Intra- and intermolecular atomic-scale interactions in the receptor binding domain of SARS-CoV-2 spike protein: implication for ACE2 receptor binding†
Received
11th June 2020
, Accepted 22nd July 2020
First published on 5th August 2020
Abstract
The COVID-19 pandemic poses a severe threat to human health with unprecedented social and economic disruption. Spike (S) glycoprotein in the SARS-CoV-2 virus is pivotal in understanding the virus anatomy, since it initiates the early contact with the ACE2 receptor in the human cell. The subunit S1 in chain A of S-protein has four structural domains: the receptor binding domain (RBD), the n-terminal domain (NTD) and two subdomains (SD1, SD2). We report details of the intra- and inter-molecular binding mechanism of RBD using density functional theory, including electronic structure, interatomic bonding and partial charge distribution. We identify five strong hydrogen bonds and analyze their roles in binding. This provides a pathway to a quantum-chemical understanding of the interaction between the S-protein and the ACE2 receptor with insights into the function of conserved features in the ACE2 receptor binding domain that could inform vaccine and drug development.
Introduction
The outbreak of the coronavirus disease in 2019 (COVID-19) has rapidly emerged as a detrimental pandemic with no end in sight. It has claimed thousands of lives worldwide and is continuing with unabashed lethality.1–3 The scientific community has been fully mobilized to address this unprecedented and devastating crisis. All scientific organizations, academic institutions, and public and private funding agencies in many countries responded immediately to facilitate the scientific research and development to combat the coronavirus in different aspects and capacities, with a growing number of focused research publications.4–9 One of the most important reports concerns the determination of the structure of the SARS-CoV-2 virus.4 The spike (S) glycoprotein seems to be not only the key component in understanding the anatomy of the virus, but also plays a pivotal role for potential vaccine development, being closely connected with the angiotensin converting enzyme (ACE2) in human cells,10 found on the outer surface of cells in lungs, arteries, heart, kidney and intestines. While arguably state-of-the-art, the experimental resolution of the cryo-electron microscopy (cryo-EM) structural probe is still quite limited and computational modeling based on quantum biology11–15 is therefore widely acknowledged as a viable complement to increase the structural resolution, thus enabling a more accurate investigation of the interatomic interaction and binding mechanisms. In this context, the cryo-EM structural study of the spike (S) glycoprotein in the prefusion conformation4,16–18 has prompted a surge of fundamental physical and bio-medical research at the atomistic level using large-scale computational methods,4,16–18 which we will amplify by a state-of-the-art density functional theory approach, elucidating the electronic structure, interatomic bonding and partial charge (PC) distribution of the protein with implication for the ACE2 receptor binding.
SARS-CoV-2 exhibits four different structural proteins: spike (S), envelope (E), membrane (M), and nucleocapsid (N) proteins. The spike (S) protein is directed outward from the lipid membrane matrix, while the two other proteins (E and M) are located between the spikes. The role of the fourth protein, the nucleocapsid protein, is to condense the 29
900 nucleotide long ss-RNA genome, which in the virus amplification cycle seizes the cell protein machinery. The S-protein, consisting of subunits S1 and S2, plays a crucial role in the first contact between the virus and the ACE2 receptor. The S1 subunit binds to the host ACE2 receptor and the S2 subunit is activated by the host serine protease TMPRSS2, which promotes membrane fusion. Once inside the cell, SARS-CoV-2 hijacks the host to transcribe, replicate and translate its RNA genome into different virus proteins that are used to reassemble, encapsulate and exocytose the newly formed virions from the cell.
The viral load was found to correlate with comorbidities and/or mutagenic properties contributing to disease in lung tissues19,20 and recent studies elucidate pieces of the pathology puzzle surrounding COVID-19. It is suggested that as infection progresses, immune imbalance occurs; interferon levels dwindle, limiting the attenuation of viral replication, and IL-6 expression becomes significantly elevated, thus promoting inflammation and accumulation of macrophages at the site of infection. Specifically, damage in the lungs is attributed to the death of Type II lung pneumocytes which compromises the air exchange and enable fluid inflow to the lungs.
The cryo-EM 3D-structure of SARS-CoV-2 with a 3.5 Å resolution is known and is deposited in PDB (ID: 6VSB).4 The reported structure for the spike protein in the prefusion conformation has three chains A, B, C (Fig. 1a), with each chain containing S1 and S2 subunits. S1 consists of the receptor binding domain (RBD), the n-terminal domain (NTD), and subdomains SD1 and SD2 (Fig. 1b). The A chain defines the “up” conformation corresponding to the receptor-accessible state, and the B and C chains the “down” conformations corresponding to receptor-inaccessible states.4 The focal point of our analysis is specifically the RBD of the S-protein, which interacts with the ACE2 receptor of the cell and is thus involved in the crucial step of the virus infectivity. In what follows, we describe a density functional theory (DFT) calculation of the atomic-scale interaction and intramolecular binding in the RBD domain of the S-protein, as well as of the intermolecular interaction with the subdomains SD1–SD2, both part of the S1 subunit. The output of the calculations includes the electronic structure, the interatomic bonding, the partial charge distribution, as well as a detailed investigation of the hydrogen bonding (HB) in RBD and in SD1–SD2. With the PC distributions on every residue of RBD and SD1–SD2 subunit known, we then explore the polar electrostatic (ES) interaction at specific locations that may play a crucial role in the interaction between the S-protein RBD and the ACE2 receptor in the post-fusion structure.
 |
| Fig. 1 The S-protein in SARS-CoV-2 consisting three chains: (a) chain A (tan), chain B (dark green), and chain C (pink). (b) The five structural domains in chain A are color coded as NTD (blue), RBD (green), SD1 (tan), SD2 (tan), and S2 (orange). The side legend shows the AA sequences with the same color code as for chain A. The range of the AA sequence not mentioned below chain A in the side bar indicates the missing position coordinates in 6VSB. (c) Ribbon structure for RBD, and (d) for SD1–SD2. (e) Ball-and-stick structure for NTD and (f) for S2. Color for atoms: grey (C), red (O), blue (N), white (H) and yellow (S). | |
Result
Refined structure of the S-protein
Table 1 gives a summary of the components of chain A in the S-protein, including the corresponding amino acid (AA) sequence numbers according to the PDB data (ID 6VSB).4 However, information on some flexible segments of the AA sequences are missing due to either technical difficulties encountered in resolving them in the experiment or because they were deemed to be not essential for biological interactions related to coronavirus.4 The smallest domain SD1 has 24 residues and a total of 391 atoms, with H atoms added to the PDB data using the Chimera software.21 NTD, RBD, SD1 and SD2 are parts of subunit S1. The subunit S2 has 433 residues and 6622 atoms.
