DOI:
10.1039/D0MA00472C
(Paper)
Mater. Adv., 2020,
1, 2888-2896
Complementary techniques to analyse pericellular matrix formation by human MSC within hyaluronic acid hydrogels†
Received
2nd July 2020
, Accepted 15th October 2020
First published on 16th October 2020
Abstract
Hydrogels are widely used as mimics of the native extracellular matrix as their physical and biological properties can be tuned over a wide range to match those of the native tissue. Cells encapsulated within hydrogels have recently been reported to modify their local surroundings by secreting and assembling proteins pericellularly, which in turn impacts their fate. As a result, methods to characterise and visualise the secreted matrix are becoming increasingly important in the development of regenerative therapies and in understanding cell behaviour within 3D matrices. Here, by combining fluorescent non-canonical amino acid tagging with confocal Raman spectral imaging, we aimed to create 3D maps of human mesenchymal stromal cells (hMSC) and their secreted matrix when embedded within hydrogels. To demonstrate the value of our combined technique in a tissue engineering context, we cultured hMSC in Dopa-modified hyaluronic acid-based hydrogels and treated cultures with the 2-oxyglutarate analogue dimethyloxalyglycine (DMOG), which mimics the cellular effects of physiological hypoxia and can both promote the chondrogenic differentiation of progenitor cells and enhance cartilage-like matrix formation. Quantitative analyses of the distribution of newly synthesised proteins combined with principal components analyses of Raman spectra showed that DMOG prompted encapsulated cells to secrete more protein pericellularly than did untreated controls. Our findings demonstrate that it is possible to visualise both the 3D secreted matrix and cellular contents using simple, unbiased, inexpensive techniques, providing complementary information on cells and their secreted matrix when encapsulated within 3D hydrogels.
Introduction
Modifiable, water-swollen polymer networks called hydrogels are widely used in tissue engineering and fundamental biological studies as mimics of the native extracellular matrix (ECM). Hydrogels are used to both form potentially regenerative tissues and understand how physical and biological properties of cells’ 3D surroundings impact processes such as stem cell fate specification.1,2 Hydrogels’ physical and biological properties are often tuned to direct cellular behaviours under the premise that encapsulated cells will detect such cues and respond in a reproducible and predictable manner.3 However, recent reports have shown that encapsulated cells are not passive sensors of hydrogel cues. Rather, human mesenchymal stromal cells (hMSC) encapsulated within both poly(ethylene glycol) (PEG)- and hyaluronic acid (HA)-based hydrogels quickly modify their local surroundings by assembling secreted proteins around themselves.4–6 Over time, this secreted pericellular matrix (PCM) masks and overrides cues provided by the hydrogel, providing its own instruction to cells. As a result, the secreted PCM is emerging as a critical regulator of cell–biomaterial interactions that can be harnessed to direct cellular behaviours in both tissue/disease modelling and regenerative medicine.7 Moreover, efforts to engineer tissues such and bone and cartilage are highly dependent on cells’ ability to produce an appropriate and extensive ECM. Therefore, methods to characterise and visualise the secreted PCM within 3D hydrogels are likely to prove crucial in developing regenerative therapies and understanding cell behaviour in 3D models of both health and disease.
Immunostaining methods, first described in the mid-twentieth century,8 have revolutionised biology by allowing researchers to visualise the location of specific proteins within tissues. However, such methods require prior knowledge of the proteins one expects to detect to choose appropriate antibodies, and preparation methods can be time-consuming. Therefore, when studying the complex secreted proteome of a mammalian cell, a priori antibody selection may lead to “confirmation bias” and potentially limit the identification of novel cellular regulators within the PCM. Proteomics techniques using mass spectrometry take the opposite approach, as they are capable of identifying all of the proteins in a given sample;9 however, standard techniques often do not provide positional information, which precludes an understanding of how secreted proteins assemble around a cell in 3D and may thus signal back to it. Desorption electrospray ionisation (DESI) combined with mass spectrometry10 and emerging single cell proteomics techniques11 may one day provide the positional information of immunostaining techniques with the unbiased specificity of proteomics; however, such approaches are limited to a small number of labs with specialised equipment and are currently expensive to implement. Therefore, alternative techniques or a combination of techniques that can provide information about the distribution and composition of the secreted proteome using inexpensive and accessible tools are required by the research community.
Fluorescent non-canonical amino acid tagging (FUNCAT) techniques, which replace a canonical amino acid in cell culture medium with an analogue containing a bio-orthogonal functional group to which a fluorophore can be “clicked”,12 are available as highly reliable kits. FUNCAT allows for 3D visualisation of the spatial distribution of proteins that have been translated whilst cultures were treated with the amino acid analogue, allowing visualisation of the secreted PCM with minimal further manipulation. However, FUNCAT labels all proteins that incorporate the amino acid analogue and provides no information on non-proteinaceous components of either the cells (lipids, nucleic acids, etc.) or other components of the ECM (sugars, e.g.). Raman spectroscopy is an unsupervised, label-free technique based on the inelastic scattering of monochromatic light that has been used extensively to identify the biochemical fingerprint of cells,13 tissues14,15 and ECM formed by cells in culture.16–19 Raman spectroscopy can be utilised with no additional labelling as a 3D confocal imaging technique, and whilst not capable of specifically identifying biological species, can distinguish between proteins, lipids, and nucleic acids, amongst other biologics. Raman spectroscopy also requires little to no sample preparation20 and is relatively accessible to many laboratories.
