Development and evaluation of novel science and chemistry identity measures
Received
2nd October 2019
, Accepted 1st April 2020
First published on 6th April 2020
Abstract
Identity has been proposed as a mechanism to increase persistence within Science, Technology, Engineering and Mathematics (STEM) education programs. To assess the impact of identity on STEM persistence, measures that produce valid and reliable data within a given STEM discipline need to be employed. Therefore, this study developed and evaluated the functioning of science and chemistry identity measures in the context of university-level chemistry courses. The developed measures were administered to students enrolled in general and organic chemistry courses at four universities across the United States. Validity and reliability evidence for the data provided by the novel measures was supported using confirmatory factor analysis and McDonald's omega. Additionally, two competing structural equation models (SEMs), designed to explore the relations between mastery experiences, verbal persuasion, situational interest, and science or chemistry identity, were tested and compared to previously reported results. Both SEMs produced acceptable data-model fit, therefore a superior model was chosen based on theoretical support. Within both SEMs, the direct pathway (relation) between mastery experiences and identity was nonsignificant. The more supported model proposed that the relation was indirect and facilitated through verbal persuasion and situational interest. While the indirect relation was supported in both courses, the predominate pathway varied by course. Limitations of the science identity measure, recommendations for future use of the Measure of Chemistry Identity (MoChI), and suggestions for the facilitation of positive identity formation within chemistry classrooms are discussed.
Introduction
In 2012, it was reported that less than 40% of students entering college with the intention to major in STEM actually pursue and obtain a STEM degree (President's Council of Advisors on Science and Technology, 2012). One of the suggestions proposed by the council to aid in retention, was to focus on changes within learning environments, such as active learning. One proposed mechanism by which learning environments can increase student retention is through identity (Chang et al., 2011; Estrada et al., 2011; Graham et al., 2013; Shedlosky-Shoemaker and Fautch, 2015; Flowers and Banda, 2016). Within this study, identity is defined as being recognized as a certain “type of person” in a specific context (Gee, 2000). Therefore, science identity is conceptualized as being recognized as a “science person” in a science context, such as a classroom. Chemistry identity is similarly conceptualized as being recognized as a “chemistry person” in a chemistry context. In order for educators and education researchers to be able to evaluate the impacts of a learning environment on STEM student identity, measures of identity that provide valid and reliable data (Furr and Bacharach, 2008) need to be available. While there are several measures of various STEM identities available in the literature (Hazari et al., 2010; Cass et al., 2011; Chemers et al., 2011; Estrada et al., 2011; Godwin et al., 2013; Stets et al., 2017; Vincent-Ruz and Schunn, 2018), they have not been operationalized specifically to identity as a “science or chemistry person” or are operationalized to disciplines other than chemistry. Therefore, to make such an assessment instrument available to the chemistry education community, a new instrument must be specifically designed or a prior instrument must be realigned and tested within the new context.
When an assessment instrument is developed to measure a psychological attribute, such as identity, the data provided by that instrument needs to show evidence of validity and reliability, which account for systematic and random error, respectively. Establishing these evidences allows an educator or researcher with data to support the meaning of the results and subsequent inferences drawn from them. Validity evidence can be provided through multiple sources including content validity, structural validity, response process validity, and relations with other variables (Furr and Bacharach, 2008). While it is always necessary to provide evidence of data validity, the sources should match the intended use of a measure (American Educational Research Association et al., 2014). Reliability evidence can be provided through various means including test–retest and single administration reliability estimates such as alpha and omega (Komperda et al., 2018a, 2018b) and should be provided every time an instrument is used within a new sample. It is crucial that measures shown to produce valid and reliable data are used in education studies so that practitioners can make the most evidence-based decisions regarding their classroom instruction.
To design a STEM identity measure, each construct involved in identity formation needs to be framed by an appropriate theory and well defined within that theory. These provide the basis for content and structural validity (Furr and Bacharach, 2008). Carlone and Johnson (2007) described a science identity framework consisting of three sub-constructs: recognition, performance, and competence (Fig. 1A). To operationalize identity within physics, the physics identity framework (Hazari et al., 2010) built upon and modified the science identity framework and described three sub-constructs of physics identity: interest, recognition, and performance/competence (Fig. 1B). Hosbein and Barbera (2020) built upon the physics identity framework to operationalize science and chemistry identity, aligning the sub-constructs of identity with mindset, situational interest, verbal persuasion, vicarious experiences, and mastery experiences. While alignment of the physics identity framework to science and chemistry identity has been explored through qualitative methods (Hosbein and Barbera, 2020), the newly aligned sub-constructs have not been quantitatively investigated.
Theoretical framework
The constructs of interest, recognition, and performance/competence have been hypothesized to play a role in identity development within the physics identity framework (Fig. 1B). Their relations have been explored in prior studies through structural equation modeling (SEM) for math (Cribbs et al., 2015), physics (Godwin et al., 2013), and science (Godwin et al., 2013) identities. SEM is a family of related measurement methods that can provide information about causal inference. Testable models are constructed from a priori relations grounded in theory. While SEM is a method of causal inference, it does not provide an absolute model of causal relations between variables. Instead, it can be used to provide support for a theory described in the literature and the causal relations described by that theory (Kline, 2016).
The sub-constructs of physics identity have been used within an SEM framework to explore relations between performance/competence, recognition, and interest with an identity indicator item. This identity indicator consists of a single item, “I see myself as a [
] person”, where the brackets have been replaced with discipline terms such as physics (Hazari et al., 2010; Godwin et al., 2016), math (Cass et al., 2011; Cribbs et al., 2015), and science (Godwin et al., 2013). Models that have been previously tested through SEM are provided in Fig. 2, where an oval represents a latent variable (i.e., not directly measured), a rectangle represents a measured variable (such as a single item), a curved line with a double-headed arrow represents a correlational relation, and a straight line with a single arrow represents a causal relation. A baseline model (Fig. 2A) was originally tested with performance/competence, recognition, and interest correlated to each other and each predicting identity. Alternatively, Cribbs et al. (2015) hypothesized that “competency beliefs (i.e., performance/competence) might precede and facilitate other perceptions that explain an individual's identity development (p. 1056).” To test this hypothesis, an alternative model (Fig. 2B) was tested with an indirect relation between performance/competence and identity, facilitated through interest and recognition (Cribbs et al., 2015). This alternative model had improved data-model fit when compared to the baseline model and has been used in subsequent studies (Godwin et al., 2016; Cheng et al., 2018).
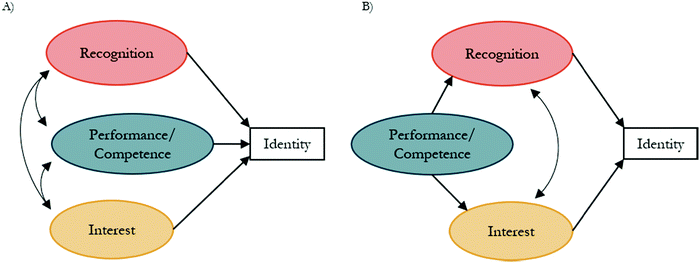 |
| Fig. 2 (A) Baseline and (B) alternative models with proposed relations between recognition, performance/competence, interest, and identity based on the physics identity framework. | |
In a recent qualitative study, Hosbein and Barbera (2020) proposed alignment between the constructs described in the physics identity framework and the constructs of mastery experiences, verbal persuasion, and situational interest (see color coded categories in Fig. 1B and C). Support for these constructs and their relations to the alternative model lie within the theories of Social Cognitive Theory (SCT) (Bandura and National Inst. of Mental Health, 1986), situational interest (Hidi and Renninger, 2006), and the science identity theory as proposed by Carlone and Johnson (2007). Within SCT, “When people aim for master valued levels of performance, they experience a sense of satisfaction (Locke et al., 1970). The satisfactions derived from goal attainments foster intrinsic interest (Bandura and National Inst. of Mental Health, 1986).” This statement provides support that one's mastery experiences may precede their interest. Additionally, within the four-phase theory of interest (Renninger and Hidi, 2011), situational interest has been shown to be marginally impacted by an individual's knowledge and values, providing further support that mastery experiences (equated to knowledge in this case) may precede interest. The relation of interest with verbal persuasion is indirectly described within SCT, “…interest grows from satisfactions derived from fulfilling challenging standards and from self-percepts of efficacy gained through accomplishments and other sources of efficacy information (Bandura and National Inst. of Mental Health, 1986).” This suggests that verbal persuasion could precede interest, however, the relation is not explicitly described and could be correlational. This description within SCT also provides further support of the directional relation between mastery experiences and interest. When describing the relation between verbal persuasion and mastery experiences, directionality is not specified within SCT, however, there is support for directionality within the context of science identity theory. An indirect effect of mastery experiences on identity through verbal persuasion is supported in the description of identity by Carlone and Johnson (2007); a person performs tasks that illustrate their competence in a way that an individual is recognized by others as a credible science person. While some support exists for directional relations between constructs, some relations are not explicitly described as directional. Therefore, it is unclear whether the constructs share correlational, or directional relations. Hence, both the baseline and alternative models (Fig. 2A and B) will be modified to include mastery experiences, verbal persuasion, and situational interest and the relations tested.
Purpose and rationale for the study
The first aim of this study was to provide further validity evidence of the alignment between the sub-constructs within the physics identity framework (Fig. 1B) and the science and chemistry identity framework (Fig. 1C). The alignment between the sub-constructs of identity have been previously explored qualitatively (Hosbein and Barbera, 2020). To further support the alignment, their quantitative relations need to be investigated and compared to the previously explored relations of the physics identity framework (Fig. 2). The second aim of this study was to interpret the modeled relations between mastery experience, verbal persuasion, situational interest and identity. By modeling these relations, we can further understand how these constructs contribute to the formation of science or chemistry identity. These two aims will aid in the creation of an assessment tool that practitioners can use to study the impacts of classroom practices, such as active learning, on the formation of identity.
While science identity has been measured on undergraduate populations, it may be more appropriate to target discipline-specific identities when focusing on higher education classroom environments. Affective measures using science and discipline-specific wording have been shown to function differently depending on wording and class type (Glynn et al., 2011; Salta and Koulougliotis, 2015; Komperda et al., 2018a). While the minor wording change of “science” to “chemistry” may seem insignificant, validity evidence is required to support that the measure functions equally in both wording versions. Additionally, exploring any changes in science or chemistry identity as a result of changes in classroom practice is dependent on an instrument that has been shown to function within each wording type and environment under study. Therefore, both science and chemistry identity measures were used within this study. Additional support for the interpretation of the data provided by the measures comes from the use of cognitive interviews to establish evidence of response process validity.
The two aims were carried out through the following research questions:
(1) To what extent do the modeled relations of the physics identity framework align with the science and chemistry identity framework?
(2) What are the relations between mastery experiences, verbal persuasion, situational interest and a
(a) science identity indicator?
(b) chemistry identity indicator?
Methods
Survey participants
After obtaining institutional review board (IRB) approval for the study, chemistry instructors at four different United States universities were contacted to aid in student recruitment. This convenience sample of instructors were known to the authors and selected based on the size and type of course they taught. The recruitment sample consisted of students enrolled in undergraduate chemistry courses at two Northwestern universities, one Southwestern university, and one Midwestern university, selected through convenience sampling. To sample a range of student levels (e.g., by major and year in degree), the selected courses included organic and general chemistry targeted toward science majors. A total of 855 organic and 1311 general chemistry students were recruited for participation in the study. Demographics collected included age, race/ethnicity, and gender. Students were offered a small amount of extra credit at the discretion of the instructor for participation in the surveys.
Instrument scales
Mastery experiences and verbal persuasion.
The mastery experience and verbal persuasion scales were adapted from the Sources of Middle School Mathematics Self-Efficacy Scale (Usher and Pajares, 2009). The instrument was developed in response to the lack of a measure targeted toward the four sources of self-efficacy (mastery experiences, verbal persuasion, vicarious experiences, and physiological state) in middle school mathematics. While this instrument also contained a vicarious experiences scale, a construct shown to align with recognition (Hosbein and Barbera, 2020), it was not used because vicarious experiences is an indirect form of recognition and therefore verbal persuasion was more appropriately aligned with the perception of recognition for the purpose of this study. Items for the four sources were developed according to SCT (Bandura and National Inst. of Mental Health, 1986), which encompasses the theory of self-efficacy. Scales aligned with these sources were iteratively developed over three rounds of data collection and psychometric analysis. Although the measure was tailored in wording for middle school students, the theory was rooted in SCT (with items reviewed by Bandura himself) and items on the measure mirrored already existing sources of self-efficacy measures developed in a college setting (Lent et al., 1996; Fencl and Scheel, 2003). For this reason, as well as the support provided by the psychometric analysis performed on the measure, the verbal persuasion and mastery experience subscales from the Sources of Middle School Mathematics Self-Efficacy Scale were chosen. Each scale contained six items on a six-point Likert-type response scale ranging from definitely false to definitely true.
Situational interest.
The initial and maintained interest scales developed by Ferrell and Barbera (2015) were used in this study to measure situational interest. The two measures were originally operationalized in psychology (Harackiewicz et al., 2008) and adapted to a general chemistry context (Ferrell and Barbera, 2015). Both initial and maintained interest measures were chosen in order to capture the interest of students at the beginning and end of a course. Both measures contained items from two constructs: feeling- and value-related interest (Schiefele, 1991). Feeling-related interest items were tied to emotional arousal and value-related items were tied to importance/utility. The initial interest measure contained seven items; four feeling- and three value-related interest items. The maintained interest measure contained eight items; four feeling- and four value-related interest items. All items were on five-point Likert-scale ranging from strongly disagree to strongly agree with a “neutral” response.
Scale modifications.
Items on all scales were duplicated to create separate “science” and “chemistry” versions. Items that did not use either phrase were not duplicated. Wording changes were made to the items on each scale, as needed, to reflect the constructs of science and chemistry, specifically in an undergraduate course setting. For example, the mastery experience and verbal persuasion scales were originally operationalized for middle school mathematics. To modify these items, the word “math” was modified to “science” and “chemistry”. Additionally, items containing words in the context of middle school were changed to reflect undergraduate courses. For example, the item “I got good grades in math on my last report card” was modified to “I have gotten good course grades in [
]”, where the bracket is replaced with chemistry or science to create each version. The initial and maintained interest scales were originally operationalized specifically for general chemistry; therefore, for the purpose of using the scale in multiple undergraduate chemistry courses, items were modified to remove this specificity. For example, the item “I think that what we will study in general chemistry will be important for me to know” was modified to “I think that what we will study in this class will be important for me to know”. All original and corresponding modified items are included in Table 5 within Appendix 1.
