A geospatially resolved database of hydraulic fracturing wells for chemical transformation assessment†
Received
1st November 2019
, Accepted 20th January 2020
First published on 10th February 2020
Abstract
Hydraulically fractured wells with horizontal drilling (HDHF) accounted for 69% of all oil and gas wells drilled and 670
000 of the 977
000 producing wells in 2016. However, only 238 flowback and produced water samples have been analyzed to date for specific organic chemicals. To aid the development of predictive tools, we constructed a database combining additive disclosure reports and physicochemical conditions at respective well sites with the goal of making synthesized analyses accessible. As proof-of-concept, we used this database to evaluate transformation pathways through two case studies: (1) a filter-based approach for flagging high-likelihood halogenation sites according to experimental criteria (e.g., for a model compound, cinnamaldehyde) and (2) a semi-quantitative, regionally comparative trihalomethane formation model that leverages an empirically derived equation. Study (1) highlighted 173 wells with high cinnamaldehyde halogenation likelihood based on combined criteria related to subsurface conditions and oxidant additive usage. Study (2) found that trihalomethane formation in certain wells within five specific basins may exceed regulatory limits for drinking water based on reaction-favorable subsurface conditions, albeit with wide uncertainty. While experimentation improves our understanding of subsurface reaction pathways, this database has immediate applications for informing environmental monitors and engineers about potential transformation products in residual fluids, guiding well operators' decisions to avoid unwanted transformations. In the future, we envision more robust components incorporating transformation, transport, toxicity, and other physicochemical parameters to predict subsurface interactions and flowback composition.
Environmental significance
Of the 670 000 active wells that leverage horizontal drilling with hydraulic fracturing in the United States, specific organic chemical measurements for only 238 flowback waters exist in the public domain. We built a geospatially resolved database that unifies well-specific geochemical parameters and chemical additive disclosures to prioritize future measurements, flag regions of concern or critical treatment needs, and avoid unwanted subsurface chemistries a priori. Leveraging existing transformation models that draw on these inputs, we demonstrate this framework's utility to highlight qualitative and quantitative chemical transformation estimates. While an important first step, the vision for this database is as a predictive tool that serves industry and regulatory sectors seeking to improve the safety and sustainability of the natural gas extraction industry.
|
Introduction
Flowback waters from hydraulic fracturing coupled with horizontal drilling (HDHF) comprise residual additives from the injection fluid, geogenic hydrocarbons, and formation derived ions and metals. Depending on the well, estimates show that 10–40% of injected waters return to the surface after fracturing.3 Kondash and Vengosh investigated 10 major shale plays and calculated that an approximate total of 803 billion L (803 million m3; 212 billion gal) flowback water returned to the surface between the early 2000s and 2015.4 From 2011 until 2016, water usage in HDHF increased up to 770% per well.5 While investigating the effects of HDHF on nearby ecosystems and populations, researchers have linked HDHF operations and waste to spill incidents,6 groundwater contamination,7,8 and potential downstream effects on treatment.9,10 Thus, these waters represent important yet complex sources of waste to be managed, treated, or remediated. However, to date, only 238 flowback and produced samples have been analyzed to identify specific organic compounds in the published literature.2
This small number of analyses have included inorganic11–17 and organic2,18–25 components of flowback water, but the vast well-to-well diversity (spatially and temporally13,21) and comparably complex suites of disclosed additives26,27 pose massive hurdles for complete characterization at the field's current pace. In particular, a high degree of uncertainty remains regarding the nature of anthropogenic additives themselves and their capacities to persist or transform in the subsurface.28 While we acknowledge that organic chemical transformations represent only a fraction of the total risk presented by HDHF activities (e.g., induced seismicity,29 fugitive gas emissions,30,31 and increased traffic32,33 and socioeconomic impacts34,35), organic chemical transformations are among the most difficult to anticipate, monitor, and rectify. Industrial ingredient reports have been disclosed through FracFocus,36 a publicly available online database, and by the United States Congress.37 Generally, these chemicals serve a wide array of purposes to optimize hydraulic fracturing operations,26 from (1) controlling the chemical environment within wells to prevent degradation (e.g., biocides and scaling inhibitors) to (2) altering the fluid's physicochemical properties in situ.26,27 Equipped with an understanding of subsurface conditions,38 researchers have simulated the shale well parameter space in laboratory systems to interrogate and characterize subsurface reaction pathways, demonstrating conditions or additives that can either mitigate or encourage transformation. Analyses of cinnamaldehyde (a corrosion inhibitor),39 glutaraldehyde (a biocide),40 and polyacrylamide (a friction reducing polymer)41 have elucidated problematic halogenation, agglomeration, and degradation pathways, respectively. However, without predictive well models these results serve only to explain past detections in the field, ultimately a reactive response to an emerging issue.
To move the field towards predictive mitigation, modeling tools are needed to anticipate transformations ahead of additive usage or wastewater management. So far, a number of modeling tools exist to predict chemical interactions in environmental systems, including EPI Suite42 for structure-based physicochemical parameter estimation, enviPath43 for biotransformation pathway prediction, or the EPA's Chemical Transformation Simulator.44–46 However, these models do not cover all relevant functional groups or transformation types and cannot account for complex mixtures, let alone the parameter ranges of hydraulically fractured shale wells. As researchers continue to investigate the unique chemistry encouraged by the shale well parameter space, evaluation tools are important to relate laboratory simulations back to wells in the field.
In this study, we appended comprehensive well condition data to existing FracFocus disclosure reports, allowing for unified filtering and searching by ingredient disclosures and corresponding subsurface parameters on a well-specific basis. To illustrate its utility, we applied this modified database to two case studies across disclosed well reports: (1) assessing the impact and opportunity for halogenation39 using cinnamaldehyde as an example, and (2) predicting the formation of trihalomethanes47 from disinfection-like halogenation pathways. Both of these studies leveraged qualitative or quantitative criteria prescribed from laboratory results and equations and linked them to FracFocus chemical disclosures. While we note critically that these models cannot be validated en masse currently, their utility in a sample-limited space is clear. By demonstrating regions of high incidence and estimating product formations based on well conditions, we have provided a framework that can inform a sampling strategy for eventual model verification. In addition, the qualitative and quantitative evaluations are useful for environmental monitors and well operators to anticipate or mitigate HDHF additive transformations.
Methods
Database sources and quality control
We compiled data from multiple sources into a unified database including hydraulic fracturing well locations, their disclosed chemicals, subsurface properties, and produced fluid compositions. Hydraulic fracturing well reports were downloaded as comma-separated-variable (CSV) files from FracFocus36 on April 1, 2019 and read into R Studio for collection and analysis. We utilized the United States Geological Survey (USGS) Produced Waters Database48 (V2.3 updated December 8, 2017) for produced water quality data (i.e., Cl−, Br−, and I− concentrations, pH, and dissolved organic carbon (DOC) measurements) and location information (e.g. basin names). Finally, we accessed the “Aggregated Well Data” dataset from the National Geothermal Data System49 (NGDS) for measurements of “bottom hole temperatures” (the temperature of the well at the target formation) for oil and gas wells nationwide. Note that these datasets comprise data collections from all types of wells, not merely HDHF operations, as our intention was to collect a high-resolution map of subsurface geochemical conditions regardless of surface operations.