HA-based hydrogels are known to promote the chondrogenesis of hMSC,21 which is important as ECM formation is central in regenerative strategies for cartilage repair. We have reported previously on HA-based hydrogels that cross-link via a visible light-mediated reaction between methacrylate (MA) and di-thiol PEG in the presence of eosin-Y (Fig. 1).22 These hydrogels also contain Dopa groups, the active component of the mussel foot protein, which is well known for its adhesive properties.23 When HA is modified with both MA and Dopa, the Dopa moieties do not participate in cross-linking, but rather function as a scavenging group, reducing kinetic chain formation.22 In this system, the Dopa in its reduced form can later oxidise, allowing for biological interactions. Hydrogels containing the Dopa modification (MA–HA–Dopa) adhere to cartilage tissue and support the viability of encapsulated hMSC, likely by binding serum proteins to provide sites for integrin-mediated interactions.22
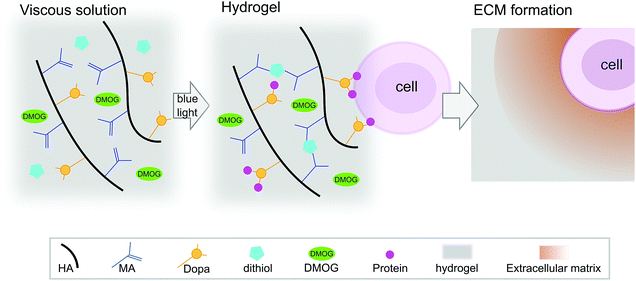 |
| Fig. 1 Hydrogel reaction scheme and cell encapsulation. Hyaluronic acid (HA) was modified with both methacrylate (MA) and 3,4-dihydroxyphenylalanine (Dopa) groups. DMOG and/or human marrow stromal cells (hMSC) were then added to viscous precursor solutions and MA–HA–Dopa hydrogels formed using a standard surgical light by reacting dithiol PEG with the MA moieties on the HA. Upon oxidation of the Dopa, hMSC attach to hydrogels (likely by interacting with serum proteins that bind to the Dopa groups) and survive when encapsulated within them. These hydrogels have previously been shown to support hMSC viability and allow for pericellular matrix formation.22 | |
Hypoxia is known to drive chondrogenesis in vitro and in vivo24,25 and plays important roles in both the secretion and maintenance of the cartilage ECM.26,27 Therefore, means to harness hypoxia for cartilage tissue engineering are widely pursued.28,29 The 2-oxyglutarate analogue dimethyloxalyglycine (DMOG) is able to ectopically stabilise the 1α component of the hypoxia inducible factor (HIF) complex at normoxia and thus stimulate cellular responses that mimic those elicited by low oxygen pressure.30 DMOG upregulates the expression of HIF target genes and prompts hMSC to adopt a more chondrogenic transcription profile compared that in hMSC cultured under basal conditions.31 Moreover, Sathy et al. have demonstrated that long-term release of DMOG from hydrogels stimulates encapsulated MSC to create a cartilage-like proteoglycan-rich matrix.32 Here, we aimed to use MA–HA–Dopa hydrogels to demonstrate that by combining Raman spectroscopy and FUNCAT, we could visualise and characterise an expected DMOG-driven increase in the secretion of PCM by encapsulated hMSC, in a cartilage tissue engineering context. Our findings show that it is possible to visualise both the 3D secreted matrix, as well as cellular components using simple, unbiased, and inexpensive techniques.
Materials and methods
Hydrogel synthesis
Double modified HA monomers (methacrylate, MA; 3,4-dihydroxyphenylalanine, Dopa) were synthesised as previously described.22 Firstly, HA (100–150 kDa, LifeCore Biomedical) was reacted with methacrylic anhydride (20-fold excess relative to primary HA hydroxyl groups, Santa Cruz Biotechnology) in an aqueous environment (pH 8). The reaction mixture was incubated at room temperature (RT) for 4 h and its pH adjusted by addition of NaOH (pH 8). The mixture was then cooled to 4 °C and allowed to proceed for a further 20 h. The resulting MA–HA monomer was then precipitated in ice-cold ethanol (5-fold excess) and the product collected by centrifuging at 2025 g for 15 min. The resulting pellet was then washed twice with ethanol, dried under vacuum, and resuspended in dH2O (pH 5). MA–HA was dopaminated via a carbodiimide coupling (EDC/NHS, from Sigma-Aldrich) by reacting MA–HA with dopamine hydrochloride (Sigma-Aldrich) for 4 h (dH2O, pH 5) in the dark. The resulting MA–HA–Dopa monomer was purified via acidic dialysis (3.5 kDa MWCO tubing in pH 5, Sigma-Aldrich) for 48 h, followed by pH neutral dialysis (dH2O, pH 7) for 24 h. The final product was lyophilised and stored at −20 °C, protected from light.
The degree of modification was determined by proton NMR (Avance 400 MHz NMR spectrometer, Bruker), and was defined as the number of substituents per 100 hydroxyl groups in HA by correlating the respective signals to the peak at δ 2.1 ppm (C(
O)CH3 in HA). Degree of methacrylation was quantified by integrating the signals at δ 5.68 and 6.13 ppm (C
CH2 of the conjugated methacrylate). Degree of dopamination was determined by integrating the signals at δ 6.5–7.2 ppm (ortho and meta coupling position of the catechol ring).
Cell culture
Human mesenchymal stromal cells (hMSC) were provided by the Imperial College Healthcare Tissue Bank (HTA license 12275), which is part of the National Institute for Health Research Biomedical Research Centre at Imperial College London (12/WA/0196). Samples used for these experiments were issued from sub-collection R16052. hMSC were sourced from iliac crest bone marrow aspirates (healthy paediatric donors with informed consent of their parents). Cells were cultured in αMEM with FBS (10%, Gibco Life Technologies) and rhFGF (5 ng mL−1, R&D Systems) under standard conditions and passaged at ∼80% confluency up to passage 7.
