DOI:
10.1039/C9RA05187B
(Paper)
RSC Adv., 2020,
10, 4928-4941
Metabolomics-driven identification of perturbations in amino acid and sphingolipid metabolism as therapeutic targets in a rat model of anorexia nervosa disease using chemometric analysis and a multivariate analysis platform†
Received
8th July 2019
, Accepted 25th November 2019
First published on 29th January 2020
Abstract
It is important to explore novel therapeutic targets and develop an effective strategy for the treatment of anorexia nervosa. In this work, serum samples were analyzed using ultra-performance liquid chromatography coupled with quadrupole time-of flight mass spectrometry (UPLC/Q-TOF MS) coupled with chemometric analysis and multivariate analysis to obtain the metabolites and their corresponding pathways. In addition, knock-in and knock-down of the key enzyme in vivo was performed to verify the reliability of the obtained metabolic pathway, which is closely associated with the anorexia nervosa pathomechanism and the potential targets. There were significant differences in the biochemical parameters between the model group and the control group. A total of 26 potential biomarkers were identified to resolve the difference between the control and model rats, which were closely related to amino acid metabolism, sphingolipid metabolism, arachidonic acid metabolism, the citrate cycle, and so forth. According to the ingenuity pathway analysis, we further elucidated the relationship between the gene, protein, and metabolite alteration in anorexia nervosa, which are involved in cellular compromise, lipid metabolism, small molecule biochemistry, cell signaling, molecular transport, nucleic acid metabolism, cell morphology, cellular function and maintenance. Arginosuccinate synthetase (ASS) deficiency was accompanied by a significant downregulation of the β-endorphin and ghrelin in the animal models. The metabolites and pathways obtained using the metabolomics strategy may provide valuable information for the early treatment for anorexia nervosa.
1. Introduction
Anorexia nervosa is a common and frequently-occurring disease in children, in which the appetite of a child is weak for a long period of time, the food intake is significantly reduced, and it can even result in food refusal.1,2 Previous research has shown that major disparities exist between different regions of the world and the prevalence of anorexia nervosa, with Latin America, Africa, and certain areas of the USA, such as those with a high population of Hispanic people, having a strikingly low rate of anorexia nervosa compared with Western countries such as those in Europe and other areas of the USA, China and Japan. The rate of prevalence of anorexia nervosa in China amounts to more than 1%, however, it accounts for less than 0.01% in Africa. In recent research conducted in Asia, children suffering from anorexia nervosa were mostly female.3,4 Long-term anorexia nervosa can lead to malnutrition, body weight loss, hypoimmunity, growth retardation and secondary infections, in addition, it also affects the mental health of children causing a huge burden on families and society.5 Anorexia nervosa in children is associated with various factors such as improper feeding, mental stress, and an irregular lifestyle.6 Studies have shown that the infant digestive system is not perfect at birth and can only be adapted to dairy foods. Improving the function of the digestive system requires food, adding food supplements in time can avoid the occurrence of anorexia nervosa in children. It can damage the digestive function if complementary foods are added too early, and this can lead to indigestion and obesity in children.7 At present, a gastric enzyme mixture, dry yeast tablets, morphine, amitriptyline and other drugs are used by clinical doctors in Western countries. In Asian countries such as China, acupuncture and chiropractic treatments are also used, as well as traditional Chinese medicine.8,9 Children as a special group have their own unique physiological characteristics, and drug treatment for pediatric diseases is much more complicated than for adults.10 In recent years, the development of novel therapeutics to treat anorexia nervosa has not kept pace with the increase in its prevalence, and one of the bottlenecks in the progress of such therapeutic advances is an incomplete understanding of the pathophysiology of anorexia nervosa in children.11 Therefore, there is an urgent need to find a new approach to deal with this issue.
Metabolomics is an emerging subject following on from genomics, transcriptomics and proteomics.12,13 Metabolomics offers a “top-down” approach and is based on the fact that metabolites reflect the cell environment, cell nutritional status, drug function and other environmental factors.14–17 In general, the subject of metabolomics concerns small molecule compounds with a molecular weight of 1000 or less. Compared with other omics, it has the following characteristics: subtle changes in gene and protein expression can be amplified on metabolites; metabolomics research does not require whole genome sequencing or a large number of databases to be established which express sequence tags; the number of metabolites is much smaller than that of genes and proteins; the kinds of metabolite are found to be roughly similar in different biological samples using metabolic research methods.18,19 This can not only determine how the different organisms respond after being disturbed by various internal and external environments, but also distinguish the phenotypic differences between different individuals of the same species and help to elucidate the mechanisms of individual differences.20 In addition, metabolomics can clarify drug targets or receptors through pharmacokinetics and provide insights into the changes in endogenous metabolites produced by drugs for guiding individualized drug treatment and evaluating clinical safety.21 In larger data, various analytical techniques are widely used such as nuclear magnetic resonance (NMR), gas chromatography-mass spectrometry (GC-MS), and liquid chromatography-mass spectrometry (LC-MS).22–24 Compared to GC-MS and NMR technology platforms, the sample detection requirements for LC-MS are low, and the technique demonstrates a high sensitivity, wide linear range, and it can also achieve good detection results for metabolites with a large difference in concentration. Combined with different mass analyzers, LC-MS meets the qualitative and quantitative requirements for small molecule materials and plays an important role in nontargeted and targeted metabolomics.25,26 However, to date, metabolomic studies of anorexia nervosa in children are still limited and methodologically heterogenous. Despite promising findings, there are no translatable biomarkers for this disorder in clinical practice.27
The aim of this study was to reveal the metabolites and biological pathway that can distinguish children with anorexia nervosa from healthy children based on ultra-performance liquid chromatography mass spectrometry (UPLC-MS) combined with multivariate data analysis and pathway analysis, which provides a novel method to probe the potential pathological mechanisms, therapeutic targets and the potential for novel drug development. Meanwhile, a knock-in and knock-down key enzyme in vivo assay was carried out to verify the reliability of the obtained metabolic pathway, which is closely related to the anorexia nervosa pathomechanism. A total of 26 potential biomarkers were identified to reflect the pathological changes in anorexia nervosa disease, and the metabolism of amino acids and sphingolipids were regarded as potential targets for capturing it.
2. Materials and methods
2.1 Chemicals and reagents
Methanol, acetonitrile and formic acid at HPLC grade were acquired from Merck Chemicals (Darmstadt, Germany). Distilled water (18.25 Ω) for the mobile phase and for preparation of the aqueous solutions was purchased from Wahaha purified water company (Hangzhou, China). Leucine enkephalin, with a purity of more than 99.0% was purchased from Sigma-Aldrich (St. Louis, MO, USA). Isofluorane, physiologic saline solution and sodium hydroxide were purchased from Sea Sky Bio Technology Co. Ltd (Beijing, China). The assay kit for serum β-endorphin and ghrelin was purchased from Merck Chemicals (Darmstadt, Germany). The assay kits for the serum total cholesterol (TC), total protein (TP), albumin (Alb), motilin (MTL) and gastrin (GAS) were obtained from Sigma Aldrich (St. Louis, MO). Other reagents and chemicals were of analytical grade.
2.2 Establishing the animal model
Male and female specific pathogen free (SPF) grade Sprague Dawley (SD) rats, 40–45 days old, were purchased from the Vital River Laboratory Animal Technology Co., Ltd (Beijing, China). They were subjected to one week of acclimatization under which the conditions were set as follows: pathogen-free, standard rodent pellet and water, 22–26 °C room temperature, 45 ± 5% relative humidity and a 12 h diurnal cycle. To avoid any external interference factors, the animals and cages were homologous during the entire process. All animal experiments were performed and ratified by the Animal Care and Use Committee of Harbin Medical University and the tenets recorded in the Declaration of Helsinki. After environmental adaptation, 40 rats were selected and allocated to two groups with 20 rats in each according to their body mass and food intake in order to obtain groups of animals with a similar body mass and food intake. The rats in the model group were fed a special feed and the rats in the control group were fed conventional food for 28 days. The special feed was composed of fish pine, milk powder, sugar, corn flour, soy flour, fresh eggs, and fresh meat fat meat at a proportion of 1
:
1
:
1
:
1 1
:
2
:
1.8
:
2.
