DOI:
10.1039/D0MO00007H
(Research Article)
Mol. Omics, 2020,
16, 185-194
In-plate toxicometabolomics of single zebrafish embryos†
Received
8th January 2020
, Accepted 3rd March 2020
First published on 6th March 2020
Abstract
Toxicometabolomic studies involving zebrafish embryos have become increasingly popular for linking apical endpoints to biochemical perturbations as part of adverse outcome pathway determination. These experiments involve pooling embryos to generate sufficient biomass for metabolomic measurement, which adds both time and cost. To address this limitation, we developed a high-throughput toxicometabolomic assay involving single zebrafish embryos. Incubation, microscopy, embryo extraction, and instrumental metabolomic analysis were all performed in the same 96-well plate, following acquisition of conventional toxicological endpoints. The total time for the assay (including testing of 6 doses/n = 12 embryos per dose plus positive and negative controls, assessing conventional endpoints, instrumental analysis, data processing and multivariate statistics) is <14 days. Metabolomic perturbations at low dose were linked statistically to those observed at high dose and in the presence of an adverse effect, thereby contextualizing omic data amongst apical endpoints. Overall, this assay enables collection of high resolution metabolomic data in a high throughput manner, suitable for mode of action hypothesis generation in the context of pharmaceutical or toxicological screening.
Introduction
EU REACH (Registration, Evaluation and Authorization of Chemicals) legislation requires chemical manufacturers to carry out comprehensive hazard assessments on new products, necessitating the use of large numbers of animals (11.5 million in 2011 from the European Union alone).1 In addition to the practical challenges associated with high throughput toxicity testing (e.g. time, money) there are ethical dilemmas stemming from experimentation involving animals. Recently, the United States Environmental Protection Agency pledged to stop financing animal tests by 20352 and within the EU REACH legislation lies imbedded the 3R principle which strongly encourages a reduction in animal testing.3 Substitution of the Organization for Economic Co-operation and Development (OECD) adult fish acute toxicity test (test guideline (TG) 203) with the fish embryo acute toxicity test (TG 236) addresses some of these issues4 through low cost, multi-well plate-based in vitro testing.5 However, since TG 236 is only designed to determine lethal concentration (LC50) it offers limited insight into a chemical's mode of action (MoA).6
To address the limitation surrounding conventional toxicity testing, omics studies involving zebrafish (ZF) embryos are increasingly used by the ecotoxicological and pharmaceutical community to aid in development of Adverse Outcome Pathways (AOPs).7,8 AOPs aim to link a molecular initiating event (MIE), through a series of key events (KEs), to apical endpoints, thus following the full progression of toxicity caused by a xenobiotic.9 Toxicometabolomics and other omics techniques (e.g. transcriptomics, proteomics) are integral tools for discerning the connections that constitute AOPs. For example, metabolomics could be applied to investigate the downstream biochemical changes arising from toxicant-induced gene expression alterations (such as the cholestasis AOP9).9 However, current ZF embryo toxicometabolomic studies suffer from some limitations. For instance, few studies have linked apical endpoints to metabolomic outcomes, making it difficult to interpret the data in a regulatory context.10 Furthermore, all ZF embryo toxicometabolomic studies performed to date have employed pooling approaches, extracting between 3 and 80 embryos per replicate, in order to obtain sufficient biomass for instrumental analysis.7,8,11–13 In addition to the time and costs associated with this approach, pooling decouples apical endpoints from metabolic perturbations in individual embryos. While the analysis of single ZF embryos could facilitate direct linkages between metabolic perturbation and apical endpoints, single ZF embryo metabolomics is hampered by their small size (∼200 μg per individual at 120 hours post fertilization (hpf)), which makes them difficult to extract and ultimately generate sufficient quantities of extract for instrumental analysis. Perhaps for this reason, single ZF embryo metabolomics has, to our knowledge, only been attempted once, with a focus on studying changes in the metabolome of developing embryos.14 In that work, a 3-step homogenization scheme was followed by filtration and pre-concentration prior to liquid chromatography (LC)- and gas chromatography (GC)-mass spectrometry (MS) analysis.14 However, preparing samples in this manner is time consuming, and limits the number of replicates which are practically possible to analyze.
Here we present a novel, high-throughput toxicometabolomic assay involving single ZF embryos. Incubation, microscopy, embryo extraction, and instrumental analysis were all performed in the same 96-well plate, resulting in a total assay time of less than 2 weeks. Metabolomic effects at low dose are statistically linked to those at high dose (i.e. in the presence of an apical endpoint) which places these data into context amongst conventional toxicological endpoints. For proof of concept we chose the beta blocker propranolol (PPL), a commonly prescribed heart medicine developed in the 1960s.15 We considered PPL a suitable compound for this study not only due to the large body of literature available to support eventual findings, but also because it had a distinct documented apical endpoint (heart rate) in ZF embryos which informed our choice of doses.16 Overall, this sample preparation and analysis method is suitable for high sensitivity, high throughput MoA hypothesis generation in pharmaceutical or toxicological screening.
