DOI:
10.1039/D0AN00150C
(Paper)
Analyst, 2020,
145, 3822-3831
Sensitive mass spectrometric analysis of carbonyl metabolites in human urine and fecal samples using chemoselective modification†
Received
19th January 2020
, Accepted 13th March 2020
First published on 12th May 2020
Abstract
Metabolites with ketone or aldehyde functionalities comprise a large proportion of the human metabolome, most notably in the form of sugars. However, these reactive molecules are also generated through oxidative stress or gut microbiota metabolism and have been linked to disease development. The discovery and structural validation of this class of metabolites over the large concentration range found in human samples is crucial to identify their links to pathogenesis. Herein, we have utilized an advanced chemoselective probe methodology alongside bioinformatic analysis to identify carbonyl-metabolites in urine and fecal samples. In total, 99 metabolites were identified in urine samples and the chemical structure for 40 metabolites were unambiguously validated using a co-injection procedure. We also describe the preparation of a metabolite-conjugate library of 94 compounds utilized to efficiently validate these ketones and aldehydes. This method was used to validate 33 metabolites in a pooled fecal sample extract to demonstrate the potential for rapid and efficient metabolite detection over a wide metabolite concentration range. This analysis revealed the presence of six metabolites that have not previously been detected in either sample type. The constructed library can be utilized for straightforward, large-scale, and expeditious analysis of carbonyls in any sample type.
Introduction
The surfaces and cavities of the human body are colonized by trillions of microbes that are heavily metabolically active and constantly exchange metabolites with other microbes and their human host. These communities have been termed microbiota. The link between microbiota dysbiosis, an altered microbial composition of gut microbiota species, and disease development has been demonstrated by state-of-the-art metagenomic studies.1,2 Microbiota cells outnumber those of their host and genomic analysis has revealed that these microbes possess approximately 400 times more genetic information than the human genome.3,4 This vast genomic information has a high enzymatic potential that lead to diverse microbiota-derived metabolites different from human metabolites. The selective discovery and analysis of these abundant metabolites has the potential not only to improve our understanding of the importance of microbiota and their role in disease development, but also to uncover new drug targets or markers for specific pathologies. A range of microbes that are commonly found in the human gut produce aldehydes and ketones via different biochemical reactions such as oxidation and reduction of metabolites.5 Endogenous aldehydes and ketones can also be generated through diverse metabolic processes e.g. lipid peroxidation, inflammation, ketogenesis and carbohydrate metabolism.6 Furthermore, many carbonyl-containing metabolites are excreted through the kidney and have been detected in urine samples.7
Metabolomics aims at investigating all metabolites present in any biological sample.8–10 Metabolite extracts can either be analyzed by NMR or diverse mass spectrometric techniques. Mass spectrometric analysis has become the method of choice as it enables detection and analysis with higher sensitivity than NMR analysis.11,12 Human fecal and urine samples are two major sample types containing metabolites resulting from mammalian clearance mechanisms. Fecal samples contain an abundance of gut microbiota-derived metabolites, many of which have been described to either have beneficial or toxic properties impacting human physiology.13,14 Urine samples are the end-point of human metabolism and especially interesting as it is an ideal source for the discovery of non-invasive biomarkers that can be readily translated into diagnostic tools.15–21
