DOI:
10.1039/C9RA09660D
(Paper)
RSC Adv., 2020,
10, 2589-2597
Development and evaluation of an automated multi-channel multiplug filtration cleanup device for pesticide residue analysis on mulberry leaves and processed tea†
Received
19th November 2019
, Accepted 24th December 2019
First published on 14th January 2020
Abstract
An automated multi-channel multiplug filtration cleanup (m-PFC) device was designed and developed. m-PFC columns were suitably installed in the device. The cycle times, speed and nitrogen pressure parameters of the m-PFC column were optimized. The device was utilized to analyze the 82 pesticide residues in fresh mulberry leaves and processed tea with GC-MS/MS detection. Method validation was performed on 82 pesticide residues in fresh mulberry leaves and processed tea at spiked levels of 0.01, 0.05 and 0.5 mg kg−1. The fortified recoveries of 82 pesticides were 72–115% and the relative standard deviations were 1–15%, except for diniconazole and clodinafop-propargyl in mulberry leaves. The automated multi-channel m-PFC device was successfully applied to detect the pesticide residues in fresh mulberry leaves and processed tea samples. With comparison to the conventional QuEChERS method, the current method using this device did not need additional vortex or centrifugation steps, and could process 48–64 samples in about one hour. The automated m-PFC method saved labor and improved the precision and was shown to be efficient and practical in pesticide residue analysis.
1. Introduction
Mulberry belongs to the family Moraceae (Morus), and is a rapidly growing deciduous perennial plant, which is distributed widely around the world, especially in oriental countries. In China, the land available for mulberry is more than a million hectares. At first, mulberry leaves are used to feed silkworms, and mulberry silk is an essential raw material for silk textiles.1 Mulberry is recognized as a “medicine and food homology” plant in China. With the development of “functional food” and “wellness” tea and food made from mulberry leaves are becoming popular.2 Mulberry leaves have attracted wide attention due to their medicinal and economic value.3 Mulberry leaves contain many active components, including phenolic acids, flavonoids, alkaloids, terpenoids, and steroids.4,5 Several studies have shown that mulberry leaves have antidiabetic, hypolipidemic, and antioxidant effects.6–8 Additionally, mulberry leaves are rich in protein, amino acids, vitamins, and trace elements, and have full future application in animal production. Many studies have found that using the extract of mulberry leaves or adding mulberry leaves to livestock and poultry diets affect production performance and quality of animal products.9,10
Pesticides play an irreplaceable role in agricultural production. It can be divided into herbicide, bactericide, insecticide, and plant growth regulators according to its use. The excessive use of pesticides also contaminates the environment and endanger the health of human beings.11,12 The detection of pesticide residues is an important measure to ensure food safety. However, the traditional analysis methods of pesticide residues exist shortcomings of experiment trouble, time-consuming and laborious, narrow scope of application, and large dosage of chemical reagents. A rapid detection method is hotspots in pesticide residues research area in recent years. Dispersive solid-phase extraction (d-SPE) is a simple, rapid, and low-cost sample preparation method.13 The adsorbent is directly added to the supernatant containing the target analytes, which can effectively remove co-extract of matrix14 and achieve the better cleanup effect without other equipment.15,16 The QuEChERS method (quick, easy, cheap, effective, rugged, and safe)17 is a fast multi-residue preparation method using dispersion solid-phase extraction to clean the extract. Compared with Soxhlet extraction and solid-phase extraction, the method is simple, efficient, solvent-saving, time-saving, and widely applicable. In most cases, the sample preparation of pesticide residues is detected by manual method at present; therefore, it is difficult to detect a large number of samples quickly and accurately. For example, the extraction and cleanup of QuEChERS method still consume lots of time and labor to analyze mountains of samples. With the rapid development in science and technology, the manual work will be substituted by intelligent equipment gradually, which is also a challenge and an opportunity for pesticide residue analysis.
The multiplug filtration cleanup (m-PFC) method basing on QuEChERS was developed by our research group.18–22 It had been successfully applied to the detection of pesticides and veterinary drugs in agricultural products. In our previous studies, we focused on optimizing the times of pull and push of m-PFC column.23–25 In this study, an automated multi-channel multiplug filtration cleanup device was developed, which could improve the efficiency and precision and avoid the error causing by manual operation. In order to evaluate the automated device, the recoveries of 82 pesticides in mulberry leaf and mulberry processed tea were investigated under different parameters. The instrument parameters were optimized in this study, and then the method was successfully applied to the detection of pesticide residues in market samples.
2. Material and methods
2.1 Standards, reagents, and materials
Pesticide standards (purities in 95–99.8%) used in the study were obtained from the Institute of the Control of Agrochemicals, Ministry of Agriculture People's Republic of China. The concentration of the mixed standard stock solution was 10 mg kg−1, which was prepared by chromatographic grade acetonitrile and stored at below 20 °C. Acetonitrile was purchased from Fisher Chemicals (Fair Lawn, NJ, USA). Ultrapure water was made from a Milli-Q device (Bedford, MA, USA). The analytical reagent grade sodium chloride (NaCl) and anhydrous magnesium sulfate (MgSO4) were purchased from Sinopharm Chemical Reagent (Beijing, China). Primary secondary amine (PSA), C18 and the m-PFC column were obtained from HAMAG instrument technology Co., Ltd. (China). Multiwalled Carbon Nanotubes (MWCNTs) with particle range of diameters 7–15 nm were provided by Beijing BTMA Biotechnology Co., Ltd. (China).
