Belonging in general chemistry predicts first-year undergraduates’ performance and attrition
Received
17th February 2020
, Accepted 22nd May 2020
First published on 1st June 2020
Abstract
Feeling a sense of belonging in a learning environment can have positive effects on student success. The impact of this psychosocial variable on undergraduates’ achievement and retention has been demonstrated in STEM disciplines, especially for women within physical sciences where large disparities in gender representation persist. The current study explores the relationship between belonging and student success in undergraduate chemistry, where greater gender parity has recently emerged. In particular, this research investigates the belonging of first-year students enrolled in a two-semester General Chemistry course sequence. The study begins by examining whether students’ early sense of belonging in the course, indexed by two survey measures (perceived belonging, belonging uncertainty) varies depending on their demographics and academic preparation. The belonging measures are then used as predictors of performance in General Chemistry 1 and 2 and attrition from one semester to the next. Paralleling research in other STEM disciplines, the results show that female students, especially those from underrepresented minority groups, reported lower belonging and higher uncertainty than male students within the first weeks of the course. After accounting for demographics, preparation, and participation in a course supplemental program, the belonging measures predicted performance and attrition for all students. These findings suggest that course-level belonging in General Chemistry can have practical consequences for student success, and early disparities in belonging may have downstream effects on the retention of women and other groups underrepresented in STEM. Strategies for creating an inclusive and engaging environment that supports the success of all students are discussed.
Introduction
Extensive research has examined the factors influencing student success in undergraduate general chemistry, showing the impact of individual differences in cognitive (e.g., Cracolice and Busby, 2015), educational (e.g., Tai et al., 2005), and affective variables (e.g., Lewis et al., 2009). This introductory course has garnered ongoing attention because it is an early requirement for students planning to pursue careers in STEM or healthcare. This line of research holds special significance in the United States, where national initiatives have challenged STEM educators to help develop a more robust and equitable STEM workforce in order to maintain the nation's global standing (National Academy of Sciences, 2011; Olson and Riordan, 2012). The current study aims to support this effort and enrich the field's understanding of student success in general chemistry by assessing the predictive power of a novel affective variable, students’ sense of belonging in the course.
Chemical education research has identified a range of cognitive, educational, and affective predictors of success in undergraduate general chemistry. In terms of cognitive factors, it is well-established that mathematical ability (e.g., Tai et al., 2005; Lewis and Lewis, 2007; Xu et al., 2013), prior conceptual knowledge (e.g., Seery, 2009; Xu et al., 2013; Cracolice and Busby, 2015; Frey et al., 2018), and scientific reasoning ability (e.g., Cracolice and Busby, 2015) influence student performance in the course. Other individual differences like the tendency to learn through memorization versus abstraction of underlying concepts (Frey et al., 2017) have also proven influential. After accounting for these cognitive variables, independent effects of students’ high-school pedagogical experiences and course-taking on their general chemistry achievement are also observed (Tai et al., 2005). Once enrolled in general chemistry, participation in collaborative learning programs like Peer Led Team Learning (PLTL) has robust effects on students’ success in the course (Wilson and Varma-Nelson, 2016). In the affective or motivational domain, students’ attitudes towards chemistry (Xu et al., 2013; Villafañe and Lewis, 2016) and their chemistry self-concepts (Lewis et al., 2009) have been shown to predict performance, above and beyond conventional cognitive measures. The potential impact of other affective predictors, such as chemistry self-efficacy (Ferrell and Barbera, 2015) and chemistry identity (Hosbein and Barbera, 2020), remains an area of active research.
The current study contributes to that work by investigating the effects of two facets of course-level belonging on students’ general chemistry outcomes, including their exam performance and continuation in the course sequence. Specifically, this study examines whether belonging influences chemistry outcomes after accounting for high-school academic preparation, demographics, and PLTL participation, and it explores whether belonging is especially influential for groups underrepresented in STEM. In addition, this investigation focuses specifically on the belonging of first-year general chemistry students, who are in the midst of both major personal and academic transitions.
Belonging
Belonging refers to perceptions of connectedness, social inclusion, and having meaningful relationships with others in the target context, and it is considered a fundamental human need (Baumeister and Leary, 1995; Gere and MacDonald, 2010) that influences achievement (Cohen and Garcia, 2008). Institution-level belonging has long been linked to motivation and academic outcomes among adolescents (Goodenow, 1993; Gillen-O'Neel and Fuligni, 2013) and college students (O'Keeffe, 2013; Yorke, 2016; Slaten et al., 2018), particularly among first-year students navigating the difficult transition into college (Hoffman et al., 2002; Freeman et al., 2007; Zumbrunn et al., 2014) and students from underrepresented groups (Hurtado and Carter, 1997; Hausmann et al., 2007; Walton and Cohen, 2007, 2011; Rahman, 2013; Murphy and Zirkel, 2015). Recently, a multi-institutional study demonstrated independent effects of different levels of belonging – course, major, and university – on undergraduate STEM students’ behavioral and emotional engagement, with course-level belonging proving most influential (Wilson et al., 2015). Taken together, these findings align with an explanatory model where supportive classrooms foster course-level belonging, which is an antecedent of motivation, which in turn fosters greater engagement in learning activities and ultimately greater achievement (Zumbrunn et al., 2014). The current study extends that model, examining the independent effects of two course-level belonging measures, perceived belonging and belonging uncertainty.
Two types of research reinforce such a model of belonging in academic settings, providing causal evidence that belonging has downstream consequences for motivation and academic achievement. First, lab-based experimental psychology studies have demonstrated that subtle manipulations of students’ perceived belonging or social connectedness affect their outcomes in the target domain (Shteynberg and Galinsky, 2011; Walton et al., 2012; Master and Walton, 2013; Carr and Walton, 2014). Second, a number of applied studies have translated this research into practice using educational interventions designed to bolster student belonging. For example, when students are randomly assigned to read and reflect on evidence that uncertainty about belonging in college is (a) common among diverse students and (b) a temporary experience that will improve over time, they tend to perform better and persist at higher rates than peers who read and reflect on other topics (Walton and Cohen, 2007, 2011; Walton et al., 2015; Yeager et al., 2016b; see also Shnabel et al., 2013). Thus, monitoring and supporting student belonging holds promise as a strategy for attracting and retaining students in STEM pathways.
Belonging and identity in undergraduate STEM education
In recent years, belonging has received increased attention, alongside other affective predictors, for its potential influence on STEM undergraduates’ success (see Trujillo and Tanner, 2014 for a review). Some of this work has examined belonging and shown its overall impact on STEM outcomes regardless of student identity (Marra et al., 2012; Veilleux et al., 2013; Wilson et al., 2015). However, the majority of studies have investigated the belonging of groups underrepresented in STEM (Cheryan et al., 2009; Cheryan et al., 2011; Johnson, 2012; Rosenthal et al., 2013; Smith et al., 2013; Liptow et al., 2016; Blaney and Stout, 2017; Rattan et al., 2018) and the role of belonging in STEM achievement and retention gaps (Good et al., 2012; Stout et al., 2013; Rainey et al., 2018).
In particular, most studies have focused on STEM fields with stark gender gaps in participation, including computer science, mathematics, and physics (National Science Foundation, 2019). In other words, such studies have predominantly explored the importance of belonging in STEM disciplines and course contexts where clear representational inequities might undermine students’ feelings of belonging. The current study extends that research by examining the role of belonging in chemistry, a STEM field where greater parity has been reached, at least at the undergraduate level. Chemistry is currently the most equitable of the physical sciences in terms of bachelor's degree attainment in the U.S. Over 45% of degrees were awarded to women in 2016 (National Science Foundation, 2019), though greater inequities emerge in terms of advanced degrees and employment. While chemistry does not compare to the biological sciences, where women received a majority (61%) of all bachelor's degrees in 2016, it represents a borderline case of gender equity in undergraduate STEM education. This study therefore explores the boundary conditions of belonging effects in STEM, assessing whether belonging effects are also observed in the moderately gender-balanced discipline of chemistry.
A growing body of research has characterized the subjective experiences of women in undergraduate STEM and linked those experiences with the well-documented gender gaps in STEM participation noted above (see Lewis et al., 2016, for a physics-oriented review). For example, laboratory studies have demonstrated that classroom environments conveying masculine norms tend to reduce women's sense of belonging and their interest in pursuing computer science (Cheryan et al., 2009; Cheryan et al., 2011). Applied studies have also employed surveys and daily diaries to understand women's belonging in actual STEM courses. London and colleagues (2011) found that two psychosocial factors, (i) perceived compatibility between gender and STEM majors and (ii) perceived social support, affect both women's belonging and their persistence in a STEM major. Similarly, Rosenthal et al. (2013) showed that perceived compatibility between gender and a medical career correlates with women's interest in pre-medicine, and belonging in pre-medicine mediates that relationship.
In addition, at least two studies have linked gender differences in belonging to gaps in undergraduate STEM course performance. Good et al. (2012; study 3) conducted a longitudinal study of college calculus students, and they observed that women's (but not men's) belonging in math was undermined by environmental messages that math abilities are innate and women have lower math abilities. Stout et al. (2013) found similar results among introductory physics students: women reported lower belonging than men, especially if the women endorsed the gender stereotype that men have better physics abilities. Crucially, both studies showed that women's decreased belonging negatively impacted their course grades, because belonging predicted performance for all students.
Finally, other research has taken an intersectional approach, examining the relationship between belonging, gender, and other facets of identity. For instance, Blaney and Stout (2017) observed that, in introductory computer science, first-generation women reported lower computing self-efficacy and belonging than all other identity groups, including continuing-generation women and men regardless of generation status. In an interview-based study, Rainey and colleagues (2018) investigated the intersection of gender and race, demonstrating that white men were most likely to report feelings of belonging, while women of color were least likely. Overall, persistence in STEM majors was associated with higher belonging, suggesting that low belonging among underrepresented groups prevents broader STEM participation.
This growing literature indicates that sense of belonging can be an influential factor in undergraduates’ success in STEM, particularly for underrepresented groups. In many physical sciences, gendered environments and gender stereotypes about STEM aptitude can prompt women to doubt their belonging, with negative consequences for their course performance and persistence. However, it remains unclear how these findings apply to STEM classrooms and disciplines that are more gender balanced. By extending prior research to the field of chemistry, this study may enhance our understanding of belonging in STEM and its potential as a lever for increasing and diversifying participation in different fields.
Research objectives
This study complements chemical education research that has established the role of student affect in determining general chemistry achievement and retention (e.g., Lewis et al., 2009; Xu et al., 2013; Ferrell and Barbera, 2015; Villafañe and Lewis, 2016), focusing on course-level belonging as a predictor of student success. Taking a novel approach, this study adapts previous survey instruments to measure two different facets of student belonging in general chemistry: perceived belonging and belonging uncertainty. Perceived belonging reflects students’ overall evaluations of their fit and social relationships in general chemistry, indicating whether they generally agree or disagree that they belong. Belonging uncertainty, on the other hand, indicates the relative stability of students’ self-evaluations or their confidence about belonging in the course. The term belonging uncertainty was introduced by Walton and Cohen (2007), who argued that awareness of educational inequities and stereotypes, experiences with discrimination, and other threats to inclusion can cause students from underrepresented groups to question or doubt the quality of their social connections in educational settings (Mallett et al., 2011). To our knowledge, this is the first study to simultaneously examine the effects of belonging and belonging uncertainty on academic outcomes, as well as the first study to investigate the impact of course-level belonging in chemistry.
This investigation focuses specifically on the belonging of first-year general chemistry students, who are experiencing a critical period of personal and academic development. The transition to college is challenging for most students (Tinto, 1993), but the challenge may be compounded for students from under-resourced or underrepresented groups who may feel alienated by the cultural norms of the institution (e.g., Stephens et al., 2012) and experience low or uncertain belonging (Hurtado and Carter, 1997; Walton and Cohen, 2007). Moreover, these belonging concerns may prove especially acute in large-enrollment, lecture-based, introductory STEM courses like general chemistry, where rigorous coursework is combined with an unfamiliar learning environment and often limited opportunities for individual participation during class. Understanding how early belonging varies and impacts first-year students’ success in general chemistry may point chemical educators towards new strategies for supporting their students and retaining more talent in STEM and healthcare fields.
This research explores multiple lines of inquiry, treating general chemistry belonging as an early outcome and also as a potential predictor of success across the course sequence. The first analysis considers the possibility that students with different characteristics may enter general chemistry with different perceptions of their belonging in the course or develop different perceptions based on their first couple weeks in this introductory STEM course environment. The second analysis assesses whether early-semester measures of belonging predict performance in general chemistry, above and beyond conventional predictors. A third analysis examines whether belonging throughout the first semester of the course, General Chemistry 1, predicts student attrition from the course sequence. More precisely, this study addresses the following research questions:
1. Does early-year belonging or belonging uncertainty, measured using a pre-survey in General Chemistry 1, differ according to demographics (i.e., gender and race) and academic preparation (i.e., math abilities, content knowledge, and college-preparatory experience)?
2. Does early-semester belonging or belonging uncertainty, measured using pre-surveys in General Chemistry 1 and 2, predict students’ subsequent exam averages in each respective course?
3. Does belonging or belonging uncertainty throughout General Chemistry 1, measured at both pre- and post-survey, uniquely predict which students choose not to enroll in the second-semester course (i.e., General Chemistry 2), after accounting for demographics and academic preparation?