2.3 Conventional index and biochemical index detection
The appearance, activity and eating status of the animals was observed daily. The body weight and eating status of the rats in the control and model group were recorded on day 1, 4, 8, 12, 16, 20, 24, and 28, respectively. The rats in the two groups were fasted on the 28th day at 8 o'clock. The two groups of rats were anesthetized using 10% chloral hydrate (5 mL kg−1) by intraperitoneal injection. Blood samples from the ventral vena cava were delivered into Eppendorf tubes to be centrifuged at 3000 rpm, 4 °C for 15 min. The obtained serum samples were collected and stored at −80 °C prior to kit detection and metabolomics analysis. After the blood was collected from the rats, the cardia was ligated and the entire stomach was removed. The gastric juice was carefully collected to be centrifuged at 3000 rpm, 4 °C for 15 min. The supernatant of the gastric juice was aspirated prior to the next analysis. All animals were injected with 3% pentobarbital sodium (0.1 ml/100 g, weight) for euthanasia.
2.4 Metabolomic analysis conditions
The serum samples were analyzed using a 2.1 × 100 mm C18 reverse-phase column with a 1.8 μm particle size (Waters Corp., Milford, MA, USA) using a Waters Acquity TM UPLC system equipped with a Waters accurate quadrupole-time-of-flight (Q-TOF) mass spectrometer (5500 Q-TRAP, AB SCIEX, USA). The procedure for the metabolomic study consisted of sample preparation, metabolite separation and detection, data preprocessing and statistical analysis for metabolite identification. The conditions for the chromatographic separation and mass spectrometry were explicitly narrated as follows: the column was maintained at 35 °C, the injected sample volume was 4 μL and the flow rate was maintained at 0.4 mL min−1. The optimized mobile phase contained water (A) and acetonitrile (B), which both contained 0.1% formic acid. The UPLC gradient program was 0–1 min 100% A, 1–3 min linear from 100 to 80% A, 3–5 min linear from 80 to 45% A, 5–8 min linear from 45 to 25% A, 8–10 min linear from 25 to 100% A, and 10–12 min 100% A. The autosampler was maintained at 4 °C.
The mass spectrometry (MS) was performed in the multiple reaction monitoring mode at a mass range of 50–1200 m/z in positive and negative ionization. For the positive electrospray mode, the optimal capillary voltage and cone voltage was set at 4500 and 35 V, respectively. The cone gas was set to 50 L h−1. The extraction cone voltage was set at 4 V, and the source temperature was set at 130 °C. The desolvation gas was set to 550 L h−1 at a temperature of 400 °C. For the negative electrospray mode, the optimal capillary voltage and cone voltage was set at 4000 and 30 V, respectively. The cone gas was set to 40 L h−1. The extraction cone voltage was set at 3 V, and the source temperature was set at 110 °C. The desolvation gas was set to 550 L h−1 at a temperature of 300 °C. Under the W optics mode with a 12
000 dpi resolution, the mass spectrometry was operated by dynamic range extension. The data acquisition rate was set to 0.1 s with a 0.15 s interscan delay. Leucine-enkephalin was considered as the lock mass at a concentration of 100 ng ml−1 and a flow rate of 5 μL min−1 to ensure the accuracy and reproducibility of the positive ion mode ([M + H]+ = 556.2771) and the negative ion mode ([M − H]− = 554.2614). All of the acquired data were imported to Prognosis QI and Markerlynx XS (Waters Corporation, MA, USA) within the Masslynx software for pretreatment.
2.5 UPLC-MS method assessment
The precision and repeatability of the UPLC-Q-TOF-MS method was assessed every day, the commercial software programs Progenesis QI and MassLynx developed by Nonlinear Dynamics (Waters Corporation) were applied to process the raw data and the missing data were seemingly undetectable and set to zero, then the data were log
2 transformed and normalized to the total signal for every rat. For analytical method assessment, 20 μL of each of the samples was mixed to give a quality control sample that was tested during the analysis. On the basis of different metabolite polarities and m/z values, nine distinctive peaks were extracted for the assessment of method validation. The relative standard deviation percentage (RSD%) of the retention times and peak areas were calculated to give an evaluation index. Injection precision was performed using the continuous analysis of six replicates of the same quality control samples. The RSD% of the retention times and the peak areas were 0.63% and 2.4%. The six parallel samples were analyzed using UPLC-high definition MS (HDMS) to evaluate the sample preparation repeatability. The RSD% of the retention times and peak areas were 0.57% and 2.8%. This method presents good repeatability and precision of this research.
2.6 Metabolomics data processing
The processed data matrices were input into the EZinfo 2.0 software (Waters Corporation, Milford, MA, USA) for further multivariate data analyses such as unsupervised principal component analysis (PCA), orthogonal projection to latent structure-discriminant analysis (OPLS-DA) and a variable importance in projection (VIP) score plot. PCA after the unit variance scaling process was employed to obtain the general separation of all samples, and then the differences in the metabolic profiling between groups were revealed by multivariate predictive modeling. The contribution to the variation in the S-plots and the corresponding VIP were calculated as well in metabolic profiling. After the unit variance scaling process, the number of components in the model was optimized using a seven fold cross-validation to avoid the overfitting problem of the OPLS-DA model. In addition, 200 times permutation tests were also analyzed, in which 200 models with randomly assigned labels are constructed for the samples, and a comparison is then performed between the goodness of fit of the original model and the goodness of fit of the permuted models (Fig. S1†). Finally, the validity of the OPLS-DA models was verified using an analysis of variance (ANOVA) of the cross-validated residuals (CV-ANOVA) test. Each potential biomarker was selected if the VIP-value was more than 1.0 while the P value of the independent sample t-test was less than 0.05. All statistical tests were two-sided, and the significant level was set at P < 0.05.
The identification of potential biomarkers was performed based on exact molecular weights, the m/z element composition (MassLynx i-FIT software, Waters Corporation, Milford, MA, USA), MS, MS E fragment, and literature comparisons. Compared with the actual value, the mass tolerance of the measured m/z was set to within 20 ppm. Online information for the biomarkers was obtained from databases including the Human Metabolome Database (http://www.hmdb.ca), KEGG (http://www.kegg.com), METLIN (https://metlin.scripps.edu), MassBank (https://massbank.eu/MassBank/) and Chemspider (http://www.chemspider.com/). Under the same UPLC-MS conditions, some metabolites were confirmed by the reference standards. The discrepant metabolite biomarkers were input into the MetaboAnalyst platform (http://www.metaboanalyst.ca/) to analyze the relative metabolic pathways to compare healthy children and those with anorexia nervosa. To systematically understand the difference in the metabolism between healthy children and those with anorexia nervosa, we uploaded the differentially expressed metabolite lists (with Human Metabolome Database identification numbers) onto an Ingenuity Pathway Analysis (IPA) server, which involved canonical pathways and analysis of multiple chemical–protein interaction networks to further clarify the relationship between metabolic changes.
2.7 Enzyme analysis
Lentivirus expressing full-length rats argininosuccinate synthetase (ASS) cDNA and lentivirus containing empty plasmids (vector) were constructed by Sigma-Aldrich (St. Louis, MO). In addition, lentivirus carrying shRNA against ASS and lentivirus containing nonspecific ASS (scramble) were also constructed by Sigma-Aldrich. After cleaning, the rats were injected with the recombinant vector using a 31 G needle. No toxic effects were found after treatment with the lentiviral vector.