Prior to integration of any data sets, we performed quality control measures. From the historical FracFocus reporting, we removed all entries without a Chemical Abstracts Service (CAS) registry number, given that the primary purpose of this dataset was chemical usage and identification at reported wells (approximately 3% of entries). Furthermore, due to our case-study focus on some of the most common oxidative breakers (e.g. hypochlorite, chlorite, and persulfate salts) for analysis, we corrected “Ingredient Name” typographical errors linked to these compounds by (1) searching for “breaker” across all report “Purpose” entries (as entered by operators at the time of disclosure), (2) inspecting and manually correcting the resulting list of misspelled species, and then (3) applying those corrections to the entire database. Similarly, we filtered the NGDS database for wells containing valid “bottom hole temperature” entries, omitting those that lacked such entries. While the USGS Produced Waters Database also contained incomplete entries for variables of interest, we did not remove these, as multiple parameters could be utilized with regional spatial resolution.
FracFocus ingredient and well condition matching
To unify the datasets (i.e., append subsurface conditions estimates to each distinct FracFocus well location when such information was absent), we adopted a geospatial averaging approach based on provided coordinates (latitudes and longitudes) or geological basins. Previously reviewed in Sumner & Plata 2018a,38 the USGS and NGDS data exhibit geospatial clusters based on common source geologies and subsurface environments. In certain instances, such as the overlapping Utica and Marcellus shales, latitude and longitude cannot discern shale plays at distinct depths; yet in general geospatial trends exist at the basin level, regardless of variant shale depths. For example, in the Appalachian Basin, the median produced water halide concentrations are generally high (80
000, 692, and 10 mg L−1 Cl−, Br−, and I−, respectively) while the median well's bottom hole temperature is low (37 °C). In contrast, median halide concentrations in the Permian Basin are relatively low (54
000, 230, and 9 mg L−1 Cl−, Br−, and I−, respectively) where median temperatures are high (91 °C). Thus, while conditions certainly vary from well to well, the values of nearby wells and/or the conditions of wells in the same region can provide valuable approximations.
Our approximation approach was parameter dependent due to variability in distribution and density of available data: from (i) the use of exact ingredient disclosure data, to (ii) estimation of certain subsurface conditions values using proximally-located wells, (iii) basin-wide averages when subsurface condition data was most sparse, or (iv) some hybrid of these approaches. For example, within the USGS dataset of 114
943 produced water samples, chloride values are reported in 108
646 of those (94.5%), whereas bromide and iodide values are reported in only 6548 (5.7%) and 3659 (3.2%) samples, respectively. Based on this availability, missing chloride values were added to FracFocus wells by finding the ten closest USGS wells according to calculated coordinate distances (via reported latitudes and longitudes in both datasets) and recording the average of chloride concentrations in those ten nearest wells. This method was also used to add temperature data from the NGDS database. However, this method failed in cases where the ten wells closest to a FracFocus location lacked the necessary data available (e.g., for bromide of iodide concentrations). In these cases, we averaged basin-wide bromide and iodide concentrations data instead. Notably, DOC measurements were rare (303 of USGS wells and primarily from only the Appalachian Basin). For pH measurements, data was relatively complete (86
630 wells, 75.4% of USGS data), so a hybrid approach was taken where basin-wide averaging was employed only when the ten nearest USGS wells lacked sufficient data. For all conditions, standard deviation values were also added to wells based on the respective condition source's data sample (i.e., the ten nearest wells or basin-wide data) to capture variability. Data sources and sample sizes by parameter are summarized in Table 1.
Table 1 Database sizes, contents, and sample sizes for each data type. Data were collected across multiple sources to combine ingredient disclosures and subsurface conditions from respective wells. Chemical ingredient disclosures from all distinct well uploads came from the historical FracFocus36 archives
Reflects valid Chemical Abstract Services (CAS) registry numbers, from original 4 190 137 disclosures.
Water quality and composition measurements were extracted from the United States Geological Society (USGS) Produced Waters Database.48
Well temperature measurements came from the National Geothermal Data System.49
|
FracFocus |
Ingredient report (with CASa) |
Unique well uploads |
n, disclosures |
4 065 763a |
111 221 |
USGSb |
Total |
Cl− |
Br− |
I− |
pH |
DOC |
n, samples |
114 943 |
108 646 |
6548 |
3659 |
86 630 |
307 |
NGDSc |
Total |
Temperature |
|
|
|
n, wells |
964 521 |
133 183 |
|
|
|
Here, we note that FracFocus includes 107
144 unique well uploads from the 670
000 HDHF wells across the United States. The model presented here includes only those wells that are indexed in FracFocus, but could be extended to include all wells where the user provides the chemical additive list for a specific well. Note that the entire available geospatial dataset was presented in Sumner & Plata 2018a.38
Case study 1: cinnamaldehyde transformation criteria filtering
To demonstrate the utility of the dataset for evaluating qualitative transformation predictions (i.e., the likelihood a transformation will occur based on known “rules” or criteria for the transformation related to geochemical and geophysical conditions), we conducted a case study drawing on existing knowledge of cinnamaldehyde halogenation pathways.28,38,39,50 Beginning with a generated list of wells containing cinnamaldehyde disclosures, we set criteria for subsurface conditions reported to encourage cinnamaldehyde halogenation; e.g., high temperature (greater than or equal to 60 °C) and halide concentrations (greater than or equal to 50
000, 500, and 25 mg L−1 chloride, bromide, and iodide, respectively), plus high values of persulfate breaker disclosed (i.e., masses in the top quartile of disclosures for this oxidant). For comparison purposes, we also highlighted wells where the reaction criteria were met except for high oxidant usage. In a sense, this last categorization demonstrates the opportunities for operator decision-making to mitigate transformation irrespective of well conditions.
