DOI:
10.1039/D0RA01384F
(Paper)
RSC Adv., 2020,
10, 11971-11981
Atomic force microscopy and Raman spectra profile of blood components associated with exposure to cigarette smoking
Received
12th February 2020
, Accepted 6th March 2020
First published on 24th March 2020
Abstract
Tobacco smoke contains several compounds with oxidant and pro-oxidant properties with the capability of producing structural changes in biomolecules, as well as cell damage. This work aimed to describe and analyse the effect of tobacco smoke on human blood components, red blood cell (RBC) membrane, haemoglobin (Hb) and blood plasma by Atomic Force Microscopy (AFM) and Raman spectroscopy. Our results indicate that tobacco induced RBC membrane nano-alterations characterized by diminished RBC diameter and increased nano-vesicles formation, and RBC fragility. The Raman spectra profile suggests modifications in chemical composition specifically found in peaks 1135 cm−1, 1156 cm−1, 1452 cm−1 and intensity relation of peaks 1195 cm−1 and 1210 cm−1 of blood plasma and by change of peaks 1338 cm−1, 1357 cm−1, 1549 cm−1 and 1605 cm−1 associated with the pyrrole ring of Hb. The relevance of these results lies in the identification of a profile of structural and chemical alterations that serves as a biomarker of physiological and pathological conditions in the human blood components induced by tobacco exposure using AFM and the Raman spectroscopy as tools for monitoring them.
1. Introduction
According to a report from the World Health Organization in 2016, more than 100 million people died worldwide in the last century because of tobacco consumption. Nowadays, tobacco kills around 6 million people each year, and more than 80% of them die because of direct use, and equally important are the numbers for non-smokers exposed to cigarette smoke with close to 600
000 deaths.1
Although cigarette smoking is a preventable risk factor, it is still one of the main causes of developing cancer, as well as cardiovascular, pulmonary and other diseases worldwide.2,3 It is known that acute cigarette smoking increases the risk of disease in healthy young smokers,4 and exacerbated effect is seen on chronic smokers,5 showing the relationship between frequency and quantity of consumption.
Tobacco smoke is a complex mixture of chemicals that contains many active compounds with oxidant and pro-oxidant activity; capable of producing free radicals and increasing the oxidative stress “in vivo”. Besides, some of them are capable of interacting directly with blood components.6–8 The oxidative cell damage is the result of the increased oxidant products, decreased antioxidant protection, and/or the failure to repair the systems in the organism.9 Previous reports indicate that reactive oxygen species (ROS) promote physiological and pathological alterations related to degenerative diseases like cancer and pulmonary and cardiovascular problems.6,10–14 In specific, the ROS alters the membrane lipid bilayer and proteins of the cytoskeleton of cells impairing not only the blood quality, also they could affecting intercellular communications, because some of these structures are identified as important and critical players of this process. Currently, it is possible to detect nano-scale changes in early stages of damage and erythrocytes are emerging as a biomonitor for this goal.15,16 Nevertheless, a few studies are focused on determining the effects of tobacco cigarette on blood components, such as in RBC or plasma.17–20
AFM is useful to characterise the changes in cells to identify profiles that could indicate the development of a disease or even support the diagnosis.21–24 Recently, it was reported that the high cigarette-consumption increased membrane roughness of RBC due to changes on its surface.25 Biophysical changes at the cellular level should also include the evaluation of chemical alterations that cause them. The Raman spectroscopy is a powerful tool that provides identification of ‘‘molecular fingerprints’’, such as glucose, lipid profile, amino acids, and total protein, among others. Quantitative and qualitative changes of these molecules are related to numerous pathologies.26–28 There is a need to identify early biomarkers of exposure for cigarettes29,30 and the AFM and Raman spectroscopy presents great potential in identifying the profiles of different stress conditions and diseases that can be used for differentiation.31,32 Additionally, analysis of RBC by Raman spectroscopy and AFM compared with other biological samples has significant advantages, especially in the cases when we need repeatedly sample collection. We propose to evaluate simultaneously structural and chemical changes in RBC and blood plasma, induced by cigarette smoke exposure using AFM and Raman spectroscopy to identify potential biomarkers in healthy individuals.
2. Experimental
2.1. Volunteers
Inquiry was applied to volunteers in order to inquest personal and relevant information about their health status and cigarette consumption. Then, seventeen asymptomatic volunteers were included. Eleven of them were smoker volunteers separated in three groups. Four volunteers (3 males and 1 female) with a mean consumption of less of five cigarettes per day (smokers <5, light daily smokers); four volunteers (3 males and 1 female) with a mean consumption between five and nine cigarettes per day (smokers 5–9, moderate smoker) and three volunteers (3 males) with a consumption of ten or more cigarettes (smokers >10, moderate smokers). Smokers participants range in age from 19 to 24 years old with a mean age of 21.36 ± 1.44 years old and a body mass index (BMI) of 23.60 ± 3.51. Six non-smoker participants (non-smokers) were considered as the control group (3 males and 3 females). Their age ranged from 21 to 23 years old, with a mean age of 21.83 ± 0.98 years old and a BMI of 22.60 ± 2.14.
2.2. Samples
A total blood sample was collected from each participant using BD Vacutainer K2 EDTA tubes, following standard sampling protocol. Smears of RBC were prepared, using conventional clinical methods. Dried, un-fixed, and un-stained blood smears were used for AFM scanning. Also, cell suspensions at pH 7.4 were prepared with phosphate buffer solution (PBS) (2.7 mM of KCl, 10 mM of NaHPO4 and 1.8 mM of KH2PO4). For Raman spectroscopy analysis, RBC suspension was diluted 1
:
50 and 50 μL was dropped into poly-L-lysine-coated (0.01% v/v) silice substrate. Finally, 1 mL of total blood was centrifuged to obtain plasma, and 0.5 mL aliquot was frozen to −20 °C for further analysis.
2.3. RBC nano-structure analysis by AFM
Nano-structure of RBC membrane was analysed from images reconstructed by AFM. All analyses were conducted in non-contact mode with an AFM model Alpha300RA (WiTec, Germany). The probe had a spring constant of 42 N m−1 and a resonant frequency of 285 kHz. An optical microscope was used to select the cells zone. Cells from each smear were randomly selected and scanned for membrane analysis. To obtain the whole cell and its shape analysis, we performed 50 × 50 μm scans for each specimen, and 60 erythrocyte membrane fragments in each group (1.5 × 1.5 μm scans) were finally analysed. The total scanning points per image were 65
536; the images and their profiles were analysed in 3D and 2D modes, respectively, with the software of WiTec project FOUR v4.1.
2.4. “In vitro” RBC osmotic fragility
RBC osmotic fragility was evaluated by the Faulkner and King method33 using hypotonic conditions in PBS, pH 7.4. Briefly, 75 μL of whole blood were added to 1425 μL (1
:
20) of PBS. Then, 50 μL of cell suspension were added to 200 μL of saline solution (from 0 to 9 g L−1) and incubated for 30 min at room temperature (RT) in 96 wells plate. Afterwards, they were centrifuged at 2000 rpm for 10 min, and 100 μL of supernatant were recovered. The absorbance was scanned from 350 to 600 nm (spectrophotometer Synergy HTX, BioTek, Winooski, VT). The haemolysis percentage was determined from free Hb using the Harboe method34 and the three wavelengths polychromatic formula: Hbfree = 1.68A415 − 0.93A380 − 0.73A470.