Cell encapsulation and culture
MA–HA–Dopa was sterilised with 54 kGy gamma irradiation. Dithiol-PEG (65 mM, 1 kDa, from JenKem Technology) and eosin-Y (154 μM, Fisher Scientific) in cell culture media, were sterilised using 0.22 μm syringe filters and mixed with the monomer (3% w/v, final concentration) on an Eppendorf Thermomixer at 37 °C. These hydrogels form in <2 min and have a G′ of ∼1000 Pa.22 hMSC (1 × 106 cells mL−1) were added to the dissolved monomer and homogenised on an Eppendorf Thermomixer for 15 min at 37 °C. Hydrogels were formed using a step-growth reaction, triggered by blue light exposure (400–500 nm, 500–600 mW cm−2, 4 min, S1500 OmniCure Light System, Lumen Dynamics). Hydrogels containing cells were incubated in chondrogenic induction medium composed of high glucose Dulbecco's modified Eagle's medium (DMEM, phenol-free, glutamine-free, methionine-free, high-glucose, Gibco Life Technologies) with L-glutamine (2 mM, Fisher Scientific), dexamethasone (100 nM, Sigma-Aldrich), insulin–transferrin–selenium solution (ITS, 1%, Fisher Scientific), antibiotic/antimycotic solution (1%, Gibco Life Technologies), ascorbic acid-2-phosphate (50 μg mL−1, Sigma-Aldrich), L-proline (40 μg mL−1, Sigma-Aldrich) and TGF-β3 (10 ng mL−1, Peprotech). Groups treated with DMOG (Sigma-Aldrich) received 200 μM of the drug with the initial cell culture media. Media was changed every 3–4 days.
Imaging of secreted proteins and analysis
To visualise newly synthesised peptides and proteins, a Click-iT HPG Alexa Fluor 594 kit (Sigma-Aldrich) was used, following the manufacturer's instructions. In short, the methionine-analogue L-homopropargylglycine (HPG) was added to methionine-free cell culture media. After incubation of 21 days, hydrogels were rinsed in dH2O and permeabilised with 0.5% TritonX in PBS for 15 min at RT. Next, Alexa Fluor 594 azide was added for 30 min at RT to allow the azide group to bind to the modified alkyne group on the HPG via a copper-catalysed click-reaction. Nuclei were stained with an HSC NuclearMask (Fisher Scientific) for 30 min at RT. Samples were rinsed thoroughly in PBS, transferred onto a glass slide, cover slipped and imaged (DM16000 confocal laser scanning microscope, Leica). Laser intensity, detector gain, and acquisition settings were all kept constant between samples to facilitate quantitative comparisons. From each group, 8 or 9 cells were selected at random, but only if they had a clearly visible nucleus and a clearly outlined membrane. Images of the cell membrane, identified in differential interference contrast images (Fig. S1, ESI†), collected using a 488 nm laser and a 40× oil immersion objective with a 1.25 numerical aperture, were overlaid with fluorescence channels. The secreted matrix was analysed by generating 20 random lines extending radially from the membrane of each cell, resulting in a total of 160 or 180 lines per condition. Protein density, as a function of fluorescence intensity of the HPG positive signal was measured by analysing the brightness (arbitrary units, a.u.) of each pixel along the radii.6,33,34 In this way, mean pixel intensity was calculated at every 0.082 μm step from the cell membrane and these values summed to calculate fluorescence intensity on a per cell basis. The distance at which secreted matrix extended from the cell membrane was calculated likewise on a per cell basis by identifying the distance in radii at which fluorescence signal was no different than background.
Raman spectral imaging
Raman imaging was performed on an in-house built Raman confocal micro-spectroscope. The 532 nm laser (Laser Quantum, Stockport, UK) was coupled to the microscope body (CSE2100, CEA1400, CSN100, ZMF1030, Thorlabs Inc., Ely, UK) using a 125 μm cladding single mode fibre (Laser Quantum, Stockport, UK) and fibre collimator (Thorlabs Inc., Ely, UK). The collimated laser beam was filtered through a laser line filter (Semrock, Rochester, NY, USA) to remove unwanted spectral emissions before being reflected on a dichroic mirror (Semrock, Rochester, NY, USA) and directed to the 60×/1.0NA water immersion objective (CFI Apo NIR 60× W, 60×/1.0NA, Nikon). The Raman scattered light collected by the objective was directed back through the dichroic mirror and filtered through a long pass filter (Semrock, Rochester, NY, USA) to remove Rayleigh scattered light before entering the fibre coupler (Thorlabs Inc., Ely, UK) and 50 μm core (Thorlabs Inc., Ely, UK) through a low OH optical fibre (Thorlabs Inc., Ely, UK) acting as a pinhole to provide confocality. The Raman signal was directed to the holographic spectrometer (HS-HSG-532-LF, Andor HoloSpec, Belfast, UK) and detected on a thermoelectrically cooled back-illuminated charge coupled device (CCD, PIXIS100B, Teledyne Princeton Instruments, Birmingham, UK) spanning the spectral range from 0 to 2500 cm−1. For 2D and 3D images, single cells were chosen at random, but only if they had a clearly visible nucleus and a clearly outlined membrane, and were raster scanned using a piezoelectric stage (Physik Instrumente GmbH & CoKG, Karlsruhe, DE) with a 500 nm step size and 0.3–0.8 s acquisition time. For 3D imaging, a piezo objective mount (Thorlabs Inc., Ely, UK) was utilised and 2D images were collected at parallel planes every 5 μm spanning the entire cell.