In addition to individual item wording modifications, the mastery experience and verbal persuasion response scales were modified from a six-point Likert-type scale to a five-point Likert scale to align with the interest scales, thereby producing a consistent response scale across the entire survey. The change in wording of the original response scale, “definitely false” to “definitely true”, to the Likert response scale “strongly agree” to “strongly disagree” did not change the meaning of any of the item responses after review by the authors. Further evidence for the lack of change was gathered during cognitive interviews with students from the target populations.
Identity indicator.
Identity within this study was conceptualized as “seeing oneself as a science person in a science context” and “seeing oneself as a chemistry person in a chemistry context”. Prior studies that have used the physics identity framework have measured identity using a single indicator item as a holistic measure for modeling purposes (Cass et al., 2011; Godwin et al., 2013; Cribbs et al., 2015; Godwin et al., 2016; Verdín et al., 2018). Therefore, the identity indicator of “I see myself as a [
] person”, where the brackets were replaced with either science or chemistry, was used in this study, for the purpose of modeling the sub-construct relations to science or chemistry identity.
Survey data collection
Survey distribution occurred during the first and last weeks of the selected courses during Fall 2018. Students were recruited by the lead author through an in-class announcement delivered through a recorded video or in person. The survey was hosted on the website Qualtrics and the link to the survey was posted to the respective course websites after each in-class announcement. The first survey (time 1) contained the mastery experiences, verbal persuasion, initial feeling- and value-related interest, and the identity indicator. In the final survey (time 2), the same measures were collected but with the maintained interest scales replacing the initial interest scales. Additionally, demographics were collected at the end of the time 1 survey. Both “science” and “chemistry” versions of each scale and identity indicator were included on the survey with a five-point Likert scale at both time points. Each “science” item was immediately followed by its “chemistry” item counterpart. This was done so that students would directly compare their responses for the chemistry and science wording of the survey. Items that did not use either term only appeared once, as there was not a counter-item. Each of the items and linked pairs were randomized for each student to avoid any item order effects in the data.
Cognitive interview participants
After obtaining IRB approval, a portion of the population who participated in the time 1 survey were selected for cognitive interviews through convenience sampling during the Winter 2019 term at the authors’ home institution of Portland State University. Students from two sections of both general and organic chemistry courses were recruited through an in-class announcement and email. Emails were sent to all students enrolled in the courses for a total of 381 and 536 students in organic and general chemistry, respectively. Students did not receive compensation for their participation.
Cognitive interview data collection
Response process validity is used to provide evidence that the items on an instrument are being interpreted in the intended way by the sample under study (Krosnick and Presser, 2010). To provide this evidence, cognitive interviews are often used (Arjoon et al., 2013). At the beginning of each cognitive interview, students were provided with a copy of the survey items from the verbal persuasion, mastery experiences, initial feeling-related interest, and initial value-related interest scales on a Likert response scale. In addition, demographics, including university status (i.e., undergrad, transfer student, post-bac), gender identity, and race/ethnicity were collected. Only the initial interest scales were provided because the interviews occurred within the first half of the term and therefore maintained interest of the course may not have been formed. Students only saw one wording-type, either science or chemistry. Students read each question aloud and were asked three questions: “What is this item trying to find out from you?”, “Which response would you choose as the right response for you?”, and “Can you explain to me why you chose that response?” Follow up questions were asked for additional clarification as necessary.
Data analysis
Data cleaning.
Only students who completed both time 1 and time 2 measures were included in the dataset. A self-check item was included in the survey that read “Please select ‘disagree’ for this item”. Students who selected a response other than ‘disagree’ were removed from the dataset. Missing data was deleted based on wording type responses. For example, if a student had completed all of the science-worded items at times 1 and 2, but did not complete an item within the chemistry wording at time 1, their chemistry responses from both times 1 and 2 were removed and science responses retained. Responses from the time 1 and time 2 measures were then matched. After matching complete datasets, duplicated responses were removed based on the self-reported name of the respondents. If duplicated responses were present, the first entry was retained.
Descriptive statistics and response patterns.
Student responses to time 1 and time 2 measures were examined between wording and course types. Descriptive statistics including mean, standard deviation, median, range, skew, and kurtosis were computed. Acceptable skew and kurtosis values were between −1 and 1 (Huck, 2012). All descriptive statistics were computed using the statistical software R (Version 3.4.4).
Reliability.
Single-administration reliability is commonly reported using the statistic alpha. While this may be appropriate for certain types of data, omega is more appropriate to report when describing models with unequal item error variances and unequal factor loadings, known as congeneric models (Komperda et al., 2018a, 2018b). All models within this study were evaluated as congeneric models and therefore omega was used to provide a reliability estimate. Omega, like alpha, requires that a scale is unidimensional, therefore it was only calculated for an individual scale if evidence of adequate one-factor CFA data-model fit was obtained. Omega values mirror alpha values in their range from 0 to 1 with higher values indicating higher reliability. When latent variables are included in SEM, it is recommended that their reliability estimates fall above 0.7 (Hancock and Mueller, 2001). Although there are R packages to calculate omega (Komperda et al., 2018b), they do not take into account item error correlations present within the model. Omega values were calculated by hand using eqn (1) to incorporate item error correlations when necessary, | 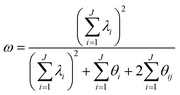 | (1) |
where λi is item factor loading, θi is item variance, and θij is the variance associated with the item error correlation.
Structural equation modeling.
Structural equation modeling occurs in two stages, measurement analysis and structural analysis. The measurement analysis stage was carried out through confirmatory factor analysis (CFA). Details for this stage are contained within Appendix 2. After measurement models were tested through CFA, the relations between constructs were investigated through full SEMs (Fig. 3). The SEMs were built upon the previously proposed models shown in Fig. 2. First, recognition was replaced by verbal persuasion, performance/competence was replaced by mastery experiences, and interest was replaced by both feeling- and value-related interest components. Next, the models were modified to reflect the effects of the measures at time 1 on the corresponding measures at time 2. To do this, autoregressive pathways were included between time 1 and time 2 constructs. Autoregressive pathways account for the direct effect of a variable on itself over time, for example, the effect of mastery experiences at time 1 on mastery experiences at time 2. Finally, maintained interest is a construct that develops over time and therefore, students were not expected to come into the course with maintained interest. To account for this, initial feeling- and value-related interest was measured at time 1 as a control for maintained feeling- and value-related interest measured at time 2. Although the model shown in Fig. 2B does not show a direct pathway between mastery experiences and identity, this pathway was tested (Cribbs et al., 2015) in the original study using the model and was found to be nonsignificant. Therefore, to re-test this pathway in the current study, it was included in the alternative model in Fig. 3.
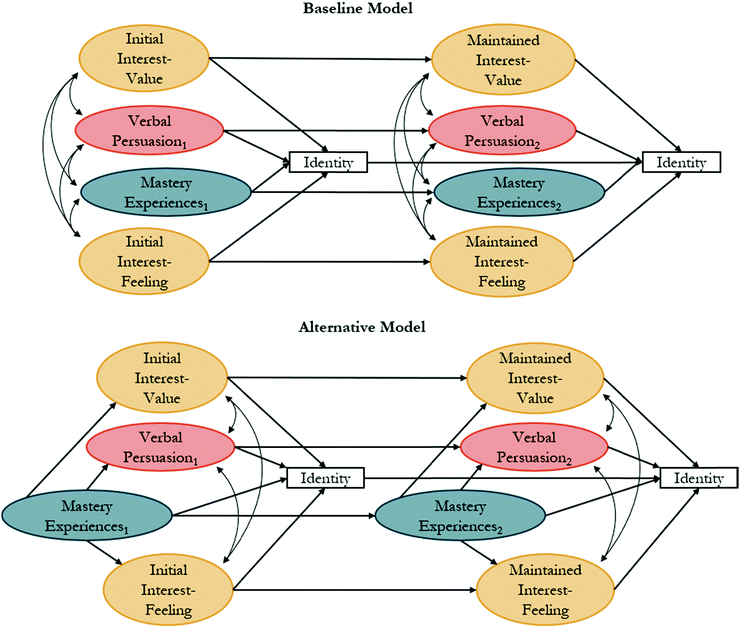 |
| Fig. 3 Proposed baseline and alternative models for SEM. | |
Before testing the full SEMs, it was necessary to show evidence of longitudinal measurement invariance, i.e., that the repeated measures from times 1 and 2 were measuring the same construct. There are various levels of measurement invariance testing. The lowest level of invariance is configural invariance, where the measures within each group (in this case mastery experiences and verbal persuasion) are shown to be invariant without holding any parameter equal between groups (i.e., time 1 and time 2) (Fischer and Karl, 2019). The next highest level of invariance is metric invariance, where measures are shown to be invariant when the factor loadings are held equal between groups. Higher levels of invariance entail holding additional parameters equal between groups, such as intercepts and error terms. It is recommended to at least provide evidence of metric invariance between repeated measures before testing a full SEM model (Newsom, 2015). Therefore, metric invariance testing was completed for the repeated measures of mastery experience and verbal persuasion for both wording types within each course. Models provided evidence of invariance if the change in chi-square was nonsignificant between configural and metric invariant models (Newsom, 2015). After showing evidence of metric invariance for repeated measures, the full SEMs within Fig. 3 were tested for each wording type within each course. To compare SEM parameters between general and organic chemistry courses within each wording type, multi-group metric invariance must be tested (Fischer and Karl, 2019). Multi-group invariance was determined by a nonsignificant change in chi-square (Cheung and Rensvold, 2002). All SEM analyses were carried out through the lavaan package (Version 0.5-23.1097) in R (Version 3.4.4). Normality in the data distributions was assessed to determine the appropriate SEM estimator. After estimation, cutoff values indicating good model fit followed that of the CFA models: CFI ≥ 0.95, RMSEA ≤ 0.06, and SRMR ≤ 0.08.
Cognitive interview analysis.
Cognitive interviews were used to assess the readability and interpretation of survey items for response process validity (Arjoon et al., 2013). Evidence for response process validity had been previously shown in the development of each measure (Usher and Pajares, 2009; Ferrell and Barbera, 2015) but with slightly different samples. However, the process was repeated here as an assurance that the slight wording changes to items and scales did not alter their meaning. Positive evidence was provided when a majority of the students’ interpretation of an item aligned with the intended meaning of the item and their responses aligned with the interpretation of the Likert-scale. If student interpretation misaligned with the intended meaning of the item or the scale, that item was noted and marked for further review by the authors.
Results and discussion
Cognitive interviews
Thirty-three students responded to emails about interview participation. Eleven students were chosen randomly and contacted to schedule an interview, three of which either canceled or did not respond to the scheduling email. A total of eight students participated in a cognitive interview; four from general chemistry and four from organic chemistry. The participants were mostly undergraduates (75%) female (63%), white (63%) or Asian (38%). These numbers were reflective of the wider survey participant characteristics. Within each course type, two students responded to the science worded version of the adapted measures while two students responded to the chemistry version of the adapted measures. No students had issues reading any particular item aloud. Items were then evaluated for appropriate responses. For example, when a student was responding to a verbal persuasion item, it would be expected that they would discuss verbal feedback from others. A specific example included the item “My chemistry instructors have told me that I’m good at chemistry” where a student who disagreed responded, “This is more like if an instructor thinks I’m good enough and singles me out and says ‘hey you’re good at this’… I don’t think that's ever happened.” The student here selected “disagree” as a response and the explanation corresponded to correct scale usage and interpretation of the item. None of the items were found to prompt inappropriate student responses within the interviews, which provided supportive information on the modifications to the response scales for mastery experiences and verbal persuasion. This was expected based on the previous response validity evidence provided by the measures in their development.
Survey data cleaning
Raw response rates for the time 1 survey were 85% and 78% for general and organic chemistry, respectively. Time 2 raw response rates were 66% and 61% for general and organic chemistry, respectively. These rates are based on week-one course enrollments. Therefore, reduction in response rates could be partially due to students dropping the course. Responses in the final dataset included those that passed four criteria: (1) the student was 18 years or older and selected to consent to the research study, (2) the student selected ‘disagree’ for the check item, (3) the student completed all of the chemistry-worded or all of the science-worded items on the survey, and (4) the student completed both time 1 and time 2 surveys. Data cleaning was performed using R (Version 3.4.4). After cleaning, there were 1,127 matched responses; 676 in general chemistry (335 science worded responses and 341 chemistry worded responses) and 451 in organic chemistry (225 science worded responses and 226 chemistry responses).
Survey participant characteristics
General chemistry participants were mostly undergraduates without a prior degree (92%), white (60%) or Asian/Pacific Islander (27%), female (65%), biology majors (38%) or other science majors (33%) with an average age of 21 ± 1.6 years. Organic chemistry participants were mostly undergraduates without a prior degree (84%), white (68%) or Asian/Pacific Islander (22%), female (68%), biology majors (55%) or other science majors (19%), with an average age of 23 ± 1.0 years.
Descriptive statistics and response patterns
Mean, standard deviation, median, minimum, maximum, skew, and kurtosis were computed for all items on the time 1 and time 2 surveys. These descriptive statistics can be found in Tables 6 and 7 within Appendix 3. The means of the items from the time 1 survey ranged from 2.89 to 4.78. Means of the items from the time 2 survey ranged from 2.95 to 4.28. The science-worded items had higher means for all items as compared to the chemistry-worded items. Within the time 1 survey, students utilized the entire magnitude of the Likert scale for all items except for the three items on the initial value-related interest scale, two items on the initial feeling-related interest scale, and one mastery experience item. Students utilized the entire magnitude of the Likert scale for all items on the time 2 survey. Therefore, it was concluded that shortening of the response scale and adding a “neutral” response for the mastery experiences and verbal persuasion scales did not cause response issues. Most items contained skew and kurtosis within the acceptable range of −1 to 1, however, some fell outside of this range with skew values as low as −1.96 and kurtosis values as high as 3.07. Based on the skew and kurtosis values, a maximum likelihood estimator with the Satorra–Bentler adjustment and robust standard errors (Satorra and Bentler, 1994) was used in all models tested with CFA and SEM.
Reliability
Prior to their use in the SEMs, all one-factor congeneric models showed evidence of acceptable data-model fit through cutoff criteria or joint model fit (Table 8). Omega values for each of these unidimensional congeneric models are reported in Table 1, with all values above the recommended cutoff of 0.7 (Hancock and Mueller, 2001). Values ranged from 0.74–0.92 for all scales in both courses for both wording types at times 1 and 2. This range of omega values provided evidence that 74% to 92% of the observed variance was explained by the items measuring each individual construct (the true construct variance) (Komperda et al., 2018b).
