DOI:
10.1039/D0SC01812K
(Edge Article)
Chem. Sci., 2020,
11, 9262-9271
Carbohydrate-binding module O-mannosylation alters binding selectivity to cellulose and lignin†
Received
29th March 2020
, Accepted 16th August 2020
First published on 19th August 2020
Abstract
Improved understanding of the effect of protein glycosylation is expected to provide the foundation for the design of protein glycoengineering strategies. In this study, we examine the impact of O-glycosylation on the binding selectivity of a model Family 1 carbohydrate-binding module (CBM), which has been shown to be one of the primary sub-domains responsible for non-productive lignin binding in multi-modular cellulases. Specifically, we examine the relationship between glycan structure and the binding specificity of the CBM to cellulose and lignin substrates. We find that the glycosylation pattern of the CBM exhibits a strong influence on the binding affinity and the selectivity between both cellulose and lignin. In addition, the large set of binding data collected allows us to examine the relationship between binding affinity and the correlation in motion between pairs of glycosylation sites. Our results suggest that glycoforms displaying highly correlated motion in their glycosylation sites tend to bind cellulose with high affinity and lignin with low affinity. Taken together, this work helps lay the groundwork for future exploitation of glycoengineering as a tool to improve the performance of industrial enzymes.
The cell walls of terrestrial plants primarily comprise the polysaccharides cellulose, hemicellulose, and pectin, as well as the heterogeneous aromatic polymer, lignin. In nature, carbohydrates derived from plant polysaccharides provide a massive carbon and energy source for biomass-degrading fungi, bacteria, and archaea, which together are the primary organisms that recycle plant matter and are a critical component of the global carbon cycle. Across the various environments in which these microbes break down lignocellulose, a few known enzymatic and chemical systems have evolved to deconstruct polysaccharides to soluble sugars.1–6 These natural systems are, in several cases, being evaluated for industrial use to produce sugars for further conversion into renewable biofuels and chemicals.
From an industrial perspective, overcoming biomass recalcitrance to cost-effectively produce soluble intermediates, including sugars for further upgrading remains the main challenge in biomass conversion. Lignin, the evolution of which in planta provided a significant advantage for terrestrial plants to mitigate microbial attack, is now widely recognized as a primary cause of biomass recalcitrance.7 Chemical and/or biological processing scenarios of lignocellulose have been evaluated8 and several approaches have been scaled to industrial biorefineries to date. Many biomass conversion technologies overcome recalcitrance by partially or wholly removing lignin from biomass using thermochemical pretreatment or fractionation. This approach enables easier polysaccharide access for carbohydrate-active enzymes and/or microbes. There are however, several biomass deconstruction approaches that employ enzymes or microbes with whole, unpretreated biomass.9,10 In most realistic biomass conversion scenarios wherein enzymes or microbes are used to depolymerize polysaccharides, native or residual lignin remains.11,12 It is important to note that lignin can bind and sequester carbohydrate-active enzymes, which in turn can affect conversion performance.13
Therefore, efforts aimed at improving cellulose binding selectivity relative to lignin have emerged as major thrusts in cellulase studies.14–25 Multiple reports in the past a few years have made exciting new contributions to our collective understanding of how fungal glycoside hydrolases, which are among the most well-characterized cellulolytic enzymes given their importance to cellulosic biofuels production, bind to lignin from various pretreatments.15,17 Taken together, these studies have demonstrated that the Family 1 carbohydrate-binding modules (CBMs) often found in fungal cellulases are the most relevant sub-domains for non-productive binding to lignin,15,17,20,26 likely due to the hydrophobic face of these CBMs that is known to be also responsible for cellulose binding (Fig. 1).27
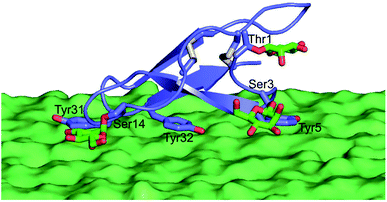 |
| Fig. 1 Model of glycosylated CBM binding the surface of a cellulose crystal. Glycans are shown in green with oxygen atoms in red, tyrosines known to be critical to binding shown in purple, and disulfide bonds Cys8–Cys25 and Cys19–Cys35 in yellow. | |
Furthermore, several studies have been published recently using protein engineering of Family 1 CBMs to improve CBM binding selectivity to cellulose with respect to lignin. Of particular note, Strobel et al. screened a large library of point mutations in both the Family 1 CBM and the linker connecting the catalytic domain (CD) and CBM.21,22 These studies demonstrated that several mutations in the CBM and one in the linker led to improved cellulose binding selectivity compared to lignin. The emerging picture is that the CBM-cellulose interaction, which occurs mainly as a result of stacking between the flat, hydrophobic CBM face (which is decorated with aromatic residues) and the hydrophobic crystal face of cellulose I, is also likely the main driving force in the CBM-lignin interaction given the strong potential for aromatic–aromatic and hydrophobic interactions.
Alongside amino acid changes, modification of O-glycosylation has recently emerged as a potential tool in engineering fungal CBMs, which Harrison et al. demonstrated to be O-glycosylated.28–31 In particular, we have revealed that the O-mannosylation of a Family 1 CBM of Trichoderma reesei cellobiohydrolase I (TrCel7A) can lead to significant enhancements in the binding affinity towards bacterial microcrystalline cellulose (BMCC).30,32,33 This observation, together with the fact that glycans have the potential to form both hydrophilic and hydrophobic interactions with other molecules, led us to hypothesize that glycosylation may have a unique role in the binding selectivity of Family 1 CBMs to cellulose relative to lignin and as such, glycoengineering may be exploited to improve the industrial performance of these enzymes. To test this hypothesis, in the present study, we systematically probed the effects of glycosylation on CBM binding affinity for a variety of lignocellulose-derived cellulose and lignin substrates and investigated routes to computationally predict the binding properties of different glycosylated CBMs.
Results
Design and chemical synthesis of CBM glycoforms
Our previous studies have shown that, compared to other common O-glycan motifs, O-mannose glycans contribute the largest increase in binding affinity of the Family 1 CBM towards BMCC. As mentioned, such O-mannose glycan structures are found naturally on the Family 1 CBM from TrCel7A and other fungal cellulase CBMs.28,30 Since we were interested in identifying CBM glycoforms that preferentially bind cellulose with high affinity, we focused our study on O-mannosylation. Accordingly, we selected 19 CBM glycoforms with systematic variations in their O-mannosylation patterns (Fig. 2, 2–20). The unglycosylated CBM 1, the glycoforms carrying Gln2 or Tyr5 mutations, and a glycoform with β-linked glucose at Ser3 instead of α-linked mannose (Fig. 2, 21–23) were also included as controls for the effects of mannosylation and amino acid sequence. The CBM variants were synthesized using our previously developed one-pot synthesis and folding procedure.32,33 The folding, identities, and homogeneity of the synthetic glycoforms were experimentally verified by circular dichroism (CD), liquid chromatography-mass spectroscopy (LC-MS), thermolysin digestion, and nuclear magnetic resonance (NMR).