Raman spectral analysis
Spectral processing was performed using in-house written algorithms in the Matlab programming environment (version 2019, MathWorks Inc.). Pre-processing of Raman spectra involved baseline correction using asymmetric least squares smoothing,35 cosmic ray removal36 and spectral smoothing by a second order Savitzky–Golay filter with a 9-point window in the spectral range 750–1760 cm−1. All individual spectra were normalised using the Euclidean vector norm to remove instrument effects. The spectra from all Raman 2D and 3D hyperspectral images were unfolded into a single matrix comprising more than 160
000 spectra. All spectra were subject to background correction using extended multiplicative signal correction with spectral interference subtraction (EMSC-SIS).37,38 Average background (water) and cell spectra identified by k-means clustering of the entire dataset were used as input to EMSC-SIS. Spectra corresponding to background were removed and the N-FINDR algorithm39 was used to unmix the remaining Raman spectra in the dataset to identify pseudo pure biochemical components (endmembers). We chose the number of components (4) that maximised the number of biochemically meaningful spectra through peak assignment and correlation with literature.40,41 The pixels in the hyperspectral images were assigned 4 abundance values from 0 to 1 according to their spectral similarities with the endmembers using a non-negative alternating least squares algorithm. Each of the 4 abundance value matrices for each image were min–max normalised and refolded back into the original shape. The images were plotted by assigning a false colour to each endmember channel.
Relative area quantification for spectral endmembers in an image was performed by counting the number of pixels with an abundance value larger than a chosen threshold and calculating the percentage to total area (in pixels) of the cells. A threshold larger than the average abundance value of all images was chosen for our application.38 Endmembers representing proteinaceous content were combined in a single relative area quantification by the logical disjunction of pixel abundance values larger than the threshold.
To investigate the extracellular regions of the Raman images for protein, all baseline corrected spectra classified as background were subject to principal component analysis (PCA) using mean-centring. The scores of principal components (PC) with loading vectors showing protein-like spectral features were used to produce pseudo spectral Raman images of the background.42 The PCM was then evaluated by generating 40 lines in each image extending radially away from cells. The scores of each pixel along the radii were used to plot the average protein distribution within the same condition as a function of distance.
Statistical analyses
Statistical analyses were performed using Prism7.04 (GraphPad, USA). A Mann–Whitney test (two-tailed) was used for comparisons between 2 groups. Error bars show standard deviations (S.D.).
Results and discussion
We encapsulated hMSC within MA–HA–Dopa hydrogels (degree of HA modification: 38% methacrylation, 20% dopamination) and either treated them with DMOG or cultured them under basal conditions. We first visualised the formation of new proteins using FUNCAT (Fig. 2A). After 21 days in culture, newly synthesised proteins were visible in the pericellular space immediately surrounding cells under both conditions (Fig. 2B and C). To better understand the distribution and density of the secreted matrix, we created fluorescence intensity profiles of the HPG signal in lines extending radially from the plasma membrane of encapsulated cells and then plotted mean fluorescence intensity as a function of distance in each condition (Fig. 2D and E). Our observations show that PCM formation is concentrated immediately around the cell membrane and extends similar distances from the cell membrane both in the absence and presence of DMOG (Fig. 2F). This is in keeping with previous reports that the secreted matrix pushes the hydrogel away from the cell membrane, and that the thickness of the secreted matrix around chondrocytes encapsulated within alginate and HA-based hydrogels is controlled by polymer density.33,34
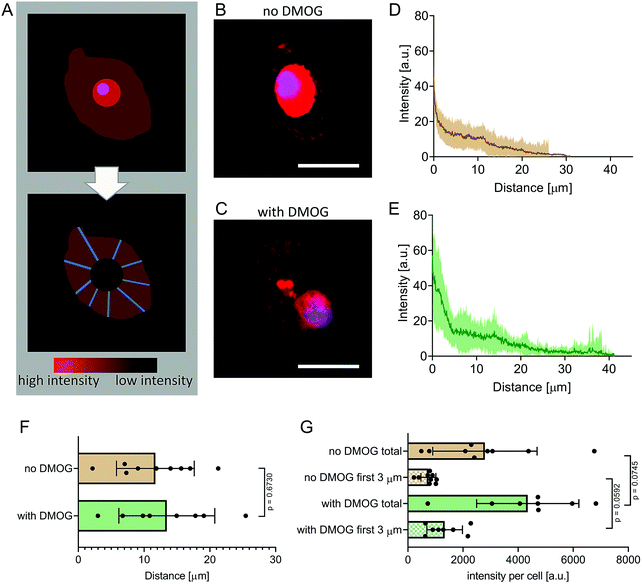 |
| Fig. 2 Fluorescent non-canonical amino acid tagging to visualise newly synthesised proteins. (A) Schematic detailing image processing strategy. Intracellular signal from synthesised proteins was removed by overlapping fluorescence images with differential interference contrast (DIC) images to identify cell membranes. Extracellular signal intensity was then quantified in line plots (n = 20 lines per cell, 8 cells per DMOG-group, 9 cells per no-DMOG-group) and radial intensity profiles determined. (B) Representative fluorescence image of an encapsulated hMSC cultured without DMOG for 21 days. Scale bar = 40 μm. (C) Representative fluorescence image of an encapsulated hMSC cultured with DMOG for 21 days. Scale bar = 40 μm. (D and E) Intensity plots as a function of distance from the cell membrane in the DMOG-treated group compared to those cultured under basal conditions (means ± S.D.). (F) Mean distance of HPG signal per cell (n = 8 or 9, Mann–Whitney test, p = 0.6730). (G) Mean fluorescence intensity of the HPG signal per cell was higher in cells treated with DMOG both within the first 3 μm from the cell membrane (p = 0.0592) and when total signal was quantified (p = 0.0745) (both Mann–Whitney test). | |
We next quantified signal intensity and found that DMOG treatment prompted hMSC to secrete more protein when compared to those cultured under basal conditions. Indeed, mean fluorescence intensity of the HPG signal per cell was higher in cells treated with DMOG both within the first 3 μm from the cell membrane (p = 0.0592) and when total signal was quantified (p = 0.0745) (Fig. 2G). Although DMOG is widely used to “mimic” hypoxia by regulating the activity of two hydroxylases that mediate HIF-1α's intracellular degradation, it also has the potential to impact other hydroxylases, including those involved in collagen biosynthesis. Previous work has shown that by limiting timing/dosing of DMOG, its chondrogenic effect on MSC can be harnessed without negatively impacting cartilage-like matrix production.31,32 Our results suggest that at the doses and timings used in this study, DMOG did not negatively impact matrix secretion, but rather enhanced it in the pericellular region.
