DOI:
10.1039/D0SC00084A
(Edge Article)
Chem. Sci., 2020,
11, 4669-4679
Engineering bio-mimicking functional vesicles with multiple compartments for quantifying molecular transport†
Received
6th January 2020
, Accepted 3rd April 2020
First published on 6th April 2020
Abstract
Controlled design of giant unilamellar vesicles under defined conditions has vast applications in the field of membrane and synthetic biology. Here, we bio-engineer bacterial-membrane mimicking models of controlled size under defined salt conditions over a range of pH. A complex bacterial lipid extract is used for construction of physiologically relevant Gram-negative membrane mimicking vesicles whereas a ternary mixture of charged lipids (DOPG, cardiolipin and lysyl-PG) is used for building Gram-positive bacterial-membrane vesicles. Furthermore, we construct stable multi-compartment biomimicking vesicles using the gel-assisted swelling method. Importantly, we validate the bio-application of the bacterial vesicle models by quantifying diffusion of chemically synthetic amphoteric antibiotics. The transport rate is pH-responsive and depends on the lipid composition, based on which a permeation model is proposed. The permeability properties of antimicrobial peptides reveal pH dependent pore-forming activity in the model vesicles. Finally, we demonstrate the functionality of the vesicles by quantifying the uptake of membrane-impermeable molecules facilitated by embedded pore-forming proteins. We suggest that the bacterial vesicle models developed here can be used to understand fundamental biological processes like the peptide assembly mechanism or bacterial cell division and will have a multitude of applications in the bottom-up assembly of a protocell.
Introduction
Giant unilamellar vesicles (GUVs) are increasingly used as bio-mimetic systems to understand a wide range of biological processes in a bottom-up approach.1,2 They are versatile tools for applications in basic science2,3 and have clinical applications specifically for drug delivery.4 Moreover, vesicles can serve as an excellent scaffold to study biological processes occurring at a membrane interface.5–7 To study specific membrane-biophysical properties, controlling the lipid-composition in giant vesicles under defined external conditions is extremely crucial and yet challenging.2,8 Several solvent-displacement techniques have been used for giant vesicle preparation with a variety of complex lipids under physiological salt conditions.9–12 Further microfluidic technologies allow for microcompartment formation in vesicles for synthetic biology applications using the solvent-displacement method.13,14 However these techniques are incompatible to investigate surface properties as organic solvents cannot be removed effectively.2,3 Alternatively, hydrogel-assisted swelling facilitated construction of vesicles under physiological conditions with various lipid compositions.8,15 The technique has since expanded the possibility to study complex lipid–protein interactions,16,17 vesicles for applications in drug delivery18 and use of vesicles as micro-reactors.19,20 However no studies have reported multicompartment formation in vesicles using this methodology.
Models systems such as giant vesicles are routinely employed to study cell-division; this lays emphasis on manipulation of membrane compositions for revealing their active role in processes like constriction.21 Importantly, recent advances in this field have frequently used giant vesicles with minimum complexity in terms of the lipid composition and buffer conditions to investigate membrane shrinkage or invagination processes22,23 and shape control.24 Other widely reported membrane biological processes using giant vesicles are the transport/release of molecules across membranes25–29 and interaction of proteins/peptides with membranes.30,31 For example, antimicrobial peptide interaction with lipid vesicles ‘mimicking’ bacterial membranes has revealed the lipid-dependent and ion-dependent transition in membranes.31,32 However, in most of these reports, minimal/no salt concentration is used to study the interaction of lipid–peptides.31–33 Despite the relevance of lipid composition-dependent activity under physiological conditions, simplified bacterial membrane mimicking models have been used in most of the reports.34,35 Notably lipid-composition-dependent release/uptake of small molecules36,37 has also been reported with significant implications in synthetic biology and the understanding of transport in bacteria. Recent advances in the direction of development of new antibiotics specifically targeting membranes38,39 also demand better bacterial-membrane mimicking models. However, to date there have been no physiologically relevant mimicking models with a well-defined lipid composition and external conditions.
In this report, we systematically construct Gram-negative and Gram-positive bacterial membrane mimicking models under controlled physiological conditions. We establish the functionality of these models by quantifying the diffusion of small molecules across membranes of varying lipid compositions. We propose that such bio-mimetic model membrane systems can find applications in synthetic chemical biology.
Results and discussion
Engineering bacterial membrane mimicking vesicles: Gram-negative and Gram-positive membrane liposomes
The Gram-negative membrane mimicking vesicles (GN vesicles) are prepared from an Escherichia coli polar lipid extract (67% phosphatidyl-ethanolamine (PE), 23.3% phosphatidyl-glycerol (PG), and 9.8% cardiolipin (CA)). The Gram-positive membrane mimicking vesicles (GP vesicles) are constructed using a ternary mixture of pure lipids (60% DOPG (1,2-dioleoyl-sn-glycero-3-phospho-(1′-rac-glycerol)), 35% lysyl-PG (1,2-dioleoyl-sn-glycero-3-[phospho-rac-(3-lysyl(1-glycerol))]), and 5% CA 1′,3′-bis[1,2-dioleoyl-sn-glycero-3-phospho]-glycerol) (Fig. 1(A) schematic of the vesicles). The gel-assisted swelling method is used to prepare both the model vesicles (Fig. 1(B) and S1†).
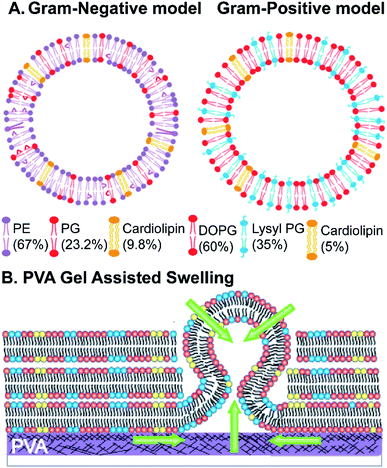 |
| Fig. 1 Schematic representing (A) Gram-negative and Gram-positive membrane model vesicles and the corresponding lipid composition and (B) PVA gel-assisted swelling of giant unilamellar vesicles. The green arrows represent the paths by which an aqueous buffer enters and swells the lipid film leading to formation of giant unilamellar vesicles. | |
A lipid film is spread on a priorly dried polyvinyl alcohol (PVA) film on a glass slide (Fig. S1(A)–(D)†) and swelled in the presence of a physiological buffer (137 mM NaCl and 2.7 mM KCl) to form vesicles (details in the ESI† Methods section). We prepared vesicles under two pH conditions, pH 5 and pH 7, to show the versatility of the model vesicles in the presence of salts. Coating lipids on the PVA film enhances the formation of GUVs upon the addition of an aqueous buffer (Fig. 2(A1) at pH 7, and Fig. 2(B1) at pH 5 for GN vesicles and Fig. 2(D1) at pH 7 and Fig. 2(E1) at pH 5 for GP vesicles). Subsequently, the vesicles are collected by pipetting the solution (Fig. 2(A2) and (B2) for GN vesicles and Fig. 2(D2) and (E2) for GP vesicles). Notably, the size of the vesicles varies slightly depending on the pH of the buffer used. The sample is collected after 30 minutes of hydration of the film and the size is determined. At pH 7, both GN and GP vesicles are slightly larger in diameter (mean and S.D.) and have values of 45 ± 18 μm (N = 279 from 65 independent batches) and 43 ± 14 μm (n = 194 from 56 independent batches) respectively, than at pH 5 where the diameter is 37 ± 15 (N = 218 from 55 independent batches) and 38 ± 15 (N = 142 from 45 independent batches) respectively (Fig. 2(C) and (F)).