Table 1 Omega values for time 1 and time 2 one-factor models
Coursea |
Wordingb |
Time 1 scales |
Time 2 scales |
Initial interest-feeling |
Verbal persuasion |
Mastery experiences |
Maintained interest-feeling |
Verbal persuasion |
Mastery experiences |
GC = general chemistry, OC = organic chemistry.
S = science, C = chemistry. * Omega value includes item error correlation errors.
|
GC |
S |
0.76* |
0.90 |
0.81* |
0.89 |
0.90 |
0.79* |
C |
0.87 |
0.90 |
0.79* |
0.90 |
0.91 |
0.79* |
|
OC |
S |
0.74* |
0.89 |
0.75* |
0.88 |
0.89 |
0.78* |
C |
0.87 |
0.91 |
0.83* |
0.92 |
0.90 |
0.80* |
Structural equation modeling
Longitudinal invariance.
Mastery experiences and verbal persuasion were the only repeated measures. Therefore, their one-factor models, across times 1 and times 2, were tested for metric invariance. Fit indices of the metric invariance models (Table 2) showed RMSEA values above the acceptable cutoff range of 0.06 for the mastery experiences scale with the chemistry wording in general chemistry, the science wording in organic chemistry, and the verbal persuasion scale with science wording in general chemistry and both wording types in organic chemistry. The RMSEA fit index has been shown to provide inflated estimates with smaller sample sizes (N ≤ 200) and low degrees of freedom (df < 50) (Hu and Bentler, 1999; Kenny et al., 2015; Taasoobshirazi and Wang, 2016). The two course types have sample sizes ranging from N = 225–341 and all of the models have df < 50. Therefore, it is possible that the combination of sample size and degrees of freedom limitations may cause issues with the reported RMSEA. Additionally, when the selected indices are not in agreement, joint criteria can be used to assess acceptable fit (Hu and Bentler, 1999; Mueller and Hancock, 2008). Joint criteria include a SRMR ≤ 0.09 with either a CFI ≥ 0.96 or RMSEA ≤ 0.06. All models show evidence of acceptable data-model fit using joint criteria. In addition to fit, a nonsignificant change in chi-square between configural and metric invariance models is required to provide evidence of metric invariance. When the Satorra–Bentler adjustment and robust standard errors are used in model estimation, a simple chi-square change cannot be calculated. Instead, the chi-square change was calculated using an adjusted calculation to account for the alternative estimator (Satorra and Bentler, 2010). Metric invariance, by time, was established for all but two of the one-factor models for both course and wording types according to the nonsignificant chi-square change values (Table 2). The mastery experience scales with both wording types in general chemistry resulted in a significant chi-square difference. Therefore, when the chi-square change is significant between nested models, the change in McDonald's Measure of Centrality (Mc) between the configural and metric invariance models can be used to measure the magnitude of non-invariance. Mc values were calculated using the R package ccpsyc (Version 0.2.1), which takes into account the Satorra–Bentler adjustments. Evidence for invariance is supported with a change in Mc ≤ 0.02 (Cheung and Rensvold, 2002). The change in Mc for mastery experiences with science and chemistry wording were 0.007 and 0.02 respectively. Therefore, these changes in Mc between configural and metric invariance models provided evidence that the amount of non-invariance was small. In addition, the Mc values for the science and chemistry worded metric models were 0.99 and 0.97, respectively. These values lie within the acceptable cutoff range off of Mc ≥ 0.96 (Sivo et al., 2006). Therefore, the data-model fit of the metric invariance model, by time, of mastery experiences with both science and chemistry wording in general chemistry was deemed acceptable. After longitudinal invariance was investigated, full SEMs were tested.
Table 2 Fit indices for metric invariance testing between correlated one-factor models at time 1 and time 2. Acceptable data-model fit indices in bold (CFI ≥ 0.95, RMSEA ≤ 0.06, SRMR ≤ 0.08). Values within models providing acceptable fit through joint criteria (SRMR ≤ 0.09 and CFI ≥ 0.96 or RMSEA ≤ 0.06) are italicized
Coursea |
Scale |
Wordingb |
df |
Δχ2sigc |
χ
2
|
CFI |
RMSEA |
RMSEA [90% CI] |
SRMR |
GC = general chemistry, OC = organic chemistry.
S = science, C = chemistry.
Δχ2sig = significance of Δχ2 between configural and metric invariance models. **p ≤ 0.001, *0.001 ≤ p ≤ 0.05.
|
GC |
Mastery experiences |
S (N = 335) |
11 |
0.00560 |
22.40* |
0.99
|
0.06
|
[0.01, 0.10] |
0.05
|
C (N = 341) |
11 |
<0.001 |
49.83** |
0.96
|
0.11 |
[0.08, 0.15] |
0.08
|
Verbal persuasion |
S (N = 335) |
22 |
0.135 |
50.77** |
0.98
|
0.07 |
[0.04, 0.10] |
0.04
|
C (N = 341) |
22 |
0.0968 |
34.29 |
0.99
|
0.05
|
[NA, 0.08] |
0.04
|
|
OC |
Mastery experiences |
S (N = 225) |
11 |
0.0872 |
22.74* |
0.98
|
0.08 |
[0.02, 0.12] |
0.05
|
C (N = 226) |
11 |
0.579 |
11.95 |
1.00
|
0.00
|
[0.00, 0.08] |
0.04
|
Verbal persuasion |
S (N = 225) |
22 |
0.520 |
45.76* |
0.98
|
0.08 |
[0.04, 0.11] |
0.04
|
C (N = 226) |
22 |
0.500 |
45.76* |
0.98
|
0.09 |
[0.06, 0.12] |
0.05
|
Baseline and alternative model testing.
Mastery experiences, verbal persuasion, and situational interest have been shown to align with the constructs of performance/competence, recognition, and interest as proposed by the physics identity framework (Hosbein and Barbera, 2020). The previously tested baseline and alternative SEMs (Fig. 2) proposed by Cribbs et al. (2015) were modified to explore the relations between mastery experiences, verbal persuasion, and feeling-related initial and maintained interest with the identity indicator (Fig. 4). As a result of the measurement portion of SEM analysis (contained in Appendix 3), the value-related initial and maintained interest scales were excluded from the SEM analysis. Baseline and alternative model fit indices for both wording and course types are contained in Table 3. All models showed acceptable data-model fit based on the cutoff criteria of all three fit indices or joint criteria cutoff ranges.
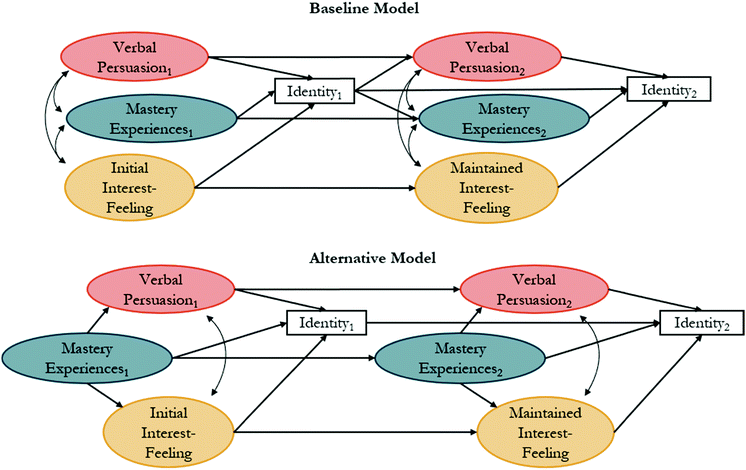 |
| Fig. 4 Baseline and alternative SEMs after the removal of initial and maintained value-related interest based on CFA results. | |
Table 3 Data-model fit for baseline and alternative structural equation models. Acceptable data-model fit indices in bold (CFI ≥ 0.95, RMSEA ≤ 0.06, SRMR ≤ 0.08). Values within models providing acceptable fit through joint criteria (SRMR ≤ 0.09 and CFI ≥ 0.96 or RMSEA ≤ 0.06) are italicized
Model |
Coursea |
Wordingb |
df |
χ
2
|
CFI |
RMSEA |
RMSEA [90% CI] |
SRMR |
GC = general chemistry, OC = organic chemistry.
S = science, C = chemistry. *p < 0.001.
|
Baseline |
GC |
S (N = 335) |
436 |
619* |
0.97
|
0.04
|
[0.03, 0.05] |
0.06
|
C (N = 341) |
437 |
690* |
0.96
|
0.05
|
[0.04, 0.05] |
0.06
|
OC |
S (N = 225) |
436 |
658* |
0.93 |
0.05
|
[0.04, 0.06] |
0.08
|
C (N = 226) |
435 |
666* |
0.95
|
0.05
|
[0.04, 0.06] |
0.07
|
|
Alternative |
GC |
S (N = 335) |
438 |
659* |
0.96
|
0.04
|
[0.04, 0.05] |
0.06
|
C (N = 341) |
437 |
708* |
0.95
|
0.05
|
[0.04, 0.05] |
0.07
|
OC |
S (N = 225) |
438 |
668* |
0.93 |
0.05
|
[0.04, 0.06] |
0.08
|
C (N = 226) |
437 |
674* |
0.95
|
0.05
|
[0.05, 0.06] |
0.07
|
It is possible for data to fit multiple proposed models. When this occurs, theoretical backing can be used to choose the more acceptable model (Kline, 2016). Within the original baseline model (Fig. 2), the direct pathway between performance/competence and identity was found to be nonsignificant (Cribbs et al., 2015). This prompted the development and testing of the alternative model. Although performance/competence was not directly related to identity, it was still thought to play a role in identity formation (Cribbs et al., 2015). While the alternative model shown in Fig. 2 does not show the direct pathway, it was tested within the original study and shown to indeed be a nonsignificant pathway (Cribbs et al., 2015). The alternative model has been re-tested and shown to provide adequate data-model fit in additional studies with multiple wording-types (math, physics, and science) (Godwin et al., 2016; Cheng et al., 2018). The results from the baseline and alternative models in Fig. 4 produced similar results, with the direct effect of mastery experiences on identity being a nonsignificant pathway for all baseline and alternative models. Within the alternative model, the indirect pathway from mastery experiences to identity through recognition was supported by Carlone and Johnson's (2007) science identity theory, which states that a person performs tasks that illustrate their competence in a way that an individual is recognized by others as a credible science person. Additionally, in this model the indirect pathway between mastery experiences and identity through situational interest was supported by both Social Cognitive Theory (SCT) (Bandura and National Inst. of Mental Health, 1986) and situational interest as described by the four-phase model of interest (Hidi and Renninger, 2006), which both describe satisfaction coming from mastery experiences or knowledge acquisition. Therefore, despite the equivalent data-model fit of both the baseline and alternative models, the alternative model was more supported by previous results (Cribbs et al., 2015; Godwin et al., 2016; Cheng et al., 2018) and theoretical backing by SCT (Bandura and National Inst. of Mental Health, 1986), situational interest (Hidi and Renninger, 2006), and science identity theory as proposed by Carlone and Johnson (2007).
Multi-group invariance.
To compare SEM parameters between models, multi-group invariance must be established. This invariance was tested between the alternative models for general and organic chemistry data with the same wording type. The change in chi-square value between configural and metric models as well as the metric model fit indices are listed in Table 4. Both course models showed a nonsignificant change in chi-square as well as acceptable data-model fit. Taken together, these results allowed for comparison of model parameters between courses within the same wording type. Due to the presence of error correlations within the science wording measurement model, that were not present in the chemistry wording model (detailed in Appendix 3), metric invariance could not be evaluated between the models. Therefore, while we can compare trends in the model parameters between wording types, it would not be appropriate to compare the magnitude of the parameters.
Table 4 Fit indices for full SEM model metric invariance testing between courses. Acceptable data-model fit indices in bold (CFI ≥ 0.95, RMSEA ≤ 0.06, SRMR ≤ 0.08)
Model |
Wordinga,b |
df |
Δχ2sigc |
χ
2metric
|
CFI |
RMSEA |
RMSEA [90% CI] |
SRMR |
S = science, C = chemistry.
GC = general chemistry, OC = organic chemistry.
Δχ2sig = significance of Δχ2 between configural and metric invariant models. *p < 0.001.
|
Alternative |
S (NGC = 335, NOC = 225) |
890 |
0.101 |
1415* |
0.95
|
0.05
|
[0.04, 0.05] |
0.07
|
C (NGC = 341, NOC = 226) |
892 |
0.101 |
1350* |
0.95
|
0.05
|
[0.04, 0.05] |
0.07
|
Interpretation of alternative SEMs.
The alternative SEMs under metric invariance conditions are displayed in Fig. 5 (science wording) and 6 (chemistry wording). Regression coefficients (β) and correlations (r) are reported in their standardized form. Standardized values represent the effect on the independent variable caused by a one standard deviation change in the dependent variable. For example, in Fig. 5, βtime
1 = 0.40 for the direct effect of verbal persuasion on the identity indicator in general chemistry. This means that for every standard deviation change in verbal persuasion, the identity indicator will increase by 0.40 of a standard deviation.
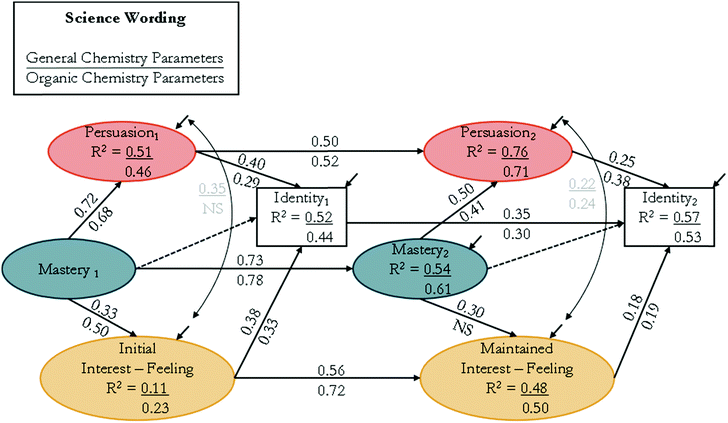 |
| Fig. 5 Metric invariant alternative SEMs for science worded identity constructs for general and organic chemistry. Standardized regression coefficients are presented in black while standardized correlation coefficients are in gray. Nonsignificant pathways (p > 0.05) are denoted with NS or by dotted arrows for nonsignificant pathways present in both courses. | |
Regression pathways and coefficients.
