DOI:
10.1039/D0RA04795C
(Paper)
RSC Adv., 2020,
10, 29873-29884
Understanding the role of galectin inhibitors as potential candidates for SARS-CoV-2 spike protein: in silico studies†
Received
31st May 2020
, Accepted 5th August 2020
First published on 13th August 2020
Abstract
The Severe Acute Respiratory Syndrome Corona Virus 2 (SARS-CoV-2) has been rapidly transmitting and leaving its footprints across the globe. Stringent measures like complete lockdown and extensive testing have been employed by many countries to slow it down in its tracks until a viable treatment is found. Therefore, in the current scenario, prompt solutions need to be uncovered to tackle the virus. In the present study, 330 galectin inhibitors were tested against SARS-CoV-2 spike (S) protein with the aid of molecular docking and molecular dynamics. Finally, the binding free energy and contributing energies were calculated for 2 top scoring ligands by using MM–GBSA method. Many of the galectin inhibitors displayed high binding score against the S protein. They were found to bind to the site of contact of S protein to ACE2. Thus, they show promise of disrupting the ACE2–S protein binding and prevent the virus from invading the host cell. Among the ligands screened, TD-139, a molecule currently in Phase IIb clinical trials, was found to be a potential hit. The present study paves the way for in vitro and in vivo testing of galectin inhibitors against SARS-CoV-2. In addition, it warrants a swift examination of TD-139 for treating COVID-19.
1. Introduction
Since the beginning of 2020 the world has found itself in a precarious situation with the emergence of ‘public enemy 1, COVID-19’ as named by the World Health Organization (WHO). The pandemic which started on 12th December 2019 has now infected more than 4.68 million people across 216 countries and territories as of 21st May 2020, resulting in over 0.3 million deaths and is still rapidly spreading.1 It was learned based on its phylogenetic characteristic and genetic structure that the causal pathogen of COVID-19 belongs to genera of beta-coronavirus (β-CoV).2 Human pathogenic Severe Acute Respiratory Syndrome Coronavirus 2 (SARS-CoV-2), is the 7th human corona virus among the other six related species namely 229E, NL63, OC43, HKU1, Middle East Respiratory Syndrome Coronavirus (MERS-CoV) and SARS-CoV.3 Unlike the related β-CoVs i.e. SARS-CoV and MERS-CoV, which caused a viral outbreak in November 2002 and September 2012 respectively, SARS-CoV-2 has shown rapid transmission and consequential morbidity.4
Variety of therapies for SARS-CoV and MERS-CoV and other viral diseases that include current and potential treatments with antiviral drugs, steroids, plasma from recovered patients and Chinese medicine are being tested on COVID-19 patients.5 Intravenous administration with remdesivir, a nucleotide analog and an antiviral drug developed by Gilead initially for the treatment of diseases caused by Ebola, Marburg, MERS and SARS viruses has been found to be efficacious in an American patient with COVID-19.6 Baricitinib, interferon-α, lopinavir/ritonavir, and ribavirin have been suggested as potential therapies for patients with acute respiratory symptoms and interactions are monitored carefully.7 Despite so many experimental and computational studies currently searching for a potential breakthrough, to date, there is no confirmed effective treatment specifically available for COVID-19.
The therapies against SARS-CoV-2 can be divided into two broad categories. One acting on the human immune system and the other acting on the coronavirus itself. The latter can be further sub-divided into two categories – one which prevents the viral RNA synthesis and replication and the second which blocks the binding to human cell receptors.8 Two groups of proteins characterize CoVs; structural proteins such as spike (S), membrane (M), nucleocapsid (N), and envelope (E), in addition to the non-structural proteins, like proteases and RNA-dependent RNA polymerase (RdRp).9,10 The S protein is the sole protein responsible for mediating viral entry into the host cell and thus a crucial recognition factor for attachment to human cellular receptor angiotensin-converting enzyme 2 (ACE2) to gain entry.11,12 The S protein protrudes out from the viral surface that gives coronaviruses a crownlike appearance by forming spikes on their surface (Fig. 1).
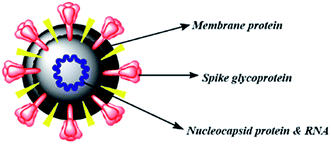 |
| Fig. 1 Schematic diagram of betacoronavirus. | |
Many computational approaches have been explored to search for small molecular inhibitors against SARS-CoV-2 main protease and RdRp.13–16 Analogous screening of potential drugs against the S protein of SARS-CoV-2 provided small molecular compounds with a high binding affinity. However, most of these compounds were not found to attach to the binding interface of the receptor binding domain (RBD) of S protein and ACE2. Fortunately, hesperidin was predicted to lie on the surface of RBD, and was suggested to disrupt the interaction of ACE2–RBD complex.8 In another development, Bioxytran, a Biotechnology company based in United States, recently concluded that a galectin inhibitor could bind to the coronavirus spikes and reduce viral load.17 Encouraged by the potential ability of a glycosylated ligand to cause disruption of ACE2 interaction and findings of Bioxytran, we decided to test other reported galectin inhibitors like TD139 (currently in Phase IIb clinical trial) and others for their ability to disrupt RBD–ACE2 complex (Fig. 2).18 In this work, the binding affinity of several monosaccharide and disaccharide galectin inhibitors with SARS-CoV-2 S protein was evaluated using molecular docking, molecular dynamics and molecular mechanics–generalized born surface area (MM–GBSA) calculations. This study provides an insight into the probable repurposing of galectin inhibitors like TD139 against SARS-CoV-2 and related coronaviruses. TD139, thus, could be used as an inhaled therapeutic for topical lung delivery, providing a valuable and swift therapy to combat COVID-19.