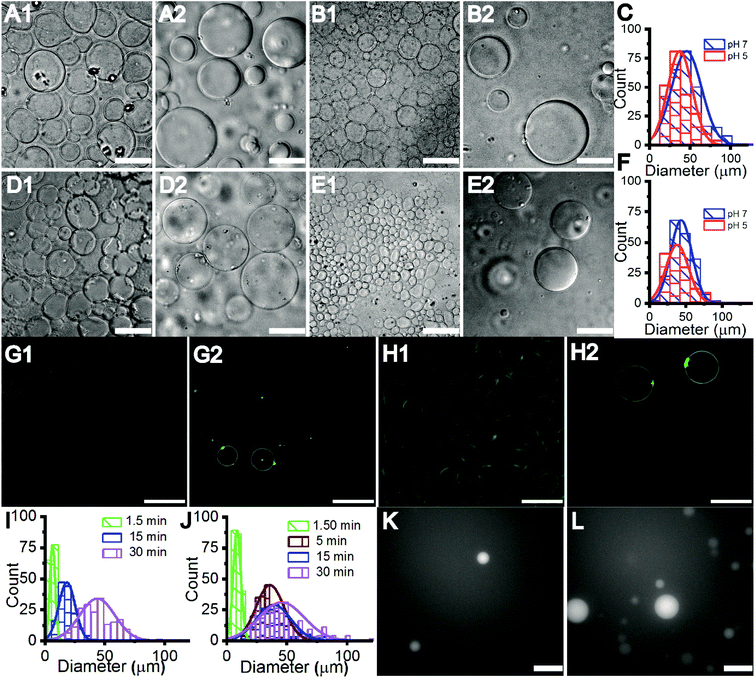 |
| Fig. 2 Phase contrast images of: Gram-negative GUVs:(A1) formation on PVA at pH 7, (A2) collected in solution after 30 minute hydration at pH 7, (B1) formation on PVA at pH 5, (B2) collected in solution after 30 minute hydration at pH 5, and (C) Histogram showing the diameter of GN GUVs at pH 7 and pH 5; Gram-positive GUVs: (D1) formation on PVA at pH 7, (D2) collected in solution after 30 minute hydration at pH 7, (E1) formation on PVA at pH 5, (E2) collected in solution at pH 5, and (F) histogram showing the diameter of GP GUVs at pH 7 and pH 5. The histograms are fitted using a normal distribution curve. Images are captured with a 40× phase contrast objective. Scale bar 50 μm for A1 to E2; Hydration time-dependent size control of Gram-negative giant vesicles with 0.1 mol% Atto-DOPE mixed with the lipid mixture; (G1) formation on PVA at 1 minute 30 seconds, (G2) vesicles in solution at 1 minute 30 s, (H1) formation on PVA at 5 min, (H2) vesicles in solution at 5 min; histogram fitted with normal distribution showing (I) size of GP vesicles and (I) size of GN vesicles at different hydration times, encapsulation of Alexa Fluor in (K). GN vesicles at 1.50 minutes, (L) GP vesicles at 30 min; images are captured with a 60× objective. Scale bar: 20 μm. | |
Further, we observed that the vesicles ‘grow’ on the PVA gel over time with their size increasing with the increase in hydration-time. The vesicle samples collected at different time points are depicted in Fig. 2(G1)–(H2) and Fig. S2(A1)–(C2)† for GN vesicles and Fig. S3† for GP vesicles. The hydration time dependent growth is used to control the size of the vesicles. Relatively homogeneous size distribution is obtained with a minimum time of hydration for both the vesicle models. At less than 2 minute time of hydration, we observe a diameter of 8 ± 3 μm for GN vesicles (N = 229 from 13 batches) and 6 ± 2 μm for GP vesicles (N = 146 from 9 independent batches). The size distribution of different time points is shown (Fig. 2(I) and (J)). In the case of GP vesicles, a continuous increase in the diameter of the vesicles is observed with increase in hydration time; at 15 minutes the diameter is 18 ± 6 μm (N = 144 from 10 independent batches) and at 30 minutes it is 43 ± 14 μm (N = 194) (Fig. 2(I)). For GN vesicles after 5 minutes of hydration, the vesicles grow to their maximum size and do not grow any further with a diameter of 35 ± 11 μm (N = 243 from 10 independent batches) and at 15 minutes, their diameter is 38 ± 14 μm (N = 205 from 10 independent batches) (Fig. 2(J)). We observed the same phenomenon of hydration time dependent growth with vesicles made from pure DOPC (Fig. S4†).
Additionally, small hydrophilic molecules such as Alexa fluor are easily encapsulated in the bacterial membrane mimicking models with very high efficiency (Fig. 2(K), (L), S2(D1) and (D2)†). For example, 1 mM Alexa Fluor 350 dye is mixed with the buffer containing salt and added on the dried lipid film to swell. Encapsulation of the water-soluble Alexa fluor dye at 1.5 minutes and 30 minutes of hydration time is shown in Fig. 2(K) and (L).