FUNCAT provides valuable visual confirmation of proteins newly synthesised and secreted by cells encapsulated within 3D hydrogels. However, it cannot identify specific proteins, and different cellular components are not distinguishable. Therefore, we next aimed to visualise hMSC and their secreted matrix when encapsulated within MA–HA–Dopa hydrogels using confocal Raman micro-spectroscopy. To accomplish this, we collected hyperspectral images of encapsulated cells at a 0.5 μm spatial resolution. Multivariate image processing of the entire hyperspectral dataset produced 4 pseudo pure spectra (endmembers) describing the biochemical composition of the cells. We then reconstructed cell images by assigning abundance values to each pixel according to their spectral similarity to the endmembers (Fig. 3A and Movie 1, ESI†). The extracted endmembers (Fig. 3B) showed typical protein Raman bands for amide I (∼1654 cm−1), amide III (∼1255 cm−1) and phenylalanine (1034 and 1003 cm−1) in the cytoplasm as well as nucleus. The spectra representing the proteinaceous cytoplasm contained 2 endmembers, which we designated as cyan and blue. The cyan endmember had a broad and intense peak in the amide III region with high intensity in the range 1250–1300 cm−1 relative to the amide I band. Previous work has shown that such spectral features are often associated with the α-helix secondary structure in proteins such as collagen.41 The blue endmember resembled a more typical cytoplasmic spectrum, similar to those reported in the literature,38,43,44 with a lower intensity amide III region (1250–1300 cm−1) relative to the amide I band. The blue endmember also exhibited a shoulder around 1610 cm−1 usually assigned to cytosine, tyrosine and tryptophan. The endmember that identified nucleus-associated spectra (red) contained bands indicative of DNA and RNA around 1340 cm−1 (nucleic acid mode) and 828 cm−1 representing the O–P–O stretch.40 The last endmember (yellow) contained spectral features associated with lipids around 1130 cm−1, 1303 cm−1 and 1674 cm−1.41
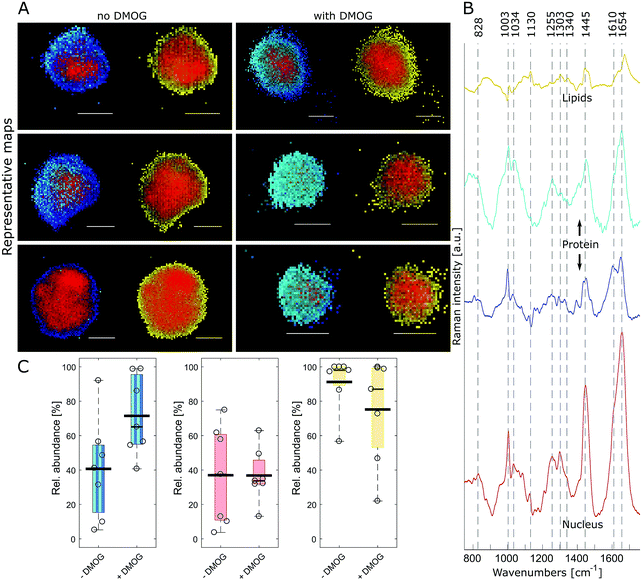 |
| Fig. 3 Raman imaging and quantitative analysis of encapsulated hMSC. (A) False colour reconstructions of 3 representative cells per condition created by spectral unmixing of Raman images showing hMSC cultured without and with DMOG. Pixels are coloured according to abundance of corresponding Raman spectral endmembers of intracellular components in (B). Image pairs show the same cell depicting either proteins (left: cyan, blue) with the nucleus (red) or lipids (right: yellow) with the nucleus. Scale bar = 10 μm. (C) Relative area quantification and comparison of the intracellular components (protein: cyan, blue; nucleus: red; lipids: yellow) for cells cultured with (n = 7) and without DMOG (n = 7). The proteinaceous content as identified by the cyan and blue endmember was larger in hMSC exposed to DMOG compared to controls (Mann–Whitney test, p = 0.053). Box plots show the median (thin black line) and 25/75th percentiles, and whiskers the maximum and minimum values in the dataset. The thick black line shows the mean and individual datapoints are indicated as circles. | |
Having identified the biochemical content of encapsulated cells, we next asked if there were any differences between cells cultured with and without DMOG. To address this, we used relative area quantification in which the number of pixels associated with the respective endmembers are counted and related to the total number of pixels in each cell (Fig. 3C). Quantification of red and yellow endmembers was similar between DMOG and controls. To investigate the total amount of intracellular protein, we performed a combined area quantification of the cyan and blue endmembers. By counting pixels with an abundance value larger than the threshold for one or the other endmember, we found that the proteinaceous content was larger in hMSC treated with DMOG compared to controls (Mann–Whitney test, p = 0.053).