2.2 GC-MS/MS conditions
The analytes were detected by Thermo Scientific TSQ8000 triple quadrupole mass spectrometer with a Trace 1310 gas chromatograph and a TriPlus AI 1310 autosampler (Thermo Fisher Scientific, San Jose, CA). The chromatographic separation column was a Rxi®-5Sil MS capillary column (20 m × 0.18 mm, 0.18 μm film thickness) (Restek, USA). The temperature program of the column was 40 °C (held for 0.6 min), increased to 180 °C at 30 °C min−1, then ramped to 280 °C at 10 °C min−1, finally up to 290 °C at 20 °C min−1 and held for 5 min. The injection port temperature was 250 °C, and the injection volume was 1 μL in splitless mode. Argon was selected as collision gas, and helium gas (99.999% purity) was used as carrier gas with a constant flow of 0.85 mL min−1. The temperature of transfer line to tandem MS and ion source all was 280 °C. The triple quadrupole MS was operated in electron ionization (EI) mode with the electron energy of 70 eV. The selected reaction monitoring (SRM) was used as an acquisition mode. The retention time, ion pair, and collision energy (CE) of each pesticide were shown in Table S1 in ESI.†18
2.3 Sample preparation
An amount of (2 ± 0.02 g) homogenized samples (fresh mulberry leaf and mulberry processed tea) were weighed into a 50 mL centrifuge tube. The standard stock solutions were spiked in blank samples at concentration levels of 0.5 mg kg−1, and the tube was placed at room temperature for 30 minutes before extraction. The samples were swelled with water (5 mL in mulberry leaf, 10 mL in mulberry processed tea) and the tube was shaken vigorously for 1 min. Then 10 mL acetonitrile was added in the tube, and the mixture was shaken by the vortex for 2 min. After that, 1 g of sodium chloride and 4 g of anhydrous MgSO4 were added in the tube which was placed in ice bath. When it returned to room temperature and was shaken for 2 min before centrifugation for 5 min at 3800 rpm for automated multi-channel m-PFC procedures.
2.4 Dispersive solid-phase extraction procedures
1 mL supernatant was transferred to a 2 mL centrifuge tube which contained the different sorbents. Then, the 2 mL tube was shaken for 2 min and centrifuged for 1 min at 10
000 rpm. Finally, the supernatant was filtered through a 0.22 μm nylon syringe filter into an autosampler vial for analysis.
In order to find the best proportion of sorbents of mulberry leaf and mulberry processed tea. Three combinations were selected as follows: (A) 10 mg MWCNTs + 150 mg anhydrous MgSO4; (B) 8 mg MWCNTs + 50 mg PSA + 150 mg anhydrous MgSO4; (C) 8 mg MWCNTs + 30 mg PSA + 20 mg C18 + 150 mg anhydrous MgSO4. Then, the best combination was packed in m-PFC column.
2.5 Automated multi-channel m-PFC device procedures
Automated multi-channel m-PFC device (Fig. 1) was based on multiplug filtration cleanup (m-PFC) which could achieve multiple solid-phase extraction and equilibrium. The cleanup and filter steps in QuEChERS method were accomplished automatically by the device. As shown in the Fig. S1,† the upper part of the device was composed of air pump and multichannel pipette, which could move vertically and horizontally to transport the extracts. Two shelves in the middle could move forward and backward. The pipette, m-PFC column, and filter were placed on the upper rack and the sample tray, buffer tray, and collection tray were on the lower shelf from left to right. The waste tank was sat at the bottom of the automated device. The automated device equipped with a signal generator, it was controlled by a computer program via connecting the signal. The automated device is composed of three parts: sample introduction, cleanup of the sample, and filter and collection. It could process multiple samples at the same time. Each sample channel had an independent air pump to ensure sufficient pressure for the sample to pass through the m-PFC column and equipped with an external source of nitrogen for cleaning complex samples. The sample was cleaned and filtered directly into a 2 mL vial. The working process of automated m-PFC device was shown in Fig. S2.† Firstly, an aliquot of 1 mL supernatant was transferred to sample tray manually. Then the multichannel pipette moved down to introduce the supernatant and transported it to m-PFC column. Secondly, the supernatant in the pipette tip were injected into the m-PFC column and increased the pressure by air pump or external source of nitrogen to let the supernatant through the sorbents completely. The purified extracts were collected in the buffer tray. Thirdly, the extracts were pipetted by multichannel pipette and were transported to filter shelf. The tips of pipette combined with filter membrane, then, the multichannel pipette moved down to the collection tray and pushed the extracts in 2 mL vial through filter membrane. Finally, the used pipettes were put into a waste tank at the bottom of device. The second step can be repeated for getting a better cleanup effect.
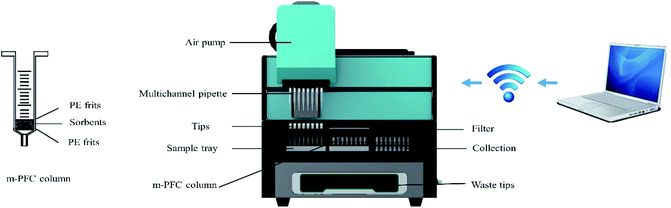 |
| Fig. 1 Schematic diagram of automated multi-channel m-PFC device. | |
3. Results and discussion
3.1 Optimization of d-SPE conditions
The common sorbents (PSA, C18, GCB, and MWCNTs) of QuEChERS method had been reported. PSA provided polar adsorption and weak anion exchange, which removed acid interfering substances and some polar pigments such as fatty acids, phenols, and carbohydrates. C18 was often used to remove non-polar substances such as lipids, which had a good cleanup effect on animal-derived agricultural products with high-fat content. GCB (graphitized carbon black)26,27 possessed special chemical structure, which adsorbed non-polar interferences such as pigments and sterols effectively. MWCNTs removed pigments and fatty acids with high efficiency.28–30
In order to clean the extract adequate, the purification effects of different combinations of PSA, C18, and MWCNTs were compared at the spiked level of 0.5 mg kg−1. The experiments were designed to transfer 1 mL acetonitrile supernatant of mulberry leaf and mulberry processed tea to 2 mL centrifugal tubes containing different amounts of sorbent mixtures (combinations A, B, and C). Mulberry leaf samples cleaned by MWCNTs appeared clear and colorless (Fig. 2(a)). Increased sorbent dosage could more sufficiently remove the impurity, but it also adsorbed target compounds. The recoveries of mostly pesticide were between 70% and 110% by combination A, and some analytes were decreased in combination B and C. The color of mulberry processed tea gradually lightened with the increase of sorbent sorts (Fig. 2(b)). Considering the complexity of mulberry processed tea, combinations A and C were selected for mulberry leaf and mulberry processed tea, respectively. The results of the recoveries were shown in Fig. 3.