Multiple compartments in vesicles
An inverse-phase precursor coating of lipids is used on the PVA-gel to construct multiple compartments of vesicles in a giant vesicle called a vesosome (Details in the Experimental section). The size-dependence on hydration time factor is exploited for the multi-compartment formation of vesicles. The smaller sized vesicles collected at 1 minute 30 seconds are mixed with lipid solution in chloroform. The emulsion mixture is then coated on the PVA film, subsequently dried to remove water/solvents and is swelled with the same physiological buffer at pH 7. We observe vesicles inside giant-vesicles of varying size (Fig. 3(A), (B) and S5†) and in some cases, we also observed aggregates of lipids (Fig. S6†). The multi-compartment vesicles are constructed using both GN vesicles (Fig. 3(A)) and GP vesicles (Fig. 3(B)). In most of the experiments, we observed fusion/budding of the internal vesicle with the main vesicle with time (Videos S1 and S2†). We also showed the multi-compartment formation of zwitterionic lipids such as DOPC using the inverse-phase precursor coating of liposomes (Video S3†). We suggest that in the presence of salts, due to electrostatic charge screening of lipid head groups, there might be no repulsion/barrier to prevent the fusion of vesicles. Accordingly, manipulating the charge on the vesicles could lead to more stable multiple compartments.12 Another strategy to create stable multiple compartments is achieved by including PEG 8000 in the buffer to the DOPC:DOPG mixture lipid film (Video S4†). However, the efficiency of encapsulating vesicles in GUVs is low compared to that of microfluidic techniques.14
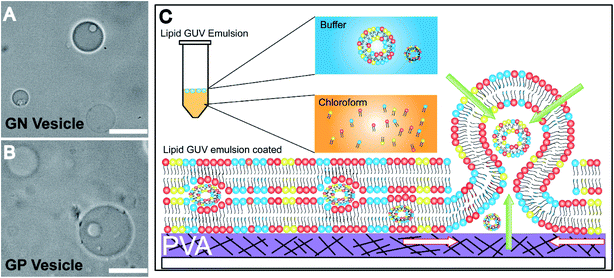 |
| Fig. 3 Microcompartments produced by the inverse phase method (A) Gram-negative vesosome, (B) Gram-positive vesosome, and (C) schematic mechanism showing the formation of microcompartments using the inverse phase coating on PVA. The aqueous buffer can enter and swell the vesicles using all the paths represented by green and white arrows, and the vesicles enter into the lumen of other vesicles using routes depicted by white arrows only. All images are captured at 60× (N.A. 0.75); conditions – 137 mM NaCl, 2.7 mM KCl, 10 mM HEPES, and pH 7. Scale bar: 20 μm. | |
The mechanism of formation of vesosomes is shown in Fig. 3(C). The preformed vesicles added in chloroform are intact in the aqueous-solvent droplets. The mixture when coated on the PVA-film forms several bilayers of lipids with preformed vesicles trapped between these layers. The addition of buffer to this vesicle-lipid film results in swelling to form a vesicle-in-vesicle mixture. The success of observing a vesosome depends on factors like entrapping a sufficient number of preformed vesicles in between the lipid layers. As the multiple compartments in GP and GN vesicles are not very stable and tend to fuse over time, we observed a lot of vesicles which had blebs on the surface with increasing time after collecting the sample. However, in the presence of PEG, the DOPC
:
DOPG multi-compartment vesicles were very stable.
This is to our knowledge the first report showing vesicle formation under a range of pH conditions in the presence of salts with such complex lipid mixtures. Most importantly, multiple compartments are constructed using the gel-assisted swelling method under physiological natural conditions. Also, previous studies have attempted to construct vesicles using single and binary lipid mixtures under non-physiological conditions, despite observing lipid and ion dependent effects on the activity of antimicrobials32,39 and have not explored specifically the acidic pH parameter for example in LPS incorporated E. coli vesicles.40
Remarkably, the Gram-negative vesicle model developed here represents the cytoplasmic membrane quite accurately. We attempted to prepare vesicles using E. coli total-lipid extracts but they had a very low tendency to form vesicles due to high concentrations of LPS in the mixture (Fig. S7(A)†). Notably, here we developed a GP vesicle model system using ternary lipid mixtures. Further, we successfully formed vesicles with varying concentrations of lysyl-PG (5% and 20% data not shown) but mixtures with >35% wt/v (up to 40% was attempted) of lysyl-PG did not form any vesicles (Fig. S7(B)†). Based on these data, we suggest that such models will have significant applications in bottom-up biology to build a simplistic isolated-model-cell.
Next, we quantified the diffusion of fluoroquinolone antibiotics under different pH conditions and studied the permeability properties of anti-microbial peptides to validate the utility and biocompatibility of the vesicles.
Bio-compatibility of the vesicles
Quantifying drug transport.
We quantified the diffusion of antibiotics by immobilizing giant vesicles in agarose for ease of imaging (details in ‘Preparation of stage for GUV imaging and immobilization in agarose for transport experiments’ and ‘Imaging’ sections in the Methods section of the ESI). The intrinsic fluorescence of the antibiotics is used as a detection system, and diffusion is measured as a change in intensity inside vesicles in a time-dependent manner (ESI text 1†).
We studied the diffusion process of antibiotics norfloxacin and ciprofloxacin inside both Gram-negative and Gram-positive membrane mimicking GUVs at pH 7 and pH 5. The vesicles used for diffusion experiments are collected after 30 minutes of hydration time. The antibiotics are amphoteric, and at pH 7 and at pH 5 they are positively charged.41 An increase in the fluorescence inside the vesicles over time indicates successful diffusion of antibiotics across membranes into the lumen of the vesicles. For example, ciprofloxacin diffusion over time inside GP vesicles is shown in Fig. 4(A) at pH 7. We observe reducing contrast inside the vesicles with respect to the outside of the vesicles for both the antibiotics with GP and GN vesicles, (Fig. S8 and S9†),which confirms the diffusion of antibiotics from outside to inside.