To investigate the pericellular regions in the Raman images, we adapted the analysis used for extracting protein distributions from FUNCAT images. We based the analysis on principal components analysis (PCA) to accommodate for the low signal to noise ratio of the background Raman spectra surrounding the cells, enabling meaningful spectral patterns to be distinguished from random noise. The first three PC loading vectors contained interpretable spectral information with features corresponding to water (PC1, 1630 cm−1), water + hydrogel (PC2, 1630 cm−1, 1410 cm−1 COO−, 948 cm−1 C–O–C)45,46 and proteinaceous content (PC3, 1660 cm−1 amide I, 1445 cm−1 CH2, 1255 cm−1 amide III, 1004 cm−1 phenylalanine) (Fig. 4B). Images were then reconstructed by plotting the scores of PC3 at each pixel, revealing the distribution of the protein-like content surrounding the cells. Positive PC3 scores correspond to spectral observations containing the most protein-like features. Mirroring the methods used for FUNCAT image analysis, PC3 scores as a function of distance from cells were extracted along 40 lines extending radially from the cell membrane (Fig. 4A). We observed a somewhat higher average PC3 score distribution (although not significant, Mann–Whitney test, p = 0.596) for cells cultured with DMOG compared to the cells cultured without DMOG within the immediate 3 μm around the cells, in which the average PC3 scores were positive for both conditions (Fig. 4C).
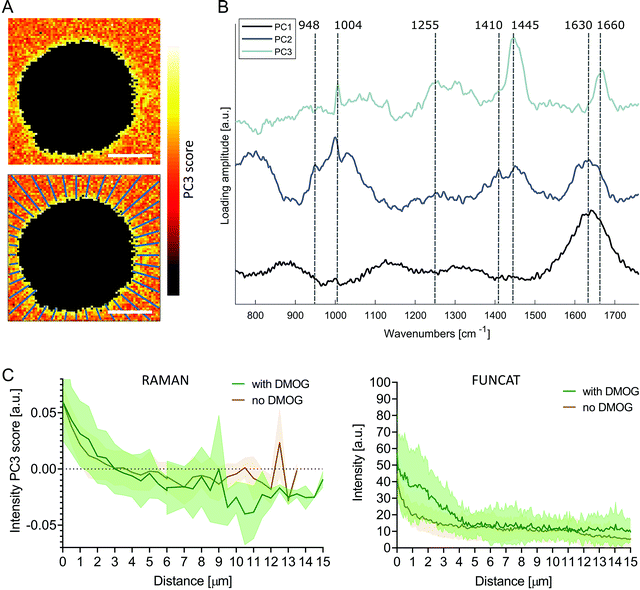 |
| Fig. 4 Principal component analysis of pericellular region in Raman images. (A) Pseudo spectral images of pericellular region (scale bars = 10 μm) using scores of principal component 3 (PC3) with corresponding loading vector in (B). The first three loading vectors show spectral features corresponding to water (PC1), water + hydrogel (PC2) and protein (PC3). PC1 explained 22% of the variance, PC2 2% and PC3 2%. (C) Average (solid line) ± standard deviation (shaded area) PC3 score distribution as function of distance from cell extracted along 40 lines extending radially as shown in (A) for cells cultured with (green, n = 7) and without (brown, n = 7) DMOG (left). Protein distribution profiles extracted from FUNCAT images are shown for comparison (right; detailed view from combined Fig. 2B and C). | |
Conclusions
Here we used a combination of FUNCAT and Raman spectral imaging to provide unbiased in situ visualisation of both cells within 3D hydrogels and their secreted matrix. Our analysis was able to confirm that the HIF mimetic DMOG could drive hMSC to produce/retain more proteinaceous matrix in their pericellular space when encapsulated within 3D hydrogels when compared to cells cultured under standard chondrogenic conditions. Standard methods to evaluate matrix synthesis within 3D hydrogels can be time-consuming and subject to confirmation bias. These two complementary techniques allow for swift analysis with little preparation time as samples can be ready for data acquisition and analysis in less than 3 h.
Many proteins contain methionine, thus HPG is incorporated into the majority of secreted proteins using the non-canonical amino-acid tagging technique.33 However, non-proteinaceous cellular and matrix components are not identified with this approach, but can be detected using Raman spectroscopy. Moreover, in addition to identifying lipids, proteins and nucleic acids, Raman spectral imaging can often be used to recognise molecules more specifically.47,48 For example, Raman-based identification of cholesterol in precursor cells could potentially be used to identify MSC that are differentiating down the chondrogenic lineage.47,48 Our findings suggest that FUNCAT analysis is more sensitive and might detect lower quantities of proteins than Raman imaging; however, Raman's ability to distinguish between biological species can provide complementary information, which may be particularly important for tissue engineering applications.
Conflicts of interest
The authors declare no conflicts of interest.
Acknowledgements
CS acknowledges a Diane Trebble PhD studentship. The authors are grateful for funding from the Rosetrees Trust and Independent Research Fund Denmark (DFF-7017-00163). EG and CS acknowledge the UK Regenerative Medicine Platform “Acellular/Smart Materials-3D Architecture”.