Materials and methods
In-plate heart rate, morphology, and mortality measurements according to TG 236
According to EU Directive 2010/63/EU on the protection of animals used for scientific purposes, early life-stages of ZF are not protected as animals until the stage of being capable of independent feeding, which in ZF is 120 hpf.17,18 Since our experiments were terminated before 120 hpf, no ethics approval was required. ZF embryos were incubated from spawning to 120 hpf with PPL. The exposure setup was guided by general conditions outlined in TG236,4 with the following deviations: slightly longer exposure time (120 hpf in the present study vs. 96 hpf under TG 236, still within the time where embryo experiments are considered to be in vitro17), an absence of pH measurements in the present work, fewer replicates (12 in the present work vs. 20 specified in TG 236), and a higher solvent concentration in the positive controls in the present study (see Notes S1, ESI† for a description of deviations from the TG 236 assay protocol). In addition, we used fewer lethal doses than what is specified under TG 236. Finally, heart rate measurements were also included as an apical endpoint, but are not required under TG 236. A single plate consisted of 6 different concentrations of PPL (n = 12 embryos/concentration), a vehicle control (n = 12 embryos) and a positive control (3.8 mg L−1 3,4-dichloroaniline (DCA); n = 12 embryos; see Fig. S1 ESI,† for plate layout). At 48 and 120 hpf we examined embryos microscopically for standard lethal and sublethal apical endpoints (i.e. completion of Gastrula, formation of somites, development of eyes, spontaneous movement, heart beat/blood circulation (boolean criteria), pigmentation, oedema, malformation of head/saccule/otoliths/tail/heart, modified structure of the corda, scoliosis, rachischisis, deformity of yolk, growth-retardation).18 One non-standard non-lethal endpoint was also measured (heart rate;16,19 Table S1, ESI†). To evaluate the potential for future cross-plate comparison, and the reproducibility of our in-plate sample preparation method, we prepared 48 non-exposed (i.e. control) embryos distributed over four plates from a second incubation event (see Text S1, ESI†).
Analysis of dose
Exposure concentrations of PPL were determined via direct injection LC-MS20 (see Text S1, ESI† for details). By measuring the exposure water in each well, we were able to determine the concentrations for all but the lowest dose (which was below the limit of quantification 0.20 μg L−1; Table S2, ESI†). The measured concentrations in the exposure medium were on average 0.49, 12, 62, 4550 and 46
540 μg L−1 at 120 hpf. The nominal dose for the lowest concentration was 0.050 μg L−1. Through analysis of exposure medium in the beginning, middle and end of the exposure, from a designated exposure medium plate, we also confirmed that doses stayed within ±10% over the duration of exposure. Therefore, we could rule out substantial degradation or biotransformation during the study (Table S3, ESI†).
In-plate sample preparation and analysis sequence
To minimize sample handling and enhance throughput for metabolomic analysis, we developed a method for in-plate extraction which allows for processing of up to several hundred embryos in <3 hours. Metabolite extraction and protein precipitation involved bead blending and sonication with methanol/chloroform (plus internal standard), followed by centrifugation (see ESI,† Text S1 for further details), after which the plate was placed directly in the autosampler of the LC for instrumental analysis. We used mixed-diameter stainless-steel beads to reproducibly homogenize the embryos, as opposed to single-sized beads which tended to leave the larvae husk intact. By gluing silicone cap-mats lined with polytetrafluoroethylene (PTFE) to each 96-well plate, up to 960 embryos (i.e. 10 × 96-well plates stacked) could potentially be homogenized simultaneously with this method. To ensure the absence of cross contamination between wells, we performed a number of a priori microscopy experiments where no sign of moisture between the wells was detectable and where empty wells close to solvent-filled wells remained dry after homogenization. Blanks were prepared in separate blank plates alongside the sample plates, but followed the exact same treatment as normal samples, and were injected prior to the analysis of real samples.
In order to avoid compromising the LC columns and MS through injection of small, insoluble organic fragments we increased the autosampler needle-height to draw extract 5 mm from the bottom of the wells (as opposed to the default setting 2 mm). In this step, the extraction beads also served to stop suction-induced turbulence from disturbing tissue remnants at the bottom of the wells. As a final precaution, we installed an in-line metal filter (0.5 μm) downstream of the autosampler but upstream of the analytical column. Each plate underwent three analyses over 74 hours: (a) a lipidomics analysis via flow-injection MS/MS, (b) non-targeted analysis using hydrophilic interaction chromatography (HILIC) positive ionization Orbitrap high resolution MS; and (c) resuspension followed by reversed phase-chromatography negative ionization Orbitrap high resolution MS (see ESI,† Text S1).
Signal-drift during both lipidomic and non-targeted analyses were corrected using the R-package batchCorr21 which required inclusion of quality control (QC) samples throughout the injection sequences. The QCs were prepared in 1.5 mL polypropylene tubes and consisted of extract of five pooled embryos, for which the same solvent to sample ratio as for plate samples was used. Blanks for the QCs were prepared in 1.5 mL polypropylene tubes and analyzed together with the plate blanks. Furthermore, we analyzed a portion of the highest concentration dose medium with both Orbitrap methods to identify features corresponding to PPL (e.g. parent ions, in-source fragments, and impurities), and remove them during processing. Collectively, analysis using all three methods yielded over 13
000 raw features per embryo.
Isolation of endogenous metabolites through data filtration
Both lipidomic and non-targeted data were processed using previously described methods developed by our group,22 the former of which was adapted from Liebisch et al.22,23 (see also ESI,† Text S1). Peaks acquired through lipidomics analysis had to be on average 10-fold higher than in blanks to be considered metabolite features. For non-targeted data, exogenous substances (e.g. PPL, its metabolites and background noise) were removed using a combination of batchCorr21 and an in-house R package comprised of a total of 6 data filters.24 The first filter used the gap-filling status recorded by Compound Discoverer (CD) to remove features not detected in any of the sequence QC injections. Features which passed the gap-filter were then subjected to sequence correction using batchCorr21 and any features displaying relative standard deviations (RSD) above 30% in the sequence QCs post correction were discarded. The third filter removed low-intensity features (i.e. those features with highest peak height <200
000 counts per second for positive electro spray ionization (ESI+) HILIC or <150
000 counts per second for ESI-reversed-phase) where after the remaining features were internal standard-corrected to account for inter-well variability in evaporation. For the fourth filter we used the annotation package “ramclustR” to detect and remove any in-source fragments of the masses predicted to be metabolites of PPL by CD, based on retention time and MS1 intensities.25 The fifth filter removed all features within 5 ppm of masses on a list of expected phase I and II metabolites of PPL generated by CD, as well as all features detected in the exposure medium and in blanks. The sixth filter removed any feature which had negative intensities and the seventh filter discarded features present in procedural blanks with peaks >40% relative to the maximum signal in samples or QCs respectively. Overall, this procedure reduced the total number of features obtained from lipidomic and Orbitrap analyses from >13
000 to <350 (Table S4, ESI†).