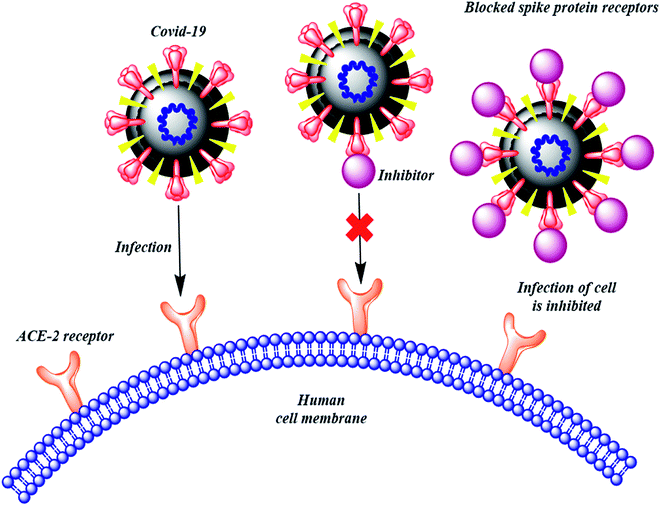 |
| Fig. 2 Mechanism of action to prevent SARS-CoV-2 entry into human cells. | |
2. Materials and methods
2.1 Dataset collection, ligand preparation and calculating ADMET properties
All the 330 galectin inhibitors which reported in 28 individually published articles were selected for study.19–46 The compounds were sketched by using the 2D-sketcher in Maestro (Schrödinger Suite 2018-4). These compounds were then prepared using the “LigPrep” module by generating low energy ionization and tautomeric states with a pH of 7.4 using OPLS-2005 force field for minimization. Further, the ligand pdb files were converted to pdbqt format using Open Babel.47 The QikProp analysis was performed for the prepared compounds in Maestro Schrödinger Suite 2018-4 to predict their ADME i.e. pharmacokinetic properties. The various physical descriptors namely, Hydrogen Bond Donors (HBD), Hydrogen Bond Acceptors (HBA), number of Nitrogen & Oxygen atoms (N & O), ring atoms, etc., for all the compounds were predicted. Further, toxicological profiling of selected ligands was performed using admetSAR48 and Toxicity Estimation Software Tool (TEST).49
2.2 Protein preparation
Target protein, SARS-CoV-2 spike protein (PDB ID: 6M0J) with resolution 2.45 Å was obtained from Research Collaboratory for Structural Bioinformatics – Protein Data Bank (RCSB – PDB).50 All bound waters and cofactors were removed, Kolmann charges were computed, polar hydrogen atoms were subsequently added and the AutoDock atom types were defined using AutoDockTools (ADT), Graphical User Interface (GUI) of AutoDock implemented in Molecular Graphics Laboratory (MGL) Tools.51
2.3 Grid generation & docking
To generate putative binding poses, we used the AutoDock Vina software.52 In general, the docking parameters were kept to the default values. The size of the docking grid was fixed at 40 Å × 40 Å × 40 Å. The X, Y, and Z coordinates of the grid box were fixed at −36.126, 32.573 and 3.383 respectively, thus encompassing the active site. The output file generated was analyzed using Discovery Studio Visualizer.53
2.4 Induced fit docking
Induced Fit Docking (IFD) gives a more accurate picture of binding by providing the flexibility to both ligand and the active site residues. Under the Schrödinger's IFD sampling protocol,54 the protein was optimized further and a grid was generated by choosing the bound ligand. A soften potential docking with van der Waals scaling of 0.5 each for protein and two top scoring ligands was performed. A maximum of 80 poses were generated for each selected ligand. Prime refinement was carried out for residues within 5 Å of the ligand. Glide redocking was carried out for structures within 30 kcal mol−1 of the best structure. The most favourable binding conformations of each ligand complex, as measured by the IFDScore, were selected for analysis.
2.5 Molecular dynamics simulations
In order to better understand the mechanism of interaction between the protein and the ligand, molecular dynamics simulations of 100 ns were performed for the two top scoring ligands using Desmond module of Schrödinger suite (2020-2).55 Aqueous biological system was built by using OPLS_2005 force field and TIP3P model was used to stimulate the water molecules. Orthorhombic periodic boundary conditions were set up to specify the shape and size of the repeating unit buffered at 10 Å distances. The physiological pH was neutralized by adding 0.15 M NaCl. 300 K temperature and 1.01325 bar pressure was maintained by using Nose–Hoover temperature coupling and Martina–Tobias–Klein method for the constant pressure, respectively. Reversible reference system propagation algorithm (REPSA), a time stepping algorithm was used for near non-bonded (2 fs), far non-bonded (6 fs), and bonded interactions (2 fs). In the end, molecular dynamics simulations (100 ns) were performed for the two top scoring ligands.
2.6 Binding free energy calculations
The MM/GBSA method (Schrödinger Release 2020-2: Prime, Schrödinger, LLC, New York, NY, 2020-2)56 was used to calculate the binding free energy (ΔGbind), for receptor–binder complex systems. One thousand snapshots were taken at 5 ns time intervals throughout the MD simulation trajectory to compute the MM/GBSA free energy difference.
3. Results
Molecular docking is an indispensable technique in the path to drug discovery and it attains an increased significance when there are no leads/hits available to direct a way forward.57 Docking studies were carried out to ascertain the binding affinity of the inhibitors. The calculated binding affinity of selected galectin inhibitors with SARS-CoV-2 S protein along with their 2D structures has been tabulated in ESI Table 1 (S1).† The pharmacokinetic properties for all the ligands commonly referred to as ADME (Absorption, Distribution, Metabolism and Excretion) properties were also calculated and can be found in ESI Table 2 (S2).†
4. Discussion
4.1 Molecular docking