According to the alternative model, mastery experiences influenced identity indirectly through verbal persuasion and feeling-related interest. This indirect effect was supported by a nonsignificant direct effect of mastery experiences on identity for all wording and course types (noted by the dotted arrows in Fig. 5 and 6).
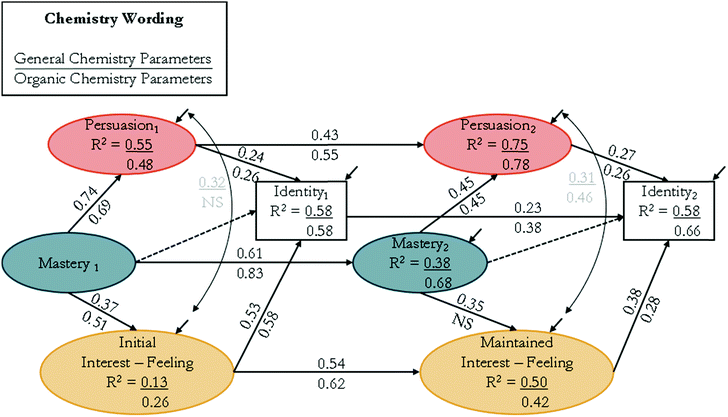 |
| Fig. 6 Metric invariant alternative SEMs for chemistry worded identity constructs for general and organic chemistry. Standardized regression coefficients are presented in black while standardized correlation coefficients are in gray. Nonsignificant pathways (p > 0.05) are denoted with NS or by dotted arrows for nonsignificant pathways present in both courses. | |
Within the science wording (Fig. 5), mastery experiences had a larger direct effect on verbal persuasion, βtime
1 = 0.72 and 0.68, compared to its direct effect on feeling-related initial interest, βtime
1 = 0.33 and 0.50, for general and organic courses, respectively. This was also true at time 2, with the direct effect of mastery experiences on verbal persuasion, βtime
2 = 0.50 and 0.41, compared to its direct effect on maintained feeling-related interest, βtime
2 = 0.30 and nonsignificant (NS), for general and organic courses, respectively. At time 1, verbal persuasion had a similar direct effect on science identity, βtime
1 = 0.40 and 0.29, when compared to the direct effect of initial feeling-related interest on science identity, βtime
1 = 0.38 and 0.33, for both general and organic chemistry, respectively. In contrast, at time 2, the direct effect of verbal persuasion on science identity, βtime
2 = 0.25 and 0.38, was larger than the direct effect of maintained feeling-related interest on science identity at time 2, βtime
2 = 0.18 and 0.19, for general and organic chemistry, respectively. The correlations between verbal persuasion and initial feeling-related interest were r = 0.35 for general chemistry and NS in organic chemistry. In contrast, at time 2, the correlations between verbal persuasion and maintained feeling-related interest were smaller at r = 0.22 for general and became significant at r = 0.24 for organic chemistry.
The SEMs for the chemistry version (Fig. 6) followed similar trends as the science worded version (Fig. 5). At time 1, the direct effect of mastery experiences on verbal persuasion, βtime
1 = 0.74 and 0.69, was larger than the direct effect of mastery experiences on initial feeling-related interest, βtime
1 = 0.37 and 0.51, for general and organic chemistry, respectively. Again, this was true at time 2 for the direct effect of mastery experiences on verbal persuasion, βtime
2 = 0.45 and 0.45, and the direct effect of mastery experiences on maintained feeling-related interest, βtime
2 = 0.35 and NS, for general and organic chemistry, respectively. Mirroring the trend in the science wording, the direct effect of mastery experiences on maintained feeling-related interest was NS for organic chemistry. Different from the science worded version, the direct effect of verbal persuasion on chemistry identity, βtime
1 = 0.24 and 0.26, was smaller than the direct effect of initial feeling-related interest, βtime
1 = 0.53 and 0.58, at time 1 for both general and organic chemistry, respectively. The direct effect of verbal persuasion on chemistry identity at time 2, βtime
2 = 0.27, was also smaller than the effect of maintained feeling-related interest, βtime
2 = 0.38, for general chemistry while the direct effect of verbal persuasion on chemistry identity at time 2, βtime
2 = 0.26, was very similar to the effect of maintained feeling-related interest, βtime
2 = 0.28, for organic chemistry. The correlations between verbal persuasion and initial feeling-related interest were r = 0.32 for general and NS for organic chemistry at time 1. At time 2, the correlations between verbal persuasion and maintained feeling-related interest were r = 0.31 for general and became significant at r = 0.46 for organic chemistry.
The larger direct effect of mastery experiences on verbal persuasion, as compared to both initial and maintained feeling-related interest, for both course and wording types suggested that when a student does well on mastery experiences, they are more likely to perceive recognition for their success rather than have their feeling-related interest stimulated. For organic chemistry, mastery experiences had a nonsignificant effect on maintained feeling-related interest for both wording types, suggesting that students’ perceived maintained feeling-related interest was not affected by success of mastery experiences. The trend in direct effects of verbal persuasion and feeling-related interest on science identity varied for both wording and course types. Within the science wording at time 1, verbal persuasion had a similar direct effect on science identity as compared to the direct effect of initial feeling-related interest on science identity for both courses. However, at time 2, verbal persuasion had a larger direct effect on science identity as compared to the direct effect of maintained feeling-related interest on science identity for both courses. Within the chemistry wording, the direct effect of verbal persuasion on chemistry identity was smaller than the direct effect of initial feeling-related interest for both courses. At time 2, in general chemistry, verbal persuasion had a smaller direct effect on identity compared to the direct effect of maintained feeling-related interest on identity while in organic chemistry, the direct effects of verbal persuasion and maintained feeling-related interest on chemistry identity were similar.
The auto-regression pathways between repeated measures of mastery experiences, verbal persuasion, and identity displayed a positive predictive relation for both wording and course types. These indicated that, on average, students scored higher on the 5-point Likert-scale on all measures at time 2. Similarly, maintained feeling-related interest was positively predicted by initial feeling-related interest. This suggested that, on average, the initial feeling-related interest of students was stable or increased by the end of the term. Although the auto-regressive pathways suggested an increase in each construct, the direct effect of each construct on identity varied over time for both wording and course type. For the science wording, the direct effect of verbal persuasion on science identity at time 1 was βtime
1 = 0.40 and 0.29 for general and organic chemistry. This same direct effect at time 2 decreased for general chemistry, βtime
2 = 0.25, and increased for organic chemistry, βtime
2 = 0.38. For the chemistry wording, the direct effect of verbal persuasion on chemistry identity at time 1, βtime
1 = 0.24 and 0.26 for general and organic chemistry, stayed stable at time 2, βtime
2 = 0.27 and 0.26. These results suggested that while students are likely to respond more positively at time 2, this did not directly reflect the impact of the sub-constructs on identity at time 2 compared to time 1. The same observation was made for the impact of feeling-related interest constructs on identity over time. While feeling-related interest increased between times 1 and 2, the impact of maintained feeling-related interest on identity was smaller than the impact of initial feeling-related interest on identity for all wording and course types.
Identity variance explained.
The relations among constructs explained a considerable amount of variance within the time 1 and time 2 identity indicators. Within the science wording at time 1, 52% and 44% of variance was explained within general and organic chemistry, respectively as compared to 57% and 53% at time 2 (Fig. 5). Within the chemistry wording at time 1, 58% of variance was explained within both general and organic chemistry as compared to 58% and 66% at time 2 (Fig. 6). While a considerable amount of variance within the identity indicators was accounted for, the amount of unexplained variance suggests that there are other constructs involved in identity (as detected by the identity indicator) that are not being captured with these measures. This was expected, as not all of the sub-constructs proposed to be a part of science or chemistry identity were measured during this study.
Conclusions
To address research question one, baseline and alternative SEMs from the physics identity framework were modified to the science and chemistry identity framework and tested through SEM with both course and wording types across both time 1 and time 2 measures (Fig. 4). Although both models provided adequate data-model fit for all course and wording types, the alternative model was chosen as the most appropriate model based on previous results (Cribbs et al., 2015; Godwin et al., 2016) as well as support from social-cognitive theory (SCT, Bandura and National Inst. of Mental Health, 1986), situational interest (Renninger and Hidi, 2011), and science identity theory (Carlone and Johnson, 2007). Providing evidence that similar relations existed between mastery experiences, verbal persuasion, and situational interest through the alternative model in Fig. 4 provided quantitative support for the alignment between these constructs.
After deciding on the most appropriate model and testing multi-group invariance between general and organic chemistry, the parameters within the alternative SEM model were interpreted to address research question two. A key finding within the alternative SEM model was the relation between mastery experiences and the identity indicators. Mastery experiences was found to have an indirect effect on identity, through verbal persuasion and feeling-related interest. These indirect paths provided evidence that success within the classroom alone may not influence identity formation. Providing positive feedback and facilitating interest after students perform a task successfully may be more meaningful to identity formation. The only exception to the indirect effect was the nonsignificant path between mastery experiences and maintained feeling-related interest for both wording types in organic chemistry. Although maintained feeling-related interest still positively predicted identity, this was not preceded by mastery experiences. This suggested that in these conditions, student success does not influence their maintained feeling-related interest.
The direct effect of verbal persuasion on identity varied between wording and course types. For the science wording, the direct effect of verbal persuasion on science identity at time 2 decreased for general chemistry and increased for organic chemistry as compared to their effects at time 1. At time 1 in general chemistry, verbal persuasion may be reflective of students’ pre-college experiences, where time 2 may be reflective of their first experiences within college. However, when students enter organic chemistry, the measure of time 1 verbal persuasion could be more reflective of college experiences and therefore, the change over time may be more reflective of experiences encountered in college. Another explanation of the varying strengths in the relation between verbal persuasion and identity could be that students enrolled in organic chemistry have previously been successful in general chemistry and may be more likely to have stronger connections between verbal persuasion and identity at the end of the course. For the chemistry wording, the direct effect of verbal persuasion on chemistry identity remained stable between times 1 and 2 for both course types. This suggested that chemistry identity was equally influenced by positive verbal feedback over time in both courses.
Initial feeling-related interest significantly and positively predicted identity for both course and wording types, suggesting that students’ incoming feeling-related interest of science or chemistry courses was reflective of their incoming identity. Additionally, maintained feeling-related interest significantly and positively predicted identity within both course and wording type, but to a lesser extent than the initial measure. This could be due to misalignment between students’ initial interest and course expectations as the course progresses, thereby potentially explaining the decrease in the predictability of identity through maintained feeling-related interest.
While we can compare trends in the model parameters between wording types, it would not be appropriate to compare the magnitude of the parameters. The chemistry- and science-wording data had slightly different SEM model specifications due to the item error correlation for the science wording of the initial feeling-related scales. Due to this difference between models, multi-group invariance between wording types could not be tested. Multi-group invariance is necessary to interpret the magnitude of parameters between groups (Fischer and Karl, 2019).
The alternative SEM model (Fig. 4) described the relations between verbal persuasion, mastery experiences, feeling-related interest, and an identity indicator at two time points. This model has theoretical support through SCT (Bandura and National Inst. of Mental Health, 1986), situational interest (Hidi and Renninger, 2006), and science identity theory (Carlone and Johnson, 2007), and the adequate fit of the data to this model supported the hypothesized relations. The alternative SEM model supported that a person performs tasks that illustrate their competence in a way that an individual is recognized by others as a credible science person (Carlone and Johnson, 2007). It also supported that interest can be facilitated through success in mastery experiences and knowledge acquisition, as noted in SCT (Bandura and National Inst. of Mental Health, 1986) and situational interest theory (Hidi and Renninger, 2006). As there is no explicit directional relation theorized between verbal persuasion and interest, the correlation between the two constructs was supported. Carlone (2012) has stated, “Of course, any boundary-defining attempts leave out other important, equally valid and rigorous, ways to bound the concept. The important thing is to understand the ways one bounds the concept and what is visible and veiled as a result (p. 9).” The constructs of the physics identity framework (Fig. 1B) have been qualitatively shown to align with the constructs of mindset, situational interest, verbal persuasion, vicarious experiences, and mastery experiences (Fig. 1C) (Hosbein and Barbera, 2020). For this study, the constructs of mastery experiences, verbal persuasion, and situational interest were chosen to mimic previous studies (Godwin et al., 2013; Cribbs et al., 2015; Godwin et al., 2016; Cheng et al., 2018) in order to explore their alignment quantitatively. We recognize that the included measures and their relations are not the only constructs involved in identity formation, and that that others, such as vicarious experiences and mindset may help to gain a more holistic view.
Implications for researchers
The end goal of this study was to provide the field of chemistry education with theoretically grounded measures of science and chemistry identity that have shown evidence of providing valid and reliable data. However, there were multiple issues with the science wording in organic chemistry throughout analysis. Under these conditions, the three-factor models of verbal persuasion, mastery experiences, and initial-interest did not show evidence of adequate fit (detailed in Appendix 3). The alternative model under the same conditions (Fig. 5) provided adequate fit through joint criteria but was on the cusp of misfit. In addition, the science wording in the initial- and maintained-interest scales showed evidence of the presence of two separate constructs, potentially due to the differences in responses with items framed in “this class” versus “science”. Given these issues, we do not recommend using the science-worded version of the identity measure until modifications and further studies can be conducted. However, the chemistry worded version, referred to as the Measure of Chemistry Identity (MoChI), did not show issues with model fit and can therefore be used in further studies to explore relations between the sub-constructs of identity within different learning environments. In addition to showing possible relations between verbal persuasion, mastery experiences, and situational interest, the alternative models in Fig. 5 and 6 provided information about identity formation over time. The MoChI could therefore be used in conjunction with identity interventions to measure the change in the sub-constructs of identity and how their relations change in magnitude over time in different classroom environments.
Implications for practitioners
Utilizing SEM in the classroom to study identity may not be practical for some practitioners due to barriers such as small course sizes or lack of training with the analysis method. Despite this, there are valuable takeaways from this research that can be connected to chemistry education practice in order to facilitate positive chemistry identity formation. Through the alternative SEM, we found that mastery experiences are not directly related to chemistry identity, but there is a direct relation from both situational interest and verbal persuasion to chemistry identity. There have been studies that provide evidence that situational interest can be affected by classroom variables such as instructors showing interest or concern for their students, instructors providing evidence of their knowledge of the subject, instructors explaining concepts to students in an understandable manner (Rotgans and Schmidt, 2011), repeated exposure to practice problems designed to improve situational interest (Rotgans and Schmidt, 2017), characteristics of lectures (Quinlan, 2019), and student perceptions of the utility and meaningfulness of interventions (Hunsu et al., 2017). Verbal persuasion can be facilitated through positive feedback to an individual from instructors or peers. However, Bandura (1997) offers a word of caution when it comes to verbal persuasion, stating that any verbal persuasion needs to be within realistic bounds. If an individual is persuaded with too many positive comments and subsequently fails, that persuader will be discredited and unable to effect change. As an instructor, providing realistic feedback to students after participation in a mastery experience such as homework or an exam may provide more impact on identity formation as opposed to providing blanket positive statements to all students. While the results contained in this research study do not provide a complete map of the relations between identity for all undergraduate STEM students, it provides a foundation for practitioners to implement and assess interventions that influence theoretically supported constructs involved in chemistry identity formation.