Methods
Study Setting
University.
The study took place at a selective, private research university in the mid-western United States during the fall 2017 through spring 2019 semesters. As of Fall 2018, institutional data indicate that the undergraduate student body is 20% Asian American, 21% underrepresented minority (i.e., Black or African American, Hispanic or Latino, American Indian, Alaska Native, Native Hawaiian, or Pacific Islander), 51% White only, 11% White multiracial, and 2% unknown (the sum exceeds 100% because students could report multiple racial or ethnic categories). The population of over 7000 full-time undergraduates is fairly balanced in terms of sex,† including 47% male and 53% female students.
General chemistry.
Each fall, approximately 700 to 800 of those students enroll in General Chemistry 1, which is the first course in a two-part introductory chemistry sequence. The course involves three one-hour lectures and a mandatory one-hour recitation each week, plus concurrent enrollment in an associated but separate laboratory course. There are ungraded clicker questions, weekly quizzes, weekly graded and ungraded homework sets, three unit exams (the lowest is dropped), and a cumulative final. General Chemistry 1 students also have the opportunity to participate in various supplemental learning opportunities. They can attend informal instructor-led help sessions, which are offered daily and provide students the chance to work with any member of the instructor team. All students are also encouraged to join a more structured activity: the department-sponsored PLTL program (Hockings et al., 2008; Frey et al., 2018). Nearly 70% of General Chemistry 1 students complete PLTL, where they spend two hours a week collaboratively solving practice problems in a group of 8–10 peers.
In the spring, between 550 to 650 General Chemistry 1 students continue on to General Chemistry 2. Those who do not continue may have lost interest or struggled in the course, or they may be required to complete only one semester of introductory chemistry for their major (i.e., electrical, mechanical, and systems engineering majors who are not prehealth). A breakdown of how many students in the current study left due to poor performance or lack of major requirements is provided in the Results section. The second semester follows the same structure as the first, including three lectures per week, mandatory recitation, and a simultaneous but separate laboratory course. Once again, students have the option to participate in help sessions and PLTL, though PLTL completion rates are lower in the spring semester (approximately 50%).
During both semesters of the course sequence, General Chemistry is divided into two or three lecture sections, but these sections share the same procedures and are treated as a single unit. For example, all instructors implement the same homework sets, quizzes, and exams. Moreover, students from all sections are intermingled during recitations, and their work is combined during a common grading process. The graduate students who lead recitation sections complete Department-led pedagogical training prior to the course, and they attend weekly meetings with their fellow graduate assistants and the General Chemistry lecturers to discuss the week's recitation problems and their facilitation.
Each semester, several faculty members collaboratively teach General Chemistry, with some teaching the lectures and others teaching recitations and overseeing the graduate assistants and supplemental course programs. During this study, the General Chemistry 1 team comprised five instructors, including three or four women depending on the semester. At least one (of three) General Chemistry 1 lecture sections was led by a woman each term. The General Chemistry 2 team comprised four instructors, including two women, but all (2) lecture sections were led by a man each term.
Social psychological interventions.
The Department of Chemistry at this institution is committed to supporting students as they transition into college. As a result, they collaborated with the research team to pilot and evaluate course-based, social-psychological interventions within the General Chemistry course sequence. Such interventions have gained prominence in educational settings because of their potential to bolster students from underrepresented or under-resourced groups as they navigate challenging academic transitions (Yeager and Walton, 2011; Yeager and Dweck, 2012; Jury et al., 2017). Two interventions may have influenced the findings in this study: a growth-mindset intervention available to all General Chemistry 1 students, and a belonging intervention piloted during spring 2018.
All General Chemistry 1 students are assigned a three-part, growth-mindset intervention intended to boost student motivation and promote effective learning strategies, and data from fall 2018 indicate that 90% of all students complete at least part of the intervention. The intervention was originally administered as part of a random-assignment classroom experiment (Fink et al., 2018), but it is now incorporated into the curriculum as part of the graded homework. Following previous research (Aronson et al., 2002; Good et al., 2003; Blackwell et al., 2007; Paunesku et al., 2015; Yeager et al., 2016a; Yeager et al., 2016b), the intervention involves short reading and writing activities designed to subtly foster a growth mindset about intelligence, or a belief that intellectual abilities can be increased through effort, effective study strategies, and help from others. The experimental results revealed a selective benefit of the growth-mindset intervention among underrepresented minority students, whose General Chemistry 1 cumulative final exam scores were approximately 5 points higher in the mindset versus control condition, even after accounting for preparation (Fink et al., 2018). Consistent with previous research, the mindset intervention had a null effect among White participants, who exhibited no disparities in performance in the control condition (e.g., based on gender).
In addition, students enrolled in General Chemistry 2 in spring 2018 were randomized into a belonging condition or control condition as part of a different classroom experiment. This intervention followed the format of the mindset intervention; it included three reading and writing assignments administered via the graded homework, earning completion credit. Following previous work (Walton and Cohen, 2007, 2011; Yeager et al., 2016b) and an intervention guide from The College Transition Collaborative (Walton et al., 2017), the belonging condition asked participants to read and reflect on testimonials from former General Chemistry students, which conveyed that uncertainty about belonging in college-level chemistry is common among all students and will dissipate over time. In contrast, the control condition asked participants to read and reflect on student testimonials that described how all students lack academic extracurriculars early in college but increase their engagement over time. The reflection prompts asked students in both conditions to explain in writing how the themes from their assigned readings relate to their own experiences.
Over 90% of consenting first-year students in spring 2018 participated in the intervention. However, analyses indicated no effect of condition on students’ belonging or their course performance (see Appendix 1, Tables 6–8). The experimental groups were therefore combined and retained in this study, in order to maximize power and examine two full years of General Chemistry outcomes. To ensure that inclusion of the spring 2018 semester did not produce spurious results, relevant regression analyses were re-run without those data. Appendix 1 reports on those follow-up analyses, and the Discussion will consider the potential influence of the social-psychological interventions on the results of this study.
Participants
All students enrolled in General Chemistry 1 in Fall 2017 and 2018 (N = 1479) were invited to participate in this study, which was approved by the university's Institutional Review Board, and receive extra credit in their laboratory course as compensation. Approximately 89% (N = 1316) of enrolled students provided informed consent, and the sample was later narrowed to first-year students only (N = 1041) for three reasons. First, a key objective of the study was to examine belonging and its impact during the transition into college-level STEM. Second, restricting the sample to first-year students simplified the attrition analysis, because it largely eliminated students required to take only one semester of General Chemistry for their engineering majors. For example, Electrical Engineering majors only need General Chemistry 1 and customarily enroll in their second or third year, whereas Biomedical Engineering majors need both semesters of General Chemistry and enroll during their first year. Finally, only first-year students are required to complete an online assessment of their incoming content knowledge, which is a key academic preparation variable in the analyses below. Participants missing any of the survey or background data were excluded, leaving a final sample of 739 first-year students.
Variables
Demographics.
Student characteristics were obtained from the registrar's office, which uses The Common Application to collect this information during admissions. Sex was reported as a binary variable (female, male); intersex was not a response option. While students had the option to share more about their gender identities on the Application (e.g., identification as gender non-conforming, genderqueer, or transgender), the research team did not have access to that information. Race and ethnicity were combined to create a three-category race variable (Asian, underrepresented minority (URM), White).
Preparation.
Math scores from the ACT, a standardized admissions test in the United States (ACT, 2018), provided an index of students’ mathematical abilities. Such scores have been shown to correlate with general chemistry performance (Tai et al., 2005; Xu et al., 2013; Frey et al., 2018). When students provided scores from the SAT, another standardized admissions test, they were converted to ACT equivalents using concordance tables (Dorans, 1999). The Chemistry Department's Online Diagnostic (OD) exam, which is administered to all first-year students who enroll in General Chemistry 1, assessed students’ incoming chemistry content knowledge (Shields et al., 2012; Frey et al., 2018). Performance on STEM-related Advanced Placement (AP) exams (College Board, 2019) was also used as an index of students’ experience with college-level coursework. AP exams evaluate students’ discipline-specific academic skills after year-long, college-level courses taken during high school. A composite “AP proportion” measure was created based on scores from four AP exams: Biology, Calculus, Chemistry, and Physics. Specifically, AP proportion represents the proportion of STEM AP exams where students earned a score of 4 or 5 (out of 5). AP proportion scores therefore range from 0 to 1, with a 0.25 increase for each exemplary AP score. Previous work has demonstrated a significant correlation between this measure and General Chemistry performance (Fink et al., 2018; Frey et al., 2018).
Belonging.
A six-item survey measured students’ belonging. The survey items were adapted from several psychology studies (Walton and Cohen, 2007, 2011; London et al., 2011), and each question was assessed on a six-point agreement scale (1 = strongly disagree, 2 = disagree, 3 = mildly disagree, 4 = mildly agree, 5 = agree, 6 = strongly agree). Factor analysis confirmed that the survey comprises two separate scales gauging different aspects of sense of belonging (see Appendix 2, Table 9). The belonging scale contains four items examining students’ social relationships and their overall feelings of fit in the target course: “I feel like I fit in the General Chemistry course,” “I feel comfortable with my peers and classmates in the General Chemistry course,” “I feel comfortable with my instructors in the General Chemistry course,” and “Setting aside my performance in class, I feel like I belong in the General Chemistry course.” The uncertainty scale includes two items probing the relative stability and performance contingency of students’ perceived belonging: “I feel uncertain about my belonging in the General Chemistry course (i.e., sometimes I feel that I belong and sometimes I don’t)” and “When I don’t perform well, I feel like maybe I don’t belong in the General Chemistry course.” Responses on each scale were averaged to create composite scores.
PLTL participation.
PLTL participation was coded as a binary variable indicating whether students met the program requirements for PLTL completion (no, yes). Once students enroll in PLTL, attendance becomes mandatory, and students are allowed no more than two excused absences. PLTL participants therefore include students who enrolled in a PLTL session and missed no more than two (out of eleven) sessions. The no-PLTL group includes students who did not enroll in the first place, who decided to drop from the PLTL program, and who were dismissed because of too many absences.
Exam average.
Exam average was calculated following the course instructors’ procedure. Students’ highest two (out of three) unit exam scores were combined with their scores on the cumulative final to determine their exam average (percent correct). In other words, the lowest unit exam was dropped, before the remaining scores were averaged together with the final.
Attrition.
Attrition from the General Chemistry sequence was coded as a binary variable reflecting student decisions to leave the course sequence between the first and second semester. General Chemistry 1 students who completed General Chemistry 2 in the immediately following semester were marked “0” for persisting, while those who did not complete the second semester were marked “1” for attrition from the course sequence. If a student took the second semester at a delay, e.g., taking General Chemistry 1 during Fall 2017 and General Chemistry 2 during Spring 2019, they were included in the attrition group (note: less than 1% of students in the Fall 2017 completed General Chemistry at a delay). Thus, the coding scheme identifies which first-year students began but did not complete the typical General Chemistry trajectory expected for most STEM majors and those in the pre-health pathway.
Procedure
The belonging survey was administered in recitation by the General Chemistry 1 and 2 graduate assistants twice per semester (up to four times total per student). The early-semester survey occurred during the first recitation session (week two of fifteen), providing an initial assessment of belonging perceptions after several lectures. The late-semester survey occurred during the last recitation session with a quiz (week twelve), when students have extensive experience with the course but have not yet taken the third unit exam or cumulative final. Each survey measure is named according to its timing, semester, and scale. For example, the General Chemistry 1 measures include early-semester GC1 belonging, early-semester GC1 uncertainty, late-semester GC1 belonging, and late-semester GC1 uncertainty. Students were assured that the course instructors would not see their individual surveys, which were collected by the General Chemistry Administrative Assistant and delivered to the research team. At the end of each term, exam grades and PLTL data were obtained from the instructors and student background information was requested from the registrar's office.
Analysis
All analyses were run with the open-source software R (R Core Team, 2019). Besides base functions, the analyses also utilized functions from the lsr (Navarro, 2015), lm.beta (Behrendt, 2014), and emmeans packages (Lenth, 2019). All analyses included the categorical demographic variables (i.e., sex and race), PLTL participation, and a two-way interaction between sex and race. The categorical variables were treatment-coded with the following reference groups: female students, Asian students, and students who did not complete PLTL. The continuous academic preparation variables (i.e., ACT math, AP proportion, and OD) were always centered and included as covariates, both out of theoretical interest and because they reduced the error variance.
Survey analysis.
ANCOVAs were used to analyze the early-year survey measures, because they allow for straightforward interpretation of both main effects and interactions between categorical variables. Early-semester GC1 belonging served as the dependent variable in the first ANCOVA, with early-semester GC1 uncertainty as the dependent variable in the second ANCOVA.
Performance analysis.
Multiple regression was used for the performance analysis, with the regression coefficients providing an index of the practical impact of each performance predictor. The first model predicted General Chemistry 1 exam averages, followed by a second model predicting General Chemistry 2 exam averages. Besides the variables above, these analyses included early-semester belonging and belonging uncertainty (centered) in the target course as predictors, plus an interaction between PLTL and AP proportion (Frey et al., 2018). To accommodate exam variation across academic years, both models were also run with z-scored exams; however, the pattern of results did not change. Raw results are therefore reported below, so the coefficients can be directly interpreted in terms of exam outcomes.