2.5. Blood plasma Raman spectroscopy
The blood plasma samples were thawed immediately before the analysis with Raman spectroscopy, following the protocol proposed by Li P. et al.,.35 Raman spectra were recorded with a Confocal Raman Microscope model Alpha300RA (WiTec, Germany). The analysis was performed with a 532 nm laser, using a single 1200 lines per mm dispersion grating. A 100× objective was used to collect the back-scattered radiation. The spectra were acquired in static mode, with a centre at 1500 cm−1 to cover the range 525 cm−1 to 2350 cm−1, with the spectral resolution of 1 cm−1. We used 20 mW laser power on the sample and 2 × 10 seconds acquisition time to obtain a better signal to noise ratio in each spectrum. The instrument was calibrated by silice at 520 cm−1 before Raman scanning.35 Each spectrum was background subtracted by a line function in the 600–1800 cm−1 region and normalized to peak 1005 cm−1. An average spectrum was calculated for representative images but individual spectra were considered for statistical analysis (n = 170).
2.6. Hb RBC Raman spectroscopy
The changes in Raman spectra of smokers' Hb RBC were analysed according to Acosta-Elias M. et al.36 Raman measurements were carried out using solid-state laser excitation of 532 nm with 20 seconds of accumulation time and 2 mW of laser power and 1 μm spot size. The Raman analysis was performed from at least 20 RBC. Each spectrum was background subtracted by a line function in the 600–1800 cm−1 region and normalized to peak 775 cm−1. An average spectrum was calculated for representative images but the total of individual spectra were considered for statistical analysis (n = 340).
2.7. Statistical analysis
The Anderson-Darling normality test was done. The statistical analysis for membrane changes roughness, nano-vesicles formation, osmotic fragility test, and intensity relation of peaks was performed using the non-parametric Kruskal–Wallis test and Mood's median test. While RCB diameter was analysed by One-Way ANOVA test. The significance level (alpha) was set at 0.05. The principal component analysis (PCA) was used to differentiate between Raman spectra of smoker and non-smoker volunteers. All statistical values were calculated using environment R version 3.0.33 (http://www.R-project.org).
3. Results and discussion
3.1. RBC nano-structure analysis by AFM
Cell morphology is a good indicator of damage when changes arise from metabolic process modifications.37 Moreover, disturbances in the micro-rheological properties can produce consequences in function and biophysical parameters for the RBC that can lead to physiological and pathological conditions.38 Previous studies refer that structural changes can promote erythrocyte shape conversion.20,38,39 Here, we analysed the effect of smoke cigarette consumption on RBC membrane. In our analysis, no shape changes were observed in RBC (data not showed); however, membrane changes at nano-level were detected in smoker cigarette groups. Fig. 1 shows representative RBC membrane of non-smoker and smoker participants. The Fig. 1a illustrates the smokers' RBC topography with the calculated mean square roughness (nm) ± standard deviation. RBC membrane roughness in non-smokers was found as 3.410 ± 0.659 nm, while the calculated roughness for smokers were as follow: 3.961 ± 0.773 nm in smokers <5, 4.057 ± 1.137 nm in smokers 5–9 and 3.551 ± 0.799 nm in smokers >10. Although the average RBC membrane roughness was increased in smokers, not significant differences were found. The profiles RBC surfaces showing the nano-depressions are showed in Fig. 1b. Masilamani V. et al.,25 reported an increase membrane roughness in erythrocytes of smokers (5.5 ± 3.1 nm) compared with the erythrocytes of non-smokers (1.2 ± 0.2 nm). The differences in our results could be explained by the frequency and quantity of cigarette consumption. Since Masilamani V. et al.,25 reported a mean consumption of 24 cigarettes, and our participants can be considered from light daily to moderate smokers and not as heavy smokers as considered by Masilamani V. et al. It has been reported that quantity and frequency of consumption of cigarettes can influence on the cell damage.9,40 Another reason for the differences can be the age of volunteers. Oxidative stress parameters of middle-aged and older subjects suggests a progressive and slow decline of antioxidant status in healthy elderly with an increase in plasma SH group oxidation.41 The formation of nano-structures on surface RBC has been associated with oxidative processes and the reduction of antioxidant activity.42,43 The smoke cigarette diminishes enzymes with antioxidant activity, and by itself, the smoke presents oxidant activity,44 and there is evidence that smoking increases the ageing process inducing changes on the cells and impacting in the capability of RBC to deform.45–48 We examined the number of nano-vesicles in the analysed membrane surface of smoker volunteers. We observed an increase in the number of nano-vesicles of smokers <5 (1.73 ± 1.52), and smokers 5–9 (1.34 ± 1.30), but only smokers >10 (2.2 ± 1.65) were statistical different (p < 0.01) compared with non-smokers (0.73 ± 0.78). The vesiculation phenomenon was previously reported in smokers by Pretorius E. et al.,20 while Acosta-Elias M.49 and AlZahrani K. et al.,43 reported the formation of nano-vesicles provoked by gamma radiation, that induces similar conditions like oxidative stress than cigarette smoke.