Limitations
It is important to emphasize that validity and reliability are not properties of an instrument itself. These are properties of the data produced by an instrument (Arjoon et al., 2013; Komperda et al., 2018b). The data produced by the MoChI has been shown to have evidence of validity and reliability. It is of note that the models utilized in this study were modified post hoc. Post hoc model modifications can be data driven and therefore, models should be verified using other samples. To expand the justification for and generalizability of the MoChI and alternative model, cross-validation studies with similar and different populations are warranted (MacCallum et al., 1992). The organic chemistry course type had a small sample sizes of 225 and 226 for the science and chemistry worded versions of the survey. These numbers may not provide enough power to unequivocally confirm model fit for some of the models with small degrees of freedom, therefore these measures should be tested with larger sample sizes. The students who participated in this study saw both science and chemistry worded items on their survey, allowing them to directly compare their responses. To provide further evidence for the functioning of these measures, future distributions should separate the wording types upon administration and re-evaluate the models.
One item on the initial value-related interest scale was not performing well according to its psychometric characteristics with the science wording (detailed in Appendix 3). The consequence of this was removal of both the initial and maintained value-related interest scales. The high mean, skew, and kurtosis of this item suggested a ceiling effect (Salkind, 2010). This should be explored further and the items modified to capture a wider range of the value-related initial interest construct. Another potential cause behind the poor performance of the item was because some items on the scale referred to “science” while others referred to “this class”. Students may not explicitly reference “this class” when responding to an item that contained “science” in it and therefore two unique constructs may have existed within the single scale. This should be explored further and the value-related interest scales edited to only reflect the class of interest.
Within this study, identity was conceptualized in a simplistic way, mimicking previous studies (Cribbs et al., 2015; Godwin et al., 2016; Cheng et al., 2018). Identity as a construct is complex and one item may not fully capture a student's science or chemistry identity. Further research is required to explore the degree to which identity can be represented by a single measure with multiple indicator variables or if this single indicator captures enough of the identity construct.
Finally, SEM does not confirm a “true” model (Mueller and Hancock, 2008). This was evident by the adequate fit of both SEMs (Fig. 4) with the sample data in this study. Therefore, the alternative SEM model should be interpreted as one possible explanation for the relations between identity sub-constructs. Further support of these relations should be provided through interviews that are designed to target the directional relations between constructs. To keep the models in their most simple form for comparison to the models previously used to test the physics identity framework, additional longitudinal relations were not tested in the alternative model (Cribbs et al., 2015). For example, the relation between identity at time 1 and verbal persuasion at time 2. The longitudinal effects of identity on the constructs should be investigated in future studies.
Conflicts of interest
There are no conflicts to declare.
Appendix 1: items
Table 5 Original and revised scale items. Bracketed portion replaced with science or chemistry
Mastery experiences |
Original |
Revised |
I make excellent grades on math tests. |
I get excellent grades on [ ] exams. |
I have always been successful with math. |
I have been successful with [ ] in the past. |
Even when I study very hard, I do poorly in math. |
Even with I study very hard, I do poorly in [ ]. |
I got good grades in math on my last report card. |
I have gotten good course grades in [ ]. |
I do well on math assignments. |
I do well on non-exam [ ] assignments. |
I do well on even the most difficult math assignments. |
I do well on even the most difficult non-exam [ ] assignment. |
Verbal persuasion |
Original |
Revised |
My math teachers have told me that I am good at learning math. |
My [ ] instructors have told me that I am good at [ ]. |
People have told me that I have a talent for math. |
People have told me that I have a talent for [ ]. |
Adults in my family have told me what a good math student I am. |
Someone that is important to me (e.g., a family member, a friend, etc.) has told me what a good [ ] student I am. |
I have been praised for my ability in math. |
I have been praised for my ability in [ ]. |
Other students have told me that I’m good at learning math. |
Other students have told me that I’m good at [ ]. |
My classmates like to work with me in math because they think I’m good at it. |
My classmates or labmates like to work with me in [ ] because they think I’m good at [ ]. |
Initial interest |
Feeling-related |
Original |
Revised |
I am fascinated by chemistry. |
I am fascinated by [ ]. |
I chose to take general chemistry because I’m really interested in the topic. |
I chose to take this class because I’m really interested in the topic. |
I am really excited about taking this class. |
Same |
I am really looking forward to learning more about chemistry. |
I am really looking forward to learning more about [ ]. |
Value-related |
Original |
Revised |
I think the field of chemistry is an important discipline. |
I think [ ] is important. |
I think that what we will study in general chemistry will be important for me to know. |
I think that what we will study in this class will be important for me to know. |
I think that what we will study in general chemistry will be worthwhile for me to know. |
I think that what we will study in this class will be worthwhile for me to know. |
Maintained interest |
Feeling-related |
Original |
Revised |
What we are learning in chemistry class this semester is fascinating to me. |
What we are learning in class is fascinating to me. |
This semester, I really enjoy the material we cover in class. |
I really enjoy the [ ] material we cover in this class. |
I am excited about what we are learning in chemistry class this semester. |
I am excited about what we are learning in this class. |
To be honest, I don’t find the chemistry material we cover in class interesting. |
To be honest, I don’t find the [ ] material we cover in class interesting. |
Maintained interest cont. |
Value-related |
Original |
Revised |
What we are studying in chemistry class is useful for me to know. |
What we are studying in this class is useful for me to know. |
The things we are studying in chemistry this semester are important to me. |
The things we are studying in this class are important to me. |
What we are learning in chemistry this semester is important for my future goals. |
What we are learning in this class is important for my future goals. |
What we are learning in chemistry this semester can be applied to real life. |
What we are learning in this class can be applied to real life. |
Identity item |
Original |
Revised |
I see myself as a [ ] person. |
same |
Table 6 Descriptive statistics for time 1 items by course and wording conditions
Item |
Scalea |
Courseb |
Wordingc |
Mean |
Std dev. |
Median |
Min. |
Max. |
Skew |
Kurtosis |
II-V = initial interest value-related, II-F = initial interest feeling-related, ME = mastery experiences, VP = verbal persuasion.
GC = general chemistry, OC = organic chemistry.
S = science, C = chemistry.
|
I think [ ] is important |
II-V |
GC |
S |
4.73 |
0.47 |
5 |
3 |
5 |
−1.40 |
0.77 |
C |
4.57 |
0.58 |
5 |
2 |
5 |
−1.17 |
1.28 |
OC |
S |
4.78 |
0.47 |
5 |
3 |
5 |
−1.96 |
3.07 |
C |
4.63 |
0.56 |
5 |
3 |
5 |
−1.21 |
0.48 |
|
I think that what we will study in this class will be important for me to know |
II-V |
GC |
S |
4.3 |
0.70 |
4 |
2 |
5 |
−0.84 |
0.82 |
C |
4.28 |
0.70 |
4 |
2 |
5 |
−0.81 |
0.68 |
OC |
S |
4.31 |
0.71 |
4 |
2 |
5 |
−0.82 |
0.48 |
C |
4.30 |
0.72 |
4 |
2 |
5 |
−0.79 |
0.35 |
|
I think that what we will study in this class will be worthwhile for me to know |
II-V |
GC |
S |
4.31 |
0.67 |
4 |
2 |
5 |
−0.69 |
0.32 |
C |
4.31 |
0.67 |
4 |
2 |
5 |
−0.75 |
0.61 |
OC |
S |
4.22 |
0.77 |
4 |
1 |
5 |
−0.97 |
1.48 |
C |
4.20 |
0.78 |
4 |
1 |
5 |
−0.93 |
1.33 |
|
I am fascinated by [ ] |
II-F |
GC |
S |
4.44 |
0.71 |
5 |
2 |
5 |
−1.15 |
0.95 |
C |
3.95 |
0.88 |
4 |
2 |
5 |
−0.54 |
−0.40 |
OC |
S |
4.56 |
0.62 |
5 |
3 |
5 |
−1.06 |
0.05 |
C |
4.11 |
0.85 |
4 |
1 |
5 |
−0.85 |
0.42 |
|
I chose to take this class because I’m really interested in the topic |
II-F |
GC |
S |
3.46 |
1.04 |
4 |
1 |
5 |
−0.19 |
−0.77 |
C |
3.45 |
1.03 |
3 |
1 |
5 |
−0.17 |
−0.76 |
OC |
S |
3.39 |
1.08 |
3 |
1 |
5 |
−0.15 |
−0.84 |
C |
3.43 |
1.05 |
3 |
1 |
5 |
−0.16 |
−0.84 |
|
I am really excited about taking this class |
II-F |
GC |
S |
3.79 |
0.91 |
4 |
1 |
5 |
−0.39 |
−0.40 |
C |
3.78 |
0.92 |
4 |
1 |
5 |
−0.35 |
−0.49 |
OC |
S |
3.79 |
1.07 |
4 |
1 |
5 |
−0.56 |
−0.54 |
C |
3.82 |
1.04 |
4 |
1 |
5 |
−0.54 |
−0.50 |
|
I am really looking forward to learning more about [ ] |
II-F |
GC |
S |
4.39 |
0.63 |
4 |
2 |
5 |
−0.67 |
0.14 |
C |
4.14 |
0.79 |
4 |
1 |
5 |
−0.72 |
0.34 |
OC |
S |
4.52 |
0.58 |
5 |
3 |
5 |
−0.69 |
−0.54 |
C |
4.20 |
0.77 |
4 |
2 |
5 |
−0.71 |
0.06 |
|
I get excellent grades on [ ] exams |
ME |
GC |
S |
3.45 |
0.87 |
4 |
1 |
5 |
−0.33 |
−0.12 |
C |
3.11 |
0.85 |
3 |
1 |
5 |
−0.24 |
−0.06 |
OC |
S |
3.67 |
0.97 |
4 |
1 |
5 |
−0.40 |
−0.47 |
C |
3.37 |
1.10 |
3 |
1 |
5 |
−0.24 |
−0.71 |
|
I have been successful with [ ] in the past |
ME |
GC |
S |
4.14 |
0.73 |
4 |
1 |
5 |
−0.69 |
0.80 |
C |
3.65 |
0.97 |
4 |
1 |
5 |
−0.57 |
−0.08 |
OC |
S |
4.22 |
0.71 |
4 |
1 |
5 |
−0.79 |
1.25 |
C |
3.91 |
0.90 |
4 |
1 |
5 |
−0.85 |
0.76 |
|
Even when I study very hard, I do poorly in [ ] |
ME |
GC |
S |
3.8 |
0.92 |
4 |
1 |
5 |
−0.78 |
0.36 |
C |
3.63 |
0.99 |
4 |
1 |
5 |
−0.70 |
0.08 |
OC |
S |
3.94 |
0.96 |
4 |
1 |
5 |
−1.05 |
0.95 |
C |
3.73 |
1.10 |
4 |
1 |
5 |
−0.65 |
−0.39 |
|
|
|
|
|
|
|
|
|
|
|
I have gotten good course grades in [ ] |
ME |
GC |
S |
4.11 |
0.73 |
4 |
1 |
5 |
−0.73 |
1.03 |
C |
3.63 |
0.94 |
4 |
1 |
5 |
−0.52 |
−0.04 |
OC |
S |
4.24 |
0.73 |
4 |
1 |
5 |
−1.03 |
1.83 |
C |
3.94 |
1.02 |
4 |
1 |
5 |
−0.97 |
0.51 |
|
|
|
|
|
|
|
|
|
|
|
I do well on non-exam [ ] assignments |
ME |
GC |
S |
4.04 |
0.66 |
4 |
2 |
5 |
−0.23 |
−0.08 |
C |
3.89 |
0.72 |
4 |
1 |
5 |
−0.33 |
0.25 |
OC |
S |
4.18 |
0.63 |
4 |
1 |
5 |
−0.59 |
2.03 |
C |
4.04 |
0.69 |
4 |
1 |
5 |
−0.78 |
1.82 |
|
I do well on even the most difficult non-exam [ ] assignments |
ME |
GC |
S |
3.31 |
0.86 |
3 |
1 |
5 |
−0.16 |
−0.02 |
C |
3.18 |
0.86 |
3 |
1 |
5 |
−0.03 |
0.22 |
OC |
S |
3.62 |
0.85 |
4 |
1 |
5 |
−0.58 |
0.24 |
C |
3.46 |
0.85 |
4 |
1 |
5 |
−0.39 |
0.12 |
|
My [ ] instructors have told me that I am good at [ ] |
VP |
GC |
S |
3.3 |
0.94 |
3 |
1 |
5 |
−0.42 |
−0.04 |
C |
3.01 |
0.92 |
3 |
1 |
5 |
−0.24 |
0.16 |
OC |
S |
3.39 |
0.97 |
3 |
1 |
5 |
−0.13 |
−0.48 |
C |
3.14 |
0.97 |
3 |
1 |
5 |
0.01 |
−0.25 |
|
People have told me that I have a talent for [ ] |
VP |
GC |
S |
3.34 |
1.00 |
3 |
1 |
5 |
−0.12 |
−0.73 |
C |
2.89 |
0.91 |
3 |
1 |
5 |
0.13 |
−0.01 |
OC |
S |
3.54 |
0.98 |
4 |
1 |
5 |
−0.28 |
−0.62 |
C |
3.17 |
1.48 |
3 |
1 |
5 |
−0.06 |
0.07 |
|
Someone that is important to me (e.g., a family member, a friend, etc.) has told me what a good [ ] student I am |
VP |
GC |
S |
3.53 |
1.03 |
4 |
1 |
5 |
−0.36 |
−0.65 |
C |
3.11 |
0.96 |
3 |
1 |
5 |
0.07 |
−0.33 |
OC |
S |
3.86 |
0.98 |
4 |
1 |
5 |
−0.69 |
−0.13 |
C |
3.54 |
0.98 |
4 |
1 |
5 |
−0.17 |
−0.79 |
|
I have been praised for my ability in [ ] |
VP |
GC |
S |
3.33 |
0.99 |
3 |
1 |
5 |
−0.38 |
−0.4 |
C |
2.94 |
0.95 |
3 |
1 |
5 |
−0.14 |
−0.48 |
OC |
S |
3.54 |
0.95 |
4 |
1 |
5 |
−0.31 |
−0.23 |
C |
3.24 |
0.97 |
3 |
1 |
5 |
0.01 |
−0.50 |
|
Other students have told me that I’m good at [ ] |
VP |
GC |
S |
3.52 |
0.97 |
4 |
1 |
5 |
−0.28 |
−0.65 |
C |
3.18 |
0.92 |
3 |
1 |
5 |
−0.11 |
−0.25 |
OC |
S |
3.72 |
0.89 |
4 |
1 |
5 |
−0.40 |
−0.37 |
C |
3.54 |
0.92 |
4 |
1 |
5 |
−0.30 |
−0.20 |
|
|
|
|
|
|
|
|
|
|
|
My classmates or labmates like to work with me in [ ] because they think I’m good at [ ] |
VP |
GC |
S |
3.37 |
0.80 |
3 |
1 |
5 |
−0.11 |
0.09 |
C |
3.19 |
0.74 |
3 |
1 |
5 |
−0.01 |
0.51 |
OC |
S |
3.62 |
0.85 |
4 |
1 |
5 |
−0.19 |
−0.16 |
C |
3.45 |
0.86 |
3 |
1 |
5 |
−0.07 |
−0.10 |
|
I see myself as a [ ] person |
Identity |
GC |
S |
3.94 |
0.92 |
4 |
1 |
5 |
−0.66 |
0.00 |
C |
3.15 |
0.96 |
3 |
1 |
5 |
0.07 |
−0.46 |
OC |
S |
4.23 |
0.80 |
4 |
1 |
5 |
−1.17 |
2.21 |
C |
3.28 |
1.03 |
3 |
1 |
5 |
−0.02 |
−0.59 |
Table 7 Descriptive statistics for time 2 items by course and wording conditions
Item |
Scalea |
Courseb |
Wordingc |
Mean |
Std dev. |
Median |
Min. |
Max. |
Skew |
Kurtosis |
MI-V = maintained interest value-related, MI-F = maintained interest feeling-related, ME = mastery experiences, VP = verbal persuasion.