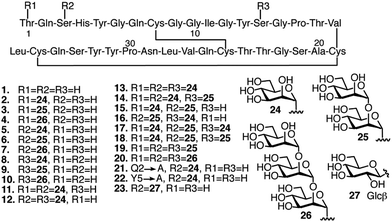 |
| Fig. 2 Glyco-variants of the TrCel7A CBM. The sequence is shown with three-letter abbreviation for each amino acid numbered from the N-terminus. Disulfide bonds indicated with solid black lines connecting Cys residues. Glycans indicated as R1, R2 and R3 attached to the respective amino acids in the sequence. Glycosylation patterns and amino acid mutations indicated for each of the 23 individual CBM glycoforms used in this study. | |
Preparation and characterization of substrates for binding studies
The structure of the cellulose substrate is thought to play a large role in CBM binding.34–36 Cellulose can exist across a wide range of organizational states from highly crystalline to mostly amorphous, and the crystallinity of cellulose is known to change as a result of certain treatment conditions.34 This degree of crystallinity is often expressed in terms of a crystallinity index (CI) value.37 With this information in mind, we decided to investigate not only the binding of the synthetic glycoforms to BMCC,32,33 but also to a series of cellulose substrates with systematically varied CI, including: Avicel® PH-101, Clean Fractionation (CF) cellulose, and phosphoric acid-swollen cellulose (PASC).
The CI of different cellulose substrates was estimated using a previously developed solid-state 13C-NMR method. Briefly, the signal from the C4 of the glucose residue was used to determine the relative amounts of crystalline and amorphous structure in each of the cellulose substrates (Fig. 3). The C4 signal in a given cellulose sample is split into two slightly overlapping peaks that represent the crystalline and amorphous regions.37 The peak at 89 ppm is characteristic of the crystalline cellulose structure and the peak at 84 ppm is that of the amorphous cellulose structure. CI is taken as the ratio of the area under the peak corresponding to the crystalline C4 signal and the total C4 signal. As expected, the commercially available microcrystalline cellulose, Avicel, had a high CI, (61%), which is only slightly lower than BMCC CI (70%). A cellulose substrate which was generated from corn stover via the Clean Fractionation process (we refer to this substrate as CF cellulose),38 exhibited a CI of 38%. PASC, which was prepared from Avicel® PH101 by using 88% (wt/vol) phosphoric acid, had the lowest CI of 14% and hence was the least crystalline or most amorphous of these samples.37
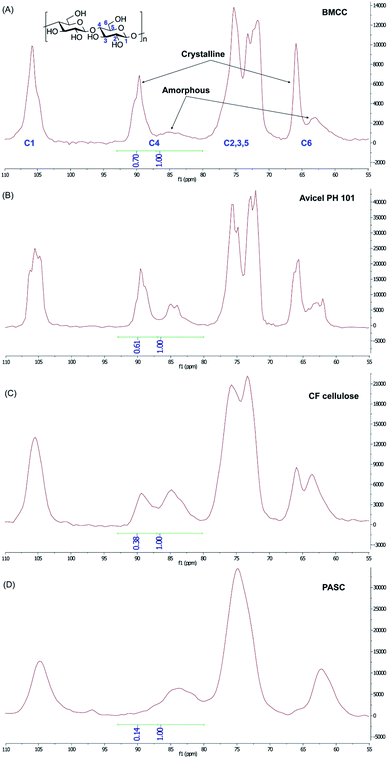 |
| Fig. 3 Whole solid state 13C NMR spectra for the determination of the CI of (A) BMCC, (B) Avicel PH 101, (C) CF cellulose, and (D) PASC. The peaks for different carbon atoms of the glucose repeat unit (numbered in blue) in different cellulose structures (crystalline vs. amorphous) were assigned according to previously published data.37 Based on the integration of the crystalline and amorphous C4 peaks, the CI was determined to be 70% for BMCC, 61% for Avicel PH 101 cellulose, 38% for CF cellulose and 14% for PASC. | |
Similarly, we hypothesized that the structure of the lignin substrate may also play a role in CBM binding based on previous work from Rahikainen et al.15 In parallel to studies of cellulose binding, we chose to investigate two lignin substrates: a lignin derived from corn stover via the same clean fractionation process mentioned above (we refer to this substrate as CF lignin) and a commercially available lignin derived from the Kraft process.
Effects of O-mannosylation on CBM binding
After preparing and characterizing the substrates, we used our previously developed, mass spectroscopy-based method to test the binding affinity of each CBM glycoform towards the three cellulose substrates and two lignin substrates.32,33 For each of the 115 possible CBM-substrate pairs, we collected binding saturation data at 4 °C and used a Langmuir isotherm to fit the resulting curves. These low temperature studies enabled direct comparison to previous binding data also gathered at this temperature.39,40 From this analysis, we could derive the value for Kads, which correlates with the strength of CBM binding for each of the CBM-substrate pairs studied. These binding experiments were conducted in triplicate. To elucidate the potential site-specific, size-specific and synergistic effects of CBM glycans on the binding affinity, the results were divided into six sets, including the unglycosylated CBM 1, monoglycosylated CBMs (2–4, 5–7, 8–10), multi-glycosylated CBMs (11–20), and CBM glycoforms with varied amino acid sequence or glycan structure (21–23) (ESI, Fig. S1 and S2†).
From the results, it can be seen that many of the CBM glycoforms studied show increased cellulose binding affinity when compared to the unglycosylated CBM, although these increases are often only modest (ESI, Fig. S1†). Five notable exceptions to this observation are CBMs 5, 13, 14, 16, and 19, whose Kads values are about three to seven times of that of the unglycosylated CBM control. CBMs 5 and 16 showed large binding affinity increases towards Avicel cellulose, but small or no increases in affinity towards CF cellulose or PASC. CBM 13 was found to bind much better to CF cellulose and bound only marginally better to the other two cellulose substrates. Interestingly, this result is the reverse of the observed binding preference of CBM 19. CBM 14 displayed relatively large increases in binding affinity towards all three of the cellulose substrates tested here.
O-Mannosylation is, as hypothesized, able to decrease the binding to the lignin substrates, particularly for Kraft lignin (ESI, Fig. S2†). Almost half of the glycoforms studied, mainly those with O-mannose glycans at multiple glycosylation sites, such as 14, 16, 17, 18, 19, and 20, exhibit lower binding affinity towards lignin than the unglycosylated CBM. Our results also show that O-mannosylation at Ser3 leads to a more substantial decrease in the affinity than O-mannosylation at either Thr1 or Ser14, which reveals that O-mannosylation can affect the lignin binding in a site-specific manner.