Table 1 Structural domains of S-protein of SARS-CoV-2
Structural domain |
Chain A |
No. of AA |
No. of atoms |
With H |
NTD |
27–304 |
226 |
1745 |
3459 |
SD1 |
305–328 |
24 |
196 |
391 |
RBD |
335–521 |
144 |
1074 |
2100 |
SD2 |
522–672 |
132 |
972 |
1912 |
S2 |
687–1146 |
433 |
3324 |
6626 |
Chain A |
27–1146 |
959 |
7311 |
14 486 |
The most important domain in Table 1 is the RBD, which is pivotal for our study. RBD is a very large biomolecule with 144 residues and a total of 2100 atoms. The NTD domain is larger than the RBD with 226 residues and 3459 atoms. The full picture of the SARS-CoV-2 is illustrated in Fig. 1a and the structural domains of the S-protein are shown in Fig. 1b. The ribbon structures of RBD and SD1–SD2 are shown in Fig. 1c and d, and those in Fig. 1e and f show the ball-and-stick structure of NTD and S2, respectively. Obviously, ab initio DFT calculations of such large proteins are extremely challenging. Fortunately, the strategy we designed and the methods we developed22–26 can successfully meet such a challenge. In the present work, the calculations are restricted to RBD and SD1–SD2 only.
Structural relaxation of these large biomolecules is very important for two reasons. (1) The atomic data deposited in PDB do not include H atoms. They are added by using Chimera software and thus may not be in the optimal position in the equilibrium structure. (2) The actual data obtained from cryo-EM analysis have limitations. It is conceivable that they can be further optimized to higher accuracy by computational modeling and test calculations show substantial differences in the relaxed and unrelaxed structures. In the present case, the RMSD (root mean square deviation) in x, y and z position coordinates between the initial unrelaxed structure and the final VASP-relaxed structure are 0.48 Å, 0.51 Å and 0.55 Å, respectively. This corroborates the need for structural optimization of the S-protein prior to the DFT calculations in order to obtain realistic results on the electronic structure and bonding of these complex biomolecular systems.
Electronic structure and partial charge (PC) distribution
The electronic structure of RBD is calculated using the OLCAO method with the VASP optimized structure as input (see Method section). The calculated total density of states (TDOS) and atom-resolved partial DOS (PDOS) is shown in Fig. S1 in the ESI.† The PDOS can also be resolved into each of the 144 individual amino acid but they are not shown here. RBD has a HOMO–LUMO gap of about 2.4 eV. Of particular interest is that the states near HOMO and LUMO have significant contributions from sulfur atoms which are present only in the CYS residue. It is also obvious that the sharp peaks below −18.0 eV originate from the localized 2s orbitals of O, C and N in different residues of the RBD. Similar TDOS and PDOS for SD1–SD2 is shown in Fig. S2c and d (ESI†).
The calculated PCs in units of the electron charge (e) on each of the 2100 atoms in RBD are grouped into 144 amino acids and shown in Fig. 2a, as well as listed in Table S1 (ESI†). It shows that the residues in RBD can be both positively or negatively charged with several of them having very large PCs. They are: ARG355, LYS356, LYS378, LYS386, ARG408, SER443, ARG454, ARG509 and PRO521 with PC values of (1.005 e, 0.818 e, 0.742 e, 0.465 e, 0.884 e, 0.885 e, 1.054 e, 0.660 e, and 1.170 e), and LEU335, ARG357, ASP364, ASP389, ASP442, TYR449, PRO491, VAL503, and TYR505 with PC of (−0.789 e, −1.007 e, −0.595 e, −0.498 e, −0.701 e, −0.898 e, −0.973 e, −0.909 e, and −0.575 e). It is noted that the PRO491 is large and negative, whereas PRO521 is large and positive reflecting different intramolecular polar interactions within RBD. The PCs on other amino acids are smaller (Table S1, ESI†) and fluctuate between positive or negative. Naturally, the terminal residues LEU335 and PRO521 have respectively large negative, and large positive, PCs. The total PC for RBD is 0.00 e since an isolated macromolecule is charge neutral. Fig. 2b–e show the PC distribution on the solvent accessible surface in RBD in two different orientations. We can see the most positively (negatively) charged residues are ARG355, LYS356, ARG408, SER443, ARG454 and PRO521 (ARG357, TYR449, PRO491 and VAL503). These amino acids appear to be strictly located at the solvent accessible surface and not in the interior region of the RBD. It is also obvious that the positive and negative PCs do not pertain only to canonical AAs with dissociable groups27 (deprotonated ASP, GLU and TYR, protonated ARG, LYS and HIS) but also highlight other non-standard AAs with large PCs that are driven by the local molecular environment. The detailed magnitude of the PC on each residue is extremely important in order to ascertain the nature of polar interactions between different amino acids in the same domain or between different domains. Similar PC distributions for 156 residues in SD1–SD2 are shown in Fig. 5 and listed in Table S2 (ESI†).