3. Results
3.1 Conventional index and biochemical index analysis
Rats in the control group had shiny hair and were active, and the rats in the model group presented as being exasperated, having dry stools and hair, and showed reduced activity. On the first to the eighth day of the experiment, the average daily food intake of the model group was about 30% lower than that of the control group. The average daily food intake for the model group from the eighth to the 28th day was 40% to 50% lower than that of the control group, these significant differences can be seen in Fig. 1A. On the first to the eighth day of the experiment, the average body weight of the rats in the model group was less than 10% of the control group, and there was no significant difference compared with the control group. On the eighth to the 28th day, the average body weight of the rats in the model group was lower than that of the control group by 10% to 15%, this significant difference can be seen in Fig. 1B.
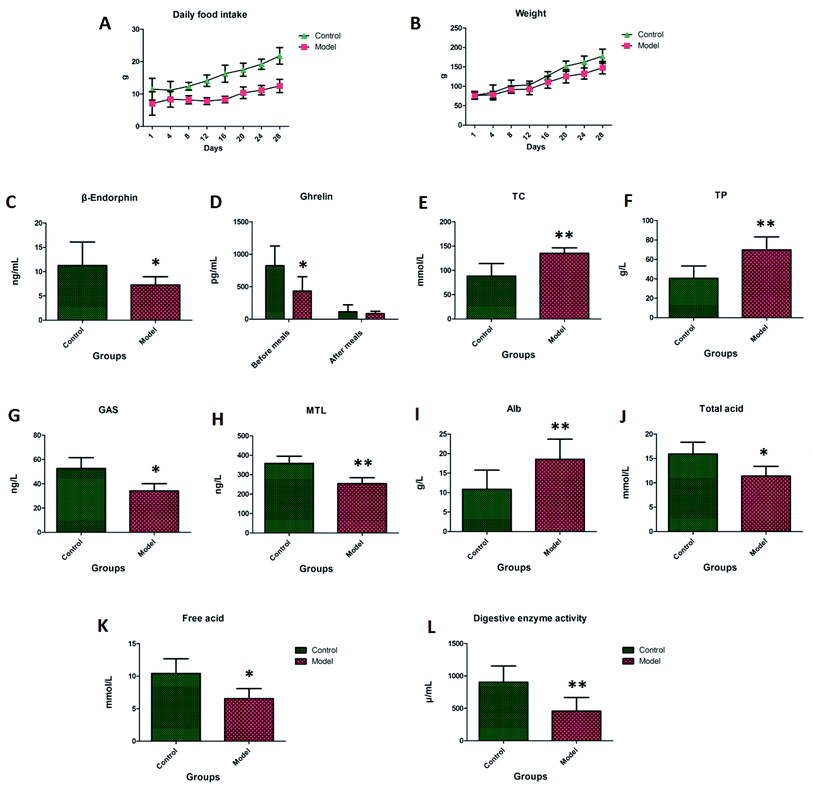 |
| Fig. 1 The average daily food intake, body weight in different days and biochemical index detection. Note: (A) daily food intake; (B) body weight; (C) β-endorphin; (D) ghrelin; (E) TC; (F) TP; (G) GAS; (H) MTL; (I) Alb; (J) total acid; (K) free acid; (L) digestive enzyme activity. | |
In Fig. 1C–J, the β-endorphin, MTL and GAS found in the blood samples of the model group were lower than those in the control group, and the TC, TP and Alb in the blood samples were higher than those in the control group. The ghrelin level in the control group compared with the model group was significantly higher than that after the meal, and the ghrelin level was significantly lower after the meal. The level of ghrelin in the control group was significantly higher than that in the model group. There was no significant difference between the two groups after a meal, which indicated that the ghrelin level may be the most important factor affecting appetite. The total acid and free acid in the gastric juice of the model group were significantly lower than those in the control group. The pepsin activity of the model group was lower than that of the control group, indicating that the model rats had digestive dysfunction.
3.2 Metabolite discovery and identification
According to the conditions described above, serum samples from 20 control rats and 20 model rats were subjected to UPLC-Q-TOF-MS metabonomic profiling. After data processing, the obtained data were imported into EZinfo 2.0 software for multiple statistical analyses. In Fig. 2A and 3A, distinct metabolic profiles could be observed from the OPLS-DA score plots between the control group and the model group, and the separation indicated that the model rats appear to present metabolic changes. In the corresponding loading-plots (Fig. 2B and 3B), the ions of the metabolites furthest away from the origin are likely to be considered as the differentiating metabolites. The S-plot (Fig. 2C and 3C) and the VIP score plot (Fig. 2D and 3D) obtained after the OPLS-DA analysis were used for performing metabolite visualization and discovery, which demonstrated that the distance of the ions from the origin possesses a positive correlation with the alteration of the metabolic trajectory and the variables contributing to cluster and discrimination.
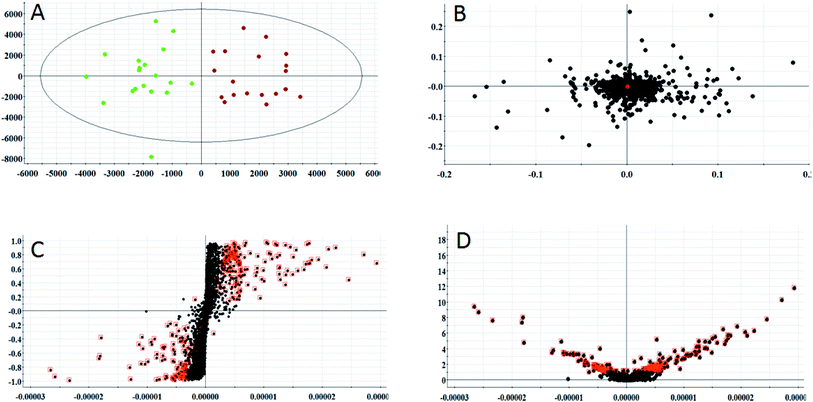 |
| Fig. 2 Multivariate analysis from metabolites in anorexia nervosa rats. (A) OPLS-DA score plot of serum metabolites for clustering of the control and model group in the positive ion mode. (B) Loading plot for the OPLS-DA model of serum metabolites for clustering of the control and model group in the positive ion mode. (C) S-plot of the OPLS-DA model of serum metabolites for clustering of the control and model group in the positive ion mode. (D) VIP-plot of the OPLS-DA model of serum metabolites for clustering of the control and model group in the positive ion mode. | |
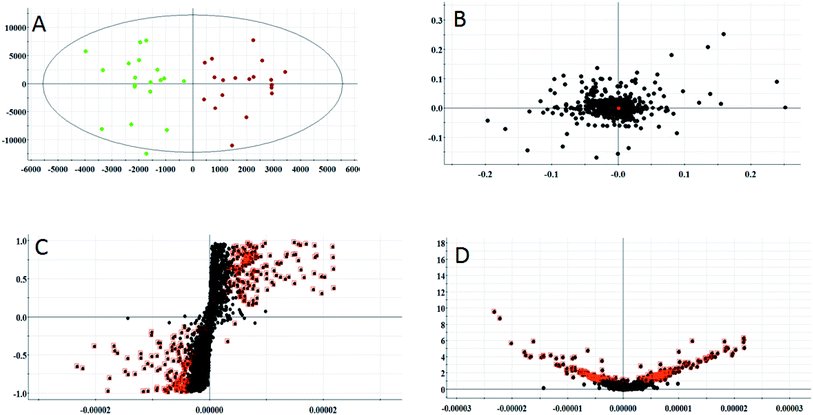 |
| Fig. 3 Multivariate analysis from metabolites in anorexia nervosa rats. (A) OPLS-DA score plot of the serum metabolites for clustering of the control and model group in the negative ion mode (total explained variance A = 99%). (B) Loading plot of the OPLS-DA model of serum metabolites for clustering of the control and model group in the negative ion mode. (C) S-plot of the OPLS-DA model of serum metabolites for clustering of the control and model group in the negative ion mode. (D) VIP-plot of the OPLS-DA model of serum metabolites for clustering of the control and model group in the negative ion mode. | |
On the basis of the VIP > 1 of the OPLS-DA model and the P < 0.05 of the nonparametric Mann–Whitney U test, potential biomarker ions with the exact m/z were selected, and the differential ions between each of the two groups were selected and identified by the RT–m/z pairs and tandem MS fragmentation in online databases. When the mass difference between the observed and theoretical mass was < 10 ppm, the metabolite name was reported. In Table S1,† a total of 26 metabolites were identified, including valyl-alanine, L-glutamine, citric acid, L-arginine, isocitric acid, uric acid, 3-hydroxybutyric acid, L-leucine, γ-carboxyglutamic acid, L-phenylalanine, pyruvate, D-ornithine, glycocholic acid, taurodeoxycholic acid, SM (d18:1/22:0), D-tryptophan, D-glutamic acid, arachidonic acid, sphingosine, LysoPE (0:0/20:0), Cer (d18:0/18:0), LysoPC (17:0), PE (15:0/24:1(15Z)), L-lactic acid, LysoSM (d18:0) and palmitic amide. Compared with the control group, 18 metabolite levels were downregulated and eight metabolites were upregulated, the difference in the brightness of the color presents the concentration changes in the biomarker in the heatmap (Fig. 4) and the relative signal intensities of the serum metabolites are shown in Fig. 5.