GC = general chemistry, OC = organic chemistry.
S = science, C = chemistry.
|
What we are studying in this class is useful for me to know |
MI-V |
GC |
S |
3.88 |
0.85 |
4 |
1 |
5 |
−0.85 |
1.02 |
C |
3.91 |
0.84 |
4 |
1 |
5 |
−0.88 |
1.16 |
OC |
S |
3.99 |
0.92 |
4 |
1 |
5 |
−0.87 |
0.51 |
C |
4.01 |
0.90 |
4 |
1 |
5 |
−0.88 |
0.63 |
|
The things we are studying in this class are important to me |
MI-V |
GC |
S |
3.74 |
0.95 |
4 |
1 |
5 |
−0.65 |
0.17 |
C |
3.78 |
0.93 |
4 |
1 |
5 |
−0.68 |
0.22 |
OC |
S |
3.85 |
0.95 |
4 |
1 |
5 |
−0.67 |
0.14 |
C |
3.88 |
0.93 |
4 |
1 |
5 |
−0.71 |
0.31 |
|
What we are learning in this class is important for my future goals |
MI-V |
GC |
S |
3.90 |
1.00 |
4 |
1 |
5 |
−0.76 |
−0.02 |
C |
3.94 |
0.98 |
4 |
1 |
5 |
−0.82 |
0.13 |
OC |
S |
4.12 |
0.89 |
4 |
1 |
5 |
−1.23 |
1.95 |
C |
4.12 |
0.90 |
4 |
1 |
5 |
−1.33 |
2.29 |
|
What we are learning in this class can be applied to real life |
MI-V |
GC |
S |
3.81 |
0.89 |
4 |
1 |
5 |
−0.73 |
0.48 |
C |
3.84 |
0.88 |
4 |
1 |
5 |
−0.72 |
0.43 |
OC |
S |
3.95 |
0.91 |
4 |
1 |
5 |
−0.78 |
0.26 |
C |
3.96 |
0.90 |
4 |
1 |
5 |
−0.85 |
0.45 |
|
What we are learning in class is fascinating to me |
MI-F |
GC |
S |
3.59 |
1.02 |
4 |
1 |
5 |
−0.65 |
0.06 |
C |
3.63 |
1.01 |
4 |
1 |
5 |
−0.67 |
−0.56 |
OC |
S |
3.77 |
0.99 |
4 |
1 |
5 |
−0.49 |
0.17 |
C |
3.83 |
0.97 |
4 |
1 |
5 |
−0.53 |
−0.42 |
|
I really enjoy the [ ] material we cover in this class |
MI-F |
GC |
S |
3.73 |
0.89 |
4 |
1 |
5 |
−0.63 |
0.37 |
C |
3.66 |
0.91 |
4 |
1 |
5 |
−0.51 |
0.09 |
OC |
S |
3.88 |
0.89 |
4 |
1 |
5 |
−0.54 |
−0.21 |
C |
3.82 |
0.93 |
4 |
1 |
5 |
−0.70 |
0.27 |
|
I am excited about what we are learning in class |
MI-F |
GC |
S |
3.60 |
0.93 |
4 |
1 |
5 |
−0.38 |
−0.24 |
C |
3.62 |
0.93 |
4 |
1 |
5 |
−0.44 |
−0.12 |
OC |
S |
3.75 |
0.97 |
4 |
1 |
5 |
−0.42 |
−0.56 |
C |
3.79 |
0.94 |
4 |
1 |
5 |
−0.47 |
−0.36 |
|
To be honest, I don’t find the [ ] material we cover in class interesting |
MI-F |
GC |
S |
3.63 |
1.05 |
4 |
1 |
5 |
−0.58 |
−0.33 |
C |
3.57 |
1.10 |
4 |
1 |
5 |
−0.54 |
−0.52 |
OC |
S |
3.95 |
0.94 |
4 |
1 |
5 |
−0.93 |
0.51 |
C |
3.85 |
1.02 |
4 |
1 |
5 |
−0.79 |
0.01 |
|
I get excellent grades on [ ] exams |
ME |
GC |
S |
3.36 |
0.98 |
3 |
1 |
5 |
−0.35 |
−0.33 |
C |
3.02 |
1.10 |
3 |
1 |
5 |
−0.05 |
−0.74 |
OC |
S |
3.61 |
0.93 |
4 |
1 |
5 |
−0.5 |
0.00 |
C |
3.22 |
1.04 |
3 |
1 |
5 |
−0.11 |
−0.56 |
|
I have been successful with [ ] in the past |
ME |
GC |
S |
4.08 |
0.76 |
4 |
1 |
5 |
−0.86 |
1.13 |
C |
3.63 |
0.97 |
4 |
1 |
5 |
−0.54 |
−0.14 |
OC |
S |
4.28 |
0.72 |
4 |
1 |
5 |
−1.12 |
2.24 |
C |
3.93 |
0.93 |
4 |
1 |
5 |
−0.93 |
0.58 |
|
Even when I study very hard, I do poorly in [ ] |
ME |
GC |
S |
3.64 |
1.05 |
4 |
1 |
5 |
−0.67 |
−0.08 |
C |
3.35 |
1.22 |
4 |
1 |
5 |
−0.38 |
−0.88 |
OC |
S |
3.77 |
0.99 |
4 |
1 |
5 |
−1.01 |
0.78 |
C |
3.59 |
1.12 |
4 |
1 |
5 |
−0.65 |
−0.40 |
|
I have gotten good course grades in [ ] |
ME |
GC |
S |
3.96 |
0.80 |
4 |
1 |
5 |
−0.72 |
0.73 |
C |
3.60 |
0.96 |
4 |
1 |
5 |
−0.52 |
−0.21 |
OC |
S |
4.13 |
0.80 |
4 |
1 |
5 |
−1.19 |
2.16 |
C |
3.91 |
0.92 |
4 |
1 |
5 |
−0.87 |
0.63 |
|
I do well on non-exam [ ] assignments |
ME |
GC |
S |
4.08 |
0.73 |
4 |
1 |
5 |
−0.73 |
1.31 |
C |
4.01 |
0.74 |
4 |
1 |
5 |
−0.58 |
0.62 |
OC |
S |
4.17 |
0.70 |
4 |
1 |
5 |
−0.96 |
2.28 |
C |
4.04 |
0.73 |
4 |
1 |
5 |
−0.68 |
1.09 |
|
I do well on even the most difficult non-exam [ ] assignments |
ME |
GC |
S |
3.51 |
0.91 |
4 |
1 |
5 |
−0.38 |
−0.07 |
C |
3.42 |
0.92 |
3 |
1 |
5 |
−0.32 |
−0.09 |
OC |
S |
3.68 |
0.89 |
4 |
1 |
5 |
−0.53 |
0.07 |
C |
3.50 |
0.92 |
4 |
1 |
5 |
−0.34 |
−0.26 |
|
My [ ] instructors have told me that I am good at [ ] |
VP |
GC |
S |
3.20 |
0.96 |
3 |
1 |
5 |
−0.16 |
−0.41 |
C |
2.95 |
0.88 |
3 |
1 |
5 |
0.02 |
0.16 |
OC |
S |
3.30 |
1.05 |
3 |
1 |
5 |
−0.18 |
−0.67 |
C |
3.08 |
0.99 |
3 |
1 |
5 |
0.08 |
−0.37 |
|
People have told me that I have a talent for [ ] |
VP |
GC |
S |
3.36 |
0.95 |
3 |
1 |
5 |
−0.25 |
−0.42 |
C |
2.99 |
0.97 |
3 |
1 |
5 |
0.03 |
−0.23 |
OC |
S |
3.70 |
0.95 |
4 |
1 |
5 |
−0.45 |
−0.56 |
C |
3.30 |
1.00 |
3 |
1 |
5 |
−0.08 |
−0.62 |
|
Someone that is important to me (e.g., a family member, a friend, etc.) has told me what a good [ ] student I am |
VP |
GC |
S |
3.60 |
1.01 |
4 |
1 |
5 |
−0.59 |
−0.28 |
C |
3.21 |
1.00 |
3 |
1 |
5 |
0.04 |
−0.71 |
OC |
S |
3.87 |
0.97 |
4 |
1 |
5 |
−0.72 |
−0.05 |
C |
3.55 |
1.06 |
4 |
1 |
5 |
−0.38 |
−0.59 |
|
I have been praised for my ability in [ ] |
VP |
GC |
S |
3.36 |
1.00 |
3 |
1 |
5 |
−0.31 |
−0.44 |
C |
3.06 |
1.00 |
3 |
1 |
5 |
−0.05 |
−0.38 |
OC |
S |
3.67 |
0.97 |
4 |
1 |
5 |
−0.46 |
−0.40 |
C |
3.33 |
1.00 |
3 |
1 |
5 |
−0.18 |
−0.47 |
|
Other students have told me that I’m good at [ ] |
VP |
GC |
S |
3.50 |
0.97 |
4 |
1 |
5 |
−0.42 |
−0.34 |
C |
3.32 |
1.02 |
3 |
1 |
5 |
−0.22 |
−0.55 |
OC |
S |
3.87 |
0.82 |
4 |
1 |
5 |
−0.73 |
0.99 |
C |
3.59 |
0.93 |
4 |
1 |
5 |
−0.40 |
−0.18 |
|
My classmates or labmates like to work with me in [ ] because they think I’m good at [ ] |
VP |
GC |
S |
3.47 |
0.87 |
3 |
1 |
5 |
−0.16 |
−0.10 |
C |
3.41 |
0.86 |
3 |
1 |
5 |
−0.08 |
−0.07 |
OC |
S |
3.74 |
0.76 |
4 |
1 |
5 |
−0.37 |
0.52 |
C |
3.53 |
0.84 |
4 |
1 |
5 |
−0.19 |
0.04 |
|
I see myself as a [ ] person |
Identity |
GC |
S |
3.85 |
0.97 |
4 |
1 |
5 |
−0.89 |
0.63 |
C |
3.02 |
1.05 |
3 |
1 |
5 |
−0.05 |
−0.56 |
OC |
S |
4.18 |
0.82 |
4 |
1 |
5 |
−1.03 |
1.34 |
C |
3.42 |
1.04 |
3 |
1 |
5 |
−0.17 |
−0.64 |
Table 8 Congeneric data-model fit of one-factor scales
Scale |
Coursea |
Wordingb |
df |
χ
2
|
CFI |
RMSEA |
RMSEA [90% CI] |
SRMR |
GC = general chemistry, OC = organic chemistry.
