DOI:
10.1039/C9NA00724E
(Minireview)
Nanoscale Adv., 2020,
2, 3083-3094
Agricultural nanodiagnostics for plant diseases: recent advances and challenges
Received
16th November 2019
, Accepted 6th July 2020
First published on 6th July 2020
Abstract
Crop diseases caused by pathogenic microorganisms pose severe threats to the global food supply. Effective diagnostic tools for timely determination of plant diseases become essential to the assurance of agricultural sustainability and global food security. Nucleic acid- and antibody-based molecular assays are gold-standard methodologies for the diagnosis of plant diseases, but the analyzing procedures are complex and laborious. The prominent physical or chemical properties of nanomaterials have enabled their use as innovative and high-performance diagnostic tools for numerous plant pathogens and other important disease biomarkers. Engineered nanomaterials have been incorporated into traditional laboratory molecular assays or sequencing technologies that offer notable enhancement in sensitivity and selectivity. Meanwhile, nanostructure-supported noninvasive detection tools combined with portable imaging devices (e.g., smartphones) have paved the way for fast and on-site diagnosis of plant diseases and long-term monitoring of plant health conditions, especially in resource-poor settings.
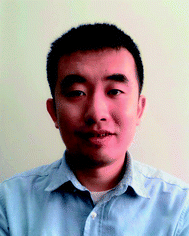 Zheng Li | Zheng Li obtained Ph.D. degree from the University of Illinois at Urbana-Champaign in 2017. From 2017–2019 he worked successively at University of Illinois and North Carolina State University as a postdoctoral scholar. He joined Shenzhen University as an assistant professor in 2020. His research is focused on array-based sensors, wearable detectors, and Lab-on-a-Chip. He has published over 15 first-authored papers in Chemical Review, Angewandte Chemie, Nature Plants, Chemical Science, Analytical Chemistry, and ACS Sensors in the past five years. He compiled 2 book chapters, held 2 U.S. patents, and is currently serving as the guest editor of Frontiers in Chemistry. |
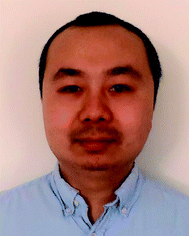 Tao Yu | Tao Yu received his Ph.D. degree from the School of Chemistry and Chemical Engineering, Nanjing University, China, in 2017. He is currently a postdoctoral researcher at North Carolina State University. His research interest includes nanomaterials, microfluidic chips, and point-of-care detection. |
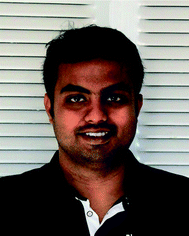 Rajesh Paul | Rajesh Paul received his B. Sc. in Chemical Engineering from Bangladesh University of Engineering and Technology (BUET) in 2013. He then worked as a faculty member at BUET for three years. He is currently a Ph.D. candidate in the Department of Chemical and Biomolecular Engineering at North Carolina State University. His research interests include point-of-care diagnostics, molecular assays, and biosensors for the detection of human and plant diseases. |
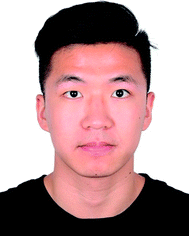 Jingyuan Fan | Jingyuan Fan is a M.S. student in Polymer Science and Engineering in the Organic Semiconductor Laboratory at Zhejiang University, China. His research interests mainly focus on the synthesis of polymers and small molecules with electrical and optical properties, and their nanoscale morphology control in organic solar cells and sensors. He received his B.S. degree from the Department of Polymer Science and Engineering at Zhejiang University in 2020. |
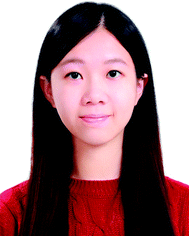 Yuming Yang | Yuming Yang is a master student at Wageningen University, the Netherlands. She is currently studying for master's Biobased Sciences with a focus on biorefinery and conversion. Yuming cares about the nature and environment. During her study in Wageningen, she finds a passion in biobased chemistry and technology. She hopes to pursue a career which enables the transition from a fossil-based to a bio-based economy by developing sustainable conversion processes. In her free time, she likes to ride her bike around town and along the riverbank. |
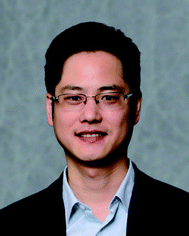 Qingshan Wei | Qingshan Wei is an assistant professor in the Department of Chemical and Biomolecular Engineering, and a member of the Emerging Plant Disease and Global Food Security Cluster at NC State. He received his Ph.D. degree in Chemistry from Purdue University in 2012, and completed postdoctoral training in the Departments of Electrical Engineering and Bioengineering at UCLA between 2012–2016. His research aims to develop novel diagnostic assays and sensors for point-of-care detection of human and plant diseases. He is the recipient of the NSF CAREER Award (2020), Nano Research Young Innovator Award in NanoBiotech (2018), and the Chancellor's Award for Postdoctoral Research (Honorable Mention) at UCLA (2014). |
1. Introduction
Nowadays, over one billion people in the world are suffering from varying degrees of starvation due to the lack of basic staples and insufficient nutritional intake.1 This situation is largely attributed to the continuous increase of global populations, and reduction of agricultural productivity due to social, economic, and environmental reasons. Crop disease caused by pathogen infection and pest attack is one of the main constraints of agricultural production and has become one of the critical global issues.2 For example, the annual losses of main economic crops including potato, maize, peanut, and soybean due to pathogen infections are estimated to be 10–25% of total production.3,4 In order to reduce the spread of plant diseases and facilitate the management of most severe infections, the development of advanced diagnostic tools for early and precise determination of common plant diseases becomes essential.
Nanotechnology is one of the most intriguing and thriving areas of science, which has profound influence on a large number of subjects including science, engineering, medicine, and agriculture.4,5 Nanomaterials are ideal candidates for the analysis of plant pathogens as their dimensions typically fall in the range of 1 to 100 nm that can provide enhanced surface-to-volume ratio and unique chemical, optical, and electrical properties, which are not observed in the bulk counterparts. Nanoscale materials can also interact with biomolecular targets in a more efficient way due to their small sizes and fast diffusion rates. Nanomaterials can be prepared in many different morphologies ranging from spherical particles, cubes, rods, wires, plates, prisms, core–shell structures, to more complicated 3D architectures, which could undergo shape transformation or agglomeration that alters their chemical or physical properties in response to various external stimuli.6 This essentially becomes one of the most common sensing mechanisms of nanomaterials. Breakthroughs in nanotechnology achieved in the recent decade allow for the preparation of nanomaterials for a broad range of biosensing or bioimaging applications, especially those for healthcare monitoring.7–9 A wide range of micro- or nanostructured platforms or sensors have been developed and integrated into the standard molecular diagnostic toolbox that provides extraordinary sensitivity and spatiotemporal resolution.10 The field of agricultural biosensors is also emerging in the past few years.11–14 Due to their cost-effectiveness and field portability, nanosensors have already demonstrated tremendous promise for accurate detection of pathogens that cause severe infections of various economically important crops and plants.15–19
In this minireview, we focus on the emerging use of engineered nanomaterials as potential diagnostic tools in agricultural settings. The design and operation of a variety of nanomaterial-based sensors and detection platforms will be discussed. We will particularly examine studies from the last few years, where the most state-of-the-art nanosensors or nanostructures were reported as easy-to-use devices for plant disease diagnosis. Based on whether nanoparticles (referring to 0D, 1D, or 2D nanostructures with at least one dimension less than 100 nm) are actively used in the sensor devices, we generally divided emerging nanodiagnostic tools for agricultural applications into two main categories: (1) nanostructured devices and tools (e.g., microneedle patch, nanopore, and wearable devices), and (2) nanoparticle-based sensors (e.g., gold nanoparticle, quantum dot, and array-based nanosensors). Finally, insights will be given to the current challenges and future directions in the development of nanodiagnostic tools for plant health monitoring.