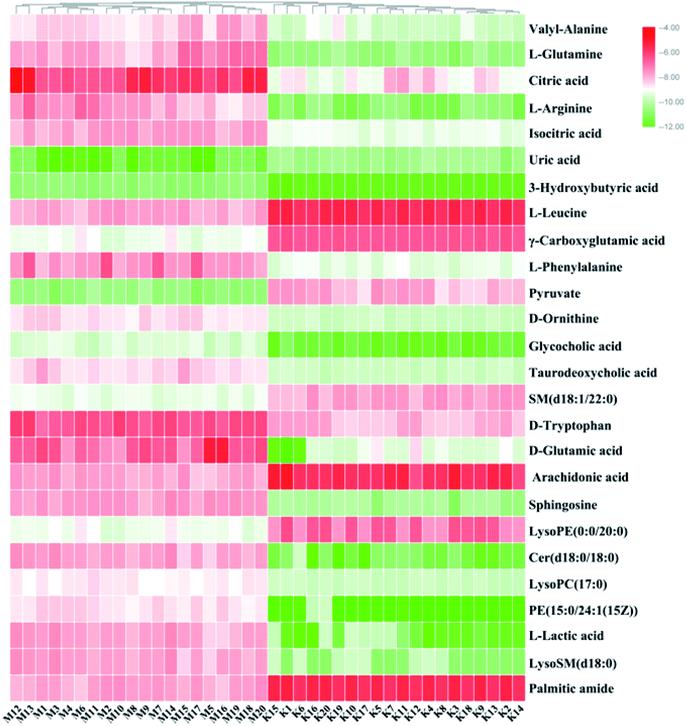 |
| Fig. 4 Heatmap visualization for significant changes in potential biomarker candidates between the control and model groups. Compared with the control group, the levels of 18 metabolites were downregulated and eight metabolites were upregulated, the difference in the brightness of the color presents the concentration changes of the biomarkers in the heatmap. | |
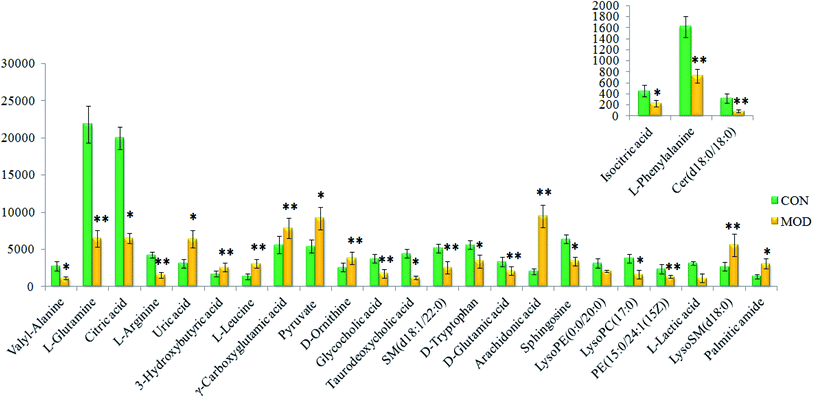 |
| Fig. 5 Relative signal intensities of serum metabolites in different groups identified using UPLC-MS. * represents p < 0.05 and ** represents p < 0.01. | |
3.3 Metabolic pathway and network analysis
The free web-based tool with pathway topology analysis based on the MetaboAnalyst platform, MetPA analysis, showed that the differentiated biomarkers were mainly involved in D-arginine and D-ornithine metabolism, D-glutamine and D-glutamate metabolism, arachidonic acid metabolism, alanine, aspartate and glutamate metabolism, sphingolipid metabolism, phenylalanine metabolism, the citrate cycle (TCA cycle), the synthesis and degradation of ketone bodies, glycosylphosphatidylinositol-anchor biosynthesis, arginine and proline metabolism, and glycerophospholipid metabolism (Fig. 6). The metabolite–metabolic pathway interaction network from KEGG is shown in Fig. 7, which mainly refers to L-glutamine, citric acid, L-arginine, isocitric acid, uric acid, L-leucine, L-phenylalanine, pyruvate, D-ornithine, arachidonic acid, sphingosine, L-lactic acid, PE, Cer and SM. The gene–metabolite interaction network enables the exploration and visualization of interactions between functionally related metabolites and genes, which mainly came down to arachidonic acid (254 genes), L-arginine (206 genes), citric acid (165 genes) and L-glutamine (133 genes), SM (d18:1/18:0) (66 genes), uric acid (60 genes), L-leucine (59 genes) and L-phenylalanine (50 genes), as shown in Fig. 8. The metabolite–metabolite interaction network involved in D-glutamic acid, L-glutamine, L-arginine, L-phenylalanine, L-leucine, arachidonic acid, uric acid, isocitric acid, L-lactic acid and D-tryptophan highlights the potentially functional relationship (Fig. 9) in line with reactions from similar chemical structures and molecular activities. The IPA that was generated using six networks displays cellular compromise, lipid metabolism, small molecule biochemistry, cell signaling, molecular transport, nucleic acid metabolism, cell morphology, cellular function and maintenance disorder in the model rats, which mainly refers to 1-carboxyglutamic acid, 1-heptadecanoyl-2-hydroxy-sn-glycero-3-phosphocholine, citric acid, 3-hydroxybutyric acid, hexadecanamide, C18 dihydroceramide, d18:1/22:1 sphingomyelin and isocitric acid activity, and the merged network is shown in Fig. 10.