Statistical analysis
Statistical analysis for apical observations were carried out using Student's t-test for heart rates and Pearson's Chi-squared test for mortality. Inter-plate reproducibility was assessed using principal component analysis (PCA). For metabolomics data from the PPL exposure statistical analysis was performed using the R-package MUVR26 which performs minimal variable selection through recursive variable elimination by repeated double cross-validation (Table S5, ESI†). In order to both characterize the MoA of PPL and to obtain data suitable for benchmarking of exposure, we developed and refined two random forest (RF) models: a classification and a regression model.
Metabolite identification through retention time and MS2-based fingerprint matching
Metabolite features measured by non-targeted analysis and selected by the models were putatively identified using mzCloud (through CD), Metlin,27 Metfrag28 and Sirius + CSI:FingerID.29,30 For compounds where there was no clear consensus regarding identification between these four software, we used the structure proposed by Sirius + CSI:FingerID due to its performance in inter-method comparisons.31 Following putative identification, we procured authentic standards and determined a similarity score between sample and standard MS2 spectra using the R-script “NTScreeneR”32 (see ESI,† Data S1). All candidate metabolite features displayed a similarity score >0.85, which we deemed sufficient to confirm their identities.
Ecotoxicological evaluation
Determination of a benchmarking dose (BMD) for the metabolomic perturbations connected to the change in heart rate was carried out by using the RF regression model to predict the four concentrations not used to train the model (i.e. 0.05 μg L−1, 0.49 μg L−1, 12 μg L−1 and 62 μg L−1). For these four dose-groups the Q2-values for all groups together and separately were calculated (see ESI,† eqn (S1)). The predicted concentrations were then entered into the US Environmental Protection Agency (EPA) BMD calculating tool BMDS 3.1.33 For the BMDS analysis the default preset settings were used (i.e. BMR type “Std. Dev.”, BMRF of 1, confidence level of 0.95, distribution set to “normal”, variance set to “constant” and the “use dataset adverse direction” option for polynomial restriction).
Results and discussion
Apical endpoints
Significant mortality was only observed in the positive controls, both at 48 and 120 hpf. We observed significantly lower heart rates (relative to negative controls) in both the positive control and the 46
540 μg L−1 exposure group at 48 hpf (Fig. 1a), as well as the 46
540 and 4550 μg L−1 groups at 120 hpf (Fig. 1b). These results align well with previously determined no- and lowest-observed effect concentrations (NOEC and LOEC, respectively) for decreased heart rate due to PPL exposure in ZF embryos (3500 μg L−1 and 7000 μg L−1 respectively at 48 hpf19). At 120 hpf all living embryos had hatched.
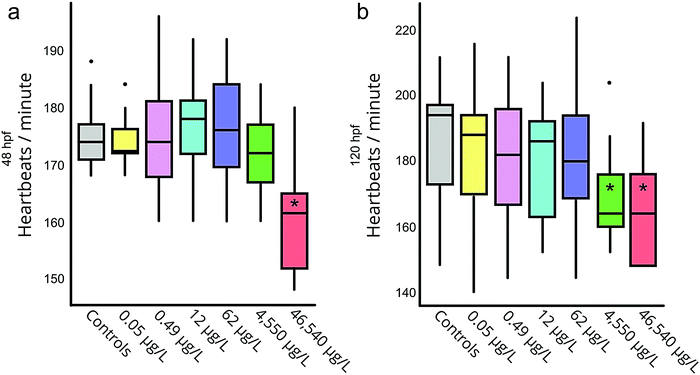 |
| Fig. 1 Heart rate of embryos measured at 48 hpf (a) and 120 hpf (b) for the range of exposure concentrations. Heart rate was measured through microscopy and determined by counting the number of heartbeats of the embryo during 15 seconds. Groups with significantly lower heart rate compared to controls (p < 0.05) are marked by *. | |
Reproducibility of between-plate controls
After instrumental analysis (i.e. non-targeted analysis and lipidomics) and a data processing approach slightly modified to facilitate batch comparison (see ESI,† Text S1) we used PCA to obtain an unbiased measure of between-plate variability in the measured metabolome. Evaluating the PCA score plots for both the lipidomics (Fig. 2a) and non-targeted data (Fig. 2b), as well as a combination thereof (Fig. 2c) it is not surprising that only the non-targeted data scores plot shows signs of outliers (Fig. 2a). Non-targeted metabolomics is not only susceptible to in-sequence drift but also drift between batches, both in mass and baseline signal.21 To counteract these phenomena we prepared a batch QC sample which, together with batchCorr, was used to normalize the baseline between the non-targeted data of the four plates.21 Since the outliers are not present in the lipidomics data (Fig. 2b) we conclude that the deviation of the outliers is not inherent to the embryos or the sample preparation but rather stems from the analysis. Interestingly, the extreme samples in the non-targeted data are almost completely attenuated in the first 2 PCs by combining the datasets (Fig. 2c). Altogether, by utilizing the full functionality of batchCorr,21 we were able to show that ZF embryos can be reproducibly incubated, homogenized and analyzed using our in-plate sample preparation and analysis methods.