Based on molecular docking analysis some key observations were made. The thiodigalactoside and disaccharide derivatives with rigid substituents/linkers like triazoles and amides showed better binding energy than inhibitors with freely rotating linkers as observed for ligand 2. This is quite probable as they won't quite fit in the binding site due to their flexibility. The aryl flanking groups as substituents led to better scores due to their engagement in stacking interactions with amino acid side chains present in the S protein. When compared to disaccharides, monosaccharides showed significantly lesser binding affinity towards the SARS-CoV-2 S protein. This is reasonable since the interface of RBD–ACE2 complex encompasses an appreciably large area and the monosaccharide derivatives are unable to fit completely in the cavity of RBD. Majority of ligands screened were able to show a better binding score than hesperidin (−7.3 kcal mol−1), which was taken as a reference and is reported to bind to the SARS-CoV-2 RBD–ACE2 interface. Top 4 molecules (all disaccharides) with the best binding affinity were picked to study their interactions with the S protein while one monosaccharide was chosen with an appreciably high binding energy in comparison to other monosaccharides and similar or higher to that of many disaccharides. The 2D and 3D interaction diagrams of ligand 3, 125, 152, 213 and 238 along with their respective docking scores can be found in Fig. 3 & 4 respectively. Among the top ligands, ligand 238 is TD139, a galectin inhibitor, is currently in Phase IIb clinical trials to treat idiopathic pulmonary fibrosis.18
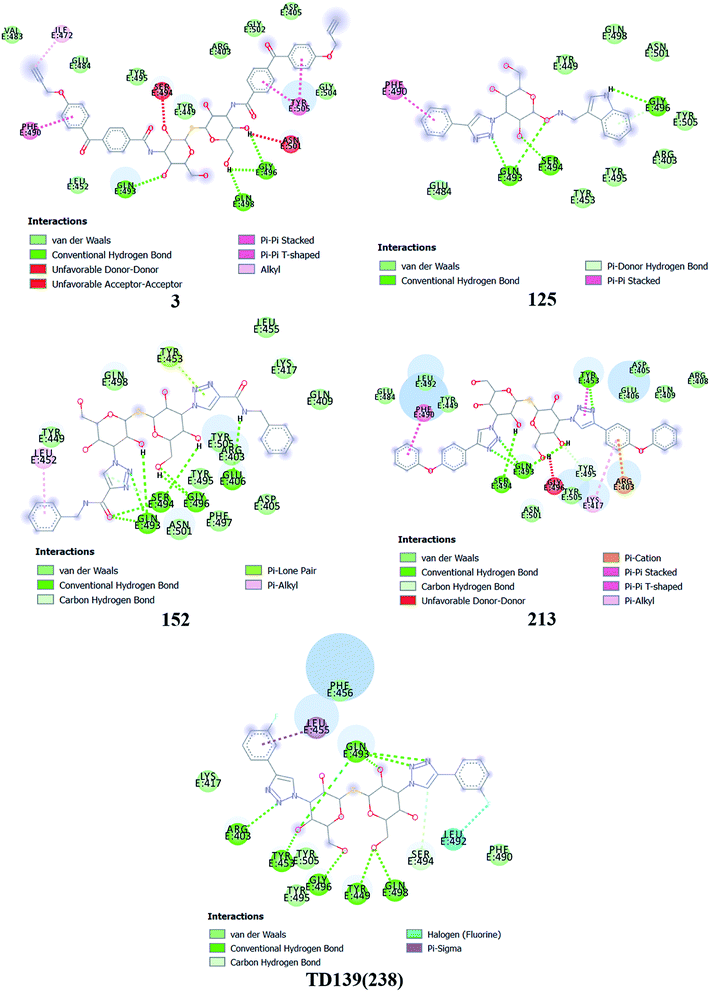 |
| Fig. 3 2D interaction diagram for the top 5 ligands. The binding energy for these ligands in kcal mol−1 were found as follows – ligand 3 (−8.3), 125 (−7.9), ligand 152 (−8.5), ligand 213 (−8.9) and ligand 238 (−8.5). | |
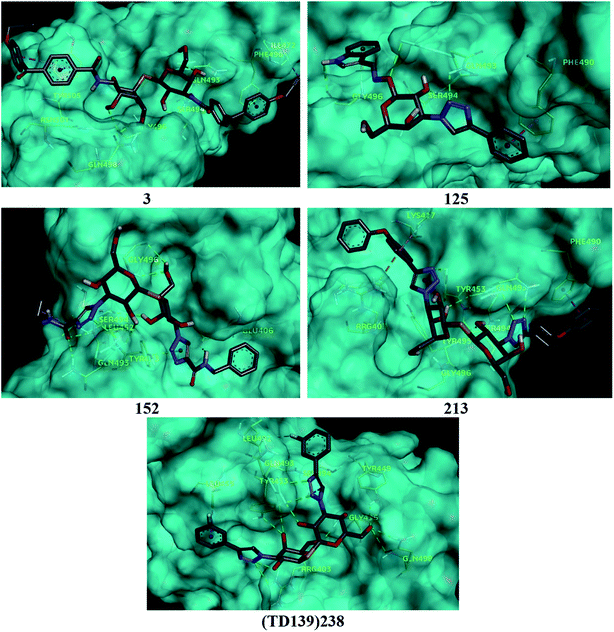 |
| Fig. 4 3D interaction diagram for the top 5 ligands. | |
As mentioned before, small molecules were able to bind strongly to the S protein, however, they were unable to bind to the interface of RBD–ACE2 complex which is crucial to stop the entry of the virus into the human cell. Thus, it was pertinent to check whether the screened ligands were able to bind to the SARS-CoV-2 RBD. The contact residues of SARS-CoV-2 RBD involved in binding to ACE2 include Lys417, Gly446, Tyr449, Tyr453, Leu455, Phe456, Ala475, Phe486, Asn487, Tyr489, Gln493, Gly496, Gln498, Thr500, Asn501, Gly502 and Tyr505.58 On examination of the interactions of the screened ligands, the following was observed – ligand 3 displayed H-bond interactions with Gln493, Gly496 (2 H-bond interactions) at a distance of 2.68, 2.53, 2.81 & 2.09 Å respectively. Van der Waals interactions were seen with Arg403, Asp405, Tyr449, Leu454, Val483, Glus484, Tyr495, Gly502, Gly504. Hydrophobic (Alkyl, Pi–Pi stacked & Pi–Pi T shaped) interactions with Ile472, Phe490 & Tyr505 were also displayed. Ligand 125 exhibited H-bond interactions with Gln493 (2 H-bond interactions), Ser494 & Gly496 at a distance of 2.98, 2.46, 1.88 & 3.02 Å respectively. Van der Waals interactions were observed with Arg403, Tyr449, Tyr453, Glu484, Tyr495, Gln498, Asn501 & Tyr505. Hydrophobic (Pi–Pi stacked & Pi–Donor H bond) interactions were displayed with Phe490 & Gly496 respectively. Ligand 152 unveiled H-bond interactions with Glu406, Gln493 (2 H-bond interactions), Ser494 (2 H-bond interactions) & Gly496 (2 H-bonds interactions) at a distance of 3.09, 2.22, 2.88, 2.14, 2.54, 2.36 & 2.49 Å respectively. Van der Waals interactions were shown with Arg403, Asp405, Gln409, Lys417, Tyr449, Leu455, Tyr495, Phe497, Gln498, Asn501 & Tyr505. Hydrophobic (Pi–Alkyl & Pi–Lone pair) interactions with Leu452 & Tyr453. Ligand 213 displayed H-bond interactions with Tyr453, Gln493 (3 H-bond interactions), Ser494 and Tyr495 at a distance of 2.53, 2.18, 2.68, 2.23, 3.02, 3.61 Å respectively. Van der Waals interactions were seen with Asp405, Glu406, Arg408, Gln409, Tyr449, Glu484, Leu492, Asn501 & Tyr505. Hydrophobic (Pi–Cation, Pi–Alkyl, Pi–Pi T shaped & Pi–Pi stacked) interactions were displayed with Arg403, Lys417, Tyr453 & Phe490 respectively. Ligand 238 (TD-139) displayed H-bond interactions with Arg403 (2 H-bond interactions), Tyr449, Tyr453, Gln493 (4 H-bond interactions), Ser494, Gly496, Gln498 (2 H-bond interactions) at a distance of 2.90, 3.02, 2.31, 2.81, 2.86, 2.64, 2.28, 3.30, 3.31, 1.90, 2.24 & 3.02 Å respectively. Van der Waals interactions were seen with Lys417, Phe456, Phe490, Tyr495 & Tyr505 along with hydrophobic (Pi–Sigma) interactions with Leu455. In all the ligands, a common feature was observed throughout. The free hydroxyls present on the sugar moiety were involved in the polar interactions while aryl groups linked to the core structure via a linker were involved in varied pi stacking interactions. In addition, another key observation was made regarding the linker. In all, the top scoring ligands, the linker was found to be a rigid and considerably longer. An aryl group linked via a triazole was seen in ligand 125, 213 and 238 while an aryl linked via a triazolo amide was sighted in ligand 152. Ligand 3 on the other hand contained an aryl linked via a benzamide derivative.
