Increasing chemistry students’ knowledge, confidence, and conceptual understanding of pH using a collaborative computer pH simulation†
Received
10th October 2019
, Accepted 9th January 2020
First published on 13th January 2020
Abstract
The purpose of this quasi-experimental non-equivalent pretest posttest control group research was to determine whether or not the use of a collaborative pH computer simulation has an impact on the knowledge, confidence, and conceptual understanding of fundamental pH concepts for undergraduate Chemistry II students. A purposeful sample of 59 Chemistry II undergraduate students were administered pre/post assessments and surveys. Results indicated higher pH knowledge, pH confidence, and pH conceptual understanding among those students who had completed the collaborative computer simulation modules (23 students/intervention group) instead of the alternative traditional classroom assignments (36 students/control group).
Introduction
Undergraduate students in chemistry courses are entering and completing these courses with a lack of understanding of fundamental chemistry concepts and/or critical chemistry misconceptions (Kind, 2004; Cetingul and Geban, 2005; Barke et al., 2010). Stieff and Wilensky (2003) studied students’ chemistry misunderstandings and found that most of them occurred because of the abstract and complex nature of the science of chemistry. The three levels of chemical representation: macroscopic, submicroscopic, and symbolic (Gilbert and Treagust, 2009) further complicate chemistry comprehension. The relationship among the three representations is known as the “triplet relationship” and the submicroscopic level is particularly difficult to comprehend due to its abstract nature. In addition, another problem with comprehension seems to arise from the language used by teachers, textbook writers, and scientists that does not adequately portray the three levels as separate from one another but instead blurs them, requiring students to shift from one level to another without written prompts, a skill most have not yet acquired and may also result in the formation of a common misconception “of transmission of substance macroscopic properties to its submicroscopic particles (Rodic et al., 2018, p. 395). Thus, for optimum chemical understanding, students must have an understanding of and be able to navigate among all three levels (Smith and Villarreal, 2015). In addition, Kozma and Russell (1997) found that students enter college chemistry courses with a variety of everyday life experiences, all of which occurred at macroscopic levels, yet in chemistry they are immediately thrust into the foreign and imperceptible submicroscopic environment of the particles of matter further complicated by the fact that these particles are represented by a wide range of symbols. This new, complicated perspective has proven to be challenging for many students.
Several research studies have shown that when students engage with supplemental multimedia websites to enhance traditional lecture instruction, larger learning gains can be realized, and interest, engagement, and attentiveness increase (Hannafin et al., 2009; Lamb and Annetta, 2009; Lamb et al., 2011). For example, Bayraktar's (2001) meta-analysis revealed that of 42 computerized science simulation (sim) studies conducted between 1971 and 1999, all but one positively impacted student achievement. Liao (1998) conducted a similar meta-analysis of 52 studies regarding the impact of computer science sims on student learning and, like Bayraktar, Liao found that computer-assisted science simulations positively impacted students’ science achievement. The most recent meta-analysis of 62 science sim instruction studies by Smetana and Bell (2012) reflected the overwhelming effectiveness of computerized science sims on students’ science learning, including the facilitation of conceptual change. Of the types of computer-assisted science instruction discussed in the literature, simulations and interactions were identified as most effective (Holzinger et al., 2009), followed by tutorials and drill-and-practice (Howard, 2009).
When science educators incorporate instructional technologies into traditional pedagogy, they offer students new possibilities of observing and experiencing abstract concepts in ways not possible with traditional lecture-based instruction. Particularly suited to computer sims is the study of chemistry. Because utilizing computer sims as tools for accessing these abstract phenomena has been instrumental in increasing student comprehension of abstract chemical concepts, we opted to utilize a pH computer simulation, the PhET Interactive Simulations project (University of Colorado Boulder). In addition, we chose to incorporate the use of the pH sim while students worked collaboratively in groups of three or four. As previously mentioned, and further substantiated by Smetana and Bell (2012), those chemical processes that take place at the macroscopic level have submicroscopic elucidations often expressed in symbolic or mathematical terminology that are very difficult for students to visualize. According to Stieff and Wilensky (2003), computerized visualization tools such as simulations, can be effective tools for the development of representation competence among chemistry students. “Multi-representational visualizations of imperceptible objects and phenomena make explicit the information embedded in external representations with interactive visual displays, thus helping students perceive the relationship between the representing and represented world (Stieff and Wilensky, 2003, p. 1139). Thus, utilizing computer sims as tools for accessing these abstract phenomena has been instrumental in increasing student comprehension of abstract chemical concepts.