The detection of ketone and aldehyde molecules by liquid chromatography mass spectrometry (LC-MS) remains a challenge due to their poor ionization properties.22 The development of chemical biology tools in this newly developed research field is limited in comparison with other well-established ‘omics’ fields.23–27 These tools are required to allow for selective and advanced metabolite analysis. Chemoselective modification strategies for targeted carbonyl metabolite analysis represent an effective approach to facilitate detection and identification of a metabolite class of interest. Different derivatisation reagents, such as hydrazines and alkoxamines, or methods for chemoselective capture of ketone bodies for quantification with antibodies have been developed to facilitate the targeted discovery and analysis of unknown carbonyl metabolites in human samples.22,28–32
Mass spectrometric modification methods are typically limited by interference from the sample matrix and other metabolites, leading to ion suppression. To overcome these restraints, we have recently developed chemoselective probe 1.25,33 This probe can be charged with any reactive moiety to investigate metabolites in biological samples (Fig. 1A). This advanced chemical biology tool was immobilized to magnetic beads to efficiently separate captured metabolites from the sample matrix and unreacted metabolites using a magnet. This procedure leads to reduced mass spectrometric background interference and an enhanced level of detection of up to six orders of magnitude.25,33
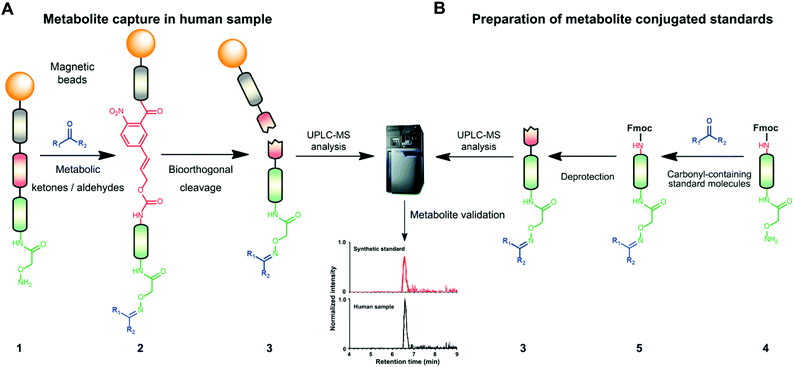 |
| Fig. 1 Chemoselective probe procedure and metabolite validation. (A) Procedure for the capture and extraction of carbonyl-containing metabolites from human samples. (B) Preparation of metabolite-conjugate standards (3) Validation of captured metabolites using metabolite-conjugate standards. Chemical structures are depicted in Schemes S1 and S2.† | |
Herein, we describe the chemoselective and mass spectrometric analysis of carbonyl-containing metabolites in urine and fecal samples collected from pancreatic cancer patients. This study represents the first application of this chemoselective probe methodology for analysis of urine samples. Furthermore, we have prepared a metabolite-conjugate library of 94 carbonyl-containing metabolites for structure validation in a pooled fecal sample to maximize metabolite coverage. This metabolite library is applicable to large scale carbonyl screening in any type of biological sample with enhanced mass spectrometric sensitivity.
Experimental section
General
All reagents and solvents were purchased from Sigma-Aldrich or Fischer Scientific and were used without further purification. A Fecal Metabolites Library was purchased from MetaSci. LC-MS grade solvents were used for UHPLC-ESI-MS analysis. Solutions were concentrated in vacuo on a Heidolph or an IKA rotary evaporator. Thin Layer Chromatography (TLC) was performed on silica gel 60 F-254 plates. Chromatographic purification of products was accomplished using flash column chromatography on Merck silica gel 60 (40–63 μm). All synthesized compounds were ≥95% pure as determined by NMR. NMR spectra were recorded on a Bruker 600 MHz spectrometer (1H NMR: 600.18 MHz, 13C NMR: 150.92 MHz), Agilent 400 MHz spectrometer (1H NMR: 399.97 MHz, 13C NMR: 100.58 MHz), or Varian 300 MHz spectrometer (13C NMR: 75.43 MHz). Chemical shifts are reported in parts per million (ppm) on the δ scale from an internal standard. Multiplicities are abbreviated as follows: s = singlet, d = doublet, t = triplet, q = quartet, m = multiplet. Glass vials used for handling magnetic beads were microwave vials from Biotage (0.2–0.5 mL or 0.5–2.0 mL). High-resolution mass spectra were acquired on a SYNAPT G2-S High Definition Mass Spectrometer (HDMS) using an electrospray ionization (ESI) source with an AQCUITY UPLC I-class system and equipped with a Waters ACQUITY UPLC BEH C18 column (2.1 × 75 mm, 1.7 μm particle size).