Case study 2: empirical THM formation modeling
To illustrate the potential for our geospatially resolved dataset to evaluate quantitative predictions, we developed a case study utilizing an empirical equation for trihalomethane (THM) formation to predict potential transformations in FracFocus reported wells. Here, we note explicitly that influences from biodegradation25,51,52 (or biotransformation43,53,54), physical mixing (e.g., relative contribution of injected fluid versus formation water), and chemical transport53,55–58 on quantitative outputs are not considered, but could be added to such models in the future when sufficient data and theory are available. Starting with Chowdhury et al.'s59 thorough review of THM generation models, we selected one model by Hong et al.47 for its basis in natural water and construction around water quality parameters relevant to subsurface hydraulic fracturing fluids; i.e., bromide concentration, pH, DOC, and temperature. Hong et al.47 described an empirical model (eqn (1)) to predict the formations of trihalomethane based on multiple regression analysis of various chlorination conditions applied to raw (untreated) samples from the Dongjiang River in Hong Kong:47 |  | (1) |
where, THMtot is the total concentration of THMs in μg L−1 predicted to form in t hours, Cl2 is the effective chlorination dosage in mg L−1 (adjusted to diatomic chlorine equivalents), DOC is the dissolved organic carbon concentration in mg L−1, T is the temperature in degrees Celsius, and [Br−] is the bromide concentration in mg L−1. Model statistics are those provided by Hong et al.47
For this analysis, we selected wells where sodium hypochlorite or sodium chlorite were disclosed as breakers. As chlorine-based oxidants, these compounds are directly applicable to disinfecting agents60,61 but have also been shown to form THMs when applied as breakers to guar-crosslinked fracturing fluids.50 We focused this analysis around five different regions with the greatest disclosure rates of these breakers for comparison purposes: the Williston Basin, the Permian Basin, the Gulf Coast Basin, the Denver Basin, and the Anadarko Basin. Inputs included the appended pH, temperature, and bromide concentrations for each well location. When choosing a timescale to model, we opted to bridge the differing scales between expected reaction time from the model's source study (6–168 h)47 and the wide-ranging, potentially months-long, shut in times as flowback fluids return to the surface, as consistent with the temporal analyses by Rosenblum et al.13,21 and Stringfellow and Camarillo.62 This factor is further complicated by the existence of “shut-in” times, where the well is closed and water is not allowed to return to the surface for some period of time that can be widely variable. To deal with this uncertainty, we chose a time of 7 days (168 hours) for initial simulations and extended this value to 28 days (672 hours; extrapolated outside the bounds of the original model by Hong et al.) in subsequent sensitivity analyses. Thus, while the time presents the greatest uncertainty and heterogeneity across the operation space, the results are presented such that the relative effect can be gauged by model users (or specified by an operator who knows the actual time constraint). To calculate an effective chlorine dosage, we adjusted disclosed masses of sodium hypochlorite and sodium chlorite by converting according to their molecular weights and oxidation capacities to Cl2 (based on per mole half-reaction stoichiometry):
|  | (2) |
where MW are the molecular weights (70.90, 74.44 and 90.33 g mol
−1 for diatomic chlorine, sodium hypochlorite, and sodium chlorite, respectively) and e
− is the number of electrons transferred in the oxidation half-reaction (2 for Cl
2 and both oxidants). In these basins, DOC measurements were not frequently available and could not be approximated by regional values. Therefore, as a proxy for DOC in this analysis, we used the disclosed concentration of guar (often the dominant organic chemical additive by mass
26,50) and adjusted the value according to the carbon fraction of guar's molecular mass (0.4 based on an average formula of C
6H
12O
6). Carbohydrates such as guar are known trihalomethane precursors,
63 and measurements of guar gum have been made in flowback fluids by measuring total carbohydrate content.
62 Critically, we note the disparity of a disinfection model (
i.e., the chlorination of DOC in water) and the HDHF system (
i.e., the breaking of crosslinked guar). Clearly, there will be quantitative differences, even though both ostensibly generate THMs and respond to the same physicochemical input parameters. Nevertheless, the results are to be taken with great caution and are shown here for illustrative purposes. Additionally, they provide a first approximation of potential THM formation rates in these wells.
To simulate error and incorporate the variability of subsurface conditions at each well in our model, we employed a stochastic approach. Well-by-well, we used the respective appended mean and standard deviation values to construct normal distributions (n = 10) reflecting each well's subsurface condition variability. Values less than zero for which negative numbers have no meaning were forced to zero. From those distributions, input selection was randomized to generate 50 simulated THM calculations for each well. From these 50 values, we calculated a “predicted THM concentration” mean and standard deviation for the well.
Finally, we performed a sensitivity analysis to investigate the influences of each input parameter to modeled THM formation. This analysis was conducted by re-modelling all wells (i.e., using the stochastic approach described above) while fixing all but the test variable model input. For each test input, we incrementally varied the individual parameter across relevant ranges (from their nationwide values) and recorded the subsequent change in predicted THM formation. Specifically, T was varied from 50 to 100 °C by 2 °C, pH was varied from 5.5 to 8.5 by 0.1, Br was varied from 40 to 1000 mg L−1 by 40 mg L−1, t was varied from 12 to 672 hours (four weeks) by 12 hours, and (Cl2/DOC) was varied from 0.02 to 1 by 0.02 (a unitless ratio of diatomic chlorine to carbon concentration).
Results and discussion
HDHF database development and contents
We developed a database unifying FracFocus chemical ingredient disclosure reports (as of April 1, 2019) with produced water and well conditions (e.g., temperature, pH, Cl−, Br−, and I−) from multiple sources. Spanning “Job Start Dates” from April 7, 2010 until March 19, 2019, the downloaded FracFocus well reporting included 4
190
037 chemical disclosures, 4
065
763 of which contained valid CAS registry numbers. Those disclosures included 2880 unique CAS registry numbers and 107
144 distinct well sites (of the 127
781 total on FracFocus). For context, of the 2880 unique CAS numbers, only 1200 appeared on the NIH CACTUS library64 of chemical structures, suggesting that many of the disclosed chemicals are either (1) rare and relatively unstudied, (2) proprietary, or (3) erroneous entries. We note that FracFocus does not cover all well operations, as the Energy Information Agency estimated that around 670
000 hydraulically fractured wells were producing in 2016 (ref. 1) (i.e., FracFocus reports data on approximately 16% of wells). The unified database enables searching across historical FracFocus disclosures and filtering by criteria such as ingredient usage and concentration, water quality parameters, and subsurface well conditions. In this study, we present two case studies to demonstrate the database's utility as a framework for evaluating the existing scope and future opportunity for chemical transformations in the shale well subsurface. Future work would enable one to fully develop and host this database to enable public usage and encourage other researchers in the field to bolster to its predictive capacities with more experimental results and modeling components.
