DOI:
10.1039/D0RA00429D
(Paper)
RSC Adv., 2020,
10, 12166-12182
Ameliorating amyloid aggregation through osmolytes as a probable therapeutic molecule against Alzheimer's disease and type 2 diabetes†
Received
15th January 2020
, Accepted 16th February 2020
First published on 25th March 2020
Abstract
Large numbers of neurological and metabolic disorders occurring in humans are induced by the aberrant growth of aggregated or misfolded proteins. Alzheimer's disease (AD) and type 2 diabetes (T2D) are two of the most prevalent disorders that lead to toxic protofibrils of amyloid beta (Aβ) and human islet amyloid polypeptide (hIAPP) in the form of intrinsically disordered proteins (IDPs). IDPs are important functional proteins or peptides that have no common structures and are found in various organisms; they play an imperative role in multiple biological mechanisms, changing their folding and unfolding patterns depending on the environment. Osmolytes are low molecular weight naturally occurring small molecules present in almost all organisms that act as denaturants or counter-denaturants, helping to alter the environmental surroundings under stressful or pathological conditions. These molecules aid in imparting steadiness on accumulated proteins and defending them from aggregating. In the current study, we performed an advanced sampling technique, replica-exchange molecular dynamics (REMD) simulations, to investigate the activities of osmolytes, with guanidine hydrochloride (G-HCL) acting as a denaturant and L-proline (L-PRO) acting as a counter-denaturant, and to explore the regulation and aggregation of Aβ and hIAPP. We report that G-HCL and L-PRO have noticeable natural effects on Aβ and hIAPP, leading to conformation modulation. Our results highlight that G-HCL attenuates peptide aggregation and transitions peptides into unfolded conformations, while L-PRO helps produce folded conformations of Aβ and hIAPP.
Introduction
Alzheimer's disease (AD) and type 2 diabetes (T2D) are two of the most prevalent neurodegenerative disorders in the current worldwide population. AD is the most common neurodegenerative disease of the central nervous system, ahead of Parkinson's disease. The disease affects approximately 50 million people worldwide and 5.8 million people of all ages in the US suffer from AD or related forms of dementia.1 AD is most common in elderly populations of around 65 years of age. Reports of death from other diseases, like heart disease and prostate cancer, have been reduced, whereas reports of death due to AD have enhanced by 145%.1 The global cost of the disease in 2018 for around 16 million people was nearly $234 billion, with about 18.5 billion hours of care provided to people with AD or related dementias, and this is estimated to be around $290 billion in 2019.1 Pathologically, AD is identified by extracellular plaques of amyloid β (Aβ) and intracellular neurofibrillary tangles of hyper-phosphorylated tau. The most common symptoms include dizziness, nausea, vomiting, diarrhea, loss of appetite, abdominal pain, and salivation. On the other hand, T2D is the most common type of diabetes found in the world's population. T2D is marked as a metabolic disease, described by hyperglycemia and insulin resistance. T2D is caused due to islet amyloid deposits consisting predominantly of misfolded and aggregated islet amyloid polypeptide (IAPP), which are detected in more than 90% of patients with T2D.2 Around 9.4 percent of the U.S population has diabetes, which includes 30.2 million adults aged 18 years and over. In reports from the Centers for Disease Control and Prevention (CDC), 79
535 deaths occur every year because of diabetes. The estimated cost of T2D is about $327 billion, including $237 billion in medical costs and $90 billion in reduced productivity.3 Increased thirst, frequent urination, increased hunger, unintended weight loss, fatigue and blurred vision are some of the serious symptoms of T2D. In accordance with pathophysiology, AD and T2D are associated with Aβ and hIAPP due to the abnormal accumulation of these proteins in the brains4–6 and pancreatic islets of patients.7–9 Several ways have been mentioned in previous studies by which Aβ and hIAPP can lead to AD and T2D pathogenesis; nevertheless, the most prevalent mechanism states that the aberrant aggregation of peptides leads to soluble oligomeric states called protofibrils, which are generally toxic and result in neuronal cell death in the case of AD and β-cell dysfunction in the case of T2D.10–15
Intrinsically disordered proteins (IDPs) are anomalous polypeptide chains without any stable tertiary structures in the physiological environment in vitro. IDPs remain functional despite the absence of a well-defined structure, opposing the concept that the functional activity of a protein is elucidated by its structure. Several studies in previous years have explained the functional importance of IDPs,16–18 and the most fascinating aspect, connected to IDPs having over 40 amino acid residues, is that they make up more than 33% of known sequenced eukaryotic proteins.19,20 Such IDPs are now contemplated as playing a pivotal role in the prior steps of the development of several protein conformational diseases.21 hIAPP and Aβ are prominent IDPs responsible for amyloid formation and conformational diseases.22 hIAPP, also known as amylin, is a 37 residue small peptide co-secreted with insulin from β-cells inside the islets of Langerhans in the pancreas, which is involved in amyloid aggregation, causing T2D; Aβ is a 42 residue peptide predominantly found in the brains of people with AD and Down syndrome. Both hIAPP and Aβ are significant components of amyloid plaques and share several features, including having similar β-sheet secondary structure conformations.22 Several in vivo and in vitro studies have been performed in previous years to investigate AD23,24 and T2D,25–27 which have provided basic structural and overall dimensional details relating to IDPs but have rarely provided a unified image of the overall proteins. In order to understand the conformational dynamics of IDPs and the methods of structural ensemble recognition involving various binding neighbors and small molecule inhibitors, primarily, sampling techniques (knowledge and physics based) are used in silico accompanied by structural data obtained from experiments.28 Grasso et al. investigated the conformational dynamics and stabilities of S-shaped and U-shaped Aβ assemblies because the C-terminal residues are involved in many interactions.29 They also claimed that the U-shaped motif is significantly described by distortions leading to an assembly with higher disorder.29 Alexandre et al. analysed the importance of the conserved disulfide bond in hIAPP between two cysteine at positions 2 and 7 using a combination of experimental studies and MD simulations.30 A wild type fragment (hIAPP1–8) led to amyloid aggregation through a different pathway than a fragment without disulfide bonds and a fragment having cysteine mutated to serine at positions 2 and 7; hence, they claimed that an intact disulfide bond might help protect from amyloid aggregation because of decreased interpeptide hydrogen bonding30. Moreover, IDP amyloid aggregation can harm the membranes of related cells and can induce various neurodegenerative diseases. It has been observed that small IDP oligomers are majorly toxic and, because of the enhanced aggregation, it is very difficult to identify the structures of IDP oligomers experimentally in the complex membrane surroundings. On the contrary, MD simulation studies provide atomistic insights into the structural dynamics of IDP amyloid aggregation. Recently, Liu et al. showed the structural propensities of dimeric hIAPP in the membrane surroundings, where they claimed that N terminal β-sheet structure formation occurred before C terminal β-sheet structures were formed in the final aggregates, and they also included insights into various other secondary structural intermediate changes31. Similarly, the putative structures of oligomeric Aβ11–40 trimers with a dipalmitoyl phosphatidylcholine (DPPC) lipid bilayer membrane were determined by Son et al.32
One factor that can influence protein folding and interactions with cell membranes and interfaces is small co-solutes, known as osmolytes, which influence and counterbalance the osmotic pressure of the cell and the cellular environment.33 The denaturation mechanism of a well-known osmolyte, urea, has been the focus of most previous research.34–36 While the extensive efforts devoted to this subject in the past decades, and the ongoing controversial discussions, justify the exclusive role urea has been given until now, it remains to be determined how other denaturants or even counter-denaturants work. Is the urea mechanism transferable to other denaturants? Can common traits be found among osmolytes with stabilizing or destabilizing effects on proteins? To address these questions, and to put the results obtained for urea in our previous work37 into a more general framework, we investigated the interactions of Aβ and hIAPP with another denaturant, guanidine hydrochloride (G-HCL), and a counter-denaturant, L-proline (L-PRO).
Other than urea, G-HCL is the most frequently used denaturant.38–40 Its chemical structure is similar to that of urea, except that a third amino group, instead of an oxygen atom, is bound to the carbon atom. With the resulting positive charge, G-HCL is a monovalent cation. Hence, it is always accompanied by an anion in an electrically neutral salt. Because ions themselves are known to have a significant effect on protein stability,41 the ionic character of G-HCL, as well as the presence of additional anions, renders the total effect of G-HCL salts on proteins highly complex. For instance, at high concentrations, G-HCL is an even stronger denaturant than urea, whereas it has a stabilizing effect on proteins at low concentrations.42 Alexander et al. found that G-HCL at low concentrations shows osmolyte-like stabilizing effects on D-glucose/D-galactose-binding proteins.43 G-HCL was also found to show biphasic behavior during amyloid aggregation, increasing the aggregation kinetics at low G-HCL concentrations and decreasing the aggregation kinetics at high G-HCL concentrations.44 Several studies on the inactivation and unfolding of related proteins have been done, leading to denaturation due to G-HCL.45–47 Not much is known about the origin of the denaturing effect of G-HCL or even if it is similar to that of urea. While some studies have suggested that the mechanisms of these two denaturants are similar,48 other findings support the view that the mechanisms differ.38 Various MD simulation studies have been performed related to G-HCL38,49,50 but these were found to be less efficient for the conformational sampling of several IDPs. Therefore, using advanced sampling techniques, e.g., REMD simulations, to examine such aspects might be quite effective for obtaining insights into conformational ensembles of Aβ and hIAPP in the presence of G-HCL.
Unlike urea or G-HCL, the protecting amino acid osmolyte L-PRO helps stabilize the native structures of proteins by undergoing interactions with solvent water and excluding it from the protein surface51. L-PRO plays a vital role in the folding and unfolding of proteins,52,53 and protein modulation and aggregation.54 L-PRO also plays a pivotal role in promoting the utilitarian forms of proteins.55 Furthermore, it is known for domain swapping56,57 and amyloid fibril formation through protein aggregation.58–60 In previous studies, it was reported that L-PRO is responsible for amorphous aggregates in polyQ,61 polymorphism in glucagon,62 and inhibiting the aggregation of both lysozyme63 and insulin.64 However, in a recent study, L-PRO was considered as a switch for the conformational changes leading to amyloid fibril formation.65 Hence, we can say that L-PRO has varied effects on different IDPs and proteins. Therefore, it will be interesting to explore the behavior of L-PRO on Aβ and hIAPP.