It is also worth noting that monosaccharides substituted at C2 position or disaccharides substituted at positions other than the C3 (188, 189, 200 and 257); ligands where the pyranose core was linked to a large hydrophobic moiety via a freely rotatable bond (compare 2 to 3, 12, 19 to 17, 32, 39 to 42, 46 to 47 and 125 to 126) and pyranose units with relatively smaller substitutions (34, 36, 39, 54, 57, 71, 74, 75, 85, 176 among many more) consistently performed poorly in the rankings based on docking score. Interestingly, similar to what was pointed out before, it was observed that among all the top scoring ligands, thiodigalactoside core with a C3 substituted unit linked to a hydrophobic moiety via triazole or amide was a common link (1, 3, 109, 110, 151, 152, 210, 213, 214 and others).
The above evidence clearly suggests that many of the galectin inhibitors screened in the study are able to bind strongly to the SARS-CoV-2 RBD and more importantly exhibit interaction with the contact residues of RBD which are responsible for binding to ACE2. Consequently, they have phenomenal potential to disrupt the SARS-CoV-2 and ACE2 interaction and thus prevent the virus from infesting the host cell.
4.2 ADMET properties
All the five ligands showed good partition coefficient (QPlog
Po/w: octanol/water partition coefficient) values which were in the acceptable range (−2 to 6.5). The predicted aqueous solubility (QPlog
S) of ligand 125, 152 & TD139 were in the acceptable range (−6.5 to 0.5). The PSA has a great influence on the oral bioavailabity of the molecules. The optimum value of PSA (7-200 Å) was exceeded by three ligands (3, 152 & 213) whereas the other two ligands (125 & TD139) has the PSA value in acceptable range. As the molecules taken for the study are polar saccharide molecules, they have high molecular weight and a greater number of OH groups (H-bond donor & acceptors) which led to the 3 violations in the Lipinski's rule of five in all ligands except ligand 125 (Table 1). All further studies hereon were performed for ligand 213 (the best ranked ligand) and TD139 (currently in clinical trials and thus having the potential to be marketed early).
Table 1 ADME properties for the top 5 ligands
Ligand no. |
Mol wt. |
HB donor |
HB acceptor |
QPlog Po/w |
QPlog S |
PSA |
N & O |
Rule of 5 |
Rule of 3 |
Ring atoms |
3 |
880.919 |
9 |
24.6 |
1.69 |
−6.878 |
278.853 |
16 |
3 |
3 |
36 |
125 |
449.465 |
4 |
12.5 |
1.563 |
−4.534 |
132.847 |
10 |
0 |
0 |
26 |
152 |
726.76 |
8 |
24.1 |
−0.95 |
−5.166 |
279.673 |
18 |
3 |
2 |
34 |
213 |
796.85 |
6 |
20.1 |
3.085 |
−7.557 |
219.196 |
16 |
3 |
3 |
46 |
238 (TD139) |
648.637 |
6 |
19.1 |
0.765 |
−5.438 |
198.55 |
14 |
3 |
2 |
34 |
The in silico toxicity profiling was carried out for ligand 213 and TD-139. The median lethal dose (LD50) of a compound describes the ability of a compound to kill 50% of the test population. LD50 was predicted in silico in a rat model. In our study, we found that ligand 213 was predicted to have a LD50 value of 2.4156 mol kg−1 while for TD139 this value was slightly higher at 2.5355 mol kg−1. The human Ether-à-go-go Related Gene (hERG) encodes potassium channels, which are responsible for the normal repolarization of the cardiac action potential. Blockage of these channels could lead to fatal cardiac problems.59 Therefore, it is essential for any potential drug to not inhibit it. Both the molecules were predicted to be non-inhibitors of hERG. At last, it is important to test for toxicity arising from carcinogenic and mutagenic properties of molecules in addition to its reproductive toxicity. Both, TD139 and ligand 213 were predicted to be non-carcinogens and non-mutagens. However, ligand 213 was predicted to have developmental toxicity. Developmental toxicity includes any effect interfering with normal development, both pre and post birth. This includes embryotoxic effects such as abortion, organ toxicity, teratogenic effects, reduced growth, impaired post-natal mental and physical development among others.49 Thus, this needs to be carefully evaluated when considering ligand 213's therapeutic potential for a disease. The results of the toxicity profiling are summarized in Table 2.
Table 2 Toxicity profile for the most promising inhibitors
Ligand no. |
LD50 (mol kg−1) |
hERG inhibition |
Carcinogenicity |
AMES mutagenicity |
Developmental toxicity |
213 |
2.4156 |
Negative |
Negative |
Negative |
Yes |
238 (TD139) |
2.5355 |
Negative |
Negative |
Negative |
No |
4.3 Induced fit docking
To account for movements in the protein structure, side chains and beyond, we employed the IFD protocol, which is crucial for accurate docking of the ligand. IFD protocol of Schrödinger allows incorporation of receptor flexibility by adjusting the receptor structure based on the docked ligand. The IFDScore obtained with the IFD protocol detailed in the Material and methods section was found to be in accordance with the docking scores. Ligand 213 was predicted to have IFDScore of −7904.77 while it was predicted to be −7878.48 for TD139. However, an interesting point to note was while both the ligands bound to a similar site as they did in rigid docking, the interactions were significantly different (Fig. 5).