Because absolute binding affinities are difficult to compare across substrates, to further explain the differences in binding caused by glycosylation and identify the substrate specificity of each glycoform, the percent change in binding affinity, relative to the unglycosylated CBM control, was also calculated and plotted as a heat map (Fig. 4 and S3†). From the heat map, it is evident that glycosylation was observed to move binding affinity in both positive and negative directions depending on the glycoform and substrate being considered. For example, relative to CBM 1, CBM 5 exhibited a similar increase in binding affinity towards PASC, CF cellulose and CF lignin, but a large increase in binding towards Avicel cellulose and a large decrease in binding towards Kraft lignin. Other glycoforms, such as CBM 8, displayed little change in binding affinity towards any of the substrates as compared to the unglycosylated control (ESI, Tables S1–S3†).
Based on the analysis of the percent change in binding affinity, the glycoforms can be roughly divided into three groups. Those in group I either have greatly decreased binding affinity to cellulose substrates or have no changes in the binding to all substrates; those in group II have stronger binding to cellulose substrates and weaker binding to at least kraft lignin; those in group III have stronger binding to all cellulose substrates and weaker binding to all lignin substrates. From the data in Fig. 4, it appears that glycoforms that contain the same mannose structures distributed across all available sites (13, 19, and 20), have the most beneficial glycosylation pattern for improving binding selectivity to cellulose over lignin. The addition of monomannosyl residues to the Ser3 and Ser14 position also provided a substantial increase in binding specificity. Interestingly, we have previously proposed that glycans at these same sites can act as an extended cellulose-binding surface for the CBM in earlier structural and computational work.41 The effects of glycosylation on binding affinity observed here could be an empirical illustration of that hypothesis. Although a single dimannosyl residue at the Ser3 site could also be helpful for increasing the preference for cellulose binding, the binding specificity of CBM 6 is not as high as those of the multi-mannosylated variants.
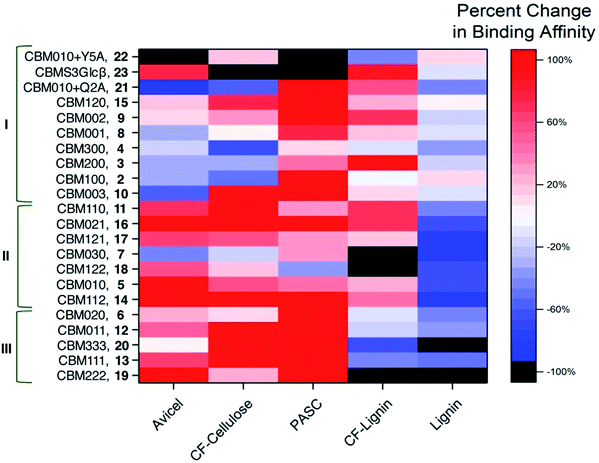 |
| Fig. 4 Changes in binding affinities resulting from glycosylation. Percent change in binding affinity towards the given substrate for each CBM glycoform relative to unglycosylated wild-type CBM 1 expressed as a heat map. The color varies from red (indicating a 100% or more increase in binding affinity) to white (indicating no change in binding affinity) to blue (indicating almost 100% decrease in binding affinity). Black is for CBM glycoform substrate pairs that displayed complete loss of binding. CBM glycoforms are named according to their glycosylation patterns (i.e., CBM100 representing the glycoform containing a single mannose at Thr1, CBM111 representing the glycoform containing a single mannose at Thr1, Ser3, and Ser14, CBM010 + Q2A representing the glycoform containing a single mannose α-linked to Ser3 and a Gln-to-Ala mutation at position 2, and CBMS3Glcβ representing the glycoform containing a single glucose β-linked to Ser3). Based on changes in binding affinities, the glycoforms are divided into three groups (I, II and III). They are ordered so that poor cellulose binders (group I) are at the top, unselective binders to cellulose and lignin (group II) are in the middle, and CBMs highly selective for binding to cellulose (group III) are at the bottom. | |
We also compared the changes in maximum binding (Bmax) caused by glycosylation (ESI, Fig. S4 and Table S4†). The heat map revealed that most glycosylation had negative effects on the maximum binding of CBM glycoforms to cellulose and lignin. At the same time, no glycosylation pattern was observed to selectively increase the Bmax value for cellulose binding.
We further investigated how temperature might affect the binding selectivity of the CBM. For this work, we chose three glyco-variants (10, 12, and 14) that represented each of the three primary groups of binding preference outlined above and the unglycosylated form CBM 1. Binding to both Avicel cellulose and Kraft lignin was measured at 30 °C. The results revealed that the binding affinity of each CBM for Avicel cellulose was slightly increased at the elevated temperature, while the affinity of most of them for Kraft lignin was decreased (ESI, Tables S1, S2 and S5†). For example, CBM 12, which had a Kads value for Avicel cellulose of 0.39 ± 0.07 μM−1 and for Kraft lignin of 0.38 ± 0.12 μM−1 at 4 °C, had the value for Avicel cellulose of 0.44 ± 0.10 μM−1 and for Kraft lignin of 0.25 ± 0.08 μM−1 at 30 °C.
Relationships of collective motions to binding specificities
Given the findings of this study and previous studies,22,33 it is possible that further improvements to binding affinity and selectivity for cellulose over lignin would result from a combination of amino acid sequence mutations and specific glycosylation patterns. A comprehensive investigation of this topic would involve the preparation and analysis of a large number of CBM glycoforms with all possible combinations of variations in glycosylation and mutation. Because such a process would be currently impractical, it would instead be advantageous to look for a simple way to predict, before synthesis, particular glycoforms most likely to result in large improvements in binding properties.
The fact that the attachment of O-mannosyl glycans at multiple glycosylation sites caused the most detectable changes in binding specificity prompted us to determine if there are collective motions between different glycosylation sites, and, moreover, the correlation between collective motions and binding to different substrates.42 Inspired by previous work, we used molecular dynamics (MD) to simulate and follow the motions of the side chain oxygens that link glycans to the peptide backbone.42–44 The average correlation between the motions of different pairs of glycosylation sites was calculated with the following equation:
where
Va and
Vb are motion vectors calculated for the individual Ser/Thr side-chain oxygen atoms
a and
b in each of the
N frames. Average correlation values calculated this way can vary between +1 and −1. A value of +1 indicates completely correlated motion between a pair of oxygen atoms (the vectors are in the same direction) and a value of −1 indicates completely anti-correlated motions (the vectors point in opposite directions). Any values between +1 or −1 imply lesser amounts of correlation or anti-correlation. 0 implies no correlation, which means that the vectors are in perpendicular or random directions.
The results of this analysis revealed that all of the correlation values were positive values, meaning that at least some amount of correlation was always present between glycan sites and no anti-correlated movements were found (ESI, Fig. S5 and Table S6†). Also, as would be expected, the amount of correlation decreases as the distance between two oxygen atoms increases.43 For example, correlations between Thr1 and Ser3 side chains, which are the closest pair in this study, are mostly above 0.3; while for the other pairs (either Thr1 and Ser14 or Ser3 and Ser14) the values range from about 0.05 to 0.13. These data also show that glycosylation of the CBM at multiple sites leads to more significant increases in the correlations, a trend that mirrors that of binding affinity towards cellulose.