Ethical approval
Patient fecal and urine samples were obtained in accordance with the World Medical Association Declaration of Helsinki and all patients gave written informed consent. Approval for the study was obtained from the ethical committee at Karolinska Institutet Hospital (Ethical approval number: Dnr 2017/290-31). Fecal and urine samples were collected using routine clinical collection protocols and all patient codes have been removed in this publication. All samples were stored at −80 °C.
UHPLC-MS analysis
Mass spectrometric analysis was performed on an Acquity UPLC system connected to a Synapt G2 Q-TOF mass spectrometer, both from Waters Corporation (Milford, MA, USA). The system was controlled using the MassLynx software package v 4.1, also from Waters. The separation was performed on an Acquity UPLC® BEH C18 column (1.7 μm, 100 × 2.1 mm) from Waters Corporation. The mobile phase consisted of a combination of 0.1% formic acid in MilliQ water (A) and 0.1% formic acid in LC-MS grade methanol (B). The column temperature was 40 °C and the mobile phase gradient applied was as follows: 0–2 min, 0% B; 2–15 min, 0–100% B; 15–16 min, 100% B; 16–17 min, 100–0% B; 17–21 min, 0% B, with a flow rate of 0.2 ml min−1.
Samples were introduced into the Q-TOF using positive electrospray ionization. The capillary voltage was set to 2.50 kV and the cone voltage was 40 V. The source temperature was 100 °C, the cone gas flow 50 L min−1 and the desolvation gas flow 600 L h−1. The instrument was operated in MSE mode, the scan range was m/z = 50–1200, and the scan time was 0.3 s. A solution of sodium formate (0.5 mM in 2-propanol: water, 90
:
10, v/v) was used to calibrate the instrument and a solution of leucine-encephalin (2 ng μl−1 in acetonitrile: 0.1% formic acid in water, 50
:
50, v/v) was used for the lock mass correction at an injection rate of 30 s.
Six LC-MS injections each were analyzed for the bead cleavage product and for the control sample (fecal sample) as well as three injections for the urine sample experiment and the corresponding control sample. For the first 90 s of the analysis, the output of the UHPLC system was diverted to the waste.
Activation of carbonyl-specific chemoselective probe 8
The bead-bound, unactivated probe was prepared as reported previously.25,33 The unactivated probe 8 (320 nmol) were suspended in a solution of (Boc-aminooxy) acetic acid NHS ester 9 (10 mg, 37.4 μmol), EDCI (10 mg, 64.4 μmol) and DIPEA (10 μL, 104.2 μmol) in DCM (300 μL). The suspension was agitated at 25 °C in a ThermoMixer (1500 rpm) for 16 h. After the reaction was completed, the supernatant was removed, and the beads were washed with THF (3 × 300 μL). The beads were then suspended in a solution of TFA (300 μL) and agitated in a ThermoMixer (1500 rpm) for 3 h. The beads were then washed with phosphate buffer (2 × 300 μL, 50 mM, pH 6.5).
Preparation of fecal metabolite extracts
A scalpel was used to collect approximately 30 mg of the frozen fecal sample (stored at 80 °C). The sample was transferred into a tube containing specified matrix D (MP Biomedicals) and dissolved in 100 μL ultrapure water and 400 μL LC-MS grade MeOH. The mixture was vortexed and subsequently homogenized by a FastPrep 24 homogenizer (3 cycles, 6.5 m s−1, 40 s, MP Biomedicals). The mixture was stored at −20 °C for at least 1 h for precipitation before the centrifugation (18
620g, 5 min, 4 °C). The supernatant was taken out from tube D into Eppendorf tubes, and the solvent volume was reduced from the supernatant through vacuum centrifugation. The residue was suspended in phosphate buffer (50 μL, pH 6.5, 50 mM) and combined into one Eppendorf tube.