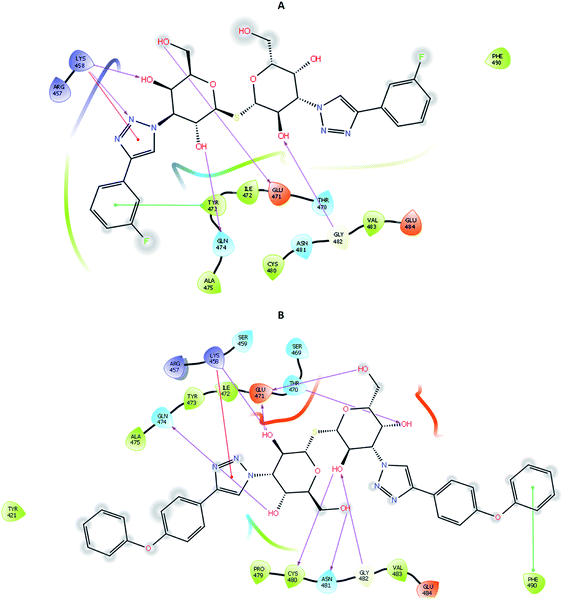 |
| Fig. 5 2D interaction diagram of (A) TD139 and (B) 213 on docking using IFD protocol. | |
4.4 Molecular dynamics and binding free energy
Proteins are dynamic macromolecules under physiological conditions, and therefore the results derived from molecular docking is less persuasive. Molecular docking predicts the spatial orientation of a ligand in the active pocket of the protein. However, molecular dynamics simulations, in addition to the fit of the binding pocket, takes into account factors like a conformational stability and flexibility.
Molecular dynamics trajectory was used to examine the equilibration of dynamics over period of time. Configuration root mean square deviation (RMSD) for TD139-6M0J with respect to its initial structure was found to increase and 6M0J complex was found to converge at 45 ns.
The RMSD of the C-alphas, heavy atoms, backbone and side chains of TD139-6M0J and 213-6M0J displayed little fluctuations in between, but maintained constant values during the major course of the simulation. At 10 ns, RMSD values of C-alphas, backbone side chains and heavy atoms were 1.312, 1.327, 2.169 and 1.594 Å, respectively, for TD-139-6M0J. At the end of 100 ns, the observed RMSD values of C-alphas (Fig. 6), heavy atoms, backbone and side chains were 2.082, 2.424, 2.080 and 2.937 Å, respectively. TD139 after initial fluctuations maintained a constant RMSD of 1.6 Å. At 10 ns, RMSD values of C-alphas, backbone side chains and heavy atoms were 1.329, 1.801, 1.350 and 2.423 Å, respectively, for 213-6M0J. At the end of 100 ns, the observed RMSD values of C-alphas (Fig. 6), heavy atoms, backbone and side chains were 1.757, 2.213, 1.726 and 2.923 Å, respectively. 213 maintained a constant RMSD initially but later showed fluctuations between 1.7 and 4.3 Å throughout the simulation. Protein–ligand interactions of both the complexes were examined during the course of MD simulation. Fig. 7 demonstrates the type of protein–ligand contacts exhibited by the complexes employed in MD simulation. Mainly, there are three types of protein–ligand interactions: H-bonds, hydrophobic and water bridges. TD139 displayed number of H-bond interactions either directly or mediated by water molecule. Interactions with the residues Asn370, Asn481, Gly482 were seen along with strong and consistent interaction with Glu471, Ile472 and Gln474 during the course of MD simulation. However, no hydrophobic interactions could be observed. In the results obtained from the MD studies, it was found that the hydroxyls of pyranose unit and nitrogen atoms belonging to the triazole were responsible for the strong interactions in TD139. This suggests that hydrogen bonding plays a significant role in accommodating and stabilizing TD-139 at the binding site. Fig. 8 illustrates timeline representation of ligand–protein contacts including both H-bond and hydrophobic interactions for ligands TD139 and 213 with the receptor during 100 ns MD simulation period. Further, ligand–protein interactions of 6M0J-213 complex were also analyzed. 213 displayed hydrophobic interactions with the residues Tyr449, Leu452, Tyr473 and Phe490. In addition, it displayed hydrogen bond interaction with Thr479, Glu471, Ile472 and Gln474. The initial stability of 6M0J can be assigned to its ability to form H-bond which quickly disappears beyond 50 ns along with the stability of ligand. This further strengthens our conclusion that H-bond interactions are crucial for stabilization of ligand within the binding site.