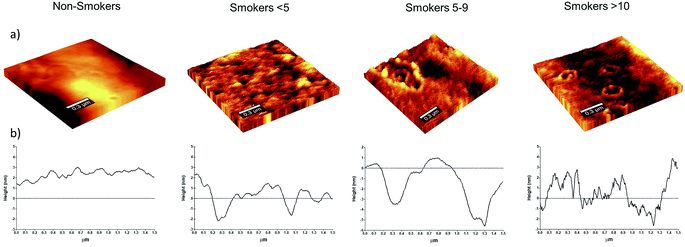 |
| Fig. 1 RBC membrane by AFM. (a) Nano-structure of RBC membrane of non-smokers and smokers volunteers and mean square roughness (mean ± SD), n = at least 15 cells per group. (b) Profile line showing membrane depressions. | |
Our results show the changes in RBC at nano-level with nano-vesicles formation in smokers with a consumption of ten cigarettes. A drastic alteration in roughness is associated to membrane-skeletal alterations. Although not significant differences were found, we identified a slight increased in membrane roughness of smoker's volunteers. Interestingly, we observed a recovering of membrane roughness in smoker >10 to the level of non-smokers that coincide with the maximum number of nano-vesicles formation. Leal J. et al., describe in a review that RBC generate micro-vesicles to remove damaged cell constituents such as oxidized haemoglobin and damaged membrane constituents. This damage leads to a weakening of the binding between the lipid bilayer and the cytoskeleton, and thereby to membrane micro-particle shedding.50 These structures, as vesicles, were reported previously by SEM and AFM analysis and named as ‘‘bubble-like’’ extensions that are present all around on RBC membrane surfaces of smokers in combination with a decreased roughness.20 This fact has been also related with the ageing process where the formation of vesicles provoked the loss of membrane fragments of RBC reducing the surface roughness and becoming to the cell more fragile.51 During storage, as well as oxidative process, membrane lipid oxidation and cytoskeletal protein oxidation can dislocate the plasma membrane and cytoskeleton.52,53 This micro-vesiculation could be considered irreversible storage lesions with oxidation of proteins and deformability of RBC.52,54 In order to determine if nano-vesicles formation had an impact in the RBC, we analysed RBC diameter (μm). Our analysis revealed statistical differences (P < 0.001) in the RBC diameter (mean ± SD) of smokers 5–9 (8.32 ± 0.68) and smokers >10 (8.68 ± 0.66) compared with non-smokers (9.50 ± 0.70), while in smokers <5 (9.62 ± 0.75) no differences were found. This indicates that nano-vesicles formation and its shedding can be related with diminish RBC size, as previously reported.55 Cigarette induced changes in structure by nano-vesicles formation and diminish RBC size that could be considered as an accelerated ageing cells process and inducing a lost of RBC deformability.45–48
3.2. “In vitro” RBC osmotic fragility
Studies have shown that the deformability of RBC decreases progressively during oxidative exposure,37,56 increasing the fragility of RBC and amplifying the erythrocyte haemolysis.57 In this phenomenon, the generation oxidative stress leads to the formation of nano-structures.42,43 Previous reports indicate that consumption of cigarette compromise the structure of RBC20 becoming it more fragile.58–60 As previous work reported,25 the tobacco smoke presents the capability of inducing changes that increase the haemolysis of the RBC.25 We performed an osmotic shock test to defy the endurance of RBC membrane of our smoker groups with stressing saline solution (NaCl) at different concentration. Fig. 2 shows that near to physiological conditions there was not differences among groups. Nevertheless, we found a significant increase (p < 0.001) in the haemolysis percentage in smokers 5–9 and smokers >10 compared with non-smokers when cells were challenged with 3.6 g L−1 and 2.6 g L−1 of NaCl. No differences were observed in smokers <5 compared with non-smokers. Previously, Asgary et al.,7 showed that smokers RBC were more susceptible to haemolysis compared with non-smokers. Here, the appearing of early haemolysis of smoker's volunteers (smokers 5–9 and smokers >10) at 3.6 g L−1 of NaCl compared with non-smokers controls corroborates that cigarette smoke increases the fragility of RBC membrane indicating that changes observed in membrane RBC (nano-vesicles formation and RBC diameter diminished) can be related with the deterioration of the structure of erythrocytes.
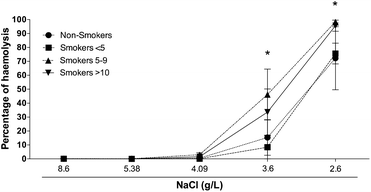 |
| Fig. 2 RBC osmotic fragility. The percentage of haemolysis increases significantly (p < 0.001) in the RBC of smokers 5–9 and smokers >10 in comparison with RBC of non-smoker participants. | |
3.3. Raman spectroscopy of blood plasma
Raman spectroscopy is a technique that follows molecular dynamics. Raman spectroscopy of body fluids is an alternative method that minimizes invasion in the analysis compared with others.61–64 Blood plasma contains several biomolecules as proteins, lipids, glucose, vitamins, hormones, and inorganic materials, as well as waste products of the metabolism; the changes in these biomolecules can be very good indicators of the health status of a person.65,66 Although blood plasma Raman spectrum it is complex to obtain without Surface-Enhanced Raman Spectroscopy (SERS), there are some successful examples for it.35,67,68 Fig. 3 shows the obtained Raman spectra of blood plasma. The most intense bands observed were 1005 cm−1, 1156 cm−1 and 1517 cm−1 corresponding to carotenoid assignments, due to the enhance resonance of this structures induced by the used laser in our experiment.69,70 We observed a significant increase in peak 1156 cm−1 associated to carotenoid and proteins vibrations in smokers >10. It is known that carotenoids are antioxidant obtained from diet and that they are stored in lipid tissue.71,72 The importance of this finding arise in the possibility that its release to the blood plasma it is related with protection against the oxidative effect of smoke cigarette.73 A new peak (at 1195 cm−1) appears in smoker's blood plasma spectra as a deformation of peak at 1210 cm−1 that is associated to C–C6H5 stretching mode in tyrosine and phenylalanine74 and ν18 (δ: CmH).73,75 The intensity relation between peaks 1210 cm−1 and 1195 cm−1 was significantly (p < 0.05) modified in smokers (Fig. 3). The mean relation intensity and standard deviations were found as follow: non-smokers (2.32 ± 0.33), smokers <5 (1.14 ± 0.13), smokers 5–9 (1.21 ± 0.38), smokers >10 (0.99 ± 0.16). This result indicates that peak 1210 cm−1 is being deformed by smoke cigarette. The band 1664 cm−1 was decreased only in smokers 5–9 and smokers >10 compared with non-smokers and smokers <5. The band 1664 cm−1 is named amide I and corresponds to the sum of coupled modes of the polypeptide backbone, the major contribution to the amide I modes comes from the C
O stretching of the peptide carbonyl groups.76,77
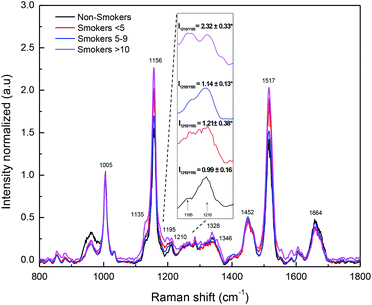 |
| Fig. 3 Average of Raman spectra of blood plasma. Black line represents the average of non-smokers (n = 6, no. of spectra 100), red line represents the average of smokers <5 (n = 4, no. of spectra 40). Blue line represents the average of smokers 5–9 (n = 4, no. of spectra 40) and magenta line represents the average smokers >10 (n = 3, no. of spectra 30). Only relevant peaks from analysis are marked. Intensity relation between peak 1210/1195 is showed in figure amplification. Asterisks indicate significant differences compared with non-smokers (p < 0.05). | |