Preparation of urine metabolite extracts
Ice cold methanol (400 μL) was added to urine sample aliquots (100 μL) for protein precipitation. Each sample was vigorously shaken for 30 s and then cooled at 4 °C for 30 min. The supernatant was taken out and transferred into empty Eppendorf tubes and the solvents were removed from the supernatant through vacuum centrifugation. The residue was redissolved in phosphate buffer (200 μL, pH 6.5, 50 mM).
Treatment of fecal or urine metabolite extracts
The activated beads 1 were used to treat the fecal extract (8 × 50 μL in pH 6.5 phosphate buffer, 50 mM) or each urine extract (200 μL in pH 6.5 phosphate buffer, 50 mM,). The mixture was shaken for 16 h at 1500 rpm and 25 °C (Scheme S1†). The fecal extract solution was removed from the beads, which were washed with THF (2 × 300 μL) before resuspension in THF (300 μL).
Bioorthogonal cleavage of Noc
The suspension of beads was transferred to a glass vial. Triphenylphosphine (97.0 μL, 12.9 mM in THF, 1.25 μmol) and dimethylbarbituric acid (90.0 μL, 30.7 mM in THF, 2.76 μmol) solutions were added to the vial, followed by palladium(II) acetate solution (84.0 μL, 6.53 mM in THF, 549 nmol). The vial was quickly sealed, and a stream of nitrogen was passed through until approximately half the volume of the suspension remained. The vial was agitated at intervals with vigorous shaking and the reaction was allowed to continue for 5 h. In parallel, a sample of unmodified beads was treated under the same cleavage conditions as the activated beads treated with fecal or urine extract and used as control sample. The supernatant was removed from the beads using magnetic separation and the solvent was removed using a vacuum centrifuge. The residues were redissolved in MeOH (30 μL each) and triphenylphosphine as well as triphenylphosphine oxide were precipitated through the addition of water (120 μL each). The suspension was centrifuged (benchtop centrifuge, 12
000g, 5 min), the supernatant transferred, and the solvent again removed using a centrifuge. The residues were redissolved with a water/acetonitrile solution (95
:
5 v/v) and submitted for UPLC-MS analysis.
Method validation
Metabolites were extracted from a urine sample aliquot (100 μL) as described in the above Experimental section. The solvent was removed from the supernatant by vacuum centrifugation and the residue was redissolved in phosphate buffer (290 μL, pH 6.5, 50 mM). The two water samples (100 μL) were prepared in parallel using the same procedure as control samples. A butanone solution (10 μL of a 30 μM butanone stock solution) was spiked into each of these three samples, followed by chemoselective probe treatment and bioorthogonal cleavage as described before. An internal standard mixture (10 μL, 10 μg mL−1 phenylalanine-13C9 and 20 μg mL−1 benzoic acid-13C6) was spiked into each solution. Samples were analyzed by UPLC-MS analysis. Peak areas for each extracted ion chromatogram were integrated using MassLynx 4.1 (waters). The recovery rate was calculated by comparing the peak area ratios of the urine with the average of both aqueous samples (Table S4†).
Preparation of Fmoc-protected simplified chemical probe 12
Simplified chemical probe 12 was prepared as previously reported in an optimized adaptation of our previously reported procedure. Compound 11 (5 mg, 11.2 μmol) was combined with (Boc-aminooxy) acetic acid NHS ester (5 mg, 27.4 μmol) in DCM (1 ml).33 EDCI (5.3 mg, 33.7 μmol) and DIPEA (11.8 μL, 33.7 μmol) were added into the reaction solution before it was stirred at room temperature for 18 h. The solvents were removed under reduced pressure and the residue was purified by flash column chromatography (5
:
95 MeOH/DCM rising to 1
:
9 MeOH/DCM) to yield the product as a white solid (6.3 mg, 91%).
Preparation of metabolite-conjugated standards
A solution of the Fmoc-protected probe 12 (50 μl, 1.0 mM in MeOH, 50 nmol) was evaporated under reduced pressure. The residue was combined with DCM (50 μl) and TFA (100 μl). The solution was shaken at 1500 rpm for 2 h, before the solvents were removed under reduced pressure. The residue was then combined with a solution of a single aldehyde/ketone standard (10 equiv. each in 400 μl, 50 mM, pH 6.5 ammonium acetate buffer). The resulting solution was then shaken at 1500 rpm for 18 h at 25 °C. The solvents were then removed under reduced pressure, and the residues were treated with piperidine (100 μl) and shaken at 1500 rpm for 4 h at 25 °C. The piperidine was then removed under reduced pressure, and the residue was redissolved in MeOH (100 μl) followed by water (400 μl). The solution was diluted as necessary in a solution of water and acetonitrile (95
:
5 v/v) before being submitted for UPLC-MS analysis (Scheme S2†).
Data analysis
Data files from the LC-MS analysis were converted into the NetCDF file format using the Databridge software from MassLynx 4.1 (Waters). The XCMS software was used to perform peak detection and alignment of the LC-MS chromatograms, in R studio.34,35 Data processing was performed by excluding all the m/z value with less than 279.1451 (the m/z value corresponding to the monoprotonated probe with no captured metabolite). Features more abundant in the control sample and less than five-fold increase in the feces sample set were eliminated from the data analysis. From each feature 279.1451 Da were subtracted (corresponding to the mass of the probe) and compared to the human metabolome database in order to find plausible candidates for the parent metabolites. Metabolite validation was performed using MassLynx 4.1 (Waters). Based on the mass and retention time from metabolite-conjugates library, the extracted ion chromatogram (EIC) for each metabolite conjugate was used to confirm the identity of the corresponding metabolite and the correct regioisomers.
Results and discussion
Chemoselective probe procedure
The key advantage of our immobilized chemical probe to magnetic beads is the bioorthogonal cleavage site p-nitrocinnamyloxy-carbonyl (Noc, depicted in compound 2) that can be cleaved under mild conditions using palladium (0) catalysis to avoid conversion of labile functional groups (Fig. 1A).36 This is in stark contrast to other bioorthogonal cleavage sites, which typically require harsh UV irradiation, or acidic or basic conditions.37 We have also developed a straightforward synthetic approach to prepare reference compounds for validation of their chemical structures (Fig. 1B). The corresponding carbonyl-containing metabolites were conjugated with simplified probe 4 and reference conjugates 3 obtained after deprotection of the Fmoc-protecting group.
In this study, we have further exploited the properties of this chemoselective probe procedure for analysis with enhanced mass spectrometric sensitivity of carbonyl-containing metabolites in human fecal and urine samples. The full-length chemical probe was immobilized to carboxylic acid-activated magnetic beads followed by deprotection of the Boc group through treatment with trifluoroacetic acid.33 The probe was then charged with the Boc-protected alkoxyamine moiety using peptide coupling conditions and activated through Boc-deprotection (Fig. 1A). Activated chemical probe 1 was incubated with metabolite extracts prepared from individual urine samples or a pooled fecal sample (Fig. 2A). After incubation, the captured carbonyl-containing metabolites 2 were separated from the unreacted metabolite mixture using a magnet and cleaved under mild and bioorthogonal conditions using Pd(0) catalysis. Released metabolite-conjugates 3 were analyzed by UPLC-MS followed by either (i) bioinformatic data analysis using the XCMS metabolomics R package or (ii) comparison with a prepared metabolite library (Fig. 2A).