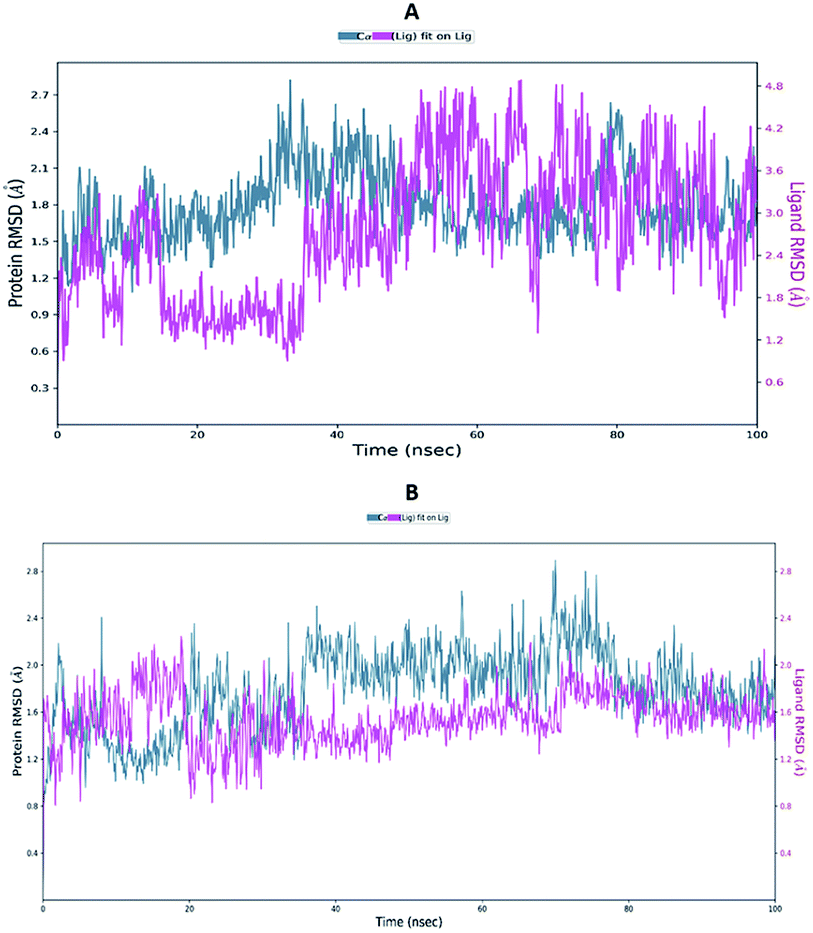 |
| Fig. 6 Average RMSD of C-alphas of protein and ligand complexes (A) 213-6M0J and (B) TD139-6M0J during molecular dynamics simulation (100 ns). | |
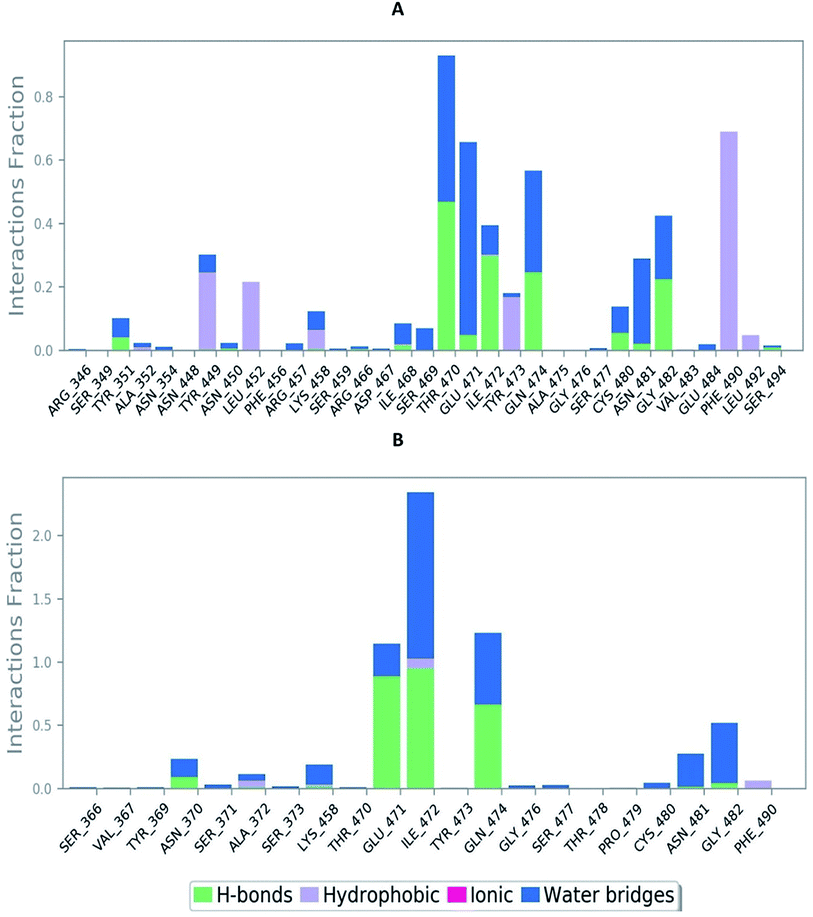 |
| Fig. 7 Bar-chart representation of protein–ligand contacts of (A) 213-6M0J and (B) TD139-6M0J during molecular dynamics simulation (100 ns). | |
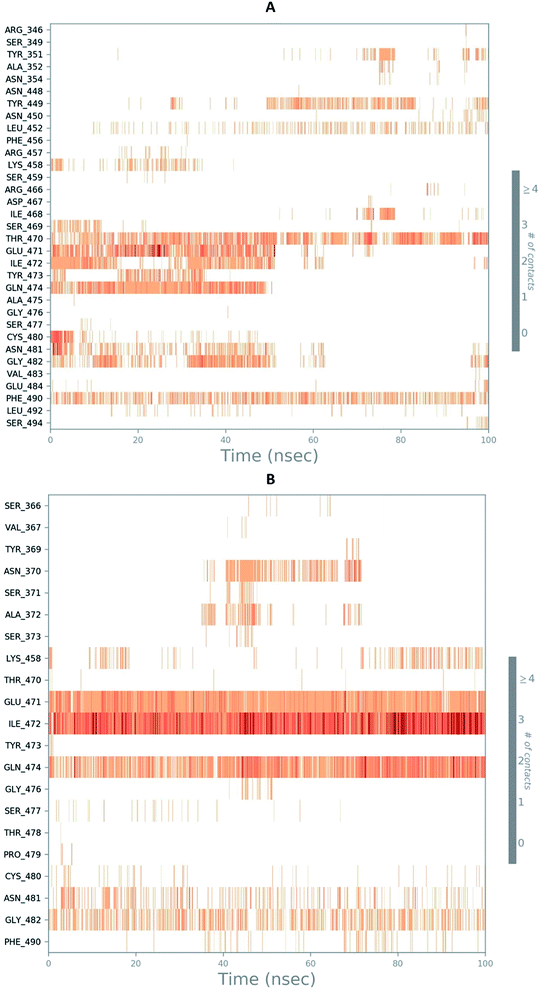 |
| Fig. 8 Timeline representation of ligand–protein contacts (A) 213-6M0J and (B) TD139-6M0J during molecular dynamics simulation (100 ns). | |
The negative values of ΔGbind indicate that the selected compounds favourably interact with the receptor. The free energy of binding values and parameters contributing towards it are summarized in Table 3. The ΔGbind for ligand 213 is −54.11 kcal mol−1 while it is −45.58 kcal mol−1 for TD139. On further investigating the contribution of each energy term it was apparent that van der Waals interaction play a crucial role. In addition, the contribution of H bond and coulombic term was more for TD139 when compared to ligand 213 which is consistent with the MD studies.
Table 3 Prime MMGBSA binding energy of ligandsa
Ligand no. |
ΔGbind |
ΔGbind Coulomb |
ΔGbind covalent |
ΔGbind H-bond |
ΔGbind lipo |
ΔGbind solv. GB |
ΔGbind vdW |
ΔGbind packing |
ΔGbind – total free energy of binding, ΔGbind Coulomb – Coulomb energy, ΔGbind covalent – covalent binding energy, ΔGbind H-bond – hydrogen-bonding correction, ΔGbind lipo – lipophilic energy, ΔGbind solv. GB – generalized Born electrostatic solvation energy, ΔGbind vdW – van der Waals energy and ΔGbind packing – pi–pi packing correction. |
213 |
−54.11 |
−15.03 |
4.30 |
−1.55 |
−21.17 |
21.56 |
−38.91 |
−3.31 |
238 (TD139) |
−45.58 |
−20.52 |
4.81 |
−2.33 |
−12.80 |
23.48 |
−35.20 |
−3.02 |
5. Conclusion
The coronavirus (SARS-CoV-2) outbreak came to light on December 31, 2019 when China informed the WHO of a cluster of cases of pneumonia of an unknown cause in the city of Wuhan. Subsequently, this viral infection has indeed got viral and spread to the rest of the world. The present study was aimed at discovering small molecular inhibitors that could inhibit SARS-CoV-2 by binding to the spike protein and inevitably prevent the virus from infiltrating the host cell. Virtual screening was carried out with reported galectin inhibitors based on evidence gathered from literature. Several ligands showed promising binding affinity and interaction with residues responsible for binding of ACE2 to SARS-CoV-2 RBD, which strongly suggests the potential of the inhibitors to disrupt the RBD–ACE2 interactions. Among the many promising ligands, 213 and TD139 came to light as an effective inhibitor. Luckily TD139 is also currently in phase IIb clinical trials to treat idiopathic pulmonary fibrosis and has shown no adverse side effect. Thus, the results of our study warrants the testing of TD-139 and related galectin inhibitors for in vitro and in vivo testing against SARS-CoV-2 and related coronaviruses to halt the spread of the virus in present and the future.