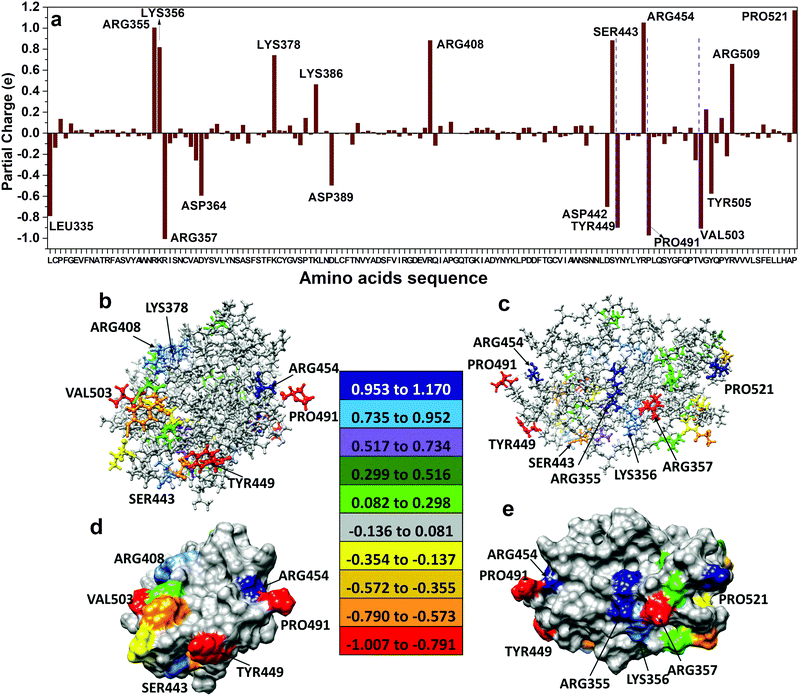 |
| Fig. 2 PC distribution in RBD. (a) For each amino acid in the sequence 335–521, from left to right, except for 444–448,455–490, and 501–502 indicated by three purple dashed lines. Amino acids with positive and negative PCs higher than 0.4 e and lower than −0.4 e, respectively, are marked. (b) and (c) PC distribution in different orientations, shown in ball-and-stick rendering. (d) and (e) PC distribution on the solvent accessible surface in different orientations. The color bar shows total PC for different amino acids from red (very negative) to blue (very positive). The navy blue, light blue, and red amino acids are identified explicitly. | |
Intramolecular bonding
The complexity of intramolecular bonding within RBD is revealed in the distribution of the bond order (BO) values and the corresponding bond length (BL) for every pair of atoms in RBD, as shown in Fig. 3a. There are 12 different types of interatomic pairs, including the O⋯H and N⋯H hydrogen bonds (HBs) with BL greater than 1.5 Å and a maximum BO of about 0.12 e. This will be discussed in more detail later. The strongest bonds are expectedly associated with C–O and C–C bonds in the residues containing them, with many of them actually double bonds. They are followed by other strong covalent bonds N–C, C–H, N–H, O–H etc. It should be pointed out that these bonds all have similar BLs but show very different ranges of BO values, reflecting the specific molecular environment of the 144 amino acids within RBD. It is noted that S–S bonds with considerable bond strength (BO = 0.17 e at BL = 2.03 Å) are present in RBD from the CYS residues.
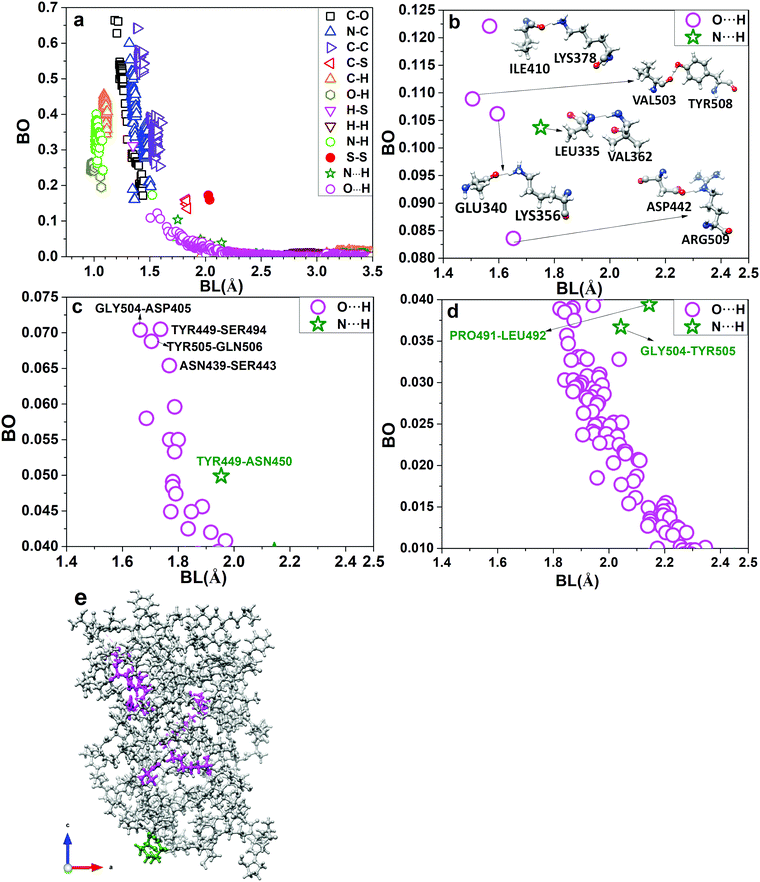 |
| Fig. 3 Interatomic bonding and hydrogen bonding (O⋯H and N⋯H) in RBD. (a) BO vs. BL distribution for different atomic pairs. (b) Strong HBs showing participating residues. (c) Same as (b) for HBs with medium strength. (d) Same as (b) for HBs weak strength. (e) Ball-and-stick figure of RBD showing distribution of AA involved in strong O⋯H (pink) and N⋯H (green) bonding. | |
We now discuss the presence of the vast number of HBs in RBD. Most of them are O⋯H bonds with some of them N⋯H bonds. We can divide them into three groups according to their strength: (i) strong HBs with BO values 0.080–0.125; (ii) medium HBs with BO values 0.040–0.075; and (iii) weak HBs with BO values 0.01–0.04, as shown in Fig. 3b–d, respectively. Fig. 3b shows five strong HBs, four O⋯H from the AA pairs of ILE410-LYS378, VAL503-TYR508, GLU340-LYS356, and ASP442-ARG509, and only one N⋯H HB from LEU335-VAL362 pair. In the medium strength group of Fig. 3c, there are eighteen O⋯H HBs and one N⋯H HB. In the weak HB group of Fig. 3d, there are many O⋯H and two N⋯H HBs with BO values ranging from 0.01 to 0.04. Their numbers are very large and thus can make significant contributions to the total HBs of internal cohesion in the RBD domain. We show the atomic scale sketch of the strong HBs displayed in Fig. 3e. These HBs are shown as dotted lines and can be traced to the residues located in the RBD. Amino acids involved in five strong HBs are shown in pink (O⋯H) and green (N⋯H) color in Fig. 3e. All HBs for RBD are listed in Table S3 (ESI†).