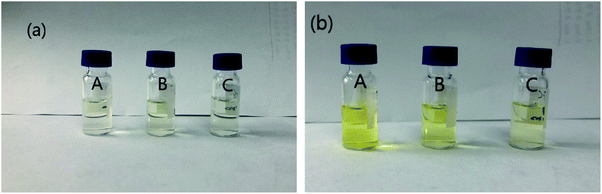 |
| Fig. 2 The visualization check of different combinations sorbents on fresh mulberry leaf (a) and mulberry processed tea (b) at the spiked level of 0.5 mg kg−1. (A) 10 mg MWCNTs + 150 mg MgSO4; (B) 8 mg MWCNTs + 50 mg PSA + 150 mg MgSO4; (C) 8 mg MWCNTs + 30 mg PSA + 20 mg C18 + 150 mg MgSO4. | |
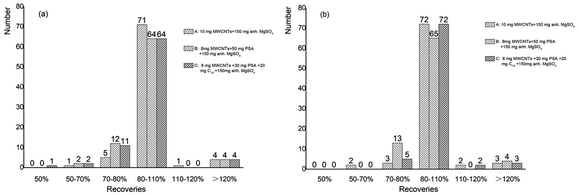 |
| Fig. 3 Histograms (X-axis was recoveries, Y-axis was the number of pesticides) about mulberry leaf (a) and mulberry processed tea (b) by three different cleanup procedures. | |
3.2 Optimization of automated multi-channel m-PFC device
The three parts of the automated device had its parameters (as shown in Table 1). The parameters of sample introduction and filter were just a transfer process and had no significant effect on results. The parameters of m-PFC column were optimized at a spiked level of 0.5 mg kg−1.
Table 1 The parameters of method about automated multi-channel m-PFC device
Method |
Parameter |
Sample introduction |
Volume: 1.0 mL |
Capture speed:5 mL min−1 |
Number of introduction: 1 |
Compensation volume: 1.0 mL |
M-PFC column |
Pushing speed: mL min−1 |
Waiting time: 5 s |
Nitrogen pressure: psi |
Duration: 10 s |
Number of cycles |
Buffer position: yes |
Filter |
Speed: 10 mL min−1 |
Waiting time: 5 s |
Nitrogen pressure: 30 psi |
Duration: 10 s |
3.2.1 Optimization of m-PFC column cycles. The m-PFC columns were packed with the optimized sorbents in the d-SPE procedure. The circles of m-PFC column were optimized in four levels of 0, 1, 2, and 3 by keeping other parameters invariant. The sample passed through the m-PFC column once, when the set value of cycle was zero. The color of supernatant of fresh mulberry leaf got lighter as cycle times rise. The recoveries of some pesticides were more than 110%, when the cycle was over two. The processed tea was more complex than leaf of mulberry. For mulberry processed tea, the more cycles were, the darker of color was. The recoveries of some pesticides increased sharply when the cycle was three. Based on our previous experiments, the interferences desorbing from the sorbents were more easily with the increase of cycle number. Cycle times of two and one were chosen for mulberry leaf and mulberry processed tea considering the recoveries and cleanup performance. The recoveries at different cycle times were shown in Fig. S3.†
3.2.2 Optimization of nitrogen pressure. In order to ensure that the extract passed through the m-PFC column completely, four levels of nitrogen pressure 0, 4, 8, and 12 psi were optimized. Nitrogen pressure had little effect on recoveries, and the average recoveries for most pesticides were in 70–110%. The recoveries of pesticides stabilized and relative standard deviation got smaller with the increase of nitrogen pressure. When nitrogen pressure was 12, the recoveries of some pesticides in fresh mulberry leaves were more than 110%. For mulberry processed tea, the matrix was complex and the resistance was higher passing through the column. Therefore, the final nitrogen pressure of fresh mulberry leaf and mulberry processed tea was 8 and 12 psi.
3.2.3 Optimization of pushing speed. The different speed of supernatant passing through the m-PFC column was controlled by an air pump. Five speeds (1, 2, 3, 4 and 5 mL min−1) of pushing were optimized, and the results were shown in Fig. 4. Box plot was drawn from five eigenvalues: maximum, minimum, median, and two quartiles. The value of distance over 1.5 times of quartile difference was defined as the outlier point, which was represented by “o” in the graph. The color of mulberry leaf and mulberry processed tea gradually deepened with the increase of pushing speed. The speed of mulberry leaf (a) through the column was more than 3 mL min−1, the color was obviously deepened. The average recoveries of 82 pesticides were better when speed was 1 and 3 mL min−1. In terms of dispersion degree, the recovery of 3 mL min−1 was concentrated. The average recovery was higher and the color was lighter in mulberry processed tea when the pushing speed was 2 mL min−1. Finally, the pushing speed through m-PFC column of mulberry leaf and mulberry processed tea was determined to 3 mL min−1 and 2 mL min−1.