S = science, C = chemistry. *0.001 ≤ p ≤ 0.05. ‡p ≥ 0.05.
|
Initial feeling-related interest (time 1) |
GC |
S (N = 335) |
1 |
0.333‡ |
1.00
|
0.00
|
[0.00, 0.00] |
0.003
|
C (N = 341) |
2 |
7.63* |
0.99
|
0.10 |
[0.03, 0.19] |
0.02
|
OC |
S (N = 225) |
1 |
0.025‡ |
1.00
|
0.00
|
[0.00, 0.00] |
0.001
|
C (N = 226) |
2 |
5.87* |
0.99
|
0.11 |
[NA, 0.21] |
0.02
|
|
Mastery experiences (time 1) |
GC |
S (N = 335) |
4 |
5.54‡ |
1.00
|
0.04
|
[0.00, 0.11] |
0.02
|
C (N = 341) |
4 |
11.4* |
0.99
|
0.08 |
[0.03, 0.14] |
0.03
|
OC |
S (N = 225) |
4 |
10.0* |
0.98
|
0.09 |
[0.02, 0.16] |
0.04
|
C (N = 226) |
4 |
8.04* |
0.99
|
0.07 |
[0.00, 0.15] |
0.03
|
|
Verbal persuasion (time 1) |
GC |
S (N = 335) |
9 |
27.6* |
0.98
|
0.09 |
[0.06, 0.14] |
0.03
|
C (N = 341) |
9 |
12.2‡ |
1.00
|
0.04
|
[0.00, 0.09] |
0.02
|
OC |
S (N = 225) |
9 |
27.6* |
0.98
|
0.09 |
[0.06, 0.14] |
0.03
|
C (N = 226) |
9 |
18.0* |
0.99
|
0.08 |
[0.02, 0.13] |
0.03
|
|
Maintained feeling-related interest (time 2) |
GC |
S (N = 335) |
2 |
2.40‡ |
1.00
|
0.03
|
[0.00, 0.12] |
0.008
|
C (N = 341) |
2 |
0.480‡ |
1.00
|
0.00
|
[0.00, 0.07] |
0.003
|
OC |
S (N = 225) |
2 |
5.76‡ |
0.99
|
0.10 |
[NA, 0.20] |
0.02
|
C (N = 226) |
2 |
0.738‡ |
1.00
|
0.00
|
[0.00, 0.10] |
0.006
|
|
Mastery experiences (time 2) |
GC |
S (N = 335) |
4 |
3.47‡ |
1.00
|
0.00
|
[0.00, 0.09] |
0.02
|
C (N = 341) |
4 |
4.11‡ |
1.00
|
0.01
|
[0.00, 0.09] |
0.02
|
OC |
S (N = 225) |
4 |
4.08‡ |
1.00
|
0.01
|
[0.00, 0.11] |
0.021
|
C (N = 226) |
4 |
1.17‡ |
1.00
|
0.00
|
[0.00, 0.05] |
0.01
|
|
Verbal persuasion (time 2) |
GC |
S (N = 335) |
9 |
13.6‡ |
1.00
|
0.05
|
[0.00, 0.09] |
0.02
|
C (N = 341) |
9 |
13.3‡ |
0.99
|
0.05
|
[0.00, 0.10] |
0.02
|
OC |
S (N = 225) |
9 |
20.6* |
0.98
|
0.08 |
[0.04, 0.13] |
0.03
|
C (N = 226) |
9 |
27.4* |
0.97
|
0.10 |
[0.06, 0.15] |
0.03
|
Appendix 2: confirmatory factor analysis
Methodology
A structural equation model (SEM) contains two components: measurement and structural. The measurement component of the model consists of the indicator items and scales used to measure latent variables. The structural component of the model consists of the proposed relations between the measured latent variables. Before a full SEM is investigated, the measurement portion of the model needs to be tested (Mueller and Hancock, 2008). This was done through confirmatory factor analysis (CFA). CFA was chosen over exploratory factor analysis (EFA) because the scales used had strong a priori hypotheses for their factor structure (Kline, 2016). CFAs were performed using R (Version 3.4.4) and the lavaan package (Version 0.5-23.1097). One-, two-, and multi-factor measurement models for time 1 and time 2 data were tested independently. One-factor models were used to justify reliability estimates that require unidimensional scales (Komperda et al., 2018a, 2018b) as well as to test measurement invariance over time (Newsom, 2015). Two two-factor models were evaluated (feeling- with value-related interest and mastery experiences with verbal persuasion) to mimic previous analysis of the factor structure of the scales within the literature (Usher and Pajares, 2009; Ferrell and Barbera, 2015), providing additional evidence for their functioning in the new contexts. Multi-factor models were tested to provide support for the full SEM analysis (Mueller and Hancock, 2008). In all models, the science and chemistry worded items were separated and tested. To complete each data set, the unduplicated items (i.e., those that contained the phrase “this class” instead of “science” or “chemistry”) were included in both versions during analysis.
Normality in the data distributions was assessed to determine the appropriate CFA estimator. Continuous data is an assumption of the maximum likelihood estimators and while the Likert-scale technically provides ordinal data, the scale can be thought of as continuous when it contains five or more response options and is approximately normally distributed (Dolan, 1994). In evaluating appropriate data-model fit, three fit indices and a standard set of cutoff values were utilized: CFI ≥ 0.95, RMSEA ≤ 0.06, and SRMR ≤ 0.08 (Hu and Bentler, 1999). Modification indices were used to determine if post-hoc modifications of each model were necessary.
Appendix 3: results
Two-factor models
Eight correlated two-factor CFA models were tested, feeling- with value-related interest and mastery experiences with verbal persuasion for both wording and course types and time 1 and 2. All scales were slightly modified in order to operationalize them to non-specific college chemistry courses. To ensure that the scales were functioning as intended, two-factor models were chosen based on the previous use of the scales (Usher and Pajares, 2009; Ferrell and Barbera, 2015) and to provide an initial check for any measurement error before moving to a multi-factor CFA model containing all scales.
Interest scales
When analyzing the two-factor initial feeling- and value-related interest scales, modification indices suggested error correlations between some of the items present on the time 1 survey. Four of the seven initial interest items contained the phrase “this class”, for example, “I chose to take this class because I’m really interested in the topic.” The remaining three items either contained the word “chemistry” or “science”, for example, “I am really looking forward to learning more about [science].” The modification indices suggested error correlations between the three items containing the word “science”. It is likely that when students read these items, they equated “this class” with “chemistry”, as the survey was given in chemistry courses, but students may not necessarily respond to the item in the context of their class when presented with the “science” wording. Results from the cognitive interviews further supported this modification. An example of a student response to “I think chemistry is important”, included, “I think it's really important because chemistry is used to solve a whole bunch of stuff. And like I don’t know—see how chemical reactions work—figure out how things are made and how things work” which described content that a student would learn within their chemistry course. Alternatively, when students responded to the item “I think science is important”, they mentioned science outside of the classroom, such as,“It makes me think about, ‘is science an important part of the world?’ is it valuable to you, or do you perceive it as valuable to everybody” and, “I think science is one of the most important jobs there is.” Based on the modification indices and qualitative support, the errors of the items containing the word “science” were correlated within the initial interest scales for subsequent models.
With the noted error correlations, the two-factor initial feeling- and value-related interest time 1 survey models had acceptable data-model fit according to cutoff criteria for both wording and course types (Table 9). The initial feeling- and value-related interest two-factor models fell within the joint criteria range for all wording and course types. Fit indices for time 2 maintained feeling- and value-related interest two-factor models suggested adequate fit for all wording and course types (Table 9).
Table 9 Data-model fit for correlated two-factor models with error correlations. Acceptable data-model fit indices in bold (CFI ≥ 0.95, RMSEA ≤ 0.06, SRMR ≤ 0.08). Values within models providing acceptable fit through joint criteria (SRMR ≤ 0.09 and CFI ≥ 0.96 or RMSEA ≤ 0.06) are italicized
Scales |
Coursea |
Wordingb |
df |
χ
2
|
CFI |
RMSEA |
RMSEA [90% CI] |
SRMR |
GC = general chemistry, OC = organic chemistry.
S = science, C = chemistry. *p ≤ 0.001.
|
Initial feeling-and value-related interest (time 1 survey) |
GC |
S (N = 335) |
10 |
30.4* |
0.98
|
0.09 |
[0.05, 0.13] |
0.04
|
C (N = 341) |
13 |
34.5* |
0.98
|
0.08 |
[0.05, 0.11] |
0.04
|
OC |
S (N = 225) |
10 |
6.16 |
1.00
|
0.00
|
[0.00, 0.05] |
0.02
|
C (N = 226) |
13 |
35.7* |
0.97
|
0.10 |
[0.06, 0.14] |
0.04
|
|
Maintained value-and feeling-related interest (time 2 survey) |
GC |
S (N = 335) |
19 |
24.7 |
1.00
|
0.04
|
[0.00, 0.07] |
0.02
|
C (N = 341) |
19 |
33.6 |
0.99
|
0.06
|
[0.02, 0.09] |
0.03
|
OC |
S (N = 225) |
19 |
33.9 |
0.98
|
0.07 |
[0.03, 0.10] |
0.03
|
C (N = 226) |
19 |
23.4 |
1.00
|
0.04
|
[0.00, 0.08] |
0.03
|
Although both time 1 and time 2 interest models provided evidence of adequate fit, there were issues with localized fit for one item on the initial value-related interest scale. The item “I think [
] is important.” had low loading values of 0.41 and 0.27 for the science wording in the general and organic chemistry courses, respectively. In addition to the low loadings, the item had high means, skew, and kurtosis across both wording and course types (4.57 to 4.78, −1.96 to −1.17, and 0.77 to 3.07, respectively). The high means, skew, and kurtosis of this item mirror high values previously reported for all items on the value-related initial interest scale before modification (Ferrell, 2016). This suggested that a ceiling effect (Salkind, 2010) exists within the scale and the high values are an artifact of the scale itself i.e., there is not enough variation of the construct being captured in student responses. Due to these issues, the item was removed. However, after discarding the item, only two items remained on the initial value-related interest scale, which created an issue for further analysis using the scale as three or more items per factor are required for CFA modeling (Kline, 2016). Therefore, the initial value-related interest scale was removed from further analysis. Additionally, the maintained value-related interest scale was removed from further analysis as the control of initial value-related interest was no longer available. The initial and maintained feeling-related interest scales were then tested as one-factor CFA models for both wording and course types. These analyses were to ensure that the one-factor scale would function without the value-related interest component. Both one-factor models provided evidence of adequate fit according to cutoff criteria or joint cutoff criteria for both wording and course types (Table 8).
Mastery experiences and verbal persuasion.
When testing the correlated two-factor mastery experiences and verbal persuasion model, modification indices suggested error correlations between two pairs of mastery experience items. The first error correlation was between the item “I have been successful with [
] in the past” and “I have gotten good course grades in [
]” and the second error correlation was suggested between the items, “I do well on non-exam [
] assignments” and “I do well on even the most difficult non-exam [
] assignments”. In the former pair, both items referenced past experiences with science or chemistry at the course level while the context of the remaining items inquired about present experiences at the exam level. The latter pair of items were very similarly worded and deemed redundant. The item “I do well on non-exam [
] assignments” was removed from further analysis based on lower factor loadings within both wording and course types as compared to the alternative item. Item errors were correlated for the first pair and the two-factor CFAs were re-run. All subsequent data-model fit values (Table 10) suggested adequate fit when using selected cutoff criteria or joint criteria.
Table 10 Data-model fit for correlated two-factor models with error correlation. Acceptable data-model fit indices in bold (CFI ≥ 0.95, RMSEA ≤ 0.06, SRMR ≤ 0.08). Values within models providing acceptable fit through joint criteria (SRMR ≤ 0.09 and CFI ≥ 0.96 or RMSEA ≤ 0.06) are italicized
Scales |
Coursea |
Wordingb |
df |
χ
2
|
CFI |
RMSEA |
RMSEA [90% CI] |
SRMR |
GC = general chemistry, OC = organic chemistry.
S = science, C = chemistry. *p ≤ 0.001.
|
Mastery experiences and verbal persuasion (time 1 survey) |
GC |
S (N = 335) |
42 |
63.6 |
0.99
|
0.05
|
[0.02, 0.03] |
0.03
|
C (N = 341) |
42 |
65.7 |
0.99
|
0.05
|
[0.02, 0.07] |
0.04
|
OC |
S (N = 225) |
42 |
76.2* |
0.96
|
0.07 |
[0.04, 0.09] |
0.05
|
C (N = 226) |
42 |
92.5* |
0.96
|
0.08 |
[0.06, 0.10] |
0.06
|
|
Mastery experiences and verbal persuasion (time 2 survey) |
GC |
S (N = 335) |
42 |
59.5 |
0.99
|
0.04
|
[0.01, 0.06] |
0.03
|
C (N = 341) |
42 |
94.9* |
0.96
|
0.07 |
[0.05, 0.09] |
0.06
|
OC |
S (N = 225) |
42 |
56.8 |
0.98
|
0.04
|
[0.00, 0.07] |
0.04
|
C (N = 226) |
42 |
76.1* |
0.97
|
0.07 |
[0.04, 0.09] |
0.05
|
Three-factor models
After providing evidence for acceptable fit for the correlated two-factor models of mastery experiences and verbal persuasion and single-factor models of feeling-related interest, correlated three-factor models were tested to ensure the full measurement model provided adequate fit before moving to full SEMs. Any item error correlations present within previous models were retained. Fit indices for the correlated three-factor models (shown in Table 11) suggested adequate model fit for all wording and course types with the exception of the time 1 model consisting of the science wording within organic chemistry. The fit indices of 0.94, 0.07, and 0.09 for the CFI, RMSEA, and SRMR were just shy of the cutoff values. This was taken into consideration during further analysis as this misfit of the measurement model could contribute to misfit during SEM. Details including factor loadings and error terms for all correlated three-factor models are included in Fig. 7–10.
Table 11 Data-model fit for correlated three-factor measurement models consisting of feeling-related initial or maintained interest, verbal persuasion, and mastery experiences. Acceptable data-model fit indices in bold (CFI ≥ 0.95, RMSEA ≤ 0.06, SRMR ≤ 0.08). Values within models providing acceptable fit through joint criteria (SRMR ≤ 0.09 and CFI ≥ 0.96 or RMSEA ≤ 0.06) are italicized
Survey |
Coursea |
Wordingb |
df |
χ
2
|
CFI |
RMSEA |
RMSEA [90% CI] |
SRMR |
GC = general chemistry, OC = organic chemistry.