The calculated Spearman's ranked correlation coefficient was used to determine the relationship between the amount of coordination in the motion of the glycan sites and binding affinity. As shown in Fig. 5 and Table S7,† our results suggest that the motion correlation is moderately predictive of binding affinity. The binding affinity to both Avicel cellulose and Kraft lignin were highly correlated with amount of coordination observed between glycosylation sites. Importantly, these associations were opposite in sign, indicating that more coordination in glycan motions was correlated with binding affinity towards cellulose, but anti-correlated with binding affinity towards lignin. While the motions of glycan sites Ser3 and Ser14 were less coordinated with one another than those of Ser3 and Thr1, they were slightly more predictive of binding affinity, particularly in the case of the Kraft lignin substrate, which gave a strong anti-correlation. The other cellulose and lignin substrates tested here displayed similar trends, although the magnitude of those trends is less.
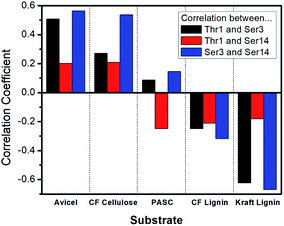 |
| Fig. 5 Correlation between coordinated glycan site movement and binding affinity. For each substrate, the Spearman's ranked correlation coefficient between the binding affinity and amount of coordination of glycan site movement is displayed as a bar. Positive values represent positive correlations, negative values represent anti-correlations. A value of +1.0 indicates perfect correlation and a value of −1.0 indicates perfect anti-correlation. Black bars are correlations based on coordination between glycan sites at Thr1 and Ser3, red bars are those based on Thr1 and Ser14, and blue bars are those based on Ser3 and Ser14. Each bar represents data derived from binding affinity and glycan site dynamics calculations for all 23 CBM variants. | |
Discussion
While lignocellulose is the most abundant carbon source for renewable production of fuels and chemicals, the biological conversion of that stored potential energy and carbon to biofuels is currently hindered by serious challenges. Many factors, including the structural integrity of cellulose and the difficulty in separating non-cellulose biopolymers, contribute to the slow and costly enzymatic hydrolysis of cellulose. Previous work demonstrating that cellulases can target different substrates via their CBMs shows that engineering CBMs is a feasible approach to direct cellulase specificity towards the preferred polysaccharide substrates and minimize their adsorption to lignin.45 Most of the work in this area thus far has focused on the functional significance of the amino acid sequences. For example, the substrate-binding sites were systematically mutated to different residues to determine if the binding properties of the CBMs would be affected.16,17 Recently, it was found that glycosylation can also modulate the binding ability of CBMs.32,33 To develop better industrial cellulases, it is of interest to determine if glycosylation can differentially regulate CBM binding selectivity.
Naturally, many fungal cellulases are O-glycosylated and evidence points to O-mannose type glycans on TrCel7A.29,31,46 To explore the potential effects of this type of glycosylation on the binding specificity of TrCel7A CBM, we performed this comparative study using synthetic homogenous glycoforms. Our studies complement previous studies by others and clearly demonstrate the value of this approach for providing definitive information regarding the biochemical roles of glycosylation. Our ability to readily synthesize a large collection of homogeneous glyco-variants that carry systematic variations in both glycan structure and amino acid sequence greatly facilitated this research.
Since the planned commercial use for the fungal cellulases studied here is lignocellulose hydrolysis, our aim is to engineer CBMs showing both a strong binding affinity to cellulose and at the same time, low binding affinity to lignin.11,15 It is clear from our previous studies that many O-mannosylated CBM variants display enhanced binding affinity towards the highly crystalline cellulose substrate, BMCC.32,33 The results obtained here also show that such beneficial effects of binding extend to three less crystalline types of cellulose. On the other hand, glycoforms that weakly bound to one type of cellulose tended to bind the other cellulose substrates poorly as well. This observation indicates that glycosylation alone may not have an ability to allow CBMs to discriminate between celluloses of differing crystallinity. This conclusion supports previous work that attributes the well-known increase in hydrolysis rate of amorphous cellulose to an increase in the reactivity of the substrate rather than an increased ability for cellulases to bind the unstructured substrates.37 It is also clear after examining our data that there are exceptions to this observation, most notably in the monoglycosylated CBMs. For example, CBM 5 was found to bind only to Avicel cellulose with high affinity and the other two cellulose substrates poorly. Previously we have found that monomannosylation of Ser3, as is the case for CBM 5, significantly increased CBM binding affinity towards BMCC.32,33 Comparing their CI values, BMCC and Avicel are far more crystalline than either of the other two cellulose forms studied here. This, along with our binding data implies that monomannosylation of the CBM at Ser3 may selectively increase binding affinity toward crystalline cellulose substrates, while exhibiting little effect on disordered cellulose binding. This is an interesting observation that warrants further study. Interestingly, although most of the CBM glycoforms we examined were poor binders to kraft lignin, more than half of the glycoforms showed some increase in CF lignin binding capacity. This may be due to the residual presence of cellulose in the CF lignin, which is less purified than the kraft lignin sample.13 Collectively, these results suggest that mannose glycans impart beneficial effects for substrate specificities and that glycosylation of multiple sites may be a useful strategy to achieve selective binding of cellulose over lignin during the saccharification process.
Importantly, the availability of the large set of data provides an ideal opportunity to look for a simple method to screen CBM variants for better binding selectivity. By systematically examining the relationship between the determined binding properties and the calculated degree of coordination that the glycosylation sites display in their movements using MD simulations, we found that the level of coordination in such motions was correlated, and hence predictive, of binding affinity to both cellulose and lignin substrates. Our results have clearly shown that the movements of the Ser3 and Ser14 sites are most closely associated with binding affinity, a finding which is consistent with their hypothesized involvement in cellulose binding.41 These two sites span the majority of the binding interface and run roughly parallel to the line of three tyrosine side chains that are known to be critical for binding (Fig. 1). It is possible that this relationship to the binding interface explains the fact that Ser3 and Ser14 motions were the most correlated with binding affinity, since any movement of these two residues is likely to affect the arrangement of the binding interface residues. By the same logic, modifications to these residues that restrict the motions of these residues will almost certainly affect the motions of the binding interface. Since glycans have been shown by many others to strongly affect the dynamics of local amino acids, it is possible that in the case of the CBM studied here, those changes in local dynamics are one factor leading to the observed effects of glycosylation on substrate binding.47,48 Taken together, the results from our computational studies suggest that the combination of computational prediction and experimental characterization could be a useful tool for developing CBM glycoforms displaying further improved substrate specificity.