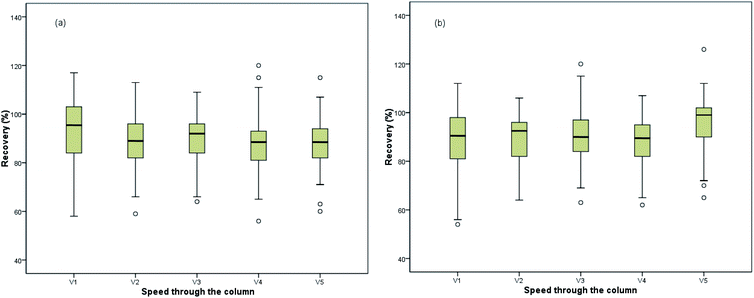 |
| Fig. 4 Box plots (X-axis was pushing speed, Y-axis was recoveries) about mulberry leaf (a) and mulberry processed tea (b) at different pushing speed. | |
Meanwhile, the waiting time of passing through the column and the duration of external nitrogen were optimized at 5, 10, and 15 seconds, while the impact on recoveries was not serious. In order to clean and collect all the supernatant in the column as far as possible, the waiting time and duration were determined to be 5 and 10 seconds, respectively.
3.3 Cross-contamination of automated device
In order to study the cross-contamination of pipette and sample tray during the movement of automated device, and the leakage of sample while using nitrogen. Standard solutions of 1 mL 0.5 mg kg−1 pyridaben and difenoconazole were crossed into the sample tray and 200 mg anhydrous magnesium sulfate was filled in the m-PFC column. The cross-contamination was studied when the cycle times of m-PFC column were 0, 1, and 2 (n = 6). The residue amount of pyridaben and difenoconazole in each sample was determined, and the results were shown in Table S2.† Difenoconazole was not found in standard solution of pyridaben and pyridaben was also not found in difenoconazole solution. The minimum detectable concentration of pyridaben and difenoconazole were 0.001 mg kg−1 and 0.005 mg kg−1. Obviously, there was no cross-contamination of samples when using the automated device.
3.4 Comparison of primarily automated m-PFC and auto m-FC
The three kinds of automated equipment were studied on mulberry processed tea spiked with the pesticides at 0.5 mg kg−1. Primarily automated m-PFC22 was developed to instead the process of push–pull of manual m-PFC, for holding the supernatant through the column at a constant speed. Rotation axis of the equipment was designed to lift the shelf up and down and pull and push the syringe piston, which let extracts circulate through the column to 2 mL of centrifuge tubes. However, there would be a few surpluses of the extracts, when the rotation axis pulled the syringe piston to introduce the extracts in centrifuge tubes. The extracts were pushed into the centrifugal tube after one cycle, mixing with the extracts which had not been cleaned. After cleanup, the extracts were manually filtered through a 0.22 μm filter membrane. Automated multi-filtration cleanup (Auto m-FC)31 introduced the supernatant through the extraction needle, and the pump syringe push extracts to the m-FC column through the pipeline. The pipeline must be flushed by acetonitrile after transporting one sample. The results of the three kinds of automated equipment were shown in Table 2. Recoveries and RSDs were no significant differences. Automated multi-channel m-PFC device could process 48–64 samples one time and each sample took less than a minute. It had an external nitrogen source, which provided sufficient pressure for the supernatant to pass through the m-PFC column completely. The device had a separate sample tray, buffer tray, and collection tray and filtered through a 0.22 μm filter membrane to 2 mL vial automatically. The majority of matrix effects performed enhancing effects by gas chromatography. As the total ion chromatogram (TIC) shown in Fig. S4,† automated multi-channel m-PFC device (III) had lower response in the mass spectrometry than other methods. It showed that the interferences of matrix were less and matrix effects were weaker.
Table 2 Comparison of the proposed method with other automated m-PFC methods
Method |
Recoveries (%) |
RSD (%) |
Sample number of batch process |
Cleanup time cost per sample (min) |
Automated filter |
Automated multi-channel m-PFC |
73–116 |
2–13 |
48–64 |
0.8 |
Yes |
Automated m-FC |
76–118 |
5–17 |
24 |
2–3 |
No |
Primary automated m-PFC |
72–124 |
1–19 |
6 |
2 |
No |
3.5 Method validation