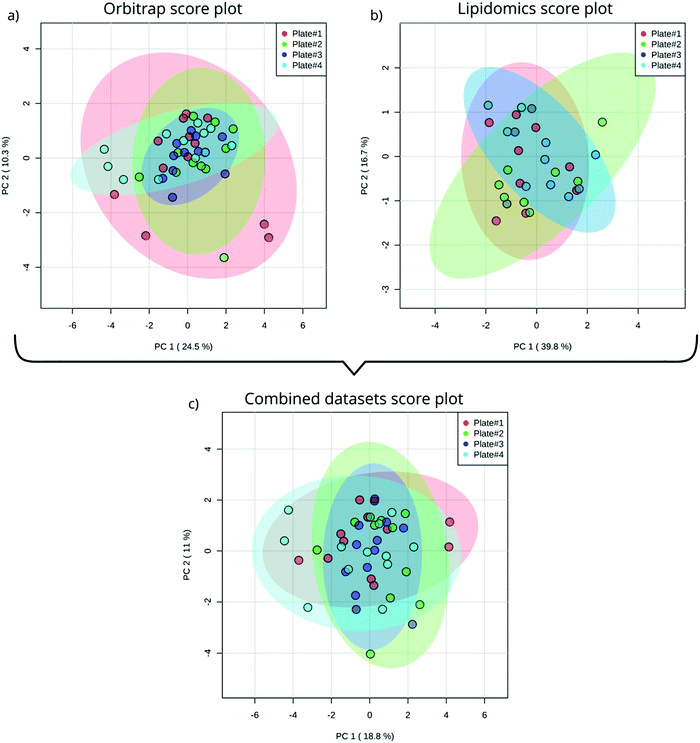 |
| Fig. 2 PCA score plot of single ZF embryo data from four different plates acquisitioned by Orbitrap utilizing HILIC chromatography (a); lipidomics analysis (b) and a combination of both analyses (c). | |
PPL exposure modelling
For the classification model we used all PPL exposure concentrations as well as negative controls as input which generated a model based on 34 of the 311 metabolite features. Unsurprisingly, the majority of misclassification of samples occurred between similar dosing concentrations (i.e. doses within 1 order of magnitude; Fig. 3a). However, when we considered misclassification as only those samples which were incorrectly classified by over an order of magnitude, the misclassification-rate decreased from 51% to only 24% (Fig. 3a). Permutation analysis of the model with randomized sample labels revealed that the model was highly statistically significant (n = 100, p = 1.16 × 10−10) which confirmed that there was no overfitting. In comparison, the RF regression model was constructed using only the negative control along with the groups in which statistically significant apical endpoints were observed (i.e. heart rate perturbations at 46
540 and 4550 μg L−1; Fig. 3b). This model resulted in a selection of 10 metabolite features, 7 of which were also identified by the classification model (Fig. 4). The Q2 and p-value for 100 permutations were 0.67 and 9.2 × 10−5 respectively. In summary, we obtained two adequately accurate and non-overfitted RF models of PPL exposure using MS-data collected from single ZF embryos through RF analysis.
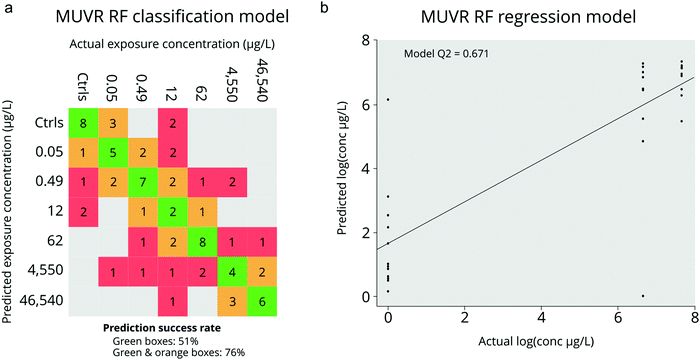 |
| Fig. 3 Results of MUVR random forest (RF) modelling. Panel a shows results of the RF classification model with actual and predicted exposure groups in the columns and rows, respectively (green for correct predictions, orange for prediction within one order of magnitude and red for erroneous predictions). Panel b shows the results of the RF regression model, trained on the two exposure groups with lower heart rate at 120 hpf (i.e. 4550 and 46 540 μg L)−1 and the negative controls (Q2 = 0.67, p = 9.2 × 10−5). | |
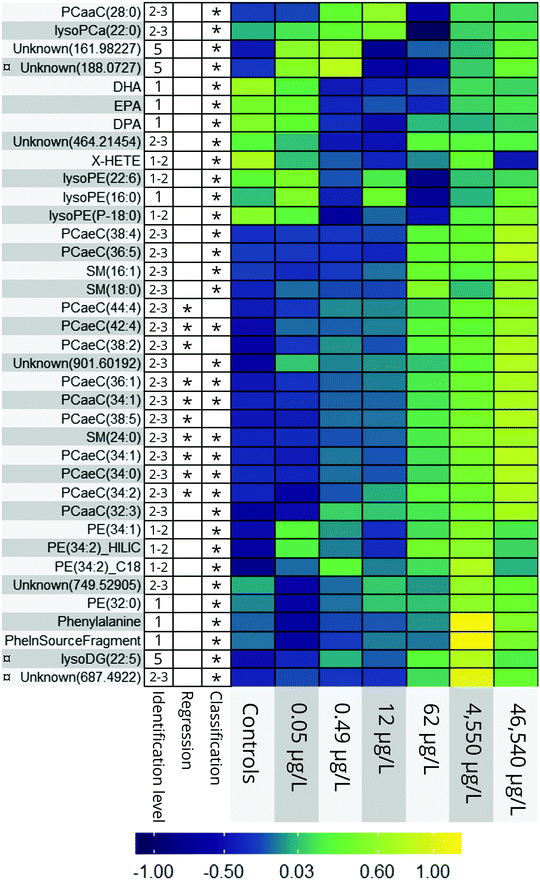 |
| Fig. 4 Heatmap of all endogenous metabolites elected by the classification and regression random forest models. 1–5 = level of putative identification as suggested by Schymanski et al.48 = no MS2 data gathered. | |
PPL mode of action
The relative, scaled abundance of the 11 structurally confirmed, 20 putatively identified, and the 6 unidentified metabolite features elected by the two models were plotted on a heat map to obtain a biochemical overview of the exposure (Fig. 4). Both monotonic and non-monotonic dose-responses were observed, highlighting the multi-faceted and complex perturbation of the metabolome caused by PPL exposure.