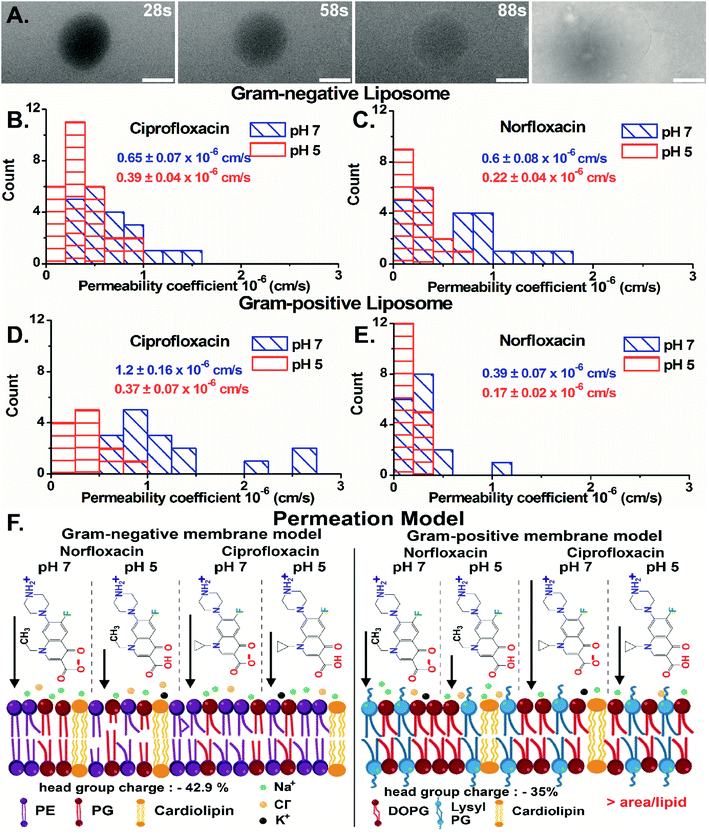 |
| Fig. 4 (A) Diffusion of the antibiotic ciprofloxacin in a time dependent manner across the Gram-positive GUV model at pH 7; histogram of the antibiotic permeability coefficients at pH 7 and pH 5 across Gram-negative vesicles for (B) ciprofloxacin and (C) norfloxacin and Gram-positive vesicles for (D) ciprofloxacin and (E) norfloxacin. (F) Schematic of the permeation model along with chemical structures of the antibiotics at respective pH and the lipid packing is shown in GN and GP vesicles. The arrow beside the antibiotics represents the diffusion rate qualitatively. | |
Importantly, we have quantified the diffusion rates by calculating the fluorescence intensity inside the vesicle which is a direct measure of the antibiotic concentration using ImageJ-Fiji software (details in ESI text 1.1 and 1.2†). For GN vesicles and GP vesicles, the scatter plot of the normalized intensity (ΔI = (Iout − Iin)/Iout) of the antibiotics, norfloxacin and ciprofloxacin, at pH 5 and pH 7 at two-time points is plotted in Fig. S10(A) and (B).† The ΔI calculated at two different time points is used to determine the permeability coefficient individually for each vesicle (as described in eqn (1) in the Experimental Section - analysis). The histograms of permeability coefficients are shown in Fig. 4(B) and (C) for GN vesicles and Fig. 4(D) and (E) for GP vesicle models respectively. The mean and standard errors of the mean are calculated from the statistics represented in the histograms. In GN vesicles, norfloxacin at pH 7 has a permeability of 0.6 ± 0.08 × 10−6 cm s−1 (mean ± S.E. of the mean calculated from 26 individual GUVs), and at pH 5 the value is 0.22 ± 0.04 × 10−6 cm s−1 (n = 16). Similar results are obtained for ciprofloxacin where at pH 7 it has a higher permeability coefficient of 0.65 ± 0.07 × 10−6 cm s−1 (n = 21) as compared to pH 5 when the coefficient is 0.39 ± 0.04 × 10−6 cm s−1 (n = 17) (Fig. 4(B) and (C)).
In GP vesicles, norfloxacin at pH 7 has a permeability coefficient of 0.39 ± 0.07 × 10−6 cm s−1 (mean and S.E. of the mean from n = 11), whereas at pH 5 the value is less than half with a coefficient of 0.17 ± 0.022 × 10−6 cm s−1 (n = 17). Similar results are obtained for ciprofloxacin where at pH 7 it has a better permeability coefficient of 1.2 ± 0.16 × 10−6 cm s−1 (n = 16) as compared to pH 5 where the coefficient is 0.37 ± 0.07 × 10−6 cm s−1 (n = 12) (Fig. 4(D) and (E)).
Based on these data, we conclude that the zwitterionic form of both antibiotics (at pH 7) diffuses better across model membranes than the cationic form which predominantly exists at pH 5. Our results showed that norfloxacin has higher permeability in Gram-negative membrane mimicking liposomes, whereas ciprofloxacin has much better transport in Gram-positive liposomes. We suggest that the differences observed in the permeability coefficients of antibiotics are due to different lipid compositions in the vesicles. There are two main molecular differences in both the vesicle models. For example, the lipid head groups in GN bacterial liposomes (total 42.9% negative charge on the head group) are more negatively charged than those in GP membrane mimics (35% negative charged) (Fig. 4(F)) making it more susceptible to polar compounds like norfloxacin. Another important difference in the models is the packing of the lipid chain. GN vesicles consist of a mixture of different chain lengths of lipids with a significant proportion of C16
:
0 (36%) (Fig. S11†) as opposed to GP vesicles, which have a uniform chain length (18
:
1 in a mixture). Our results indicate that the increased order of packing, due to the presence of mainly saturated lipid chains in Gram-negative liposomes, lowers the diffusion of hydrophobic molecules. In contrast, disordered packing due to unsaturated lipids35 in Gram-positive vesicles provides higher area/lipid chain lengths and hence promotes the diffusion of ciprofloxacin more effectively. Several orders of magnitude of difference are observed in the permeability coefficients of antibiotics reported in simplified mimicking models37,42 as compared to the value reported here. Notably, our studies are conducted in the presence of salts and therefore, electrostatic charge screening of antibiotics can validate the high transport rate of antibiotics at pH 5 as compared to that in previous studies.42 Fluoroquinolone antibiotics have a lower efficacy at pH 5 as compared to pH 7 in vivo.43 The slower accumulation rates of these antibiotics at acidic pH observed in our mimicking models explain the in vivo activity as opposed to no transport rates observed in simplified models. The drug diffusion data further validate and indeed emphasize the use of bacterial models developed here.
Antimicrobial peptide, dermcidin, and its permeability properties
To validate the versatility of the vesicle models, we studied the interaction of dermcidin peptide DCD-1L (Fig. 5(A)) with Gram-positive model vesicles under two pH conditions. Dermcidin is an antimicrobial peptide produced in the human sweat glands, cleaved or processed into truncated peptides and secreted in human sweat.44 DCD-1L is a processed dermcidin, a rare anionic peptide with 48 residues. We incubated the DCD-1L peptide with GP vesicles at a concentration of 0.5 μM at pH 5 and of 0.5–200 μM at pH 7. We studied the transport of membrane impermeant fluorescent dye Alexa Fluor 350 into the vesicle lumen in the presence and absence of the dermcidin peptide. The analysis is performed similar to antibiotic diffusion experiments, using ImageJ on uneven-illumination corrected images. Iin, the intensity of the dye inside the vesicles and Iout, the intensity of the dye outside the vesicles are calculated and plotted (Fig. 5(B) and (C)) for the vesicles without (control) and with the peptide. At pH 5, we observed an increase in Iin/Iout, in the presence of the dermcidin peptide (0.5 μM) depicting transport of the dye from the outside to the lumen of the vesicle. At a higher concentration of dermcidin (like 10–20 μM) incubation, we observed very few vesicles surviving. There is no increase observed in the case of the control at pH 5 (Fig. 5(B)N > 3 from 3 independent batches). The normalised intensity of individual vesicles at pH 5 shows the stochastic process of dye uptake (Fig. S12 A†). Up to 30% of the vesicles at pH 5 show no change in intensity over time, which might be due to low incubation times used in these experiments or the lamellarity of the vesicles. At pH 5, there is an increase in the intensity inside the lumen of the vesicles with time, unlike the control (Fig. 5(D) and (E)). In contrast at pH 7, there is no change in Iin/Iout at 0.5 μM (Fig. S12 B†) and at the highest concentration of dermcidin used i.e. 50 μM (Fig. 5(C)N > 4, 3 independent batches). At pH 7, even at 200 μM incubation the pre-formed GUVs show no change in the normalised fluorescence intensities (Fig. S12(B), more details in the text in the ESI below the figure†). We observe no change in the fluorescence intensity in the lumen of the vesicles over time at pH 7 even at the highest concentration used (50 μM), similar to the control (Fig. 5(F) and (G)).