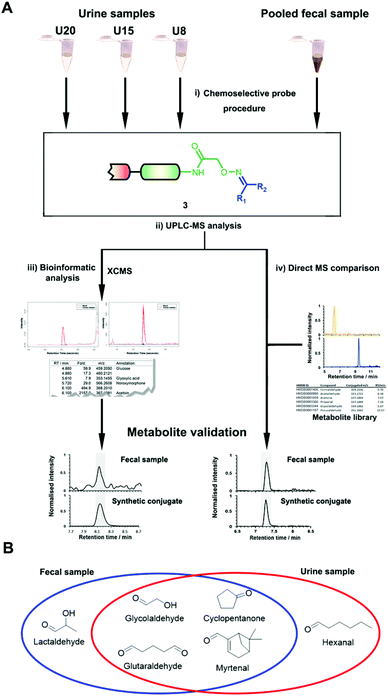 |
| Fig. 2 (A) Overview of the analysis of human samples using the chemoselective probe methodology using either a bioinformatic workflow (left) or comparison with a metabolite library. A pooled fecal sample constituted from eight samples and three individual urine samples were analyzed. (i) Chemoselective probe treatment; (ii) UPLC-MS analysis of captured metabolites; (iii) bioinformatic analysis using XCMS; and (iv) metabolite validation using synthetic metabolite conjugate standards through co-injection experiments. (B) Discovered and validated new metabolites in each sample type that have not been report before according to HMDB. | |
Carbonyl-metabolite analysis in urine samples
Urinary carbonyl-containing metabolites have so far not been analyzed in the context of pancreatic cancer. As carbonyl-containing compounds are highly reactive and can interact with other biomolecules, leading to the degradation of regulatory proteins and enzymes as well as DNA modifications we have applied our method to the analysis of patient urine samples.38 Several urinary aldehydes/ketones have been linked to the development of other diseases, for example, 3-hydroxykynurenine has been suggested to be a potential urinary biomarker for Alzheimer's disease,39 while other studies have proposed that urinary metabolites such as cortisol, 11-deoxycortisol, and 21-deoxycortisol, should be considered as biomarkers for Parkinson's disease.40 Formaldehyde and acetaldehyde have also been classified as group 1 human carcinogens by the International Agency for Research on Cancer (IARC) due to their carcinogenic properties through DNA adduct formation that dysregulates cellular growth.41 Malondialdehyde is a well-known biomarker for oxidative stress as it is a metabolic end product from the oxidation of lipids.42
To explore carbonyl-compounds in pancreatic cancer patients, we have now utilized our chemoselective procedure for analysis of this compound class in human urine samples (Fig. 2A). Bioinformatic analysis of the metabolomics dataset revealed on average 1191 significantly altered features in the three samples. These features were reduced by excluding those which had either: (1) A mass smaller than the linker adduct of 279.1451 Da. (2) More abundant features in the control sample. (3) Less than five-fold more abundant in the treated sample. The candidate features were annotated by searching the Human Metabolome Database (HMDB) and METLIN for chemical structures with matching molecular masses.35,43 These filters led to a total of 99 ketones and aldehydes (Table S1†), which are more metabolites compared to other studies of carbonyl metabolites in human urine samples.29,44,45 The structure of metabolites was validated through preparation of conjugate metabolites. This procedure requires commercially available carbonyl-containing metabolites that were incubated with simplified probe 4 (Fig. 1B). This was followed by removal of the Fmoc-protecting group of intermediate 5, removal of the solvent, reconstitution, and subsequent UPLC-MS analysis of each conjugate 3 separately. It is of importance to note that the preparation of compounds with the general structure 3 follows a simple and efficient procedure using a low volume of 100 μL and a reaction time of only 24 h and does not require any purification step.