2. Methods for diagnosis of plant diseases
Detection and identification of plant diseases could be achieved by both direct and indirect methods.20,21 Direct approaches typically involve analysis of plant pathogens (e.g., bacteria, oomycetes, fungi, and viruses) or biomolecular markers (e.g., nucleic acids, proteins, and carbohydrates) isolated from infected plant tissues. Indirect diagnosis recognizes plant diseases through changes in physiological or histological indices such as leaf surface temperature or humidity, spectroscopic features of plant tissues, morphology, growth rate, and emissions of volatile organic compounds (VOCs). A wide variety of spectroscopic,22,23 electrochemical,24 or molecular technologies25 could serve as direct or indirect detection methods (Fig. 1a). This minireview will summarize several mainstream categories of nanoscale techniques for agricultural diagnosis, in both direct or indirect fashions, including microneedle patches, nanopore sequencing platform, plant wearables, and nanoparticle or array-based sensors. The development of nanodiagnostic tools for AgBio science is in its infancy (Fig. 1b). While many nanosensing tools have been previously prepared and demonstrated for human health monitoring, the applications of nanosensors to agriculture started relatively recently in around 2009. Other new sensing technologies such as nanopore sequencing and array-based nanosensors only moved into the AgBio area in around 2016, and the development of plant wearable and microneedle tools is even latter (Fig. 1b). In recent years, the adoption and application of new technologies to the field of agriculture and plant science has been even more accelerated. For example, the newly developed CRISPR technology has already found a wide range of applications in agriculture and food industry.26
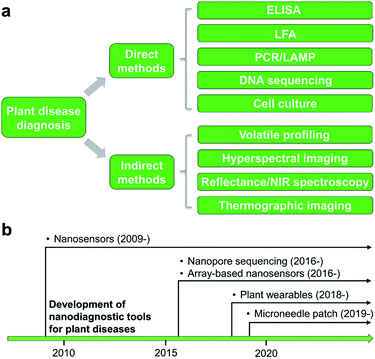 |
| Fig. 1 Methods for plant biotic stress monitoring. (a) Categories of different direct or indirect techniques for plant disease detection. (b) Chronological advances in nanoscale tools for plant disease diagnosis. | |
2.1 Nanostructured devices and tools
2.1.1 Microneedle patches.
Conventional diagnostics in agriculture are based on nucleic acid amplification and protein-based molecular assays, such as enzyme-linked immunosorbent assay (ELISA),27 polymerase chain reaction (PCR),28 loop-mediated isothermal amplification (LAMP),29–31 and recombinase polymerase amplification (RPA).32 These measurements have been extensively carried out for routine laboratory analysis of biomolecules to identify specific pathogens and strains. Despite the high sensitivity and specificity toward particular pathogens, traditional molecular diagnostic tests suffer from several major drawbacks such as complicated sample preparation, long assay time, and expensive instrument, which limit their application and use in the field.33 The integration of nanotechnology with standard biomolecular analyses could possibly provide a solution to improving sensitivity, portability, and versatility of traditional diagnostics that are often performed in centralized laboratories.10
Micro-analytical devices such as skin-penetrating microneedle (MN) patches are attractive for healthcare applications due to their high efficiency in noninvasive delivery of drug molecules, extraction of diagnostic analytes, and in situ monitoring of key clinical parameters. Although the size of the microneedles is typically in the micron scale (e.g., 5–10 μm tip diameter), the needle matrix can be loaded with functional nanoparticles or engineered with nanoporous structures to enhance sensing and sample interaction (e.g., fluid extraction). Microneedle patches have been extensively employed in nanomedicine for transdermal drug delivery34 and subdermal biosensing35 in human patients. However, few studies are available for point-of-care (POC) diagnosis of plant diseases. Recently, we demonstrated a polymer-based microneedle patch in combination with real-time PCR (i.e., quantitative PCR, or qPCR) technique for rapid and sensitive detection of P. infestans, an oomycete that causes the serious potato and tomato disease known as late blight (Fig. 2a and b).36,37 One of the major challenges for on-site plant disease detection through molecular assays is to isolate specific biomarkers from robust and rigid plant tissues. To overcome this obstacle, a prototype microneedle patch made of polyvinyl alcohol (PVA) was developed that is sufficiently strong to break the cell walls of plant leaf tissues to release plant or pathogenic DNA.36,37 The developed DNA extraction method is simple enough to reduce sample preparation time from several hours to less than 1 min. The isolated DNA is ready for amplification without the need for any additional sample purification steps. Despite the lack of specificity in isolation of nucleic acids and other intracellular analytes in the current microneedle design, integration of effective microneedle-patch techniques with miniaturized DNA amplification assays38,39 opens up a path for facile, rapid, and field-deployable amplification and diagnosis of plant pathogens.
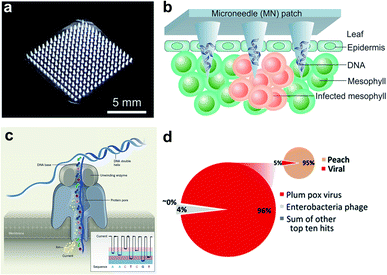 |
| Fig. 2 Microneedle-based plant DNA extraction and nanopore sequencing platform. (a) Photograph of a polymeric microneedle patch. (b) Illustration of plant pathogen DNA (Phytophthora infestans) extraction by applying MN patches on plant leaves. The microneedles can penetrate the leaf tissue and absorbs DNA on the surface of tips. (c) Principle of the handheld nanopore sequencing device (MinION) developed by Oxford Nanopore Technologies. (d) Alignment of reads produced by MinION from Prunus persica (peach) datasets to the total viral genomes using nanopore sequencing of whole transcriptome amplification. The target viruses are plum pox virus (PPV) and Candidatus Liberibacter asiaticus, two major viral pathogens of stone fruits. The inset shows the number of total viral reads versus the number of reads mapping to the P. persica genome. Figure panels reproduced from ref. 36 with permission from American Chemical Society, copyright 2019; ref. 47 with permission from American Phytopathological Society, copyright 2018. | |
2.1.2 Nanopore sequencing platform.
The development of single-molecule sequencing technology has brought about impressive advancements in the detection accuracy and sensitivity of probing DNA structure variations for both clinical and agricultural studies.40,41 As a third-generation sequencing (TGS) technique, the nanopore sequencing platform uses a motor protein to transport a single-stranded DNA or RNA through either a protein or a solid-state nanopore to generate nucleotide-dependent electronic current signals (Fig. 2c),42 which enables facile analysis of pathogenic polynucleotides. Compared to earlier generations of sequencing techniques, nanopore sequencing platform takes advantages of real-time and long reads of sequence data, fast running times, scalable genome mapping, and small sample loadings.43–46
A large number of latest studies using nanopore sequencing platforms have involved in the plant pathogen diagnosis. For example, Chalupowicz et al. have successfully set up a standard protocol using a handheld sequencing system developed by Oxford Nanopore Technologies (named “MinION”) for diagnosis of different plant bacteria, viruses, fungi, and phytoplasma, including P. digitatum in lemon and S. lycopersicum in tomato. The total assay time is within 2 h, and the results are comparable to conventional diagnostic procedures (e.g., PCR and ELISA).46 Badial et al. demonstrated the capability of nanopore sequencing coupled with whole transcriptome amplification for relatively rapid detection (within 24 h) of two viral species, Candidatus Liberibacter asiaticus and plum pox virus, in the peach (Fig. 2d).47 Filloux et al. reported high genome mapping results achieved by MinION by predicting the presence of several plant virus species, including Dioscorea bacilliform virus, Yam mild mosaic virus and Yam chlorotic necrosis virus, in a water yam plant.48 Despite some challenges remaining in the present equipment such as high per-read error rate and poor discriminatory ability among similar sequences,48 continuous breakthroughs in nanopore technology will lead to the creation of more powerful sequencing platforms.