In this study, we performed 100 ns all-atom explicit solvent REMD simulations on Aβ and hIAPP under the influence of G-HCL and L-PRO. To put our work into a broader framework, we characterized Aβ and hIAPP, where the counter-denaturant L-PRO supports protein folding and amyloid fibril formation, whereas the denaturant G-HCL supports protein unfolding and inactivation. We used these osmolytes to show the regulation and aggregation of IDPs through an intensive conformational sampling technique, REMD simulations, and to check the modulation of the conformations of Aβ and hIAPP. Initiated from extended coiled structures, REMD showed that in the presence of the denaturant G-HCL, Aβ and hIAPP peptides transiently underwent the formation of more random coils and loops, while the influence of the counter-denaturant L-PRO acts oppositely on the morphologies of Aβ and hIAPP, showing β-sheet formation along with reduced α-helix formation. Several statistical and quantitative analysis results accompanying this study provide a detailed understanding of the effects of different osmolytes on various IDPs.
Materials and methods
Peptide preparation
Aqueous solution 3D structures of AD-associated Aβ1–42 (PDB ID: 1ZOQ) and type 2 diabetes (T2D)-associated hIAPP1–37 (PDB ID: 2L86) were downloaded from the RCSB PDB.66 The sequence of Aβ1–42 is 1DAEFRHDSGYEVHHQKLVFFAEDVGSNKGAIIGLMVGGVVIA42, and that of hIAPP1–37 is 1KCNTATCATQRLANFLVHSSNNFGAILSSTNVGSNTY37. The peptides were preprocessed and optimized by enumerating missing atoms and amending the overlapping coordinates; furthermore, the energy was minimized via an optimized force field. To avoid any bias in the preliminary secondary structures, the extended coil states of the Aβ and hIAPP monomers were obtained through simulations at 500 K. Moreover, to minimize the effects of unanticipated outcomes from uncapped and non-neutralized peptide ends, amidation and acetylation were performed at the C and N terminals of both monomer peptides. The nomenclature for the six systems of Aβ and hIAPP peptides involving osmolytes is as follows: AβG-HCL+Water and hIAPPG-HCL+Water (G-HCL and water); AβL-PRO+Water and hIAPPL-PRO+Water (L-PRO and water); and AβWater and hIAPPWater (water).
Replica exchange molecular dynamics (REMD)
REMD simulations involving the six systems (AβG-HCL+Water, AβL-PRO+Water, AβWater, hIAPPG-HCL+Water, hIAPPL-PRO+Water, and hIAPPWater) were performed on GROMACS.67 Newton's equations of motion were unified with a time step of 2 fs, using the leapfrog algorithm integrator. The van der Waals interactions were calculated, including both long and short range electrostatic forces, and the particle mesh Ewald algorithm68 was implemented with the algorithm, with fast Fourier transforms used to reduce the MD simulation impediments. Furthermore, periodic boundary conditions were used to control the system size effects, and to derive the Aβ and hIAPP topologies, an all-atom optimized potentials for liquid simulations (OPLS)69 forcefield was used. Moreover, three-point rigid water with transferable intermolecular potential70 was utilized as the solvent, incorporating charged ions to nullify the charges of Aβ and hIAPP.
At the beginning, all six systems were NPT equilibrated (i.e., a static number of atoms, and constant temperature and pressure) at 300 K and an isotropic pressure of 1 bar, employing the Berendsen weak-coupling thermostat and a barostat71 to achieve box dimension equilibrium at a compressibility of 4.5 × 10−5 bar−1. In addition, NVT equilibrium conditions (i.e., a static number of atoms, and constant temperature and volume) were applied with a Nosé–Hoover thermostat72 and an advanced Hamiltonian associated superficially with a heat-bath to accomplish precise thermodynamic ensembles. The peptide and water bonds were constrained via the linear constraint solver algorithm73 and SETTLE algorithm,74 respectively. The two algorithms use Lagrange multipliers and a symplectic integrator to constrain the chemical bonds.
REMD simulations were performed to enhance the sampling in such scenarios; various independent replicas were run with slightly variant ensembles, exchanging the coordinates of the replicas within the ensembles periodically. In recent studies, temperatures were selected and distributed based on an exponential spacing law to perform REMD studies.75 Another technique widely used in previous studies76 was a web server (http://folding.bmc.uu.se/remd),77 which we utilized for temperature selection in REMD simulations ranging from 298 to 501 K for 32 replicas, having 20% average exchange probability within replica purses every 2 ps. The peptide in each of the six systems was simulated for 100 ns per replica, and further analysis was performed on the last 80 ns of each timeframe. The osmolytes G-HCL and L-PRO were used according to models developed by Nozaki and Tanford78 and at specific concentrations (i.e., 5 M for G-HCL and 2 M for L-PRO), as previously described.37
MD analysis
A series of MD simulation analyses were performed via GROMACS tools and using in-house scripts. Initially, energy factors or distance restraint data from the energy files were extracted using the GROMACS in-built facility “g_energy.” Furthermore, we confirmed that all physicochemical properties of the system had attained equilibrium, where their averages no longer altered as a function of time. The easiest way to calibrate stability was through measuring the root mean square deviation (RMSD) using “g_rms.” Similarly, root mean square fluctuation (RMSF) analysis was performed to calculate the peptide flexibility, using “g_rmsf,” thus determining the variations in the C-alpha atom coordinates from a neutral position. Intramolecular hydrogen bonds were considered using “g_hbond” to predict the nature of the peptide folding or unfolding, and hydrogen bonds were calculated with an oxygen–hydrogen–nitrogen angle of 30° or less, along with an oxygen–hydrogen distance of 2.5 Å or less.
To detect the compactness of Aβ and hIAPP, the radius of gyration (Rg) about the center of mass was determined with the help of “g_gyrate,” and the end-to-end peptide distance (Ree) was calculated utilizing “g_polymer.” Ree was determined from the center of mass of the acetylated terminal (i.e., the C terminal) to the center of mass of the amidated terminal (i.e., the N terminal). Secondary structure analysis was also implemented via “do_dssp,” which uses a pattern-recognition process involving hydrogen-bonded and geometric features. Peptide clustering analysis was performed to identify similar structures sampled during the MD simulations, using “g_cluster” with a cutoff of 0.35 nm. The most frequently used clustering algorithms applied to MD trajectories developed by Daura79 were utilized for peptide conformations that were clustered together, in order to compare various orientations of protein backbones without terminals, and the clusters were grouped together depending on the RMSD. The radial distribution functions (RDFs) of water, the denaturant (G-HCL), and the counter-denaturant (L-PRO), corresponding to the peptide surface and center of mass of the osmolyte, were measured using “g_rdf”.
Population density analysis
Population density analysis was achieved using GNUPLOT v5.0, a portable command line program widely used for graphing on various operating systems. Population density analysis takes into consideration the well-known quantities of associated phenomena and ramifies them across a landscape based on the quantity determined at each location and the spatial relationships between the locations of the determined quantities. Population density graphs presenting a function of Rg and Ree in each case were plotted in GNUPLOT v5.0. Salt bridge analysis was also performed on nearby and distant residues displayed in the population density plot.
Results
This study was designed to investigate the roles played by osmolytes (G-HCL and L-PRO) in the modulation and aggregation of intrinsically disordered Aβ and hIAPP, which are responsible for AD and T2D, respectively. We performed REMD simulations on six systems: Aβ and hIAPP in the presence of G-HCL and water (AβG-HCL+Water and hIAPPG-HCL+Water), L-PRO and water (AβL-PRO+Water and hIAPPL-PRO+Water), and water only (AβWater and hIAPPWater). A comprehensive computational approach was used to obtain conformational insights into the effects of G-HCL and L-PRO on amyloids.
REMD simulations of Aβ and hIAPP in G-HCL, L-PRO, and water
REMD studies were performed on a total of 32 replicas in the temperature range of 298–500 K, and further analysis was conducted at 300 K. Simulation convergence assessments were performed where the average acceptance ratio was found to be higher than 20% for each case, and the convergence of the REMD simulations was checked using the trajectories, which showed that the temperature space was explored widely by each replica throughout the simulation time (Fig. S1A and B†). Further, the distributions of the potential energy of each replica along the REMD simulation time were determined and were also found to converge (Fig. S1C†). RMSD analysis of the peptides gave insight into their structural conformations during REMD simulations, providing an explanation for the peptide stability and whether the simulation had equilibrated. The average backbone RMSD values for all Aβ and hIAPP systems varied (0.5–1.5 nm and 0.5–2.5 nm, respectively) and they adopted multiple conformations through the entire REMD simulation time period (Fig. 1(A)). The existence of stable peptide RMSD values until the end of the simulation implied that the simulations were ideal for further precise analysis.
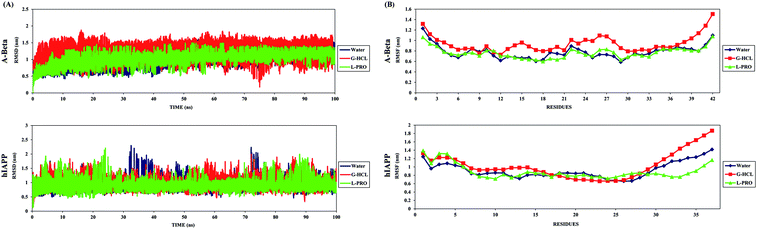 |
| Fig. 1 (A) Root mean square deviation (RMSD) plots for Aβ [top] and hIAPP [bottom] in the presence of water, G-HCL, and L-PRO, demonstrating that the peptides adopt various conformations. (B) Root mean square fluctuation (RMSF) plots for all residues of Aβ [top] and hIAPP [bottom] in the presence of water, G-HCL, and L-PRO. | |
An RMSF graph for each residue was determined, and the peaks showed local fluctuations along the peptide during the REMD simulations. Both terminals (the N and C terminals) fluctuated more than any other part of the peptides (Fig. 1(B)). Furthermore, residues 10–28 of Aβ were found to fluctuate more in the presence of G-HCL and similar fluctuations were seen in the presence of L-PRO and water. In the case of hIAPP, all three systems showed similar fluctuations, with a small increase in the presence of G-HCL from residues 13–17. Both Aβ and hIAPP in the presence of G-HCL showed more fluctuating residues, which are responsible for amyloid formation.