Qualitative criteria filtering: cinnamaldehyde transformation case study
Across all historical FracFocus reporting, cinnamaldehyde appeared in disclosures for 14
175 out of 107
144 distinct wells (13.2%). To isolate wells in which reactive halogen species might form and add to cinnamaldehyde (a probe chemical for other α-unsaturated aldehydes), we screened for wells with subsurface conditions that met or exceeded the experimental “requirements” determined in Sumner and Plata:39 Cl− and Br− concentrations of at least 50
000 and 500 mg L−, respectively, and a temperature of at least 60 °C. Of the total cinnamaldehyde wells, 1726 met those reaction criteria, notably in the Williston (n = 494), Anadarko (220), Permian (513), and Gulf Coast (344) basins (Fig. 1), which tend to have elevated temperatures. These higher temperatures can activate persulfate (disclosed as a “breaker”) to sulfate radical, which is capable of oxidizing concentrated halides to reactive species that can go on to halogenate other additives.39,50,65–67 Thus, we classified co-disclosures of persulfate at the same site (n = 6352) as being of higher halogenation forming potentials. Finally, to isolate the wells with the highest likelihood of halogenation, we highlighted wells with disclosed persulfate usage in the top 25th percentile of reported concentrations and satisfied the other reaction conditions. We note that this persulfate threshold is not a precise requirement for transformation and that persulfate availability is also a function of competing species within the fluid;50,66,68 nevertheless, we viewed this final criterion as a conservative estimate for flagging wells with the greatest opportunities for halogenation.
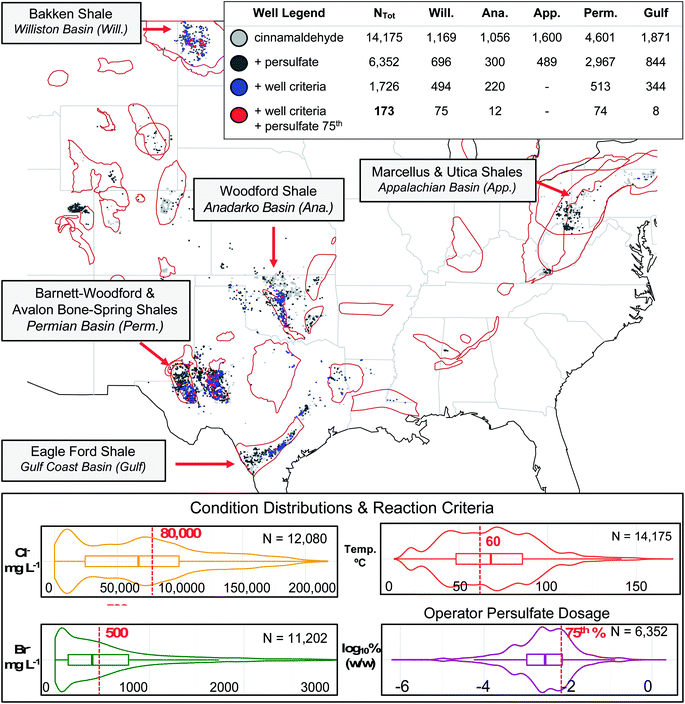 |
| Fig. 1 Hydraulically fractured well sites with cinnamaldehyde ingredient disclosures and other halogenation-pathway promoting criteria. In the inset, horizontal violin plots depict the density distributions of relevant subsurface parameters (i.e., chloride and bromide concentration; temperature) and disclosed ammonium persulfate concentrations. The box plot contains a dark line that indicates the median value of the data, whereas the edges of the box define the ±25th percentile distributions about the median. Amplitudes about the horizontal axis correspond to the relative frequency of disclosures reported at that value. The selected reaction criteria are indicated with a vertical, dashed red line in each inset plot, and N indicates the number of data reflected in the violin plot. Wells meeting the reaction criteria and high breaker dosage were clustered due to (a) geospatial trends dictating the relevant conditions and (b) common breaker usage among shale plays. These trends are demonstrated across the highlighted shale plays/basins: the Williston Basin (Will.), the Anadarko Basin (Ana.), the Appalachian Basin (App.), the Permian Basin (Perm.), and the Gulf Coast Basin (Gulf). | |
Notably, 173 wells passed each of these filters: 75 in the Williston Basin, 12 in the Anadarko Basin, 74 in the Permian Basin, and 8 in the Gulf Coast Basin. Interestingly, in regions like the Appalachian Basin where cinnamaldehyde disclosure was high (n = 1600), zero wells were flagged for the halogenation pathway, as none of them met all of the subsurface conditions needed to promote that reaction due to relatively low well temperatures in that region. This highlights the danger of applying sweeping generalizations to flowback water chemistry. Furthermore, while a large subset of the cinnamaldehyde wells in the Gulf Coast basin met the subsurface condition criteria due to high well temperatures and elevated salinities (344 out of 1871; 18%), just 8 of those 344 (2.3%) wells had sufficient persulfate usage to merit designation as a likely transformation site. In contrast, 75 of the 494 (15%) criteria-matching wells in the Williston Basin and 74 of the 513 (14%) criteria-matching wells in the Permian Basin met the persulfate concentration threshold, indicative of more aggressive breaker usage by operators in the Bakken, Barnett-Woodford, and Avalon Bone-Spring Shales of North Dakota and west Texas.
Ultimately, the undesirable cinnamaldehyde halogenation pathway requires the coincidence of encouraging subsurface conditions along with certain high levels of breaker usage by the well operator. By highlighting wells with halogenation promoting conditions, this database and analysis can caution against aggressive oxidant usage at (or near) those sites of transformation product concern in order to mitigate hazardous product formation. Furthermore, environmental monitors and industrial operators screening for such contaminants can be made aware of the potential occurrence of halogenated species when managing wastewaters or inspecting nearby spill sites based on our conservative well “flagging”, saving time and cost associated with comprehensive analysis. By narrowing the potential transformation sites down from tens-of-thousands to less than two hundred, we have made the scale of such site analyses or sampling programs for verification markedly more feasible. Finally, we identify utility for such a well-specific predictive tool, especially in a highly complex and heterogeneous reaction parameter space, and this tool may help industry avoid a degree of trial-and-error experimentation when optimizing their own oil and gas production as a function of chemical additive choice.
Quantitative modeling and parameter sensitivity: THM formation case study
We predicted concentration distributions of THMs, with error, across shale-active basins (Fig. 2a), by applying an empirical THM formation model47 to wells disclosing sodium chlorite and sodium hypochlorite usage (two common oxidative “breakers”). Additionally, we highlighted the distribution of predicted THMs in the wells of five regions with the highest disclosure of those two oxidants: Williston Basin (n = 2461), the Permian Basin (980), the Gulf Coast Basin (3825), the Denver Basin (2190), and the Anadarko Basin (966) (Fig. 2b). Recall that the error calculations account for both a distribution of input parameter values due to geospatial variability, each with propagated uncertainties, and then random selections of those inputs for stochastic simulations (n = 50). That is, because we are unable to validate the model, we intentionally capture a very large uncertainty window.