Intermolecular bonding and implications
In order to investigate the intermolecular interactions of the RBD with other units in the S-protein, apart from, and in addition to, the intra-molecular interaction discussed above, we show the results of similar calculation for the SD1–SD2 subdomains (see Fig. 4a). The SD1–SD2 interact with the RBD as illustrated in Fig. 4b. The closest separation between these two biomolecules is only 1.33 Å between residue PRO521 in RBD and residue ALA522 in SD2 (Fig. 4c–e). Before we discuss any interactions between RBD and other possible domains such as ACE2 receptors, it is prudent to first delve into the smaller SD1–SD2 subdomains separately as a viable example. Fig. 5a shows the PC distribution of residues in SD1–SD2. Just like the RBD in Fig. 2a, they contain residues with large positive and negative PCs with many of them being of the same type as in the RBD. This is of course due to the specificity of the 22 amino acids. The specific values of PCs in SD1 and SD2 are listed in Table S2 (ESI†). Fig. 5b–e show the PC distribution on the solvent accessible surface in SD1–SD2 in two different orientations. Their distribution is somewhat different from the one exhibited by the RBD in Fig. 2b–e. SD1–SD2 seem to be less evenly distributed as it has higher standard deviation in comparison with RBD. The most positively (negatively) charged residues are ARG328 (SER305). SER305, with largest negative PC, and ALA672, with high positive PC, lie at the far ends of the elongated molecular assembly.
 |
| Fig. 4 Interface structure and bonding between RBD (green) and SD1 (red)-SD2 (tan). (a) Ribbon structure; and (b) ball-and-stick structure. (c) Sketch of interactions between RBD and SD2 with bonding residues marked. (d) Atomic-scale structure of possible bonding between residues CYS525 and CYS391. (e) Atomic-scale structure of possible bonding between residues ALA522 and PRO521. | |
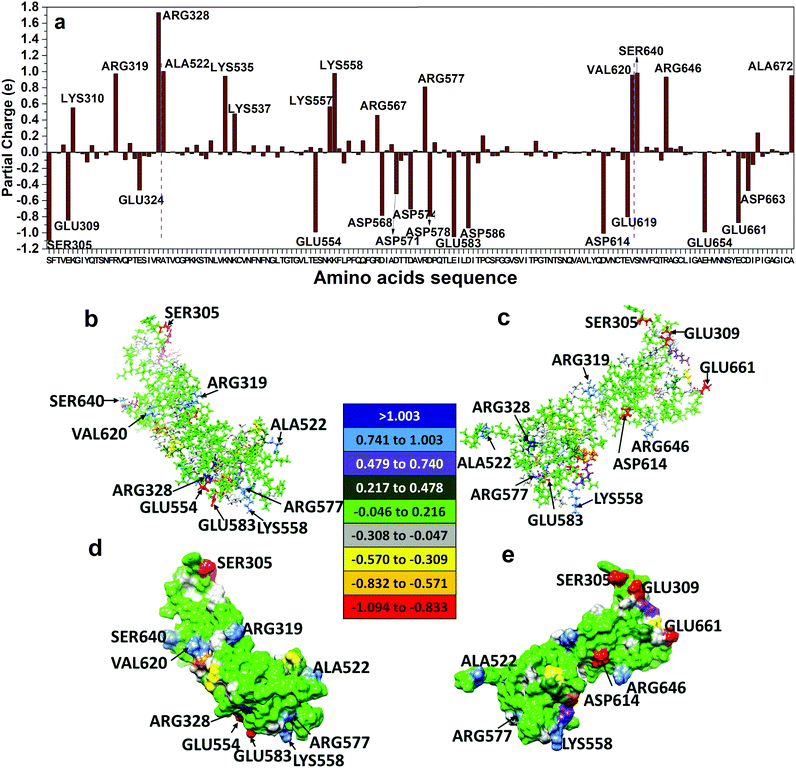 |
| Fig. 5 PC distribution in SD1–SD2. (a) For each amino acid in sequence 305–672, from left to right, except for 329–521 and 621–639 indicated by two purple dashed lines. Amino acids with positive and negative PCs higher than 0.4 e and lower than −0.4 e, respectively, are marked. (b) and (c) PC distribution in different orientations shown in ball-and-stick representation. (d) and (e) PC distribution on the solvent accessible surfaces in different orientations. The color bar shows total PC for different amino acids from red (very negative) to blue (very positive). The navy blue, light blue, and red amino acids are identified explicitly. | |
In Fig. S3 (ESI†), we display the HBs in SD1–SD2 divided into strong, medium and weak, in the same manner as in Fig. 3 for RBD, since both are large biomolecules consisting of similar residues in different sequences. Also noted is that there are no N⋯H HBs compared to RBD. A somewhat conspicuous fact is a very strong O⋯H bond between ASP574-LYS557 with BO value of 0.168 e that is very rare. A closer inspection of the geometry shows that the O⋯H BL is 1.48 Å, which is shorter than other HBs. All HBs for SD1–SD2 are listed in Table S4 (ESI†).
RBD-ACE2 interaction
The ab initio computational results for the RBD pave the way to a fundamental understanding of interactions between the S-protein and the angiotensin-converting enzyme 2 (ACE2) in the post-fusion conformation. The full-length structure for the ACE2 has recently been determined by cryo-EM10 and deposited in the PDB (ID: 6M18). The initial contact between RBD of the S-protein and ACE2 is through to be the peptidase domain (PD). The possible binding mechanism is of pivotal importance and has vital implications for vaccine design. In fact, as of April 20th, there are 93 candidate vaccines against SARS-CoV-2 being developed around the world, two of which target the S-protein and are already in clinical trials.28 About 40–50% of these vaccine candidates target the S-protein. Further, numerous entities specifically disclose usage of the RBD region of the SARS-CoV-2 SD1 in their vaccine design: (1) FluGen and University of Hong Kong29 both leverage a combination of live-attenuated influenza and the RBD subunit of S-protein, (2) U.S. Army Institute of Infectious Disease30 employs H. pylori-derived ferritin as a nanocarrier of the RBD subunit, (3) Biological E Limited31 delivers RBD subunit with a proprietary adjuvant, (4) Saiba GmbH31 expresses RBD subunit on a proprietary VLP, and (5) RNACure Biopharma32 deliver mRNA vaccines encoding the RBD subunit. RBD-based vaccines against SARS-CoV-2 hold significant promise given that RBD-vaccines against SARS-CoV were successfully developed in 2017 and that neutralizing monoclonal antibodies against SARS-CoV RBD derived from convalescent patients also bind to the SARS-CoV-2 RBD as discussed and reviewed elsewhere.33,34
The PD is itself a large domain with almost 600 residues (19-615),10 almost twice the combined size of RBD and SD1–SD2 (300 residues), so that the combined size of RBD in S-Protein and PD in ACE2 is 640 residues. Although such large calculations are possible using the present computational scheme with support from the current generation of supercomputing facilities, it is prudent to attack such problems by limiting the calculation to a selected region of the RBD-PD interface, which contains around 200 residues. A prime example is the weaker interface shown in Fig. 2C of ref. 10 involving GLN139 and GLN175. There could be other possible interface regions of interest such as those displayed in Fig. 4B–D of the same ref. 10 which involve a network of hydrogen bonds. Conservation of amino acids 451–509, including the receptor-binding domain ACE2 contact residues 455–505, has recently been studied for human SARS-CoV and SARS-CoV-2, three bat, as well as one pangolin coronavirus strain (cf.Fig. 1a in35). As expected, the ACE2 contact residues have a low conservation level (of only about 37%). It is therefore interesting that one of the three residues in this area with significant negative PC values (PRO491, see Fig. 2a and 6) is conserved across all strains. Moreover, VAL503 forms part of a strong HB (see Fig. 3(b) and 6). This suggests that this charged residue and this HB might play important roles in ACE2 receptor binding.