Interactions between the belonging measures and demographic variables were also tested by individually adding them to the baseline performance models. Nested model comparisons were used to determine whether the interactions significantly improved the explanatory power and fit of the models. These supplemental analyses, which showed no substantive changes to the effects observed in the original models, are described in Appendix 3.
Attrition analysis.
Logistic regression was used for the attrition analysis due to the dichotomous dependent variable. This analysis included four belonging measures (centered) as predictors: both early- and late-semester GC1 belonging and GC1 uncertainty. Parallel to the performance analysis, the attrition model also included an interaction between AP proportion and PLTL, and interactions between the belonging measures and demographic variables were later evaluated through nested model comparisons with the baseline model (see Appendix 4). In addition, General Chemistry 1 exam average was included as a predictor in the attrition model.
Assessment of multi-collinearity.
Multi-collinearity occurs when regression predictors are highly correlated, making it difficult to attribute variance in the data to individual variables (Graham, 2003). While multi-collinearity does not necessarily invalidate an analysis, it makes it less sensitive, especially for small effects. Three strategies were therefore used to assess the presence of multi-collinearity in the current regression analyses: bivariate correlations, Variance Inflation Factors (VIFs), and model reduction. These procedures suggested a stable pattern of results that was not subject to a high degree of multi-collinearity (see Appendix 5). Therefore, full regression models including all the predictors described above were retained.
Significance and effect size.
Significance was evaluated at α = 0.05. Tukey HSD adjustments were applied to p-values from post hoc tests to correct for multiple comparisons. Although all models incorporated the academic preparation covariates, unadjusted means (M) and standard errors (SE) are reported below. Partial eta-squared (ηp2) provided effect-size estimates for the ANCOVAs, with 0.01, 0.06, and 0.14 indicating small, medium, and large effect-sizes, respectively (Richardson, 2011). For the linear regression analyses, unstandardized regression coefficients (b) provided absolute effect-size estimates, and standardized regression coefficients (β) provided relative effect-size estimates. Logistic regression also generates unstandardized regression coefficients, but they are more challenging to interpret because they reflect the impact of each predictor on the log odds of the target outcome. To facilitate interpretation, the coefficients were converted into odds ratios (i.e., the odds of attrition divided by the odds of retention) through exponentiation. In addition, key effects are described in terms of their impact on the predicted probability of attrition, which was estimated for a modeled student who had average academic preparation scores and belongs to the reference group of Asian, female, PLTL non-completers.
Results
Demographic effects on early-year belonging surveys
Belonging.
As shown in Table 1, OD and AP proportion both had small, positive effects on early-semester GC1 belonging, while the effect of ACT math was not significant. With academic preparation accounted for, a small main effect of sex emerged: early in the semester, male students (M = 5.00, SE = 0.03) reported higher belonging in General Chemistry 1 than female students (M = 4.80, SE = 0.03). There was also a significant effect of race. Raw means indicate minimal differences in the early-semester GC1 belonging of Asian (M = 4.88, SE = 0.03), underrepresented (M = 4.84, SE = 0.06), and White (M = 4.90, SE = 0.04) students. However, pairwise comparisons indicate higher belonging among underrepresented students compared to Asian students, after accounting for differences in preparation, t(730) = −2.44, p = 0.04 (other comparisons n.s.). This result therefore reflects a confound between the race and academic preparation variables: underrepresented students entered the course with significantly lower ACT, AP, and OD scores than Asian and White students (p's < 0.05). Because the ANCOVA examined students’ belonging scores after normalizing for differences in preparation (i.e., raising underrepresented students’ scores and lowering Asian and White students’ scores to the same level), underrepresented students’ belonging scores appear relatively high, all else being equal.
Table 1 ANCOVA testing for variation in early-semester GC1 belonging
Variable |
SS |
df |
MS |
F
|
η
p
2
|
*p < 0.05. N = 739. OD refers to the Online Diagnostic of incoming content knowledge. AP proportion reflects how many STEM AP exams (Biology, Calculus, Chemistry, Physics) students earned a 4 or 5 on. |
ACT math |
0.16 |
1 |
0.16 |
0.45 |
<0.01 |
AP proportion |
2.18 |
1 |
2.18 |
5.99* |
0.01 |
OD |
4.11 |
1 |
4.11 |
11.31* |
0.02 |
Sex |
5.71 |
1 |
5.71 |
15.68* |
0.02 |
Race |
2.38 |
2 |
1.19 |
3.27* |
0.01 |
Sex × race |
2.52 |
2 |
1.26 |
3.47* |
0.01 |
Residuals |
265.54 |
730 |
0.36 |
|
|
Crucially, there was a significant interaction between sex and race. As illustrated by Fig. 1, underrepresented males (M = 5.11, SE = 0.07) reported significantly higher early-semester GC1 belonging than underrepresented females (M = 4.70, SE = 0.08), t(730) = −3.39, p = 0.01. The sex effect proved marginally significant among White students, t(730) = −2.80, p = 0.06 (male: M = 5.04, SE = 0.06; female: M = 4.81, SE = 0.04), and non-significant among Asian students, p > 0.99 (male: M = 4.92, SE = 0.05; female: M = 4.84, SE = 0.04).
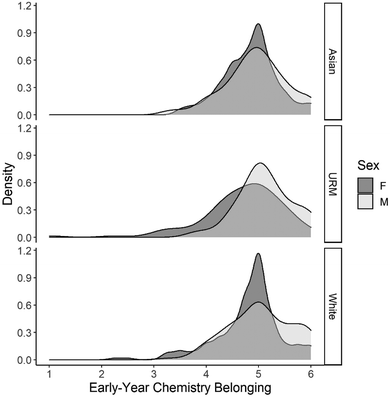 |
| Fig. 1 Density estimates of early-semester GC1 belonging scores within each racial group and sex. Note: density estimates were calculated with a Gaussian kernel and a bandwidth equaling the standard deviation of the kernel. | |
Belonging uncertainty.
Similar to the belonging results, OD had a small, significant effect and AP proportion had a small, marginal effect on early-semester GC1 belonging uncertainty, while ACT math did not (Table 2). In terms of demographics, the results showed a significant main effect of sex on uncertainty. Female students (M = 3.46, SE = 0.05) reported more uncertainty than male students (M = 2.84, SE = 0.07), and this tendency for female students to report more uncertainty was consistent across each racial group (sex × race n.s.; Fig. 2). Race also had a marginal effect on early-semester GC1 belonging uncertainty. While the raw means once again indicate minimal differences (Asian: M = 3.10, SE = 0.07; URM: M = 3.23, SE = 0.10; White: M = 3.28, SE= 0.07), pairwise comparisons show that after adjusting for preparation, underrepresented students reported marginally less uncertainty than White students, t(730) = −2.15, p = 0.08 (other comparisons n.s.). As above, this result stems from significant differences in the academic preparation scores of underrepresented students compared to their Asian and White peers. Because the ANOVA evaluated students’ uncertainty after normalizing for differences in preparation, underrepresented students appear to have relatively low uncertainty on balance.
Table 2 ANCOVA testing for variation in early-semester GC1 belonging uncertainty
Variable |
SS |
df |
MS |
F
|
η
p
2
|
*p < 0.05. † 0.05 < p < 0.10. N = 739. OD refers to the Online Diagnostic of incoming content knowledge. AP proportion reflects how many STEM AP exams (Biology, Calculus, Chemistry, Physics) students earned a 4 or 5 on. |
ACT math |
0.08 |
1 |
0.08 |
0.07 |
<0.01 |
AP proportion |
4.28 |
1 |
4.28 |
3.59† |
0.01 |
OD |
22.49 |
1 |
22.49 |
18.88* |
0.03 |
Sex |
45.07 |
1 |
45.07 |
37.84* |
0.05 |
Race |
5.89 |
2 |
2.95 |
2.47† |
0.01 |
Sex × race |
3.29 |
2 |
1.65 |
1.38 |
<0.01 |
Residuals |
864.7 |
729 |
1.19 |
|
|
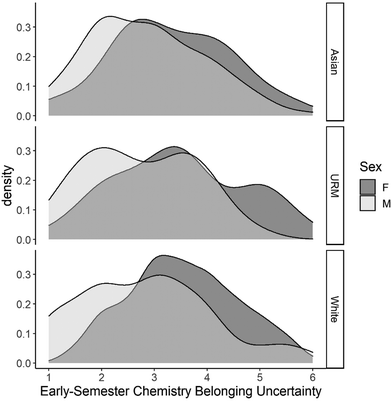 |
| Fig. 2 Density estimates of early-semester GC1 belonging uncertainty within each racial group and sex. Note: density estimates were calculated with a Gaussian kernel and a bandwidth equaling the standard deviation of the kernel. | |
Early-semester belonging predicts general chemistry 1 exam performance
As expected from previous research (Tai et al., 2005; Xu et al., 2013; Frey et al., 2018), all three preparation variables were significant, positive predictors of General Chemistry 1 performance (Table 3). After accounting for preparation, PLTL completion also significantly predicted course performance: PLTL completers (M = 69.8, SE = 0.57) scored over five points higher on exams than PLTL non-completers (M = 64.3, SE = 1.07). Replicating a recent study (Frey et al., 2018), this PLTL effect interacted with AP proportion, reflecting how the PLTL benefit increased in magnitude as students’ AP preparation decreased.
Table 3 Multiple regression testing for predictors of general chemistry 1 exam performance
Predictor |
b
|
β
|
t
|
*p < 0.05. Note: General Chemistry 1 exam average is the dependent variable. OD refers to the Online Diagnostic of incoming content knowledge. AP proportion reflects how many STEM AP exams (Biology, Calculus, Chemistry, Physics) students earned a 4 or 5 on. Continuous variables were centered around their sample means. Categorical variables were treatment coded with PLTL non-completers, female, and Asian students as reference levels. R2 = 0.45. N = 739. |
(Intercept) |
15.54 |
0 |
2.34* |
ACT math |
1.25 |
0.23 |
6.25* |
AP proportion |
14.89 |
0.33 |
5.84* |
OD |
0.18 |
0.28 |
8.10* |
Early-semester GC1 belonging |
1.77 |
0.08 |
2.41* |
Early-semester GC1 uncertainty |
−0.33 |
−0.03 |
−0.80 |
PLTL (yes) |
5.42 |
0.17 |
6.12* |
Sex (M) |
−1.52 |
−0.05 |
−1.18 |
Race (URM) |
−4.10 |
−0.12 |
−2.64* |
Race (White) |
−0.57 |
−0.02 |
−0.49 |
Sex (M) × race (URM) |
1.36 |
0.02 |
0.60 |
Sex (M) × race (White) |
0.11 |
0.003 |
0.06 |
AP proportion × PLTL |
−8.66 |
−0.16 |
−3.06* |
The only significant demographic predictor of General Chemistry 1 performance was race. Underrepresented minority students (M = 58.4, SE = 1.24) received lower exam averages than their Asian peers (M = 72.7, SE = 0.73); the regression coefficient indicates a four-point difference after adjusting for preparation. Pairwise comparisons showed that underrepresented students also scored marginally lower than White students (M = 68.9, SE = 0.71), t(725) = −2.31, p = 0.05, with no significant difference between Asian and White students (p = 0.81). The effect of sex and the sex by race interactions were not significant.
Crucially, after accounting for preparation, PLTL participation, and demographics, early-semester GC1 belonging was a significant predictor of General Chemistry 1 performance. The higher students’ belonging at the start of the semester, the better their subsequent exam scores. Specifically, a one-point increase in early-semester GC1 belonging corresponded to an increase of 1.77 points on exams. In contrast, early-semester GC1 belonging uncertainty was not a significant predictor, i.e., it did not account for any additional variance in students’ General Chemistry 1 performance above and beyond the other variables. To examine whether the belonging effect varied across demographic groups, two-way interactions between the belonging measures and demographic variables were also considered, but none of the interactions significantly improved the baseline model's fit (see Appendix 3).
Early-semester belonging and uncertainty predict general chemistry 2 exam performance
Among participants who continued to the second semester of General Chemistry, we observed similar performance results (Table 4). All three preparation variables (OD, AP proportion, ACT math) were significant predictors of General Chemistry 2 exam average, with more prepared students earning higher scores. PLTL participation also predicted performance, with PLTL completers (M = 79.3, SE = 0.76) scoring higher than non-completers (M = 70.5, SE = 0.95). The effect of PLTL interacted with AP proportion, indicating a larger PLTL benefit among first-year students with less AP experience.