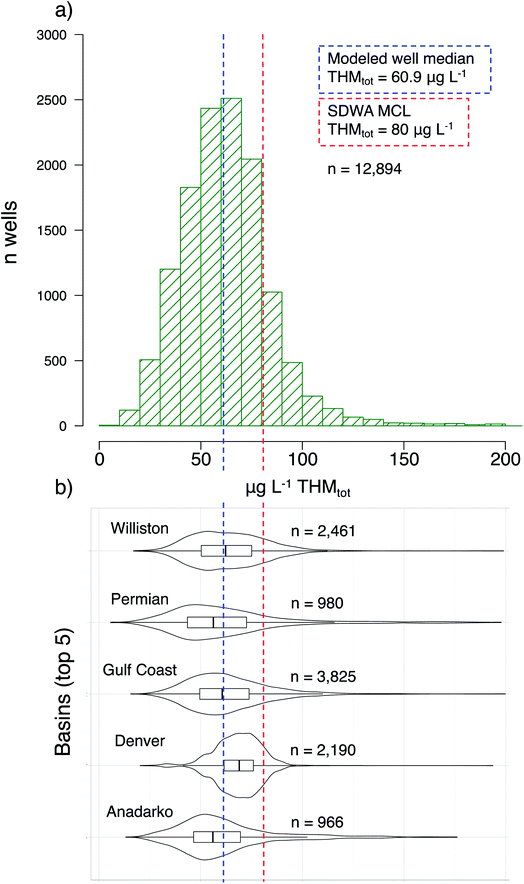 |
| Fig. 2 Predicted well THM concentration distributions for (a) all modeled US wells and (b) wells within five selected basins. Distributions are composed of median predicted values at each well from n = 50 simulations. The five highlighted basins with high sample sizes (Williston, Permian, Gulf Coast, Denver, and Anadarko) were selected to investigate potential differences in magnitudes and ranges regionally. The overall median well value (60.9 μg L−1) and SDWA MCL (80 μg L−1) are overlaid for comparative context. | |
For context, the “Maximum Contaminant Level” (MCL) of total THM as regulated by the Safe Drinking Water Act (SDWA) is 80 μg L−1. Across all 12
894 modeled wells, the median predicted THM concentration was 60.9 μg L−1 (Fig. 2). Within the highlighted basins, the predicted concentrations varied slightly: the Anadarko Basin had a median predicted concentration of 56.3 μg L−1 while the Denver Basin had a median predicted concentration of 69.0 μg L−1. However, even while these two basins held the largest gap between median concentrations, that difference is not significant (p > 0.05) due to their wide tailing distributions. More interestingly, 2243 wells were predicted to exceed the THMtot MCL of 80 μg L−1. Of note across the five highlighted basins: 166 wells in the Anadarko, 298 in the Denver, 666 in the Gulf Coast, 196 in the Permian, and 441 in the Williston Basin had mean THM values that exceeded the MCL. Again, we emphasize that these calculated concentrations are not precise predictors, considering the model was developed in the context of river-water disinfection rather than subsurface oxidation of fracturing fluids. Nonetheless, this model accounts for many of the important chemical parameters and governing principles identified in Sumner and Plata,39 and the application here can provide reasonable first estimates for comparing THM formation risk regionally.
To capture the influence of input value selection on THM prediction outputs and probe the effects of each input to our model, we performed a sensitivity analysis (Fig. 2b). That is, while our model already accounts for variability of input mean, we performed an exercise to determine how sensitive the basin-wide outputs were to changes in those respective input values. For each parameter, we modeled the resulting nation-wide median and standard deviations for the incremental parameter values across their relevant ranges, as determined by existing parameter reviews38 (Fig. 3).
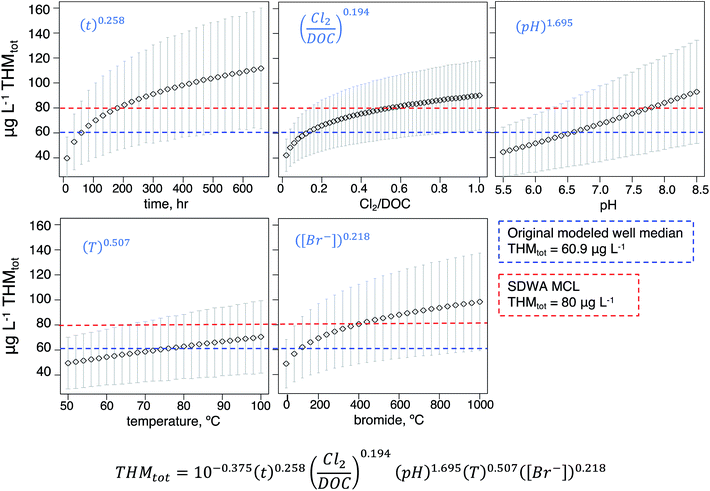 |
| Fig. 3 Sensitivity analysis of THMtot model equation for five input parameters. Time, the Cl2 dose to DOC ratio, pH, temperature, and bromide concentration, were each held constant at values across selected intervals to investigate the sensitivity of these inputs on the empirical THMtot equation at ranges relevant to well parameters. | |
Individual sensitivities generally reflected a parameter's relative exponentials in the model equation, yet the magnitude of effect on final predicted THMtot also depended on the total variability in the selected parameter range. Across the tested temperature range, the median predicted well concentration varied linearly by about 20 μg L−1: 49.5 vs. 70.5 μg L−1 at 50 and 100 °C, respectively. From pH 5.5 to 8.5, the median predicted well concentration spanned a larger linear range from 44.4 to 92.7 μg L−1, indicating a higher response to predicted THM formation due to higher pH, consistent with the model equation's relatively high exponent (i.e., pH1.695) and with the disinfection chemistry literature.61,69,70 The remaining model input parameters (time, bromide concentration, and the ratio of chlorine dosage to DOC) resulted in logarithmic predicted THMtot responses (Fig. 3); i.e. initial parameter increases corresponded to greater increases in THMtot but the responses diminished toward the latter end of the ranges. From t = 12 to t = 192 hours (8 days), the median predicted well concentration roughly doubled from 39.7 to 81.4 μg L−1. Similar trends resulted from the dosage/DOC and bromide sensitivity analyses. From Cl2/DOC = 0.02 to 0.2, the median predicted THMtot jumped from 42.2 to 66.1 μg L−1 but at Cl2/DOC = 1.0 saw about the same increase of about 24 μg L−1 to 90.2. From Br− = 40 to 360 mg L−1, the median predicted THMtot increased from 48.8 to 78.8 μg L−1 and then to 98 μg L−1 at the highest tested value of 1000 mg L−1. Overall, model outputs were most sensitive to pH and bromide levels (and clearly time, as the model is a kinetic one), whereas well temperature had a smaller effect. This information can be used to prioritize ancillary data collection at well sites; for example, Br− measurement should be encouraged as they are only collected at 5.7% of wells nationally, whereas pH measurements are more standard and widely available.