Contradictory effects of G-HCL and L-PRO on Aβ and hIAPP
The response of osmolytes in terms of protein aggregation is crucial, since they can act as drugs for diseases related to amyloid formation. In this study, we showed that the stabilizer L-PRO (2 M) and denaturant G-HCL (5 M) osmolytes had remarkably different effects on the peptide conformations. The addition of G-HCL helped the formation of unfolded structures in the cases of both AβG-HCL+Water and hIAPPG-HCL+Water, while L-PRO (AβL-PRO+Water and hIAPPL-PRO+Water) inhibited unfolded conformations from emerging in the dense and folded structures of Aβ and hIAPP. In the case of the water systems, both peptides were more prone to exhibiting compact folded forms, and there were few cases of β-sheet formation.
Population density graphs: Rg vs. Ree
Population density graphs were sketched as functions of Rg and Ree for all six systems (Fig. 2). After urea, G-HCL is the most effective denaturant, known to enhance the formation of unfolded conformations, and L-PRO has a stabilizing effect similar to trimethylamine N-oxide (TMAO). The population density graphs show that the incorporation of G-HCL guided population shifts in both AβG-HCL+Water and hIAPPG-HCL+Water to unfolded conformations. However, the addition of L-PRO led to population shifts in both AβL-PRO+Water and hIAPPL-PRO+Water to folded structures, identical to those of AβWater and hIAPPWater, respectively.
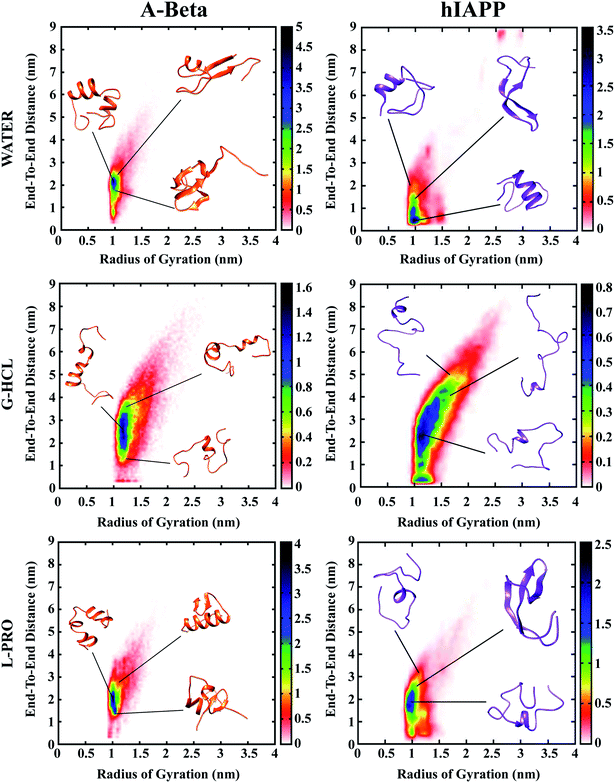 |
| Fig. 2 Population density analysis for Aβ and hIAPP, showing peptide end to end distance (Ree), i.e., C to N terminal, and radius of gyration (Rg) around the center of mass. A blue colour implies heavily populated conformations, whereas red and yellow colours indicate lesser populated conformations. | |
In the population density graphs, the blue regions represent densely populated areas, and the yellow, green, red, and white regions represent continuously less-densely populated areas. The relative observations from the population density graphs for all six systems affirm that the blue regions are complementary for AβWater, hIAPPWater, AβL-PRO+Water, and hIAPPL-PRO+Water, but in the cases of AβG-HCL+Water and hIAPPG-HCL+Water, the blue regions shifted to higher coordinates.
Table 1 shows the Ree and Rg values measured for all six systems. The results in Table 1 clearly show that AβG-HCL+Water and hIAPPG-HCL+Water had higher Ree and Rg values (3.252 and 3.420 nm; and 1.386 and 1.461 nm, respectively), which led the peptides to adopt extended unfolded conformations. In the cases of AβL-PRO+Water and hIAPPL-PRO+Water, the Ree and Rg values (2.545 and 2.155 nm; and 1.146 and 1.139 nm, respectively) demonstrated that the peptides were compact, folded, and further stabilized. The control systems (i.e., AβWater and hIAPPWater) had Ree and Rg values (2.316 and 2.227 nm; and 1.084 and 1.192 nm, respectively) similar to those of AβL-PRO+Water and hIAPPL-PRO+Water. Population density plots for Rg and Ree were also determined in different time windows of 0–25, 25–50, 50–75 and 75–100 ns in order to check the REMD convergence throughout the simulation time (Fig. S2†).
Table 1 Average peptide end to end distance (Ree) and radius of gyration (Rg) values
Peptide in water and osmolytes |
Average Ree |
Average Rg |
AβWater |
2.316 nm |
1.084 nm |
AβG-HCL+Water |
3.252 nm |
1.386 nm |
AβL-PRO+Water |
2.545 nm |
1.146 nm |
hIAPPWater |
2.227 nm |
1.192 nm |
hIAPPG-HCL+Water |
3.420 nm |
1.461 nm |
hIAPPL-PRO+Water |
2.155 nm |
1.139 nm |
Intramolecular hydrogen bonding probability
The presence of G-HCL led to drastic changes, with the decreased formation of intramolecular hydrogen bonds leading to unfolded and extended conformations of AβG-HCL+Water and hIAPPG-HCL+Water (Fig. 3), whereas L-PRO had minimal effects on intramolecular hydrogen bonding, having a highest probability of more than 9.3% (24 hydrogen bonds) in the case of AβL-PRO+Water and 9.6% (13 hydrogen bonds) in the case of hIAPPL-PRO+Water. Similar results were found for AβWater and hIAPPWater, leading to folded and compact conformations. However, AβG-HCL+Water and hIAPPG-HCL+Water only had probabilities of 8.9% (19 hydrogen bonds) and 8.9% (18 hydrogen bonds), respectively. Similar intramolecular hydrogen bond probability percentage (%) values were also found in four different time frames, as seen in the case of Fig. S2,† when investigating the convergence of all the REMD simulations (Fig. S3†).
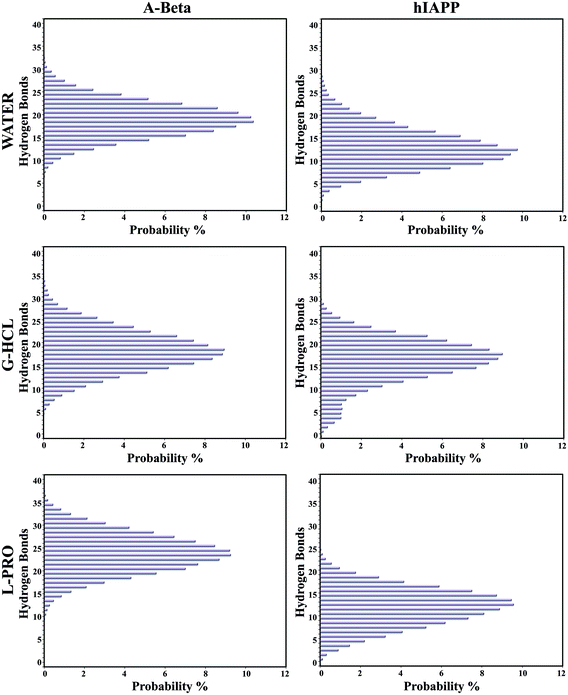 |
| Fig. 3 Probability percentage (%) values of intramolecular hydrogen bond (C O to N–H) formation. | |
Population density graphs: salt bridge formation
Population density graphs were sketched for salt bridges between adjacent residues (D23 & K28) and distant residues (D23 & K16) in the cases of AβWater, AβG-HCL+Water, and AβL-PRO+Water (Fig. 4). No salt bridge formation occurred in hIAPP due to the absence of negatively charged or acidic amino acids (like aspartic acid and glutamic acid) as acidic protein side chains. Peptide unfolding occurred in AβG-HCL+Water, confirming salt bridge formation between adjacent residues (D23 & K28) rather than distant residues (D23 & K16). However, in the case of AβWater, more salt bridge formation occurred between distant residues (D23 & K16) rather than between adjacent residues (D23 & K28). Both of these systems showed comparable numbers of salt bridges, with fewer formed in AβG-HCL+Water than in AβWater. However, a drastic decrease in the number of salt bridges formed between adjacent residues (D23 & K28) and an increase in distant residue bridges (D23 & K16) occurred for AβL-PRO+Water.
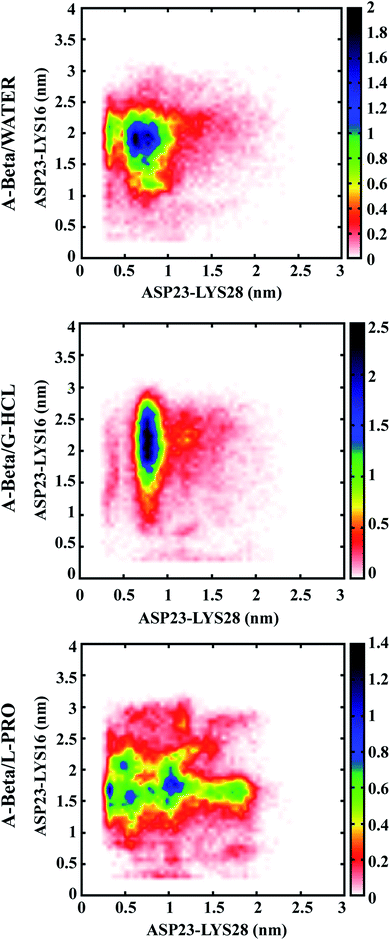 |
| Fig. 4 Population density analysis of monomeric salt bridges formed between ASP (D) and LYS (K) of Aβ. | |
Based on the results obtained from intramolecular hydrogen bonding and salt bridge formation studies, we concluded that an increase in salt bridge formation compensated for a decrease in intramolecular hydrogen bonds. Therefore, both AβL-PRO+Water and AβWater showed similar patterns without conformational disparity. Similar plots were generated in four different time windows (as in Fig. S2†), to check the convergence of REMD throughout the simulation time (Fig. S4†).