Table 4 Multiple regression testing for predictors of general chemistry 2 exam performance
Predictor |
b
|
β
|
t
|
*p < 0.05. When the dependent variable is z-scored, effect is only marginally significant, p = 0.08. Note: General Chemistry 2 exam average is the dependent variable. OD refers to the Online Diagnostic of incoming content knowledge. AP proportion reflects how many STEM AP exams (Biology, Calculus, Chemistry, Physics) students earned a 4 or 5 on. Continuous variables were centered around their sample means. Categorical variables were treatment coded with PLTL non-completers, female, and Asian students as reference levels. R2 = 0.52. n = 592. |
(Intercept) |
24.37 |
0 |
7.47 |
ACT math |
1.17 |
0.19 |
5.19* |
AP proportion |
13.22 |
0.27 |
5.94* |
OD |
0.17 |
0.24 |
6.67* |
Early-semester GC2 belonging |
1.71 |
0.07 |
2.09* |
Early-semester GC2 uncertainty |
−1.79 |
−0.13 |
−3.74* |
PLTL (yes) |
7.11 |
0.23 |
7.86* |
Sex (M) |
1.04 |
0.03 |
0.69 |
Race (URM) |
−3.61 |
−0.10 |
−2.03*a |
Race (White) |
0.70 |
0.02 |
0.53 |
Sex (M) × race (URM) |
−1.53 |
−0.03 |
−0.61 |
Sex (M) × race (White) |
−3.18 |
−0.07 |
−1.54 |
AP proportion × PLTL |
−6.49 |
−0.09 |
−2.24* |
As above, the only significant demographic predictor was race.‡ Underrepresented minority students (M = 63.7, SE= 1.57) scored lower than Asian students (M = 80.0, SE = 0.93) on the General Chemistry 2 exams, with the regression coefficient showing a 3.6-point difference after adjusting for other variables. Pairwise comparisons showed that underrepresented minority students also received lower scores than White students (M = 75.7, SE = 0.81), t(578) = −2.44, p = 0.04, while the difference between Asian and White students was not significant (p = 0.77). Sex did not significantly influence students’ exam averages, nor did sex by race interactions.
In contrast to the first-semester results, both belonging measures uniquely predicted students’ exam averages in General Chemistry 2, after accounting for preparation, demographics, and PLTL participation. Early-semester GC2 belonging had a positive effect on performance, with a one-point increase in belonging predicting an increase of 1.71 points on exams. Early-semester GC2 uncertainty had the expected, complementary effect, with a one-point increase in uncertainty predicting a decrease of 1.79 points on exams. The standardized regression coefficients indicate that the belonging predictors were less influential than the preparation predictors; nonetheless, they had an independent and practical impact on student outcomes in the course. Once again, interactions between the belonging measures and demographic variables were also tested, showing no significant impact on the baseline model fit (see Appendix 3).
Late-semester belonging in general chemistry 1 predicts attrition from general chemistry sequence
Among the first-year students in our study, there was 11.8% (n = 87 out of 739) attrition from first to second semester of General Chemistry. Course grades indicate that students who left the course sequence struggled at higher rates than students who continued on to General Chemistry 2. Specifically, 40.2% of those who left received a C or lower in General Chemistry 1 (n = 35 out of 87), compared to just 13.5% of those who persisted (n = 88 out of 652). Very few students appear to have left due to their majors: only 9.2% (n = 8 out of 87) of those who left the sequence reported intentions to major in non-prehealth, engineering fields that do not require General Chemistry 2.
As one might expect, AP proportion had a negative effect on attrition: that is, students with more college-preparatory experience (i.e., higher AP proportion scores) were less likely to leave between General Chemistry 1 and 2 (Table 5). For a modeled student who is Asian, female, and a PLTL non-completer with average academic preparation scores, a one-point increase in AP proportion decreased the predicted probability of attrition from 24.2% to 0.6%. Parallel to the performance results, this AP proportion effect interacted with PLTL, such that AP proportion became less influential when students completed PLTL. In other words, PLTL mitigated the tendency for students with less college-preparatory experience to leave between General Chemistry 1 and 2, although PLTL had no overall effect on attrition from the course sequence.
Table 5 Logistic regression testing for predictors of course-sequence attrition
Predictor |
b
|
SE |
z
|
Odds ratio |
* p < 0.05. Note: leaving the General Chemistry course sequence (vs. persisting to General Chemistry 2) is the dependent variable. OD refers to the Online Diagnostic of incoming content knowledge. AP proportion reflects how many STEM AP exams (Biology, Calculus, Chemistry, Physics) students earned a 4 or 5 on. Continuous variables were centered around their sample means. Categorical variables were treatment coded with PLTL non-completers, female, and Asian students as reference levels. N = 739. |
(Intercept) |
−5.16 |
2.26 |
−2.29* |
— |
ACT math |
0.15 |
0.07 |
2.27* |
1.17 |
AP proportion |
−1.82 |
0.88 |
−2.05* |
0.16 |
OD |
−0.01 |
0.01 |
−0.79 |
0.99 |
Early-semester GC1 belonging |
−0.15 |
0.25 |
−0.62 |
0.86 |
Early-semester GC1 uncertainty |
0.14 |
0.15 |
0.90 |
1.15 |
Late-semester GC1 belonging |
−0.85 |
0.20 |
−4.22* |
0.43 |
Late-semester GC1 uncertainty |
0.03 |
0.14 |
0.19 |
1.03 |
GC1 exam average |
−0.04 |
0.01 |
−2.84* |
0.97 |
PLTL (yes) |
−0.05 |
0.30 |
−0.18 |
0.95 |
Sex (M) |
0.36 |
0.46 |
0.79 |
1.44 |
Race (URM) |
0.15 |
0.54 |
0.29 |
1.17 |
Race (White) |
0.46 |
0.42 |
1.11 |
1.59 |
Sex (M) × race (URM) |
−0.88 |
0.81 |
−1.09 |
0.41 |
Sex (M) × race (White) |
−0.04 |
0.59 |
−0.07 |
0.96 |
AP proportion × PLTL |
1.98 |
1.00 |
1.97* |
7.21 |
ACT math was also a significant predictor, but it had an unexpected effect. Students with stronger math abilities (i.e., higher ACT math scores) were more likely to leave General Chemistry after one semester than students with weaker abilities. Given the narrow range of ACT math scores observed in this sample (M = 33.03, SD = 2.56), this effect may have had little practical impact, and it is unlikely to generalize to other student populations. The final preparation predictor, OD score, was not a significant predictor.
None of the demographic variables influenced student attrition from the course sequence. Among all students, performance in General Chemistry 1 proved to be an influential predictor: students who earned higher exam scores were less likely to leave after one semester. Specifically, for the modeled student (i.e., an average-preparation Asian, female, PLTL non-completer), the predicted probability of attrition was 21.4% if her exam average was below the sample mean compared to 3.9% if her average was above the mean.
Crucially, the only other significant predictor was late-semester GC1 belonging, which had a negative effect on attrition. For the modeled student, as her late-semester GC1 belonging increased from 4 to 5 to 6, her predicted probability of attrition decreased from 20.2% to 6.2% to 0.6%, respectively. None of the other belonging measures – early-semester GC1 belonging and uncertainty, and late-semester GC1 uncertainty – significantly contributed to the model. Finally, interactions between each of the belonging measures and demographic variables were also tested, showing little impact on the attrition model (Appendix 4).
Discussion
This investigation extends previous research on undergraduate belonging and success in STEM, which has typically focused on physical sciences with stark gender disparities, into the more gender-balanced discipline of chemistry. Specifically, this study examined two facets of first-year students’ sense of belonging, their course-level belonging and belonging uncertainty, in a two-semester general chemistry course sequence. Early-year belonging measures were examined as an important metric of first-year students’ affect during the challenging transition to college. The belonging measures were then assessed as potential predictors of general chemistry exam performance and attrition from the course sequence, after accounting for high-school academic preparation, demographics, and PLTL participation. Below, three key findings are summarized and interpreted relative to prior research.
Finding 1: academic preparation and gender affect early-year belonging and belonging uncertainty
Similar to other studies about more gendered physical science courses (Good et al., 2012; Stout et al., 2013), early-year GC1 belonging and belonging uncertainty were associated with academic preparation. Specifically, the preparation variables OD and AP proportion, but not ACT math, showed small but significant correlations with the early-year belonging measures. The more content knowledge (OD) and college-preparatory experience (AP proportion) incoming students had, the higher their sense of belonging and the lower their uncertainty early in the course sequence. In contrast, incoming mathematics ability was not influential. While these effects are not surprising, they shed light on how different facets of students’ academic background can independently affect their self-perceptions.
These results differ from the exact findings of prior research, which showed significant correlations between students’ ACT/SAT math scores and their belonging in math (Good et al., 2012) and physics (Stout et al., 2013). The difference across studies may be meaningful, e.g., reflecting a difference in the perceived importance of quantitative abilities for each course. If students believe math abilities to be more important for success in calculus and physics courses than in chemistry, then their belonging in those contexts may be more dependent on quantitative skills. Alternatively, the difference in results may be an artefact of the different analyses in each study. In the studies cited above, math scores were the only index of students’ academic preparation. This contrasts with the current study, where inter-correlations among the three academic covariates may have prevented detection of significant effects (Graham, 2003). Regardless, the results of this study support the conclusion that students with weaker academic preparation feel less belonging and more uncertainty upon entering college-level STEM courses.
Also consistent with previous research (Good et al., 2012; Stout et al., 2013; Blaney and Stout, 2017; Rainey et al., 2018), there was a main effect of gender on both early-semester GC1 belonging and belonging uncertainty. Male students reported more early-year belonging, while female students reported more early-year uncertainty. For belonging only, the effect of gender interacted with race, indicating that the gender difference was strongest among underrepresented minority students, with a marginal gender effect among White students and none among Asian students. This interactive pattern parallels the work of Blaney and Stout (2017) and Rainey et al. (2018), whose intersectional studies demonstrated that students who identify with multiple underrepresented groups (e.g., first-generation women and women of color, respectively) are especially vulnerable to low belonging in STEM.
Thus, gendered belonging gaps are not limited to those physical sciences where women remain drastically underrepresented at the undergraduate level. Instead, women can also experience lower belonging or more doubts in physical sciences like chemistry and other STEM disciplines where bachelor's degree attainment has become more equitable (National Science Foundation, 2019) and classrooms are more likely to be gender-balanced. This finding aligns with work from the field of gender studies, which illustrates how persistent cultural stereotypes about women's STEM abilities continue to undermine their participation in STEM. Even when women have positive attitudes toward fields like math, negative stereotypes can hurt their interest and performance (Shapiro and Williams, 2012). In a society with gendered expectations about learners’ potential in STEM, anticipating or feeling low belonging can dissuade women from continuing in STEM majors (Thoman et al., 2014; Tellhed et al., 2017).
Moreover, gender gaps can interact with other aspects of students’ identity to alleviate or increase perceptions of identity threat, i.e., the perception that one's identity is a liability (Cohen and Garcia, 2008), and influence belonging accordingly. In this study, the main effects of race on early-semester GC1 belonging outcomes did not indicate an overall tendency for underrepresented students to report lower belonging or higher uncertainty. However, the significant interaction of gender and race on early-year belonging indicates that women of color were particularly susceptible to low belonging at the outset of general chemistry. Thus, these results support the idea that students who identify with multiple groups underrepresented in STEM may face compound challenges to belonging.
Finding 1 implies that students with less academic preparation or from underrepresented demographic groups feel less belonging and more uncertainty as they enter introductory-level STEM courses, or they develop those belonging concerns within the first few weeks. While this may seem discouraging, it points towards an opportunity for instructors to disrupt these negative perceptions at the start of a course. Indeed, more than one theoretical model argues that a supportive and inclusive classroom environment provides the foundation for undergraduate students’ sense of belonging, motivation, and achievement (e.g., Cohen and Garcia, 2008; Zumbrunn et al., 2014). Moreover, decades of program evaluation has demonstrated that these principles extend beyond the classroom, as honors programs designed to foster the academic skills and support networks of underrepresented or under-resourced student groups have consistently increased their success, retention, and advancement in STEM fields (e.g., the Treisman model, Fullilove and Treisman, 1995; the Meyerhoff Scholars Program, Maton et al., 2000; the Biology Scholars Program, Matsui et al., 2003; and the SAGE project, Hall et al., 2014). Several strategies for cultivating an inclusive classroom and instilling the belief that all students can succeed are discussed below in the Practical Strategies section.
Finding 2: early-semester belonging and uncertainty predict general chemistry exam performance
The second part of this study examined the impact of course-level belonging and belonging uncertainty on General Chemistry 1 and 2 exam performance, after accounting for student background and participation in PLTL. Replicating previous studies of this course (Fink et al., 2018; Frey et al., 2018), stronger academic preparation predicted higher exam averages in both semesters. In both courses, PLTL completion also predicted better performance, and this effect interacted with AP proportion. This result extends previous research, showing that PLTL is an important resource for less-prepared students not only in General Chemistry 1 (Frey et al., 2018), but throughout the course sequence. In terms of demographics, the only statistically significant finding was a racial achievement gap.
Most importantly, the belonging measures significantly predicted first-years’ general chemistry performance above and beyond the other variables. In General Chemistry 1, only early-semester belonging (not belonging uncertainty) was a significant predictor: a one-point increase in early-semester GC1 belonging was associated with 1.77 point increase in exam average. In General Chemistry 2, both belonging measures added to the predictive power of the performance model. The effects were similar in magnitude to the first-semester course, with a one-point increase in early-semester GC2 belonging predicting a 1.71 point increase on exams, and a one-point decrease in early-semester GC2 uncertainty predicting a 1.79 point increase on exams. Combined, these two effects indicate a cumulative 3.5 point advantage on exams for students who begin General Chemistry 2 with one point more belonging and one point less uncertainty than their peers. While these belonging effects are modest in size, they provide useful information to researchers and practitioners aiming to understand and maximize student success in general chemistry. From the student perspective, they also have practical importance, because 3.5 points on exams could make the difference between letter grades.