Implications
We have developed an evaluation framework using a unified, searchable database capable of linking experimental transformation studies (which simulate subsurface conditions based on measurements in the field) back to the wells that meet the prescribed conditions promoting those transformations. For environmental monitors, this tool might suggest target contaminants at a spill site or flag potentially overlooked transformation products. For example, halogen-substituted additives often have similar or enhanced toxicities and recalcitrance,71–76 yet would not appear on any initial disclosure reports. For wastewater treatment engineers, knowledge of incoming water quality could potentially alter treatment strategies. For well operators, this tool can highlight wells or regions of future explorations where systematic additive choices or conservative usage could prevent such transformations a priori. These approaches could help avoid costly mitigation strategies (e.g., measures to reduce THM formation) and protect public ecological health.
A major goal of this study was to meaningfully synthesize the wealth of publicly available data for assessing transformation models, seeking to bridge analyses from single-ingredient simulation studies to nation-wide trends suggesting transformation potential. While the presented predictions have not been verified, the model provides critical information needed to inform a rigorous evaluation. For example, the output of the model can now guide the collection of both positive and negative controls at strategic sites across the country, as well as a spread of quantitative measurements across the identified basins. This strategy is necessary given the logistical and analytical hurdles to comprehensive flowback sampling2,28 (e.g., current limitations of ad hoc strategies for flowback water compositional analysis only where sample access is granted or available). To date, we are limited to a handful of observations that confirm the presence of halogenated chemicals, including THMs, in basins where their formation was anticipated by the model.19,21,28,77
As of now, the two case studies investigated here offer just a limited vision for the scope of this evaluation tool to leverage known subsurface pathways and well parameters. While experimental capacities improve and the field characterizes more subsurface transformation pathways and physicochemical interactions, this framework provides a critical first step towards truly predictive modeling of flowback composition and environmental risk. As such, the approach presented here can and should be modified to enable a complete and multi-faceted suite of fate predictions. We envision this platform will stimulate researchers to populate the missing and necessary data. For example:
(i) Enhanced, geospatially resolved knowledge of mineralogy and organic-carbon content could enable ingredient-specific estimates for surface partitioning, governing whether additives or transformation products return to the surface with the flowback fluids.
(ii) Permeability and fracture measurements along the well horizon could inform subsurface transport phenomena and their effects on flowback interactions and composition (e.g., improved understanding of the physical mixing processes).
(ii) Knowledge of downhole-relevant microbial activity, proteins, and their transformation capabilities25,52,78 relative to subsurface conditions could improve existing biopathway models.43
(iv) Organic transformation models,44–46 modified to incorporate mixtures and adjust for extreme physical/chemical conditions could estimate product formation extents and kinetics.
(v) Toxicity measurements79–81 or estimates, exposure models,82 and population data could be appended, akin to the EPA's Risk Screening Environmental Indicators (RSEI) model,83 using FracFocus ingredient disclosures and input in lieu of the Toxic Release Inventory (TRI).
Ultimately, the scope and growth of hydraulic fracturing fluids as hazardous wastes for treatment, management, or remediation demands continued attention. The database framework presented here should serve as a foundation for more ambitious model components that can apply, in real time, emerging transformation studies to inform final flowback compositions. In turn, this information will inform better public health, economic, gas production, and treatment optimization for more sustainable development of this important energy industry.
Conflicts of interest
There are no conflicts of interest to declare.
Acknowledgements
The authors acknowledge support from the United States National Science Foundation Graduate Research Fellowship Program for support of AJS.
References
-
US Energy Information Administration, Annual Energy Outlook 2018 with projections to 2050, 2018 Search PubMed.
- J. L. Luek and M. Gonsior, Water Res., 2017, 123, 536–548 CrossRef CAS PubMed.
- A. Vengosh, R. B. Jackson, N. Warner, T. H. Darrah and A. Kondash, Environ. Sci. Technol., 2014, 48, 8334–8348 CrossRef CAS PubMed.
- A. Kondash and A. Vengosh, Environ. Sci. Technol. Lett., 2015, 4(10), 276–280 CrossRef.
- A. J. Kondash, N. E. Lauer and A. Vengosh, Sci. Adv., 2018, 4, eaar5982 CrossRef CAS PubMed.
- G. T. Llewellyn, F. Dorman, J. L. Westland, D. Yoxtheimer, P. Grieve, T. Sowers, E. Humston-Fulmer and S. L. Brantley, Proc. Natl. Acad. Sci. U. S. A., 2015, 112, 6325–6330 CrossRef CAS PubMed.
- B. D. Drollette, K. Hoelzer, N. R. Warner, T. H. Darrah, O. Karatum, M. P. O'Connor, R. K. Nelson, L. A. Fernandez, C. M. Reddy, A. Vengosh, R. B. Jackson, M. Elsner and D. L. Plata, Proc. Natl. Acad. Sci. U. S. A., 2015, 112, 13184–13189 CrossRef CAS PubMed.
- E. G. Elliott, X. Ma, B. P. Leaderer, L. A. McKay, C. J. Pedersen, C. Wang, C. J. Gerber, T. J. Wright, A. J. Sumner, M. Brennan, G. S. Silva, J. L. Warren, D. L. Plata and N. C. Deziel, Environ. Res., 2018, 167, 550–557 CrossRef CAS PubMed.
- G. J. Getzinger, M. P. O'Connor, K. Hoelzer, B. D. Drollette, O. Karatum, M. A. Deshusses, P. L. Ferguson, M. Elsner and D. L. Plata, Environ. Sci. Technol., 2015, 49, 8347–8355 CrossRef CAS PubMed.
- K. M. Parker, T. Zeng, J. Harkness, A. Vengosh and W. A. Mitch, Environ. Sci. Technol., 2014, 48, 11161–11169 CrossRef CAS PubMed.
- L. O. Haluszczak, A. W. Rose and L. R. Kump, Appl. Geochem., 2013, 28, 55–61 CrossRef CAS.
- J. S. Harkness, G. S. Dwyer, N. R. Warner, K. M. Parker, W. A. Mitch and A. Vengosh, Environ. Sci. Technol., 2015, 49, 1955–1963 CrossRef CAS PubMed.
- J. Rosenblum, A. Nelson and B. Ruyle, Sci. Total Environ., 2017, 596, 369–377 CrossRef PubMed.
- T. L. Tasker, W. D. Burgos, M. A. Ajemigbitse, N. E. Lauer, A. V. Gusa, M. Kuatbek, D. May, J. D. Landis, D. S. Alessi, A. M. Johnsen, J. M. Kaste, K. L. Headrick, F. D. H. Wilke, M. McNeal, M. Engle, A. M. Jubb, R. D. Vidic, A. Vengosh and N. R. Warner, Environ. Sci.: Processes Impacts, 2019, 21, 224–241 RSC.
- N. R. Warner, R. B. Jackson, T. H. Darrah, S. G. Osborn, A. Down, K. Zhao, A. White and A. Vengosh, Proc. Natl. Acad. Sci. U. S. A., 2012, 109, 11961–11966 CrossRef CAS PubMed.
- B. Ouyang, D. J. Renock, M. A. Ajemigbitse, K. Van Sice, N. R. Warner, J. D. Landis and X. Feng, Environ. Sci.: Processes Impacts, 2019, 21, 339–351 RSC.