Conclusions
By synthesizing, characterizing, and systematically analyzing a collection of differently glycosylated CBM variants, we were able to reveal the beneficial effects that glycosylation can have on CBM binding preferences across multiple biomass-derived cellulose and lignin substrates. We have shown that most of the glycoforms studied here bind to ordered and disordered cellulose equal well, meaning that the glycosylation patterns examined here do not cause an increased preference for a specific type of cellulose. More importantly, our results suggested that specific patterns of O-glycosylation can lead to simultaneous increases in cellulose binding and deceases in affinity for lignin. Lignin content in biomass is an historical problem for enzymatic depolymerization of biomass polysaccharides, largely due to its ability to bind and sequester cellulases.11,15 Our findings thus provide a conceptually new means to reduce lignin-driven enzyme inhibition, while simultaneously increasing cellulase binding affinity towards cellulose. In addition, based on the large set of experimentally derived CBM binding data, we were able to use MD simulation to correlate different binding properties to variation in the collective motion of pairs of glycosylation sites. The findings presented here thus will enable future optimization of the substrate specificity for this CBM, which would be an advance towards more efficient cellulases via glycoengineering.46
Methods
Materials and methods
All commercial reagents and solvents were used as purchased without further purification. Unless otherwise indicated, all reactions and purifications were performed under air atmosphere at room temperature. All LC-MS analyses were performed using a Waters Acquity™ Ultra Performance LC system equipped with Acquity UPLC® BEH 300 C4, 1.7 μm, 2.1 × 100 mm column at flow rates of 0.3 and 0.5 mL min−1. The mobile phase for LC-MS analysis was a mixture of H2O (0.1% formic acid, v/v) and acetonitrile (0.1% formic acid, v/v). All preparative separations were performed using a LabAlliance HPLC solvent delivery system equipped with a Rainin UV-1 detector and a Varian Microsorb 100-5 C18, 250 × 21.4 mm column at a flow rate of 16.0 mL min−1. The mobile phase for HPLC purification was a mixture of H2O (0.05% TFA, v/v) and acetonitrile (0.04% TFA, v/v). Mass spectrometric analysis done with a Waters SYNAPT G2-S LC-MS system was used to confirm the identity and homogeneity of the synthetic CBM glycoforms. Solid-state 13C-NMR was done on a Varian INOVA 400 MHz NMR instrument equipped with a 4 mm cross-polarization magic angle spinning (CP-MAS) probe. MALDI-TOF mass spectrometric analysis done using a AB/Sciex Voyager DE-STR system was used to determine the binding affinity.32
Synthesis of Fmoc-glycoamino acids
The Fmoc-glycoamino acid building blocks Fmoc-Ser(Ac4Manα)-OH, Fmoc-Thr(Ac4Manα)-OH, Fmoc-Ser(Ac4Glcβ)-OH, Fmoc-Ser(Ac4Manα2Ac3Manα)-OH, Fmoc-Thr(Ac4Manα2Ac3Manα)-OH, Fmoc-Ser(Ac4Manα2Ac3Manα2Ac3Manα)-OH and Fmoc-Thr(Ac4Manα2Ac3Manα2Ac3Manα)-OH were prepared using procedures adapted from previous work.33,49
Synthesis of CBM variants
The variants were synthesized following our previous work.32 Briefly, automated peptide synthesis was conducted on a Pioneer® continuous flow peptide synthesizer (Applied Biosystems) using low-loading Fmoc-Leu-NovaSyn® TGT resin (Merck Millipore). Fmoc-amino acids/Fmoc-glycoamino acids (4.0 equiv.), HATU (4.0 equiv.) and DIEA (8.0 equiv.) were used for the amino acid coupling reactions. A mixture of DMF/piperidine/DBU (100/2/2, v/v) was used for Fmoc-deprotection. After synthesis, the peptides/glycopeptides were deprotected and simultaneously cleaved from the resin support by treating with TFA/TIS/H2O (95/2.5/2.5, v/v) for 45 min. TFA was blown off under a stream of N2 and the crude peptides/glycopeptides were collected by precipitation with cold ether and centrifugation. The crude peptides/glycopeptides were then dissolved MeCN/H2O (50/50, v/v) and lyophilized to dryness for the next synthetic steps without further purification.
For the unglycosylated CBM 1, the lyophilized crude peptide was dissolved in the folding buffer (0.2 M Tris–acetate, 0.33 mM oxidized glutathione, 2.6 mM reduced glutathione, pH 8.2) and stirred under helium for 12 h. The solution was then concentrated to a small volume using 3 kDa cut-off centrifugal filter units (Amicon). After HPLC purification, the pure fractions were combined and lyophilized to give the desired product as a white solid.
For the glycosylated CBM variants 2–23, the crude glycopeptides were dissolved in hydrazine solution (hydrazine/H2O, 5/100, v/v) and stirred for 30 min under helium. The reaction was quenched with acetic acid solution (AcOH/H2O, 5/100, v/v). The resulting mixture was diluted at 1/40 (v/v) with the folding buffer and stirred under helium for 12 h. The solution was then concentrated and purified by HPLC to afford the desired product as a white solid.
Preparation of CF cellulose and lignin
Following previous work closely,38 whole corn stover (10 g) in a single-phase mixture of methyl isobutyl ketone (MIBK)/acetone/H2O with sulfuric acid (0.025 to 0.1 M) was loaded into a 316 stainless steel pressure reactor. The reactor was sealed and heated in an electric heating block at 120 or 140 °C for 56 minutes. After the reaction, the reactor was cooled in ice water. The reaction mixture was separated into a solid fraction and an aqueous fraction via filtration. The solid fraction was thoroughly washed first with the same solvent (200 mL) followed by deionized H2O (650 mL). The solid fraction was air-dried to obtain the CF cellulose.38 Moisture content in the CF cellulose was measured by the moisture analyzer. The combined black filtrate (filtrate and wash liquors) was mixed with MIBK (50 mL) in a separatory funnel, shaken, and allowed to stand for 1 hour to separate aqueous and organic phases. The aqueous layer was extracted with MIBK (28 mL) two times. MIBK layers were combined and washed with deionized H2O and brine, followed by evaporation in a Rotavap to remove MIBK. The dried contents in the flask were further dried in a vacuum oven at 35 °C for 4 d to obtain the CF lignin.38
Solid state NMR
Solid state 13C-CP/MAS-NMR spectra were collected for each sample using a 400 MHz NMR operating at 100.63 MHz at 23 °C and equipped with a 4 mm Revolution CP/MAS Probe. Spinning speed was 10
000 Hz, relaxation delay of 3.0 s. Peak assignments were based on those of Park.37 The amorphous peak was taken as 80 to 87 ppm and the crystalline peak was taken as 87 to 93 ppm.
Determination of binding affinity
Binding affinity was measured as described previously in our work.32 Briefly, lyophilized CBM variants were suspended and serially diluted in 50 mM sodium acetate and 50 mM sodium chloride buffer (pH 5.0). CBM suspensions were added 1
:
1 with 2.4 mg mL−1 substrate in 50 mM sodium acetate and 50 mM sodium chloride (pH 5.0; total volume = 100 μL) in microcentrifuge tubes containing magnetic stir bars. The samples were stirred to equilibrium at 1100 rpm at 4 °C for 2 h before centrifugation at 14000g for 10 min. Two 10 μL aliquots were taken from the supernatant and analyzed by quantitative MALDI-TOF MS to calculate unbound CBM concentration. This process was repeated for three independent trials. Data were fitted to a single-site Langmuir adsorption model using OriginPro software.