Finding 2 aligns with previous research showing that belonging in STEM influences the success of all students, having an overall effect on achievement that can perpetuate initial gender disparities in belonging (Good et al., 2012; Stout et al., 2013). The current study also expands upon those findings, showing separate effects of course-level belonging and belonging uncertainty on exam averages in General Chemistry 2. The fact that these belonging measures remain influential in the second semester was somewhat surprising, because General Chemistry 1 and 2 are tightly connected at this institution, sharing the same structure, policies, and even some of the instructors. This similarity led to the expectation that students might acclimate and feel secure in their belonging by second semester, dampening any effects of belonging on performance in General Chemistry 2. Instead, the persistent belonging effects suggest that instructors must continually be aware of and address belonging concerns throughout the course sequence. Although the instructors may feel very comfortable with students by the end of General Chemistry 1, first-year students may need ongoing support and encouragement to maintain belonging and achievement, especially if they come from underrepresented groups.
Finding 3: late-semester belonging in general chemistry 1 predicts attrition from the course sequence
The final part of this study examined the relationship between course-level belonging during General Chemistry 1 and attrition from the course sequence, once again adjusting for academic preparation, demographics, and PLTL participation, as well as performance in General Chemistry 1. In terms of preparation, the key result was a significant effect of college-preparatory experience: students with lower AP proportion scores were more likely to leave the course sequence between semesters. Given that General Chemistry 1 performance is accounted for in the attrition model, with better performance significantly lowering the odds of attrition, the effect of AP proportion is particularly noteworthy. This result demonstrates the value of college-preparatory coursework: above and beyond its contribution via better course performance, such coursework helps first-year students persist through early college-level STEM requirements.
No demographic predictors were significant. Contrary to previous research (Mitchell et al., 2012; Lewis, 2014), PLTL participation did not have an overall effect on attrition from general chemistry. However, a significant interaction between AP proportion and PLTL emerged, indicating that PLTL participation neutralized the tendency for less-prepared students to depart from the course sequence after General Chemistry 1. In other words, PLTL increased the equity of the course sequence, because PLTL participants with little AP experience were no more likely to leave after General Chemistry 1 than PLTL participants with extensive AP experience. This novel result adds to the evidence showing PLTL's many benefits (Wilson and Varma-Nelson, 2016).
Crucially, the attrition analysis included four belonging variables: early- and late-semester GC1 belonging and belonging uncertainty. Only late-semester GC1 belonging had a significant, negative effect on attrition. That is, the higher a student's belonging towards the end of General Chemistry 1, the less likely they were to depart from the course sequence rather than completing General Chemistry 2 as well.
To our knowledge, this represents the first study to demonstrate an overall impact of belonging on retention specifically within general chemistry. This result both complements and diverges from prior studies, which focused specifically on the impact of belonging on women's persistence in STEM majors (London et al., 2011) and the pre-medicine pathway (Rosenthal et al., 2013). The current study did not detect a selective or increased effect of belonging on the attrition rates of women or other underrepresented groups. Instead, it revealed that after accounting for variation in academic preparation and performance in General Chemistry 1, belonging can affect all students’ decisions about whether to complete the general chemistry course sequence. Such results help motivate further investigation of students’ subjective experiences and belonging in STEM classrooms, especially using qualitative methods that might illuminate how and why different students’ belonging decreases, increases, or remains stable over time.
Limitations
While the findings of this study shed light on the role of belonging in students’ success in general chemistry, they are subject to some important limitations. As with any education research conducted at a single college or university, the results of this study may not generalize across institutions. They may replicate at other selective, private, research universities, or they may prove to be unique to this specific context and student population. The extra credit that participants received as compensation for enrolling in this study may have also influenced its findings, although that credit comprised a very small portion of students’ laboratory grade (0.5%). A related issue is the usage of instructor-written exams rather than a standardized assessment like the American Chemical Society General Chemistry exam. Without a standardized exam, the current results cannot be directly compared with other studies. Regardless, this research reinforces the important role that belonging in particular and student affect in general can play in determining undergraduates’ outcomes in chemistry and STEM (e.g., Trujillo and Tanner, 2014; Wilson et al., 2015).
Another limitation of this study is its relatively simplistic treatment of student demographics. The race variable collapsed across unique ethnicities, and the sex variable served as an imperfect proxy for gender, which does not necessarily reflect students’ self-defined gender identities. While such simplifications are common research practices, which often mitigate sample size issues and streamline analysis, they prevent a more nuanced understanding of students’ subjective experiences in chemistry and STEM learning environments. The evidence-base would therefore benefit from large-scale quantitative studies that might overcome sample size concerns to examine belonging across an array of groups, as well as qualitative research that delves deeply into the experiences of underrepresented or under-resourced groups of interest (e.g., Rainey et al., 2018). In general, qualitative research examining all students’ experiences and belonging in chemistry is needed. The large sample of participants in this study made quantitative methods more feasible; however, this approach does not enable deep exploration of the underpinnings and impact of belonging. Qualitative investigations may foster understanding of the possible routes to belonging and the ways that environmental factors modulate belonging and its effects on student success.
As a final limitation, this study may provide a conservative estimate of potential belonging effects in general chemistry, due to the social psychological interventions administered during the course sequence. Most students complete a growth-mindset intervention in General Chemistry 1 (Fink et al., 2018), and a subset also completed a belonging intervention in General Chemistry 2, although the latter showed no effects on student affect or performance (Appendix 1). While these interventions target different social psychological processes (Yeager and Walton, 2011), both are intended to improve students’ subjective experiences in the classroom, especially among underrepresented groups. These activities, combined with other strategies adopted by the General Chemistry instructor team to create an inclusive classroom (e.g., transparent syllabi, active learning pedagogy), may have reduced group-wise variation in belonging and washed out effects of belonging on performance and attrition. Future studies may reveal more robust effects if they examine belonging in undergraduate chemistry courses that place less emphasis on student attitudes and inclusion.
Measures of belonging
A unique feature of the current study is its usage of two belonging measures, perceived belonging and belonging uncertainty, to represent different aspects of students’ sense of belonging. Prior research has not addressed the separability of these two constructs, but the current results and validation analyses (Appendix 2) suggest they are at least partially distinct, with independent effects on student performance in General Chemistry 2. More work is needed to clarify the relationship between these measures and to evaluate whether one tends to be more sensitive to demographic or contextual variation in student perceptions. In addition, research that examines the conceptual overlap between belonging uncertainty and phenomena like imposter syndrome (Cockley et al., 2015; Tao and Gloria, 2019) could synthesize multiple lines of research and advance the field's understanding of gender differences in STEM outcomes.
In general, both the education and psychology communities might benefit from research that refines and expands upon current measures of belonging. With some exceptions (e.g., Good et al., 2012), many studies of belonging in undergraduate STEM education, including this one, have utilized quite brief surveys adapted from previous work. These instruments often ask about students’ general perceptions of belonging in a STEM context (e.g., I feel like I fit in the General Chemistry course) or about one or two specific components of belonging (e.g., I feel comfortable with my instructors in the General Chemistry course). However, Hirsch and Clark (2019) recently argued that multiple pathways towards student belonging exist, which can interact and should therefore be studied in tandem. Thus, the development of multifaceted, validated measures of student belonging could clarify what it means to belong in STEM settings and point towards more precise interventions for supporting student belonging and success.
Practical strategies for supporting belonging and student success
Current evidence supports an explanatory model where the STEM classroom environment influences self-perceptions of belonging, which modulate students’ motivation and engagement in the course, with consequences for achievement (Zumbrunn et al., 2014). Moreover, this affect-cognition-behavior chain is thought to be cyclic and self-reinforcing, such that negative self-perceptions contribute to maladaptive learning strategies and poor performance, which beget more negative perceptions, and so on (Yeager and Walton, 2011). While this model suggests that low belonging can set chemistry students on a downward trajectory, it also indicates multiple points for intervention. The strategies outlined below, which focus on students’ potential to excel, their interests and goals, and collaborative learning with others, represent core tenets of several successful, large-scale initiatives aimed at improving the belonging and success of underrepresented and under-resourced groups (Fullilove and Treisman, 1995; Maton et al., 2000; Matsui et al., 2003; Hall et al., 2014).
One strategy aims to directly boost belonging by creating an inclusive and supportive environment. Several studies have demonstrated that students are sensitive to environmental factors like gendered classrooms (Cheryan et al., 2009; Cheryan et al., 2011), as well as messages about the fixedness of learners’ abilities (Good et al., 2012; Canning et al., 2019) and gender stereotypes about quantitative skills (Good et al., 2012). Therefore, instructors can examine whether masculine or majority norms dominate their classroom or course materials and work to incorporate more diverse representation. In addition, instructors can explicitly convey a growth mindset, i.e., a belief that all learners’ can grow and improve, in their syllabus and course policies. This message can be reinforced at critical points in the semester (e.g., after each exam has been taken or exam grades are posted) when students struggle to maintain positive self-beliefs.
Another strategy is to target student motivation, rather than belonging per se. Motivation encompasses a number of different process, but one process with both intuitive appeal and theoretical grounding is student interest. Harackiewicz and colleagues (2016a, 2016b) have argued that spurring and growing interest in course content can set students on a path of persistent engagement and success, and they offer several concrete strategies for cultivating it. For example, course assignments known as utility-value interventions prompt students to explain in writing how a course topic is useful to them or relates to their personal interests and goals (Canning and Harackiewicz, 2015). Such interventions can improve the interest of all undergraduates and offer performance benefits to underrepresented groups, reducing achievement gaps (Harackiewicz et al., 2016a, 2016b). In general, both formal and informal strategies to personalize and connect a course with students’ own interest can help diverse students succeed.
A final way to mitigate potential effects of low belonging is to target student engagement through active learning pedagogies. Extensive research has demonstrated that student-centered tasks where undergraduates generate their own knowledge or co-construct it with peers tend to improve the performance of all students (Freeman et al., 2007), with larger effects among students from under-resourced or underrepresented groups (Haak et al., 2011). Thus, if belonging concerns trigger disengagement, active learning may disrupt that process and prompt students to re-engage. In addition, the process of exchanging ideas with peers may actually increase students’ sense of community and ultimately improve their belonging (Wilton et al., 2019).
Conclusion
This study has illustrated that first-year undergraduates’ course-level belonging and belonging uncertainty can impact their achievement and persistence throughout general chemistry, which is an important requirement for students interested in many STEM and healthcare professions. When women and other underrepresented groups experience low belonging or high uncertainty early in the course sequence, as observed here, such belonging effects may contribute to inequities in post-graduate STEM education and the workforce. Therefore, even in disciplines like chemistry where near gender parity has been achieved at the undergraduate level, there remains a need for STEM educators to carefully assess the culture and expectations conveyed by their classroom environments and pedagogical practices. A consistent message that everyone belongs and is capable of learning, which is sustained throughout the academic year, may become a self-fulfilling prophecy of success for diverse students.
Conflicts of interest
There are no conflicts of interest.
Appendix 1: analyses confirming null effect of belonging intervention in general chemistry 2 Spring 2018
A total of 325 first-year students enrolled in General Chemistry 2 in Spring 2018 and participated in the random-assignment experiment examining the effects of a belonging intervention. Three ANCOVAs were conducted to test for intervention effects on participants’ final exam scores, late-semester GC2 belonging, and late-semester GC2 belonging uncertainty. All three models included ACT math, AP proportion scores, and OD scores as covariates indexing students’ academic preparation. The models predicting late-semester GC2 belonging outcomes also included exam average as a covariate. In terms of categorical variables, the model included sex, race, and their two-way interaction. Finally, the main effect of interest was intervention condition (belonging vs. control), which was allowed to interact with the demographic variables. As shown in Tables 6–8, no significant effects of intervention condition were found.