Principal Component Analysis (PCA) is useful to reduce the dimensionality of the original data matrix retaining the maximum amount of variability. Besides, PCA allows samples visualization in a two dimensional space identifying the directions in which most of the information is retained. Then, it is possible to explain the differences among samples by means of factors from data sets and, at the same time, to determine which variables contribute the most for such differences.78 We examined the scores and loadings for the most important PCs, as determined from percentage variance plots, were used to investigate changes in the spectral features of Raman data. First, a multivariate analysis of variance was carried out finding significant difference among groups (Wilks = 0.0367, F = 6.82 p-value = 0.00, Lawley–Hotelling = 7.06, F = 10.45, p-value = 0.00). Next, the first derivative of the variance of Raman spectra bands was calculated to identify the signals with maximum variance among groups. We found that the bands with major variance were those between 1100 cm−1 to 1600 cm−1. Once the major variance bands were selected, the PC analysis was performed and based on correlation matrix. Examination of the cumulative variance revealed that two components reflected 92.7% (PC1 and PC2) of the variance of the system. The coefficient that defines the weight of the original variable in the PC's can be investigated to understand which bands are responsible for the ranking of samples. P1135 (−0.6974), P1156 (−0.9247), P1328 (0.2131), P1346 (0.6107), P1452 (0.9054), and P1664 (0.9713) were highly loaded on the first PC. While, P1135 (0.6276), P1156 (0.2999), P1328 (0.8549), P1346 (0.6642), P1452 (0.1387), and P1664 (0.0017) were loaded on the second PC explaining most of the variability. The Fig. 4 shows the plot of the first PC versus the second PC of the blood plasma Raman spectra for smoker and non-smoker volunteers. No differences were found between smokers <5 and non-smokers. Although, not obvious clusters were observed, separated plots of smokers >10, including one volunteer of smokers 5–9 were identified. We consider that this lack of clustering can be due to complexity of the sample and small sample. However, the separation of four of the six smokers 5–9 and smokers >10 (moderate smokers) from the non-smokers (controls) and smokers <5 (light smokers) is indicator that some changes in components of blood plasma are occurring. It could be interesting to prove if SERS amplify the spectrum and discriminate more efficiently between smokers and non-smokers, since it have been reported that blood plasma spectrum is significantly improve using this procedure.66,79–81 The main contribution to PC was due to peaks 1135 cm−1, 1156 cm−1, and 1452 cm−1. Researchers have been used the intensity of band 1135 cm−1 (due to the C–O stretching mode) to estimate glucose concentration artificially added to serum blood.82 Our data show an increase of intensity of this band indicating that glucose is increased in smoker group compared with our non-smoker volunteers. This fact was reported previously by Bornemisza P. and Suciu I., 198083 who determined that glucose concentration increase in smokers. The band 1156 cm−1 is associated to ν(C–N), proteins (protein assignment) and ν(C–C), carotenoid.84,85 The band 1452 cm−1 associated to CH2CH3 deformation and proteins. While, the band 1664 cm−1 have been associated with amide I in cell samples.77 A study showed that cigarette smoke extract causes conformational changes in protein.86 Specific components of smoke cigarette have been also analysed observing that they are capable to interrupt the protein-receptor interaction.87 Proteins are also target of reactive oxygen species altering the structure and function.88 Previous reports showed that smoke cigarette induces changes in the profile of proteins of sperm and epididymis and fraction proteins in serum.89–91 Changes in peaks associated to proteins in our experiments may indicate that cigarette consumption is affecting the compounds of blood plasma including proteins in it, although as it was reported, the susceptibility of compounds is differentially affected,92,93 and further investigation is needed to determine if SERS procedure improve the spectra in blood plasma analysis between smokers and non-smokers and which components have been specifically affected.
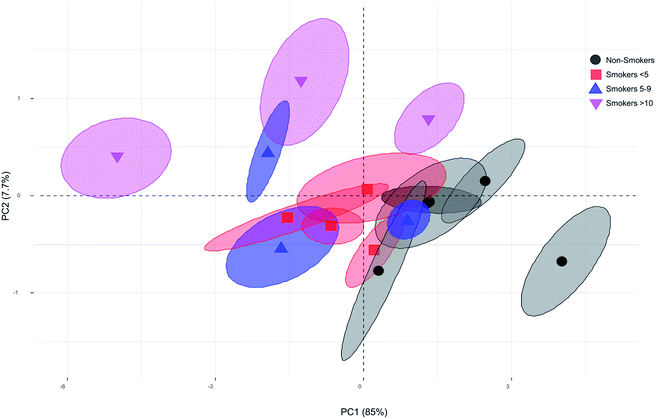 |
| Fig. 4 Score plot PC1 vs. PC2 for blood plasma. Ellipses are confidant area. Black circles are non-smokers; red squares are smokers <5; blue triangles are smokers 5–9; magenta inverted triangles are smokers >10. | |
3.4. Raman spectroscopy of smokers' RBC
We examined the potential structural changes and oxygenation states in haemoglobin (Hb) associated with different vibration mode in smoke cigarette volunteers by Raman Spectroscopy. Our results are in agreement with other studies about Raman spectrum of single blood cells reported with similar results to ours.94–97 This technique has been used to study changes in Hb structure associated to functionality.98,99 The Hb spectrum was analysed from 600 cm−1 to 1800 cm−1 region looking for displacements peak, peak intensity changes and the comparison of haemoglobin derivatives such as oxygenated and deoxygenated states. Fig. 5a shows the mean values of typical Hb Raman spectra from RBC obtained with a laser excitation of 532 nm. We present the most important differences in the Raman spectrum of erythrocyte profiles between non-smokers and smokers. Significant changes were observed in the region of 1000 cm−1 to 1800 cm−1. As we observed in blood plasma, smoke cigarettes affects some Hb molecule structures depending on consumption. We observed a displacement of the band 1120 cm−1 to 1130 cm−1 in smokers >10 compared with non-smokers (Fig. 5b). This band is associated to asymmetric pyrrole half-ring stretching vibrations corresponding to vibration ν22.97 In Fig. 5c, we present the increase in the peak at 1150 cm−1 associated to ν(Pyr half-ringasym) in the smokers 5–9 and smokers >10. This change was accompanied with the formation of a new peak at 1145 cm−1 (unassigned peak) in smoker >10. In contrast, a decrease was observed at the region of 1400–1300 cm−1 (Fig. 5d) associated with vibration modes of pyrrolic ring stretching, the comparison of the non-smokers with smokers >10 shows a diminishing in the intensity and a shape change in peak at 1365 cm−1. Additionally, a significant diminishing was observed in the intensities of peaks at 1336 cm−1, 1357 cm−1 associated with vibrations ν41 and ν4d(Pyr half-ringsym), respectively. While in region of the spin state of Fe in the protoporphyrin, the peak 1547 cm−1 and 1605 cm−1 associated with vibrations ν11(CβCβ)67 and ν19, respectively (Fig. 5e). No changes were observed between non-smokers and smoker <5, indicating that exposure it is important to modify the Hb spectrum. Previous reports measured the haemoglobin oxygen saturation showing that peaks of 1375 cm−1, 1590 cm−1, 1640 cm−1 would increase with high O2 saturation.96,100,101 We also monitored the oxygenation states, which are associated with different mode vibrations in the Raman spectra using the bands ν4, ν10 and ν13. These bands are present in the oxygenation state of Hb.49 In our experiment, no changes associated with oxygenation states of Hb were observed. We hypothesize that the amount of cigarettes consumed by our volunteers is not enough to make changes the capacity of the function of Hb, and as reported before, these peaks did not appear increased because the O2 saturation of the blood is too low.100
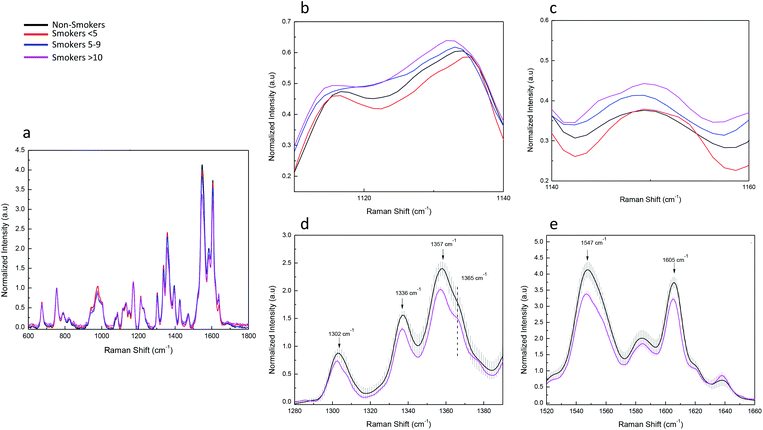 |