Calculation of the average correlation and the Spearman's ranked correlation coefficient
The CBM variants 1–23 were built and modeled based on the structure of TrCel7A CBM with O-mannose residues at Thr1, Ser3, and Ser14 (PDB ID: 2MWK). The His4 residue was kept protonated since the binding affinity measurement was performed at pH 5.0. The Amber ff14SB force field and atomic charges50 were used for protein residues and the GLYCAM06 force field and atomic charges51 were used for the glycans. Each variant was first solvated in a TIP3P water box, maintaining at least 12 Å between any protein atoms and the box boundaries. Then molecular dynamics simulations were performed using the Amber 14.0 package.52 Long-range interactions were treated with particle mesh Ewald summation using mesh density of ∼1 point/Å in each cell direction during simulations. The system was first equilibrated for 10 ns, then a 20 ns production simulation was performed with NPT ensemble at 4 °C and 1 atm pressure. During the production simulation, the trajectories were saved in every 1 ps, which were then used to compute the average correlation (AvgCorr) between collective motions by the ptraj module in the Amber package. The results were shown in Fig. S5 and Table S6.† The Spearman's ranked correlation coefficients between these AvgCorr values and binding affinity were then computed using the binding affinity values. Spearman's coefficients were grouped into five categories based on the strength of the indicated correlation as based on common statistic practice (Table S7†).53
Author contributions
All authors have given approval to the final version of the manuscript. Y. L., X. G., P. K. C., H. L., Z. T. designed research; Y. L., X. G., P. K. C., Y. R., B. M., H. L. performed research; G. T. B. contributed essential material; Y. L., X. G., P. K. C., S. S., H. L., Z. T. analyzed data; Y. L., X. G., P. K. C., S. S., M. E. H., G. T. B., H. L., Z. T. wrote the paper.
Conflicts of interest
The authors declare no competing financial interest.
Acknowledgements
We would like to thank the National Natural Science Foundation of China (Grant number: 91853120), the National Major Scientific and Technological Special Project of China (Grant number: 2018ZX09711001-013), the National Key R&D Program of China (Grant number: 2018YFE0111400), the State Key Laboratory of Bioactive Substance and Function of Natural Medicines, Institute of Materia Medica, and the Chinese Academy of Medical Sciences and Peking Union Medical College, and the NIH Research Project Grant Program (R01 EB025892) for funding. This work was also authored in part by Alliance for Sustainable Energy, LLC, the Manager and Operator of the National Renewable Energy Laboratory for the U.S. Department of Energy (DOE) under Contract No. DE-AC36-08GO28308. Funding to MEH and GTB was provided by U.S. Department of Energy Office of Energy Efficiency and Renewable Energy Bioenergy Technologies Office. Computer time was provided by the National Renewable Energy Laboratory Computational Sciences Center supported by the DOE Office of EERE under contract number DE-AC36-08GO28308. We thank Rui Katahira for providing the CF substrate.
References
- G. Xu and B. Goodell, Mechanisms of wood degradation by brown-rot fungi: chelator-mediated cellulose degradation and binding of iron by cellulose, J. Biotechnol., 2001, 87(1), 43–57 CrossRef CAS PubMed.
- C. M. Fontes and H. J. Gilbert, Cellulosomes: highly efficient nanomachines designed to deconstruct plant cell wall complex carbohydrates, Annu. Rev. Biochem., 2010, 79, 655–681 CrossRef CAS PubMed.
- D. Floudas, M. Binder, R. Riley, K. Barry, R. A. Blanchette, B. Henrissat, A. T. Martínez, R. Otillar, J. W. Spatafora and J. S. Yadav, The Paleozoic origin of enzymatic lignin decomposition reconstructed from 31 fungal genomes, Science, 2012, 336(6089), 1715–1719 CrossRef CAS PubMed.
- R. Brunecky, M. Alahuhta, Q. Xu, B. S. Donohoe, M. F. Crowley, I. A. Kataeva, S.-J. Yang, M. G. Resch, M. W. W. Adams and V. V. Lunin, Revealing nature's cellulase diversity: the digestion mechanism of Caldicellulosiruptor bescii CelA, Science, 2013, 342(6165), 1513–1516 CrossRef CAS PubMed.
- C. M. Payne, B. C. Knott, H. B. Mayes, H. Hansson, M. E. Himmel, M. Sandgren, J. Stahlberg and G. T. Beckham, Fungal cellulases, Chem. Rev., 2015, 115(3), 1308–1448 CrossRef CAS PubMed.
- K. V. Solomon, C. H. Haitjema, J. K. Henske, S. P. Gilmore, D. Borges-Rivera, A. Lipzen, H. M. Brewer, S. O. Purvine, A. T. Wright and M. K. Theodorou, Early-branching gut fungi possess a large, comprehensive array of biomass-degrading enzymes, Science, 2016, 351(6278), 1192–1195 CrossRef CAS PubMed.
- M. E. Himmel, S.-Y. Ding, D. K. Johnson, W. S. Adney, M. R. Nimlos, J. W. Brady and T. D. Foust, Biomass recalcitrance: engineering plants and enzymes for biofuels production, Science, 2007, 315(5813), 804–807 CrossRef CAS PubMed.
- P. S. Chundawat, G. T. Beckham, M. E. Himmel and B. E. Dale, Deconstruction of lignocellulosic biomass to fuels and chemicals, Annu. Rev. Chem. Biomol. Eng., 2011, 2, 121–145 CrossRef PubMed.
- D. G. Olson, J. E. McBride, A. J. Shaw and L. R. Lynd, Recent progress in consolidated bioprocessing, Curr. Opin. Biotechnol., 2012, 23(3), 396–405 CrossRef CAS PubMed.
- D. Chung, M. Cha, A. M. Guss and J. Westpheling, Direct conversion of plant biomass to ethanol by engineered Caldicellulosiruptor bescii, Proc. Natl. Acad. Sci. U. S. A., 2014, 111(24), 8931–8936 CrossRef CAS PubMed.
- A. Berlin, N. Gilkes, A. Kurabi, R. Bura, M. Tu, D. Kilburn and J. Saddler, Weak lignin-binding enzymes: a novel approach to improve activity of cellulases for hydrolysis of lignocellulosics, Appl. Biochem. Biotechnol., 2005, 121–124, 163–170 CrossRef CAS PubMed.
- R. H. Narron, H. Kim, H. M. Chang, H. Jameel and S. Park, Biomass pretreatments capable of enabling lignin valorization in a biorefinery process, Curr. Opin. Biotechnol., 2016, 38, 39–46 CrossRef CAS PubMed.