S-protein undergoes a conformational change during infection, in which the RBD of S1 carries out a hinge-like conformational movement into a receptor-binding active state.36 In a recent paper37 this mechanism has been analyzed for SARS-CoV-2 (6VSB), and it has been shown that an interaction between SER359 located in RBD and PRO561 located in SD2 is critically important for this conformational change. None of these amino acids have large PCs in our computations. However, interestingly, we have obtained a negative value (−0.046, Table S1, ESI†) for the former, and a positive value (0.139, Table S2, ESI†) for the latter, making this interaction indeed favorable. In SARS-CoV (5X5B), by contrast, this interaction is mediated by SER346 and PRO547, that have been mutated to ARG346 and THR547 in SARS-CoV-2 (6VSB). Both amino acids have positive PCs in our computation (0.030 and 0.068, respectively) preventing an interaction between these two sites. We observe several factors in our computations that may impact the formation of this vital SER359-PRO561 contact: (i) a cluster of residues with two larger positive PCs (ARG355; LYS356) and the largest negative PC (ARG357) (Fig. 2a) are surface accessible (Fig. 2e), and are proximal to SER359. (ii) Two of the strong HB bonds in Fig. 3b involve residues located three amino acids down- and upstream of SER359: GLU340-LYS356 and LEU335-VAL362, respectively. LYS356 and VAL362, in particular, could therefore potentially play a role in facilitating the SER359-PRO561 contact. In Fig. 6, we indicate our calculated data on PC and HB (from Fig. 2a and 3b) in the RBD on an alignment of SARS-CoV-2, SARS-CoV, and three bat strains using data from ref. 35 This shows how residue-specific data from our computational calculations correlate with conservation of AA sequences.
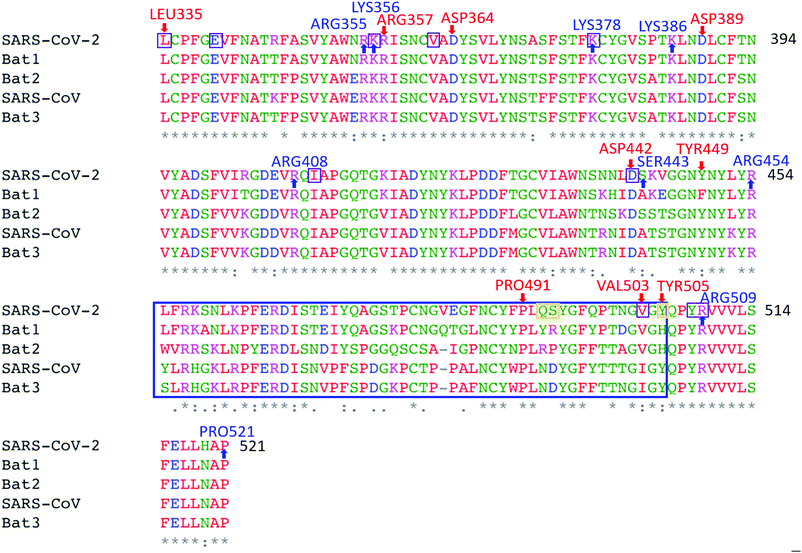 |
| Fig. 6 Characterization of the SARS-CoV-2 (6VSB) RBD. Alignment with other sequences (NCBI GenBank accession codes AY278741, MN996532, KY417146 and MK211376) reveals conserved amino acids (marked by stars). 130 AAs in the range 335–521, i.e., about 72%, are conserved, dropping to only 37% in the area corresponding to the ACE2 receptor binding contact residue area according to Andersen et al. (blue box; 455–505). The AAs indicated by red and blue arrows have negative and positive PCs lower than −0.4 e and higher than 0.4 e, respectively, and correspond to those labelled in Fig. 2a. 90% of the 10 purple-boxed AAs with strong HBs are conserved, and around 60% have high positive or low negative PCs. Position coordinates of AAs 455–490 & 501–502 in the ACE2 receptor binding contact residue area are missing in PDB-file 6VSB. Among the remaining ones, PRO491 is conserved whereas VAL503 and TYR505 are not. The orange boxes denote the three AAs in direct contact with the ACE2 receptor according to Andersen et al. One coincides with TYR505, with negative PC, and VAL503 forms part of a strong HB, suggesting that these charged residues and HBs may play important roles in ACE2 receptor binding. | |
This analysis demonstrates that the PC and HB values from our computations shed light on how mutations may affect the molecular mechanisms underpinning infection, such as ACE2 receptor binding and the conformational change that is critically important for infection. It also demonstrates how mutations in SARS-CoV-2 compared with related genomes may have resulted in higher infectivity.
Electrostatic interaction
The role of electrostatic interactions in intermolecular binding is well known.38 It is comprised of two components. The first one is structural, dependent on the distribution of PCs along the solvent accessible surface of the protein directly benefitting from the present study of the PCs in RBD and the two subdomains SD1 and SD2. The other one is thermodynamic, relating the equilibrium distribution of the mobile charges in the bathing solution to the structural charge on the protein.39 However, to quantify the detailed role of electrostatic interactions in the intra-protein stability and inter-protein interactions, one would need to include the aqueous solvent on some level, specifically the aqueous protons [pH], as well as the salt ions into the formulation. At present this is still not feasible. What could be feasible are the detailed ab initio calculations with atomistically resolved protein and a few strategically positioned waters and ions, consistent with the fact that the protein carries a net charge, depending on pH and ionic strength of the solution. The protonation–deprotonation reactions at the solvent exposed AA would need to be included through a phenomenological pKa, while the non-bonded water and the salt ions could be included on a continuum dielectric level. The coarse grained phenomenological and the atomistic microscopic description could then be bridged with a variant of the on-the-fly adaptive resolution simulation DNA.40 However, for a complex system such as SARS-CoV-2 our ab initio approach, that fully incorporates the structure of the protein but is weak in terms of the protein–bathing solution interactions, seems to be the best bet.