The best parameter of device had been obtained to be able to make the precision and trueness well after cleanup process by optimization. Under this optimal condition, the fortified recoveries of 82 pesticides were studied at three concentration levels (0.01, 0.05 and 0.5 mg kg−1) by spiking standard pesticides for a blank sample. The validated parameters of the automated multi-channel m-PFC method were specificity, linear range, fortified recovery, precision, limit of detection (LOD), and limit of quantification (LOQ). The results were shown in Table 3. Linearity was evaluated by a calibration curve at five concentrations (0.01, 0.05, 0.1, 0.5, 1 mg kg−1), and the correlation coefficient (R2) was greater than 0.99. Precision was a measure of accidental error, expressing as repeatability and reproducibility. In this study, the relative standard deviation (RSD) was used to represent the precision. The limit of detection (LOD) and limit of quantification (LOQ) were defined as signal-to-noise (S/N) ratio of 3 and 10 at the lowest concentration of each analyte, respectively. LOQs were the lowest spike level (0.01 mg kg−1) of the method's validation in this study and the LOQs were regarded as LODs in respect of method validation.32
Table 3 Average recoveries and RSDs at three spiked level (n = 5), R2 of calibration curve
Pesticide |
Mulberry leaf |
Mulberry leaf tea |
Recovery, % (RSD, %) |
R2 |
Recovery, % (RSD, %) |
R2 |
0.01 mg kg−1 |
0.05 mg kg−1 |
0.5 mg kg−1 |
0.01 mg kg−1 |
0.05 mg kg−1 |
0.5 mg kg−1 |
Methomyl |
91(6) |
108(6) |
83(8) |
0.9998 |
107(9) |
101(11) |
92(8) |
0.9974 |
Dichlorvos |
74(10) |
89(8) |
107(8) |
0.9992 |
89(7) |
83(10) |
96(6) |
0.9995 |
Trichlorfon |
101(3) |
93(6) |
96(2) |
0.9992 |
93(6) |
88(6) |
97(4) |
0.9991 |
Etridiazole |
93(1) |
101(2) |
93(6) |
0.9995 |
98(7) |
95(8) |
93(5) |
0.9998 |
Propoxur |
81(7) |
95(3) |
97(4) |
0.9996 |
91(0) |
97(4) |
88(4) |
0.9998 |
Propachlor |
100(8) |
95(7) |
100(7) |
0.9993 |
76(1) |
79(9) |
86(7) |
0.9998 |
Ethoprophos |
76(3) |
99(1) |
97(3) |
0.9991 |
89(9) |
101(8) |
99(4) |
0.9991 |
Trifluralin |
85(3) |
98(5) |
103(4) |
0.9982 |
106(6) |
87(6) |
85(4) |
0.9978 |
Phorate |
75(2) |
83(4) |
101(3) |
0.9985 |
100(3) |
93(6) |
91(3) |
0.9990 |
Atrazine |
80(11) |
98(3) |
92(5) |
0.9991 |
87(4) |
97(13) |
97(5) |
0.9998 |
Propazine |
78(4) |
90(7) |
97(2) |
0.9998 |
90(9) |
86(7) |
92(3) |
0.9993 |
Clomazone |
101(2) |
93(3) |
97(4) |
0.9988 |
79(3) |
100(5) |
100(4) |
0.9998 |
Propyzamide |
92(5) |
99(3) |
93(8) |
0.9993 |
84(7) |
99(3) |
107(5) |
0.9996 |
Triallate |
92(4) |
95(8) |
99(5) |
0.9990 |
103(8) |
80(9) |
81(4) |
0.9959 |
Pirimicarb |
108(8) |
83(11) |
92(8) |
0.9982 |
89(6) |
94(7) |
98(4) |
0.9991 |
Acetochlor |
95(5) |
95(4) |
103(7) |
0.9984 |
87(1) |
85(10) |
101(2) |
0.9976 |
Propisochlor |
107(7) |
100(4) |
101(4) |
0.9983 |
92(10) |
86(9) |
104(4) |
0.9985 |
Metribuzin |
79(6) |
92(10) |
95(60 |
0.9995 |
111(7) |
83(7) |
102(4) |
0.9994 |
Tolclofos-methyl |
104(2) |
89(9) |
90(6) |
0.9986 |
99(3) |
90(5) |
96(3) |
0.9997 |
Propanil |
86(8) |
92(6) |
91(6) |
0.9995 |
92(7) |
84(9) |
97(7) |
0.9990 |
Metalaxyl |
104(7) |
91(6) |
107(7) |
0.9955 |
109(5) |
93(9) |
108(4) |
0.9993 |
Ametryne |
107(2) |
92(9) |
86(11) |
0.9975 |
112(10) |
98(10) |
105(5) |
0.9992 |
Acephate |
91(3) |
92(9) |
96(7) |
0.9980 |
83(6) |
74(5) |
98(1) |
0.9992 |
Prometryn |
83(2) |
87(8) |
95(8) |
0.9998 |
92(6) |
76(10) |
102(3) |
0.9996 |
Pirimiphos-methyl |
88(11) |
94(7) |
92(8) |
0.9976 |
106(14) |
102(11) |
102(4) |
0.9980 |
Fenitrothion |
90(4) |
92(7) |
98(3) |
0.9997 |
80(3) |
77(8) |
105(3) |
0.9987 |
Dimethoate |
82(5) |
93(4) |
98(2) |
0.9991 |
77(9) |
85(6) |
99(6) |
0.9991 |
Malathion |
90(12) |
91(9) |
96(4) |
0.9978 |
89(14) |
88(9) |
101(5) |
0.9983 |
Metolachlor |
97(6) |
91(7) |
99(4) |
0.9983 |
82(2) |
98(4) |
104(3) |
0.9987 |
Chlorpyrifos |
89(4) |
84(12) |
76(10) |
0.9987 |
88(3) |
76(6) |
78(4) |
0.9995 |
Diethofencarb |
91(3) |
88(9) |
88(7) |
0.9993 |
99(8) |
83(4) |
99(1) |
0.9991 |
Triadimefon |
103(3) |
97(10) |
102(8) |
0.9986 |
111(2) |
104(2) |
108(0) |
0.9991 |
Pendimethalin |
80(9) |
82(1) |
74(8) |
0.9980 |
85(10) |
82(9) |
84(4) |
0.9989 |
Thiamethoxam |
101(15) |
92(6) |
97(5) |
0.9995 |
107(10) |
110(6) |
100(9) |
0.9997 |
Fipronil |
88(8) |
96(6) |
96(5) |
0.9992 |
109(11) |
99(10) |
84(8) |
0.9995 |
Penconazole |
101(8) |
94(4) |
96(6) |
0.9995 |
107(6) |
91(10) |
102(4) |
0.9992 |
Phenthoate |
105(3) |
96(9) |
98(5) |
0.9992 |
108(9) |
97(4) |
103(3) |
0.9988 |
Procymidone |
81(2) |
92(4) |
94(6) |
0.9980 |
99(10) |
88(12) |
102(3) |
0.9993 |
Triadimenol |
103(7) |
92(7) |
104(4) |
0.9999 |
101(9) |
85(11) |
104(4) |
0.9997 |
Methidathion |
93(9) |
85(7) |
96(6) |
0.9994 |