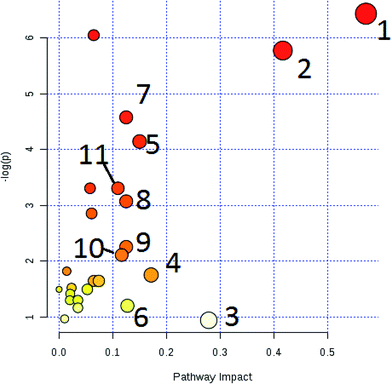 |
| Fig. 6 Altered metabolomic pathways of 26 biomarkers observed in the serum sample using MetPA. (1): D-arginine and D-ornithine metabolism (impact value = 0.571); (2): D-glutamine and D-glutamate metabolism (impact value = 0.416); (3): arachidonic acid metabolism (impact value = 0.287); (4): alanine, aspartate and glutamate metabolism (impact value = 0.171); (5): sphingolipid metabolism (impact value = 0.150); (6): phenylalanine metabolism (impact value = 0.127); (7): TCA cycle (impact value = 0.125); (8): synthesis and degradation of ketone bodies (impact value = 0.125); (9): glycosylphosphatidylinositol (GPI)-anchor biosynthesis (impact value = 0.125); (10): arginine and proline metabolism (impact value = 0.116); (11): glycerophospholipid metabolism (impact value = 0.109). | |
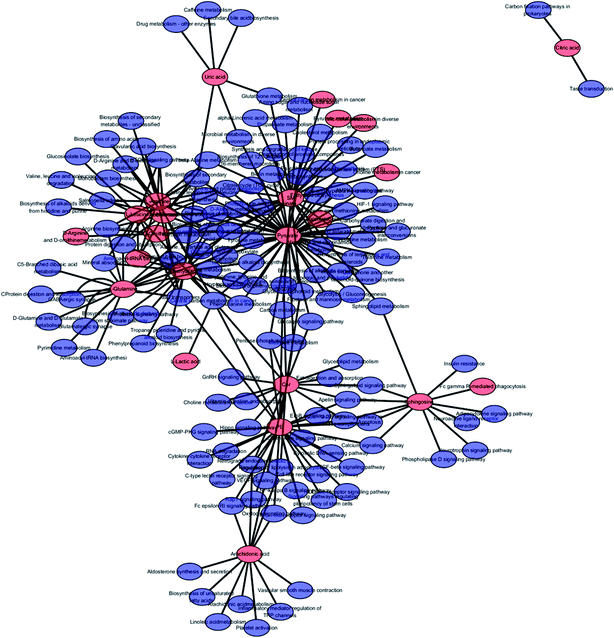 |
| Fig. 7 The metabolite–metabolic pathway interaction network from KEGG in anorexia nervosa rats, red symbols represent the metabolites and blue symbols represent the metabolic pathways. | |
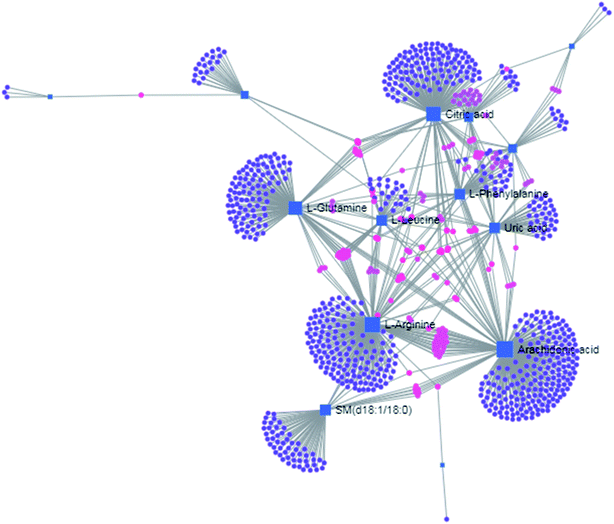 |
| Fig. 8 The gene–metabolite interaction network in anorexia nervosa rats, including arachidonic acid (254 genes), L-arginine (206 genes), citric acid (165 genes), L-glutamine (133 genes), SM (d18:1/18:0) (66 genes), uric acid (60 genes), L-leucine (59 genes) and L-phenylalanine (50 genes). | |
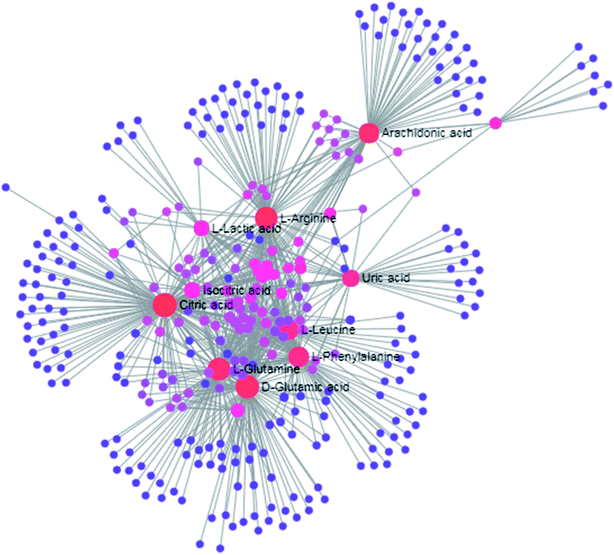 |
| Fig. 9 The metabolite–metabolite interaction network involved in D-glutamic acid, L-glutamine, L-arginine, L-phenylalanine, L-leucine, arachidonic acid, uric acid, isocitric acid, L-lactic acid and D-tryptophan highlights the potential functional relationship. | |
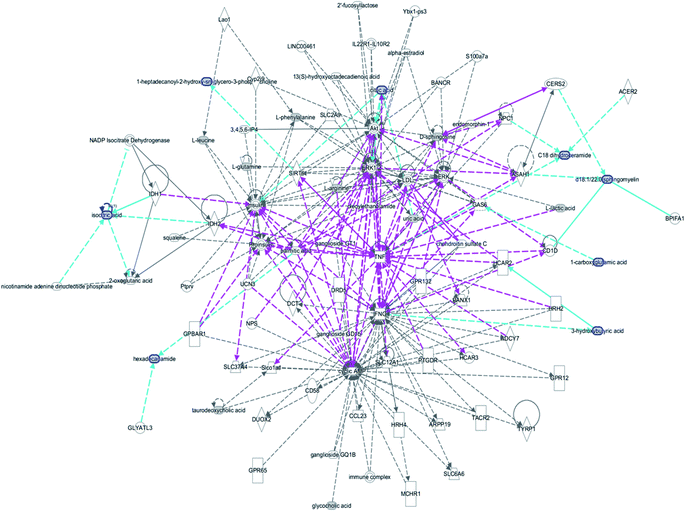 |
| Fig. 10 IPA prediction networks related to the anorexia nervosa pathomechanism in this study, including cellular compromise, lipid metabolism, small molecule biochemistry, cell signaling, molecular transport, nucleic acid metabolism, cell morphology, cellular function and maintenance disorder in the model rats, which mainly refers to 1-carboxyglutamic acid, 1-heptadecanoyl-2-hydroxy-sn-glycero-3-phosphocholine, citric acid, 3-hydroxybutyric acid, hexadecanamide, C18 dihydroceramide, d18:1/22:1 sphingomyelin and isocitric acid activity. | |
3.4 Biological verification
We hypothesized that the upregulation of endogenous arginine by ASS exerts favorable therapeutic effects on anorexia nervosa in children. ASS is a key enzyme in the synthesis of arginine from citrulline, and the knock-down of ASS has been linked to the downregulation of arginine. ASS expression was reduced after anorexia nervosa was caused by the stimulation of being fed a diet of special feed composed of fish pine, milk powder, sugar, corn flour, soy flour, fresh eggs, and fresh meat fat at a proportion of 1
:
1
:
1
:
1 1
:
2
:
1.8
:
2. The knock down of ASS suppressed the expression of β-endorphin and ghrelin, which provides strong evidence of a change in appetite. We further investigated the expression of ASS in anorexia nervosa juvenile rats and found that ASS expression was significantly downregulated in model rats. Meanwhile, ASS overexpression resulted in significant upregulation of arginine in rats. ASS overexpression increased the expression of β-endorphin and ghrelin in model rats. In contrast, ASS deficiency exacerbated progression of the disease. Taken together, these results identified ASS as a potential therapeutic target for the treatment of anorexia nervosa.