Effect of mutation
Mutations are always important in evolving biological systems, and in virology in particular. Generally speaking, a mutation refers to an error in DNA or RNA code,41 and can be both good as well as bad, depending on the effect it has on the proteome. RNA viruses such as SARS-CoV-2 have high mutation rates and evolve rapidly to adapt to local environmental conditions. In addition, it is not clear if there are any mutationally conserved sites in the coronavirus, and one would need to probe the differences in binding between the viral spike and the many recombinant ACE2s, a direction that has so far not been pursued. Computational modeling may offer some insights, as demonstrated in our earlier work, on the effects of a mutation in the consensus protein−RNA recognition motif on the strength of their interactions that was traced to the increased hydrogen bonding in the case of the single-stranded RNA MS2 virus.26
Solvation effect
Presently, almost all published work on SARS-CoV-2 and its S-protein seldom delves into the effect of the aqueous bathing solution. However, the water environment, be it in drops or aerosols, seems to be crucial in mitigating the spread, infection and transmission of the virus. That the effect of water molecules might be crucial follows first from the protonation–deprotonation equilibrium at the dissociable AAs related to electrostatic interaction, but also from the fact that the lipid membrane shell of the pleomorphic SARS-CoV-2 is composed of phospholipids and embedded protein amphiphilic moieties, that both strongly interact with water. Solvation interactions are quite complex and partitioned into the hydrophobic and hydration components42 that both depend on the HB configuration of water molecules between themselves and with the solvent exposed moieties of the protein and membrane. We are still far from bringing into the modeling fold the solvation interactions and water HB configurations, as the deposited SARS-CoV-2 PDB data do not even contain the H atoms, much less the water molecules. The solvation effect can thus only be studied either on an all-atom level by adding water molecules at strategically chosen locations of the protein structure, and investigating its effect on various aspects related to binding, Parts of the solvation problem could be implemented on the ab initio level in the study of the RBD/ACE2 interface interaction in relation to vaccine/drug development by inserting small molecules or peptides at key locations between proteins in screening for candidates for vaccines or drugs, or by replacing critical elements such as S by Se in CYS residues, as selenium deficiency appears to have a connection with the high death rate under coronavirus infection, similar to the case of hantavirus.43
Implications on vaccine and drug development as well as screening and monitoring
Computational analysis and detailed understanding of the structure of SARS-CoV-2 to enable the development of effective and safe vaccines. Safety concerns are present in the form of antibody dependent enhancement (ADE) of infection – as was observed in previous studies investigating SARS and MERS vaccine candidates.34,44–46 Clinical data from SARS-CoV-2 patient serum suggest disease severity is positively correlated with IgG titer.45,47,48 Therefore, a detailed structural understanding of how neutralizing antibodies interact with SARS-CoV-2 is highly critical. Computational models may help to differentiate between targets that are neutralizing vs. those that induce undesired ADE or other adverse immune effects. To this end, the concerted computational informatics and immunological screening of antibodies derived from patient sera have helped predict various B- and T-cell epitopes of the SARS-CoV-2 S-protein.
The above discussion made it clear that effective methods for monitoring and screening are both needed for tracing infections and for monitoring those that have been infected and may be immune due to acquisition of neutralizing antibodies. These latter tests are still in the development stage and require further validation. Detailed structural analysis combined with accurate computational modeling will help the development of such detection devices.
Summary
In summary, we provided a detailed ab initio DFT computational study at the basic atomic and amino acid level of interactions in the RBD domain of the SARS-CoV-2 spike protein. This unprecedented large-scale DFT calculation closes an important gap in our fundamental understating of the SARS-CoV-2 virus. The conclusions reached in this study are as follows:
(1) We demonstrate a detailed computational strategy, using a highly optimized structure of high accuracy, to unravel the interatomic bonding in the complex S-protein of the SARS-CoV-2 virus.
(2) Our calculations show both positive and negative PC distributions in the RBD domain. An amino acid with a large PC value is highly conserved among the ACE2 receptor binding contact residues, suggesting that it could be important for ACE2 receptor binding.
(3) We identified the strong HBs between specific residues in the RBD, which furthermore implies the importance of including solvent effects in SARS-CoV-2 research. One of the HBs is associated with an ACE2 receptor binding contact residue, suggesting a role in receptor binding.
(4) A cluster of residues with large positive PCs and a large negative PC, as well as two with strong HBs, are proximal to SER359, which forms part of the SER359-PRO561 interaction that is crucial for the conformational change into the receptor-binding active state. They may therefore play a vital role in this conformational change, and thus be important for infection.
(5) Our calculation on the SD1–SD2 subdomains identified possible interaction sites between specific residues of RBD and SD1–SD2 in these two biomolecular units.
(6) We demonstrate that accurate computational modeling could be a potent method, in combination with sequence conservation, to understand the molecular mechanism underpinning viral infection.
(7) The fundamental understanding of the SARS-CoV-2 virus enabled by our computational analysis provides intimate details of the nature of the infection process that could accelerate the discovery of vaccines and drugs to combat the COVID-19 pandemic.
Methods
Structural relaxation
The Vienna ab initio simulation package (VASP),49 which is highly effective for structure optimization, was employed to relax the existing experimentally determined structures for different domains of the S-protein in order to be utilized as input for the DFT calculations. We have used the projector augmented wave (PAW) method with the Perdew–Burke–Ernzerhof (PBE) exchange correlation functional.50 PBE is one of the most popular generalized gradient approximation (GGA) potentials and is reasonably accurate for biomolecular systems. A relatively high energy cutoff of 500 eV and the stringent electronic convergence criterion of 10−4 eV were adopted. The force convergence criteria for ionic relaxation was set at −10−2 eV Å−1. We used single k-point calculations since our models are in the form of large supercells and a single k-point calculation at the zone center is sufficient. It has been successfully demonstrated in many of our recent studies in bio-molecular systems, organic, inorganic and metallic crystals and glasses.22–26 All VASP calculations were carried out at the National Energy Research Scientific Computing (NERSC) facility at Lawrence Berkeley Laboratory and the HPC clusters of the University Missouri Research Computing Support Services (RSCC).
Electronic structure and interatomic bonding
The electronic structure calculations were based on the all-electron orthogonalized linear combination of atomic orbitals (OLCAO) method51 and applied to the VASP-relaxed structure as input. The merits of the OLCAO method is well documented and it is especially effective for large complex biomolecular systems such as COVID-19 virus. In particular, the OLCAO method can provide the effective charge (Q*) or partial charge (PC) on each atom as well as the bond order (BO) values ραβ between any pairs of atoms. The two are defined as | 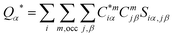 | (1) |
|  | (2) |
In the above equations, Siα,jβ are the overlap integrals between the ith orbital in αth atom in the jth orbital in βth atom. Cmjβ are the eigenvector coefficients of the mth occupied band. The PC (ΔQα = Q0α − Qα*) is the deviation from the neutral charge Q0α from the effective charge Qα* on the same atom α. The BO, which is basis-dependent only for short-ranged atomic orbitals, defines the relative strength of the bond. Comparisons of BO calculation using different basis or methods should thus be treated with caution. The atomic-scale interactions based on DFT calculations are critical for providing the accurate information necessary for their fundamental understanding. As RBD and SD1–SD2 have a total of 2100 and 2303 atoms, respectively, it is obviously quite challenging to obtain accurate atomic partial charges and bond order values between all pairs of atoms. More details on the OLCAO method can be found in ref. 51.