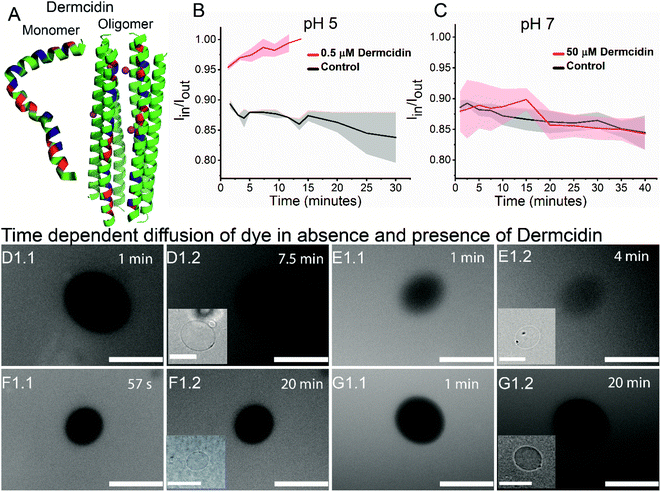 |
| Fig. 5 Antimicrobial peptide permeability properties. (A) Dermcidin monomer and dermcidin oligomer stabilised by Zn2+ interacting with anti-parallel monomers made in the PyMOL Molecular Graphics System (the green colored residues are neutral, red colored are negatively charged and blue colored are positively charged residues at neutral pH and only two monomers are color coded in the oligomer for ease of viewing). Line graph depicting the Iin/Iout values with time (s) at (B) pH 5 and (C) pH 7. (D) Time-dependent uptake of Alexa Fluor 350 in the Gram-positive vesicle: without dermcidin at pH 5 (D1.1) at 1 minute, (D1.2) at 7.5 minutes, with dermcidin at pH 5 (E1.1) at 1 minute, and (E1.2) at 4 minute, without dermcidin at pH 7 (F1.1) at 57 seconds, (F1.2) at 20 minutes, and with dermcidin at pH 7 (G1.1). At 1 minute, and (G1.2) at 20 minutes. Scale bar 20 μm. | |
We can conclude that at pH 5, dermcidin has pore-forming activity that allows the uptake of small molecular weight molecules (like Alexa Fluor in this case). At pH 5 or under acidic conditions, dermcidin has zero net charge45 which might promote binding to the highly negatively charged GP vesicles and oligomerisation to form pores large enough to transport small molecules as reported in multilamellar liposomes at pH 6.46 However, at pH 7 this activity is not seen and no uptake of dye is observed in the vesicles suggesting the absence of large size oligomers of the peptide. At pH 7 the peptide is negatively charged and forms Zn2+ stabilised oligomers,47 and similar oligomeric pores can be expected to form at pH 5.
Functionality of liposomes
We investigated the unilamellarity of the vesicles by reconstitution of membrane proteins that form specific pores in the vesicles to confirm the functionality of liposomes.48 Here, we used a pore-forming toxin, alpha-hemolysin derived from Staphylococcus aureus, which assembles in the membranes to form uniform heptameric pores.49,50 We incubated the pre-formed GN vesicles with alpha-hemolysin monomers (0.4–0.6 μM) at both pH 5 and pH 7. Then we investigated the uptake of membrane-impermeant dye Alexa Flour 350 across vesicles without any protein and with alpha-hemolysin in a time dependent manner (Fig. 6(A)). Notably, membrane pores can span only in single lipid bilayers and not in multilamellar membranes. Therefore, the uptake and visualization of the dye is possible only in the unilamellar vesicles and not multilamellar membranes.
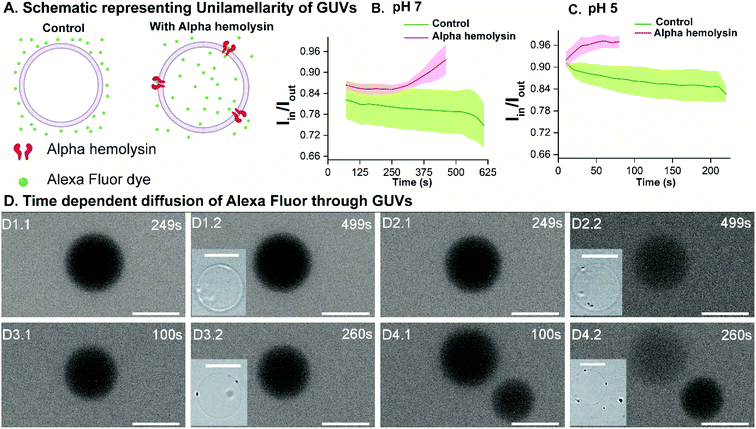 |
| Fig. 6 Unilamellarity of vesicles. (A) Schematic representing the control vesicle (without alpha hemolysin) and the influx of Alexa Fluor through the proteoliposome. Line graph depicting the Iin/Iout values with time (s) at (B) pH 7 and (C) pH 5. (D) Time-dependent uptake of Alexa Fluor 350 in the Gram-negative vesicle, without alpha hemolysin at pH 7 (D1.1) at 249 s and (D1.2) at 499 s, with alpha hemolysin at pH 7 (D2.1) at 249 s and (D2.2) at 499 s, without alpha hemolysin at pH 5 (D3.1) at 100 s and (D3.2) at 260 s, and with alpha hemolysin at pH 5 (D4.1) at 100 s and (D4.2) at 260 s. Scale bar: 20 μm. | |
The analysis is performed similarly to peptide permeability experiments as described above and in the ESI.† In the case of protein reconstituted liposomes, Iin increases with time, indicating diffusion of dye across the protein inside the vesicle lumen over time under both pH conditions. Consequently, an increase in Iin/Iout is observed (Fig. 6(B) and (C)). Importantly, in the control liposomes, Iin/Iout does not change and even decreases slightly due to bleaching over a more extended period as compared to proteo-liposomes (Fig. 6(C)). Additionally, this establishes the non-leaky nature of the vesicles embedded in agarose for at least 10–15 minutes. We observe an increase in the fluorescence intensity inside proteo-vesicles (Fig. 6(D2.1) and (D2.2) at pH 7 and Fig. 6(D4.1) and (D4.2) at pH 5) as opposed to their corresponding controls (Fig. 6(D1.1), (D1.2) pH 7 and (D3.1), (D3.2) pH 5) in a time-dependent manner. Notably, some of the vesicles are still dark with little/no significant change in intensity inside the vesicles over time, which we propose to be most likely due to the varying degree of protein reconstitution in the vesicles (One vesicle remains dark in Fig. 6(D4.1) and (D4.2)). Another hypothesis might be that some proportion (close to 5–10%) of the vesicles are multilamellar, as observed for other methods as well.12 Also, we present the data for different individual GUVs measured at both pH 5 (n = 8) and pH 7 (n = 9) and corresponding Iin/Iout changing at different rates confirming varying protein reconstitution in different GUVs (Fig. S13†). The change in Iin in different vesicles is observed at different time points and this revalidates varying protein reconstitution efficiencies.