| Fig. 5 Analysis of Raman spectroscopy of erythrocytes. Black line represents the average of non-smokers participants (n = 120). Red line represents the average of smokers <5 (n = 80), blue line represents the average of smokers 5–9 (n = 80). The magenta line represents the average of smokers >10 (n = 60). | |
The principal component analysis (PCA) was used to successfully differentiate between smoker and non-smoker volunteers. The same strategy was follow for haemoglobin as blood plasma Raman spectra data. The multivariate analysis of variance shows significant difference among groups (Wilks = 0.00285, F = 6.275, p-value = 0.00, Lawley–Hotelling = 12.99779, F = 10.688, p-value = 0.00). Then, the first derivative of the variance of Raman spectra bands was calculated to identify the signals with maximum variance among groups. We found that the bands with major variance were those between 1300 cm−1 to 1606 cm−1 (not showed). In this case, the PC analysis and cumulative variance revealed that PC1 and PC2 reflected 91.8% of the variance of the system. The coefficient that defines the weight of the original variable in the PC's can be investigated to understand which bands are responsible for the ranking of samples. P1338 (0.8354), P1357 (0.9188), P1549 (0.9501) and P1606 (0.8951) were highly loaded on the first PC. While, P1338 (0.5511), P1357 (−0.0708), P1549 (−0.1010) and P1606 (−0.3283) were loaded on the second PC explaining most of the variability. The Fig. 6 shows the plot of the first PC versus the second PC of the Raman spectra of Hb from smokers and non-smokers. As we observed for blood plasma the non-smokers and smokers <5 were found together in the first quadrant, but in this case, two of four smokers 5–9 and all smokers >10 were found separated and clustered in the third quadrant. These results indicate that non-smokers and smokers <5 have more intense bands than smokers 5–9 and smokers >10 and that selected band for PCA are good indicators for moderate exposure to smoke cigarette by haemoglobin analysis. The non-smokers and smokers <5 have positive value of PC2, while smokers 5–9 and smokers >10 have negative values (Fig. 6). The first component (PC1) is a weighted sum of the bands corresponding to the core size and three principal modes assigned to pyrrole ring stretching vibrations (1300 cm−1 to 1650 cm−1).97 The second component (PC2) contrasts the bands in the core size (or spin state marker) with the principal modes assigned to pyrrole ring stretching vibrations. Taking into account the coefficients of the variables in PC2, it can assume that the non-smokers and smokers <5 have a greater amplitude into pyrrole ring stretching modes than smokers 5–9 and smokers >10. We infer that, haemoglobin structure from smokers 5–9 and smokers >10 are different compared with non-smokers and smokers <5. By taking advantage of PCA, we can see the most significant differences in the Raman spectra of the smokers 5–9 and smokers >10 (moderate smokers) compared with non-smokers, thus providing strong support for the establishment of the standard method of exposure differentiation based on haemoglobin quality analysis.
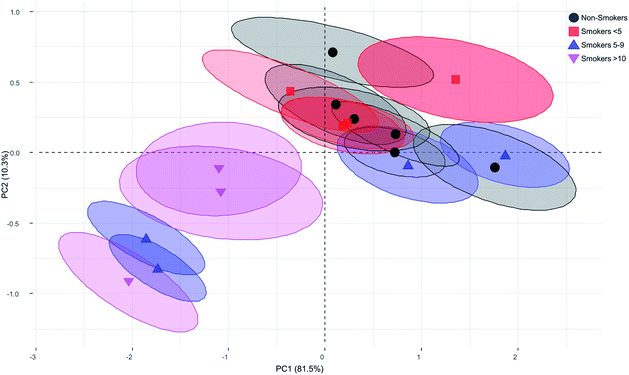 |
| Fig. 6 Score plot PC1 vs. PC2 for haemoglobin. Ellipses are confidant area. Black circles are non-smokers controls; red squares are smokers <5; blue triangles are smokers 5–9; magenta inverted triangles are smokers >10. | |
Biological markers for the assessment of exposure to a variety of environmental carcinogens have been widely used in both basic and clinical research. There is a need to find new markers to understand the molecular determinants of diseases or stress process.102,103 The exposure to tobacco smoke presents an ideal environment to find, characterize, and refine biological markers, especially for those carcinogens found in tobacco.29,30,93 Changes in secondary and tertiary structures of Hb in cigarette smokers and impaired function have been described previously104 Additionally, the finding subtle changes in proteins as amino acid residues moved toward or behind more hydrophobic region in biomarkers, in addition to impaired amino acid interaction with each other and their environment, could be a sign of an increased susceptibility to disease or risk. In the preventive medicine, to find this kind of biomarkers is mandatory. Table 1 summarize the profiles of AFM and Raman spectrum of smoker volunteers. As we have shown, it is possible to identify changes in the membrane and Hb structure of RBC, and in blood plasma spectra, using AFM and Raman spectroscopy. Tobacco smoke is altering the concentrations of some components of the blood plasma, as observed by increase in the intensity of specific peaks associated to previous indicator as glucose. In addition, we observed that there are changes in the structure of some of the compounds (mainly proteins, by the vibrational modes observed). We also identified changes in the shape of the peaks associated with these components in the blood plasma, coupled with the change in the structure of haemoglobin by changes, not only in the peaks of the haem group, but also on the pyrrole structure. These results indicate that tobacco smoke exposure could be monitored even at low consumption cigarette doses and that blood plasma and RBC are good indicators of this exposure. Our work could be used as basis for future analyses in order to identify the possible biomarkers associated with tobacco consumption and in some way associate them with the particular risk of each exposed person.
Table 1 AFM and Raman spectrum profiles of smoker volunteers
Analysis |
Parameters |
Non-smokers |
Smokers (cigarettes consumption) |
<5 |
5–9 |
>10 |
AFM parameter |
Nano-vesicles |
|
|
|
Increased |
RBC diameter |
|
|
Diminished |
Diminished |
RBC fragility |
Haemolysis (%) |
|
|
Increased |
Increased |
Raman blood plasma peaks (cm−1) |
I1210/1195 |
|
Diminished |
Diminished |
Diminished |
1135 |
|
|
Increased |
Increased |
1156 |
|
|
|
Increased |
1328 |
|
|
|
Increased |
1346 |
|
|
|
Increased |
1452 |
|
|
|
Increased |
1664 |
|
|
Diminished |
Diminished |
Raman Hb peaks (cm−1) |
1120–1130 |
|
|
Displacement |
Displacement |
1145 |
Absent |
Absent |
Absent |
Present |
1150 |
|
|
Increased |
Increased |
1302 |
|
|
|
Diminished/shape change |
1336 |
|
|
|
Diminished |
1357 |
|
|
|
Diminished |
1365 |
|
|
|
Diminished/shape change |
1547 |
|
|
|
Diminished |
1605 |
|
|
|
Diminished |
4. Conclusions
As we can see in our results, AFM and Raman spectroscopy are very good techniques that require little sample preparation for the analysis. We present the changes in RBC membrane as diminishing RBC diameter and increasing formation of nano-vesicles, and RBC fragility. These results confirm previous evidence of structural damage in RBC induced by smoke cigarette analysed by AFM. Additionally, and for the first time, we report the changes in Raman spectra profile of blood plasma and Hb RBC, which can be associated with composition and structural changes in blood plasma compounds and haemoglobin of young healthy smokers. The chemical changes were found specifically on peaks 1135 cm−1, 1156 cm−1, 1452 cm−1 and relation of peak 1195 cm−1 and 1210 cm−1 of blood plasma and by change of peaks 1338 cm−1, 1357 cm−1, 1549 cm−1 and 1605 cm−1 associated to pyrrole ring of Hb and allows differentiate between non-smokers and moderate smokers cigarette exposure by Raman spectroscopy. This imply that both techniques can be used to monitoring early damage in red blood cells induced by tobacco smoke at moderate exposure in asymptomatic volunteers using suitable parameters as biological markers.