Conformational transitions of Aβ and hIAPP in G-HCL and L-PRO
Secondary structure analysis was performed on all systems of Aβ and hIAPP in the presence of G-HCL and L-PRO. Fig. 5(A) shows the secondary structure profiles for Aβ and hIAPP, with the associated coil, β-sheet, β-bridge, bend, turn, α-helix, 5-helix, and 3-helix probabilities. As evident in the figure, G-HCL was responsible for the pronounced formation of coiled secondary structures when compared to the L-PRO and water systems. AβG-HCL+Water and hIAPPG-HCL+Water showed maximum coiled secondary structure probability percentages of 59.96 and 62.16%, respectively; AβL-PRO+Water, hIAPPL-PRO+Water, AβWater, and hIAPPWater showed probability percentages of 35.36, 41.95, 31.10, and 38.99%, respectively. Furthermore, the β-sheet secondary structure probability percentages were quite low for both AβG-HCL+Water and hIAPPG-HCL+Water (0.28 and 0.13%, respectively) when compared values of 9.73, 17.38, 18.36, and 19.95% for AβL-PRO+Water, hIAPPL-PRO+Water, AβWater, and hIAPPWater, respectively. Here, the β-sheet element was fixed in both control systems (i.e., AβWater and hIAPPWater). Subsequently, the other secondary structures showed lower probability percentages, as depicted in Fig. 5(A). Moreover, elaborate residue-specific secondary structure probability percentages were calculated, as shown in Fig. 5(B). The β-sheet probability was prominent at the N terminal hydrophobic residues of AβL-PRO+Water and AβWater; correspondingly, hIAPPL-PRO+Water, hIAPPG-HCL+Water, and hIAPPWater showed similar β-sheet probabilities. The α-helix probabilities were apparently quite high in the middle region residues of AβG-HCL+Water, AβL-PRO+Water, and AβWater, as well as at the C terminal residues of hIAPPL-PRO+Water, hIAPPG-HCL+Water, and hIAPPWater. Other residue-specific secondary structure probabilities are shown in the ESI (Fig. S5†). Furthermore, we also performed similar work in four different time windows to check the convergence of the REMD simulations (Fig. S6†).
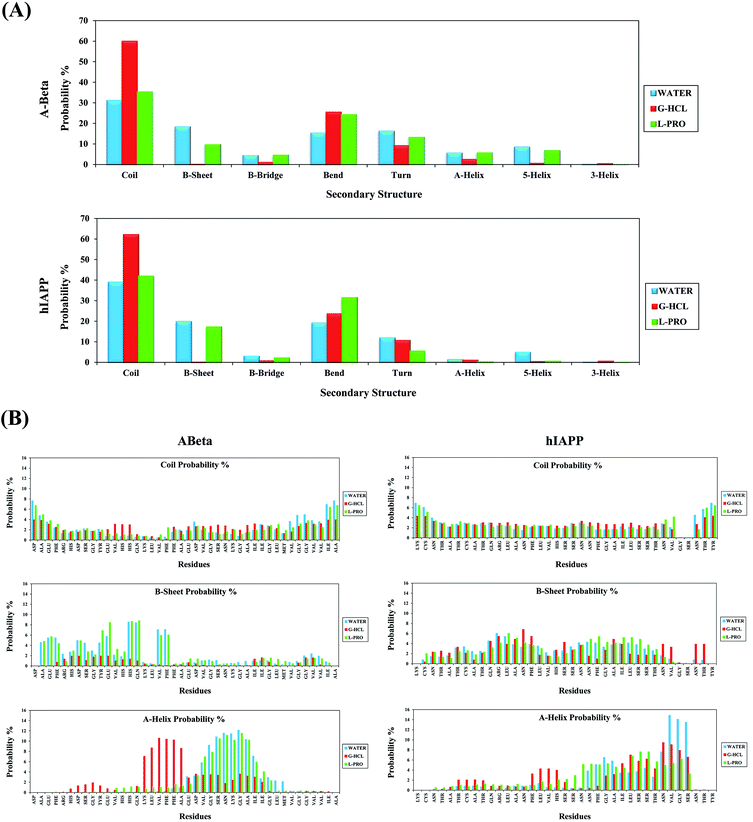 |
| Fig. 5 (A) Probability (%) of secondary structure formation for Aβ and hIAPP. (B) Detailed residue specific probability (%) of secondary structures [coil (top), β-sheet (middle), and α-helix (bottom)] for Aβ and hIAPP. | |
Clustering of Aβ and hIAPP in G-HCL and L-PRO
Each REMD simulation assembled ample conformational ensembles for every REMD simulated temperature. To examine the 3D conformations of Aβ and hIAPP, we implemented cluster analysis of the monomer conformations generated in all six systems. This analysis helps to identify the more populated structures and divides the low-temperature structures developed from OPLS-treated REMD simulations into new clusters with analogous geometric characteristics. Hence, we obtained the salient structural properties of all six systems via highlighting precise structures that were representative of their particular clusters. Using a chain-independent main-chain RMSD cutoff value of 0.35 nm, the monomer conformations in all six systems (AβG-HCL+Water, AβL-PRO+Water, AβWater, hIAPPG-HCL+Water, hIAPPL-PRO+Water, and hIAPPWater) were separated into different clusters. The centers of the three most dominant clusters and their ensembles are delineated in Fig. 6. In the control systems (i.e., AβWater and hIAPPWater), the most dominant conformations had cluster probabilities of 2.88 and 2.92%, respectively, having folded and compact conformations, with β-sheet formation in the subsequent clusters, unlike the native structure. Similarly, AβL-PRO+Water and hIAPPL-PRO+Water had folded conformations with enhanced β-sheet structures, showing cluster probabilities of 3.13 and 3.84%, respectively, for the most dominant conformations. Conversely, AβG-HCL+Water and hIAPPG-HCL+Water both showed denatured peptides, with cluster probabilities of 0.54 and 0.55%, respectively, for the most dominant conformations. Also, the most dominant clusters in four different time windows (as in Fig. S2†) were observed to check the REMD convergence throughout the time period of simulation (Fig. S7†).
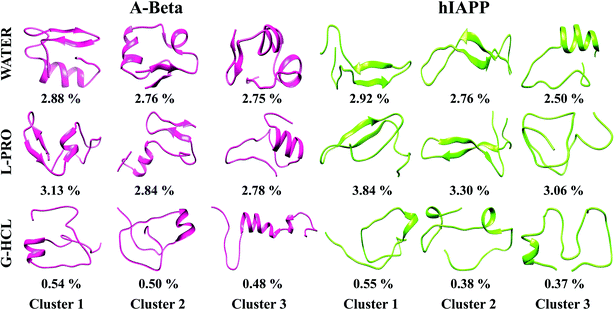 |
| Fig. 6 The ideal conformations of the three most populated clusters of Aβ and hIAPP obtained under different conditions. The corresponding time spent in each conformation over the production run of all systems is shown by the percentage value. | |
Peptide dehydration in G-HCL and L-PRO containing systems
The dehydration states of Aβ and hIAPP were determined around 5 Å of the peptide surfaces. To explain the dehydration of the peptides in all six systems, the quantities of water molecules around 5 Å of the peptide surfaces were calculated.
Table 2 shows the number of water molecules and osmolytes present within 5 Å of the entire peptide surfaces for all six systems. As per Table 2, the quantities of water molecules in the cases of AβG-HCL+Water and hIAPPG-HCL+Water were significantly decreased, only being hydrated by 326 and 257 water molecules, respectively, whereas AβWater and hIAPPWater were hydrated by 545 and 454 water molecules, respectively. These significant reductions in the numbers of water molecules can be explained in terms of the mechanism of G-HCL molecules, which are more prone to seek the side chains and backbones of Aβ and hIAPP, thus leading to the elimination of water molecules from the peptide surfaces (Fig. S8†). Furthermore, decreased numbers of water molecules were also observed in the cases of AβL-PRO+Water and hIAPPL-PRO+Water (i.e., from 545 to 360 and from 454 to 299, respectively, with AβWater and hIAPPWater as the respective standards) but to a smaller extent than the observed decreases in the cases of AβG-HCL+Water and hIAPPG-HCL+Water. Therefore, reduced numbers of L-PRO molecules28and22 were bound to the surfaces of AβL-PRO+Water and hIAPPL-PRO+Water, respectively, leading to AβWater
:
AβL-PRO+Water and hIAPPWater
:
hIAPPL-PRO+Water ratios of 12.85 and 13.59, respectively. Conversely, 77 and 55 G-HCL molecules were very closely bound to the surfaces of AβG-HCL+Water and hIAPPG-HCL+Water, respectively, leading to AβWater
:
AβG-HCL+Water and hIAPPWater
:
hIAPPG-HCL+Water ratios of 4.23 and 4.67, respectively.
Table 2 The peptide dehydration forms of the six systems around 0.5 nm of the surfaces of Aβ and hIAPP in their most dominant conformations
Aβ and hIAPP peptide system |
Water + osmolyte |
Water (# of molecules) |
Osmolyte (# of molecules) |
Water/osmolyte |
AβG-HCL+Water |
Water + G-HCl |
326 |
77 |
4.23 |
AβWater |
Water |
545 |
0 |
N/A |
AβL-PRO+Water |
Water + L-Pro |
360 |
28 |
12.85 |
hIAPPG-HCL+Water |
Water + G-HCl |
257 |
55 |
4.67 |
hIAPPWater |
Water |
454 |
0 |
N/A |
hIAPPL-PRO+Water |
Water + L-Pro |
299 |
22 |
13.59 |
A similar ratio of water to urea (4.5) was obtained for denatured deca-alanine peptides,80 conforming with our results. This clearly explains that G-HCL densely surrounds and prefers enhanced electrostatic interactions with the side chains of Aβ and hIAPP compared to L-PRO, which is dispersed at the peptide surfaces.