Also critical for optimal learning gains by students utilizing computer sims is the amount and type of guidance they receive while interacting with the simulations. A recent study examining guidance levels associated with a chemistry computerized simulation revealed that less guidance resulted in students who were more focused on the content, while significant guidance prompted students to be most concerned with the investigation process rather than the content (Bonawitz et al., 2011). Similar studies investigating levels of guidance afforded students in pedagogical contexts revealed that student-led investigations are often more productive and more thorough than those led by instructors (Kirschner et al., 2006; Mostmans et al., 2012). It is important to note however, that a lack of guidance can leave students unsure of procedures and may result in less interaction with the sim (Kirschner et al., 2006; Mostmans et al., 2012).
Computer-supported collaborative learning (CSCL)
Computer-Supported Collaborative Learning (CSCL) is defined in the literature as a learning science associated with students who learn together with computer-assisted support (Phielix et al., 2010). CSCL software is beneficial when utilized in small groups because users learn through interaction with others and the software. Group members become privy to how others learn, ask questions of, and teach the other members, articulate and argue about their ideas, and make collective decisions (Phielix et al., 2010).
Phielix et al. (2010) found that CSCL results in higher levels of achievement, more sophisticated decision making, increased exposure to diverse viewpoints, and an increase in frequency of complex and thought-provoking discussions among students when compared to traditional classroom learning. Also impacting the perceived success of CSCL is the degree of interaction it offers. The greater the amount of interaction between users and the computer simulation, the more intuitive CSCL becomes (Lou et al., 2001; Bennett et al., 2010).
The research literature addressing computer simulation as an instructional tool usually presents this form of instruction on an individual or small group basis, with small groups being optimal due to peer mediation and interaction (Smetana and Bell, 2012). However, according to Bennett et al. (2010), small group interaction is most successful when members are provided instruction in effective collaboration. One cannot assume, therefore, that simply putting students in small groups will result in productive collaboration (Smetana and Bell, 2012). Also instrumental to effective group work is students’ and teachers’ prior experience and comfort with collaborative learning (Kulick and Kulick, 1978). Collaborative learning with a computerized simulation, when facilitated appropriately, can allow for the co-construction of knowledge among all group members through conversations with each other and the instructor while interacting with the simulation.
Also critical to collaborative learning success is the careful assignment of individuals to groups. Fiechtner and Davis (1984) identified characteristics of groups often associated with students’ negative collaborative learning experiences including: self-selected groups, homogenous groups, groups of less than four or more than seven members, and short-term groups (those created for one task and then immediately disbanded). According to Vygotsky (1978), team members should be individually evaluated for ability and potential group contribution before being assigned to a group. Other considerations for group assignment include gender, culture, language, age, and learning context preferences. Vygotsky's (1978)Zone of Proximal Development (ZPD) suggests that weaker students can gain knowledge and skills from stronger students as they interact with one another in groups. Thus, heterogeneous groups are preferred as homogeneous grouping could result in groups that are too accomplished to complete a task or groups lacking requisite skills making them unable to perform a task successfully (Vygotsky, 1978). Furthermore, Volman and van Eck (2001) found that males in mixed gender groups engaging in computer simulations tend to dominate females in the groups, commandeering the simulation activity. Females, on the other hand, prefer engaging in communication and forging relationships with other group members (Hannan, 2011) and are often better than males at visualizing graphical representations (Volman and van Eck, 2001).
The physics education technology (PhET) project
The PhET Interactive Simulations project at the University of Colorado Boulder was utilized in this research study. The PhET project contains 127 interactive simulations (sims) free for public use, 30 of which are related to chemistry. PhET sims are utilized by K-12 educators, college instructors, and university faculty to encourage students to learn about scientific processes. The research literature contains numerous examples of studies conducted utilizing the PhET sims, including research regarding their use in classroom settings, students’ perceptions of learning with PhET sims, the development of PhET simulations, and inclusivity and group dynamics in relation to in-class PhET sim usage. For example, Watson (2019) examined how utilizing the PhET sim along with the 5E instructional model enhanced students’ pH understandings, Hale-Hanes (2015) investigated students’ modeling and scientific inquiry skills in acid–base chemistry using the PhET pH simulation. Additionally, Moore et al. (2014) shared resources for integrating PhET sims into introductory chemistry courses, while Roll et al. (2014) examined the impact scaffolding has on knowledge and attitudes in online simulations, including PhET. Moreover, there have been several studies conducted concerning PhET simulations and group dynamics. For example, Wieman et al. (2010) investigated the use of PhET simulations in different educational settings, including small groups, while Keller et al. (2006) examined the use of PhET simulations in different introductory undergraduate environments to include groups using peer instruction. Finally, Stephens and Clement (2015) conducted a comparative case study regarding the use of sims in whole class versus small group settings.