In total, we have validated 40 metabolites present in at least one of these three samples through co-injection experiments with these synthetic conjugate-standards. This number is higher than previous reports,46 which stems from our method separating the captured metabolites from the matrix as well an increase in mass spectrometric sensitivity by several orders-of-magnitude. Of these compounds, 35 have been reported previously in human samples according to the Human Metabolome Database (HMDB).43 The high sensitivity of our methods led to the discovery and confirmation of the five metabolites glycolaldehyde, cyclopentanone, glutaraldehyde, hexanal, and myrtenal, which have not yet been reported in this human sample type and demonstrates the versatility of our method (Fig. 2B). Examples of the co-injection experiments for structure validation are given in Fig. S1.† We have also determined the recovery of our procedure to be 47–58% using butanone as a model compound that was not present in analyzed urine samples (Table S4†).43 This recovery rate is consistent with comparable methods and confirms the high coverage of metabolites detected using our qualitative discovery method.47,48
Our analysis also revealed a total of 13 metabolites that were detected in all three samples including acetone, propanal, glycolaldehyde, pyruvic acid, hydroxyacetone, cyclopentanone, glyceraldehyde, cyclohexanone, 4-hydroxyphenylpyruvic acid, retinal, cortexolone, tolualdehyde, and daidzein (Table 1). With the exception of cyclopentanone, these metabolites are commonly produced in the human body or consumed through diet. An overview of captured metabolites has been compiled detailing metabolic sources and biological importance (Table S3†).43 While six metabolites were present in two of these patients, 21 metabolites were only detected in one of these three individuals. Comparison of the carbonyl-content in a larger sample cohort from individuals and pancreatic cancer patients can be performed to identify pattern for selective biomarkers or reveal individual metabolic fingerprints.
Table 1 Validated carbonyl-containing metabolites in human urine samples sorted by molecular weight
Construction of a carbonyl-metabolite conjugate library
One challenge of metabolomics analysis is the validation of exact chemical structures of regioisomers.49,50 Metabolites that have been catalogued in databases such as HMDB, Sirius, MZmine, and Metlin can be identified through MS/MS fragmentation comparison.35,43,51–56 A second compound validation method is co-injection of a synthetic or commercially available reference compound with the natural metabolite to compare the chromatographic and mass spectrometric properties and unambiguously distinguish regioisomers.
Our method was also developed to unequivocally distinguish and validate the chemical structure of metabolites through co-injection of synthetic standards with the human sample for validation of a series of known and unknown metabolites.25,33 As aldehydes and ketones are important metabolites present in most mammalian sample types and metabolomics studies are aimed at analysing as many metabolites as possible in parallel, we further expanded the number of synthetic metabolite conjugate standards to 94. For a comprehensive analysis of microbiome metabolism, we have also prepared standards for 39 aldehyde/ketone-containing metabolites that are part of a commercially available Fecal Metabolites Library.
Conjugated standards were prepared as described vide supra using the activated simplified chemical probe 4. Each metabolite was conjugated and after Fmoc-deprotection analysed separately by UPLC-MS (Fig. 1 and 3A). Our library list is composed of the monoisotopic mass of the conjugate, the retention time and the HMDB ID number through analysis of each extracted ion chromatogram (EIC) (Table S2†). The reaction of hydroxylamines with carbonyl compounds can in some cases produce geometric isomers; where both E and Z isomers were observed the stronger signal was taken into consideration. The library contains a variety of compound classes including sugars, aliphatic aldehydes/ketones, and coenzymes.