102(8) |
96(1) |
86(4) |
0.9990 |
Butachlor |
79(1) |
92(7) |
98(4) |
0.9984 |
113(8) |
102(11) |
98(2) |
0.9988 |
Paclobutrazol |
87(12) |
94(5) |
100(3) |
0.9995 |
94(7) |
95(5) |
100(4) |
0.9994 |
Hexythiazox |
77(6) |
93(7) |
90(3) |
0.9991 |
89(3) |
107(9) |
96(5) |
0.9993 |
Napropamide |
89(12) |
83(7) |
88(7) |
0.9994 |
82(4) |
95(9) |
92(3) |
0.9992 |
Hexaconazole |
94(7) |
92(1) |
94(6) |
0.9995 |
84(12) |
83(11) |
98(3) |
0.9996 |
Pretilachlor |
97(11) |
90(6) |
97(5) |
0.9987 |
101(6) |
96(2) |
105(2) |
0.9990 |
Isoprothiolane |
94(12) |
91(4) |
98(2) |
0.9993 |
102(7) |
92(5) |
91(3) |
0.9988 |
Profenofos |
98(2) |
81(11) |
79(7) |
0.9993 |
92(9) |
83(14) |
83(4) |
0.9988 |
Oxadiazon |
90(8) |
92(8) |
96(3) |
0.9999 |
106(7) |
91(7) |
98(3) |
0.9992 |
Fludioxonil |
98(5) |
85(12) |
79(5) |
0.9990 |
87(2) |
87(6) |
96(3) |
0.9998 |
Myclobutanil |
89(5) |
95(8) |
100(5) |
0.9999 |
102(7) |
101(2) |
105(3) |
0.9998 |
Buprofezin |
104(9) |
109(9) |
97(4) |
0.9994 |
93(8) |
88(6) |
83(6) |
0.9991 |
Flusilazole |
78(10) |
93(5) |
95(3) |
0.9990 |
91(7) |
94(7) |
103(4) |
0.9990 |
Trifloxystrobin |
99(11) |
93(7) |
97(4) |
0.9992 |
95(8) |
98(3) |
106(4) |
0.9996 |
Kresoxim-methyl |
87(7) |
86(6) |
95(2) |
0.9993 |
93(5) |
102(9) |
102(1) |
0.9991 |
Carboxin |
101(14) |
89(4) |
90(5) |
0.9999 |
106(6) |
90(6) |
85(6) |
0.9997 |
Chlorfenapyr |
110(5) |
87(8) |
98(6) |
0.9988 |
97(8) |
82(4) |
93(5) |
0.9990 |
Cyproconazole |
82(4) |
89(7) |
98(4) |
0.9998 |
103(7) |
98(2) |
105(3) |
0.9991 |
Diniconazole |
63(9) |
68(2) |
62(7) |
0.9991 |
85(5) |
90(8) |
97(7) |
0.9990 |
Oxadixyl |
101(13) |
89(6) |
101(4) |
0.9999 |
100(6) |
99(4) |
101(4) |
0.9998 |
Triazophos |
79(8) |
78(8) |
80(5) |
0.9994 |
84(7) |
99(6) |
102(2) |
0.9993 |
Propiconazole |
97(11) |
92(6) |
95(2) |
0.9992 |
106(10) |
101(5) |
109(4) |
0.9991 |
Clodinafop-propargyl |
62(3) |
55(9) |
57(5) |
0.9994 |
86(9) |
83(7) |
100(4) |
0.9998 |
Tebuconazole |
84(9) |
98(2) |
89(7) |
0.9993 |
85(6) |
98(4) |
107(4) |
0.9995 |
Propargite |
110(2) |
91(6) |
96(2) |
0.9993 |
96(3) |
87(4) |
99(3) |
0.9996 |
Epoxiconazol |
75(4) |
72(8) |
86(6) |
0.9992 |
90(7) |
95(6) |
101(2) |
0.9991 |
Iprodione |
93(2) |
94(7) |
90(5) |
0.9998 |
98(6) |
92(11) |
83(9) |
0.9997 |
Bifenthrin |
86(6) |
80(7) |
90(5) |
0.9990 |
92(7) |
81(4) |
74(5) |
0.9995 |
Lambda-cyhalothrin |
91(7) |
78(3) |
94(4) |
0.9995 |
102(4) |
89(5) |
95(3) |
0.9991 |
Fenpropathrin |
95(12) |
85(5) |
102(5) |
0.9985 |
90(4) |
81(9) |
94(6) |
0.9993 |
Triticonazole |
76(5) |
81(5) |
91(3) |
0.9997 |
100(6) |
95(4) |
102(1) |
0.9998 |
Pyriproxyfen |
83(7) |
77(9) |
75(5) |
0.9990 |
76(3) |
81(9) |
87(3) |
0.9995 |
Pyridaben |
86(6) |
80(6) |
89(6) |
0.9992 |
89(5) |
86(5) |
84(3) |
0.9995 |
Beta-cypermethrin |
95(11) |
87(4) |
91(4) |
0.9997 |
90(5) |
100(3) |
84(6) |
0.9996 |
Flumioxazin |
101(12) |
91(6) |
94(5) |
0.9998 |
100(6) |
94(6) |
107(3) |
0.9999 |
Esfenvalerate |
79(6) |
75(7) |
87(5) |
0.9998 |
85(4) |
92(2) |
87(5) |
1.0000 |
Difenoconazole |
76(5) |
91(9) |
86(7) |
0.9998 |
81(4) |
93(6) |
102(3) |
0.9995 |
Indoxacarb |
110(6) |
86(6) |
94(3) |
0.9998 |
91(5) |
103(12) |
100(4) |
0.9996 |
Deltamethrin |
99(9) |
86(4) |
92(6) |
0.9994 |
114(8) |
102(11) |
79(8) |
0.9998 |
Azoxystrobin |
86(12) |
87(9) |
95(6) |
0.9999 |
85(2) |
80(2) |
98(4) |
0.9999 |
Dimethomorph |
87(8) |
74(5) |
99(2) |
0.9996 |
104(7) |
92(7) |
98(4) |
0.9997 |
Famoxadone |
99(4) |
84(6) |
95(3) |
0.9998 |
111(8) |
98(10) |
100(3) |
0.9995 |
Matrix effect (ME) could lead to signal response of analyte enhanced or weakened because the existence of matrix co-extractives affected the ionization of target compounds.33,34 The matrix effects of automated m-PFC device and d-SPE methods in mulberry leaf and mulberry processed tea were investigated by comparing the slope ratio of matrix-matched standards and solvent standards. The results are summarized in Table S3 in ESI.† The values of ME were less than 0.9 regarding as matrix weakening effect, and matrix effect could be ignored when the values were in 0.9–1.1. The matrix enhancement effect (ME > 1.1) was observed in most target compounds. For the majority of pesticides, the ME values of m-PFC method were lower than that of d-SPE. The automated m-PFC device method had better cleanup performance.