Utilized in this study was the PhET acid–base solutions sim (see Fig. 1), an investigation into how various acids and bases affect solution's pH. The primary capabilities of this sim include observation of the effects of an acid/base's strength and initial concentration on pH and conductivity, and its microscopic, macroscopic, and symbolic representations (Chamberlain et al., 2014).
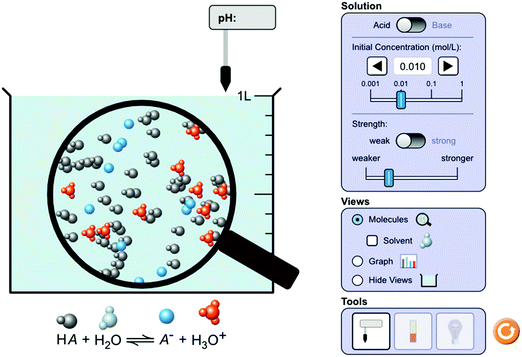 |
| Fig. 1 Acid–base solutions PhET simulation. Image by PhET interactive simulations and used with permission. | |
Research purpose and questions
This study aimed to determine whether or not the use of a collaborative pH computer simulation had an impact on the pH knowledge (ability to solve standard problems), confidence, and conceptual understanding of undergraduate Chemistry II students. The following research questions guided this study:
1. Does participation in a pH sim activity increase pH knowledge?
2. Is there a statistically significant mean difference in pH knowledge between the computer sim and traditional group?
3. Does participation in a pH sim activity increase pH confidence levels?
4. Does participation in a pH sim activity increase pH conceptual understanding?
5. Is there a statistically significant mean difference in pH conceptual understanding between the computer sim and traditional group?
Methods
Overview
This quasi-experimental research was comprised of two groups: (a) computer sim group/intervention and (b) the traditional/control group. Twenty-three students participated in a collaborative pH computer simulation activity, while 36 students did not participate in the computer simulation and instead completed the alternative traditional reading-based classroom assignments. To address Research Questions 1 through 5, all 59 students were administered an assessment and survey after covering the pH-related chapters in a General Chemistry II course, prior to and following participation in the optional collaborative sim activity.
Participants
A purposeful sample of 59 Chemistry II undergraduate students participated in this study. The majority of the participants were women comprising 71.2% (n = 42) of the sample. Regarding race/ethnicity, the majority of the participants identified themselves as Caucasian or White (42.4%, n = 25), Hispanic or Latino (32.2%, n = 19), Asian (16.9%, n = 10), and Black or African American (3.4%, n = 2). Biological Sciences majors made up 64.4% (n = 38) of the population, while 1.7% (n = 1) were Physics majors, 13.6% (n = 8) were Fitness and Human Performance majors, 5.1% (n = 3) were Environmental Science majors, 5.1% (n = 3) were Pre-engineering, and 3.4% (n = 2) were Chemistry majors. Table 1 provides a breakdown of participant demographics overall and per group.
Table 1 Participant demographics
|
Overall (n = 59) |
Computer sim group (n = 23) |
Traditional group (n = 36) |
1. Gender |
Male |
17 |
6 |
11 |
Female |
42 |
17 |
25 |
2. Race/ethnicity |
Asian |
10 |
5 |
5 |
Caucasian/White |
25 |
8 |
17 |
Black/African American |
2 |
1 |
1 |
Hispanic/Latino |
19 |
7 |
12 |
Native American |
1 |
0 |
1 |
Two or more races |
2 |
2 |
0 |
3. College major |
Art & Design |
1 |
0 |
1 |
Biological Science |
38 |
14 |
24 |
Chemistry |
2 |
1 |
1 |
Computer Science |
1 |
0 |
1 |
Environmental Science |
3 |
0 |
3 |
Fitness & Human Performance |
8 |
5 |
3 |
Interdisciplinary Studies |
1 |
1 |
0 |
Mechanical Engineering |
1 |
0 |
1 |
Physics |
1 |
0 |
1 |
Pre-Engineering |
3 |
2 |
1 |
Computer sim group
Twenty-three of the 59 students participated in a collaborative pH computer simulation activity (intervention/computer sim group). The individual student groups participating in the activity consisted of 3–4 students who were selected by the professor of record based on pre-test scores, gender, age, and student classification. Attempts were made to create groups that consisted of male and female students, lower and higher pretest scorers, older and younger students, and lower and higher student classifications. Groups were formed first by the instructor according to the previously mentioned criteria, then time selections occurred according to student group members’ availability. The majority of these participants were women comprising 73.9% (n = 17) of the sample. Regarding race/ethnicity, the majority of the participants identified themselves as Caucasian or White (34.8%, n = 8), Hispanic or Latino (30.4%, n = 7), Asian (21.7%, n = 5), and Black or African American (4.3%, n = 1). Biological science majors made up 61.0% (n = 14) of the participant population, while 21.8% (n = 5) were Fitness and Human Performance majors, 8.6% (n = 2) were Pre-engineering, and 4.3% (n = 1) were Chemistry majors.