Table 6 ANCOVA testing effect of belonging intervention on Spring 2018 final exam scores
Variable |
SS |
df |
MS |
F
|
η
p
2
|
* p < 0.05. n = 325. OD refers to the Online Diagnostic of incoming content knowledge. AP proportion reflects how many STEM AP exams (Biology, Calculus, Chemistry, Physics) students earned a 4 or 5 on. |
ACT math |
749 |
1 |
749 |
4.28* |
0.01 |
AP proportion |
3421 |
1 |
3421 |
19.53* |
0.06 |
OD |
9060 |
1 |
9060 |
51.73* |
0.14 |
Sex |
52 |
1 |
52 |
0.30 |
<0.01 |
Race |
460 |
2 |
230 |
1.31 |
<0.01 |
Intervention condition |
115 |
1 |
115 |
0.65 |
<0.01 |
Sex × race |
140 |
2 |
70 |
0.40 |
<0.01 |
Sex × intervention condition |
2 |
1 |
2 |
0.005 |
<0.01 |
Race × intervention condition |
497 |
2 |
249 |
1.42 |
0.01 |
Residuals |
54 643 |
312 |
175 |
|
|
Table 7 ANCOVA testing effect of belonging intervention on Spring 2018 late-semester GC2 belonging
Variable |
SS |
df |
MS |
F
|
η
p
2
|
* p < 0.05. † 0.05 < p < 0.10. n = 325. OD refers to the Online Diagnostic of incoming content knowledge. AP proportion reflects how many STEM AP exams (Biology, Calculus, Chemistry, Physics) students earned a 4 or 5 on. |
ACT math |
0.08 |
1 |
0.08 |
0.17 |
<0.01 |
AP proportion |
0.04 |
1 |
0.04 |
0.08 |
<0.01 |
OD |
1.71 |
1 |
1.71 |
3.63† |
0.01 |
Sex |
0.11 |
1 |
0.11 |
0.24 |
<0.01 |
Race |
0.06 |
2 |
0.03 |
0.06 |
0.01 |
Intervention condition |
0.52 |
1 |
0.52 |
1.11 |
<0.01 |
Exam Average |
18.49 |
1 |
18.49 |
39.14* |
0.11 |
Sex × race |
0.04 |
2 |
0.02 |
0.04 |
<0.01 |
Sex × intervention condition |
0.17 |
1 |
0.17 |
0.36 |
<0.01 |
Race × intervention condition |
0.71 |
2 |
0.36 |
0.76 |
<0.01 |
Residuals |
146.92 |
311 |
0.47 |
|
|
Table 8 ANCOVA testing effect of belonging intervention on Spring 2018 late-semester GC2 belonging uncertainty
Variable |
SS |
df |
MS |
F
|
η
p
2
|
* p < 0.05. n = 325. OD refers to the Online Diagnostic of incoming content knowledge. AP proportion reflects how many STEM AP exams (Biology, Calculus, Chemistry, Physics) students earned a 4 or 5 on. |
ACT math |
0.21 |
1 |
0.21 |
0.15 |
<0.01 |
AP proportion |
1.74 |
1 |
1.75 |
1.26 |
<0.01 |
OD |
3.48 |
1 |
3.48 |
2.53 |
0.01 |
Sex |
1.80 |
1 |
1.80 |
1.31 |
0.02 |
Race |
0.15 |
2 |
0.08 |
0.06 |
<0.01 |
Intervention condition |
0.41 |
1 |
0.41 |
0.30 |
<0.01 |
Exam average |
26.08 |
1 |
26.08 |
18.97* |
0.06 |
Sex × race |
2.30 |
2 |
1.15 |
0.84 |
0.01 |
Sex × intervention condition |
0.05 |
1 |
0.05 |
0.04 |
<0.01 |
Race × intervention condition |
0.14 |
2 |
0.07 |
0.05 |
<0.01 |
Residuals |
427.6 |
311 |
1.38 |
|
|
Despite the null effects of the belonging intervention on student outcomes, concern remained that the experiment in spring 2018 might have unduly influenced the results of this study. As a result, the General Chemistry 2 performance model and the attrition model were both re-run with the spring 2018 semester excluded. For performance, two changes were observed in the reduced model. First, the effect of early-semester GC2 belonging on General Chemistry 2 exam averages no longer reached significance, though the regression coefficient showed only a modest decrease of 0.18 points in magnitude (b = 1.53, β = 0.07, t(235) = 1.26, p = 0.21). The effect of early-semester GC2 belonging uncertainty on General Chemistry 2 exams remained significant (b = −1.65, β = −0.13, t(235) = −2.25, p = 0.03). In addition, the interaction between AP proportion and PLTL became marginally significant (b = −8.25, β = −0.13, t(235) = −1.81, p = 0.07). In the attrition model, the only shift was that the unexpected, positive effect of ACT math on attrition failed to reach significance (b = 0.13, SE = 0.11, z(300) = 1.18, p = 0.24).
These changes may be due at least partially to a reduction in power. With the exclusion of all spring 2018 students (n = 344), the General Chemistry 2 performance sample shrank from 592 to 248 students, and the attrition sample shrank from 739 to 395 students. A post hoc power calculation indicates that the chances of detecting a small effect in the General Chemistry 2 performance model, for example, decreased from 96% to 72% with the reduced sample. Because the descriptive pattern of results remained essentially unchanged, despite some fluctuations in significance, results from the full sample were reported in the main text.
Appendix 2: factor analysis of belonging survey
A combination of explanatory factor analysis (EFA) and confirmatory factor analysis (CFA) of the pre-survey data from General Chemistry 1 (Fall 2017 and 2018) was used to determine the factor structure of the belonging survey. The original belonging survey comprised seven items, including the six items listed in the Methods section and one additional item: “People in the General Chemistry course are a lot like me.” To maximize the sample, responses from all available consenting students were used regardless of their year in school and provision of other measures (N = 1238). This sample was randomly split in half, with EFA performed on one half of the data (N = 619) and CFA performed on the other (n = 619). All factor analyses were conducted using the R package lavaan (Rosseel, 2012).
Description of data
None of the survey items were missing more than 1.4% of responses. Mean values ranged from 2.87 to 5.12 (out of 6). Item skew ranged from |0.09| to |1.10| and kurtosis ranged from |0.44| to |2.29|. Mardia's multivariate normality test indicated that these are significant skewness and kurtosis values (Psych package, Revelle, 2017). Inter-item correlations ranged from −0.06 to 0.60.
Factor analysis parameters
A principal axis estimator, which is one of the most commonly used estimators for ordinal and non-normal data (Knekta et al., 2019), was selected to extract variance for the EFA. As required for by lavaan, only complete cases (i.e., observations with responses to all seven survey items) were included. An oblique factor rotation (oblimin) was implemented, because of the expectation that any potential subscales would be correlated. To determine the number of factors, several methods were used, including visual analysis of a skree plot, eigenvalues greater than one, and parallel analysis. These metrics indicated anywhere from one to three factors.
EFAs
An initial round of EFAs was conducted, testing one-, two-, and three-factor structures for the original belonging survey of seven items. The three-factor model did not converge correctly. Instead, it produced a Heywood case error (i.e., a pattern loading greater than 1), suggesting that the seven-item belonging survey did not support such a complex factor structure. The one- and two-factor structures did converge and accounted for 39% and 49% total variance, respectively. The pattern coefficients for all items exceeded a minimum threshold of 0.40 in both models. However, the communality values for the item listed above (“…a lot like me”) were low in both models (h2 ≤ 0.28), indicating that its variance was not well-explained by the available factors. As a result, this item was removed from the survey.
A second set of EFAs was constructed using one-factor and two-factor structures to explain the abridged six-item survey, and the pattern coefficients are presented in Table 9. The one-factor model accounted for 40% total variance, while the two-factor model accounted for 53% variance. The two-factor model also showed higher communality values on average than the one-factor model. Finally, the two-factor structure aligns with the literature and the development of this instrument: items 1 through 4 were drawn from previous surveys on students’ perceived belonging (London et al., 2011; Walton and Cohen, 2011), while items 5 and 6 were have been used to measure students’ belonging uncertainty (Walton and Cohen, 2007, 2011). For these reasons, the two-factor solution was chosen for further analysis.
Table 9 Final belonging survey items and EFA pattern coefficients
|
One-factor structure |
Two-factor structure |
1 |
1 |
2 |
1. I feel like I fit |
0.84 |
0.64 |
|
2. I feel comfortable with my peers and classmates |
0.69 |
0.83 |
|
3. I feel comfortable with my instructors |
0.55 |
0.66 |
|
4. Setting aside my performance, I feel like I belong |
0.67 |
0.55 |
|
5. I feel uncertain about my belonging |
−0.61 |
|
0.76 |
6. If I don’t perform, I feel like maybe I don’t belong |
−0.48 |
|
0.67 |
CFAs
Following Knekta et al. (2019), three model fit indices were used to evaluate whether the two-factor structure adequately fit the second half of the General Chemistry 1 sample. The comparative fit index (CFI) provided a relative fit index, the standardized root-mean-square residual (SRMR) provided an absolute fit index, and root-mean-square error of approximation (RMSEA) provided a parsimony-adjusted fit index. The thresholds for acceptability were CFI > 0.95, SRMR < 0.08, and RMSEA < 0.06. The CFA validating the two-factor structure for the belonging survey yielded the following fit indices: CFA = 0.962, SRMR = 0.031, and RMSEA = 0.098. Thus, two out of three measures indicated adequate fit, with the poor RMSEA score suggesting that the model was more complex than justified by the survey data.
As an additional test of the two-factor structure, another CFA was conducted on the pre-survey data from General Chemistry 2 (Spring 2018 and 2019), once again using all available data (N = 1111). This CFA produced the fit indices CFA = 0.984, SRMR = 0.023, and RMSEA = 0.066, with the 90% confidence interval for the RMSEA score (0.045–0.088) including the threshold for acceptability. The results therefore paralleled the findings above, showing acceptable relative and absolute fit, but borderline parsimony-adjusted fit.
Because the majority of fit indices were satisfactory, the two-factor model was accepted as the final structure for this survey. This decision was further justified by the theoretical considerations presented above: the distinction between perceived belonging (items 1–4) and belonging uncertainty (5–6) aligns with the literature. Nonetheless, the results of these factor analyses suggest that future research would benefit from the development or usage of more elaborated belonging surveys (e.g., Good et al., 2012). A wider range of survey items would not only enable more robust measurement and instrument validation, but it might also foster a more nuanced theory of belonging.
Appendix 3: performance models testing interactions between belonging measures and demographics
To evaluate the stability of the belonging effects across demographic groups, two-way interactions between the demographic variables (i.e., sex and race) and belonging variables (i.e., early-semester belonging and belonging uncertainty) were individually added to the General Chemistry 1 and 2 performance models (see Tables 3 and 4, respectively). Nested model comparisons were then used to determine if the addition of each interaction significantly improved the fit of the baseline models. Specifically, these comparisons used an F test to determine whether the baseline model's residual variance was significantly reduced when a new term was added. For example, if early-semester GC1 belonging had a larger effect on the General Chemistry 1 performance of female versus male students, then adding an interaction between sex and belonging should improve the accuracy of the model and significantly reduce its residual variance.
None of the model comparisons reached significance, although two marginal comparisons emerged (Tables 10 and 11). For the General Chemistry 1 model, the interaction of early-semester GC1 belonging and sex marginally improved the model's fit. The regression coefficient for this interaction revealed that the positive effect of early-semester GC1 belonging on exam averages was more than two points larger among male students compared to female students (b = 2.39, β = 0.07, t(725) = 1.84, p = 0.07; see Fig. 3). For the General Chemistry 2 model, the interaction of early-semester GC2 belonging uncertainty and sex marginally improved the model's fit. The regression coefficient for this interaction indicates that the negative effect of early-semester GC2 uncertainty on General Chemistry 2 exam performance was 1.5 points larger for female compared to male students (b = 1.51, β = 0.07, t(578) = 1.82, p = 0.07; see Fig. 4). On the whole, these results suggest that early-semester belonging and belonging uncertainty had fairly consistent effects on student achievement, with some minor variations in magnitude based on sex.
Table 10 Model comparisons adding interactions to the general chemistry 1 performance model
Interaction |
Change in residual |
df |
F
|
† 0.05 < p < 0.10. Note: comparable results were found when z-scored exam averages were used as the dependent variable in the baseline and nested models. |
Sex × early-semester GC1 belonging |
369.86 |
1 |
3.39† |
Sex × early-semester GC1 belonging uncertainty |
152.52 |
1 |
1.40 |
Race × early-semester GC1 belonging |
97.06 |
2 |
0.64 |
Race × early-semester GC1 belonging uncertainty |
131.16 |
2 |
0.55 |
Table 11 Model comparisons adding interactions to the general chemistry 2 performance model
Interaction |
Change in residual |
df |
F
|
† 0.05 < p < 0.10. Note: comparable results were found when z-scored exam averages were used as the dependent variable in the baseline and nested models. |
Sex × early-semester GC2 belonging |
0.59 |
1 |
0.01 |
Sex × early-semester GC2 belonging uncertainty |
383.74 |
1 |
3.31† |
Race × early-semester GC2 belonging |
74.82 |
2 |
0.32 |
Race × early-semester GC2 belonging uncertainty |
65.97 |
2 |
0.28 |
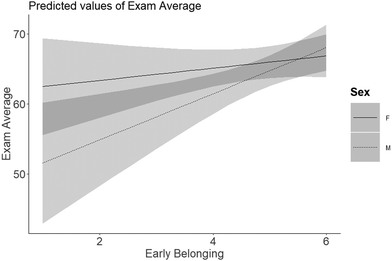 |
| Fig. 3 Marginal effects plot of the early-semester GC1 belonging and sex interaction from the General Chemistry 1 performance model. Depicts the predicted exam averages for female (solid line) and male (dotted line) students who report different levels of early-semester GC1 belonging, while holding all other predictors in the performance model constant. | |
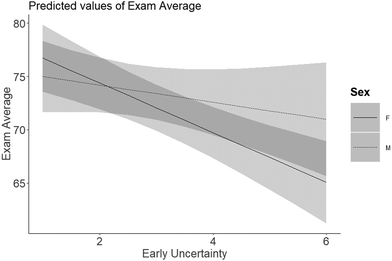 |
| Fig. 4 Marginal effects plot of the early-semester GC2 uncertainty and sex interaction from the General Chemistry 2 performance model. Depicts the predicted exam averages for female (solid line) and male (dotted line) students who report different levels of early-semester GC2 uncertainty, while holding all other predictors in the performance model constant. | |
Appendix 4: attrition model testing interactions between belonging measures and identity
Nested model comparisons were also used to test for variation in belonging effects on attrition from the General Chemistry sequence. In this case, the comparisons used Chi-square tests to determine whether the addition of two-way interactions between the demographic variables and belonging variables significantly reduced the residual deviance of the baseline model. As shown in Table 12, only the interaction of sex and late-semester GC1 belonging uncertainty significantly improved the model's fit.