- M. J. Selig, S. Viamajala, S. R. Decker, M. P. Tucker, M. E. Himmel and T. B. Vinzant, Deposition of lignin droplets produced during dilute acid pretreatment of maize stems retards enzymatic hydrolysis of cellulose, Biotechnol. Prog., 2007, 23(6), 1333–1339 CrossRef CAS PubMed.
- H. Palonen, F. Tjerneld, G. Zacchi and M. Tenkanen, Adsorption of Trichoderma reesei CBH I and EG II and their catalytic domains on steam pretreated softwood and isolated lignin, J. Biotechnol., 2004, 107(1), 65–72 CrossRef CAS PubMed.
- J. L. Rahikainen, R. Martin-Sampedro, H. Heikkinen, S. Rovio, K. Marjamaa, T. Tamminen, O. J. Rojas and K. Kruus, Inhibitory effect of lignin during cellulose bioconversion: the effect of lignin chemistry on non-productive enzyme adsorption, Bioresour. Technol., 2013, 133, 270–278 CrossRef CAS PubMed.
- J. L. Rahikainen, U. Moilanen, S. Nurmi-Rantala, A. Lappas, A. Koivula, L. Viikari and K. Kruus, Effect of temperature on lignin-derived inhibition studied with three structurally different cellobiohydrolases, Bioresour. Technol., 2013, 146, 118–125 CrossRef CAS PubMed.
- R. Martín-Sampedro, J. L. Rahikainen, L.-S. Johansson, K. Marjamaa, J. Laine, K. Kruus and O. J. Rojas, Preferential adsorption and activity of monocomponent cellulases on lignocellulose thin films with varying lignin content, Biomacromolecules, 2013, 14(4), 1231–1239 CrossRef PubMed.
- E. M. Nordwald, R. Brunecky, M. E. Himmel, G. T. Beckham and J. L. Kaar, Charge engineering of cellulases improves ionic liquid tolerance and reduces lignin inhibition, Biotechnol. Bioeng., 2014, 111(8), 1541–1549 CrossRef CAS PubMed.
- D. W. Sammond, J. M. Yarbrough, E. Mansfield, Y. J. Bomble, S. E. Hobdey, S. R. Decker, L. E. Taylor, M. G. Resch, J. J. Bozell and M. E. Himmel, Predicting enzyme adsorption to lignin films by calculating enzyme surface hydrophobicity, J. Biol. Chem., 2014, 289(30), 20960–20969 CrossRef CAS PubMed.
- D. Gao, C. Haarmeyer, V. Balan, T. A. Whitehead, B. E. Dale and S. P. Chundawat, Lignin triggers irreversible cellulase loss during pretreated lignocellulosic biomass saccharification, Biotechnol. Biofuels, 2014, 7(1), 175 CrossRef PubMed.
- K. L. Strobel, K. A. Pfeiffer, H. W. Blanch and D. S. Clark, Engineering Cel7A carbohydrate binding module and linker for reduced lignin inhibition, Biotechnol. Bioeng., 2016, 113(6), 1369–1374 CrossRef CAS PubMed.
- K. L. Strobel, K. A. Pfeiffer, H. W. Blanch and D. S. Clark, Structural insights into the affinity of Cel7A carbohydrate-binding module for lignin, J. Biol. Chem., 2015, 290(37), 22818–22826 CrossRef CAS PubMed.
- J. M. Yarbrough, A. Mittal, E. Mansfield, L. E. Taylor, S. E. Hobdey, D. W. Sammond, Y. J. Bomble, M. F. Crowley, S. R. Decker and M. E. Himmel, New perspective on glycoside hydrolase binding to lignin from pretreated corn stover, Biotechnol. Biofuels, 2015, 8(1), 1 CrossRef PubMed.
- K. A. Pfeiffer, H. Sorek, C. M. Roche, K. L. Strobel, H. W. Blanch and D. S. Clark, Evaluating endoglucanase Cel7B-lignin interaction mechanisms and kinetics using quartz crystal microgravimetry, Biotechnol. Bioeng., 2015, 112(11), 2256–2266 CrossRef CAS PubMed.
- C. N. Haarmeyer, M. D. Smith, S. P. Chundawat, D. Sammond and T. A. Whitehead, Insights into cellulase-lignin non-specific binding revealed by computational redesign of the surface of green fluorescent protein, Biotechnol. Bioeng., 2017, 114(4), 740–750 CrossRef CAS PubMed.
- H. Palonen and L. Viikari, Role of oxidative enzymatic treatments on enzymatic hydrolysis of softwood, Biotechnol. Bioeng., 2004, 86(5), 550–557 CrossRef CAS PubMed.
- P. J. Kraulis, G. M. Clore, M. Nilges, T. A. Jones, G. Pettersson, J. Knowles and A. M. Gronenborn, Determination of the three-dimensional solution structure of the C-terminal domain of cellobiohydrolase I from Trichoderma reesei. A study using nuclear magnetic resonance and hybrid distance geometry-dynamical simulated annealing, Biochemistry, 1989, 28(18), 7241–7257 CrossRef CAS PubMed.
- M. J. Harrison, A. S. Nouwens, D. R. Jardine, N. E. Zachara, A. A. Gooley, H. Nevalainen and N. H. Packer, Modified glycosylation of cellobiohydrolase I from a high cellulase-producing mutant strain of Trichoderma reesei, Eur. J. Biochem., 1998, 256(1), 119–127 CrossRef CAS PubMed.
- G. T. Beckham, Z. Dai, J. F. Matthews, M. Momany, C. M. Payne, W. S. Adney, S. E. Baker and M. E. Himmel, Harnessing glycosylation to improve cellulase activity, Curr. Opin. Biotechnol., 2012, 23(3), 338–345 CrossRef CAS PubMed.
- C. B. Taylor, M. F. Talib, C. McCabe, L. Bu, W. S. Adney, M. E. Himmel, M. F. Crowley and G. T. Beckham, Computational investigation of glycosylation effects on a Family 1 carbohydrate-binding module, J. Biol. Chem., 2012, 287(5), 3147–3155 CrossRef CAS PubMed.
- A. Amore, B. C. Knott, N. T. Supekar, A. Shajahan, P. Azadi, P. Zhao, L. Wells, J. G. Linger, S. E. Hobdey, T. A. Vander Wall, T. Shollenberger, J. M. Yarbrough, Z. Tan, M. F. Crowley, M. E. Himmel, S. R. Decker, G. T. Beckham and L. E. Taylor, 2nd, Distinct roles of N- and O-glycans in cellulase activity and stability, Proc. Natl. Acad. Sci. U. S. A., 2017, 114(52), 13667–13672 CrossRef CAS PubMed.
- L. Chen, M. R. Drake, M. G. Resch, E. R. Greene, M. E. Himmel, P. K. Chaffey, G. T. Beckham and Z. Tan, Specificity of O-glycosylation in enhancing the stability and cellulose binding affinity of Family 1 carbohydrate-binding modules, Proc. Natl. Acad. Sci. U. S. A., 2014, 111(21), 7612–7617 CrossRef CAS PubMed.