We further quantified the permeability coefficients as described by eqn (1) in the Analysis section. At pH 7 the permeability coefficient of Alexa Fluor dye is 0.17 ± 0.04 × 10−6 cm s−1 and at pH 5 the value is slightly higher, 0.27 ± 0.08 × 10−6 cm s−1. The higher rate at pH 5 might indicate better insertion efficiency of the pore into the liposomes at the same concentrations of alpha-hemolysin, in agreement with previous reports.12,28,51 Also, previous reports have reconstituted for example, ABC transporters in giant vesicles from E. coli extracts, however under non-physiological conditions.52 In our study, we have reconstituted membrane proteins in complex E. coli lipid vesicles in the presence of high salt concentrations and a range of pH conditions close to natural membrane conditions. Here we clearly demonstrate the versatility of the vesicle models constructed here for controlled uptake of hydrophilic molecules via membrane protein pores.
Conclusion
We have successfully engineered Gram-negative and Gram-positive membrane mimicking models resembling natural membranes. The key advantage is that the vesicle preparation requires no special equipment for high yield and can be adapted to encapsulate biomolecules. Multi-compartment vesicles are formed with a range of lipid/lipid mixtures under physiological conditions. The utility of the vesicles is validated by quantifying the antibiotic transport rate through membranes. Controlled uptake of antibiotics is possible by modulating ions and lipid-composition. The models are utilized to study the permeability properties of antimicrobials.39 Quantifying uptake of hydrophilic molecules via membrane proteins reconstituted in the vesicle model substantiates the functionality of the vesicles. Such bacterial membrane mimicking models have not been reported in the literature to our knowledge. Our bacterial models created here could be adapted for studying important biological processes like cell division53,54 or for bottom-up synthetic biology to build microreactors or simplistic-cells.
Experimental section
Optical setup
The fluorescence imaging of GUVs is performed using an EVOS FL Auto2 epifluorescence inverted microscope and a Carl Zeiss Axio imager2 upright microscope with an Apotome module. The camera used in EVOS for imaging is a high sensitivity 1.3 MP CMOS monochrome camera with 1328 × 1048 pixels. A 40× phase contrast objective (N.A. – 0.65×) and 60× objective (N.A. – 0.75) are used for both bright field and fluorescence imaging of GUVs.
The camera used in Carl Zeiss is Axiocam 503 monochrome with 2.83 megapixels: 1936 (H) × 1460 (V) sensor pixel count. A 40× objective with a numerical aperture of 0.75 is used to spot GUVs in agarose and subsequent fluorescence measurements with antibiotics/peptides are performed.
A DAPI filter cube (Ex: 357/44; Em: 447/60) is used for epi-fluorescence imaging since the excitation and emission wavelength of auto-fluorescing antibiotics/dye used here falls in this range. A GFP filter cube (Ex: 470/40; Em: 525/50) in the apotome module is used for imaging of vesicles with an Atto-labelled lipid incorporated in them.
Giant unilamellar vesicle formation
Briefly, giant unilamellar vesicles (GUVs) mimicking the membrane of Gram-negative bacteria are prepared using an E. coli polar extract consisting of 67% phosphatidylethanolamine (PE), 23.2% phosphatidyl glycerol (PG), and 9.8% cardiolipin (CA). For GUVs mimicking a Gram-positive bacterial membrane, a mixture of 60% w/v 1,2-dioleoyl-sn-glycero-3-phospho-(1′-rac-glycerol) (sodium salt) (DOPG), 35% w/v 1,2-dioleoyl-sn-glycero-3-[phospho-rac-(3-lysyl(1-glycerol))] (chloride salt) (lysyl PG) and 5% w/v 1′,3′-bis[1,2-dioleoyl-sn-glycero-3-phospho]-glycerol (sodium salt) (cardiolipin/CA) is used to form vesicles. The mixture is formulated based on the lipid composition found in a Gram-positive bacterial membrane such as that of Staphylococcus aureus.55 For fluorescence imaging of vesicles, 0.1 mol% Atto-DOPE 488 is added in both the vesicle model chloroform solutions. The GUVs are formed using the gel-assisted method.8 All lipid stocks are prepared in chloroform (100%), and a lipid stock of desired concentration (0.5–1 mg mL−1, 20 μL) is added onto the polyvinyl alcohol (PVA) film and spread evenly till the chloroform evaporates. The slides are further kept in a desiccator for 30 minutes to completely evaporate the solvent. Buffer with a composition of 137 mM NaCl, 2.7 mM KCl in 5 mM acetate (for pH 5), or 10 mM HEPES (for pH 7) (150 μL) is added unless otherwise mentioned to the lipid film for GUV formation and sealed using a greased O-ring. The sample is incubated in a water bath at 40 °C for 30 minutes. The growth of vesicles is viewed under a microscope. The vesicles are collected using cut tips transferred into Eppendorf tubes and imaged in solution. The details of the vesicle formation are provided in the ESI Methods section (Fig. S1†). All the antibiotic/membrane protein/peptide dye transport assays are performed on vesicles without any fluorescent lipid as described above.
Time-dependent vesicle growth
Buffer is added to the lipid films for the formation of GUVs. For GN and GP vesicles preheated buffer containing 137 mM NaCl and 2.7 mM KCl in 10 mM HEPES (pH 7) is added while for DOPC vesicles buffer containing 50 mM KCl and 100 mM sucrose in 10 mM HEPES (pH 7) is added. The PVA film is imaged and then the sample is collected at 1.30, 5, 15, and 30 minutes for GP and GN vesicles at 2, 15 and 30 minutes for DOPC. The collected vesicles are then imaged using an epifluorescence microscope with a 60× objective (N.A. 0.75).
Encapsulation
To check the encapsulation efficiency of the vesicles, 1 mM Alexa Fluor 350 is added to 137 mM NaCl and 2.7 mM KCl in 10 mM HEPES (pH 7) buffer to hydrate the dried lipid film. The samples are collected at 1.30 and 30 minutes. The collected GUV sample is then diluted at least 3 times in the respective buffer and imaged in the epifluorescence microscope with a 60× objective (N.A. 0.75). The dilution is performed to reduce/dilute the fluorescence signal from the un-encapsulated dye in solution to image better the vesicles with encapsulated dye molecules.