Compliance with ethical standards
The experiments of this work were approved by the Ethics Committee of Sonora University, and complied with institutional standards and the principles expressed in the declaration of Helsinki. Informed consents were obtained from participants of this study.
Conflicts of interest
The authors declare that they have no competing interests.
Acknowledgements
We are grateful to the CONACYT and SEP of Mexico for financial support, under projects PDPCPN-2014-248982, INFR-2016-01-268666, INFR-2018-01-293642, and PRODEP/INPTC/511-6/17-13125 for the grant of Victor M. Escalante L. We thank to Yanik Deana for his technical support. We acknowledge to Dr Carlos A. Velázquez-Contreras and Dr Amir D. Maldonado-Arce for allowing us to work in their laboratories.
References
- S. A. Glantz and W. W. Parmley, Circulation, 1991, 83, 1–12 CrossRef CAS PubMed.
- C. Iribarren, I. S. Tekawa, S. Sidney and G. D. Friedman, N. Engl. J. Med., 1999, 340, 1773–1780 CrossRef CAS PubMed.
- K. K. Teo, S. Ounpuu, S. Hawken, M. R. Pandey, V. Valentin, D. Hunt, R. Diaz, W. Rashed, R. Freeman, L. Jiang, X. Zhang, S. Yusuf and I. S. Investigators, Lancet, 2006, 368, 647–658 CrossRef.
- A. Mahmud and J. Feely, Hypertension, 2003, 41, 183–187 CrossRef CAS PubMed.
- J. W. Kim, C. G. Park, S. J. Hong, S. M. Park, S. W. Rha, H. S. Seo, D. J. Oh and Y. M. Rho, Blood Pressure, 2005, 14, 80–85 CrossRef CAS PubMed.
- A. L. Bluhm, J. Weinstein and J. A. Sousa, Nature, 1971, 229, 500 CrossRef CAS PubMed.
- S. Asgary, G. Naderi and A. Ghannady, Exp. Clin. Cardiol., 2005, 10, 116–119 CAS.
- S. A. Hulea, R. Olinescu, S. Nita, D. Crocnan and F. A. Kummerow, J. Environ. Pathol., Toxicol. Oncol., 1995, 14, 173–180 CAS.
- P. Mazzone, W. Tierney, M. Hossain, V. Puvenna, D. Janigro and L. Cucullo, Int. J. Environ. Res. Public Health, 2010, 7, 4111–4126 CrossRef CAS PubMed.
- T. Nagamma, K. Anjaneyulu, J. Baxi, P. Dayaram and P. Singh, Asian Pac. J. Cancer Prev., 2011, 12, 313–316 CAS.
- R. S. Barua, J. A. Ambrose, S. Srivastava, M. C. DeVoe and L. J. Eales-Reynolds, Circulation, 2003, 107, 2342–2347 CrossRef CAS PubMed.
- A. Valavanidis, T. Vlachogianni and K. Fiotakis, Int. J. Environ. Res. Public Health, 2009, 6, 445–462 CrossRef CAS PubMed.
- D. Morrison, I. Rahman, S. Lannan and W. MacNee, Am. J. Respir. Crit. Care Med., 1999, 159, 473–479 CrossRef CAS PubMed.
- M. R. Law, J. K. Morris and N. J. Wald, Bmj, 1997, 315, 973–980 CrossRef CAS PubMed.
- J. Khoory, J. Estanislau, A. Elkhal, A. Lazaar, M. I. Melhorn, A. Brodsky, B. Illigens, I. Hamachi, Y. Kurishita, A. R. Ivanov, S. Shevkoplyas, N. I. Shapiro and I. C. Ghiran, PLoS One, 2016, 11, e0141206 CrossRef PubMed.
- K. Hensley, K. A. Robinson, S. P. Gabbita, S. Salsman and R. A. Floyd, Free Radical Biol. Med., 2000, 28, 1456–1462 CrossRef CAS PubMed.
- M. Tsuchiya, A. Asada, E. Kasahara, E. F. Sato, M. Shindo and M. Inoue, Circulation, 2002, 105, 1155–1157 CrossRef CAS PubMed.
- A. Kocyigit, O. Erel and S. Gur, Clin. Biochem., 2001, 34, 629–633 CrossRef CAS PubMed.
- R. M. Johnson, Y. Ravindranath, M. S. ElAlfy and G. Goyette Jr, Blood, 1994, 83, 1117–1123 CrossRef CAS PubMed.
- E. Pretorius, J. N. du Plooy, P. Soma, I. Keyser and A. V. Buys, Nitric Oxide, 2013, 35, 42–46 CrossRef CAS PubMed.
- R. Yang, N. Xi, C. K. Fung, K. Seiffert-Sinha, K. W. Lai and A. A. Sinha, J. Nanosci. Lett., 2011, 1, 87–101 CAS.
- M. Lekka, D. Gil, K. Pogoda, J. Dulinska-Litewka, R. Jach, J. Gostek, O. Klymenko, S. Prauzner-Bechcicki, Z. Stachura, J. Wiltowska-Zuber, K. Okon and P. Laidler, Arch. Biochem. Biophys., 2012, 518, 151–156 CrossRef CAS PubMed.
- P. D. Antonio, M. Lasalvia, G. Perna and V. Capozzi, Biochim. Biophys. Acta, Biomembr., 2012, 1818, 3141–3148 CrossRef PubMed.
- M. Li, D. Dang, L. Liu, N. Xi and Y. Wang, IEEE Trans. NanoBioscience, 2017, 16, 523–540 Search PubMed.
- V. Masilamani, K. AlZahrani, S. Devanesan, H. AlQahtani and M. S. AlSalhi, Sci. Rep., 2016, 6, 21095 CrossRef CAS PubMed.
- A. C. Filho, L. Silveira Jr, A. L. Yanai and A. B. Fernandes, Lasers Med. Sci., 2015, 30, 247–253 CrossRef PubMed.
- J. Griffiths, Anal. Chem., 2007, 79, 3975–3978 CrossRef CAS PubMed.
- F. M. Lyng, D. Traynor, I. R. Ramos, F. Bonnier and H. J. Byrne, Anal. Bioanal. Chem., 2015, 407, 8279–8289 CrossRef CAS PubMed.
- C. M. Chang, S. H. Edwards, A. Arab, A. Y. Del Valle-Pinero, L. Yang and D. K. Hatsukami, Cancer Epidemiol., Biomarkers Prev., 2017, 26, 291–302 CrossRef CAS PubMed.