Conflicts of interest
There are no conflicts to declare.
Acknowledgements
Authors are thankful to DoP, Ministry of Chemicals & Fertilizers, Govt. of India, New Delhi, for the award of NIPER fellowship and supporting the work. Authors are also thankful to Vinod Devaraji, Schrodinger, Bengaluru for molecular dynamics studies.
References
- World Health Organization, WHO Coronavirus Disease (COVID-19) Dashboard, https://covid19.who.int/, accessed 21 May 2020 Search PubMed.
- N. Zhu, D. Zhang, W. Wang, X. Li, B. Yang, J. Song, X. Zhao, B. Huang, W. Shi, R. Lu and P. Niu, N. Engl. J. Med., 2020, 382, 727–733 CrossRef PubMed.
- D. Wu, T. Wu, Q. Liu and Z. Yang, Int. J. Infect. Dis., 2020, 94, 44–48 CrossRef PubMed.
- R. Hilgenfeld and M. Peiris, Antiviral Res., 2013, 100, 286–295 CrossRef PubMed.
- M. Cascella, M. Rajnik, A. Cuomo, S. C. Dulebohn and R. Di Napoli, Features, evaluation and treatment coronavirus (COVID-19), StatPearls Publishing, Treasure Island, 2020, https://www.ncbi.nlm.nih.gov/books/NBK554776/ Search PubMed.
- A. Abdelmaksoud, M. Goldust and M. Vestita, Dermatol Ther., Advance Online Publication, 2020, p. e13360 Search PubMed.
- J. Stebbing, A. Phelan, I. Griffin, C. Tucker, O. Oechsle, D. Smith and P. Richardson, Lancet Infect. Dis., 2020, 20, 400–402 CrossRef PubMed.
- C. Wu, Y. Liu, Y. Yang, P. Zhang, W. Zhong, Y. Wang, Q. Wang, Y. Xu, M. Li, X. Li and M. Zheng, Acta Pharm. Sin. B, 2020, 10(5), 766–788 CrossRef PubMed.
- F. Li, Annu. Rev. Virol., 2016, 3, 237–261 CrossRef CAS PubMed.
- I. M. Ibrahim, D. H. Abdelmalek, M. E. Elshahat and A. A. Elfiky, J. Infect., 2020, 80(5), 554–562 CrossRef CAS.
- B. J. Bosch, R. van der Zee, C. A. de Haan and P. J. Rottier, J. Virol., 2003, 77, 8801–8811 CrossRef CAS PubMed.
- P. Zhou, X. L. Yang, X. G. Wang, B. Hu, L. Zhang, W. Zhang, H. R. Si, Y. Zhu, B. Li, C. L. Huang and H. D. Chen, Nature, 2020, 579, 270–273 CrossRef CAS.
- M. T. ul Qamar, S. M. Alqahtani, M. A. Alamri and L. L. Chen, J. Pharm. Anal., 2020 DOI:10.1016/j.jpha.2020.03.009.
- R. Yu, L. Chen, R. Lan, R. Shen and P. Li, Int. J. Antimicrob. Agents, 2020, 106012 CrossRef CAS PubMed , in press.
- P. Sang, S. H. Tian, Z. H. Meng and L. Q. Yang, RSC Adv., 2020, 10, 15775–15783 RSC.
- A. A. Elfiky, J. Biomol. Struct. Dyn., 2020, 1–15 Search PubMed.
- BioXyTran Inc, Bioxytran Releases Details on a Novel Carbohydrate Galectin Inhibitor designed to Eliminate COVID-19 in Patients, https://www.bioxytraninc.com/press-releases/bioxytran-releases-details-on-a-novel-carbohydrate-galectin-inhibitor-designed-to-eliminate-covid-19-in-patients, accessed 21 May 2020 Search PubMed.
- ClinicalTrials.gov, A study to test the efficacy and safety of inhaled TD139 in subjects with idiopathic pulmonary fibrosis, https://clinicaltrials.gov/ct2/show/NCT03832946, accessed 21 May 2020 Search PubMed.
- A. Dahlqvist, F. R. Zetterberg, H. Leffler and U. J. Nilsson, MedChemComm, 2019, 10, 913–925 RSC.
- R. Kumar, M. M. Ignjatovic, K. Peterson, M. Olsson, H. Leffler, U. Ryde, U. J. Nilsson and D. T. Logan, ChemMedChem, 2019, 14, 1528–1536 CrossRef CAS PubMed.
- J. Stegmayr, F. Zetterberg, M. C. Carlsson, X. Huang, G. Sharma, B. Kahl-Knutson, H. Schambye, U. J. Nilsson, S. Oredsson and H. Leffler, Sci. Rep., 2019, 9, 1–12 CrossRef CAS PubMed.
- R. Kumar, K. Peterson, M. M. Ignjatovic, H. Leffler, U. Ryde, U. J. Nilsson and D. T. Logan, Org. Biomol. Chem., 2019, 17, 1081–1089 RSC.
- K. Peterson, R. Kumar, O. Stenstrom, P. Verma, P. R. Verma, M. Hakansson, B. Kahl-Knutsson, F. Zetterberg, H. Leffler, M. Akke and D. T. Logan, J. Med. Chem., 2018, 61, 1164–1175 CrossRef CAS PubMed.
- F. R. Zetterberg, K. Peterson, R. E. Johnsson, T. Brimert, M. Hakansson, D. T. Logan, H. Leffler and U. J. Nilsson, ChemMedChem, 2018, 13, 133–137 CrossRef CAS.
- J. Dion, T. Advedissian, N. Storozhylova, S. Dahbi, A. Lambert, F. Deshayes, M. Viguier, C. Tellier, F. Poirier, S. Teletchea and C. Dussouy, ChemBioChem, 2017, 18, 2428–2440 CrossRef CAS.
- C. Atmanene, C. Ronin, S. Teletchea, F. M. Gautier, F. Djedaini-Pilard, F. Ciesielski, V. Vivat and C. Grandjean, Biochem. Biophys. Res. Commun., 2017, 489, 281–286 CrossRef CAS PubMed.
- V. K. Rajput, A. MacKinnon, S. Mandal, P. Collins, H. Blanchard, H. Leffler, T. Sethi, H. Schambye, B. Mukhopadhyay and U. J. Nilsson, J. Med. Chem., 2016, 59, 8141–8147 CrossRef CAS PubMed.