2.1.3 Plant wearables.
With recent advances in the technology of micro-electro-mechanical systems (MEMS), more and more implantable and wearable electronics using conductive nanomaterials have emerged as sensor components for long-term and on-demand monitoring of plant VOCs or other biomarkers. Plant wearable becomes a new frontier of crop diagnostics, which relies on ultrathin and ultra-lightweight nanosensor design to attach flexible sensor devices directly on plant tissues such as leaves for continuous monitoring. For example, Oren et al. developed a roll-to-roll fabrication method of a graphene-based wearable sensor that can monitor water evaporation from plant leaves (Fig. 3a and b).49 The sensing mechanism is based on changes in the electrical resistance of graphene in different humidity environments. Im et al. reported a wearable plant drought stress sensor based on a different mechanism, where the variation of the capacitance of printed 100 nm-think gold electrodes on a flexible polyimide (PI) was recorded in real-time to monitor local humidity.50 Nassar et al. developed a lightweight and multiplexed plant wearable that integrates temperature (resistance), humidity (capacitance), and strain sensors (resistance) to monitor plant's local microclimate and plant growth (Fig. 3c and d).51 The butterfly-shaped multisensory platform was fabricated by transfer printing of 180 nm thick gold electrodes onto flexible polyimide (PI)/polydimethylsiloxane (PDMS) substrate. The same group also demonstrated a flexible CMOS-enabled sensor platform that is equipped with a bare die battery, microcontroller, and a bare die chip for simultaneous light, temperature, and humidity measurement.52 Lei et al. have demonstrated disintegrable and biocompatible pseudo-CMOS flexible circuits that can be attached to the rough surface of plants such as an avocado.53 Kim et al. developed a technique to prepare vapor-printed polymer electrodes, which can be directly printed on living plant tissues for long-term monitoring of drought and photodamage.54
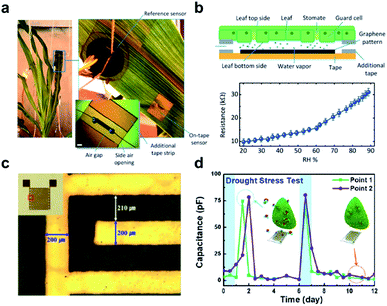 |
| Fig. 3 Wearable sensors for the monitoring of plant health. (a) A photograph of the graphene-based relative humidity (RH) sensor for the detection of water movement from the roots to the lower and upper leaves within the plant. (b) Schematic illustration of the construction and detection mechanism of the RH sensor (upper figure). The lower figure shows the resistance measurement of this sensor as a function of RH. (c) Optical image and real image (inset) of a flexible polyimide-based plant drought sensor. (d) The drought stress responses over time on the Nicotiana tabacum leaf. The blue arrow points to normal conditions, while a red arrow points to drought. Figure panels reproduced from ref. 49 with permission from Wiley, copyright 2017; ref. 51 with permission from MDPI, copyright 2018. | |
2.2 Nanoparticle-based sensors
Imaging techniques, such as thermographic and hyperspectral imaging, have been used in the field for indirect detection of plant disease. However, many of them have notable limitations, such as susceptibility to parameter changes of the environment and lack of specificity for disease subtypes or strains.55,56 Rapid progresses in chemo- or biosensing technology have led to a broad range of successful applications, such as quality assessment of medicinal and industrial products.57 Recently, several nanoparticle-based chemo- or biosensors have been proposed and commercialized for agricultural diagnosis.11,13,58,59 Depending on transduction mechanisms of the designed sensory interactions, the analytes could be recognized by characteristic optical or electrical outputs of the nanosensors. The sensor's detection specificity could be enhanced by selective chemical interactions or by the use of biospecific recognition elements such as DNA oligos, antibodies, aptamers, and enzymes. The detection sensitivity could be improved by the use of surface-enhanced optical properties (e.g., surface plasmon resonance (SPR)) or electron-conductive nanoscale substrates such as carbon-based nanomaterials (e.g., graphene or carbon nanotube) as transducers.60 In this section, we will highlight three main classes of nanosensors or nanobiosensors, including metal or metalloid nanoparticles, quantum dots, and array-based nanosensors. A list of representative nanosensors or biosensors used for plant disease diagnosis, their detection mechanisms, and associated performance is summarized in Table 1.
Table 1 Summary of nanoparticle-based sensors for plant pathogen or disease marker detection
Target |
Sensor component |
Sensor Fabrication |
Detection mechanism |
Sensitivity |
Specificity |
Toxicity |
Ref. |
Nanoparticle.
Surface-enhanced Raman spectroscopy.
Tris(bipyridine)ruthenium(II) chloride.
Immunoglobulin G.
Matrix-assisted laser desorption/ionization-time of flight mass spectroscopy.
3-Mercaptopropionic acid.
Quantum dot.
Polyethylenimine.
Carbon dot.
Förster resonance energy transfer.
Rhodamine 123.
Single-walled carbon nanotube.
Volatile organic compounds.
|
P. syringae
|
Au NPa-ssDNA |
Nanoparticle functionalization and DNA hybridization |
Electrochemistry |
214 pM |
Unresponsive to B. cinerea and F. oxysporum |
Low |
62
|
P. ramorum and P. lateralis |
Ag NP-ssDNA |
Nanoparticle functionalization and DNA hybridization |
SERSb |
N/A |
Unresponsive to P. lateralis |
Low |
64
|
Aflatoxins |
Ag NRc |
Surface functionalization |
SERS |
5 × 10−5 M for Aflatoxin B1 |
Discriminable among Aflatoxin B1, B1, G1 and G2 |
Low |
66
|
B. thuringiensis and B. subtilis |
Pt NP-IgGd |
Surface immunological functionalization |
MALDI-TOF MSe |
∼102 CFU |
Discriminable between two bacteria |
Low |
67
|
X. campestris
|
Si NP-Rubpy-IgG |
Surface immunological functionalization |
Fluorescence quenching |
3 × 102 CFU |
N/A |
Moderate |
68
|
R. solanacearum
|
Au NP-ssDNA |
Surface functionalization |
Colorimetry |
7.5 ng |
No specific band revealed by PCR |
Low |
69
|
F. oxysporum
|
CdSe/ZnS-MPAf QDg |
Surface functionalization |
Fluorescence |
25 μg mL−1 |
N/A |
High |
73
|
Arabidopsis thaliana
|
CdSe-PEIh QD |
Surface functionalization |
Fluorescence |
10 μg mL−1 |
N/A |
High |
75
|
V. mali
|
CDi |
Surface functionalization |
Fluorescence |
pH range of 2.13–9.34 |
No interference from ions |
Low |
79
|
F. avenaceum
|
CD |
Surface functionalization |
Fluorescence |
N/A |
Discriminable between P. aeruginosa and F. avenaceum |
Low |
80
|
3′-Diphosphate-5′-diphosphate |
Tb(III)-CD |
Metal ligation |
Fluorescent ratiometry |
50 nM |
Unresponsive to analogues including ATP, CTP, and UTP |
Moderate |
81
|
Citrus tristeza
|
CdTe QD-CD |
Surface immunological functionalization |
FRETj |
520 ng mL−1 |
N/A |
High |
84
|
Citrus tristeza
|
CdTe QD-Rdk |
Surface immunological functionalization |
FRET |
220 ng mL−1 |
N/A |
High |
85
|
p-Ethylguaiacol |
TiO2 and SnO2 NP |
Surface functionalization |
Electrochemistry |
35–62 nM |
Slightly responsive to p-ethylphenol, unresponsive to 3-octanone and 1-octen-3-ol |