S = science, C = chemistry. *p ≤ 0.001.
|
Time 1 |
GC |
S (N = 335) |
85 |
142* |
0.97
|
0.05
|
[0.03, 0.06] |
0.06
|
C (N = 341) |
86 |
149* |
0.97
|
0.05
|
[0.04, 0.07] |
0.05
|
OC |
S (N = 225) |
85 |
157* |
0.94 |
0.07 |
[0.05, 0.08] |
0.09 |
C (N = 226) |
86 |
154* |
0.96
|
0.07 |
[0.05, 0.08] |
0.06
|
|
Time 2 |
GC |
S (N= 335) |
86 |
115 |
0.99
|
0.04
|
[0.02, 0.05] |
0.04
|
C (N = 341) |
86 |
168* |
0.97
|
0.06
|
[0.05, 0.07] |
0.06
|
OC |
S (N = 225) |
86 |
127 |
0.97
|
0.05
|
[0.03, 0.07] |
0.05
|
C (N = 226) |
86 |
151* |
0.96
|
0.06
|
[0.05, 0.08] |
0.05
|
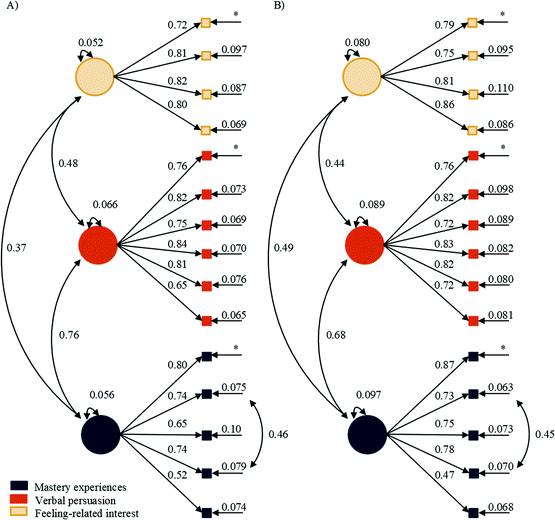 |
| Fig. 7 Localized estimates for time 1, chemistry worded, three-factor correlated models within (A) general and (B) organic chemistry. * indicates the reference variable for the model. | |
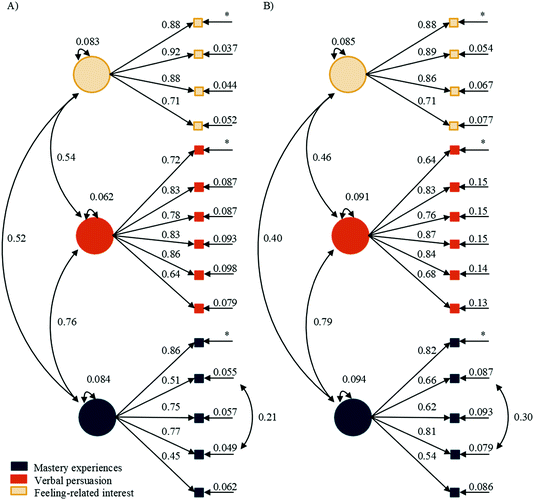 |
| Fig. 8 Localized estimates for time 2, chemistry worded, three-factor correlated models within (A) general and (B) organic chemistry. * indicates the reference variable for the model. | |
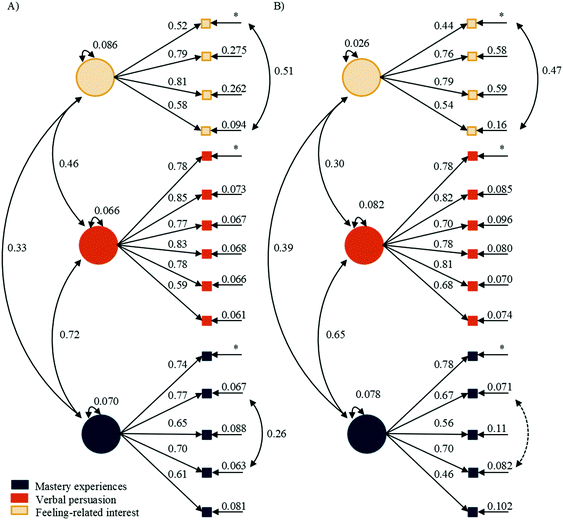 |
| Fig. 9 Localized estimates for time 1, science worded, three-factor correlated models within (A) general and (B) organic chemistry. Dashed line indicates non-significant path. * indicates the reference variable for the model. | |
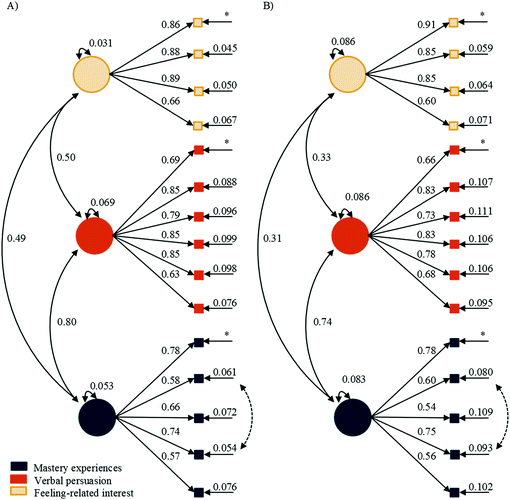 |
| Fig. 10 Localized estimates for time 2, science worded, three-factor correlated models within (A) general and (B) organic chemistry. Dashed lines indicate non-significant paths. * indicates the reference variable for the model. | |
References
- American Educational Research Association, American Psychological Association and National Council on Measurement in Education, (2014), Standards for Educational and Psychological Testing, Washington, DC: American Educational Research Association.
- Arjoon J. A., Xu X. and Lewis J. E., (2013), Understanding the State of the Art for Measurement in Chemistry Education Research: Examining the Psychometric Evidence, J. Chem. Educ., 90(5), 536–545.
- Bandura A. and National Inst. of Mental Health, (1986), Prentice-Hall series in social learning theory: Social foundations of thought and action: A social cognitive theory, Englewood Cliffs, NJ: Prentice-Hall, Inc.
- Bandura A., (1997), Self-efficacy: The exercise of control, New York, NY: Worth Publishers.
- Carlone H. B., (2012), Identity Construction and Science Education Research: Learning, Teaching, and Being in Multiple Contexts, in Varelas M. (ed.), Rotterdam, Netherlands: SensePublishers, pp. 9–25.
- Carlone H. B. and Johnson A., (2007), Understanding the science experiences of successful women of color: Science identity as an analytic lens, J. Res. Sci. Teach., 44(8), 1187–1218.
- Cass C. A. P., Hazari Z., Cribbs J., Sadler P. M. and Sonnert G., (2011), Examining the impact of mathematics identity on the choice of engineering careers for male and female students, Rapid City, SD.
- Chang M. J., Eagan M. K., Lin M. H. and Hurtado S., (2011), Considering the Impact of Racial Stigmas and Science Identity: Persistence Among Biomedical and Behavioral Science Aspirants, J. High. Educ., 82(5), 564–596.
- Chemers M. M., Zurbriggen E. L., Syed M., Goza B. K. and Bearman S., (2011), The role of efficacy and identity in science career commitment among underrepresented minority students, J. Soc. Issues, 67(3), 469–491.
- Cheng H., Potvin G., Khatri R., Kramer L. H., Lock R. M. and Hazari Z., (2018), Examining physics identity development through two high school interventions, Washington, DC.
- Cheung G. W. and Rensvold R. B., (2002), Evaluating goodness-of-fit indexes for testing measurement invariance, Struct. Equ. Model., 9(2), 233–255.
- Cribbs J. D., Hazari Z., Sonnert G. and Sadler P. M., (2015), Establishing an Explanatory Model for Mathematics Identity, Child Dev., 86(4), 1048–1062.
- Dolan C. V., (1994), Factor analysis of variables with 2, 3, 5 and 7 response categories: A comparison of categorical variable estimators using simulated data, Brit. J. Math. Stat. Psy., 47(2), 309–326.
- Estrada M., Woodcock A., Hernandez P. R. and Schultz P. W., (2011), Toward a model of social influence that explains minority student integration into the scientific community, J. Educ. Psychol., 103(1), 206.
- Fencl H. and Scheel K., (2003), Pedagogical approaches, contextual variables, and the development of student self-efficacy in undergraduate physics courses, Madison, WI.
- Ferrell B., (2016), Evaluation of students' interest, effort beliefs, and self-efficacy in general chemistry, PhD thesis, University of Northern Colorado.
- Ferrell B. and Barbera J., (2015), Analysis of students' self-efficacy, interest, and effort beliefs in general chemistry, Chem. Educ. Res. Pract., 16(2), 318–337.
- Fischer R. and Karl J. A., (2019), A Primer to (Cross-Cultural) Multi-Group Invariance Testing Possibilities in R, Front. Psychol., 10, 1–18.
- Flowers A. M. and Banda R., (2016), Cultivating science identity through sources of self-efficacy, J. Multicultural Educ., 10(3), 405–417.
- Furr R. M. and Bacharach V. R., (2008), Psychometrics: an introduction, Thousand Oaks, CA: Sage Publications.
- Gee J. P., (2000), Identity as an Analytic Lens for Research in Education, Rev. Res. Educ., 25, 99–125.
- Glynn S. M., Brickman P., Armstrong N. and Taasoobshirazi G., (2011), Science motivation questionnaire II: Validation with science majors and nonscience majors, J. Res. Sci. Teach., 48(10), 1159–1176.
- Godwin A., Potvin G., Hazari Z. and Lock R. M., (2013), Understanding engineering identity through structural equation modeling, Oklahoma City, OK.
- Godwin A., Potvin G., Hazari Z. and Lock R. M., (2016), Identity, Critical Agency, and Engineering: An Affective Model for Predicting Engineering as a Career Choice, J. Eng. Educ., 105(2), 312–340.
- Graham M. J., Frederick J., Byars-Winston A., Hunter A.-B. and Handelsman J., (2013), Increasing Persistence of College Students in STEM, Science, 341(6153), 1455.
- Hancock G. R. and Mueller R. O., (2001) presented in part at the Structural equation modeling, present and future: a festschrift in honor of Karl Jöreskog, Uppsala, Sweden.
- Harackiewicz J. M., Durik A. M., Barron K. E., Linnenbrink-Garcia L. and Tauer J. M., (2008), The role of achievement goals in the development of interest: Reciprocal relations between achievement goals, interest, and performance, J. Educ. Psychol., 100(1), 105–122.
- Hazari Z., Sonnert G., Sadler P. M. and Shanahan M.-C., (2010), Connecting high school physics experiences, outcome expectations, physics identity, and physics career choice: A gender study, J. Res. Sci. Teach., 47(8), 978–1003.
- Hidi S. and Renninger K. A., (2006), The four-phase model of interest development, Educ. Psychol., 41(2), 111–127.
- Hosbein K. N. and Barbera J., (2020), Alignment of theoretically grounded constructs for the measurement of science and chemistry identity, Chem. Educ. Res. Pract., 21(1), 371–386.
- Hu L.-t. and Bentler P. M., (1999), Cutoff criteria for fit indexes in covariance structure analysis: Conventional criteria versus new alternatives, Struct. Equ. Modeling, 6(1), 1–55.
- Huck S., (2012), Reading Statistics and Research, Boston, MA: Pearson.
- Hunsu N. J., Adesope O. and Van Wie B. J., (2017), Engendering situational interest through innovative instruction in an engineering classroom: what really mattered? Instr. Sci., 45(6), 789–804.
- Kenny D. A., Kaniskan B. and McCoach D. B., (2015), The Performance of RMSEA in Models With Small Degrees of Freedom, Sociol. Methods Res., 44(3), 486–507.
- Kline R. B., (2016), Principles and Practice of Structural Equation Modeling, New York, NY: The Guilford Press.
- Komperda R., Hosbein K. N. and Barbera J., (2018a), Evaluation of the influence of wording changes and course type on motivation instrument functioning in chemistry, Chem. Educ. Res. Pract, 19, 184–198.
- Komperda R., Pentecost T. C. and Barbera J., (2018b), Moving beyond Alpha: A Primer on Alternative Sources of Single-Administration Reliability Evidence for Quantitative Chemistry Education Research, J. Chem. Educ., 95(9), 1477–1491.
- Krosnick J. A. and Presser S., (2010), Handbook of survey research, in Wright J. D. and Marsden P. V. (ed.), San Diego, CA: Elselvier, 2nd edn, pp. 263–314.
- Lent R. W., Lopez F. G., Brown S. D. and Gore J. P. A., (1996), Latent Structure of the Sources of Mathematics Self-Efficacy, J. Vocat. Behav., 49(3),
292–308.
- Locke E. A., Cartledge N. and Knerr C. S., (1970), Studies of the relationship between satisfaction, goal-setting, and performance, Organ. Behav. Hum. Perform., 5(2), 135–158.
- MacCallum R. C., Roznowski M. and Necowitz L. B., (1992), Model modifications in covariance structure analysis: the problem of capitalization on chance, Psychol. Bull., 111(3), 490.
- Mueller R. O. and Hancock G. R., (2008), Best practices in quantitative methods, Thousand Oaks, CA: Sage Punlications, Inc., ch. 32.
- Newsom J. T., (2015), Longitudinal Structural Equation Modeling: A Comprehensive Introduction, New York, NY: Routledge, ch. 4.
- President's Council of Advisors on Science and Technology, (2012), Engage to Excel: Producing One Million Additional College Graduates with Degrees in Science, Technology, Engineering, and Mathematics. Report to the President, Washington, DC.
- Quinlan K. M., (2019), What triggers students’ interest during higher education lectures? personal and situational variables associated with situational interest, Stud. High. Educ., 44(10), 1781–1792.
- Renninger K. A. and Hidi S., (2011), Revisiting the Conceptualization, Measurement, and Generation of Interest, Educ. Psychol., 46(3), 168–184.
- Rotgans J. I. and Schmidt H. G., (2011), The role of teachers in facilitating situational interest in an active-learning classroom, Teach. Teach. Educ., 27(1), 37–42.
- Rotgans J. I. and Schmidt H. G., (2017), Interest development: Arousing situational interest affects the growth trajectory of individual interest, Contemp. Educ. Psychol., 49, 175–184.
- Salkind N. J., (2010), Encyclopedia of Research Design, Thousand Oaks, CA: SAGE Publications, Inc., vol. 1-0.
- Salta K. and Koulougliotis D., (2015), Assessing motivation to learn chemistry: adaptation and validation of Science Motivation Questionnaire II with Greek secondary school students, Chem. Educ. Res. Pract., 16(2), 237–250.
- Satorra A. and Bentler P. M., (1994), Latent Variable Analysis: Applications to Developmental Research, in Eye A. v. and Clogg C. C. (ed.), Newbury Park, CA: Sage, pp. 399–419.
- Satorra A. and Bentler P. M., (2010), Ensuring positiveness of the scaled difference Chi-square test statistic, Psychometrika, 75(2), 243–248.
- Schiefele U., (1991), Interest, Learning, and Motivation, Educ. Psychol., 26(3/4), 299.
- Shedlosky-Shoemaker R. and Fautch J. M., (2015), Who Leaves, Who Stays? Psychological Predictors of Undergraduate Chemistry Students’ Persistence, J. Chem. Educ., 92(3), 408–414.
- Sivo S. A., Fan X., Witta E. L. and Willse J. T., (2006), The Search for “Optimal” Cutoff Properties: Fit Index Criteria in Structural Equation Modeling, J. Exp. Educ., 74(3), 267–288.
- Stets J. E., Brenner P. S., Burke P. J. and Serpe R. T., (2017), The science identity and entering a science occupation, Soc. Sci. Res., 64, 1–14.
- Taasoobshirazi G. and Wang S., (2016), The performance of the SRMR, RMSEA, CFI, and TLI: An examination of sample size, path size, and degrees of freedom, J. Appl. Quant. Methods, 11(3), 31–39.
- Usher E. L. and Pajares F., (2009), Sources of self-efficacy in mathematics: A validation study, Contemp. Educ. Psychol., 34(1), 89–101.
- Verdín D., Godwin A., Kirn A., Benson L. and Potvin G., (2018), Understanding How Engineering Identity and Belongingness Predict Grit for First-Generation College Students, Crystal City, VA.
- Vincent-Ruz P. and Schunn C. D., (2018), The nature of science identity and its role as the driver of student choices, Int. J. Stem Educ., 5(1), 48.
|
This journal is © The Royal Society of Chemistry 2020 |
Click here to see how this site uses Cookies. View our privacy policy here.