- J. S. Harkness, T. H. Darrah, N. R. Warner, C. J. Whyte, M. T. Moore, R. Millot, W. Kloppmann, R. B. Jackson and A. Vengosh, Geochim. Cosmochim. Acta, 2017, 208, 302–334 CrossRef CAS.
- I. Ferrer and E. M. Thurman, Anal. Bioanal. Chem., 2015, 407, 6417–6428 CrossRef CAS PubMed.
- S. J. Maguire-Boyle and A. R. Barron, Environ. Sci.: Processes Impacts, 2014, 16, 2237–2248 RSC.
- W. Orem, C. Tatu, M. Varonka, H. Lerch, A. Bates, M. Engle, L. Crosby and J. McIntosh, Int. J. Coal Geol., 2014, 126, 20–31 CrossRef CAS.
- J. Rosenblum, E. M. Thurman, I. Ferrer, G. Aiken and K. G. Linden, Environ. Sci. Technol., 2017, 51, 14006–14015 CrossRef CAS PubMed.
- J. L. Luek, M. Harir, P. Schmitt-Kopplin, P. J. Mouser and M. Gonsior, Water Res., 2018, 136, 200–206 CrossRef CAS PubMed.
- J. L. Luek, M. Harir, P. Schmitt-Kopplin, P. J. Mouser and M. Gonsior, Environ. Sci.: Processes Impacts, 2019, 21, 206–213 RSC.
- J. D. Rogers, E. M. Thurman, I. Ferrer, J. S. Rosenblum, M. V. Evans, P. J. Mouser and J. N. Ryan, Environ. Sci.: Processes Impacts, 2019, 21, 256–268 RSC.
- D. Kekacs, B. Drollette, M. Brooker, D. Plata and P. Mouser, Biodegradation, 2015, 1–17 Search PubMed.
- M. Elsner and K. Hoelzer, Environ. Sci. Technol., 2016, 50, 3290–3314 CrossRef CAS.
- W. T. Stringfellow, J. K. Domen, M. K. Camarillo, W. L. Sandelin and S. Borglin, J. Hazard. Mater., 2014, 275, 37–54 CrossRef CAS PubMed.
- K. Hoelzer, A. J. Sumner, O. Karatum, R. K. Nelson, B. D. Drollette, M. P. O'Connor, E. L. D'Ambro, G. J. Getzinger, P. L. Ferguson, C. M. Reddy, M. Elsner and D. L. Plata, Environ. Sci. Technol., 2016, 50, 8036–8048 CrossRef CAS PubMed.
- A. A. Holland, Bull. Seismol. Soc. Am., 2013, 103, 1784–1792 CrossRef.
- R. W. Howarth, R. Santoro and A. Ingraffea, Clim. Change, 2011, 106, 679–690 CrossRef CAS.
- O. Schneising, J. P. Burrows, R. R. Dickerson, M. Buchwitz, M. Reuter and H. Bovensmann, Earth's Future, 2014, 2, 548–558 CrossRef CAS.
- T. V. Bartholomew and M. S. Mauter, ACS Sustainable Chem. Eng., 2016, 4, 3728–3735 CrossRef CAS.
- M. J. Small, P. C. Stern, E. Bomberg, S. M. Christopherson, B. D. Goldstein, A. L. Israel, R. B. Jackson, A. Krupnick, M. S. Mauter, J. Nash, D. W. North, S. M. Olmstead, A. Prakash, B. Rabe, N. Richardson, S. Tierney, T. Webler, G. Wong-Parodi and B. Zielinska, Environ. Sci. Technol., 2014, 48, 8289–8297 CrossRef CAS PubMed.
- N. C. Deziel, Z. Humeau, E. G. Elliott, J. L. Warren and L. M. Niccolai, PLoS One, 2018, 13, e0194203 CrossRef PubMed.
- E. G. Elliott, P. Trinh, X. Ma, B. P. Leaderer, M. H. Ward and N. C. Deziel, Sci. Total Environ., 2017, 576, 138–147 CrossRef CAS PubMed.
- FracFocus chemical disclosure registry, accessed 2014–2019.
-
H. A. Waxman, E. J. Markey and D. DeGette, Chemicals used in hydraulic fracturing, United States House of Representatives Committee on Energy and Commerce Minority Staff, 2011 Search PubMed.
- A. J. Sumner and D. L. Plata, Environ. Sci.: Processes Impacts, 2018, 20, 318–331 RSC.
- A. J. Sumner and D. L. Plata, Environ. Sci. Technol., 2018, 52, 9097–9107 CrossRef CAS PubMed.
- G. A. Kahrilas, J. Blotevogel, E. R. Corrin and T. Borch, Environ. Sci. Technol., 2016, 50, 11414–11423 CrossRef CAS PubMed.
- B. Xiong, Z. Miller, S. Roman-White, T. Tasker, B. Farina, B. Piechowicz, W. D. Burgos, P. Joshi, L. Zhu, C. A. Gorski, A. L. Zydney and M. Kumar, Environ. Sci. Technol., 2018, 52, 327–336 CrossRef CAS PubMed.
- M. L. Card, V. Gomez-Alvarez, W.-H. Lee, D. G. Lynch, N. S. Orentas, M. T. Lee, E. M. Wong and R. S. Boethling, Environ. Sci.: Processes Impacts, 2017, 19, 203–212 RSC.
- J. Wicker, T. Lorsbach, G. Martin, E. Schmid, D. Latino, S. Kramer and K. Fenner, Nucleic Acids Res., 2016, 44, 502–508 CrossRef PubMed.
- C. Tebes-Stevens, J. M. Patel, W. J. Jones and E. J. Weber, Environ. Sci. Technol., 2017, 51, 5008–5016 CrossRef CAS PubMed.
- K. Wolfe, N. Pope, R. Parmar, M. Galvin, C. Stevens, E. Weber, J. Flaishans and T. Purucker, BYU School Archive, 2016 Search PubMed.
- P. G. Tratnyek, E. J. Bylaska and E. J. Weber, Environ. Sci.: Processes Impacts, 2017, 19, 188–202 RSC.
- H. C. Hong, Y. Liang, B. P. Han, A. Mazumder and M. H. Wong, Sci. Total Environ., 2007, 385, 48–54 CrossRef CAS PubMed.
-
M. S. Blondes, K. D. Gans, J. J. Thordsen, M. E. Reidy, B. Thomas, M. A. Engle and E. L. Rowan, US Geological Survey National Produced Waters Geochemical Database v2. 3 (PROVISIONAL), United States Geological Survey, 2016 Search PubMed.
-
D. D. Blackwell, C. Chickering Pace and M. C. Richards, Recovery Act: Geothermal Data Aggregation: Submission of Information into the National Geothermal Data System, Final Report DOE Project DE-EE0002852 June 24, 2014, Golden, CO, United States, 2014 Search PubMed.