Table 12 Model comparisons adding interactions to the general chemistry retention model
Interaction |
Change in residual |
df |
* p < 0.05. |
Sex × early-semester GC1 belonging |
0.16 |
1 |
Sex × late-semester GC1 belonging |
0.21 |
Sex × early-semester GC1 belonging uncertainty |
2.59 |
Sex × late-semester GC1 belonging uncertainty |
4.43* |
|
Race × early-semester GC1 belonging |
0.13 |
2 |
Race × late-semester GC1 belonging |
0.50 |
Race × early-semester GC1 belonging uncertainty |
1.17 |
Race × late-semester GC1 belonging uncertainty |
1.89 |
To interpret this interaction, follow-up logistic regression models were constructed to separately examine the attrition of female and male students. These models revealed that late-semester GC1 belonging uncertainty did not have a significant effect on the attrition of either group (p's > 0.37). Instead, the interaction above reflects how these non-significant effects patterned in opposite directions. Among female students, late-semester GC1 belonging uncertainty had a positive (ns) relationship with students’ decisions to leave general chemistry (β = 0.17, SE = 0.19, z = 0.90, odds ratio = 1.19). Among male students, there was a negative (ns) relationship between late-semester GC1 belonging uncertainty and attrition (β = −0.14, SE = 0.21, z = −0.66, odds ratio = 0.87).
Overall, these findings support the conclusion that belonging had relatively stable effects on student persistence in the general chemistry sequence. The key finding that late-semester GC1 belonging predicted students’ attrition from second-semester general chemistry (see Table 5) did not differ across demographic groups. Only minor sex-based variation emerged, which parallels the performance findings.
Appendix 5: assessment of multi-collinearity in regression analyses
As a first step towards evaluating the presence of multi-collinearity in the performance and attrition regression analyses, bivariate correlations among all continuous variables from the full sample were calculated. This does not include the General Chemistry 2 belonging measures, which were only available for first-year students who continued on to the second semester. The conventional thresholds for small, medium, and large correlations are 0.1, 0.3, and 0.5, respectively (Cohen, 1988). As shown in Table 13, the largest observed correlation is r = 0.55, suggesting that the variables (early- and late- semester GC1 belonging) are strongly related but far from redundant.
Table 13 Pearson correlation coefficients among continuous predictors
|
ACT |
AP |
OD |
E-S GC1 belonging |
L-S GC1 belonging |
E-S GC1 uncertainty |
L-S GC1 uncertainty |
GC1 exam |
Note: E-S indicates early-semester, and L-S indicates late-semester. N = 739. |
ACT |
1 |
0.44 |
0.49 |
0.14 |
0.18 |
−0.15 |
−0.19 |
0.49 |
AP |
|
1 |
0.50 |
0.19 |
0.21 |
−0.19 |
−0.24 |
0.47 |
OD |
|
|
1 |
0.20 |
0.24 |
−0.23 |
−0.28 |
0.54 |
E-S GC1 belonging |
1 |
0.55 |
−0.53 |
−0.35 |
0.21 |
L-S GC1 belonging |
1 |
−0.35 |
−0.53 |
0.41 |
E-S GC1 uncertainty |
|
1 |
0.52 |
−0.18 |
L-S GC1 uncertainty |
|
|
1 |
−0.41 |
GC1 exam |
|
|
|
|
1 |
The second strategy used to assess multi-collinearity involved calculation of Variance Inflation Factors (VIFs) for each regression model. When a specific predictor is affected by multi-collinearity, the standard error of its regression coefficient increases, making the analysis less likely to detect significance even when the effect is real (i.e., a false negative/type II error; Graham, 2003). VIFs gauge the degree to which the standard error of each regression coefficient has been exaggerated due to multi-collinearity. There is no consensus about the VIF threshold for severe multi-collinearity; although many scholars have pointed towards VIFs greater than 10 as problematic, others have argued that VIFs as low as 2 can be cause for concern (Graham, 2003). In the current study, the largest VIFs were 4.14 in the General Chemistry 1 performance model, 2.43 in the General Chemistry 2 performance model, and 3.86 in the attrition model, all corresponding to the AP proportion predictor. The majority of other VIFs were less than 2. Given that AP proportion proved significant in all regression analyses, it seems that multi-collinearity did not cause false negatives.
Finally, the regression analyses were re-run with only those variables and interactions found to be significant, in order to check the stability and interpretation of the initial results. For the General Chemistry 1 performance model, the most substantive change pertained to the effect of early-semester GC1 belonging, which increased slightly in magnitude and become significant at a lower α (b = 1.99, β = 0.09, t(730) = 3.14, p = 0.002). For the General Chemistry 2 performance model, the only change applied to the effect of race, indicating a larger difference between under-represented minority students and the Asian reference group, which was also significant at a lower α (b = −4.28, β = −0.11, t(582) = −2.97, p = 0.003). For the attrition model, the primary change applied to the interaction between AP proportion and PLTL, which became only marginally significant (b = 1.73, SE = 0.96, z(732) = 1.80, p = 0.07). On the whole, the pattern of results remained unchanged, suggesting they are fairly stable and not subject to a high degree of multi-collinearity.
Acknowledgements
This research was funded by an Inclusive Excellence grant from the Howard Hughes Medical Institute (Award No. 42008723; PI: Regina Frey) to Washington University in St. Louis. We thank Jia Luo, Rick Schneider, and the General Chemistry Assistants in Instruction for their help in administering this study, and we are grateful to the General Chemistry instructor team for their support of this project. We also thank Mike Cahill for his IRB and data collection efforts and his statistical support, and Liana Bloom and Ethan Wiseman for their assistance in entering the survey data.
References
- ACT, (2018), About ACT, viewed November, 2019, https://www.act.org.
- Aronson J., Fried C. B. and Good C., (2002), Reducing the effects of stereotype threat on African American college students by shaping theories of intelligence, J. Exp. Soc. Psychol., 38(2), 113–125.
- Baumeister R. F. and Leary M. R., (1995), The need to belong: desire for interpersonal attachments as a fundamental human motivation, Psychol. Bull., 117(3), 497–529.
- Behrendt S., (2014), lm.beta: Add Standardized Regression Coefficients to lm-Objects, R package version 1.5-1, https://CRAN.R-project.org/package=lm.beta.
- Blackwell L. S., Trzesniewski K. H. and Dweck C. S., (2007), Implicit theories of intelligence predict achievement across an adolescent transition: a longitudinal study and an intervention, Child Dev., 78(1), 246–263.
- Blaney J. M. and Stout J. G., (2017), ‘Examining the relationship between introductory computing course experiences, self-efficacy, and belonging among first-generation college women’, 2017 ACM SIGCSE Technical Symposium on Computer Science Education, 8–11 March, 2017, pp. 69–74.
- Canning E. A. and Harackiewicz J. M., (2015), Teach it, don’t preach it: the differential effects of directly-communicated and self-generated utility–value information, Motiv. Sci., 1(1), 47–71.
- Canning E. A., Muenks K., Green D. J. and Murphy M. C., (2019), STEM faculty who believe ability is fixed have larger racial achievement gaps and inspire less student motivation in their classes, Sci. Adv., 5(2), eaau4734.
- Carr P. B. and Walton G. M., (2014), Cues of working together fuel intrinsic motivation, J. Exp. Soc. Psychol., 53, 169–184.
- Cheryan S., Plaut V. C., Davies P. G., and Steele C. M., (2009), Ambient belonging: how stereotypical cues impact gender participation in computer science, J. Pers. Soc. Psychol., 97(6), 1045–1060.
- Cheryan S., Meltzoff A. N. and Kim S., (2011), Classrooms matter: the design of virtual classrooms influences gender disparities in computer science classes, Comput. Educ., 57(2), 1825–1835.
- Cohen J., (1988). Statistical Power Analysis for the Behavioral Sciences, 2nd edn, Hillsdale, NJ: Erlbaum.
- Cohen G. L. and Garcia J., (2008), Identity, belonging, and achievement: a model, interventions, implications, Curr. Dir. Psychol. Sci., 17(6), 365–369.
- Cokley K., Awad G., Smith L., Jackson S., Awosogba O., Hurst A., Stone S., Blondeau L. and Roberts D., (2015), The roles of gender stigma consciousness, impostor phenomenon and academic self-concept in the academic outcomes of women and men, Sex Roles, 73, 414–426.
- College Board, (2019), viewed November, 2019, https://www.collegeboard.org/.
- Cracolice M. S. and Busby B. D., (2015), Preparation for college General Chemistry: more than just a matter of content knowledge acquisition, J. Chem. Educ., 92(11), 1790–1797.
- Dorans N. J., (1999), Correspondences between ACT™ and SAT® I scores, College Board Research Report 99-1, New York: The College Board.
- Ferrell B. and Barbera J., (2015), Analysis of students' self-efficacy, interest, and effort beliefs in general chemistry, Chem. Educ. Res. Pract., 16(2), 318–337.
- Fink A., Cahill M. J., McDaniel M. A., Hoffman A., and Frey R. F., (2018), Improving general chemistry performance through a growth mindset intervention: selective effects on underrepresented minorities, Chem. Educ. Res. Pract., 19(3), 783–806.
- Freeman T. M., Anderman L. H. and Jensen J. M., (2007), Sense of belonging in college freshmen at the classroom and campus levels, J. Exp. Educ., 75(3), 203–220.
- Frey R. F., Cahill M. J. and McDaniel M. A., (2017), Students’ concept-building approaches: a novel predictor of success in chemistry courses, J. Chem. Educ., 94(9), 1185–1194.
- Frey R. F., Fink A., Cahill M. J., McDaniel M. A., and Solomon E. D., (2018), Peer-Led Team Learning in General Chemistry I: Interactions with Identity, Academic Preparation, and a Course-Based Intervention, J. Chem. Educ., 95(12), 2103–2113.
- Fullilove R. E. and Treisman P. U., (1995), Mathematics achievement among African American undergraduates at the University of California, Berkeley: an evaluation of the mathematics workshop program. J. Negro Educ., 59(3), 463–478.
- Gere J. and MacDonald G., (2010), An update of the empirical case for the need to belong, J. Indiv. Psychol., 66(1), 93–115.
- Gillen-O'Neel C. and Fuligni A., (2013), A longitudinal study of school belonging and academic motivation across high school, Child Dev., 84(2), 678–692.
- Good C., Aronson J. and Inzlicht M., (2003), Improving adolescents' standardized test performance: an intervention to reduce the effects of stereotype threat, J. App. Devel. Psychol., 24(6), 645–662.
- Good C., Rattan A. and Dweck C. S., (2012), Why do women opt out? Sense of belonging and women's representation in mathematics, J. Pers. Soc. Psychol., 102(4), 700–717.
- Goodenow C., (1993), Classroom belonging among early adolescent students: relationships to motivation and achievement, J. Early Adol., 13(1), 21–43.
- Graham M. H., (2003), Confronting multicollinearity in ecological multiple regressions, Ecology, 84(11), 2809–2815.
- Haak D. C., HilleRisLambers J., Pitre E. and Freeman S., (2011), Increased structure and active learning reduce the achievement gap in introductory biology, Science, 332(6034), 1213–1216.
- Hall D. M., Curtin-Soydan A. J. and Canelas D. A., (2014), The Science Advancement through Group Engagement program: leveling the playing field and increasing retention in science, J. Chem. Ed., 91, 37–47.
- Harackiewicz J. M., Canning E. A., Tibbetts Y., Priniski S. J. and Hyde J. S., (2016a), Closing achievement gaps with a utility-value intervention: disentangling race and social class, J. Pers. Soc. Psychol., 111(5), 745–765.
- Harackiewicz J. M., Smith J. L. and Priniski S. J., (2016b), Interest matters: the importance of promoting interest in education, Policy Insights Behav. Brain Sci., 3(2), 220–227.
- Hausmann L. R., Schofield J. W. and Woods R. L., (2007), Sense of belonging as a predictor of intentions to persist among African American and white first-year college students, Res. Higher Educ., 48(7), 803–839.
- Hirsch J. L, and Clark M. S., (2019), Multiple paths to belonging that we should study together, Perspect. Psychol. Sci., 14(2), 238–255.
- Hockings S. C., DeAngelis K. J. and Frey R. F., (2008), Peer-led team learning in general chemistry: implementation and evaluation, J. Chem. Educ., 85(7), 990–996.
- Hoffman M., Richmond J., Morrow J. and Salomone K., (2002), Investigating “sense of belonging” in first-year college students, J. Coll. Stud. Ret.: Res., Theory, Pract., 4(3), 227–256.
- Hosbein
K. N. and Barbera J., (2020), Alignment of theoretically grounded constructs for the measurement of science and chemistry identity, Chem. Educ. Res. Pract., 21(1), 371–386.
- Hurtado S. and Carter D. F., (1997), Effects of college transition and perceptions of the campus racial climate on Latino college students' sense of belonging, Sociol. Educ., 70(4), 324–345.
- Johnson D. R., (2012), Campus racial climate perceptions and overall sense of belonging among racially diverse women in STEM majors, J. Coll. Stud. Dev., 53(2), 336–346.
- Jury M., Smeding A., Stephens N. M., Nelson J. E., Aelenei C. and Darnon C., (2017), The experience of low-SES students in higher education: psychological barriers to success and interventions to reduce social-class inequality, J. Soc. Issues, 73(1), 23–41.
- Knekta E., Runyon C. and Eddy S., (2019), One size doesn’t fit all: using factor analysis to gather validity evidence when using surveys in your research, CBE – Life Sci. Educ., 18(1), rm1.