Conflicts of interest
There are no conflicts to declare.
Acknowledgements
This research used the resources of the National Energy Research Scientific Computing Center supported by DOE under Contract No. DE-AC03-76SF00098 and the Research Computing Support Services (RCSS) of the University of Missouri System. PA was supported in part from funds provided by the University of Missouri-Kansas City, School of Graduate Studies. NL is supported by the National Natural Science Foundation of China (Grant No. 11604249), the Fok Ying-Tong Education Foundation for Young Teachers in the Higher Education Institutions of China (Grant No. 161008), the Basic Research Program of Shenzhen (Grant No. JCYJ20190809120015163), the Overseas Expertise Introduction Project (111 project) for Discipline Innovation of China (B18038). M. S. was supported in part by CBI training program NIH T32GM135142. NS is supported for work on SARS-CoV-2 by the National Science Foundation RAPID CMMI-2027668 and CBET-2032196. RT acknowledges funding via an EPSRC Established Career Fellowship (EP/R023204/1), a Royal Society Wolfson Fellowship (RSWF\R1\180009) and a Joint Wellcome Trust Investigator Award (110145 & 110146). RP would like to acknowledge the support of the 1000-Talents Program of the Chinese Foreign Experts Bureau, and of the University of the Chinese Academy of Sciences, Beijing. WC is supported for work on SARS-CoV-2 by the National Science Foundation RAPID DMR/CMMT-2028803.
References
- J. F.-W. Chan, S. Yuan, K.-H. Kok, K. K.-W. To, H. Chu, J. Yang, F. Xing, J. Liu, C. C.-Y. Yip and R. W.-S. Poon, Lancet, 2020, 395, 514–523 CrossRef CAS.
- C. Huang, Y. Wang, X. Li, L. Ren, J. Zhao, Y. Hu, L. Zhang, G. Fan, J. Xu and X. Gu, Lancet, 2020, 395, 497–506 CrossRef CAS.
- WHO, Coronavirus Disease 2019 (COVID-19): Situation Report 98 (WHO, 2020), https://www.who.int/emergencies/diseases/novel-coronavirus-2019/situation-reports.
- D. Wrapp, N. Wang, K. S. Corbett, J. A. Goldsmith, C.-L. Hsieh, O. Abiona, B. S. Graham and J. S. McLellan, Science, 2020, 367, 1260–1263 CrossRef CAS PubMed.
- W. Tai, L. He, X. Zhang, J. Pu, D. Voronin, S. Jiang, Y. Zhou and L. Du, Cell. Mol. Immunol., 2020, 1–8, DOI:10.1038/s41423-020-0400-4.
- F. Wu, S. Zhao, B. Yu, Y.-M. Chen, W. Wang, Z.-G. Song, Y. Hu, Z.-W. Tao, J.-H. Tian and Y.-Y. Pei, Nature, 2020, 579, 265–269 CrossRef CAS PubMed.
- R. Lu, X. Zhao, J. Li, P. Niu, B. Yang, H. Wu, W. Wang, H. Song, B. Huang and N. Zhu, Lancet, 2020, 395, 565–574 CrossRef CAS.
- X. Ou, Y. Liu, X. Lei, P. Li, D. Mi, L. Ren, L. Guo, R. Guo, T. Chen and J. Hu, Nat. Commun., 2020, 11, 1–12 Search PubMed.
- M. Yuan, N. C. Wu, X. Zhu, C.-C. D. Lee, R. T. So, H. Lv, C. K. Mok and I. A. Wilson, Science, 2020, 1–9, DOI:10.1126/science.abb7269.
- R. Yan, Y. Zhang, Y. Li, L. Xia, Y. Guo and Q. Zhou, Science, 2020, 367, 1444–1448 CrossRef CAS PubMed.
- J. C. Brookes, Proc. R. Soc. A, 2017, 473, 20160822 Search PubMed.
- Quantum Biology: Powerful Computer Models Reveal Key Biological Mechanism, https://www.sciencedaily.com/releases/2007/01/070116133617.htm.
-
Computational biochemistry and biophysics, ed. O. M. Becker, A. D. MacKerell Jr, B. Roux and M. Watanabe, Marcel Decker, Inc., New York, 2001 Search PubMed.
-
Theoretical biochemistry: Processes and properties of biological systems, ed. L. A. Eriksson, Elsevier B. V. Science, Amsterdam, 2001 Search PubMed.
-
Quantum biochemistry: Electronic Structure and Biological Activity, ed. C. F. Matta, Wiley-VCH, Weinheim, 2010 Search PubMed.
- J. Wang, J. Chem. Inf. Model., 2020, 3277–3286 CrossRef CAS PubMed.
- J. Zou, J. Yin, L. Fang, M. Yang, T. Wang, W. Wu and P. Zhang, ChemRxiv, 2020, 1–13, DOI:10.26434/chemrxiv.11902623.v2.
- M. Smith and J. C. Smith, ChemRxiv, 2020, 1–29, DOI:10.26434/chemrxiv.11871402.v4.
- C. Ziegler, S. J. Allon, S. K. Nyquist, I. Mbano, V. N. Miao, Y. Cao, A. S. Yousif, J. Bals, B. M. Hauser and J. Feldman, Cell, 2020, 181(5), 1016–1035 CrossRef CAS PubMed.
- D. Blanco Melo, B. Nilsson Payant, W. Liu, S. Uhl, D. Hoagland, R. Møller, T. Jordan, K. Oishi, M. Panis, D. Sachs, T. Wang, R. Schwartz, J. Lim, R. Albrecht and B. tenOever, Cell, 2020, 181(5), 1036–1045 CrossRef CAS PubMed.
- E. F. Pettersen, T. D. Goddard, C. C. Huang, G. S. Couch, D. M. Greenblatt, E. C. Meng and T. E. Ferrin, J. Comput. Chem., 2004, 25, 1605–1612 CrossRef CAS PubMed.
- L. Poudel, A. M. Wen, R. H. French, V. A. Parsegian, R. Podgornik, N. F. Steinmetz and W. Y. Ching, ChemPhysChem, 2015, 16, 1451–1460 CrossRef CAS PubMed.