It is well-documented that PPL strongly interacts with the phosphatidate phosphatase (PAP)/phospholipase D (PLD) pathway, causing inhibition of PAP and induction of PLD (Fig. 5a).34–36 The PAP/PLD pathway is a major component of glycerophospholipid metabolism in which a number of other enzymes have been documented to be affected by PPL exposure (Fig. 5a). Phospholipids such as phosphatidylcholine (PC), -ethanolamine (PE), -inositol (PI), -serine (PS), -glycerol (PG) and phosphatidic acid (PA) as well as the fatty acids (FA) which they are composed of are not only structural components of the lipid membrane but also signaling molecules governing important cellular and physiological mechanisms such as cytokinesis,37 apoptosis,38 neurotransmission39 and inflammation.40 Thus, it is no surprise that a large number of PC lipids show signs of a monotonic increase in abundance over the exposure doses in this study (Fig. 4). Interesting to note is that our RF regression model is solely based on compounds with an increase in relative concentration over increasing exposure doses.
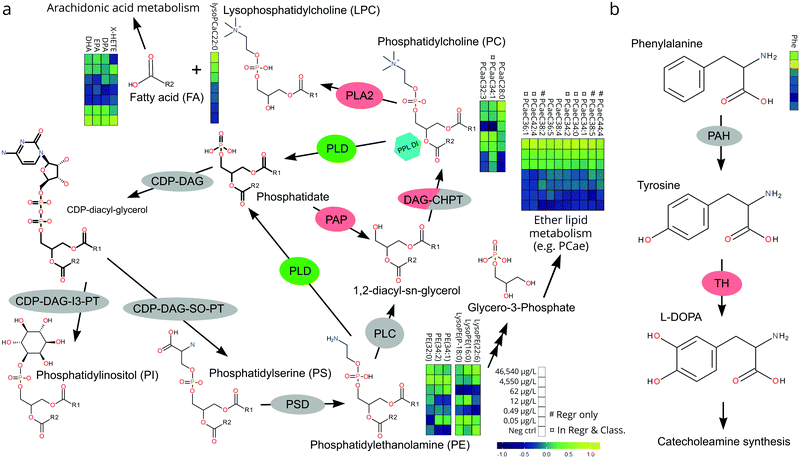 |
| Fig. 5 Pathways of phospholipid (a) and tyrosine (b) metabolism implicated in the mode of action of PPL. Enzymes are color-coded for up-regulation or induced activity (green), down-regulation or reduced activity (red) or no documented effect (gray) associated with PPL. The hexagon indicates a direct interaction of PPL (PPL DI) with phopshatidylo choline lipids. Metabolite heatmaps are arranged from the highest concentration (top) to the negative control (bottom). # = only in regression model; = both in regression and classification model. | |
We hypothesize that the chain of events that induces PC lipids with one ether-bound FA also explains the increase of PE lipids in higher exposure doses (Fig. 4). When PPL induces PLD and inhibits PAP a build-up of PA will occur36 which will lead to a subsequent increase in PI and PS.41 PS can be further metabolized into PE which in turn can be metabolized into glycerol-3-phosphate, one of the main starting molecules for ether lipid metabolism (Fig. 5a). Also, many enzymes which are either directly or indirectly affected by PPL can be found within the ether lipid metabolism pathway (e.g. phospholipase A2 (PLA2),42 PAP, PLD and PLC43). So, despite the lack of detailed knowledge on how PPL interacts with ether lipid metabolism, our findings in literature are indicative of a MoA which would explain the stepwise increase and subsequent decline in PE and the monotonic increase in PC ether lipids over the exposure concentrations.
The heart rate-lowering MoA of PPL through blockage of β-adrenergic receptors is well studied.44 Activation of these receptors result in adenylyl cyclase activation and conversion of ATP into cAMP. cAMP then activates protein kinase A which leads to contraction through phosphorylation of ryanodine receptors and L-type Ca2+ channels.44 There is, however, an alternative way in which PPL influences this pathway, through the PLD-induced increase in PA.45 It has been shown that PPL induced build-up of PA activates type 4 cAMP-specific phosphodieseterase (PDE4) which turns cAMP into 5′AMP, thus inhibiting cAMP-activation of protein kinase A.45 This alternative pathway constitutes a strong link between the lipid metabolites elected for our regression model and the apically observed heart rate reduction in the most highly exposed embryos.