References
- S. Khetan, M. Guvendiren, W. R. Legant, D. M. Cohen, C. S. Chen and J. A. Burdick, Nat. Mater., 2013, 12, 458–465 CrossRef CAS.
- N. Huebsch, P. R. Arany, A. S. Mao, D. Shvartsman, O. A. Ali, S. A. Bencherif, J. Rivera-Feliciano and D. J. Mooney, Nat. Mater., 2010, 9, 518–526 CrossRef CAS.
- D. A. Foyt, M. D. A. Norman, T. T. L. Yu and E. Gentleman, Adv. Healthcare Mater., 2018, 7, e1700939 CrossRef.
- U. Blache, Q. Vallmajo-Martin, E. R. Horton, J. Guerrero, V. Djonov, A. Scherberich, J. T. Erler, I. Martin, J. G. Snedeker, V. Milleret and M. Ehrbar, EMBO Rep., 2018, 19, e45964 CrossRef.
- S. A. Ferreira, M. S. Motwani, P. A. Faull, A. J. Seymour, T. T. L. Yu, M. Enayati, D. K. Taheem, C. Salzlechner, T. Haghighi, E. M. Kania, O. P. Oommen, T. Ahmed, S. Loaiza, K. Parzych, F. Dazzi, O. P. Varghese, F. Festy, A. E. Grigoriadis, H. W. Auner, A. P. Snijders, L. Bozec and E. Gentleman, Nat. Commun., 2018, 9, 4049 CrossRef.
- C. Loebel, R. L. Mauck and J. A. Burdick, Nat. Mater., 2019, 18, 883–891 CrossRef CAS.
- U. Blache, M. M. Stevens and E. Gentleman, Nat. Biomed. Eng., 2020, 4, 357–363 CrossRef.
- A. H. Coons, H. J. Creech and R. N. Jones, Exp. Biol. Med., 1941, 47, 200–202 CrossRef CAS.
- S. A. Ferreira, P. A. Faull, A. J. Seymour, T. T. L. Yu, S. Loaiza, H. W. Auner, A. P. Snijders and E. Gentleman, Biomaterials, 2018, 176, 13–23 CrossRef CAS.
- J. M. Wiseman, D. R. Ifa, Y. Zhu, C. B. Kissinger, N. E. Manicke, P. T. Kissinger and R. G. Cooks, Proc. Natl. Acad. Sci. U. S. A., 2008, 105, 18120–18125 CrossRef.
- V. Marx, Nat. Methods, 2019, 16, 809–812 CrossRef CAS.
- C. M. McLeod and R. L. Mauck, Sci. Rep., 2016, 6, 38852 CrossRef CAS.
- H. Autefage, E. Gentleman, E. Littmann, M. A. Hedegaard, T. Von Erlach, M. O'Donnell, F. R. Burden, D. A. Winkler and M. M. Stevens, Proc. Natl. Acad. Sci. U. S. A., 2015, 112, 4280–4285 CrossRef CAS.
- C. P. Tarnowski, M. A. Ignelzi, Jr. and M. D. Morris, J. Bone Miner. Res., 2002, 17, 1118–1126 CrossRef.
- H. Autefage, F. Allen, H. M. Tang, C. Kallepitis, E. Gentleman, N. Reznikov, K. Nitiputri, A. Nommeots-Nomm, M. D. O'Donnell, C. Lange, B. M. Seidt, T. B. Kim, A. K. Solanki, F. Tallia, G. Young, P. D. Lee, B. F. Pierce, W. Wagermaier, P. Fratzl, A. Goodship, J. R. Jones, G. Blunn and M. M. Stevens, Biomaterials, 2019, 209, 152–162 CrossRef CAS.
- S. Loaiza, S. A. Ferreira, T. M. Chinn, A. Kirby, E. Tsolaki, C. Dondi, K. Parzych, A. P. Strange, L. Bozec, S. Bertazzo, M. A. B. Hedegaard, E. Gentleman and H. W. Auner, Biomaterials, 2018, 183, 102–113 CrossRef CAS.
- E. Gentleman, R. J. Swain, N. D. Evans, S. Boonrungsiman, G. Jell, M. D. Ball, T. A. Shean, M. L. Oyen, A. Porter and M. M. Stevens, Nat. Mater., 2009, 8, 763–770 CrossRef CAS.
- A. A. Volponi, E. Gentleman, R. Fatscher, Y. W. Pang, M. M. Gentleman and P. T. Sharpe, J. Dent. Res., 2015, 94, 1568–1574 CrossRef CAS.
- N. D. Evans, R. J. Swain, E. Gentleman, M. M. Gentleman and M. M. Stevens, Eur. Cells Mater., 2012, 24, 211–223 CrossRef CAS.
- K. J. I. Ember, M. A. Hoeve, S. L. McAughtrie, M. S. Bergholt, B. J. Dwyer, M. M. Stevens, K. Faulds, S. J. Forbes and C. J. Campbell, npj Regener. Med., 2017, 2, 12 CrossRef.
- C. Chung, M. Beecham, R. L. Mauck and J. A. Burdick, Biomaterials, 2009, 30, 4287–4296 CrossRef CAS.
- C. Salzlechner, T. Haghighi, I. Huebscher, A. R. Walther, S. Schell, A. Gardner, G. Undt, R. M. P. da Silva, C. A. Dreiss, K. Fan and E. Gentleman, Adv. Healthcare Mater., 2020, e1901134, DOI:10.1002/adhm.201901134.
- P. K. Forooshani and B. P. Lee, J. Polym. Sci., Part A: Polym. Chem., 2017, 55, 9–33 CrossRef.