Fig. 7 shows the peptide dehydration profiles of Aβ and hIAPP in the presence of G-HCL and L-PRO, representing the enhanced dehydration in the case of G-HCL, but reduced dehydration in the case of L-PRO. Similar dehydration patterns for Aβ in the presence of urea and TMAO were obtained in our previous study,37 and for α-synuclein, showing the depletion of water from its surface.81
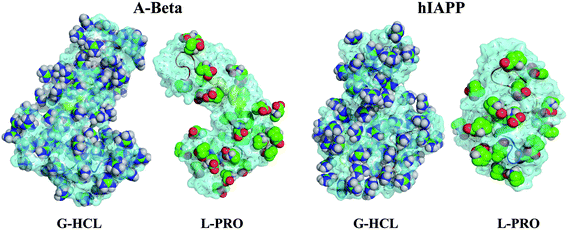 |
| Fig. 7 The structural resolution of water, G-HCL, and L-PRO 0.5 nm from the Aβ and hIAPP surfaces. The systems with G-HCL display peptide dehydration and the systems with L-PRO display mild peptide dehydration. Aβ and hIAPP are displayed with cartoon representations (rainbow); water molecules are displayed as lines (heteroatom colors: red and grey); and the surfaces (aqua-blue) and osmolytes are displayed using sphere and dot representation (heteroatom colors: G-HCL: blue, green, and grey; L-PRO: green, red, and grey). | |
The distribution of G-HCL and L-PRO around the peptide surface
The capability of an osmolyte to attract water molecules plays an important role in determining the peptide hydration profile, and the mechanisms involved in the distribution patterns of G-HCL and L-PRO for each residue at specific distances (i.e., 0.25–0.50, 0.50–0.75, and 0.75–1.00 nm) from the Aβ and hIAPP backbones were determined (Fig. 8). Based on the figure, it is evident that G-HCL accumulates around the peptide hydrophobic residues, which explains the prominent reduction in the number of water molecules around the peptide surface. Furthermore, although L-PRO also clusters around the peptide hydrophobic residues, it does not do so to the same extent that G-HCL does. We also analysed the distributions of osmolytes at different distances from the peptide surface in four different time windows in order to investigate the convergence of every REMD simulation performed in this study (Fig. S9†). Previous studies have shown the importance of proline residues in relation to the conformational changes leading to amyloid formation.65
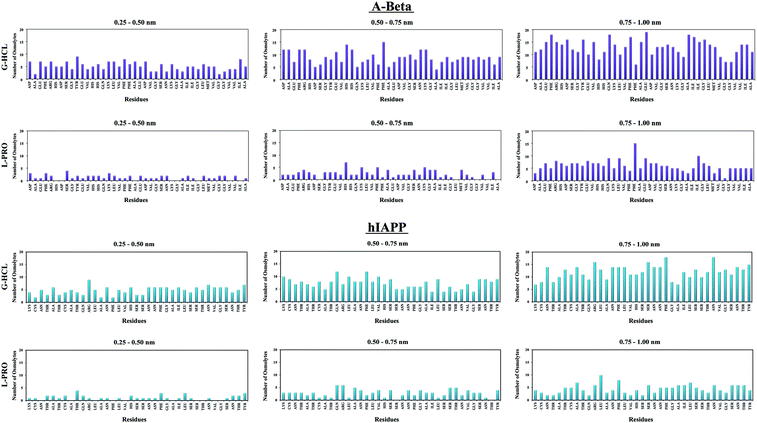 |
| Fig. 8 The residue-specific distributions of osmolytes at various distances from the backbones of Aβ and hIAPP for the most dominant peptide conformations. The positively charged side chains are heavily surrounded by G-HCL, whereas L-PRO does not show this type of activity. | |
The effects of G-HCL and L-PRO hydration on peptide stabilization
To illustrate the antithetical natures of Aβ and hIAPP in the presence of G-HCL and L-PRO, we computed the RDFs of water, G-HCL, and L-PRO with respect to the full peptide surface (Fig. 9(A)). In this figure, the structures of the G-HCL solutions surrounding the peptides are considerably more distinctive than those of the L-PRO solutions. The AβWater and hIAPPWater systems show the maximum hydration peaks relative to the peptide surfaces, having two hydration peaks at 0.04 and 1.5 nm, respectively. In the presence of osmolytes, the hydration shells preferentially changed in the cases of the AβG-HCL+Water, AβL-PRO+Water, hIAPPG-HCL+Water, and hIAPPL-PRO+Water systems. All four systems showed distinctive decreases in both the first and second hydration shells. As G-HCL has a higher dipole moment than L-PRO, the interaction of G-HCL with the peptide surfaces was closer (0.04 nm) than those of L-PRO, as shown in Fig. 9(A). Furthermore, modifications in the densities of surface water were found to complement the calculated results in Table 2. Fig. S8† shows that G-HCL tends to bind to surrounding G-HCL and the peptide surfaces more compactly than L-PRO. Previous studies reported that, despite the formation of extensive hydrogen bonds, G-HCL is mainly involved in short-term stacking interactions with itself82 and also with various planar groups.50,82,83 These stacking interactions allow for an understanding of the behaviour of G-HCL around the surfaces of Aβ and hIAPP. The results here correlate with the reduced number of intramolecular hydrogen bonds in the G-HCL treated systems, leading to unfolded conformations of both peptides. Similarly, the RDFs of water, G-HCL and L-PRO with respect to the peptide surfaces were analysed in different time windows to investigate the REMD simulation convergence (Fig. S10†).
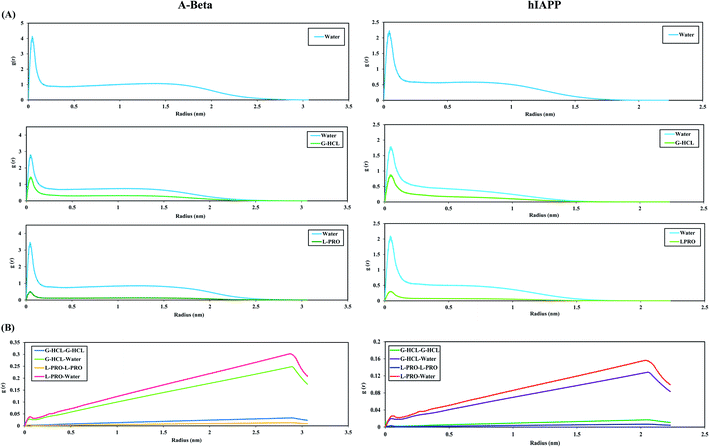 |
| Fig. 9 (A) The radial distribution functions, g(r), of water, G-HCL and L-PRO relative to the surfaces of Aβ and hIAPP peptides. (B) The radial distribution functions, g(r), of G-HCL and L-PRO in bulk water relative to their centers of mass. | |
Conversely, L-PRO enhances protein stability, as its pyrrolidine ring decreases the conformational ability of the protein backbone in its unfolded form. The RDFs of osmolytes in bulk water, relative to their center of mass, were also calculated (Fig. 9(B)). Because G-HCL has a higher dipole moment than L-PRO, the G-HCL–G-HCL association was found to be greater than the L-PRO–L-PRO association.
Superposition of conformational ensembles and their importance for regulation
Various modeled peptides have been considered as IDPs, showing completely diverse properties except for all having organized 3D structures84–86. Both Aβ and hIAPP in the presence of G-HCL and L-PRO showed various conformational ensembles of structures that interconvert simultaneously. G-HCL and L-PRO help in the regulation of these peptides in order to obtain some groups of conformations, as in the case of deviations of the conformational ensembles. Here, a few groups of conformations occurred, but no contemporary structures could be obtained in the monomeric state. Based on this result, this study states that each conformational ensemble present within the entire conformational arena of Aβ peptides sampled together was complementary; the same applies in the case of hIAPP.
The unfolded and extended structures of Aβ and hIAPP were stable throughout the REMD simulations when no hydrogen bonds were permitted (or salt bridges in the case of Aβ). It is known that salt bridge interactions have a particular tendency to affect various backbone conformations. Therefore, irrespective of salt bridge breakage, Aβ peptides change their conformations. This happens because of entropy changes during the transitions and a lack of an energetic drawback due to the absence of hydrogen bonds. Hence, various interchanging conformational structures are possible within the hydrogen bonded conformations and non-hydrogen bonded conformations (i.e., unfolded structures for salt bridges related to compact Aβ conformations, and hIAPP conformations without salt bridges).
When the salt bridge structure is converted to a hydrogen bond stable structure, a prominent transformation of energy occurs as a result of a decrease in entropy and an increase in enthalpy (Fig. 10). A lesser chance of salt bridge breakage leads to extended peptide structures with a loss of entropy due to various main salt bridge structures, and energy is equilibrated via increasing the conformational entropy of the system. Conformational changes of Aβ and hIAPP occur with the help of osmolytes (e.g., G-HCL and L-PRO), and extended peptide interactions between G-HCL and Aβ or hIAPP are stable, whereas L-PRO is similar to water, having moderate effects on both peptides. Furthermore, despite the simultaneous conversion of structures having fewer hydrogen-bonded ensembles and more salt bridge ensembles, both Aβ and hIAPP endured in the folded form in L-PRO treated systems because of crowding effects.
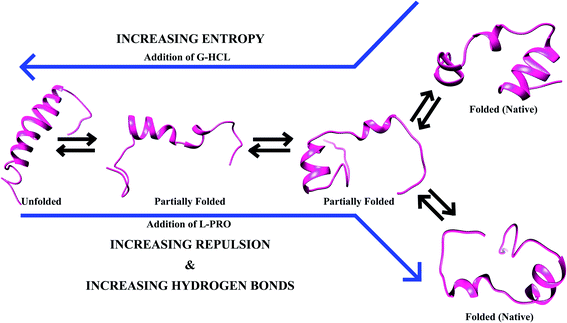 |
| Fig. 10 Substantial aid from the surroundings results in the dominant conformations. The inclusion of osmolytes makes compact conformations less favorable (G-HCL breaks hydrogen bonds; moving from native to unfolded Aβ and hIAPP) and extended conformations favorable (L-PRO makes intramolecular hydrogen bonds; moving from unfolded to native Aβ and hIAPP). | |
Conformational changes due to intramolecular hydrogen bonds and salt bridges play vital roles in maintaining the balance between several conformational ensembles of IDPs. Therefore, disparities within the shifts in equilibrium were prominent on either side. A critical observation due to these disparities is that none of the new structures appeared in the monomeric stage. This result shows that every ensemble was found to be complementary and was sampled synchronously over the entire conformational space available to Aβ and hIAPP peptides.
Discussion
Several diseases, such as neurodegenerative disorders, cancers, and cardiovascular diseases, involve IDPs. Drug discoveries for these diseases based on explorations of disease protein structures and functions could be of great importance. Aβ and hIAPP are two such IDPs responsible for amyloid aggregation and toxicity, leading to AD and T2D, respectively. Aβ is the major component of extracellular senile plaques, is intrinsically disordered, and is considered the hallmark of a brain affected by AD and associated amyloidopathies. hIAPP is a peptide hormone that plays an integral role in β-cell dysfunction and death by forming islet amyloid aggregates during T2D. Various studies have reported that conformational changes in these IDPs can lead to their aggregation in brains with AD and in pancreatic β-cells exposed to T2D, which thus supports the characterization of the structures of both Aβ and hIAPP.87 As is known, IDPs are highly dynamic in nature and have ample conformational ensembles, restricting, to a limited extent, the use of experimental methods to design new drugs.88 To study the conformational transitions (i.e., folding/misfolding) of various IDPs, a computational technique called REMD can be used for various replicas simulated over a wide range of temperatures.