4. Discussion
Reduced levels of sphingolipids and phospholipid metabolites in the model group, such as LysoSM (d18:0), LysoPC (17:0), PE (15:0/24:1(15Z)) and sphingosine indicates sphingolipid and phospholipid metabolic disorders in anorexia nervosa rats. Sphingolipids (SPL) are a class of amphoteric lipids containing a sphingosine skeleton with one long chain of fatty acids attached to one end and a polar alcohol at the other end, including sphingomyelin, cerebroside, and gangliosides.28,29 The congenital defect in phospholipase leads to accumulation of sphingomyelin in tissues causing liver and splenomegaly, and this can even affect the central nervous system and become life-threatening.30 Phospholipase is involved in regulating cell growth, differentiation, aging, programmed cell death and many important signal transduction processes. SPL is present in cell membranes such as those in animals, plants and fungi, and is contained in the tissues of the central nervous system at high levels.31 Hydrolysis of intracellular sphingomyelin is catalyzed by neurophospholipidase in lysosomes. The hydrolysate is ceramide, and choline phosphate and can continue to be metabolized. SPL is synthesized in hepatocytes to form lipoproteins, and changes in the lipoprotein components may lead to an increase in atherosclerosis. Studies have shown that SPL not only helps to treat atherosclerosis, but also has a positive therapeutic effect on insulin resistance, diabetes and cancer.32,33 The basic structure of the glycerophospholipids is a phosphatidic acid with a substituent group attached to the hydroxyl group of the phosphate, and this can be classified into six different types of glycerophospholipids according to the different substituents. As a component of bile and membrane surfactants, this participates in the formation of biofilms, and participates in the transmission and recognition of proteins by cell membranes.34,35 Abnormal lipid metabolism refers to the absorption, utilization and metabolic disorders of lipids in children's bodies. An abnormal disorder of the lyso-PC is an effective indicator of acute and chronic inflammation and obesity in the body.36 Citric acid and isocitric acid are involved in the tricarboxylic acid cycle, and the metabolite content of these metabolites in the model group is reduced, indicating the presence of a tricarboxylic acid cycle disorder in the anorexia nervosa rats. Tricarboxylic acid is one of the main ways that organisms obtain energy. A decrease in these metabolites leads to energy acquisition disorders in the model rats and subsequent weight loss. The tricarboxylic acid cycle occurs in the mitochondria, which are a major site of intracellular oxidative phosphorylation and synthesis of adenosine triphosphate (ATP), which provides the necessary energy for cellular activities.37 Mitochondrial dysfunction can lead to neurodegenerative diseases, and mitochondrial respiratory abnormalities and localization can also cause neurodegenerative diseases. Palmitic acid and arachidonic acid play a vital role in fatty acid metabolism, the levels of palmitic acid and arachidonic acid in the model group were increased compared with the control group, indicating that the model rats have fatty acid metabolism disorders. Fatty acid is one of the main energy sources of the body, and it can release a lot of energy when there is sufficient oxygen supply. The disorder of these metabolites can cause the anorexia nervosa body to develop energy barriers. Some studies have revealed that the levels of glycerol and fatty acids (such as palmitic acid, linoleic acid, oleic acid, arachidonic acid) were increased significantly in obese rats and caused by an estrogen deficiency.38 It is speculated that estrogen has an important regulatory role in lipid metabolism. The increase in fatty acid levels may be due to a decrease in the beta oxidation of fatty acids.
Studying the metabolic pathways and biomarkers is crucial to elucidate the pathological mechanism39–44 and could provide insights into the characteristics for pharmacological effects and potential targets,45–56 and provide a better understanding of the mechanistic actions of natural products for new drugs.57–64 Chinmedomics is a powerful platform that can be used to discover biomarkers, metabolic mechanisms and potential targets for drugs.65 Valyl-alanine, L-arginine, L-leucine, L-phenylalanine, D-ornithine, D-tryptophan, D-glutamic acid are involved in the metabolism of amino acids, which present level changes to different degrees in the model group, indicating that the model rats have an amino acid metabolism disorder. Amino acid metabolites are involved in the process of protein metabolism in the body. Glutamic acid itself can be used as a drug in the metabolism of proteins and sugars in the brain, and promotes the oxidation process. Glutamate is an important component of glucose metabolism and amino acid transport and utilization, candidate genes can be selected from brain-derived neurotrophic factors, nutritional neurotrophic factors and tyrosine kinase receptor signals.66 A study was previously published that suggested that glial cell-derived neurotrophic factor (GDNF), ciliary neurotrophic factor (CNTF), and insulin-like growth factor 1 (IGF-1) promote cell survival and protect motor neurons in the body. Mutation of the tyrosine kinase signal sequence (r7w-tyro3) enhances the phenotype of ANX, indicating that normal Tyro3 protection can maintain an appetite-regulating circuit and reduce anorexia nervosa.67,68 Other psychiatric and neurodegenerative diseases are feasible through systematic analysis of eating disorders and platelet function. Glutamic acid can combine with ammonia to form non-toxic glutamine in the body, which can reduce blood ammonia, alleviate liver coma and improve mental development in children.69 The levels of glutamic acid and glutamine in the model group were lower than those in the control group, indicating that glutamate metabolism in the rats with anorexia nervosa results in inflammatory disorders. The arginine present in the body is mainly derived from three pathways: food proteins, endogenous synthesis and protein turnover. For mammals, the synthesis of endogenous arginine is mainly accomplished by the small intestinal–kidney metabolic axis, which is converted from citrulline to refined ammonia by the action of cytosolic arginine succinate synthase and arginine succinate lyase.70,71 Under the action of arginase, arginine is decomposed into ornithine and urea. Ornithine is a precursor of the synthetic polyamines, and polyamines are important for regulating cell growth and development.72 The reduced levels of arginine and ornithine in the model group can be used to explain the slow growth and low immunity of anorexia nervosa patients caused by arginine metabolism disorders. Our current study has several limitations: we need to apply key enzymes to perform gene knock-in and knock-down for biological verification; and in addition, the 26 metabolites were not validated from populations of other races and the positive results from the animal models suggested possible generalization in a different population.
5. Conclusion
In this study, a serum metabolomics strategy based on UPLC-MS detection has been performed for revealing biomarkers and pathway disorder for anorexia nervosa in children. A total of 26 metabolites and relevant pathways were discovered to distinguish the characterized information between the diseased and healthy status. Among them, large amounts of amino acid and sphingolipid metabolism were found to have metabolic abnormalities and they were widely investigated and found to be closely related to anorexia nervosa, especially arginine metabolism. These metabolites and pathways could offer a more promising way to probe the mechanism of anorexia nervosa in children, and provide successful treatments. This study also supports the need for novel diagnostic approaches and drug development.
Conflicts of interest
There are no conflicts to declare.
References
- K. A. Halmi, Anorexia nervosa: an increasing problem in children and adolescents, Dialogues Clin. Neurosci., 2009, 11(1), 100–103 Search PubMed.
- C. Jaite, F. Hoffmann and G. Glaeske, et al., Prevalence, comorbidities and outpatient treatment of anorexia nervosa and bulimia nervosa in German children and adolescents, Eat. Weight Disord., 2013, 18(2), 157–165 CrossRef PubMed.
- H. E. Erskine, H. A. Whiteford and K. M. Pike, The global burden of eating disorders, Curr. Opin. Psychiatr., 2016, 29(6), 346–353 CrossRef PubMed.
- C. Kwok, V. Kwok and H. Y. Lee, et al., Clinical and socio-demographic features in childhood vs. adolescent-onset anorexia nervosa in an Asian population, Eat. Weight Disord., 2019, 1–6 Search PubMed.
- T. L. Hernandez, Children with anorexia nervosa, Am. J. Nurs., 2012, 112(12), 12 CrossRef PubMed.
- L. Lucarelli, M. Ammaniti and A. Porreca, et al., Infantile Anorexia nervosa and Co-parenting: A Pilot Study on Mother-Father-Child Triadic Interactions during Feeding and Play, Front. Psychol., 2017, 8, 376 CrossRef PubMed.
- V. A. Klymenko and IuV. Karpushenko, Food sensitization as a factor of formation of functional diseases of the digestive system in children, Lik. Sprava, 2014, 11, 68–72 Search PubMed.
- R. Mairs and D. Nicholls, Assessment and treatment of eating disorders in children and adolescents, Arch. Dis. Child., 2016, 101(12), 1168–1175 CrossRef PubMed.
- C. Smith, S. Fogarty and S. Touyz, et al., Acupuncture and acupressure and massage health outcomes for patients with anorexia nervosa nervosa: findings from a pilot randomized controlled trial and patient interviews, J. Altern. Complement. Med., 2014, 20(2), 103–112 CrossRef PubMed.
- M. Preis and J. Breitkreutz, Pediatric Drug Development and Dosage Form Design, AAPS PharmSciTech, 2017, 18(2), 239–240 CrossRef PubMed.