A number of biologically important FAs (i.e. docosapentaenoic acid, eicosapentaenoic acid, docosahexaenoic acid, X-hydroxy eicosatetraenoic acid) showed similar non-monotonic expression patterns to one another, consistent with the response observed for a number of lysoPEs and lysoPCs (Fig. 4). The concentration of these FAs decreased in the lower dose groups (0.5–62 μg L−1) before returning to levels similar to controls at the highest doses (4540–46
540 μg L−1). This could be explained by the ability of PPL to inhibit the activation of PLA2, which facilitates hydrolysis of acyl-bound FAs from phospholipids (Fig. 5a).42 Considering that all of the affected lipids and FAs are messenger molecules, we propose that fold-change increases in higher doses might be the result of many negative and positive feedback loops interacting with each other.
The only non-lipid related metabolite feature we identified with a level 1 certainty was phenylalanine, which has also been reported in a previous metabolomics study on PPL exposure.46 The direct inhibition of tyrosine hydroxylase (TH) by PPL is a likely explanation for the relative increase of phenylalanine in the two highest exposure concentrations in our data (Fig. 5b).47 If tyrosine concentrations grow large, due to TH-inhibition, alternative pathways could be over-burdened which would ultimately result in a build-up of its precursor phenylalanine. Thus, we conclude that the metabolites elected by our models are consistent with existing literature on PPL and provide strong evidence supporting their perturbation at the exposure concentrations investigated in the present work.
Ecotoxicological evaluation
The results showed a large variation in predictability of different dose concentrations (Fig. 6): The two higher doses (12 μg L−1 and 62 μg L−1) were very close to modelled values while lower doses (0.05 μg L−1 and 0.49 μg L−1; Fig. 5) suffered from slightly worse prediction accuracy, although without any measurable bias (Fig. 6). Importantly, predicted doses at all concentrations were significantly higher than predictions of the negative control, suggesting a high potential to correctly identify the occurrence of exposure even at such low exposures that could not be accurately predicted (Fig. 6). We also used the data acquired from the model to make BMD calculations using the US EPA BMD tool (BMDS, version 3.0).33 This resulted in a log-normal model with constant variance which fulfilled all but one of the default requirements of BMDS (see ESI,† Table S6). The metabolomic BMD (lower confidence level; BMDL) and LOEC of this model were 0.0016 μg L−1 and 0.050 μg L−1 at 120 hpf, which are orders of magnitude lower than the LOEC for heart rate (7000 μg L−1 at 48 hpf;19 46
540 μg L−1 at 48 hpf in this study) which the metabolomic effect is anchored in. Unfortunately, we were unable to find any heart rate BMDL for PPL in the literature.
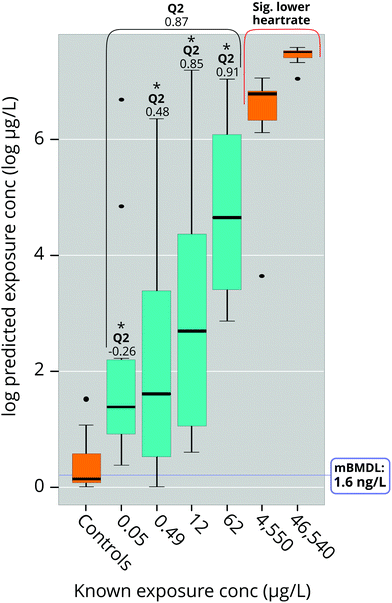 |
| Fig. 6 Exposure concentration predicted by the MUVR RF regression model trained on exposure groups marked in orange. Exposure groups marked in turquoise were not used for model construction, but still produced dose-dependent predictions. Concentrations predicted to be significantly (p < 0.05) lower than 4550 μg L−1 and higher than negative controls are denoted with *. Of note, although predictions at the lowest exposure (0.05 μg L−1) were inaccurate, the model still managed to identify these samples as exposed. mBMDL is the lower metabolomics benchmarking dose as determined by the US EPA tool “BMDS 3.0” using prediction data from the regression model. | |
Conclusions
The present work demonstrates that combining metabolomics with apical endpoints in single ZF embryos provides a robust and high throughput means of obtaining data on a chemical's MoA. The newly developed in-plate extraction procedure minimized sample handling error induced by multi-step protocols and the delicacy of small sample volumes, while also improving time-efficiency, thus enabling large-scale, high-throughput analysis. The possibility to determine metabolomic BMDs, NOECs and LOECs linked to apical endpoints in single fish embryos not only forwards the possibility to use omics data in a legislative context but it also emphasizes the question of where the threshold of an adverse effect should be drawn. Beyond molecular hazard screening, the developed in-plate sample processing method can be applied to metabolomic studies in other species or for the rapid determination of chemical transformation products. Overall, the method presented here constitutes a significant development in high-throughput toxicometabolomics in small organisms.
Ethics statement
The embryos in this study were excess material generated from animals used under permit C161.14, from the ZF facility at SciLife labs in Uppsala, Sweden. According to EU Directive 2010/63/EU on the protection of animals used for scientific purposes, early life-stages of ZF are not protected as animals until the stage of being capable of independent feeding, which in ZF is 120 hours post fertilization.33,34 Since our experiments were terminated before 120 hours post fertilization, no ethics approval was required.
Author contributions
Anton Ribbenstedt: project conceptualization, experimental design, incubation and exposure of ZF embryos, sample preparation, instrumental analysis, statistical analysis, pathway perturbation analysis, interpretation of results, manuscript preparation. Malte Posselt: contributions to experimental design, incubation and exposure of ZF embryos, dose analysis, interpretation of results, manuscript preparation. Carl Brunius: statistical analysis, interpretation of results, manuscript preparation. Jonathan P. Benskin: project conceptualization, experimental design, statistical analysis, interpretation of results, manuscript preparation.