4. Conclusion
In this study, a rapid and straightforward automated multi-channel m-PFC device combined with QuEChERS was developed to determine the 82 pesticide residues by GC-MS/MS in fresh mulberry leaf and mulberry processed tea. Meanwhile, the automated multi-channel m-PFC method was successfully applied to detect the pesticides in fresh mulberry leaf and mulberry processed tea samples which purchased from markets in Beijing and Shandong Province. Most pesticide residues were low and some pesticides were not found. The residues of propachlor and propanil in mulberry processed tea were higher, which could go up to 0.034 and 0.017 mg kg−1. Of the 82 targeted pesticides in fresh mulberry leaf, the higher residual concentrations of trichlorfon, propachlor, propanil, triadimenol and dichlorvos were found, with values ranging from 0.017 to 0.053 mg kg−1. The residues of dichlorvos in mulberry leaf and methomyl in mulberry processed tea were higher than others. Table 4 showed the results of 10 typical pesticides registering in mulberry or having residue limits in China. Maximum residue limits (MRLs) of mulberries were from China, the Codex Alimentarius Commission (CAC) and European Union (EU).
Table 4 The residual range of 10 typical pesticides in market sample
Pesticides |
Fresh mulberry leaf |
Mulberry processed tea |
MRLs (China/EU/CAC) |
Residual range (10−3 mg kg−1) |
Residual range (10−3 mg kg−1) |
10−3 mg kg−1 |
Indicating lower limit of analytical determination. Indicating the MRL values are from berries because there is no MRL for the pesticide in mulberries. |
Methomyl |
8.7–9.1 |
37.1 |
—/10a/70b |
Dichlorvos |
42.8 |
— |
—/10a/— |
Propoxur |
5.1–5.3 |
— |
—/50a/— |
Acetochlor |
5.9–6.8 |
— |
—/10a/— |
Dimethoate |
— |
7.8 |
—/10a/50b |
Malathion |
6.4–6.5 |
6.9 |
—/20a/1000b |
Chlorpyrifos |
5.0–5.2 |
5.1 |
—/10a/1000b |
Profenofos |
— |
— |
—/10a/70b |
Propargite |
— |
— |
—/10a/— |
Tebuconazole |
10.1–11.5 |
6.6–7.5 |
1500/1500/— |
In most cases, the recoveries of 82 pesticides were in 70–110% and RSDs were less than 15% by using the automated multi-channel m-PFC device. All of the validation parameters of the method met the requirements for pesticide analysis. After centrifugation, an aliquot of 1 mL supernatant of the sample was directly transferred to the sample tray of the automated device. The automated multi-channel m-PFC device didn't need additional vortex, centrifugation, and filter steps comparing with d-SPE, and the automated device handled 48–64 samples simultaneously. The automated multi-channel m-PFC device reduced the number of operating personnel, saved time, and operated easily, which achieved the full-automation of cleanup procedure of sample preparation.
Conflicts of interest
The authors have declared no conflict of interest.
Acknowledgements
This work was supported by the National Key R&D Program of China (Project No. 2018YFC1604401 and 2018YFC1604402) from the Chinese Ministry of Science and Technology.
References
- B. Bussaban, P. Kodchasee, S. Apinyanuwat, C. Kosawang and N. Jonglaekha, Plant Dis., 2017, 101, 1951 CrossRef.
- P. Pothinuch, A. Miyamoto, H. T. T. Nguyen and S. Tongchitpakdee, J. Funct. Foods, 2017, 38, 151–159 CrossRef CAS.
- E. M. Sánchez-Salcedo, P. Mena, C. García-Viguera, F. Hernández and J. J. Martínez, J. Funct. Foods, 2015, 18, 1039–1046 CrossRef.
- C. T. Horng, Z. H. Liu, Y. T. Huang, H. J. Lee and C. J. Wang, J. Food Drug Anal., 2017, 25, 862–871 CrossRef CAS PubMed.
- Y. Sheng, S. Zheng, T. Ma, C. Zhang, X. Ou, X. He, W. Xu and K. Huang, Sci. Rep., 2017, 7, 12041 CrossRef PubMed.
- J. Naowaboot, P. Pannangpetch, V. Kukongviriyapan, B. Kongyingyoes and U. Kukongviriyapan, Plant Foods Hum. Nutr., 2009, 64, 116–121 CrossRef CAS PubMed.
- Y. Wang, L. Xiang, C. Wang, C. Tang and X. He, PLoS One, 2013, 8, e71144 CrossRef CAS PubMed.