Multi-compartment vesicle formation
Vesicles are collected at 1 minute 30 seconds and they have small-sized vesicles. 5 μL of the collected GUV sample is added to 25 μL of a lipid solution in chloroform (0.5–1 mg mL−1) and tapped gently to form a lipid–GUV emulsion. 20 μL of the emulsion was coated to form a dried lipid film. After the complete evaporation of chloroform, respective buffers are added to the lipid film. For GN and GP vesicles buffer containing 137 mM NaCl and 2.7 mM KCl in 10 mM HEPES (pH 7) is added and incubated for 30 minutes at 40 °C, and for DOPC vesicles buffer containing 50 mM KCl and 100 mM sucrose in 10 mM HEPES (pH 7) is added. For better stability the buffer containing 5% w/v PEG 8000 (with 5 mM NaCl, 50 mM sucrose, 10 mM HEPES pH 7) in the case of DOPC
:
DOPG (1
:
1) vesicles is added and then incubated at 25 °C. The sample was then collected and imaged using the epifluorescence microscope with a 60× objective (N.A. 0.75).
Analysis
The permeability coefficient was determined using eqn (1), as described previously:26 | 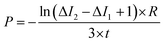 | (1) |
where P = permeability coefficient, R = radius of the vesicle, t = time between initial and final time point for measuring intensity, and t= tf − t0
Iout is the intensity outside the vesicle, I1 is intensity inside the vesicle at t = initial time, and I2 is the intensity inside the vesicle at t = tf. The fluorescence intensities are determined on the uneven-illumination corrected images (as described in detail in ESI text 1.1 and 1.2†).
Conflicts of interest
There are no conflicts to declare.
Acknowledgements
GM and KSN contributed equally to this paper. This work was supported by the ‘Innovative Young Biotechnologist Award’ by the Department of Biotechnology, Government of India (BT/11/IYBA/2018/09) awarded to HB. HB acknowledges the ‘Biotechnology Career Advancement and Reorientation Programme’ of the Department of Biotechnology, Government of India (BT/PR18365/BIC/101/487/2016). HB thanks Dr A. Ajayaghosh, the Director, CSIR-NIIST, for support for the lab facilities. HB thanks Dr Rajeev K. Sukumaran, HOD, and Dr Ramesh Kumar N for the support for the equipment. 1A and 4F are created with Biorender.com.
References
- R. Dimova, Annu. Rev. Biophys., 2019, 48, 93–119 CrossRef CAS PubMed.
- E. Rideau, R. Dimova, P. Schwille, F. R. Wurm and K. Landfester, Chem. Soc. Rev., 2018, 47, 8572–8610 RSC.
- P. Walde, K. Cosentino, H. Engel and P. Stano, ChemBioChem, 2010, 11, 848–865 CrossRef CAS PubMed.
- T. M. Allen and P. R. Cullis, Adv. Drug Delivery Rev., 2013, 65, 36–48 CrossRef CAS PubMed.
- K. Karamdad, J. W. Hindley, G. Bolognesi, M. S. Friddin, R. V. Law, N. J. Brooks, O. Ces and Y. Elani, Chem. Sci., 2018, 9, 4851–4858 RSC.
- X. Wang, L. Tian, H. Du, M. Li, W. Mu, B. W. Drinkwater, X. Han and S. Mann, Chem. Sci., 2019, 10, 9446–9453 RSC.
- S. Deshpande, S. Wunnava, D. Hueting and C. Dekker, Small, 2019, 15, 1902898 CrossRef PubMed.
- A. Weinberger, F.-C. Tsai, G. H. Koenderink, T. F. Schmidt, R. Itri, W. Meier, T. Schmatko, A. Schröder and C. Marques, Biophys. J., 2013, 105, 154–164 CrossRef CAS PubMed.
- S. Pautot, B. J. Frisken and D. A. Weitz, Proc. Natl. Acad. Sci. U. S. A., 2003, 100, 10718–10721 CrossRef CAS PubMed.
- S. Ota, S. Yoshizawa and S. Takeuchi, Angew. Chem., Int. Ed., 2009, 48, 6533–6537 CrossRef CAS PubMed.
- J. C. Stachowiak, D. L. Richmond, T. H. Li, A. P. Liu, S. H. Parekh and D. A. Fletcher, Proc. Natl. Acad. Sci., 2008, 105, 4697–4702 CrossRef CAS PubMed.
- K. Göpfrich, B. Haller, O. Staufer, Y. Dreher, U. Mersdorf, I. Platzman and J. P. Spatz, ACS Synth. Biol., 2019, 8, 937–947 CrossRef PubMed.
- Y. Elani, A. Gee, R. V. Law and O. Ces, Chem. Sci., 2013, 4, 3332–3338 RSC.
- N.-N. Deng, M. Yelleswarapu, L. Zheng and W. T. S. Huck, J. Am. Chem. Soc., 2017, 139, 587–590 CrossRef CAS PubMed.
- K. S. Horger, D. J. Estes, R. Capone and M. Mayer, J. Am. Chem. Soc., 2009, 131, 1810–1819 CrossRef CAS PubMed.
- A.-E. Saliba, I. Vonkova, S. Ceschia, G. M. Findlay, K. Maeda, C. Tischer, S. Deghou, V. van Noort, P. Bork, T. Pawson, J. Ellenberg and A.-C. Gavin, Nat. Methods, 2014, 11, 47–50 CrossRef CAS PubMed.
- I. Kusters, A. M. van Oijen and A. J. M. Driessen, ACS Nano, 2014, 8, 3380–3392 CrossRef CAS PubMed.
- A. Nieth, C. Verseux, S. Barnert, R. Süss and W. Römer, Expert Opin. Drug Delivery, 2015, 12, 1411–1424 CrossRef PubMed.
- K. Kamiya and S. Takeuchi, J. Mater. Chem. B, 2017, 5, 5911–5923 RSC.
- J. S. Hansen, K. Elbing, J. R. Thompson, N. Malmstadt and K. Lindkvist-Petersson, Chem. Commun., 2015, 51, 2316–2319 RSC.
- A. Martos, M. Jiménez, G. Rivas and P. Schwille, Trends Cell Biol., 2012, 22, 634–643 CrossRef CAS PubMed.
- T. Furusato, F. Horie, H. T. Matsubayashi, K. Amikura, Y. Kuruma and T. Ueda, ACS Synth. Biol., 2018, 7, 953–961 CrossRef CAS PubMed.
- E. J. Cabré, A. Sánchez-Gorostiaga, P. Carrara, N. Ropero, M. Casanova, P. Palacios, P. Stano, M. Jiménez, G. Rivas and M. Vicente, J. Biol. Chem., 2013, 288, 26625–26634 CrossRef PubMed.
- F. Fanalista, A. Birnie, R. Maan, F. Burla, K. Charles, G. Pawlik, S. Deshpande, G. H. Koenderink, M. Dogterom and C. Dekker, ACS Nano, 2019, 13, 5439–5450 CrossRef CAS PubMed.