Traditional group
The remaining 36 students did not participate in the computer simulation and instead completed the traditional reading-based classroom assignments (control/traditional group). The majority of these participants were women comprising 69.4% (n = 25) of the sample. Regarding race/ethnicity, the majority of the participants identified themselves as Caucasian or White (47.2%, n = 17), Hispanic or Latino (33.3%, n = 12), Asian (13.9%, n = 5), and Black or African American (2.8%, n = 1). Biological sciences was the major of 66.6% (n = 24) of the participant population, while 8.3% (n = 3) were Fitness and Human Performance majors, 8.3% (n = 3) Environmental Science, 2.8% (n = 1) were Pre-engineering, and 2.8% (n = 1) Chemistry majors.
Instrumentation
Students were required to complete a standard course pre/post assessment and perceptions survey dealing with the topic of pH, acids & bases. A multiple-choice chemistry assessments was then administered to measure knowledge (standard problem-solving skillset) of pH and was aligned to the curricular objectives/content of the undergraduate Chemistry II course. The pH Survey was a researcher-constructed instrument designed to assess student perceptions of the conceptual understanding of pH and their confidence in the subject. The survey items reflected the three levels of chemical representation: macroscopic, submicroscopic, and symbolic. Students were requested to provide responses to a combination of multiple-choice, fill-in the blank, Likert scale, and open-ended response items on the survey. The survey was aligned to the curricular objectives and content of the undergraduate Chemistry II course and was validated prior to implementation of the research by six chemical education experts.
Collaborative PhET simulation – acid–base solutions
Students in the computer sim group participated in a collaborative PhET simulation, acid–base solutions, that was offered shortly after the pH/acids&bases lecture. The week before students arrived for the collaborative computer simulation activity (orchestrated to be a series of drop-in group lab experiences which groups selected from several time slots), they were given instruction regarding effective group learning behaviors. Specifically, students were instructed in the five elements of effective cooperative learning: individual accountability, positive interdependence, face-to-face promotive interaction, group processing, and interpersonal and small group skills (Johnson and Johnson, 1975).
Student groups were then introduced to the PhET acid–base solutions simulation, were allowed time to interact with the simulation freely, and were then given a set of six pH quests/worksheets to work through together using the acid–base-solutions simulation as a learning tool. The topics of the six quests were as follows: (1) equilibria in water; (2) acids and bases; (3) strong and weak acids and bases; (4) introducing pH; (5) dependence of pH on concentration; and (6) pH of aqueous solutions. The six quests were to be approached in a sequence; the quests increased in difficulty and student teams must have successfully cleared quest one to be given permission to move on to quest two, etc.
Minimal directions were provided regarding the navigation of the sim in an effort to encourage students to figure out the various features of the sim rather than discovering them via a prescribed set of directions. Students also monitored their own understandings by making predictions based on their new knowledge and then checking those predictions against the simulation. In addition, during each of the six quests, students evaluated common student pH-related misconceptions such as: “strong acids are corrosive” and “acidic solutions contain hydronium ions while basic solutions contain hydroxide ions” and then were directed to interact with the sim or other provided data so that their findings would dispute the statements. Finally, many quests required student participants to consider themselves as teachers helping their future students understand the same concepts. For example, in Quest 2, students were asked to create guidelines for future students as to how they might “find [H3O+] (if given [OH−]), at room temperature.”