- X. Guan, P. K. Chaffey, C. Zeng, E. R. Greene, L. Chen, M. R. Drake, C. Chen, A. Groobman, M. G. Resch, M. E. Himmel, G. T. Beckham and Z. Tan, Molecular-scale features that govern the effects of O-glycosylation on a carbohydrate-binding module, Chem. Sci., 2015, 6(12), 7185–7189 RSC.
- S. P. Chundawat, G. Bellesia, N. Uppugundla, L. da Costa Sousa, D. Gao, A. M. Cheh, U. P. Agarwal, C. M. Bianchetti, G. N. Phillips Jr, P. Langan, V. Balan, S. Gnanakaran and B. E. Dale, Restructuring the crystalline cellulose hydrogen bond network enhances its depolymerization rate, J. Am. Chem. Soc., 2011, 133(29), 11163–11174 CrossRef CAS PubMed.
- J. Guo and J. M. Catchmark, Binding specificity and thermodynamics of cellulose-binding modules from Trichoderma reesei Cel7A and Cel6A, Biomacromolecules, 2013, 14(5), 1268–1277 CrossRef CAS PubMed.
- D. Gao, S. P. Chundawat, A. Sethi, V. Balan, S. Gnanakaran and B. E. Dale, Increased enzyme binding to substrate is not necessary for more efficient cellulose hydrolysis, Proc. Natl. Acad. Sci. U. S. A., 2013, 110(27), 10922–10927 CrossRef CAS PubMed.
- S. Park, J. O. Baker, M. E. Himmel, P. A. Parilla and D. K. Johnson, Cellulose crystallinity index: measurement techniques and their impact on interpreting cellulase performance, Biotechnol. Biofuels, 2010, 3, 10 CrossRef PubMed.
- R. Katahira, A. Mittal, K. McKinney, P. N. Ciesielski, B. S. Donohoe, S. K. Black, D. K. Johnson, M. J. Biddy and G. T. Beckham, Evaluation of Clean Fractionation Pretreatment for the Production of Renewable Fuels and Chemicals from Corn Stover, ACS Sustainable Chem. Eng., 2014, 2(6), 1364–1376 CrossRef CAS.
- J. Medve, J. Stahlberg and F. Tjerneld, Isotherms for adsorption of cellobiohydrolase I and II from Trichoderma reesei on microcrystalline cellulose, Appl. Biochem. Biotechnol., 1997, 66(1), 39–56 CrossRef CAS PubMed.
- T. Reinikainen, O. Teleman and T. T. Teeri, Effects of pH and high ionic strength on the adsorption and activity of native and mutated cellobiohydrolase I from Trichoderma reesei, Proteins, 1995, 22(4), 392–403 CrossRef CAS PubMed.
- R. M. Happs, X. Guan, M. G. Resch, M. F. Davis, G. T. Beckham, Z. Tan and M. F. Crowley, O-glycosylation effects on family 1 carbohydrate-binding module solution structures, FEBS J., 2015, 282(22), 4341–4356 CrossRef CAS PubMed.
- H. J. Berendsen and S. Hayward, Collective protein dynamics in relation to function, Curr. Opin. Struct. Biol., 2000, 10(2), 165–169 CrossRef CAS PubMed.
- T. Ichiye and M. Karplus, Collective motions in proteins: a covariance analysis of atomic fluctuations in molecular dynamics and normal mode simulations, Proteins, 1991, 11(3), 205–217 CrossRef CAS PubMed.
- M. Tiberti, G. Invernizzi and E. Papaleo, (Dis)similarity Index To Compare Correlated Motions in Molecular Simulations, J. Chem. Theory Comput., 2015, 11(9), 4404–4414 CrossRef CAS PubMed.
- H. J. Gilbert, J. P. Knox and A. B. Boraston, Advances in understanding the molecular basis of plant cell wall polysaccharide recognition by carbohydrate-binding modules, Curr. Opin. Struct. Biol., 2013, 23(5), 669–677 CrossRef CAS PubMed.
- E. R. Greene, M. E. Himmel, G. T. Beckham and Z. Tan, Glycosylation of cellulases: Engineering better enzymes for biofuels, Adv. Carbohydr. Chem. Biochem., 2015, 72, 63–112 CrossRef PubMed.
- B. Imperiali and S. E. O'Connor, Effect of N-linked glycosylation on glycopeptide and glycoprotein structure, Curr. Opin. Chem. Biol., 1999, 3(6), 643–649 CrossRef CAS PubMed.
- J. L. Price, E. K. Culyba, W. Chen, A. N. Murray, S. R. Hanson, C. H. Wong, E. T. Powers and J. W. Kelly, N-glycosylation of enhanced aromatic sequons to increase glycoprotein stability, Biopolymers, 2012, 98(3), 195–211 CrossRef CAS PubMed.
- L. Chen and Z. Tan, A convenient and efficient synthetic approach to mono-, di-, and tri-O-mannosylated Fmoc amino acids, Tetrahedron Lett., 2013, 54(17), 2190–2193 CrossRef CAS.
- V. Hornak, R. Abel, A. Okur, B. Strockbine, A. Roitberg and C. Simmerling, Comparison of multiple Amber force fields and development of improved protein backbone parameters, Proteins, 2006, 65(3), 712–725 CrossRef CAS PubMed.
- K. N. Kirschner, A. B. Yongye, S. M. Tschampel, J. González-Outeiriño, C. R. Daniels, B. L. Foley and R. J. Woods, GLYCAM06: A generalizable biomolecular force field. Carbohydrates, J. Comput. Chem., 2008, 29(4), 622–655 CrossRef CAS PubMed.
-
D. A. Case, V. Babin, J. T. Berryman, R. M. Betz, Q. Cai, D. S. Cerutti, T. E. Cheatham III, T. A. Darden, R. E. Duke, H. Gohlke, A. W. Goetz, S. Gusarov, N. Homeyer, P. Janowski, J. Kaus, I. Kolossváry, A. Kovalenko, T. S. Lee, S. LeGrand, T. Luchko, R. Luo, B. Madej, K. M. Merz, F. Paesani, D. R. Roe, A. Roitberg, C. Sagui, R. Salomon-Ferrer, G. Seabra, C. L. Simmerling, W. Smith, J. Swails, R. C. Walker, J. Wang, R. M. Wolf, X. Wu and P. A. Kollman, AMBER 14, University of California, San Francisco, 2014 Search PubMed.
-
D. E. Hinkle, W. Wiersma and S. G. Jurs, Applied statistics for the behavioral sciences, 5th edn, 2003 Search PubMed.
Footnotes |
† Electronic supplementary information (ESI) available: Binding affinity of each glycoform towards different substrates; calculated average correlation of motion and calculated correlation coefficients; adsorption isotherm plots of CBMs with different substrates. See DOI: 10.1039/d0sc01812k |
‡ These authors contributed equally |
|
This journal is © The Royal Society of Chemistry 2020 |