- A. J. Sumner and D. L. Plata, Environ. Sci. Technol., 2019, 53(14), 8216–8226 CrossRef CAS PubMed.
- R. A. Daly, M. A. Borton, M. J. Wilkins, D. W. Hoyt, D. J. Kountz, R. A. Wolfe, S. A. Welch, D. N. Marcus, R. V. Trexler, J. D. MacRae, J. A. Krzycki, D. R. Cole, P. J. Mouser and K. C. Wrighton, Nat. Microbiol., 2016, 1, 16146 CrossRef CAS PubMed.
- P. J. Mouser, S. Liu, M. A. Cluff, M. McHugh, J. J. Lenhart and J. D. MacRae, Environ. Eng. Sci., 2016, 33, 827–838 CrossRef CAS.
- D. E. Helbling, J. Hollender, H.-P. E. Kohler, H. Singer and K. Fenner, Environ. Sci. Technol., 2010, 44, 6621–6627 CrossRef CAS PubMed.
- M. Wang and D. E. Helbling, Water Res., 2016, 102, 241–251 CrossRef CAS PubMed.
- B. I. Escher and K. Fenner, Environ. Sci. Technol., 2011, 45, 3835–3847 CrossRef CAS PubMed.
- A. B. A. Boxall, C. J. Sinclair, K. Fenner, D. Kolpin and S. J. Maud, Environ. Sci. Technol., 2004, 38, 368A–375A CrossRef CAS PubMed.
- J. Klasmeier, M. Matthies, M. Macleod, K. Fenner, M. Scheringer, M. Stroebe, A. C. Le Gall, T. Mckone, D. Van De Meent and F. Wania, Environ. Sci. Technol., 2005, 39, 1932–1942 CrossRef PubMed.
- K. Fenner, M. Scheringer, M. MacLeod, M. Matthies, T. McKone, M. Stroebe, A. Beyer, M. Bonnell, A. Le Gall, J. Klasmeier, C. Mackay, D. van de Meent, D. Pennington, B. Scharenberg, N. Suzuki and F. Wania, Environ. Sci. Technol., 2005, 40, 53–60 Search PubMed.
- S. Chowdhury, P. Champagne and P. J. McLellan, Sci. Total Environ., 2009, 407, 4189–4206 CrossRef CAS PubMed.
-
L. K. Wang, P.-C. Yuan and Y.-T. Hung, in Physicochemical Treatment Processes, Humana Press, Totowa, NJ, 2005, pp. 271–314 Search PubMed.
-
Board on Toxicology and Environmental Health Hazards; Safe Drinking Water Committee; Assembly of Life Sciences, The Disinfection of Drinking Water: Volume 2, Washington (DC), 1980 Search PubMed.
- W. T. Stringfellow and M. K. Camarillo, Environ. Sci.: Processes Impacts, 2019, 21, 370–383 RSC.
- S. Navalon, M. Alvaro and H. Garcia, Water Res., 2008, 42, 3990–4000 CrossRef CAS PubMed.
- B. K. Shoichet, Nature, 2004, 432, 862–865 CrossRef CAS PubMed.
- C. Liang, Z.-S. Wang and N. Mohanty, Sci. Total Environ., 2006, 370, 271–277 CrossRef CAS PubMed.
- R. L. Johnson, P. G. Tratnyek and R. O. Johnson, Environ. Sci. Technol., 2008, 42, 9350–9356 CrossRef CAS PubMed.
- S. Ahn, T. D. Peterson, J. Righter, D. M. Miles and P. G. Tratnyek, Environ. Sci. Technol., 2013, 47, 11717–11725 CrossRef CAS PubMed.
- A. N. Paukert Vankeuren, J. A. Hakala, K. Jarvis and J. E. Moore, Environ. Sci. Technol., 2017, 51(16), 9391–9402 CrossRef CAS PubMed.
- T. Bond, E. H. Goslan, S. A. Parsons and B. Jefferson, Environ. Technol. Rev., 2012, 1, 93–113 CrossRef CAS.
-
R. A. Larson and E. J. Weber, Reaction Mechanisms in Environmental Organic Chemistry, Lewis Publishers, Ann Arbor, 1994 Search PubMed.
- S. D. Richardson, M. J. Plewa, E. D. Wagner, R. Schoeny and D. M. DeMarini, Mutat. Res., Mutat. Res. Genomics, 2007, 636, 178–242 CrossRef CAS PubMed.
- F. I. Hai, N. Tadkaew, J. A. McDonald, S. J. Khan and L. D. Nghiem, Bioresour. Technol., 2011, 102, 6299–6303 CrossRef CAS PubMed.
- E. K. Weisburger, Environ. Health Perspect., 1977, 21, 7–16 CrossRef CAS PubMed.
- X.-F. Li and W. A. Mitch, Environ. Sci. Technol., 2018, 52(4), 1681–1689 CrossRef CAS PubMed.
- V. K. Sharma, R. Zboril and T. J. McDonald, J. Environ. Sci. Health, Part B, 2014, 49, 212–228 CrossRef CAS PubMed.
- S. Richardson, F. Fasano and J. Ellington, Environ. Sci. Technol., 2008, 42, 8330–8338 CrossRef CAS.
- J. L. Luek, P. Schmitt-Kopplin, P. J. Mouser, W. T. Petty, S. D. Richardson and M. Gonsior, Environ. Sci. Technol., 2017, 51, 5377–5385 CrossRef CAS.
- M. V. Evans, A. J. Sumner, R. A. Daly, J. L. Luek, D. L. Plata, K. C. Wrighton and P. J. Mouser, Environ. Sci. Technol. Lett., 2019, 6, 585–591 CrossRef CAS.
- E. E. Yost, J. Stanek, R. S. DeWoskin and L. D. Burgoon, Environ. Sci. Technol., 2016, 50, 7732–7742 CrossRef CAS PubMed.
- E. E. Yost, J. Stanek, R. S. DeWoskin and L. D. Burgoon, Environ. Sci. Technol., 2016, 50, 4788–4797 CrossRef CAS PubMed.
- E. G. Elliott, A. S. Ettinger, B. P. Leaderer, M. B. Bracken and N. C. Deziel, J. Exposure Sci. Environ. Epidemiol., 2016, 27, 90–99 CrossRef PubMed.
- J. D. Rogers, T. L. Burke, S. G. Osborn and J. N. Ryan, Environ. Sci. Technol. Lett., 2015, 2, 158–164 CrossRef CAS.
- United States Environ. Prot. Agency, Off. Pollut. Prev.
Footnote |
† Electronic supplementary information (ESI) available. See DOI: 10.1039/c9em00505f |
|
This journal is © The Royal Society of Chemistry 2020 |
Click here to see how this site uses Cookies. View our privacy policy here.