Data collection & analysis
IRB permission was granted prior to collecting any data. The Chemistry II students were informed of the purpose of the study, assured confidentiality, and told they could cease participation at any time without penalty. Students who agreed to participate in the study were required to sign informed consent forms (response rate = 100%). All of the students were assessed and surveyed prior to and following the optional pH sim activity in the General Chemistry II course. This study utilized quantitative data collected within the classroom setting via paper/pencil pre/posttest and an online survey. Following data collection, quantitative data were analyzed using two tailed independent t-tests (RQ 2, 5), two tailed paired t-tests (RQ 1, 3, 4), Mann–Whitney test (RQ 3), and the Wilcoxon signed rank test (RQ 3). To assess effect size, Cohen's d and the coefficient of determination (r2) were calculated and a significance value of 0.05 was utilized.
Results
Research question 1: knowledge of pH
The results of the paired t-test for the class as a whole indicated a statistically significant mean difference did not exist in pH knowledge prior to and following the optional sim activity, t(58) = −0.814, p = 0.419 (see Table 2). Although a statistically significant mean difference was not found to exist (p > 0.05), the mean difference appeared to be higher for the group of students who completed the computerized tutorial modules (Mpre = 2.22, Mpost = 2.56) in comparison to those only completing the traditional classroom assignments (Mpre = 2.33, Mpost = 2.33). The scores of the traditional group remained the same, while the scores of the collaborative pH computer sim group increased.
Table 2 pH knowledge: pre- and post-scores
pH knowledge |
Sample size |
Mean |
Standard deviation |
t-Value |
df |
p-Value |
*Statistically significant (p < 0.05). |
1. Pre-scores |
59 |
2.29 |
1.16 |
0.814 |
58 |
0.419 |
2. Post-scores |
59 |
2.42 |
1.16 |
|
|
|
Research question 2: computer sim vs. traditional group in pH knowledge
At the beginning of the semester, a baseline equivalence was established with participants intending to participate in the collaborative pH computer simulation tutorial modules and participants not intending to complete them. The results of the independent t-test indicated there was not a statistically significant mean difference (p > 0.05) between the two groups of students in terms of pH knowledge prior to any instruction being provided, t(57) = 0.372, p = 0.712. At the end of the semester, students were assessed once more. The results of the independent t-test indicated no statistically significant mean difference between the two groups of students in terms of pH knowledge following instruction being provided, t(57) = 0.744, p = 0.460 (see Table 3). Although the groups were not found to be statistically different, the average mean scores appeared to be higher for the collaborative computer sim group (MC = 2.57) than for the traditional group (MT = 2.33).
Table 3 pH knowledge: computer sim vs. traditional group
Group |
Sample size |
Mean |
Standard deviation |
t-Value |
df |
p-Value |
*Statistically significant (p < 0.05). |
1. Traditional |
36 |
2.33 |
1.24 |
0.744 |
57 |
0.460 |
2. Computer sim |
23 |
2.57 |
1.04 |
|
|
|
Research question 3: confidence levels of pH understanding
Students were asked to respond to a survey item concerning their confidence level of pH understanding prior to and following the optional sim activity (1 = not confident at all, 4 = very confident). The results of the Wilcoxon signed rank test for the class as a whole indicated a statistically significant mean difference existed in student confidence in pH understanding, Z = −4.99, p < 0.001. In other words, student confidence in pH understanding increased. Statistically significant mean differences (p < 0.001) were also found to exist when analyzing the pre/post confidence scores for the computerized sim group and then again for the traditional group. Although the results of the Mann–Whitney test did not reveal statistically significant differences between the two groups, the mean and median differences from pre to post appeared to be higher for the computerized sim group (MDC = 0.74, Median DC = 1.0) in comparison to the traditional group (MDC = 0.55, Median DC = 0.0). In addition, at the end of the semester 100.0% of the computerized sim group claimed to be mostly confident/very confident in comparison to 82.9% of the traditional group.
Research question 4: conceptual understanding of pH
The results of the paired t-test for the class as a whole indicated a statistically significant mean difference in the conceptual understanding of pH, t(58) = 11.47, p < 0.001, d = 1.52 (large effect size), r2 = 0.372 (see Table 4). The average mean score was significantly higher after participation in the optional sim activity (M = 9.61) in comparison to prior to participation (M = 5.65). Thirty-seven percent of the variation in pH understanding can be attributed to the course.