- R. Bryant-Waugh, Feeding and Eating Disorders in Children, Psychiatr. Clin. N. Am., 2019, 42(1), 157–167 CrossRef PubMed.
- X. Li, A. Zhang and H. Sun, et al., Metabolic characterization and pathway analysis of berberine protects against prostate cancer, Oncotarget, 2017, 8, 65022–65041 Search PubMed.
- X. N. Li, A. Zhang and M. Wang, et al., Screening the active compounds of Phellodendri Amurensis cortex for treating prostate cancer by high-throughput chinmedomics, Sci. Rep., 2017, 7, 46234 CrossRef CAS PubMed.
- H. Sun, A. H. Zhang and Q. Song, et al., Functional metabolomics discover pentose and glucuronate interconversion pathways as promising targets for Yang Huang syndrome treatment with Yinchenhao Tang, RSC Adv., 2018, 8, 36831–36839 RSC.
- Y. Nan, X. Zhou and Q. Liu, et al., Serum metabolomics strategy for understanding pharmacological effects of ShenQi pill acting on kidney yang deficiency syndrome, J. Chromatogr. B: Biomed. Sci. Appl., 2016, 1026, 217–226 CrossRef CAS PubMed.
- A. Zhang, G. Yan, Y. Han and X. Wang, Metabolomics approaches and applications in prostate cancer research, Appl. Biochem. Biotechnol., 2014, 174, 6–12 CrossRef CAS PubMed.
- Y. Li, S. Qiu and A. H. Zhang, et al., High-throughput metabolomics to identify metabolites to serve as diagnostic biomarkers of prostate cancer, Anal. Methods, 2016, 8, 3284–3290 RSC.
- A. Zhang, H. Sun and G. Yan, et al., Metabolomics in diagnosis and biomarker discovery of colorectal cancer, Cancer Lett., 2014, 345, 17–20 CrossRef CAS PubMed.
- X. J. Wang, J. L. Ren and A. H. Zhang, et al., Novel applications of mass spectrometry-based metabolomics in herbal medicines and its active ingredients: Current evidence, Mass Spectrom. Rev., 2019, 38(4–5), 380–402 CrossRef CAS PubMed.
- A. Zhang, H. Sun and Y. Yuan, et al., An in vivo analysis of the therapeutic and synergistic properties of Chinese medicinal formula Yin-Chen-Hao-Tang based on its active constituents, Fitoterapia, 2011, 82, 1160–1168 CrossRef CAS PubMed.
- C. Lu, X. Niu and C. Xiao, et al., Network-based gene expression biomarkers for cold and heat patterns of rheumatoid arthritis in traditional chinese medicine, J. Evidence-Based Complementary Altern. Med., 2012, 2012, 203043 Search PubMed.
- H. Wang, G. Yan and A. Zhang, et al., Rapid discovery and global characterization of chemical constituents and rats metabolites of Phellodendri amurensis cortex by ultra-performance liquid chromatography-electrospray ionization/quadrupole-time-of- flight mass spectrometry coupled with pattern recognition approach, Analyst, 2013, 138, 3303–3312 RSC.
- A. Zhang, H. Sun and X. Wu, et al., Urine metabolomics, Clin. Chim. Acta, 2012, 414, 65–69 CrossRef CAS PubMed.
- H. Guo, X. Niu and Y. Gu, et al., Differential Amino Acid, Carbohydrate and Lipid Metabolism Perpetuations Involved in a Subtype of Rheumatoid Arthritis with Chinese Medicine Cold Pattern, Int. J. Mol. Sci., 2016, 17(10), 1757 CrossRef PubMed.
- J. Xie, A. H. Zhang and S. Qiu, et al., Identification of the perturbed metabolic pathways associating with prostate cancer cells and anticancer affects of obacunone, J. Proteomics, 2019, 206, 103447 CrossRef CAS PubMed.
- A. Zhang, H. Sun and H. Xu, et al., Cell metabolomics, OMICS: J. Integr. Biol., 2013, 17(10), 495–501 CrossRef CAS PubMed.
- A. H. Zhang, Z. M. Ma, H. Sun, Y. Zhang, J. H. Liu, F. F. Wu and X. J. Wang, High-Throughput Metabolomics Evaluate the Efficacy of Total Lignans From Acanthophanax Senticosus Stem Against Ovariectomized Osteoporosis Rat, Front. Pharmacol., 2019, 10, 553 CrossRef PubMed.
- J. Iqbal, M. T. Walsh and S. M. Hammad, et al., Sphingolipids and Lipoproteins in Health and Metabolic Disorders, Trends Endocrinol. Metab., 2017, 28(7), 506–518 CrossRef CAS PubMed.
- A. Zhang, H. Sun and Y. Han, et al., Urinary metabolic biomarker and pathway study of hepatitis B virus infected patients based on UPLC-MS system, PLoS One, 2013, 8(5), e64381 CrossRef CAS PubMed.
- G. H. Norris and C. N. Blesso, Dietary sphingolipids: potential for management of dyslipidemia and nonalcoholic fatty liver disease, Nutr. Rev., 2017, 75(4), 274–285 CrossRef PubMed.
- M. Murakami, Y. Nakatani and G. I. Atsumi, et al., Regulatory Functions of Phospholipase A2, Crit. Rev. Immunol., 2017, 37(2–6), 121–179 CrossRef PubMed.
- L. Zhang, M. Li and L. Zhan, et al., Plasma metabolomic profiling of patients with diabetes-associated cognitive decline, PLoS One, 2015, 10(5), e0126952 CrossRef PubMed.
- Q. Liang, H. Liu and H. Xing, et al., High-resolution mass spectrometry for exploring metabolic signatures of sepsis-induced acute kidney injury, RSC Adv., 2016, 6, 29863–29868 RSC.
- S. Rodriguez-Cuenca, V. Pellegrinelli and M. Campbell, et al., Sphingolipids and glycerophospholipids - The "ying and yang" of lipotoxicity in metabolic diseases, Prog. Lipid Res., 2017, 66, 14–29 CrossRef CAS PubMed.
- D. Hishikawa, T. Hashidate and T. Shimizu, et al., Diversity and function of membrane glycerophospholipids generated by the remodeling pathway in mammalian cells, J. Lipid Res., 2014, 55(5), 799–807 CrossRef CAS PubMed.
- J. M. Del Bas, A. Caimari and M. I. Rodriguez-Naranjo, et al., Impairment of lysophospholipid metabolism in obesity:altered plasma profile and desensitization to the modulatory properties of n-3P-UF As in a randomized controlled trial, Am. J. Clin. Nutr., 2016, 104(2), 266–279 CrossRef CAS PubMed.
- R. E. Patterson, S. Kalavalapalli and C. M. Williams, et al., Lipotoxicity in steatohepatitis occurs despite an increase in tricarboxylic acid cycle activity, Am. J. Physiol.: Endocrinol. Metab., 2016, 310(7), E484–E494 CrossRef PubMed.
- Bo ma, Qi Zhang and G.-ji wang, et al., GC-TOF/MS-based metabolomic profiling of estrogen deficiency-induced obesity in ovariectomized rats, Acta Pharmacol. Sin., 2011, 32(2), 270–278 CrossRef CAS PubMed.
- A. Zhang, G. Yan and Y. Han, et al., Metabolomics approaches and applications in prostate cancer research, Appl. Biochem. Biotechnol., 2014, 174, 6–12 CrossRef CAS PubMed.
- H. Zhang, A. Zhang and J. Miao, et al., Targeting regulation of tryptophan metabolism for colorectal cancer therapy: a systematic review, RSC Adv., 2019, 9, 3072–3080 RSC.
- H. Sun, A. H. Zhang and S. B. Liu, et al., Cell metabolomics identify regulatory pathways and targets of magnoline against prostate cancer, J. Chromatogr. B: Anal. Technol. Biomed. Life Sci., 2018, 1102–1103, 143–151 CrossRef CAS PubMed.