- E. Schipani, H. E. Ryan, S. Didrickson, T. Kobayashi, M. Knight and R. S. Johnson, Genes Dev., 2001, 15, 2865–2876 CAS.
- S. Provot, D. Zinyk, Y. Gunes, H. M. Kronenberg, R. S. Johnson, M. T. Longaker, A. J. Giaccia and E. Schipani, J. Bone Miner. Res., 2006, 21, S49 Search PubMed.
- S. Strobel, M. Loparic, D. Wendt, A. D. Schenk, C. Candrian, R. L. Lindberg, F. Moldovan, A. Barbero and I. Martin, Arthritis Res. Ther., 2010, 12, R34 CrossRef.
- D. Pfander, T. Cramer, E. Schipani and R. S. Johnson, J. Cell Sci., 2003, 116, 1819–1826 CrossRef CAS.
- D. K. Taheem, G. Jell and E. Gentleman, Tissue Eng., Part B, 2019, 26, 105–115 CrossRef.
- D. A. Foyt, D. K. Taheem, S. A. Ferreira, M. D. A. Norman, J. Petzold, G. Jell, A. E. Grigoriadis and E. Gentleman, Acta Biomater., 2019, 89, 73–83 CrossRef CAS.
- S. J. Bryant and K. S. Anseth, J. Biomed. Mater. Res., Part A, 2003, 64, 70–79 CrossRef.
- D. K. Taheem, D. A. Foyt, S. Loaiza, S. A. Ferreira, D. Ilic, H. W. Auner, A. E. Grigoriadis, G. Jell and E. Gentleman, Stem Cells, 2018, 36, 1380–1392 CrossRef CAS.
- B. N. Sathy, A. Daly, T. Gonzalez-Fernandez, D. Olvera, G. Cunniffe, H. O. McCarthy, N. Dunne, O. Jeon, E. Alsberg, T. L. H. Donahue and D. J. Kelly, Acta Biomater., 2019, 88, 314–324 CrossRef CAS.
- C. M. McLeod and R. L. Mauck, Sci. Rep., 2016, 6, 38852 CrossRef CAS.
- C. Loebel, M. Y. Kwon, C. Wang, L. Han, R. L. Mauck and J. A. Burdick, Adv. Funct. Mater., 2020, 1909802 CrossRef.
- P. H. Eilers, Anal. Chem., 2003, 75, 3631–3636 CrossRef CAS.
- C. J. Behrend, C. P. Tarnowski and M. D. Morris, Appl. Spectrosc., 2002, 56, 1458–1461 CrossRef CAS.
- H. Martens and E. Stark, J. Pharm. Biomed. Anal., 1991, 9, 625–635 CrossRef CAS.
- M. A. B. Hedegaard, M. S. Bergholt and M. M. Stevens, J. Biophotonics, 2016, 9, 542–550 CrossRef CAS.
- M. Hedegaard, C. Matthäus, S. Hassing, C. Krafft, M. Diem and J. Popp, Theor. Chem. Acc., 2011, 130, 1249–1260 Search PubMed.
- Z. Movasaghi, S. Rehman and I. U. Rehman, Appl. Spectrosc. Rev., 2007, 42, 493–541 CrossRef CAS.
- A. Rygula, K. Majzner, K. M. Marzec, A. Kaczor, M. Pilarczyk and M. Baranska, J. Raman Spectrosc., 2013, 44, 1061–1076 CrossRef CAS.
- H. J. Butler, L. Ashton, B. Bird, G. Cinque, K. Curtis, J. Dorney, K. Esmonde-White, N. J. Fullwood, B. Gardner, P. L. Martin-Hirsch, M. J. Walsh, M. R. McAinsh, N. Stone and F. L. Martin, Nat. Protoc., 2016, 11, 664–687 CrossRef CAS.
- K. Klein, A. M. Gigler, T. Aschenbrenner, R. Monetti, W. Bunk, F. Jamitzky, G. Morfill, R. W. Stark and J. Schlegel, Biophys. J., 2012, 102, 360–368 CrossRef CAS.
- C. Matthaus, T. Chernenko, J. A. Newmark, C. M. Warner and M. Diem, Biophys. J., 2007, 93, 668–673 CrossRef CAS.
- R. Bansil, I. V. Yannas and H. E. Stanley, Biochim. Biophys. Acta, 1978, 541, 535–542 CrossRef CAS.
- R. Ellis, E. Green and C. P. Winlove, Connect. Tissue Res., 2009, 50, 29–36 CrossRef.
- A. Villalvilla, R. Gomez, R. Largo and G. Herrero-Beaumont, Int. J. Mol. Sci., 2013, 14, 20793–20808 CrossRef.
- F. R. Maxfield and D. Wustner, Methods Cell Biol., 2012, 108, 367–393 CAS.
Footnotes |
† Electronic supplementary information (ESI) available: Movie 1: Representative 3D Raman image of a hMSC encapsulated in a MA–HA–Dopa hydrogel. Biochemical components of the cell identified using spectral unmixing data analysis highlight the nucleus (red), proteins (blue and cyan) and lipids (yellow). Fig. S1: Representative confocal images of hMSC encapsulated within a hydrogel. (A) Fluorescent non-canonical amino acid tagging (red) and nucleus (blue). (B) Differential interference contrast (DIC) images of the cells shown in A for visualisation of cell outline and (C) merged view of red and DIC channels. See DOI: 10.1039/d0ma00472c |
‡ These authors contributed equally. |
|
This journal is © The Royal Society of Chemistry 2020 |
Click here to see how this site uses Cookies. View our privacy policy here.