Aβ and hIAPP monomeric structures are known to have the characteristics of IDPs. Aβ serves various physiological functions in humans, such as being involved in antimicrobial action, tumor suppression, sealing exposures in the blood–brain barrier, encouraging recovery from brain injury, and rectifying synaptic function.89 hIAPP serves as part of the endocrine pancreas, helping with glycemic control, and is a synergistic ally of insulin and the bone metabolism.90 However, both Aβ and hIAPP have shown conformational changes in their monomeric states that can lead to amyloid aggregation, which is crucial to the pathology of AD and T2D.4,91 Comprehending the conformational changes of the monomeric states of Aβ and hIAPP is important to prevent their aggregation and, therefore, AD and T2D.
Osmolytes, a group of small intracellular organic molecules and chaperones, are vital for regulating the folding and unfolding of proteins. G-HCL shows a robust denaturing effect on IDPs at physiological concentrations,92 although it has been shown that the protecting osmolyte L-PRO redirects amyloid aggregates to non-amyloidogenic amorphous aggregates through solubilizing monomers and decreasing the assembly of early transient aggregates.61 In this study, we performed REMD simulations on Aβ and hIAPP in the presence of a denaturant osmolyte (G-HCL) and a protecting osmolyte (L-PRO), observing exceptionally significant effects in the cases of the denaturant and protective osmolytes in terms of the behavior of the peptide conformational ensembles. With G-HCL, Aβ and hIAPP were found to be in unfolded and extended conformations; however, we observed a shift in the populations toward folded and compact forms in the case of L-PRO. We also observed AD-associated Aβ and T2D-associated hIAPP, both of which are intrinsically disordered proteins that change their unfolded and folded conformations under the influence of G-HCL and L-PRO, respectively. The local conformational changes in Aβ and hIAPP could further lead to modified folding, unfolding, or binding, conclusively culminating in altered functionality. The osmolytes affect Aβ and hIAPP amyloid aggregation and the association of insoluble fibrils that lead to AD and T2D, respectively.
Many previous studies have used REMD to investigate the conformational behavior of Aβ and hIAPP. One study investigated the role of osmolytes in protein misfolding and the aggregation of amyloid peptides.37 The effects of urea and TMAO during conformational sampling through REMD helped gain understanding regarding the shifts in populations between compact and extended structures. Another study analyzed the effects of osmolytes, using circular dichroism spectroscopic data from Aβ fragments, IAPP amyloidogenic fragments, and polyglutamine peptide fragments for conformational analysis and aggregation.93 Furthermore, Mimi and Roland examined the amyloidogenic properties of hIAPP in the presence of crowders and osmolytes in terms of their effects on the aggregation pathways of hIAPP and the roles of varied neutral and charged lipid bilayer systems, including biological membranes.94 Moreover, the atomic structures and thermodynamic details of hIAPP wild-type and mutant dimers and trimers were studied via REMD.95 Atomistic simulations showed oligomerization as a result of anti-parallel β-sheet formation between C-terminal segments. The central20–29 and C-terminal30–37 segments aid in maintaining stable secondary structures and encouraging intermolecular interactions. The hydrophobic amino acids (I26 and L27) facilitate oligomeric conformations and help in the elongation of fibrils.95
We implemented the REMD technique to cross potential energy barriers between unfolded conformations and to enhance convergence toward native structures. This atomistic simulation approach determined that the unfolding and folding mechanisms of IDPs depend on the osmolytes: G-HCL, as a denaturing agent, helps α-helix destabilization followed by β-sheet destabilization, and L-PRO, as a counter-denaturing agent, helps in the stabilization of α-helices and β-sheets. Furthermore, we observed an increase in Ree and Rg values in the cases of AβG-HCL+Water and hIAPPG-HCL+Water, showing the formation of extended peptide conformations. Additionally, secondary structure analysis revealed a decrease in β-sheet formation in the cases of AβG-HCL+Water and hIAPPG-HCL+Water, whereas formation was enhanced in the cases of AβWater, hIAPPWater, AβL-PRO+Water, and hIAPPL-PRO+Water. Moreover, the hydration patterns of AβG-HCL+Water and hIAPPG-HCL+Water indicated that G-HCL confronted water molecules from both peptide surfaces, leading to the attachment of molecules at the peptide backbone and side chains. Conversely, L-PRO did not bind to the peptide backbone as much and helped to distribute water molecules around the peptide surfaces, leading to the stabilization of compact peptide structures.
Here, we report the role of osmolytes (G-HCL and L-PRO) on the regulation and aggregation of intrinsically disordered Aβ and hIAPP, displaying conformational ensembles of interconverting structures. The results from this study could enhance the understanding of the conformational behaviour of AD-associated Aβ and T2D-associated hIAPP.
Abbreviations
IDP | Intrinsically disordered protein |
Aβ | Amyloid beta |
AD | Alzheimer's disease |
hIAPP | Human islet amyloid polypeptide |
T2D | Type 2 diabetes |
REMD | Replica exchange molecular dynamics |
G-HCL | Guanidine hydrochloride |
L-PRO | L-Proline |
Author summary
Amyloid beta (Aβ) and human islet amyloid polypeptide (hIAPP) are considered to be prominent intrinsically disordered proteins (IDPs), which aggregate into neuritic plaques and toxic protofibrils, the hallmarks of Alzheimer's disease and type 2 diabetes. Here, we delineate the roles of some regulating agents, i.e., guanidine hydrochloride (G-HCL), a denaturant, and L-proline (L-PRO), a counter-denaturant, which can remarkably alter the balance of hydrogen bonds and/or salt bridges in IDPs, and successively explore the most dominant conformations. Through several replica exchange molecular dynamics (REMD) simulations, which act as atomistic simulations, we observed that the unfolding and folding of Aβ and hIAPP depends on the osmolytes, where G-HCL leads to α-helix destabilization followed by β-sheet destabilization and L-PRO leads to α-helix stabilization followed by β-sheet stabilization. These fine disturbances can drastically shift the population of IDPs from compact to extended conformations and thus can be exploited to modulate the critical aggregation mechanisms implicated in Alzheimer's disease and type 2 diabetes.
Author contributions
AK, PS and AG devised the mode of experimentation and its setup. AK performed the research work and addressed the manuscript.
Conflicts of interest
No conflicts of interest are declared by the authors regarding the content of this research article.
Acknowledgements
AG and PS are grateful to Jawaharlal Nehru University and Teri School of Advanced Studies for their management and other facilities. AG is thankful to University Grant Commission, India for the Faculty Recharge position. AK is grateful to Indian Council of Medical Research (ICMR), India for the SRF position.
References
- J. Gaugler, B. James, T. Johnson, A. Marin and J. Weuve, 2019 Alzheimer's disease facts and figures, Alzheimer's Dementia, 2019, 15(3), 321–387 CrossRef.
- A. Mukherjee, D. Morales-Scheihing, N. Salvadores, I. Moreno-Gonzalez, C. Gonzalez and K. Taylor-Presse, et al., Induction of IAPP amyloid deposition and associated diabetic abnormalities by a prion-like mechanism, J. Exp. Med., 2017, 214(9), 2591–2610 CrossRef CAS PubMed.
- M. C. Riddle and W. H. Herman, The cost of diabetes care—an elephant in the room, Diabetes Care, 2018, 41(5), 929–932 CrossRef PubMed.
- X. Zhang, Z. Fu, L. Meng, M. He and Z. Zhang, The early events that initiate β-amyloid aggregation in Alzheimer's disease, Front. Aging Neurosci., 2018, 10, 359 CrossRef CAS PubMed.
- L. Dumery, F. Bourdel, Y. Soussan, A. Fialkowsky, S. Viale and P. Nicolas, et al., β-Amyloid protein aggregation: its implication in the physiopathology of Alzheimer's disease, Pathol. Biol., 2001, 49(1), 72–85 CrossRef CAS PubMed.
- V. H. Finder and R. Glockshuber, Amyloid-β aggregation, Neurodegener. Dis., 2007, 4(1), 13–27 CrossRef CAS PubMed.
- A. Abedini and A. M. Schmidt, Mechanisms of islet amyloidosis toxicity in type 2 diabetes, FEBS Lett., 2013, 587(8), 1119–1127 CrossRef CAS PubMed.
- R. L. Hull, G. T. Westermark, P. Westermark and S. E. Kahn, Islet amyloid: a critical entity in the pathogenesis of type 2 diabetes, J. Clin. Endocrinol. Metab., 2004, 89(8), 3629–3643 CrossRef CAS PubMed.
- G. Cooper, J. Aitken and S. Zhang, Is type 2 diabetes an amyloidosis and does it really matter (to patients)?, Diabetologia, 2010, 53(6), 1011–1016 CrossRef CAS PubMed.
- S. T. Ferreira, M. V. Lourenco, M. M. Oliveira and F. G. De Felice, Soluble amyloid-b oligomers as synaptotoxins leading to cognitive impairment in Alzheimer's disease, Front. Cell. Neurosci., 2015, 9, 191 Search PubMed.
- U. Sengupta, A. N. Nilson and R. Kayed, The role of amyloid-β oligomers in toxicity, propagation, and immunotherapy, EBioMedicine, 2016, 6, 42–49 CrossRef PubMed.
- I. Benilova, E. Karran and B. De Strooper, The toxic Aβ oligomer and Alzheimer's disease: an emperor in need of clothes, Nat. Neurosci., 2012, 15(3), 349 CrossRef CAS PubMed.
- E. N. Cline, M. A. Bicca, K. L. Viola and W. L. Klein, The amyloid-β oligomer hypothesis: beginning of the third decade, J. Alzheimer's Dis., 2018, 64(s1), S567–S610 CAS.
- M. Verma, A. Vats and V. Taneja, Toxic species in amyloid disorders: oligomers or mature fibrils, Ann. Indian Acad. Neurol., 2015, 18(2), 138 CrossRef PubMed.