Low |
96
|
Ethylene |
Cu(I) tris(pyrazolyl) borate-SWCNTl |
Ball-milling |
Chemiresistor |
0.5 ppm |
Unresponsive to common solvents except acetonitrile, THF, and acetaldehyde |
Low |
99
|
Ethylene |
Pd(II)-pH dye-SiO2 microspheres |
Ultrasonic spray pyrolysis |
Colorimetry |
0.17 ppm |
Unresponsive to alcohols or esters |
Moderate |
100
|
Terpene vapor |
Au NP@sol–gel |
One-pot hydrolysis |
LSPR |
N/A |
Discriminable among cis-jasmone, α-pinene, limonene, and γ-terpiene |
Low |
101
|
Various VOCsm |
Au NR-cysteine and nanoporous dyes |
Surface functionalization |
LSPR |
0.4–1.7 ppm for hexenal; 1.8–5.2 ppm for phenol |
Discriminable among over ten VOCs |
Low |
102
|
2.2.1 Metal or metalloid nanoparticles.
Inorganic nanomaterials such as Ag, Au, Si, and various metal oxide nanoparticles form a common class of nanosensors that use recognition ligands (e.g., antibodies or DNA oligos) and signal transducers to detect and quantify molecular targets of interest.61,62 Those nanoparticles can serve as unique sensing platforms for probing interactions between the nanoparticles and bioanalytes of specific relevance to plant diseases in vitro or in living plant systems.63 Characteristic sensor responses are often recorded through changes in the surfaced-enhanced optical properties, originating from the localized surface plasmon resonance (LSPR) of nanoparticles. A myriad of self-assembled nanoparticles with oligonucleotides64–66 or proteins67 have been explored to construct sensitive and target-specific nanobiosensors. For example, Ag nanorods have been frequently employed as sensor substrates for the identification of various plant pathogens or produced toxins using surface-enhanced Raman spectroscopy (SERS),68–70 as shown in Fig. 4a. Pt nanosensors functionalized with IgG antibodies were introduced to matrix-assisted laser desorption/ionization mass spectrometry (MALDI MS) that greatly improved the sensitivity to detect plant-associated bacteria in soil and carrot (Fig. 4b).71 Fluorescent silica nanoparticles doped with a Ru(II) complex and conjugated with a secondary antibody were reported to successfully detect plant pathogens such as X. campestris that caused bacterial spot disease in nightshade plant.72 The method was highly sensitive, but the environmental concerns regarding the use of a potentially hazardous heavy metal complex were not addressed. Au nanoparticles functionalized with a specific single-stranded DNA were used to detect as low as 15 ng of genomic DNA of R. solanacearum, a soil-borne species that could cause potato bacterial wilt.73 The assay showed sufficiently high specificity toward the target DNA, yet more quantitative calibration results at the lower detectable range were unavailable.
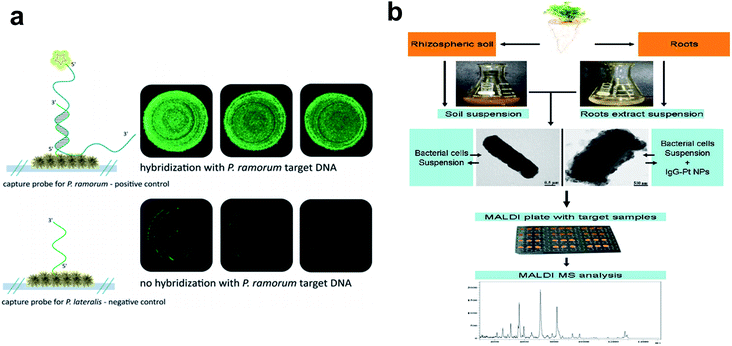 |
| Fig. 4 Metallic nanoparticles as nanobiosensors in plant pathogen detection. (a) Fluorescence microscopy to verify the functionality of the hybridization assay on an Ag nanoparticle-based SERS substrate. Top: hybridization of P. ramorum target DNA with matching P. ramorum capture probes; bottom: absence of signals for P. ramorum target DNA with non-matching P. lateralis capture probes. (b) Workflow chart showing procedures of Pt nanoparticle-assisted MALDI MS analysis of plant-associated bacteria from soil and root samples. Figure panels reproduced from ref. 68 with permission from Royal Society of Chemistry, copyright 2015; ref. 71 with permission from Elsevier, copyright 2012. | |
Nanosensors or nanobiosensors also play vital roles in detecting and controlling the use of pesticides, fertilizers, as well as many other growth parameters associated with crops, which provide timely information for precise decision making and agricultural management.74 Nevertheless, more field validation tests are needed for sensor applications in precision farming.
2.2.2 Quantum dots.
Quantum dots (QDs) are semiconductor nanocrystals that have shown tremendous potentials as optical nanosensors.75 Owing to their unique and advantageous photophysical properties, QDs have found successful applications as biosensors for plant imaging and disease identification.76 The ultra-small size of the QDs (1–10 nm) makes them ideal fluorescent imaging contrast agents for rapid in-planta uptake and transport, where the fluorescent signals can be readily captured to track their distributions in the living system (Fig. 5a). Current studies regarding plant pathogen detection were typically performed in vitro. Prats et al. reported a CdSe–ZnS core–shell QD with 3-mercaptopropionic acid coating, which could be quickly taken up by fungal hyphae.77 Despite an increasing number of endeavors on QD uptake by different model plant species, the interactive/delivery mechanisms and underlying side effects of QDs yet remains to be investigated.78,79
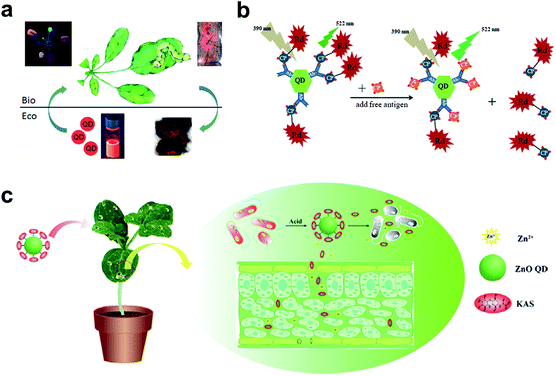 |
| Fig. 5 QDs used in the plant system. (a) Fluorescence imaging of the uptake and transport of QDs in Arabidopsis thaliana. (b) Schematic illustration of specific CTV biosensor based on FRET. (c) ZnO QDs were used to conjugate KAS for the controlled release of pesticides for the plant system. Figure panels reproduced from ref. 79 with permission from American Chemical Society, copyright 2015; ref. 89 with permission from International Frequency Sensor Association Publishing, copyright 2017; ref. 90 with permission from Elsevier, copyright 2019. | |
As a competitive alternative to conventional semiconductor QDs, the newly emerging carbon dots (CDs) have attracted wide attention largely due to the ease of preparation from fruits80 and vegetables.81 The low cytotoxicity and high biocompatibility make them valuable in bacterial and fungal imaging.82–84 Huang et al. developed a paper-based, fluorescent Tb(III)-CD probe to detect 3′-diphosphate-5′-diphosphate, the stringent of plants in response to extreme environmental conditions. The limit of detection (LOD) was down to 50 nM based on the synergistic effect between Tb(III) ions and CDs.85 In addition to inherent fluorescence enhancement or quenching, fluorescence resonance energy transfer (FRET)86 is another well-established mechanism for the construction of QD sensors. For FRET sensors, the proximity of the donors (i.e., QDs) to acceptors (e.g., gold NPs,87 carbon nanodots,88 and organic dyes89) leads to an energy transfer, which results in quenched fluorescence intensity. Safarnejad et al. reported a FRET-based complex sensor for probing Citrus tristeza virus (CTV).89 To achieve this goal, CdTe QDs conjugated with CTV coat protein (CP) and CP-labelled rhodamine dye were employed as donor–acceptor complexes. The presence of target viruses resulted in the replacement of CP-rhodamine with free CP, rendering a recovered fluorescence of QDs (Fig. 5b).
Furthermore, surface-functionalized QDs can be employed as on-demand agrochemicals for plant disease management. For example, kasugamycin (KAS), a kind of natural antibiotic, was artificially conjugated to the surface of ZnO QDs.90 The prepared KAS-ZnO QDs showed outstanding pH-responsive property and improved photostability. In the greenhouse experiment, KAS and Zn(II) species could be released in a controlled manner, which significantly alleviated the severity of bacterial fruit blotch (Fig. 5c).