Table 4 Conceptual understanding of pH: pre- and post-scores
pH understanding |
Sample size |
Mean |
Standard deviation |
t-Value |
df |
p-Value |
d-Value |
r
2
|
*Statistically significant (p < 0.05). |
1. Pre-scores |
59 |
5.65 |
2.13 |
11.47 |
58 |
<0.001* |
1.52 |
0.372 |
2. Post-scores |
59 |
9.61 |
2.99 |
|
|
|
|
|
The results of the paired t-test for the collaborative pH computer simulation group indicated a statistically significant mean difference in the conceptual understanding of pH t(22) = 10.14, p < 0.001, d = 2.3 (large effect size), r2 = 0.563 (see Table 5). The average mean score was significantly higher after participation in the sim activity (Mpre = 5.35) in comparison to before participation (Mpost = 10.50). Fifty-six percent of the variation in the conceptual understanding of pH can be attributed to a combination of the course instruction and the collaborative pH computer sim tutorial modules. Although the results of the paired t-test for the traditional group also indicated a statistically significant mean difference in pH understanding, the mean difference between pre/post scores was higher for the group that completed the collaborative pH computer sim tutorial modules (MDC = 5.15) than for the traditional group (MDT = 3.19).
Table 5 Conceptual understanding of pH: computer sim group pre- and post-scores
pH understanding |
Sample size |
Mean |
Standard deviation |
t-Value |
df |
p-Value |
d-Value |
r
2
|
*Statistically significant (p < 0.05). |
1. Pre-scores |
23 |
5.35 |
1.92 |
10.14 |
22 |
<0.001* |
2.3 |
0.563 |
2. Post-scores |
23 |
10.50 |
2.59 |
|
|
|
|
|
Research question 5: computer sim group vs. traditional group conceptual understanding of pH
At the beginning of the semester, a baseline equivalence was established with participants intending to participate in the collaborative pH computer simulation tutorial modules and participants not intending to complete them. The results of the independent t-test indicated no statistically significant mean difference between the two groups of students (MC = 5.35, MT = 5.85) in terms of pH understanding prior to any instruction being provided, t(57) = 0.877, p = 0.384. After the optional pH sim activity, students were surveyed once more. The results of the independent t-test also indicated no statistically significant mean difference between the two groups of students in terms of conceptual understanding of pH, t(57) = 1.863, p = 0.068 (see Table 6). Although a statistically significant mean difference was not found to exist between the two groups, the average mean scores appeared to be higher for the collaborative computer sim group (MC = 10.5) than for the traditional group (MT = 9.0).
Table 6 Conceptual understanding pH: computer sim vs. traditional group
Group |
Sample size |
Mean |
Standard deviation |
t-Value |
df |
p-Value |
*Statistically significant (p < 0.05). |
1. Traditional |
36 |
9.0 |
3.13 |
1.86 |
57 |
0.068 |
2. Computer sim |
23 |
10.5 |
2.59 |
|
|
|
Discussion
This research study found that collaborative computer sim group members experienced higher mean scores regarding pH knowledge and conceptual understanding, and indicated higher levels of pH-related confidence from the beginning to the end of the semester when compared to the traditional group members. The findings of this study are in agreement with several related research studies (Hannafin et al., 2009; Lamb and Annetta, 2009; Smetana and Bell, 2012) in the literature that concluded that when students engage with supplemental multimedia to enhance traditional lecture instruction, learning gains are realized. However, our findings refute those of Hawkins and Phelps (2013) who found no statistical difference in learning gains between a group of students using a computerized sim on electrochemistry (treatment) versus a group of students who were taught electrochemistry via a traditional hands-on experiment (control). Perhaps the differences between our findings and those of Hawkins and Phelps (2013) lie in the collaborative element of this investigation. When students engage in small groups in CSCL (Howard, 2009), their decision-making processes are more sophisticated, they are exposed to more diverse viewpoints, and experience an increase in frequency of complex and thought-provoking discussions.
Our sim-centered set of activities were administered to each group in the form of a set of six worksheets (“quests”) increasing in their difficulty level. If students did not answer some of the particular quest questions correctly (as checked by instructor or TAs), they were not allowed to proceed to the next level without revisiting and eventually fixing their mistakes. This format added to students' engagement, enhanced their preparedness on higher level quests. It is also worth stating that the quests provoked critical thinking as they focused on understanding/applying/analyzing, and not on recalling/listing/remembering. Example follows, where A and B are pH-related observations: “Use sim to find how A results in B. How can you summarize and rationalize B? Because of your conclusion, can observation opposite of B ever happen? Rationalize why it can or can't happen,” rather than “here is A and B that we need to know about.” Particular emphasis was placed on presenting common misconceptions college students have about pH-related concepts, and attempts at debunking them.