- K. Strimbu and J. A. Tavel, Curr. Opin. HIV AIDS, 2010, 5, 463–466 CrossRef PubMed.
- M. T. Pacheco and L. M. Moreira, Photomed. Laser Surg., 2013, 31, 463–465 CrossRef PubMed.
- S. Choi, G. B. Jung, K. S. Kim, G. J. Lee and H. K. Park, J. Nanosci. Nanotechnol., 2014, 14, 71–97 CrossRef CAS PubMed.
- R. Faulkner and J. W. King Manual of Clinical Laboratory Procedures, 1970, p. 354 Search PubMed.
- M. Harboe, Scand. J. Clin. Lab. Invest., 1959, 11, 66–70 CrossRef CAS PubMed.
- P. Li, C. Chen, X. Deng, H. Mao and S. Jin, J. Biomed. Opt., 2015, 20, 037004 CrossRef PubMed.
- M. Acosta-Elias, A. Sarabia-Sainz, S. Pedroso-Santana, E. Silva-Campa, K. Santacruz-Gomez, A. Angulo-Molina, B. Castaneda, D. Soto-Puebla, M. Barboza-Flores, R. Melendrez, S. Alvarez-Garcia and M. Pedroza-Montero, Phys. Status Solidi A, 2015, 212, 2437–2444 CrossRef CAS.
- J. R. Hess, R. L. Sparrow, P. F. van der Meer, J. P. Acker, R. A. Cardigan and D. V. Devine, Transfusion, 2009, 49, 2599–2603 CrossRef PubMed.
- V. V. Moroz, A. M. Chernysh, E. K. Kozlova, P. Y. Borshegovskaya, U. A. Bliznjuk, R. M. Rysaeva and O. Y. Gudkova, J. Crit. Care, 2010, 25, 539.e1–539.e12 CrossRef PubMed.
- P. Zachee, J. Snauwaert, P. Vandenberghe, L. Hellemans and M. Boogaerts, Br. J. Haematol., 1996, 95, 472–481 CrossRef CAS PubMed.
- J. Zhang, Y. Liu, J. Shi, D. F. Larson and R. R. Watson, Exp. Biol. Med., 2002, 227, 823–829 CrossRef CAS PubMed.
- M. Andriollo-Sanchez, I. Hininger-Favier, N. Meunier, E. Venneria, J. M. O'Connor, G. Maiani, C. Coudray and A. M. Roussel, Eur. J. Clin. Nutr., 2005, 59(Suppl 2), S58–S62 CrossRef CAS PubMed.
- C. Kozlova capital Ie, C. Chernysh capital A, V. Moroz, V. Sergunova, C. Gudkova capital O and C. Kuzovlev capital A, Exp. Cell Res., 2015, 337, 192–201 CrossRef PubMed.
- K. AlZahrani and H. A. Al-Sewaidan, Indian J. Hematol. Blood Transfus., 2017, 33, 109–115 CrossRef PubMed.
- B. Isik, A. Ceylan and R. Isik, Inhalation Toxicol., 2007, 19, 767–769 CrossRef CAS PubMed.
- K. A. Ward, C. Baker, L. Roebuck, K. Wickline and R. W. Schwartz, Age, 1991, 14, 73–77 CrossRef.
- H. Abe, M. Orita and S. Arichi, Mech. Ageing Dev., 1984, 27, 383–390 CrossRef CAS PubMed.
- J. M. Norton and P. W. Rand, Blood, 1981, 57, 671–674 CrossRef CAS PubMed.
- K. Salbas, Scand. J. Clin. Lab. Invest., 1994, 54, 411–416 CrossRef CAS PubMed.
- M. A. Acosta-Elias, A. J. Burgara-Estrella, J. A. i. Sarabia-Sainz, E. Silva-Campa, A. Angulo-Molina, K. J. Santacruz-Gomez, B. Castaneda, D. Soto-Puebla, A. I. Ledesma-Osuna, R. Melendrez-Amavizca and M. Pedroza-Montero, Int. J. Radiat. Biol., 2017, 93, 1306–1311 CrossRef CAS PubMed.
- J. K. F. Leal, M. J. W. Adjobo-Hermans and G. J. C. G. M. Bosman, Front Physiol., 2018, 9, 1–7 Search PubMed.
- M. Girasole, G. Pompeo, A. Cricenti, G. Longo, G. Boumis, A. Bellelli and S. Amiconi, Nanomedicine, 2010, 6, 760–768 CrossRef CAS PubMed.
- R. Almizraq, J. D. R. Tchir, J. L. Holovati and J. P. Acker, Transfusion, 2013, 53, 2258–2267 CAS.
- J. G. Mohanty, E. Nagababu and J. M. Rifkind, Front Physiol., 2014, 5, 1–6 Search PubMed.
- M. Prudent, J. D. Tissot and N. Lion, Transfus. Apher. Sci., 2015, 52, 270–276 CrossRef PubMed.
- A. Ciana, C. Achilli, A. Gaur and G. Minetti, Cell. Physiol. Biochem., 2017, 42, 1127–1138 CrossRef CAS PubMed.
- V. V. Moroz, A. M. Chernysh, E. K. Kozlova, V. A. Sergunova, O. E. Gudkova, S. E. Khoroshilov, A. D. Onufrievich and A. I. Kostin, Bull. Exp. Biol. Med., 2015, 159, 406–410 CrossRef CAS PubMed.
- S. W. Lee and H. S. Ducoff, Radiat. Res., 1994, 137, 104–110 CrossRef CAS PubMed.
- W. A. Pryor and K. Stone, Ann. N. Y. Acad. Sci., 1993, 686, 12–27 CrossRef CAS PubMed ; discussion 27-18..
- M. Mazhar, J. Uzma, B. Mumtaz and N. Naim Ahmad, Biomed. Sci., 2017, 3, 58–62 CrossRef.
- P. Padmavathi, V. D. Reddy, G. Kavitha, M. Paramahamsa and N. Varadacharyulu, Nitric Oxide, 2010, 23, 181–186 CrossRef CAS PubMed.
- D. N. Artemyev, A. Bratchenko, Y. A. Khristoforova, A. A. Lykina, O. O. Myakinin, T. P. Kuzmina, I. L. Davydkin and V. P. Zakharov, Proc. SPIE, 2016, 9887 Search PubMed.
- A. M. K. Enejder, T. W. Koo, J. Oh, M. Hunter, S. Sasic, M. S. Feld and G. L. Horowitz, Opt. Lett., 2002, 27, 2004–2006 CrossRef CAS PubMed.
- Y. Z. Li, R. Chen, L. Liu, S. Y. Feng and B. H. Huang, Proc. Soc. Photo-Opt. Instrum. Eng., 2005, 5630, 229–234 CrossRef.
- E. Al-Hetlani, L. Halamkova, M. O. Amin and I. K. Lednev, J. Biophot., 2019, e201960123, DOI:10.1002/jbio.201960123.
- N. Kuhar, S. Sil, T. Verma and S. Umapathy, RSC Adv., 2018, 8, 25888–25908 RSC.
- A. Bonifacio, S. Cervo and V. Sergo, Anal. Bioanal. Chem., 2015, 407, 8265–8277 CrossRef CAS PubMed.