Due to the extensive use of transition metal elements as optical nanoprobes, issues associated with their toxicity become increasingly inevitable. For field applications where diagnostic efficiency is of high priority, health risks of quantum dots could be overlooked since many of them are made of highly toxic heavy metals (Pb, Zn, and Hg).91,92 Using environmentally benign elements such as carbon or silica could potentially relieve this negative effect. Another concern for optical imaging of plant biomarkers using nanoparticles lies in the possible interferences from autofluorescence of host plant tissues due to ubiquity of biological pigments (e.g., chlorophyll or carotenoid) in plants that may have similar emission bands.93 Therefore, rational selection of excitation/emission wavelengths of engineered nanosensors is critical to the acquisition of explicit spectral information about targets in a plant body.
2.2.3 Array-based nanosensors.
Among the state-of-the-art nanosensors, one format capable of multiplexing and discrimination of different analytes is the array-based sensor assemblies composed of several chromophores or synthetic nanomaterials as an analog to the mammalian olfactory system. A common form of the array-based sensor is also known as the “electronic nose” (e-nose), which incorporates electronic transducers instead of chemical sensors in the array.94–96 The array-based approach is particularly beneficial in distinguishing highly similar analyte mixtures due to its cross-reactivity and ability to measure molecular fingerprints. The multidimensional output data of sensor arrays can be analyzed by supervised or unsupervised chemometrics, which provides highly precise and predictive results.97 Alternatively, array-based chemical sensors98 can provide optical readout (e.g., colorimetric or fluorescent), which is easier to analyze and interpret compared with e-nose signals. In addition, chemical sensor arrays are easy to fabricate, based on specific chemical interaction, which brings in much better chemical specificity, extremely cost-effective, and more resistant to environmental interference such as humidity and temperature than most e-nose devices. Chemical sensor arrays can be easily prepared by embedding various analyte-responsive dyes such as Lewis acid-base colorants, Brønsted acidic or basic colorants, solvatochromic or vapochromic dyes, and redox indicator colorants in hydrophobic nanoporous substrates such as modified silica sol–gels.96
An important indirect method for plant disease detection involves the profiling of the volatile chemical signature of diseased plants.99 This is based on the observations that diseased plants could result in the release or change of the composition of characteristic volatile organic compounds (VOCs) that are indicative of the type of biotic or abiotic stresses the host plants have experienced.100 E-noses made of metal oxides101 or conductive polymer coatings have drawn considerable attention as multiplexed gas sensors for tracing VOC biomarkers in plant stress events ranging from pathogen infection,101 pest invasion,102 to physical wounding.103 Particular emphasis is placed on the detection of ethylene, one of the most important phytohormones, using chemiresistive104 or colorimetric method.105 Of special interest is the design of a nanoplasmonic sensor array comprising AuNPs and a molecularly imprinted sol–gel (AuNPs@MISG) for selective detection of terpenes (Fig. 6a).106 Recently, we developed a smartphone-integrated VOC sensing platform that utilized plasmonic nanoparticles for early detection and differentiation of tomato late blight (within 2 days of infection) from the other fungal pathogens that lead to similar symptoms of tomato foliage (Fig. 6b).107 The gold nanoparticles were functionalized with chemical ligands that were reactive to specific leafy VOCs, and printed on paper strips to generate unique patterns of color changes for each VOC. The results were then scanned by a smartphone microscope for digital pattern recognition and differentiation. The described sensor platform will be not only useful for fingerprinting plant pathogens of interest, but also well-suited for distinguishing various abiotic stresses, such as mechanical damages, drought, and nutrition deficiency. Future research may be focused on improving sampling protocols that do not require pre-collection and concentration of VOCs prior to data analysis.
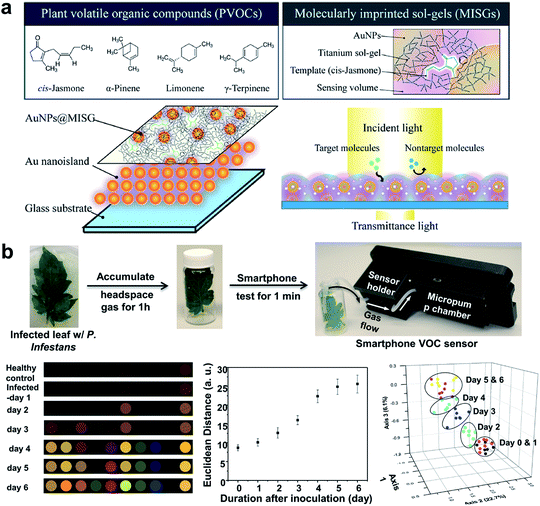 |
| Fig. 6 Different array-based nanosensor platforms for plant monitoring. (a) Schematic of AuNPs@MISG-coated Au nanoislands for selective detection of terpenes. (b) VOC sampling and detection of tomato late blight enabled by a 10-element nanostructured colorimetric sensor array using a smartphone-based detector. Early infection can be detected by the smartphone platform 2 days after inoculation. Figure panels reproduced from ref. 106 with permission from American Chemical Society, copyright 2018; ref. 107 with permission from Springer, copyright 2019. | |
Portable vapor analyzers embedded with gas chromatography (GC) tandem mass spectroscopy (MS) or ion mobility spectroscopy (IMS) represent another class of mobile tools for on-site analysis of plant health status that can determine the identity and abundance of each emitted VOC species.108–110 Due to facile device fabrication and fast target sampling, hand-held MEMS devices integrated with miniaturized GC-MS/IMS sensors are among the most effective, non-optical methods for plant health monitoring.
3. Conclusions, challenges, and perspectives
Nanoscale materials are promising candidates for plant disease detection due to the remarkable biospecificity of engineered molecular recognitions at the nanoscale, which has witnessed unprecedented development in the recent decade in combination with modern analytical techniques, including fluorescence microscopy, spectroscopic measurement, wearable sensors, and smartphone-based microscopy. In this minireview, we have highlighted the latest and most notable nanodiagnostic systems for plant disease detection in the laboratory or field settings. Owing to the rapid advances in nanotechnology and modern nanofabrication techniques in the recent decade, great progress in a variety of useful sensors, biosensors, and nanostructured platforms has been continuously emerging for plant disease analysis. One of the immediate impacts of these newly developed nanodiagnostic tools is that precision plant disease detection now becomes more accessible to the field workers or farmers. For example, many conventional laboratory tests such as nucleic acid amplification, sequencing, and VOC analysis now can be potentially performed directly in the crop field in a much faster and cost-effective fashion, due to the recent innovation of rapid plant DNA extraction technology enabled by microneedles, miniature DNA sequencing chips, and smartphone-based VOC sensors. Taking advantages of design flexibility, chemical diversity, multiplexity, and cost-effectiveness of nanomaterials, it is expected that more and more powerful nanoscale sensors and probes integrated with multimodal detection mechanisms will be developed that allow for quick detection and determination of infections caused by plant pathogens, as well as many other biotic or abiotic stresses.
However, currently available plant diagnostic tools still face three major challenges: (1) environmental impact and toxicity of engineered nanomaterials; (2) promptness of data sharing and disease forecasting; and (3) long-term sensor stability in extreme scenarios such as cold or hot weather, intensive sun exposure, and heavy wear. For the first challenge, before any nanosensors can be commercialized and deployed to the field, their safety concerns must be addressed as some of the nanoparticles such as QDs could be toxic. In particle, for nanosensors that will be left on live plants or consumable agricultural and food products, more careful toxicity testing and regulation are needed, as harmful nanomaterial residuals could potentially enter the food chain and be uptaken by the end users.
For the second concern, since the foremost prerequisite of disease diagnosis is always the timely report and forecast of infection events on site, the new generation of nanosensors are expected to be more wirelessly connected that can provide near real-time measurement. For example, continuous monitoring of VOC emission of plants is expected to provide more time dynamic information than conventional single-point measurements and therefore enable more accurate monitoring of plant stresses. In this regard, the recent development of field-portable sensor tools such as smartphone devices or plant wearables brings promisingly new opportunities to the in situ analysis of pathogens in the field by sharing and transmitting data almost in real-time. In order to support continuous measurement, sensor miniaturization, wireless data transmission, and integration with computational data processing pipelines such as machine learning and artificial intelligence (AI) will be among the critical areas to be addressed further.