Limitations
This research study is best interpreted with a consideration for its limitations. Data were collected from undergraduate Chemistry II students at one university in the Southeast United States; therefore, more empirical studies should be conducted at other colleges and universities to investigate whether or not the findings can be correlated to those at other educational institutions. Also, the sample group was relatively small and thus the study should be repeated with a larger student sample. Additional confounding factors that may have impacted the findings were the collaborative nature of the computer simulation group and the amount of contact with the course material. Finally, this study did not analyze the relationship between student learning as it relates to the individual design components of the simulation, thus student learning may have occurred as a result of group interaction or teacher facilitation rather than interaction with the sim. In future research studies, we will analyze sim and student interactions via video and audio recordings to pinpoint the role of the sim in student learning.
Conclusions
The findings of this research study indicated that the collaborative PhET sim positively impacted students’ pH conceptual understandings, which both supports and refutes the literature.
The PhET sim experience in this research investigation was a collaborative activity, which was administered in the form of a set of multiple worksheets/quests. When students did not answer some of the quest questions correctly, they were not allowed to proceed to the next level without reflecting on and eventually correcting their mistakes. The quests provoked critical thinking, and particular emphasis was placed on presenting common misconceptions college students have about pH-related concepts, and on debunking them. The groups were purposely designed and could have contributed to the positive mean differences in the treatment group members’ pH knowledge scores, their higher levels of pH-related confidence, and their increased understandings of pH from the beginning to the end of the semester as opposed to those not participating in the computer sim group. Perhaps, this approach is worth adopting for learning other chemistry topics, in addition to pH. Future studies are underway.
Conflicts of interest
There are no conflicts to declare.
References
- Barke H. D., Hazari A. and Yitbarek S., (2010), Misconceptions in chemistry: addressing perceptions in chemical education, Berlin, Germany: Springer-Verlag.
- Bayraktar S., (2001), A meta-analysis of the effectiveness of computer-assisted instruction in science education, J. Res. Tech. Ed., 34(2), 173–188.
- Bennett J., Hogarth S., Lubben F., Campbell B. and Robinson A., (2010), Talking science: the research evidence on the use of small group discussions in science teaching, Int. J. Res. Sci. Ed., 32(1), 69–95.
- Bonawitz E., Shafto P., Gweon H., Goodman N. D., Spelke E. and Schulz L., (2011), The double-edged sword of pedagogy: instruction limits spontaneous exploration and discovery, Cognition, 120, 322–330.
- Cetingul I. and Geban O., (2005), Understanding of acid-base concepts by using conceptual change approach, Hacettepe Univ. J. Educ., 29, 69–74.
- Chamberlain J. M., Lankaster K., Parson R. and Perkins K. K., (2014), How guidance affects student engagement with an interactive simulation, Chem. Educ. Res. Pract., 15, 628–638.
- Fiechtner S. B. and Davis E. A., (1984), Why some groups fail: a survey of students’ experiences with learning groups, Teach. Higher Educ., 19(3), 58–73.
- Gilbert J. K. and Treagust D., (2009), Introduction: macro, submicro and symbolic representations and the relationship between them: key models in chemical education. Multiple representations in chemical education, The Netherlands: Springer, pp. 1–8.
- Hale-Hanes C., (2015), Promoting student development of models and scientific inquiry skills in acid-base chemistry: an important skill development in preparation for AP chemistry, J. Chem. Educ., 92(8), 1320–1324.
- Hannafin M., West R., and Shepard C., (2009), Re-examining cognition during student-centered, Web-based learning, Ed. Tech. Res. Dev., 57(6), 767.
- Hannan G., (2011), Learning styles and gender, Retrieved from http://www.brainboxx.co.uk/a3_aspects/pages/LSgender.htm 2011.
- Hawkins L. and Phelps A. J., (2013), Virtual laboratory vs. traditional laboratory: which is more effective for teaching electrochemistry? Chem. Educ. Res. Pract., 14(4), 516–523.
- Holzinger A., Kickmeier-Rust M., Wassertheurer S. and Hessinger M. (2009), Learning performance with interactive simulations in medical education: lessons learned from results of learning complex physiological models with the AEMOdynamics SIMulator, Comput. Educ., 52(2), 292–301.
- Howard Y., (2009), User-friendly and learner-friendly courseware design, development, and evaluation, Dissertation submitted, University of Tasmania.
- Johnson D. and Johnson R. T., (1975), Positive interdependence: key to effective cooperation, in Hertz-Lazarowitz R. and Miller N. (ed.), Interaction in cooperative groups: the theoretical anatomy of group learning, New York: Cambridge University Press, pp. 120–141.