- C. G. Atkins, K. Buckley, M. W. Blades and R. F. B. Turner, Appl. Spectrosc., 2017, 71, 767–793 CrossRef CAS PubMed.
- H. F. Nargis, H. Nawaz, A. Ditta, T. Mahmood, M. I. Majeed, N. Rashid, M. Muddassar, H. N. Bhatti, M. Saleem, K. Jilani, F. Bonnier and H. J. Byrne, Spectrochim. Acta, Part A, 2019, 222, 1–8 CrossRef PubMed.
- M. Tatarkovic, M. Miskovicova, L. Stovickova, A. Synytsya, L. Petruzelka and V. Setnicka, Analyst, 2015, 140, 2287–2293 RSC.
- M. E. Darvin, I. Gersonde, H. Albrecht, W. Sterry and J. Lademann, Laser Phys. Lett., 2007, 4, 452–456 CrossRef CAS.
- J. Fiedor and K. Burda, Nutrients, 2014, 6, 466–488 CrossRef PubMed.
- H. Tapiero, D. M. Townsend and K. D. Tew, Biomed. Pharmacother., 2004, 58, 100–110 CrossRef CAS PubMed.
- Z. Movasaghi, S. Rehman and I. U. Rehman, Appl. Spectrosc. Rev., 2007, 42, 493–541 CrossRef CAS.
- D. P. Lau, Z. W. Huang, H. Lui, D. W. Anderson, K. Berean, M. D. Morrison, L. Shen and H. S. Zeng, Lasers Surg. Med., 2005, 37, 192–200 CrossRef PubMed.
- D. Naumann, Proc. SPIE, 1998, 3257, 245–257 CrossRef CAS.
- J. Bandekar, Biochim. Biophys. Acta, Protein Struct. Mol. Enzymol., 1992, 1120, 123–143 CrossRef CAS.
- W. T. Cheng, M. T. Liu, H. N. Liu and S. Y. Lin, Microsc. Res. Tech., 2005, 68, 75–79 CrossRef CAS PubMed.
- F. Bernal-Reyes, M. Acosta-Elias, A. J. Burgara-Estrella, O. Álvarez-Bajo, O. I. Gavotto-Nogales, F. J. Antunez-Dominguez, L. Placencia-Camacho and H. M. Sarabia-Sainz, Instrum. Sci. Technol., 2020, 1–15, DOI:10.1080/10739149.2020.1725891.
- S. Y. Feng, D. Lin, J. Q. Lin, B. H. Li, Z. F. Huang, G. N. Chen, W. Zhang, L. Wang, J. J. Pan, R. Chen and H. S. Zeng, Analyst, 2013, 138, 3967–3974 RSC.
- W. R. Premasiri, J. C. Lee and L. D. Ziegler, J. Phys. Chem. B, 2012, 116, 9376–9386 CrossRef CAS PubMed.
- D. Lin, J. Pan, H. Huang, G. Chen, S. Qiu, H. Shi, W. Chen, Y. Yu, S. Feng and R. Chen, Sci. Rep., 2014, 4, 4751–4758 CrossRef PubMed.
- X. M. Dou, Y. Yamaguchi, H. Yamamoto, H. Uenoyama and Y. Ozaki, Appl. Spectrosc., 1996, 50, 1301–1306 CrossRef CAS.
- P. Bornemisza and I. Suciu, Med. Interne., 1980, 18, 353–356 CAS.
- Z. Huang, A. McWilliams, H. Lui, D. I. McLean, S. Lam and H. Zeng, Int. J. Cancer, 2003, 107, 1047–1052 CrossRef CAS PubMed.
- J. W. Chan, D. S. Taylor, T. Zwerdling, S. M. Lane, K. Ihara and T. Huser, Biophys. J., 2006, 90, 648–656 CrossRef CAS PubMed.
- J. A. Samuels and S. Singh, JPMC, 2017, 1, 25–28 Search PubMed.
- J. L. Liu, X. J. Zhang, X. F. Wang, L. Xu, J. Y. Li and X. H. Fang, Sci. Bull., 2016, 61, 1187–1194 CrossRef CAS.
- B. S. Berlett and E. R. Stadtman, J. Biol. Chem., 1997, 272, 20313–20316 CrossRef CAS.
- X. Chen, W. Xu, M. Miao, Z. Zhu, J. Dai, Z. Chen, P. Fang, J. Wu, D. Nie, L. Wang, Z. Wang, Z. Qiao and H. Shi, Acta Biochim. Biophys. Sin., 2015, 47, 504–515 CrossRef CAS PubMed.
- Z. Zhu, W. Xu, J. Dai, X. Chen, X. Zhao, P. Fang, F. Yang, M. Tang, Z. Wang, L. Wang, D. Ma and Z. Qaio, Int. J. Biochem. Cell Biol., 2013, 45, 571–582 CrossRef CAS PubMed.
- N. Roohi, A. Mehjabeen and S. Ashraf, Punjab Univ. J. Zool., 2017, 32, 209–215 Search PubMed.
- A. Arora, C. A. Willhite and D. C. Liebler, Carcinogenesis, 2001, 22, 1173–1178 CrossRef CAS PubMed.
- M. Taniguchi, J. Iizuka, Y. Murata, Y. Ito, M. Iwamiya, H. Mori, Y. Hirata, Y. Mukai and Y. Mikuni-Takagaki, BioMed Res. Int., 2013, 2013, 168765 Search PubMed.
- A. Bankapur, E. Zachariah, S. Chidangil, M. Valiathan and D. Mathur, PLoS One, 2010, 5, e10427 CrossRef PubMed.
- J. W. Shao, H. L. Yao, L. J. Meng, Y. Q. Li, M. M. Lin, X. Li, J. X. Liu and J. P. Liang, Vib. Spectrosc., 2012, 63, 367–370 CrossRef CAS.
- B. R. Wood and D. McNaughton, Biopolymers, 2002, 67, 259–262 CrossRef PubMed.
- B. R. Wood and D. McNaughton, J. Raman Spectrosc., 2002, 33, 517–523 CrossRef CAS.
- S. Rao, S. Balint, B. Cossins, V. Guallar and D. Petrov, Biophys. J., 2009, 96, 209–216 CrossRef CAS PubMed.
- B. R. Wood, L. Hammer, L. Davis and D. McNaughton, J. Biomed. Opt., 2005, 10, 14005 CrossRef PubMed.
- I. P. Torres, J. Terner, R. N. Pittman, E. Proffitt and K. R. Ward, J. Appl. Physiol., 2008, 104, 1809–1817 CrossRef PubMed.
- B. R. Wood, B. Tait and D. McNaughton, Biochim. Biophys. Acta, Mol. Cell Res., 2001, 1539, 58–70 CrossRef CAS.
- R. G. Blasberg and J. Gelovani, Mol. Imaging, 2002, 1, 280–300 CrossRef CAS PubMed.
- E. A. Schellenberger, A. Bogdanov Jr, A. Petrovsky, V. Ntziachristos, R. Weissleder and L. Josephson, Neoplasia, 2003, 5, 187–192 CrossRef CAS PubMed.
- A. Roy, J. Sikdar, P. Seal and R. Haldar, Inhalation Toxicol., 2015, 27, 300–307 CrossRef CAS PubMed.
|
This journal is © The Royal Society of Chemistry 2020 |