- J. Eifler, R. Podgornik, N. F. Steinmetz, R. H. French, V. A. Parsegian and W. Y. Ching, Int. J. Quantum Chem., 2016, 116, 681–691 CrossRef CAS.
- L. Poudel, N. F. Steinmetz, R. H. French, V. A. Parsegian, R. Podgornik and W.-Y. Ching, Phys. Chem. Chem. Phys., 2016, 18, 21573–21585 RSC.
- P. Adhikari, M. Xiong, N. Li, X. Zhao, P. Rulis and W.-Y. Ching, J. Phys. Chem. C, 2016, 120, 15362–15368 CrossRef CAS.
- L. Poudel, R. Twarock, N. F. Steinmetz, R. Podgornik and W.-Y. Ching, J. Phys. Chem. B, 2017, 121, 6321–6330 CrossRef CAS PubMed.
- R. Zandi, B. Dragnea, A. Travesset and R. Podgornik, Phys. Rep., 2020, 847, 1–102 CrossRef CAS.
- COVID-19 Treatments and Vaccines, https://milkeninstitute.org/covid-19-tracker.
- UW-Madison, FluGen, Bharat Biotech to develop CoroFlu, a coronavirus vaccine, https://www.businesswire.com/news/home/20200402005666/en/UW%E2%80%93Madison-FluGen-Bharat-Biotech-develop-CoroFlu-coronavirus.
-
E. Wiler, The US Army's Virus Research Lab Gears Up to Fight Covid-19, https://www.wired.com/story/the-us-armys-virus-research-lab-gears-up-to-fight-covid-19/.
-
https://www.who.int/blueprint/priority-diseases/key-action/novel-coronavirus-landscape-ncov.pdf
.
- Toward an effective mRNA vaccine against 2019-nCoV, https://www.fudan.edu.cn/en/2020/0307/c344a104281/page.htm.
- W.-H. Chen, P. J. Hotez and M. E. Bottazzi, Hum. Vaccines Immunother., 2020, 1–4, DOI:10.1080/21645515.2020.1740560.
- W.-H. Chen, S. M. Chag, M. V. Poongavanam, A. B. Biter, E. A. Ewere, W. Rezende, C. A. Seid, E. M. Hudspeth, J. Pollet and C. P. McAtee, J. Pharm. Sci., 2017, 106, 1961–1970 CrossRef CAS PubMed.
- K. G. Andersen, A. Rambaut, W. I. Lipkin, E. C. Holmes and R. F. Garry, Nat. Med., 2020, 26, 450–452 CrossRef CAS PubMed.
- A. C. Walls, Y.-J. Park, M. A. Tortorici, A. Wall, A. T. McGuire and D. Veesler, Cell, 2020, 181(2), 281–292 CrossRef CAS PubMed.
- S. Roy, bioRxiv, 2020, 1–30, DOI:10.1101/2020.04.20.052290.
- R. H. French, V. A. Parsegian, R. Podgornik, R. F. Rajter, A. Jagota, J. Luo, D. Asthagiri, M. K. Chaudhury, Y.-m. Chiang and S. Granick, Rev. Mod. Phys., 2010, 82, 1887 CrossRef.
- R. J. Nap, A. L. Božič, I. Szleifer and R. Podgornik, Biophys. J., 2014, 107, 1970–1979 CrossRef CAS PubMed.
- J. Zavadlav, R. Podgornik and M. Praprotnik, J. Chem. Theory Comput., 2015, 11, 5035–5044 CrossRef CAS PubMed.
-
P.-O. Löwdin, Quantum genetics and the aperiodic solid: Some aspects on the biological problems of heredity, mutations, aging, and tumors in view of the quantum theory of the DNA molecule, Uppsala University, Sweden, 1962 Search PubMed.
- V. Parsegian and T. Zemb, Curr. Opin. Colloid Interface Sci., 2011, 16, 618–624 CrossRef CAS.
- L.-Q. Fang, M. Goeijenbier, S.-Q. Zuo, L.-P. Wang, S. Liang, S. L. Klein, X.-L. Li, K. Liu, L. Liang and P. Gong, Viruses, 2015, 7, 333–351 CrossRef PubMed.
- B. D. Quinlan, H. Mou, L. Zhang, Y. Guo, W. He, A. Ojha, M. S. Parcells, G. Luo, W. Li, G. Zhong, H. Choe and M. Farzan, Immunity, 2020, 1–24, DOI:10.2139/ssrn.3575134.
- Q. Wang, L. Zhang, K. Kuwahara, L. Li, Z. Liu, T. Li, H. Zhu, J. Liu, Y. Xu and J. Xie, ACS Infect. Dis., 2016, 2, 361–376 CrossRef CAS PubMed.
- J. Zhao, Q. Yuan, H. Wang, W. Liu, X. Liao, Y. Su, X. Wang, J. Yuan, T. Li, J. Li, S. Qian, C. Hong, F. Wang, Y. Liu, Z. Wang, Q. He, Z. Li, B. He, T. Zhang, Y. Fu, S. Ge, L. Liu, J. Zhang, N. Xia and Z. Zhang, Clin. Infect. Dis., 2020, 1–22, DOI:10.1093/cid/ciaa344.
- B. Zhang, X. Zhou, C. Zhu, F. Feng, Y. Qiu, J. Feng, Q. Jia, Q. Song, B. Zhu and J. Wang, medRxiv, 2020, 1–15, DOI:10.1101/2020.03.12.20035048.
- H. Ma, W. Zeng, H. He, D. Zhao, Y. Yang, D. Jiang, P. Zhou, Y. Qi, W. He, C. Zhao, R. Yi, X. Wang, B. Wang, Y. Xu, Y. Yang, A. Kombe, C. Ding, J. Xie, Y. Gao, L. Cheng, Y. Li, X. Ma and T. Jin, medRxiv, 2020, 1–18, DOI:10.1101/2020.04.17.20064907.
- VASP – Vienna Ab initio Simulation Package, https://www.vasp.at/.
- J. P. Perdew, K. Burke and M. Ernzerhof, Phys. Rev. Lett., 1996, 77, 3865 CrossRef CAS PubMed.
-
W.-Y. Ching and P. Rulis, Electronic Structure Methods for Complex Materials: The orthogonalized linear combination of atomic orbitals, Oxford University Press, 2012 Search PubMed.
Footnote |
† Electronic supplementary information (ESI) available. See DOI: 10.1039/d0cp03145c |
|
This journal is © the Owner Societies 2020 |
Click here to see how this site uses Cookies. View our privacy policy here.