Data availability
Datasets obtained through data processing of instrumental raw files from Thermo Scientific Quantiva and from Q Exactive Orbitrap instrumental analysis are available in the Data Dryad repository (https://doi.org/10.5061/dryad.7m0cfxppz). The raw files generated through instrumental analysis in this study, as well as result files generated by data processing software, are available from the corresponding author upon reasonable request.
Code availability
All code involved in formating data prior to employment of functions included in the “batchCorr” R-package are available through requests sent to the corresponding authors. Code related to reorganization and deconvolution of lipidomics data is available as a package for R called “metLab”, (https://github.com/parasitetwin/metLab). All functionality connected to our non-targeted data filtering procedures are available as a package for R called “ExpMetFilter”, (https://github.com/parasitetwin/ExpMetFilter).
Conflicts of interest
The authors declare no competing interests.
Acknowledgements
We thank the SciLife lab facility in Uppsala, Sweden for providing their expertise, embryos and labs to perform the incubation and apical analysis.
References
- European Commission, ‘Report from the commission to the council and the European parlament’, COM(2013) 859 Final, available at https://eur-lex.europa.eu/procedure/EN/1041492, accessed in May 2019.
- O. US EPA, Administrator Wheeler Signs Memo to Reduce Animal Testing, Awards $4.25 Million to Advance Research on Alternative Methods to Animal Testing, https://www.epa.gov/newsreleases/administrator-wheeler-signs-memo-reduce-animal-testing-awards-425-million-advance, accessed 18 September 2019.
- R. Čihák, Interdiscip. Toxicol., 2009, 2, 42–44 Search PubMed.
-
OECD, Test No. 236: Fish Embryo Acute Toxicity (FET) Test, Organisation for Economic Co-operation and Development, Paris, 2013 Search PubMed.
- European Commission, ‘White Paper – Strategy for a future Chemicals Policy’, COM(2001) 0088 Final, available at https://eur-lex.europa.eu/legal-content/EN/ALL/?uri=CELEX:52001DC0088, accessed in March 2020.
- M. Sobanska, S. Scholz, A.-M. Nyman, R. Cesnaitis, S. G. Alonso, N. Klüver, R. Kühne, H. Tyle, J. de Knecht, Z. Dang, I. Lundbergh, C. Carlon and W. D. Coen, Environ. Toxicol. Chem., 2018, 37, 657–670 CrossRef CAS PubMed.
- S. S. Y. Huang, J. P. Benskin, N. Veldhoen, B. Chandramouli, H. Butler, C. C. Helbing and J. R. Cosgrove, Aquat. Toxicol., 2017, 182, 102–112 CrossRef CAS PubMed.
- J. Fu, Z. Gong and B. C. Kelly, Environ. Toxicol. Chem., 2019, 38, 240–249 CrossRef CAS PubMed.
- C. Wittwehr, H. Aladjov, G. Ankley, H. J. Byrne, J. de Knecht, E. Heinzle, G. Klambauer, B. Landesmann, M. Luijten, C. MacKay, G. Maxwell, M. E. (Bette) Meek, A. Paini, E. Perkins, T. Sobanski, D. Villeneuve, K. M. Waters and M. Whelan, Toxicol. Sci, 2017, 155, 326–336 CrossRef CAS PubMed.
- T. Ramirez, M. Daneshian, H. Kamp, F. Y. Bois, M. R. Clench, M. Coen, B. Donley, S. M. Fischer, D. R. Ekman, E. Fabian, C. Guillou, J. Heuer, H. T. Hogberg, H. Jungnickel, H. C. Keun, G. Krennrich, E. Krupp, A. Luch, F. Noor, E. Peter, B. Riefke, M. Seymour, N. Skinner, L. Smirnova, E. Verheij, S. Wagner, T. Hartung, B. van Ravenzwaay and M. Leist, ALTEX, 2013, 30, 209–225 CrossRef PubMed.
- S. S. Y. Huang, J. P. Benskin, B. Chandramouli, H. Butler, C. C. Helbing and J. R. Cosgrove, Environ. Sci. Technol., 2016, 50, 6526–6535 CrossRef CAS PubMed.
- S.-M. Huang, F. Xu, S. H. Lam, Z. Gong and C. N. Ong, Mol. BioSyst., 2013, 9, 1372–1380 RSC.
- D. B. D. Simmons, J. P. Benskin, J. R. Cosgrove, B. P. Duncker, D. R. Ekman, C. J. Martyniuk and J. P. Sherry, Environ. Toxicol. Chem., 2015, 34, 1693–1704 CrossRef CAS PubMed.
- S. Hayashi, M. Yoshida, T. Fujiwara, S. Maegawa and E. Fukusaki, Z. Naturforsch., C: J. Biosci., 2014, 66, 191–198 Search PubMed.
- J. W. Black, A. F. Crowther, R. G. Shanks, L. H. Smith and A. C. Dornhorst, Lancet, 1964, 283, 1080–1081 CrossRef.
- J. Finn, M. Hui, V. Li, V. Lorenzi, N. de la Paz, S. H. Cheng, L. Lai-Chan and D. Schlenk, Aquat. Toxicol., 2012, 122–123, 214–221 CrossRef CAS PubMed.
- U. Strähle, S. Scholz, R. Geisler, P. Greiner, H. Hollert, S. Rastegar, A. Schumacher, I. Selderslaghs, C. Weiss, H. Witters and T. Braunbeck, Reprod. Toxicol., 2012, 33, 128–132 CrossRef PubMed.