- Lenth R., (2019), emmeans: Estimated Marginal Means, aka Least-Squares Means, R package version 1.4, https://CRAN.R-project.org/package=emmeans.
- Lewis S. E., (2014), Investigating the longitudinal impact of a successful reform in general chemistry on student enrollment and academic performance, J. Chem. Educ., 91(12), 2037–2044.
- Lewis S. E. and Lewis J. E., (2007), Predicting at-risk students in general chemistry: comparing formal thought to a general achievement measure, Chem. Educ. Res. Pract., 8(1), 32–51.
- Lewis S. E., Shaw J. L., Heitz J. O. and Webster G. H., (2009), Attitude counts: self-concept and success in general chemistry, J. Chem. Educ., 86(6), 744–749.
- Lewis K. L., Stout J. G., Pollock S. J., Finkelstein N. D. and Ito T. A., (2016), Fitting in or opting out: a review of key social-psychological factors influencing a sense of belonging for women in physics, Phy. Rev. Phys. Educ. Res., 12(2), 020110.
- Liptow E. E., Chen K., Parent R., Duerr J. and Henson D., (2016), ‘A sense of belonging: creating a community for first-generation, underrepresented groups and minorities through an engineering student success course’, ASEE's 23rd Annual Conference & Exposition, New Orleans, Louisiana, 26–29 June, 2016.
- London B., Rosenthal L., Levy S. R. and Lobel M., (2011), The influences of perceived identity compatibility and social support on women in nontraditional fields during the college transition, Basic App. Soc. Psychol., 33(4), 304–321.
- Mallett R. K., Mello Z. R., Wagner D. E., Worrell F., Burrow R. N. and Andretta J. R., (2011), Do I belong? It depends on when you ask, Cultur. Divers. Ethnic Minor. Psychol., 17(4), 432–436.
- Marra R. M., Rodgers K. A., Shen D. and Bogue B., (2012), Leaving engineering: a multi-year single institution study, J. Eng. Educ., 101(1), 6–27.
- Master A. and Walton G. M., (2013), Minimal groups increase young children's motivation and learning on group-relevant tasks, Child Dev., 84(2), 737–751.
- Maton K. I., Hrabowski III F. A. and Schmitt C. L., (2000), African American college students excelling in the sciences: college and postcollege outcomes in the Meyerhoff Scholars Program, J. Res. Sci. Teach., 37(7), 629–654.
- Matsui J., Liu R. and Kane C. M., (2003), Evaluating a science diversity program at UC Berkeley: more questions than answers, Cell Biol. Educ., 2, 117–121.
- Mitchell Y. D., Ippolito J. and Lewis S. E., (2012), Evaluating peer-led team learning across the two semester general chemistry sequence, Chem. Educ. Res. Pract., 13(3), 378–383.
- Murphy M. C. and Zirkel S., (2015), Race and belonging in school: How anticipated and experienced belonging affect choice, persistence, and performance, Teach. Coll. Rec., 117(12), 1–40.
- National Academy of Sciences, (2011), Expanding underrepresented minority participation, Washington, D.C.: The National Academies Press, available at http://grants.nih.gov/training/minority_participation.pdf.
- National Science Foundation, National Center for Science and Engineering Statistics, (2019), Women, minorities, and persons with disabilities in science and engineering: 2019, Special Report NSF 17-310, Arlington, VA, available at http://www.nsf.gov/statistics/wmpd/.
- Navarro D. J., (2015), Learning statistics with R: A tutorial for psychology students and other beginners (Version 0.5), Adelaide, Australia: University of Adelaide.
- O'Keeffe P., (2013), A sense of belonging: improving student retention, Coll. Stud. J., 47(4), 605–613.
- Olson S. and Riordan D. G., (2012), Engage to Excel: Producing One Million Additional College Graduates with Degrees in Science, Technology, Engineering, and Mathematics (Report to the President), Washington, D.C.: Executive Office of the President.
- Paunesku D., Walton G. M., Romero C., Smith E. N., Yeager D. S. and Dweck C. S., (2015), Mind-set interventions are a scalable treatment for academic underachievement, Psychol. Sci., 26(6), 784–793.
- R Core Team, (2019), R: A language and environment for statistical computing, R Foundation for Statistical Computing, Vienna, Austria, https://www.R-project.org/.
- Rahman K., (2013), Belonging and learning to belong in school: the implications of the hidden curriculum for indigenous students, Discourse: Studies Cultur. Politics Educ., 34(5), 660–672.
- Rainey K., Dancy M., Mickelson R., Stearns E. and Moller S., (2018), Race and gender differences in how sense of belonging influences decisions to major in STEM, Intl. J. STEM Educ., 5(1), 10.
- Rattan A., Savani K., Komarraju M., Morrison M. M., Boggs C. and Ambady N., (2018), Meta-lay theories of scientific potential drive underrepresented students’ sense of belonging to science, technology, engineering, and mathematics (STEM), J. Pers. Soc. Psychol., 115(1), 54–75.
- Revelle W., (2017), Psych: Procedures for psychological, psychometric, and personality research, Evanston, Illinois: Northwestern University.
- Richardson J. T., (2011), Eta squared and partial eta squared as measures of effect size in educational research, Educ. Res. Rev., 6(2), 135–147.
- Rosenthal L., Levy S. R., London B., Lobel M. and Bazile C., (2013), In pursuit of the MD: the impact of role models, identity compatibility, and belonging among undergraduate women, Sex Roles, 68(7), 464–473.
- Rosseel Y, (2012), lavaan: an R package for structural equation modeling, J. Stat. Softw., 48(2), 1–36.
- Seery M. K., (2009), The role of prior knowledge and student aptitude in undergraduate performance in chemistry: a correlation-prediction study, Chem. Educ. Res. Pract., 10(3), 227–232.
- Shapiro J. R. and Williams A. M., (2012), The role of stereotype threats in undermining girls’ and women's performance and interest in STEM fields, Sex Roles, 66(3-4), 175–183.
- Shields S. P., Hogrebe M. C., Spees W. M., Handlin L. B., Noelken G. P., Riley J. M. and Frey R. F. (2012), A transition program
for underprepared students in general chemistry: diagnosis, implementation, and evaluation, J. Chem. Educ., 89(8), 995–1000.
- Shnabel N., Purdie-Vaughns V., Cook J. E., Garcia J. and Cohen G. L., (2013), Demystifying values-affirmation interventions: writing about social belonging is key to buffering against identity threat, Pers. Soc. Psychol. Bull., 39(5), 662–676.
- Shteynberg G. and Galinsky A. D., (2011), Implicit coordination: sharing goals with similar others intensifies goal pursuit, J. Exp. Soc. Psychol., 47(6), 1291–1294.
- Slaten C. D., Elison Z. M., Deemer E. D., Hughes H. A. and Shemwell D. A., (2018), The development and validation of the university belonging questionnaire, J. Exp. Educ., 86(4), 633–651.
- Smith J. L., Lewis K. L., Hawthorne L. and Hodges S. D., (2013), When trying hard isn’t natural: women's belonging with and motivation for male-dominated STEM fields as a function of effort expenditure concerns, Pers. Soc. Psychol. Bull., 39(2), 131–143.
- Stephens N. M., Townsend S. S., Markus H. R. and Phillips L. T., (2012), A cultural mismatch: independent cultural norms produce greater increases in cortisol and more negative emotions among first-generation college students, J. Exp. Soc. Psychol., 48(6), 1389–1393.
- Stout J. G., Ito T. A., Finkelstein N. D. and Pollock S. J., (2013), How a gender gap in belonging contributes to the gender gap in physics participation, AIP Conf. Proc., 1513(1), 402–405.
- Tai R. H., Sadler P. M. and Loehr J. F., (2005), Factors influencing success in introductory college chemistry, J. Res. Sci. Teach., 42(9), 987–1012.
- Tao K. W. and Gloria A. M., (2019), Should I stay or should I go? The role of impostorism in STEM persistence, Psychol. Women Quart., 43(2), 151–164.
- Tellhed U., Bäckström M. and Björklund F., (2017), Will I fit in and do well? The importance of social belongingness and self-efficacy for explaining gender differences in interest in STEM and HEED majors, Sex Roles, 77(1-2), 86–96.
- Thoman D. B., Arizaga J. A., Smith J. L., Story T. S. and Soncuya G., (2014), The grass is greener in non-science, technology, engineering, and math classes: examining the role of competing belonging to undergraduate women's vulnerability to being pulled away from science, Psychol. Women Quart., 38(2), 246–258.
- Tinto V., (1993), Leaving College: Rethinking the Causes and Cures of Student Attrition, 2nd edn, Chicago: University of Chicago Press.
- Trujillo G. and Tanner K. D., (2014), Considering the role of affect in learning: monitoring students' self-efficacy, sense of belonging, and science identity, CBE – Life Sci. Educ., 13(1), 6–15.
- Veilleux N., Bates R., Allendoerfer C., Jones D., Crawford J. and Floyd-Smith T., (2013), ‘The relationship between belonging and ability in computer science’, 44th ACM Technical Symposium on Computer Science Education, 6–9 March, 2013, pp. 65–70.
- Villafañe S. M. and Lewis J. E., (2016), Exploring a measure of science attitude for different groups of students enrolled in introductory college chemistry, Chem. Educ. Res. Pract., 17(4), 731–742.
- Walton G. M. and Cohen G. L., (2007), A question of belonging: race, social fit, and achievement, J. Pers. Soc. Psychol., 92(1), 82–96.
- Walton G. M. and Cohen G. L., (2011), A brief social-belonging intervention improves academic and health outcomes of minority students, Science, 331, 1447–1451.
- Walton G. M., Cohen G. L., Cwir D. and Spencer S. J., (2012), Mere belonging: the power of social connections, J. Pers. Soc. Psychol., 102(3), 513–532.
- Walton G. M, Logel C., Peach J. M., Spencer S. J. and Zanna M. P., (2015), Two brief interventions to mitigate a “chilly climate” transform women's experience, relationships, and achievement in Engineering, J. Educ. Psychol., 107(2), 468–485.
- Walton G. M., Murphy M. C., Logel C., Yeager D. S. and The College Transition Collaborative, (2017), The Social-Belonging Intervention: A Guide For Use and Customization.
- Wilson S. B. and Varma-Nelson P., (2016), Small groups, significant impact: a review of peer-led team learning research with implications for STEM education researchers and faculty, J. Chem. Educ., 93(10), 1686–1702.
- Wilson D., Jones D., Bocell F., Crawford J., Kim M. J., Veilleux N., Floyd-Smith T., Bates R. and Plett M., (2015), Belonging and academic engagement among undergraduate STEM students: a multi-institutional study, Res. Higher Educ., 56(7), 750–776.
- Wilton M., Gonzalez-Niño E., McPartlan P., Terner Z., Christoffersen R. E. and Rothman J. H., (2019), Improving Academic Performance, Belonging, and Retention through Increasing Structure of an Introductory Biology Course, CBE – Life Sci. Educ., 18(4), ar53.
- Xu X., Villafane S. M. and Lewis J. E., (2013), College students’ attitudes toward chemistry, conceptual knowledge and achievement: structural equation model analysis, Chem. Educ. Res. Pract., 14(2), 188–200.
- Yeager D. S. and Dweck C. S., (2012), Mindsets that promote resilience: when students believe that personal characteristics can be developed, Educ. Psychol., 47(4), 302–314.
- Yeager D. S. and Walton G. M., (2011), Social-psychological interventions in education: they’re not magic, Rev. Educ. Res., 81(2), 267–301.
- Yeager D. S., Romero C., Paunesku D., Hulleman C. S., Schneider B., Hinojosa C., Lee H. Y., O’Brien J., Flint K., Roberts A., Trott J., Greene D., Walton G. M. and Dweck C. S., (2016a), Using design thinking to improve psychological interventions: the case of the growth mindset during the transition to high school, J. Educ. Psychol., 108(3), 374–391.
- Yeager D. S., Walton G. M., Brady S. T., Akcinar E. N., Paunesku D., Keane L., Kamentz D., Ritter G., Duckworth A. L., Urstein R., Gomez E. M., Markus H. R., Cohen G. L. and Dweck C. S., (2016b), Teaching a lay theory before college narrows achievement gaps at scale, Proc. Natl. Acad. Sci. U. S. A., 113(24), E3341–E3348.
- Yorke M., (2016), The development and initial use of a survey of student ‘belongingness’, engagement and self-confidence in UK higher education, Assess. Eval. Higher Educ., 41(1), 154–166.
- Zumbrunn S., McKim C., Buhs E. and Hawley L. R., (2014), Support, belonging, motivation, and engagement in the college classroom: a mixed method study, Instrl. Sci., 42(5), 661–684.
Footnotes |
† We alternate between the terms “gender” and “sex” in this manuscript, using them for specific purposes. Gender refers to students’ socially-constructed gender identity, and it is referenced throughout the introduction and discussion. This terminology links the current study to previous education and psychology literature exploring gender and its effects on student success in STEM. Sex refers to students’ biological sex, and it is used in the methods and results sections. As reported below, the university instrument used to gather demographic information asked for students’ sex, so this terminology most accurately represents those data. |
‡ When z-scored exam average was used as the dependent variable, this predictor was only marginally significant, p = 0.08. Z-Scoring did not impact the significance level of any other predictor. |
|
This journal is © The Royal Society of Chemistry 2020 |
Click here to see how this site uses Cookies. View our privacy policy here.