- T. J. Hsieh, H. Y. Lin, Z. Tu, T. C. Lin, S. C. Wu, Y. Y. Tseng, F. H. Liu, S. T. D. Hsu and C. H. Lin, Sci. Rep., 2016, 6, 1–9 CrossRef PubMed.
- T. Delaine, P. Collins, A. MacKinnon, G. Sharma, J. Stegmayr, V. K. Rajput, S. Mandal, I. Cumpstey, A. Larumbe, B. A. Salameh and B. Kahl-Knutsson, ChemBioChem, 2016, 17, 1759–1770 CrossRef CAS PubMed.
- M. F. Marchiori, D. E. P. Souto, L. O. Bortot, J. F. Pereira, L. T. Kubota, R. D. Cummings, M. Dias-Baruffi, I. Carvalho and V. L. Campo, Bioorg. Med. Chem., 2015, 23, 3414–3425 CrossRef CAS.
- V. K. Rajput, H. Leffler, U. J. Nilsson and B. Mukhopadhyay, Bioorg. Med. Chem. Lett., 2014, 24, 3516–3520 CrossRef CAS PubMed.
- H. Van Hattum, H. M. Branderhorst, E. E Moret, U. J. Nilsson, H. Leffler and R. J. Pieters, J. Med. Chem., 2013, 56, 1350–1354 CrossRef CAS.
- P. M. Collins, C. T. Oberg, H. Leffler, U. J. Nilsson and H. Blanchard, Chem. Biol. Drug Des., 2012, 79, 339–346 CrossRef CAS.
- C. T. Oberg, H. Leffler and U. J. Nilsson, Chimia, 2011, 65, 18–23 CrossRef.
- C. T. Oberg, A. L. Noresson, H. Leffler and U. J. Nilsson, Tetrahedron, 2011, 67, 9164–9172 CrossRef.
- B. A. Salameh, I. Cumpstey, A. Sundin, H. Leffler and U. J. Nilsson, Bioorg. Med. Chem., 2010, 18, 5367–5378 CrossRef CAS PubMed.
- J. Tejler, B. Salameh, H. Leffler and U. J. Nilsson, Org. Biomol. Chem., 2009, 7, 3982–3990 RSC.
- C. T. Oberg, H. Leffler and U. J. Nilsson, J. Med. Chem., 2008, 51, 2297–2301 CrossRef.
- M. Van Scherpenzeel, E. E. Moret, L. Ballell, R. M. Liskamp, U. J. Nilsson, H. Leffler and R. J. Pieters, ChemBioChem, 2009, 10, 1724–1733 CrossRef CAS.
- I. Cumpstey, E. Salomonsson, A. Sundin, H. Leffler and J. Nilsson, Eur. J. Chem., 2008, 14, 4233–4245 CrossRef CAS.
- I. Cumpstey, E. Salomonsson, A. Sundin, H. Leffler and U. J. Nilsson, ChemBioChem, 2007, 8, 1389–1398 CrossRef CAS.
- J. Tejler, F. Skogman, H. Leffler and U. J. Nilsson, Carbohydr. Res., 2007, 342, 1869–1875 CrossRef CAS PubMed.
- I. Cumpstey, A. Sundin, H. Leffler and U. J. Nilsson, Angew. Chem., Int. Ed., 2005, 44, 5110–5112 CrossRef CAS PubMed.
- B. A. Salameh, H. Leffler and U. J. Nilsson, Bioorg. Med. Chem. Lett., 2005, 15, 3344–3346 CrossRef CAS PubMed.
- J. Tejler, H. Leffler and U. J. Nilsson, Bioorg. Med. Chem. Lett., 2005, 15, 2343–2345 CrossRef CAS PubMed.
- P. Sorme, P. Arnoux, B. Kahl-Knutsson, H. Leffler, J. M. Rini and U. J. Nilsson, J. Am. Chem. Soc., 2005, 127, 1737–1743 CrossRef PubMed.
- N. M. O'Boyle, M. Banck, C. A. James, C. Morley, T. Vandermeersch and G. R. Hutchison, J. Cheminf., 2011, 3, 33 Search PubMed.
- http://lmmd.ecust.edu.cn/admetsar2/, accessed 12 July 2020.
- https://www.epa.gov/chemical-research/toxicity-estimation-software-tool-test, accessed 4 August 2020.
- RCSB PDB, 6M0J, Crystal structure of SARS-CoV-2 spike receptor-binding domain bound with ACE-2, https://www.rcsb.org/structure/6M0J, accessed 6 May 2020 Search PubMed.
- G. M. Morris, R. Huey, W. Lindstrom, M. F. Sanner, R. K. Belew, D. S. Goodsell and A. J. Olson, J. Comput. Chem., 2009, 30, 2785–2791 CrossRef CAS PubMed.
- O. Trott and A. J. Olson, J. Comput. Chem., 2010, 31, 455–456 CAS.
- Dassault Systèmes BIOVIA, Discovery Studio, Dassault Systèmes, San Diego, 2020 Search PubMed.
- Schrödinger Release 2020-2: Induced Fit Docking protocol; Glide, Schrödinger, LLC, New York, NY, 2016 Search PubMed; Schrödinger Release 2020-2: Prime, Schrödinger, LLC, New York, NY, 2020 Search PubMed.
- Schrödinger Release 2020-2: Desmond Molecular Dynamics System, D. E. Shaw Research, New York, NY, 2020 Search PubMed; Maestro-Desmond Interoperability Tools, Schrödinger, LLC, New York, NY, 2020 Search PubMed; Schrödinger Release 2018-3: Prime, Schrödinger, LLC, New York, NY, 2018 Search PubMed.
- Schrödinger Release 2020-2: Prime, Schrödinger, LLC, New York, NY, 2018 Search PubMed.
- A. Sethi, K. Joshi, K. Sasikala and M. Alvala, Drug Discov. Dev. Adv., IntechOpen, 2019 Search PubMed.
- J. Lan, J. Ge, J. Yu, S. Shan, H. Zhou, S. Fan, Q. Zhang, X. Shi, Q. Wang, L. Zhang and X. Wang, Nature, 2020, 581, 1–6 CrossRef PubMed.
- O. M. Bautista-Aguilera, G. Esteban, M. Chioua, K. Nikolic, D. Agbaba, I. Moraleda, I. Iriepa, E. Soriano, A. Samadi, M. Unzeta and J. Marco-Contelles, Drug Des., Dev. Ther., 2014, 8, 1893–1910 Search PubMed.
Footnote |
† Electronic supplementary information (ESI) available. See DOI: 10.1039/d0ra04795c |
|
This journal is © The Royal Society of Chemistry 2020 |
Click here to see how this site uses Cookies. View our privacy policy here.