- J. Höppener, J. Verbeek, E. De Koning, C. Oosterwijk, K. Van Hulst and H. Visser-Vernooy, et al., Chronic overproduction of islet amyloid polypeptide/amylin in transgenic mice: lysosomal localization of human islet amyloid polypeptide and lack of marked hyperglycaemia or hyperinsulinaemia, Diabetologia, 1993, 36(12), 1258–1265 CrossRef PubMed.
- P. E. Wright and H. J. Dyson, Intrinsically disordered proteins in cellular signalling and regulation, Nat. Rev. Mol. Cell Biol., 2015, 16(1), 18 CrossRef CAS PubMed.
- A. K. Dunker, C. J. Brown, J. D. Lawson, L. M. Iakoucheva and Z. Obradović, Intrinsic disorder and protein function, Biochemistry, 2002, 41(21), 6573–6582 CrossRef CAS PubMed.
- F. Pazos, N. Pietrosemoli, J. A. García-Martín and R. Solano, Protein intrinsic disorder in plants, Front. Plant Sci., 2013, 4, 363 Search PubMed.
- J. J. Ward, J. S. Sodhi, L. J. McGuffin, B. F. Buxton and D. T. Jones, Prediction and functional analysis of native
disorder in proteins from the three kingdoms of life, J. Mol. Biol., 2004, 337(3), 635–645 CrossRef CAS PubMed.
- M. M. Babu, R. van der Lee, N. S. de Groot and J. Gsponer, Intrinsically disordered proteins: regulation and disease, Curr. Opin. Struct. Biol., 2011, 21(3), 432–440 CrossRef CAS PubMed.
- C. M. Dobson, Protein misfolding, evolution and disease, Trends Biochem. Sci., 1999, 24(9), 329–332 CrossRef CAS.
- Y.-A. Lim, L. M. Ittner, Y. L. Lim and J. Götz, Human but not rat amylin shares neurotoxic properties with Aβ42 in long-term hippocampal and cortical cultures, FEBS Lett., 2008, 582(15), 2188–2194 CrossRef CAS PubMed.
- V. Tiwari, V. Solanki and M. Tiwari, In vivo and in vitro techniques used to investigate Alzheimer's disease, Front. Life Sci., 2015, 8(4), 332–347 CrossRef CAS.
- M. Jorfi, C. D'Avanzo, R. E. Tanzi, D. Y. Kim and D. Irimia, Human neurospheroid arrays for in vitro studies of Alzheimer's disease, Sci. Rep., 2018, 8(1), 2450 CrossRef PubMed.
- X. Hou, L. Sun, Z. Li, H. Mou, Z. Yu and H. Li, et al., Associations of amylin with inflammatory markers and metabolic syndrome in apparently healthy Chinese, PLoS One, 2011, 6(9), e24815 CrossRef CAS PubMed.
- F. B. Stentz and A. E. Kitabchi, Activated T lymphocytes in Type 2 diabetes: implications from in vitro studies, Curr. Drug Targets, 2003, 4(6), 493–503 CrossRef CAS PubMed.
- P. Krotee, J. A. Rodriguez, M. R. Sawaya, D. Cascio, F. E. Reyes and D. Shi, et al., Atomic structures of fibrillar segments of hIAPP suggest tightly mated β-sheets are important for cytotoxicity, Elife, 2017, 6, e19273 CrossRef PubMed.
- S. Bhattacharya and X. Lin, Recent advances in computational protocols addressing intrinsically disordered proteins, Biomolecules, 2019, 9(4), 146 CrossRef CAS PubMed.
- G. Grasso, M. Rebella, S. Muscat, U. Morbiducci, J. Tuszynski and A. Danani, et al., Conformational dynamics and stability of U-shaped and S-shaped amyloid β assemblies, Int. J. Mol. Sci., 2018, 19(2), 571 CrossRef PubMed.
- A. I. Ilitchev, M. J. Giammona, T. D. Do, A. G. Wong, S. K. Buratto and J.-E. Shea, et al., Human islet amyloid polypeptide N-terminus fragment self-assembly: effect of conserved disulfide bond on aggregation propensity, J. Am. Soc. Mass Spectrom., 2016, 27(6), 1010–1018 CrossRef CAS PubMed.
- N. Liu, M. Duan and M. Yang, Structural properties of human IAPP dimer in membrane environment studied by all-atom molecular dynamics simulations, Sci. Rep., 2017, 7(1), 7915 CrossRef PubMed.
- S. T. Ngo, H. M. Hung, K. N. Tran and M. T. Nguyen, Replica exchange molecular dynamics study of the amyloid beta (11–40) trimer penetrating a membrane, RSC Adv., 2017, 7(12), 7346–7357 RSC.
- M. Auton, J. Rösgen, M. Sinev, L. M. F. Holthauzen and D. W. Bolen, Osmolyte effects on protein stability and solubility: a balancing act between backbone and side-chains, Biophys. Chem., 2011, 159(1), 90–99 CrossRef CAS PubMed.
- L. Hua, R. Zhou, D. Thirumalai and B. Berne, Urea denaturation by stronger dispersion interactions with proteins than water implies a 2-stage unfolding, Proc. Natl. Acad. Sci. U. S. A., 2008, 105(44), 16928–16933 CrossRef CAS PubMed.
- B. J. Bennion and V. Daggett, The molecular basis for the chemical denaturation of proteins by urea, Proc. Natl. Acad. Sci. U. S. A., 2003, 100(9), 5142–5147 CrossRef CAS PubMed.
- A. Tischer and M. Auton, Urea-temperature phase diagrams capture the thermodynamics of denatured state expansion that accompany protein unfolding, Protein Sci., 2013, 22(9), 1147–1160 CrossRef CAS PubMed.
- A. Kumari, R. Rajput, N. Shrivastava, P. Somvanshi and A. Grover, Synergistic approaches unraveling regulation and aggregation of intrinsically disordered β-amyloids implicated
in Alzheimer's disease, Int. J. Biochem. Cell Biol., 2018, 99, 19–27 CrossRef CAS PubMed.
- C. Camilloni, A. G. Rocco, I. Eberini, E. Gianazza, R. Broglia and G. Tiana, Urea and guanidinium chloride denature protein L in different ways in molecular dynamics simulations, Biophys. J., 2008, 94(12), 4654–4661 CrossRef CAS PubMed.
- F. Rashid, S. Sharma and B. Bano, Comparison of guanidine hydrochloride (GdnHCl) and urea denaturation on inactivation and unfolding of human placental cystatin (HPC), Protein J., 2005, 24(5), 283–292 CrossRef CAS PubMed.
- A. K. Bhuyan, Protein stabilization by urea and guanidine hydrochloride, Biochemistry, 2002, 41(45), 13386–13394 CrossRef CAS PubMed.
- X. Tadeo, B. López-Méndez, D. Castaño, T. Trigueros and O. Millet, Protein stabilization and the Hofmeister effect: the role of hydrophobic solvation, Biophys. J., 2009, 97(9), 2595–2603 CrossRef CAS PubMed.
- M. Patra, C. Mukhopadhyay and A. Chakrabarti, Probing conformational stability and dynamics of erythroid and nonerythroid spectrin: effects of urea and guanidine hydrochloride, PLoS One, 2015, 10(1), e0116991 CrossRef PubMed.
- A. Fonin, A. Golikova, I. Zvereva, S. D'Auria, M. Staiano and V. Uversky, et al., Osmolyte-Like Stabilizing Effects of Low GdnHCl Concentrations on D-Glucose/D-Galactose-Binding Protein, Int. J. Mol. Sci., 2017, 18(9), 2008 CrossRef PubMed.
- B. Murray, J. Rosenthal, Z. Zheng, D. Isaacson, Y. Zhu and G. Belfort, Cosolute effects on amyloid aggregation in a nondiffusion limited regime: intrinsic osmolyte properties and the volume exclusion principle, Langmuir, 2015, 31(14), 4246–4254 CrossRef CAS PubMed.
- Y. Wang, H. He, L. Liu, C. Gao, S. Xu and P. Zhao, et al., Inactivation and unfolding of protein tyrosine phosphatase from Thermus thermophilus HB27 during urea and guanidine hydrochloride denaturation, PLoS One, 2014, 9(9), e107932 CrossRef PubMed.
- C. O. Nwamba and F. C. Chilaka, Kinetic Analysis of Guanidine Hydrochloride Inactivation of β-Galactosidase in the Presence of Galactose, Enzyme Res., 2012, 2012, 1–13 CrossRef PubMed.
- T. Narimoto, K. Sakurai, A. Okamoto, E. Chatani, M. Hoshino and K. Hasegawa, et al., Conformational stability of amyloid fibrils of β2-microglobulin probed by guanidine-hydrochloride-induced unfolding, FEBS Lett., 2004, 576(3), 313–319 CrossRef CAS PubMed.
- R. F. Greene and C. N. Pace, Urea and guanidine hydrochloride denaturation of ribonuclease, lysozyme, α-chymotrypsin, and β-lactoglobulin, J. Biol. Chem., 1974, 249(17), 5388–5393 CAS.
- F. Mehrnejad, M. Khadem-Maaref, M. M. Ghahremanpour and F. Doustdar, Mechanisms of amphipathic helical peptide denaturation by guanidinium chloride and urea: a molecular dynamics simulation study, J. Comput.-Aided Mol. Des., 2010, 24(10), 829–841 CrossRef CAS PubMed.
- P. E. Mason, J. W. Brady, G. W. Neilson and C. E. Dempsey, The interaction of guanidinium ions with a model peptide, Biophys. J., 2007, 93(1), L04–L6 CrossRef CAS PubMed.
- D. R. Canchi and A. E. Garcia, Cosolvent effects on protein stability, Annu. Rev. Phys. Chem., 2013, 64, 273–293 CrossRef CAS PubMed.
- S. W. Englander and L. Mayne, The nature of protein folding pathways, Proc. Natl. Acad. Sci. U. S. A., 2014, 111(45), 15873–15880 CrossRef CAS PubMed.
- W. J. Wedemeyer, E. Welker and H. A. Scheraga, Proline cis– trans isomerization and protein folding, Biochemistry, 2002, 41(50), 14637–14644 CrossRef CAS PubMed.
- A. Borgia, K. R. Kemplen, M. B. Borgia, A. Soranno, S. Shammas and B. Wunderlich, et al., Transient misfolding dominates multidomain protein folding, Nat. Commun., 2015, 6, 8861 CrossRef CAS PubMed.