- A. Zhang, S. Qiu, H. Sun, T. Zhang, Y. Guan, Y. Han, G. Yan and X. Wang, Scoparone affects lipid metabolism in primary hepatocytes using lipidomics, Sci. Rep., 2016, 6, 28031 CrossRef CAS PubMed.
- Y. Zhang, P. Liu and Y. Li, et al., Exploration of metabolite signatures using high-throughput mass spectrometry coupled with multivariate data analysis, RSC Adv., 2017, 7, 6780–6787 RSC.
- X. Liu, A. Zhang and H. Fang, et al., Serum metabolomics strategy for understanding the therapeutic effects of Yin-Chen-Hao-Tang against Yanghuang syndrome, RSC Adv., 2018, 8, 7403–7413 RSC.
- H. Xiong, A. H. Zhang and Q. Q. Zhao, et al., Discovery and screening quality-marker ingredients of Panax quinquefolius using chinmedomics approach, Phytomedicine, 2019, 152928 CrossRef PubMed.
- H. Fang, A. H. Zhang and H. Sun, et al., High-throughput metabolomics screen coupled with multivariate statistical analysis identifies therapeutic targets in alcoholic liver disease rats using liquid chromatography-mass spectrometry, J. Chromatogr. B: Anal. Technol. Biomed. Life Sci., 2019, 1109, 112–120 CrossRef CAS PubMed.
- A. H. Zhang, H. Sun and G. L. Yan, et al., Chinmedomics: A Powerful Approach Integrating Metabolomics with Serum Pharmacochemistry to Evaluate the Efficacy of Traditional Chinese Medicine, Engineering, 2019, 5, 60–68 CrossRef CAS.
- H. Sun, H. Wang and A. Zhang, et al., Berberine ameliorates nonbacterial prostatitis via multi-target metabolic network regulation, OMICS: J. Integr. Biol., 2015, 19(3), 186–195 CrossRef CAS PubMed.
- H. Sun, H. L. Zhang and A. H. Zhang, et al., Network pharmacology combined with functional metabolomics discover bile acid metabolism as a promising target for mirabilite against colorectal cancer, RSC Adv., 2018, 8, 30061–30070 RSC.
- H. Sun, H. L. Zhang and A. H. Zhang, et al., Network pharmacology combined with functional metabolomics discover bile acid metabolism as a promising target for mirabilite against colorectal cancer, RSC Adv., 2018, 8, 30061–30070 RSC.
- X. Wang, J. Li and A. H. Zhang, Urine metabolic phenotypes analysis of extrahepatic cholangiocarcinoma disease using ultra-high performance liquid chromatography-mass spectrometry, RSC Adv., 2016, 6(67), 63049–63057 RSC.
- Y. Zhao, H. Lv and S. Qiu, et al., Plasma metabolic profiling and novel metabolite biomarkers for diagnosing prostate cancer, RSC Adv., 2017, 7(48), 30060–30069 RSC.
- Y. F. Li, S. Qiu and L. J. Gao, et al., Metabolomic estimation of the diagnosis of hepatocellular carcinoma based on ultrahigh performance liquid chromatography coupled with time-of-flight mass spectrometry, RSC Adv., 2018, 8(17), 9375–9382 RSC.
- A. H. Zhang, J. B. Yu and H. Sun, et al., Identifying quality-markers from Shengmai San protects against transgenic mouse model of Alzheimer's disease using chinmedomics approach, Phytomedicine, 2018, 84–92 CrossRef CAS PubMed.
- T. Zhang, A. Zhang, S. Qiu, S. Yang and X. Wang, Current Trends and Innovations in Bioanalytical Techniques of Metabolomics, Crit. Rev. Anal. Chem., 2016, 46(4), 342–351 CrossRef CAS PubMed.
- H. Cao, A. Zhang and H. Zhang, et al., The application of metabolomics in traditional Chinese medicine opens up a dialogue between Chinese and Western medicine, Phytother. Res., 2015, 29(2), 159–166 CrossRef PubMed.
- A. Zhang, H. Sun and H. Xu, et al., Cell metabolomics, OMICS: J. Integr. Biol., 2013, 17(10), 495 CrossRef CAS PubMed.
- S. Qiu, A. Zhang, T. Zhang, H. Sun, Y. Guan, G. Yan and X. Wang, Dissect new mechanistic insights for geniposide efficacy on the hepatoprotection using multiomics approach, Oncotarget, 2017, 8(65), 108760–108770 Search PubMed.
- A. Zhang, H. Sun and G. Yan, et al., Metabolomics study of type 2 diabetes using ultra-performance LC-ESI/quadrupole-TOF high-definition MS coupled with pattern recognition methods, J. Physiol. Biochem., 2014, 70(1), 117–128 CrossRef CAS PubMed.
- A. Zhang, H. Sun and X. Wang, Emerging role and recent applications of metabolomics biomarkers in obesity disease research, RSC Adv., 2017, 7(25), 14966–14973 RSC.
- H. Sun, A. Zhang and L. Yang, et al., High-throughput chinmedomics strategy for discovering the quality-markers and potential targets for Yinchenhao decoction, Phytomedicine, 2019, 54, 328–338 CrossRef CAS PubMed.
- Y. Zhang, P. Liu and Y. Li, et al., Exploration of metabolite signatures using high-throughput mass spectrometry coupled with multivariate data analysis, RSC Adv., 2017, 7, 6780–6787 RSC.
- A. Zhang, H. Sun and Y. Han, et al., Urinary metabolic biomarker and pathway study of hepatitis B virus infected patients based on UPLC-MS system, PLoS One, 2013, 8(5), e64381 CrossRef CAS PubMed.
- H. Sun, A. H. Zhang and Q. Song, et al., Functional metabolomics discover pentose and glucuronate interconversion pathways as promising targets for Yang Huang syndrome treatment with Yinchenhao Tang, RSC Adv., 2018, 8, 36831–36839 RSC.
- X. Wang, A. Zhang and H. Sun, Future perspectives of Chinese medical formulae: chinmedomics as an effector, OMICS: J. Integr. Biol., 2012, 16(7–8), 414–421 CrossRef CAS PubMed.
- J. T. Hackett and T. Ueda, Glutamate Release, Neurochem. Res., 2015, 40(12), 2443–2460 CrossRef CAS PubMed.
- Y. R. L. B. Tovar, U. N. Ramirez and R. Lazo-gomez, Trophic factors as modulators of motor neuron physiology and survival:implications for ALS therapy, Front. Cell. Neurosci., 2014, 8, 61 Search PubMed.
- Q. Liang, H. Liu and H. Xing, et al., Urinary UPLC-MS metabolomics dissecting the underlying mechanisms of huaxian capsule protects against sepsis, RSC Adv., 2016, 6, 40436–40441 RSC.
- N. Mishra, L. H. Rodan and D. A. Nita, et al., Anti-glutamic Acid decarboxylase antibody associated limbic encephalitis in a child: expanding the spectrum of pediatric inflammatory brain diseases, J. Child Neurol., 2014, 29(5), 677–683 CrossRef PubMed.
- M. Gesto, J. L. Soengas and A. Rodríguez-Illamola, et al., Arginine vasotocin treatment induces a stress response and exerts a potent anorexigenic effect in rainbow trout, Oncorhynchus mykiss, J. Neuroendocrinol., 2014, 26(2), 89–99 CrossRef CAS PubMed.
- X. Wang, A. Zhang and X. Zhou, et al., An integrated chinmedomics strategy for discovery of effective constituents from traditional herbal medicine, Sci. Rep., 2016, 6, 18997 CrossRef CAS PubMed.
- T. Lerman-Sagie and M. Mimouni, Reversal of anorexia nervosa in a child with partial ornithine transcarbamylase deficiency by cyproheptadine therapy, Clin. Pediatr., 1995, 34(3), 163–165 CrossRef CAS PubMed.
Footnote |
† Electronic supplementary information (ESI) available. See DOI: 10.1039/c9ra05187b |
|
This journal is © The Royal Society of Chemistry 2020 |
Click here to see how this site uses Cookies. View our privacy policy here.