Lastly, more durable and robust sensors that can withstand varied environmental conditions (e.g., temperature, humidity, air pollutions, etc.) in the crop field are anticipated before any sensors can be deployed to the real field. This requires more fundamental research on the novel sensor materials, such as environmental-resistant substrates and nanoparticles. For example, volatile sensor arrays made of more durable materials, such as resilient paper or polymer substrates and photo-resistive dyes, will be needed for the field crops or sentinel plants for long-term monitoring, where the sensor signals could be regularly scanned by the mobile phone readers or wirelessly read out by electrical wearable devices. The latter holds great promise for monitoring of early infections in a large scale by remote and continuous measurement of relevant biomarkers from symptomless field plants.
Despite the remaining challenges, the recent development of miniature and cost-effective nanodiagnostic tools has shown tremendous potentials in improving plant disease diagnosis, management, and crop health monitoring in the long run. The future of AgBio sensors is indeed very bright in the coming era of digital farm and precision agriculture.
Conflicts of interest
The authors declare no conflicts of interest.
Acknowledgements
The authors sincerely thank the supports from the USDA AFRI Grant (no. 2019-67030-29311), the Chancellor’s Faculty Excellent Program, the Kenan Institute for Engineering, Technology & Science (KIETS), and the Game-Changing Research Incentive Program for Plant Sciences Initiative (GRIP4PSI) of NC State University. Z. L. also acknowledges the financial supports from Guangdong Joint Fund of Fundamental and Applied Research (No. 2019A1515110242) and Guangdong University Young Talents Project (No. 2019KQNCX127).
References
- K. Debnath, J. Agric. Econ., 2013, 64, 738–740 CrossRef.
- R. N. Strange and P. R. Scott, Annu. Rev. Phytopathol., 2005, 43, 83–116 CrossRef CAS PubMed.
- J. Kaminski and L. Christiaensen, Global Food Security, 2014, 3, 149–158 CrossRef.
- D. Kumar and P. Kalita, Foods, 2017, 6, 8 CrossRef PubMed.
- R. Prasad, A. Bhattacharyya and Q. D. Nguyen, Front. Microbiol., 2017, 8, 1014 CrossRef PubMed.
- A. A. Keller, H. Wang, D. Zhou, H. S. Lenihan, G. Cherr, B. J. Cardinale, R. Miller and Z. Ji, Environ. Sci. Technol., 2010, 44, 1962–1967 CrossRef CAS PubMed.
- D. Quesada-González and A. Merkoçi, Chem. Soc. Rev., 2018, 47, 4697–4709 RSC.
- R. Das, C. D. Vecitis, A. Schulze, B. Cao, A. F. Ismail, X. Lu, J. Chen and S. Ramakrishna, Chem. Soc. Rev., 2017, 46, 6946–7020 RSC.
- J.-J. Hu, Y.-J. Cheng and X.-Z. Zhang, Nanoscale, 2018, 10, 22657–22672 RSC.
- T. Yu and Q. Wei, Nano Res., 2018, 11, 5439–5473 CrossRef PubMed.
- S.-Y. Kwak, M. H. Wong, T. T. S. Lew, G. Bisker, M. A. Lee, A. Kaplan, J. Dong, A. T. Liu, V. B. Koman, R. Sinclair, C. Hamann and M. S. Strano, Annu. Rev. Anal. Chem., 2017, 10, 113–140 CrossRef PubMed.
- A. S. Nezhad, Lab Chip, 2014, 14, 2887–2904 RSC.
- M. Khater, A. de la Escosura-Muñiz and A. Merkoçi, Biosens. Bioelectron., 2017, 93, 72–86 CrossRef CAS PubMed.
- S. Neethirajan, K. V. Ragavan and X. Weng, Trends Food Sci. Technol., 2018, 73, 25–44 CrossRef CAS.
- M. A. Khiyami, H. Almoammar, Y. M. Awad, M. A. Alghuthaymi and K. A. Abd-Elsalam, Biotechnol. Biotechnol. Equip., 2014, 28(5), 775–785 CrossRef PubMed.
- P. L. Kashyap, S. Kumar and A. K. Srivastava, Environ. Chem. Lett., 2017, 15(1), 7–13 CrossRef CAS.
-
P. Sharma and S. Sharma, Paradigm shift in plant disease diagnostics: a journey from conventional diagnostics to nano-diagnostics, in Current trends in plant disease diagnostics and management practices, Springer, Cham, 2016, pp. 237–264 Search PubMed.
-
T. Shoala, Nanodiagnostic Techniques in Plant Pathology, in Nanobiotechnology Applications in Plant Protection, Springer, Cham, 2019, pp. 209–222 Search PubMed.
-
V. Kumar and K. Arora, Trends in nano-inspired biosensors for plants, Materials Science for Energy Technologies, 2020, vol. 3, pp. 255–273 Search PubMed.
- Y. Fang and R. P. Ramasamy, Biosensors, 2015, 5, 537–561 CrossRef CAS PubMed.
- F. Martinelli, R. Scalenghe, S. Davino, S. Panno, G. Scuderi, P. Ruisi, P. Villa, D. Stroppiana, M. Boschetti, L. R. Goulart, C. E. Davis and A. M. Dandekar, Agron. Sustain. Dev., 2015, 35, 1–25 CrossRef.
- E. Bauriegel and W. B. Herppich, Agriculture, 2014, 4, 32–57 CrossRef CAS.
- K. Golhani, S. K. Balasundram, G. Vadamalai and B. Pradhan, Inf. Process. Agric., 2018, 5, 354–371 Search PubMed.
- R. B. Valekunja, V. Kamakoti, A. Peter, S. Phadnis, S. Prasad and V. J. Nagaraj, Anal. Methods, 2016, 8, 8534–8541 RSC.
- M. Verosloff, J. Chappell, K. L. Perry, J. R. Thompson and J. B. Lucks, ACS Synth. Biol., 2019, 8, 902–905 CrossRef CAS PubMed.
- Y. Zhang, A. A. Malzahn, S. Sretenovic and Y. Qi, Nature Plants, 2019, 5, 778–794 CrossRef PubMed.
- S. Sakamoto, W. Putalun, S. Vimolmangkang, W. Phoolcharoen, Y. Shoyama, H. Tanaka and S. Morimoto, J. Nat. Med., 2018, 72, 32–42 CrossRef CAS PubMed.
- M.-J. Bergeron, N. Feau, D. Stewart, P. Tanguay and R. C. Hamelin, PloS One, 2019, 14, e0210952 CrossRef CAS PubMed.
- L. Zhang and C. Gleason, Plant Dis., 2019, 103, 12–18 CrossRef CAS PubMed.
- J.-j. Li, C. Xiong, Y. Liu, J.-s. Liang and X.-w. Zhou, Front. Plant Sci., 2016, 7, 1956 Search PubMed.
- J. B. Ristaino, A. C. Saville, R. Paul, D. C. Cooper and Q. Wei, Plant Dis., 2020, 104, 708–716 CrossRef PubMed.
- H. Y. Lau, Y. Wang, E. J. H. Wee, J. R. Botella and M. Trau, Anal. Chem., 2016, 88, 8074–8081 CrossRef CAS PubMed.
- H. Y. Lau and J. R. Botella, Front. Plant Sci., 2017, 8, 2016 CrossRef PubMed.
- K. van der Maaden, R. Luttge, P. J. Vos, J. Bouwstra, G. Kersten and I. Ploemen, Drug Delivery Transl. Res., 2015, 5, 397–406 CrossRef CAS PubMed.
- P. R. Miller, R. J. Narayan and R. Polsky, J. Mater. Chem. B, 2016, 4, 1379–1383 RSC.
- R. Paul, A. C. Saville, J. C. Hansel, Y. Ye, C. Ball, A. Williams, X. Chang, G. Chen, Z. Gu, J. B. Ristaino and Q. Wei, ACS Nano, 2019, 13, 6540–6549 CrossRef CAS PubMed.