- F. Rousseau, J. Schymkowitz and L. S. Itzhaki, Implications of 3D domain swapping for
protein folding, misfolding and function, Protein Dimerization and Oligomerization in Biology, Springer, 2012. pp. 137–152 Search PubMed.
- F. Rousseau, J. W. Schymkowitz and L. S. Itzhaki, The unfolding story of three-dimensional domain swapping, Structure, 2003, 11(3), 243–251 CrossRef CAS PubMed.
- F. Rousseau, J. Schymkowitz, H. Wilkinson and L. Itzhaki, Three-dimensional domain swapping in p13suc1 occurs in the unfolded state and is controlled by conserved proline residues, Proc. Natl. Acad. Sci. U. S. A., 2001, 98(10), 5596–5601 CrossRef CAS.
- C. M. Eakin, A. J. Berman and A. D. Miranker, A native to amyloidogenic transition regulated by a backbone trigger, Nat. Struct. Mol. Biol., 2006, 13(3), 202 CrossRef CAS.
- T. R. Jahn, M. J. Parker, S. W. Homans and S. E. Radford, Amyloid formation under physiological conditions proceeds via a native-like folding intermediate, Nat. Struct. Mol. Biol., 2006, 13(3), 195 CrossRef CAS PubMed.
- J. S. Pedersen, G. Christensen and D. E. Otzen, Modulation of S6 fibrillation by unfolding rates and gatekeeper residues, J. Mol. Biol., 2004, 341(2), 575–588 CrossRef CAS PubMed.
- T. Borwankar, C. Röthlein, G. Zhang, A. Techen, C. Dosche and Z. Ignatova, Natural osmolytes remodel the aggregation pathway of mutant huntingtin exon 1, Biochemistry, 2011, 50(12), 2048–2060 CrossRef CAS PubMed.
- F. Macchi, M. Eisenkolb, H. Kiefer and D. E. Otzen, The effect of osmolytes on protein fibrillation, Int. J. Mol. Sci., 2012, 13(3), 3801–3819 CrossRef CAS.
- D. Samuel, T. K. S. Kumar, G. Ganesh, G. Jayaraman, P.-W. Yang and M.-M. Chang, et al., Proline inhibits aggregation during protein refolding, Protein Sci., 2000, 9(2), 344–352 CrossRef CAS PubMed.
- S. Choudhary, S. N. Save, N. Kishore and R. V. Hosur, Synergistic Inhibition of Protein Fibrillation by Proline and Sorbitol: Biophysical Investigations, PLoS One, 2016, 11(11), e0166487 CrossRef.
- A. Taler-Verčič, S. Hasanbašić, S. Berbić, V. Stoka, D. Turk and E. Žerovnik, Proline residues as switches in conformational changes leading to amyloid fibril formation, Int. J. Mol. Sci., 2017, 18(3), 549 CrossRef.
- A. Kouranov, L. Xie, J. de la Cruz, L. Chen, J. Westbrook and P. E. Bourne, et al., The RCSB PDB information portal for structural genomics, Nucleic Acids Res., 2006, 34(suppl_1), D302–D305 CrossRef CAS PubMed.
- M. J. Abraham, T. Murtola, R. Schulz, S. Páll, J. C. Smith and B. Hess, et al., GROMACS: High performance molecular simulations through multi-level parallelism from laptops to supercomputers, SoftwareX, 2015, 1, 19–25 CrossRef.
- U. Essmann, L. Perera, M. L. Berkowitz, T. Darden, H. Lee and L. G. Pedersen, A smooth particle mesh Ewald method, J. Chem. Phys., 1995, 103(19), 8577–8593 CrossRef CAS.
- G. A. Kaminski, H. A. Stern, B. J. Berne, R. A. Friesner, Y. X. Cao and R. B. Murphy, et al., Development of a polarizable force field for proteins via ab initio quantum chemistry: first generation model and gas phase tests, J. Comput. Chem., 2002, 23(16), 1515–1531 CrossRef CAS PubMed.
- W. L. Jorgensen, J. Chandrasekhar, J. D. Madura, R. W. Impey and M. L. Klein, Comparison of simple potential functions for simulating liquid water, J. Chem. Phys., 1983, 79(2), 926–935 CrossRef CAS.
- H. J. Berendsen, Jv Postma, W. F. van Gunsteren, A. DiNola and J. Haak, Molecular dynamics with coupling to an external bath, J. Chem. Phys., 1984, 81(8), 3684–3690 CrossRef CAS.
- W. G. Hoover, Canonical dynamics: equilibrium phase-space distributions, Phys. Rev. A, 1985, 31(3), 1695 CrossRef PubMed.
- B. Hess, H. Bekker, H. J. Berendsen and J. G. Fraaije, LINCS: a linear constraint solver for molecular simulations, J. Comput. Chem., 1997, 18(12), 1463–1472 CrossRef CAS.
- S. Miyamoto and P. A. Kollman, Settle: an analytical version of the SHAKE and RATTLE algorithm for rigid water models, J. Comput. Chem., 1992, 13(8), 952–962 CrossRef CAS.
- R. Denschlag, M. Lingenheil and P. Tavan, Optimal temperature ladders in replica exchange simulations, Chem. Phys. Lett., 2009, 473(1–3), 193–195 CrossRef CAS.
- R. Qi, G. Wei, B. Ma and R. Nussinov, Replica Exchange Molecular Dynamics: A Practical Application Protocol with Solutions to Common Problems and a Peptide Aggregation and Self-Assembly Example, Peptide Self-Assembly, Springer, 2018. pp. 101–119 Search PubMed.
- A. Patriksson and D. van der Spoel, A temperature predictor for parallel tempering simulations, Phys. Chem. Chem. Phys., 2008, 10(15), 2073–2077 RSC.
- Y. Nozaki and C. Tanford, The solubility of amino acids, diglycine, and triglycine in aqueous guanidine hydrochloride solutions, J. Biol. Chem., 1970, 245(7), 1648–1652 CAS.
- X. Daura, K. Gademann, H. Schäfer, B. Jaun, D. Seebach and W. F. van Gunsteren, Τhe β-peptide hairpin in solution: conformational study of a β-hexapeptide in methanol by NMR spectroscopy and MD simulation, J. Am. Chem. Soc., 2001, 123(10), 2393–2404 CrossRef CAS.
- H. Kokubo, C. Y. Hu and B. M. Pettitt, Peptide conformational preferences in osmolyte solutions: transfer free energies of decaalanine, J. Am. Chem. Soc., 2011, 133(6), 1849–1858 CrossRef CAS.
- S. Jamal, A. Kumari, A. Singh, S. Goyal and A. Grover, Conformational Ensembles of α-Synuclein Derived Peptide with Different Osmolytes from Temperature Replica Exchange Sampling, Front. Neurosci., 2017, 11, 684 CrossRef.
- P. E. Mason, G. W. Neilson, J. E. Enderby, M.-L. Saboungi, C. E. Dempsey and A. D. MacKerell, et al., The structure of aqueous guanidinium chloride solutions, J. Am. Chem. Soc., 2004, 126(37), 11462–11470 CrossRef CAS PubMed.
- E. P. O'Brien, R. I. Dima, B. Brooks and D. Thirumalai, Interactions between hydrophobic and ionic solutes in aqueous guanidinium chloride and urea solutions: lessons for protein denaturation mechanism, J. Am. Chem. Soc., 2007, 129(23), 7346–7353 CrossRef.
- Ł. Mioduszewski and M. Cieplak, Disordered peptide chains in an α-C-based coarse-grained model, Phys. Chem. Chem. Phys., 2018, 20(28), 19057–19070 RSC.
- D. Song, R. Luo and H.-F. Chen, The IDP-specific force field ff14IDPSFF improves the conformer sampling of intrinsically disordered proteins, J. Chem. Inf. Model., 2017, 57(5), 1166–1178 CrossRef CAS.
- L. X. Peterson, A. Roy, C. Christoffer, G. Terashi and D. Kihara, Modeling disordered protein interactions from biophysical principles, PLoS Comput. Biol., 2017, 13(4), e1005485 CrossRef.
- V. N. Uversky, C. J. Oldfield and A. K. Dunker, Intrinsically disordered proteins in human diseases: introducing the D2 concept, Annu. Rev. Biophys., 2008, 37, 215–246 CrossRef CAS PubMed.
- S. Ambadipudi and M. Zweckstetter, Targeting intrinsically disordered proteins in rational drug discovery, Expert Opin. Drug Discovery, 2016, 11(1), 65–77 CrossRef CAS PubMed.
- H. M. Brothers, M. L. Gosztyla and S. R. Robinson, The Physiological Roles of Amyloid-β Peptide Hint at New Ways to Treat Alzheimer's Disease, Front. Aging Neurosci., 2018, 10, 118 CrossRef.
- R. Akter, P. Cao, H. Noor, Z. Ridgway, L.-H. Tu and H. Wang, et al., Islet amyloid polypeptide: structure, function, and pathophysiology, J. Diabetes Res., 2016, 2016, 1–18 CrossRef.
- D. C. R. Camargo, K. Tripsianes, K. Buday, A. Franko, C. Göbl and C. Hartlmüller, et al., The redox environment triggers conformational changes and aggregation of hIAPP in Type II Diabetes, Sci. Rep., 2017, 7, 44041 CrossRef.
- H. L. Grothe, M. R. Little, A. S. Cho, A. J. Huang and C. Yuan, Denaturation and solvent effect on the conformation and fibril formation of TGFBIp, Mol. Vision, 2009, 15, 2617 CAS.
- M. Inayathullah and J. Rajadas, Effect of osmolytes on the conformation and aggregation of some amyloid peptides: CD spectroscopic data, Data Brief, 2016, 7, 1643–1651 CrossRef PubMed.
- M. Gao and R. Winter, The effects of lipid membranes, crowding and osmolytes on the aggregation, and fibrillation propensity of human IAPP, J. Diabetes Res., 2015, 2015, 1–21 Search PubMed.
- S. Bouzakraoui and N. Mousseau, Structural and thermodynamical properties of early human amylin oligomers using replica exchange molecular dynamics: mutation effect of three key residues F15, H18 and F23, Phys. Chem. Chem. Phys., 2017, 19(46), 31290–31299 RSC.
Footnote |
† Electronic supplementary information (ESI) available. See DOI: 10.1039/d0ra00429d |
|
This journal is © The Royal Society of Chemistry 2020 |