- N.-C. Yang, K.-Y. Jhou and C.-Y. Tseng, Food Chem., 2012, 132, 1796–1801 CrossRef CAS.
- Z. Zeng, J.-j. Jiang, J. Yu, X.-b. Mao, B. Yu and D.-w. Chen, J. Integr. Agric., 2019, 18, 143–151 CrossRef CAS.
- M. H. Abdeldaiem, H. G. M. Ali and M. I. Foda, Journal of Food Measurement and Characterization, 2017, 11, 1681–1689 CrossRef.
- Y. Wang, X. Miao, H. Wei, D. Liu, G. Xia and X. Yang, Food Anal. Methods, 2016, 9, 2133–2141 CrossRef.
- P. Zohrabi, M. Shamsipur, M. Hashemi and B. Hashemi, Talanta, 2016, 160, 340–346 CrossRef CAS PubMed.
- M. Anastassiades, S. J. Lehotay, D. Štajnbaher and F. J. Schenck, J. AOAC Int., 2003, 86, 412–431 CAS.
- M. H. Petrarca, J. O. Fernandes, H. T. Godoy and S. C. Cunha, Food Chem., 2016, 212, 528–536 CrossRef CAS.
- Y. Q. Wan, X. J. Mao, A. P. Yan, M. Y. Shen and Y. M. Wu, Biomed. Chromatogr., 2010, 24, 961–968 CAS.
- X. Mao, Y. Wan, A. Yan, M. Shen and Y. Wei, Talanta, 2012, 97, 131–141 CrossRef CAS PubMed.
- M. Anastassiades, K. Maštovská and S. J. Lehotay, J. Chromatogr. A, 2003, 1015, 163–184 CrossRef CAS PubMed.
- P. Zhao, B. Huang, Y. Li, Y. Han, N. Zou, K. Gu, X. Li and C. Pan, J. Agric. Food Chem., 2014, 62, 3710–3725 CrossRef CAS PubMed.
- N. Zou, Y. Han, Y. Li, Y. Qin, K. Gu, J. Zhang, C. Pan and X. Li, J. Agric. Food Chem., 2016, 64, 6061–6070 CrossRef CAS PubMed.
- Y. Han, L. Song, S. Liu, N. Zou, Y. Li, Y. Qin, X. Li and C. Pan, Food Chem., 2018, 241, 258–267 CrossRef CAS PubMed.
- Y. Han, L. Song, N. Zou, Y. Qin, X. Li and C. Pan, J. Sep. Sci., 2017, 40, 878–884 CrossRef CAS PubMed.
- Y. Qin, J. Zhang, Y. He, Y. Han, N. Zou, Y. Li, R. Chen, X. Li and C. Pan, J. Agric. Food Chem., 2016, 64, 6082–6090 CrossRef CAS PubMed.
- Y. Qin, B. Huang, J. Zhang, Y. Han, Y. Li, N. Zou, J. Yang and C. Pan, J. Sep. Sci., 2016, 39, 1757–1765 CrossRef CAS PubMed.
- Y. Qin, J. Zhang, Y. Li, Y. Han, N. Zou, Y. Jiang, J. Shan and C. Pan, Anal. Bioanal. Chem., 2016, 408, 5801–5809 CrossRef CAS PubMed.
- Y. Qin, P. Zhao, S. Fan, Y. Han, Y. Li, N. Zou, S. Song, Y. Zhang, F. Li, X. Li and C. Pan, J. Chromatogr. A, 2015, 1385, 1–11 CrossRef CAS PubMed.
- A. Islam, H. S. Lee, J. H. Ro, D. Kim and H. Kwon, J. Sep. Sci., 2019, 42, 2379–2389 CrossRef CAS PubMed.
- Q. Zhao, G.-L. Li, Y.-F. Ning, T. Zhou, Y. Mei, Z.-Z. Guo and Y.-Q. Feng, Microchem. J., 2019, 147, 67–74 CrossRef CAS.
- S. Fan, P. Zhao, C. Yu, C. Pan and X. Li, Food Addit. Contam., Part A: Chem., Anal., Control, Exposure Risk Assess., 2014, 31, 73–82 CrossRef CAS PubMed.
- P. Zhao, L. Wang, Y. Jiang, F. Zhang and C. Pan, J. Agric. Food Chem., 2012, 60, 4026–4033 CrossRef CAS PubMed.
- Y. Han, L. Song, N. Zou, R. Chen, Y. Qin and C. Pan, J. Chromatogr. B: Anal. Technol. Biomed. Life Sci., 2016, 1031, 99–108 CrossRef CAS PubMed.
- Y. Qin, J. Zhang, Y. Li, Q. Wang, Y. Wu, L. Xu, X. Jin and C. Pan, J. Chromatogr. A, 2017, 1515, 62–68 CrossRef CAS.
- https://ec.europa.eu/food/sites/food/files/plant/docs/pesticides_mrl_guidelines_wrkdoc_2017-11813.pdf, accessed on, 15 September 2018.
- X. Liu, J. Xu, Y. Li, F. Dong, J. Li, W. Song and Y. Zheng, Anal. Bioanal. Chem., 2011, 399, 2539–2547 CrossRef CAS PubMed.
- X. Q. Liu, Y. F. Li, W. T. Meng, D. X. Li, H. Sun, L. Tong and G. X. Sun, J. Chromatogr. B: Anal. Technol. Biomed. Life Sci., 2016, 1015–1016, 1–12 CrossRef CAS PubMed.
Footnote |
† Electronic supplementary information (ESI) available. See DOI: 10.1039/c9ra09660d |
|
This journal is © The Royal Society of Chemistry 2020 |
Click here to see how this site uses Cookies. View our privacy policy here.