- R. Paul, E. Ostermann, Z. Gu, J. B. Ristaino and Q. Wei, Curr. Protoc. Plant Biol., 2020, 5, e20104 Search PubMed.
- C. Koo, M. Malapi-Wight, H. S. Kim, O. S. Cifci, V. L. Vaughn-Diaz, B. Ma, S. Kim, H. Abdel-Raziq, K. Ong, Y.-K. Jo, D. C. Gross, W.-B. Shim and A. Han, PloS One, 2013, 8, e82704 CrossRef PubMed.
- Z. K. Njiru, PLoS Neglected Trop. Dis., 2012, 6, e1572 CrossRef PubMed.
- D. M. Bickhart, B. D. Rosen, S. Koren, B. L. Sayre, A. R. Hastie, S. Chan, J. Lee, E. T. Lam, I. Liachko, S. T. Sullivan, J. N. Burton, H. J. Huson, J. C. Nystrom, C. M. Kelley, J. L. Hutchison, Y. Zhou, J. Sun, A. Crisà, F. A. Ponce de León, J. C. Schwartz, J. A. Hammond, G. C. Waldbieser, S. G. Schroeder, G. E. Liu, M. J. Dunham, J. Shendure, T. S. Sonstegard, A. M. Phillippy, C. P. Van Tassell and T. P. L. Smith, Nat. Genet., 2017, 49, 643–650 CrossRef CAS PubMed.
- S. Ardui, A. Ameur, J. R. Vermeesch and M. S. Hestand, Nucleic Acids Res., 2018, 46, 2159–2168 CrossRef CAS PubMed.
- M. Eisenstein, Nature, 2017, 550, 285–288 CrossRef PubMed.
- C. M. Watson, L. A. Crinnion, S. Hewitt, J. Bates, R. Robinson, I. M. Carr, E. Sheridan, J. Adlard and D. T. Bonthron, Lab. Invest., 2020, 100, 135–146 CrossRef CAS PubMed.
- B. D. Wilson, M. Eisenstein and H. T. Soh, Anal. Chem., 2019, 91, 6783–6789 CrossRef CAS PubMed.
- T. Charalampous, G. L. Kay, H. Richardson, A. Aydin, R. Baldan, C. Jeanes, D. Rae, S. Grundy, D. J. Turner, J. Wain, R. M. Leggett, D. M. Livermore and J. O'Grady, Nat. Biotechnol., 2019, 37, 783–792 CrossRef CAS PubMed.
- L. Chalupowicz, A. Dombrovsky, V. Gaba, N. Luria, M. Reuven, A. Beerman, O. Lachman, O. Dror, G. Nissan and S. Manulis-Sasson, Plant Pathol., 2019, 68, 229–238 CrossRef CAS.
- A. Bronzato Badial, D. Sherman, A. Stone, A. Gopakumar, V. Wilson, W. Schneider and J. King, Plant Dis., 2018, 102, 1648–1652 CrossRef PubMed.
- D. Filloux, E. Fernandez, E. Loire, L. Claude, S. Galzi, T. Candresse, S. Winter, M. L. Jeeva, T. Makeshkumar, D. P. Martin and P. Roumagnac, Sci. Rep., 2018, 8, 17879 CrossRef CAS PubMed.
- S. Oren, H. Ceylan, P. S. Schnable and L. Dong, Adv. Mater. Technol., 2017, 2, 1700223 CrossRef.
- H. Im, S. Lee, M. Naqi, C. Lee and S. Kim, Electronics, 2018, 7, 114 CrossRef CAS.
- J. M. Nassar, S. M. Khan, D. R. Villalva, M. M. Nour, A. S. Almuslem and M. M. Hussain, npj Flexible Electron., 2018, 2, 24 CrossRef.
- S. M. Khan, S. F. Shaikh, N. Qaiser and M. M. Hussain, IEEE Trans. Electron Devices, 2018, 65, 5038–5044 CAS.
- T. Lei, M. Guan, J. Liu, H.-C. Lin, R. Pfattner, L. Shaw, A. F. McGuire, T.-C. Huang, L. Shao, K.-T. Cheng, J. B.-H. Tok and Z. Bao, Proc. Natl. Acad. Sci. U. S. A., 2017, 114, 5107–5112 CrossRef CAS PubMed.
- J. J. Kim, L. K. Allison and T. L. Andrew, Sci. Adv., 2019, 5, eaaw0463 CrossRef CAS PubMed.
- L. Li, Q. Zhang and D. Huang, Sensors, 2014, 14, 20078–20111 CrossRef PubMed.
-
D. Rousseau, H. Dee, and T. Pridmore, Imaging Methods for Phenotyping of Plant Traits, in Phenomics in Crop Plants: Trends, Options and Limitations, ed. J. Kumar, A. Pratap and S. Kumar, Springer, India, New Delhi, 2015, pp. 61–74 Search PubMed.
- S.-J. Kim, S.-J. Choi, J.-S. Jang, H.-J. Cho and I.-D. Kim, Acc. Chem. Res., 2017, 50, 1587–1596 CrossRef CAS PubMed.
- J. Yin, Y. Wang and L. M. Gilbertson, Environ. Sci.: Nano, 2018, 5, 11–26 RSC.
-
M. Kaushal and S. P. Wani, Nanosensors: Frontiers in Precision Agriculture, in Nanotechnology: An Agricultural Paradigm, ed. R. Prasad, M. Kumar and V. Kumar, Springer, Singapore, 2017, pp. 279–291 Search PubMed.
- A. Mukherjee, S. Majumdar, A. D. Servin, L. Pagano, O. P. Dhankher and J. C. White, Front. Plant Sci., 2016, 7, 172 Search PubMed.
- S.-H. Wu, C.-Y. Mou and H.-P. Lin, Chem. Soc. Rev., 2013, 42, 3862–3875 RSC.
- J. A. Darr, J. Zhang, N. M. Makwana and X. Weng, Chem. Rev., 2017, 117, 11125–11238 CrossRef CAS PubMed.
- S.-Y. Kwak, M. H. Wong, T. T. S. Lew, G. Bisker, M. A. Lee, A. Kaplan, J. Dong, A. T. Liu, V. B. Koman, R. Sinclair, C. Hamann and M. S. Strano, Annu. Rev. Anal. Chem., 2017, 10, 113–140 CrossRef PubMed.
- B. Dubertret, M. Calame and A. J. Libchaber, Nat. Biotechnol., 2001, 19, 365–370 CrossRef CAS PubMed.
- I.-H. Cho and S. Ku, Int. J. Mol. Sci., 2017, 18, 2078 CrossRef PubMed.
- H. Y. Lau, H. Wu, E. J. H. Wee, M. Trau, Y. Wang and J. R. Botella, Sci. Rep., 2017, 7, 38896 CrossRef CAS PubMed.
-
L.-L. Li, Self-assembled Nanomaterials for Bacterial Infection Diagnosis and Therapy, in In Vivo Self-Assembly Nanotechnology for Biomedical Applications, H. Wang and L.-L. Li, Springer, Singapore, 2018, pp. 57–88 Search PubMed.
- S. Yüksel, L. Schwenkbier, S. Pollok, K. Weber, D. Cialla-May and J. Popp, Analyst, 2015, 140, 7254–7262 RSC.
- C. Farber, M. Mahnke, L. Sanchez and D. Kurouski, TrAC, Trends Anal. Chem., 2019, 118, 43–49 CrossRef CAS.
- X. Wu, S. Gao, J.-S. Wang, H. Wang, Y.-W. Huang and Y. Zhao, Analyst, 2012, 137, 4226–4234 RSC.
- F. Ahmad, M. A. Siddiqui, O. O. Babalola and H.-F. Wu, Biosens. Bioelectron., 2012, 35, 235–242 CrossRef CAS PubMed.
- K. S. Yao, S. J. Li, K. C. Tzeng, T. C. Cheng, C. Y. Chang, C. Y. Chiu, C. Y. Liao, J. J. Hsu and Z. P. Lin, Adv. Mater. Res., 2009, 79–82, 513–516 CAS.