- R. Nagel, Altex – Altern. Zu Tierexp., 2002, 19, 38–48 Search PubMed.
- B. Fraysse, R. Mons and J. Garric, Ecotoxicol. Environ. Saf., 2006, 63, 253–267 CrossRef CAS PubMed.
- M. Posselt, A. Jaeger, J. L. Schaper, M. Radke and J. P. Benskin, Environ. Sci.: Process Impacts, 2018, 20, 1716–1727 CAS.
- C. Brunius, L. Shi and R. Landberg, Metabolomics, 2016, 12, 173 CrossRef PubMed.
- A. Ribbenstedt, H. Ziarrusta and J. P. Benskin, PLoS One, 2018, 13, e0207082 CrossRef PubMed.
- G. Liebisch, B. Lieser, J. Rahtenberg, W. Drobnik and G. Schmitz, Biochim. Biophys. Acta, Mol. Cell Biol. Lipids, 2005, 1734, 86–89 CrossRef CAS.
-
A. Ribbenstedt, ExpMetFilter, Zenodo, 2019, https://github.com/parasitetwin/ExpMetFilter/tree/v1.0 Search PubMed.
- C. D. Broeckling, F. A. Afsar, S. Neumann, A. Ben-Hur and J. E. Prenni, Anal. Chem., 2014, 86, 6812–6817 CrossRef CAS PubMed.
- L. Shi, J. A. Westerhuis, J. Rosén, R. Landberg and C. Brunius, Bioinformatics, 2019, 35, 972–980 CrossRef CAS PubMed.
- C. A. Smith, G. O. Maille, E. J. Want, C. Qin, S. A. Trauger, T. R. Brandon, D. E. Custodio, R. Abagyan and G. Siuzdak, Ther. Drug Monit., 2005, 27, 747 CrossRef CAS PubMed.
- C. Ruttkies, E. L. Schymanski, S. Wolf, J. Hollender and S. Neumann, J. Cheminf., 2016, 8, 3 Search PubMed.
- K. Dührkop, M. Fleischauer, M. Ludwig, A. A. Aksenov, A. V. Melnik, M. Meusel, P. C. Dorrestein, J. Rousu and S. Böcker, Nat. Methods, 2019, 16, 299 CrossRef PubMed.
- K. Dührkop, H. Shen, M. Meusel, J. Rousu and S. Böcker, Proc. Natl. Acad. Sci. U. S. A., 2015, 112, 12580–12585 CrossRef PubMed.
- I. Blaženović, T. Kind, J. Ji and O. Fiehn, Metabolites, 2018, 8, 31 CrossRef PubMed.
-
J. Schollée, NTScreeneR, 2019 Search PubMed.
- J. A. Davis, J. S. Gift and Q. J. Zhao, Toxicol. Appl. Pharmacol., 2011, 254, 181–191 CrossRef CAS PubMed.
- M. Bobeszko, A. Dygas, I. Nalepa and J. Barańska, Cell. Signalling, 2000, 12, 399–404 CrossRef CAS PubMed.
- F. Pascual and G. M. Carman, Biochim. Biophys. Acta, 2013, 1831, 514–522 CrossRef CAS PubMed.
- K. E. Meier, K. C. Gause, A. E. Wisehart-Johnson, A. C. S. Gore, E. L. Finley, L. G. Jones, C. D. Bradshaw, A. F. McNair and K. M. Ella, Cell. Signalling, 1998, 10, 415–426 CrossRef CAS PubMed.
- K. Emoto and M. Umeda, Cell Struct. Funct., 2001, 26, 659–665 CrossRef CAS PubMed.
- S. Orrenius, B. Zhivotovsky and P. Nicotera, Nat. Rev. Mol. Cell Biol., 2003, 4, 552–565 CrossRef CAS PubMed.
- R. P. Bazinet and S. Layé, Nat. Rev. Neurosci., 2014, 15, 771–785 CrossRef CAS PubMed.
- P. C. Calder, Am. J. Clin. Nutr., 2006, 83, 1505S–1519S CrossRef CAS PubMed.
- M. I. Aveldaño, S. J. P. de Garcia and N. G. Bazán, J. Lipid Res., 1983, 24, 628–638 Search PubMed.
- J. Y. Vanderhoek and M. B. Feinstein, Mol. Pharmacol., 1979, 16, 171–180 CAS.
- S. N. P. Murthy, P. H. Chung, L. Lin and J. W. Lomasney, Biochemistry, 2006, 45, 10987–10997 CrossRef CAS PubMed.
- I. Y. Kuo and B. E. Ehrlich, Cold Spring Harbor Perspect. Biol., 2015, 7, a006023 CrossRef PubMed.
- M. Grange, C. Sette, M. Cuomo, M. Conti, M. Lagarde, A. F. Prigent and G. Némoz, J. Biol. Chem., 2000, 275, 33379–33387 CrossRef CAS PubMed.
- C. Gómez-Canela, T. H. Miller, N. R. Bury, R. Tauler and L. P. Barron, Sci. Total Environ, 2016, 562, 777–788 CrossRef PubMed.
- N. Tuross and R. L. Patrick, J. Pharmacol. Exp. Ther., 1986, 237, 739–745 CAS.
- E. L. Schymanski, J. Jeon, R. Gulde, K. Fenner, M. Ruff, H. P. Singer and J. Hollender, Environ. Sci. Technol., 2014, 48, 2097–2098 CrossRef CAS PubMed.
Footnote |
† Electronic supplementary information (ESI) available. See DOI: 10.1039/d0mo00007h |
|
This journal is © The Royal Society of Chemistry 2020 |