- S. Li, P. C. Hu and N. Malmstadt, Biophys. J., 2011, 101, 700–708 CrossRef CAS PubMed.
- J. Cama, C. Chimerel, S. Pagliara, A. Javer and U. F. Keyser, Lab Chip, 2014, 14, 2303–2308 RSC.
- C. Peetla, S. Vijayaraghavalu and V. Labhasetwar, Adv. Drug Delivery Rev., 2013, 65, 1686–1698 CrossRef CAS PubMed.
- J. Cama, H. Bajaj, S. Pagliara, T. Maier, Y. Braun, M. Winterhalter and U. F. Keyser, J. Am. Chem. Soc., 2015, 137, 13836–13843 CrossRef CAS PubMed.
- P. P. Parui, Y. Sarakar, R. Majumder, S. Das, H. Yang, K. Yasuhara and S. Hirota, Chem. Sci., 2019, 10, 9140–9151 RSC.
- E. Bárány-Wallje, S. Keller, S. Serowy, S. Geibel, P. Pohl, M. Bienert and M. Dathe, Biophys. J., 2005, 89, 2513–2521 CrossRef PubMed.
- L. Yang, V. D. Gordon, A. Mishra, A. Som, K. R. Purdy, M. A. Davis, G. N. Tew and G. C. L. Wong, J. Am. Chem. Soc., 2007, 129, 12141–12147 CrossRef CAS PubMed.
- Y.-F. Chen, T.-L. Sun, Y. Sun and H. W. Huang, Biochemistry, 2014, 53, 5384–5392 CrossRef CAS PubMed.
- M.-T. Lee, W.-C. Hung, F.-Y. Chen and H. W. Huang, Proc. Natl. Acad. Sci. U. S. A., 2008, 105, 5087–5092 CrossRef CAS PubMed.
- H. W. Huang, Biochim. Biophys. Acta, Biomembr., 2006, 1758, 1292–1302 CrossRef CAS PubMed.
- Y.-M. Zhang and C. O. Rock, Nat. Rev. Microbiol., 2008, 6, 222–233 CrossRef CAS PubMed.
- M. Weiss, J. P. Frohnmayer, L. T. Benk, B. Haller, J.-W. Janiesch, T. Heitkamp, M. Börsch, R. B. Lira, R. Dimova, R. Lipowsky, E. Bodenschatz, J.-C. Baret, T. Vidakovic-Koch, K. Sundmacher, I. Platzman and J. P. Spatz, Nat. Mater., 2018, 17, 89–96 CrossRef CAS PubMed.
- S. Purushothaman, J. Cama and U. F. Keyser, Soft Matter, 2016, 12, 2135–2144 RSC.
- M. C. Sousa, Nature, 2019, 576, 389–390 CrossRef CAS PubMed.
- W. Kim, W. Zhu, G. L. Hendricks, D. Van Tyne, A. D. Steele, C. E. Keohane, N. Fricke, A. L. Conery, S. Shen, W. Pan, K. Lee, R. Rajamuthiah, B. B. Fuchs, P. M. Vlahovska, W. M. Wuest, M. S. Gilmore, H. Gao, F. M. Ausubel and E. Mylonakis, Nature, 2018, 556, 103–107 CrossRef CAS PubMed.
- J. Kubiak, J. Brewer, S. Hansen and L. A. Bagatolli, Biophys. J., 2011, 100, 978–986 CrossRef CAS PubMed.
- H. Nikaido and D. G. Thanassi, Antimicrob. Agents Chemother., 1993, 37, 1393–1399 CrossRef CAS PubMed.
- M. Schaich, J. Cama, K. Al Nahas, D. Sobota, H. Sleath, K. Jahnke, S. Deshpande, C. Dekker and U. F. Keyser, Mol. Pharm., 2019, 16, 2494–2501 CrossRef CAS PubMed.
- H. J. Zeiler, Drugs Exp. Clin. Res., 1985, 11, 335–338 CAS.
- B. Schittek, R. Hipfel, B. Sauer, J. Bauer, H. Kalbacher, S. Stevanovic, M. Schirle, K. Schroeder, N. Blin, F. Meier, G. Rassner and C. Garbe, Nat. Immunol., 2001, 2, 1133–1137 CrossRef CAS PubMed.
- M. Paulmann, T. Arnold, D. Linke, S. Özdirekcan, A. Kopp, T. Gutsmann, H. Kalbacher, I. Wanke, V. J. Schuenemann, M. Habeck, J. Bürck, A. S. Ulrich and B. Schittek, J. Biol. Chem., 2012, 287, 8434–8443 CrossRef CAS PubMed.
- N. Puthumadathil, P. Jayasree, K. Santhosh Kumar, K. M. Nampoothiri, H. Bajaj and K. R. Mahendran, Biomater. Sci., 2019, 7, 3226–3237 RSC.
- C. Song, C. Weichbrodt, E. S. Salnikov, M. Dynowski, B. O. Forsberg, B. Bechinger, C. Steinem, B. L. de Groot, U. Zachariae and K. Zeth, Proc. Natl. Acad. Sci. U. S. A., 2013, 110, 4586–4591 CrossRef CAS PubMed.
- S. Deshpande, Y. Caspi, A. E. C. Meijering and C. Dekker, Nat. Commun., 2016, 7, 10447 CrossRef CAS PubMed.
- L. Song, M. R. Hobaugh, C. Shustak, S. Cheley, H. Bayley and J. E. Gouaux, Science, 1996, 274, 1859–1866 CrossRef CAS PubMed.
- Y.-L. Ying, H.-Y. Wang, T. C. Sutherland and Y.-T. Long, Small, 2011, 7, 87–94 CrossRef CAS PubMed.
- M. Chiba, M. Miyazaki and S. Ishiwata, Biophys. J., 2014, 107, 346–354 CrossRef CAS PubMed.
- E. R. Geertsma, N. A. B. Nik Mahmood, G. K. Schuurman-Wolters and B. Poolman, Nat. Protoc., 2008, 3, 256–266 CrossRef CAS PubMed.
- M. Osawa and H. P. Erickson, Proc. Natl. Acad. Sci. U. S. A., 2013, 110, 11000–11004 CrossRef CAS PubMed.
- L. D. Renner and D. B. Weibel, J. Biol. Chem., 2012, 287, 38835–38844 CrossRef CAS PubMed.
- K. Lohner, E. Sevcsik and G. Pabst, Adv. Planar Lipid Bilayers Liposomes, 2008, 6, 103–137 CAS.
Footnotes |
† Electronic supplementary information (ESI) available. See DOI: 10.1039/d0sc00084a |
‡ Equally contributed. |
|
This journal is © The Royal Society of Chemistry 2020 |
Click here to see how this site uses Cookies. View our privacy policy here.