- Keller C. J., Finkelstein N. D., Perkins K. K. and Pollock S. J., (2006), Assessing the Effectiveness of a computer simulation in introductory undergraduate environments, PERC Proceedings, AIP Conf. Proc. 883, 121, 2007.
- Kind V., (2004), Beyond Appearances: Students’ misconceptions about basic chemical ideas, 2nd edn, Retrieved from http://www.rsc.org/images/Misconceptions_update_tem18-188603.pdf.
- Kirschner P. A., Sweller J. and Clark R. E., (2006), Why minimal guidance during instruction does not work: an analysis of the failure of constructivist, discovery, problem-based, experiential, and inquiry-based teaching, Educ. Psychol., 41, 75–86.
- Kozma R., and Russell J., (1997), Multimedia and understanding: expert and novice responses to different representations of chemical phenomena, J. Res. Sci. Teach., 34(9), 949–968.
- Kulick J. A. and Kulick C. L. C., (1978), Effects of ability grouping on student achievement, Equity Excellence, 23, 22–30.
- Lamb R., and Annetta L. (2009), A pilot study of online simulations and problem-based learning in a chemistry classroom, J. Virginia Sci. Ed., 3(2), 34–50.
- Lamb R., Annetta L., Meldrum J., and Vallett D. B., (2011), Constructing and validating the science interest survey, Int. J. Sci. Math. Ed., 10(3), 643–668.
- Liao C., (1998), Effects of hypermedia versus traditional instruction on students’ achievement, J. Res. Comput. Educ., 30(A), 341–359.
- Lou Y. P., Abrami P. C. and d’Apollonia S. (2001), Small group and individual learning with technology: a meta-analysis, Rev. Educ. Res., 71(3), 449–521.
- Moore E. B., Carpenter Y., Parson R. and Perkins K. K., (2014), From demonstrations and clicker questions to guided inquiry activities: Resource for integrating PhET simulations into your introductory chemistry course, American Chemical Society Division of Chemical Education, Committee on Computers in Chemistry Education, Fall Newsletter.
- Mostmans L., Vieugels C. and Bannier S., (2012), Raise your hands or hands-on? The role of computer-supported collaborative learning in simulating intercreativity in education, Educ. Tech. Soc., 15(4), 104–113.
- Phielix C., Prins F. J. and Kirschner P. A. (2010), Awareness of group performance in a CSCL-environment: effects of peer feedback and reflection. Comput. Hum. Behav., 26(2), 151–161.
- Rodic D., Roncevic T., and Segedinac M., (2018), The accuracy of Macro-Submicro-Symbolic language of future chemistry teachers, Acta Chim. Slov., 65(2), 394–400.
- Roll I., Lee N. and Briseno A., (2014), Students’ adaptation and transfer of strategies across levels of scaffolding in an exploratory environment. in proceedings of the International Conference on Intelligent Tutoring Systems, Honolulu, HI.
- Smetana L. K. and Bell R. L., (2012), Computer simulations to support science instruction and learning: a critical review of the literature, Int. J. Sci. Educ., 34(9), 1337–1370.
- Smith K., and Villarreal S., (2015), A reply to “Reinterpretation of students’ ideas when reasoning about particle model illustrations. A response to “Using animations in identifying general chemistry students’ misconceptions and evaluating their knowledge transfer relating to particle position in physical changes” by Smith and Villarreal, Chem. Educ. Res. Pract., 16(3), 701.
- Stephens A. L. and Clement J. J., (2015), Use of physics simulations in whole class and small group settings: comparative case studies, Comp. Educ., 86, 137–156.
- Stieff M. and Wilensky U., (2003). Connected Chemistry–Incorporating interactive simulations into the chemistry classroom, J. Sci. Educ. Technol., 12, 285–302.
- Volman M. and van Eck E., (2001), Gender equity and information technology in education: the second decade, Rev. Educ. Res.,71(4), 613–634.
- Vygotsky L. S., (1978), Mind in society: the development of higher psychological processes, Cambridge, MA: Harvard University Press.
- Watson S., (2019), Virtual chemistry: using the PhET pH scale simulation and the 5E model to enhance students’ pH understanding, Sci. Teach., 87(2), 25–30.
- Wieman C. E., Adams W. K., Loeblein P. and Perkins K. K., (2010), Teaching physics using PhET simulations, Phys. Teach., 48(4), 225–227.
Footnote |
† Electronic supplementary information (ESI) available: See DOI: 10.1039/c9rp00235a |
|
This journal is © The Royal Society of Chemistry 2020 |