- S. Khaledian, M. Nikkhah, M. Shams-bakhsh and S. Hoseinzadeh, J. Gen. Plant Pathol., 2017, 83, 231–239 CrossRef CAS.
- J. S. Duhan, R. Kumar, N. Kumar, P. Kaur, K. Nehra and S. Duhan, Biotechnology Reports, 2017, 15, 11–23 CrossRef PubMed.
- G. Gao, Y.-W. Jiang, W. Sun and F.-G. Wu, Chin. Chem. Lett., 2018, 29, 1475–1485 CrossRef CAS.
- P. Wang, E. Lombi, F.-J. Zhao and P. M. Kopittke, Trends Plant Sci., 2016, 21, 699–712 CrossRef CAS PubMed.
- N. Rispail, L. De Matteis, R. Santos, A. S. Miguel, L. Custardoy, P. S. Testillano, M. C. Risueño, A. Pérez-de-Luque, C. Maycock, P. Fevereiro, A. Oliva, R. Fernández-Pacheco, M. R. Ibarra, J. M. de la Fuente, C. Marquina, D. Rubiales and E. Prats, ACS Appl. Mater. Interfaces, 2014, 6, 9100–9110 CrossRef CAS PubMed.
- B. Thalmann, A. Voegelin, E. Morgenroth and R. Kaegi, Environ. Sci.: Nano, 2016, 3, 203–212 RSC.
- Y. Koo, J. Wang, Q. Zhang, H. Zhu, E. W. Chehab, V. L. Colvin, P. J. J. Alvarez and J. Braam, Environ. Sci. Technol., 2015, 49, 626–632 CrossRef CAS PubMed.
- W. Liu, C. Li, X. Sun, W. Pan, G. Yu and J. Wang, Nanotechnology, 2017, 28, 485705 CrossRef PubMed.
- A. Sachdev and P. Gopinath, Analyst, 2015, 140, 4260–4269 RSC.
- B. S. B. Kasibabu, S. L. D'souza, S. Jha and S. K. Kailasa, J. Fluoresc., 2015, 25, 803–810 CrossRef CAS PubMed.
- X. Jin, X. Sun, G. Chen, L. Ding, Y. Li, Z. Liu, Z. Wang, W. Pan, C. Hu and J. Wang, Carbon, 2015, 81, 388–395 CrossRef CAS.
- B. S. B. Kasibabu, S. L. D'Souza, S. Jha, R. K. Singhal, H. Basu and S. K. Kailasa, Anal. Methods, 2015, 7, 2373–2378 RSC.
- B. B. Chen, M. L. Liu, L. Zhan, C. M. Li and C. Z. Huang, Anal. Chem., 2018, 90, 4003–4009 CrossRef CAS PubMed.
- L. Yuan, W. Lin, K. Zheng and S. Zhu, Acc. Chem. Res., 2013, 46, 1462–1473 CrossRef CAS PubMed.
- T. R. Shojaei, M. A. Salleh, K. Sijam, R. A. Rahim, A. Mohsenifar, R. Safarnejad and M. Tabatabaei, Spectrochim. Acta Mol. Biomol. Spectrosc., 2016, 169, 216–222 CrossRef CAS PubMed.
- T. R. Shojaei, M. A. M. Salleh, K. Sijam, R. A. Rahim, A. Mohsenifar, R. Safarnejad and M. Tabatabaei, Microchim. Acta, 2016, 183, 2277–2287 CrossRef CAS.
- M. R. Safarnejad, F. Samiee, M. Tabatabie and A. Mohsenifar, Sens. Transducers J., 2017, 213, 54–60 CAS.
- Y. Liang, Y. Duan, C. Fan, H. Dong, J. Yang, J. Tang, G. Tang, W. Wang, N. Jiang and Y. Cao, Chem. Eng. J., 2019, 361, 671–679 CrossRef CAS.
- S. Pramanik, S. K. E. Hill, B. Zhi, N. V. Hudson-Smith, J. J. Wu, J. N. White, E. A. McIntire, V. S. S. K. Kondeti, A. L. Lee, P. J. Bruggeman, U. R. Kortshagen and C. L. Haynes, Environ. Sci.: Nano, 2018, 5, 1890–1901 RSC.
- K. M. Tsoi, Q. Dai, B. A. Alman and W. C. W. Chan, Acc. Chem. Res., 2013, 46, 662–671 CrossRef CAS PubMed.
- M. L. Pérez-Bueno, M. Pineda, F. M. Cabeza and M. Barón, Front. Plant Sci., 2016, 7, 1790 Search PubMed.
- F. Röck, N. Barsan and U. Weimar, Chem. Rev., 2008, 108, 705–725 CrossRef PubMed.
- Y. Geng, W. J. Peveler and V. M. Rotello, Angew. Chem., Int. Ed., 2019, 58, 5190–5200 CrossRef CAS PubMed.
- Z. Li, J. R. Askim and K. S. Suslick, Chem. Rev., 2019, 119, 231–292 CrossRef CAS PubMed.
- W. Cuypers and P. A. Lieberzeit, Front. Chem., 2018, 6, 268 CrossRef PubMed.
- J. R. Askim, M. Mahmoudi and K. S. Suslick, Chem. Soc. Rev., 2013, 42, 8649–8682 RSC.
- A. Cellini, S. Blasioli, E. Biondi, A. Bertaccini, I. Braschi and F. Spinelli, Sensors, 2017, 17, 2596 CrossRef PubMed.
- A. Cellini, G. Buriani, L. Rocchi, E. Rondelli, S. Savioli, M. T. Rodriguez Estrada, S. M. Cristescu, G. Costa and F. Spinelli, Mol. Plant Pathol., 2018, 19, 158–168 CrossRef CAS PubMed.
- Y. Fang, Y. Umasankar and R. P. Ramasamy, Analyst, 2014, 139, 3804–3810 RSC.
- J. Laothawornkitkul, J. P. Moore, J. E. Taylor, M. Possell, T. D. Gibson, C. N. Hewitt and N. D. Paul, Environ. Sci. Technol., 2008, 42, 8433–8439 CrossRef CAS PubMed.
-
M. M. Mahmud, N. Constantino, C. Seok, F. Y. Yamaner, R. A. Dean, and O. Oralkan, A CMUT - Based Electronic Nose for Real- Time Monitoring of Volatiles Emitted by Plants: Preliminary Results, in IEEE Sensors, 2018, pp. 1–4 Search PubMed.
- B. Esser, J. M. Schnorr and T. M. Swager, Angew. Chem., Int. Ed., 2012, 51, 5752–5756 CrossRef CAS PubMed.
- Z. Li and K. S. Suslick, Anal. Chem., 2019, 91, 797–802 CrossRef CAS PubMed.
- L. Shang, C. Liu, B. Chen and K. Hayashi, ACS Sens., 2018, 3, 1531–1538 CrossRef CAS PubMed.
- Z. Li, R. Paul, T. Ba Tis, A. C. Saville, J. C. Hansel, T. Yu, J. B. Ristaino and Q. Wei, Nat. Plants, 2019, 5, 856–866 CrossRef CAS PubMed.
- R. Sharma, M. Zhou, M. D. Hunter and X. Fan, J. Agric. Food Chem., 2019, 67, 7530–7537 CrossRef CAS PubMed.
- M. M. McCartney, S. L. Spitulski, A. Pasamontes, D. J. Peirano, M. J. Schirle, R. Cumeras, J. D. Simmons, J. L. Ware, J. F. Brown, A. J. Y. Poh, S. C. Dike, E. K. Foster, K. E. Godfrey and C. E. Davis, Talanta, 2016, 146, 148–154 CrossRef CAS PubMed.
- F. Martinelli, R. Scalenghe, S. Davino, S. Panno, G. Scuderi, P. Ruisi, P. Villa, D. Stroppiana, M. Boschetti, L. R. Goulart, C. E. Davis and A. M. Dandekar, Agron. Sustain. Dev., 2015, 35, 1–25 CrossRef.
|
This journal is © The Royal Society of Chemistry 2020 |