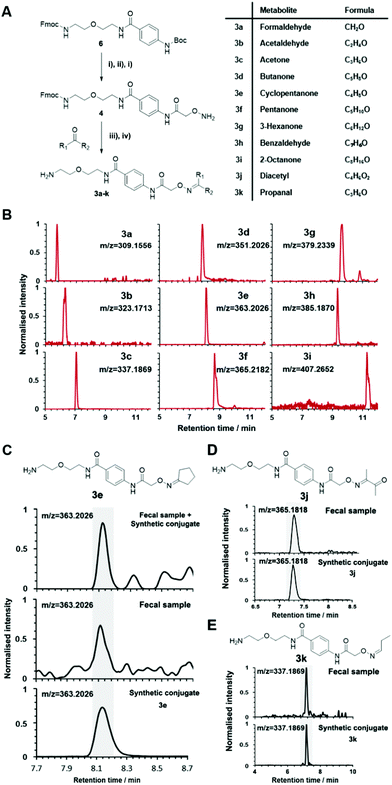 |
| Fig. 3 Synthesis of conjugates 3a–k as part of the reference library. (A) General chemical synthesis of metabolite conjugates 3. (i) TFA, DCM, 3 h, 25 °C; (ii) (Boc-aminooxy)acetic acid NHS ester, EDC, DIPEA, DCM, 18 h, 25 °C; (iii) 50 mM NH4OAc buffer, pH 6.5, 18 h, 25 °C; (iv) piperidine, 4 h, 25 °C. (B) Extracted ion chromatograms (EIC) of representative conjugated metabolite standards 3a–i. (C) Co-injection experiment of cyclopentanone conjugate 3e for validation in fecal samples. (D) Metabolite validation of diacetyl 3j. (E) Metabolite validation of propanal with conjugate 3k. | |
This library also contains several metabolites derived from microbiome-metabolism including e.g. acetone, arabinose, rhamnose, and trehalose, which have been linked to different diseases including Parkinson's disease, lung cancer, and colorectal cancer.43 Volatile and small metabolites are usually inaccessible on an LC-MS system and in common metabolomics analyses. Our method facilitates this analysis and enabled us to include metabolites such as formaldehyde, acetaldehyde and propanal. An example of the strength of our method is demonstrated through the resolution of the regioisomers o-tolualdehyde, p-tolualdehyde, and m-tolualdehyde (Fig. S2†). The EIC traces for each individual metabolite from the LC-MS analysis results in chromatographic separation that can be used for accurate assignment of these regioisomers in human samples. For metabolites with two-carbonyl moieties, we also recorded the chromatographic and mass spectrometric properties for the bis-labeled metabolite (Fig. S3†).
Our constructed reference library of 94 metabolite conjugates can be efficiently utilized for expeditious and comprehensive analysis of these metabolically important compounds in any sample type (Fig. 3B). Furthermore, our efficient procedure for the preparation of new metabolite conjugates allows this library to be readily extended to include new molecules of interest.
Comprehensive carbonyl-metabolite screening in pooled fecal samples
The versatility of this metabolite library was demonstrated by tested a fecal sample pooled from eight different patients to discover metabolic ketones and aldehydes derived from microbiota metabolism. Microbiota dysbiosis has been linked to pancreatic cancer through metagenomic analysis.57–59 Thus, metabolic investigation of fecal microbiota-derived metabolites is a crucial step towards understanding the role of microbes in the pathogenesis of pancreatic cancer. This strategy has a high potential to discover unknown bioactive metabolites and/or biomarkers.60–62 This study extends on our previous study detailing individual difference of carbonyl metabolites of three patients in this sample type.
Each fecal sample was separately extracted using a bead-based homogenizer. The supernatants of these metabolite extracts were combined in phosphate buffer and incubated with activated carbonyl-specific probe 1 following our metabolite capturing procedure. Upon LC-MS based analysis of the captured and released metabolite conjugates, we compared the obtained features with the recorded conjugate library data. Comparison of retention times and m/z values of the metabolite library and the dataset led to validation of 33 carbonyl-containing metabolites, which is about two-fold higher than validated in individual samples (Tables 2 and S2†).33 Each metabolite was validated through co-injection experiments of the fecal sample extract with the corresponding reference compounds (Fig. S4†). Most of these metabolites are known components of fecal samples. An overview of captured metabolites has been compiled detailing metabolic sources and biological importance (Table S3†). However, our procedure also identified five metabolites – glycolaldehyde, lactaldehyde, glutaraldehyde, myrtenal, and cyclopentanone – that until now have not been detected in human fecal samples. Examples of these co-injection experiments of these synthetic conjugates are presented for cyclopentanone 3e, diacetyl 3j, and propanal 3k (Fig. 3C–E).
Table 2 Validated carbonyl-containing metabolites in human fecal samples sorted by molecular weight
|
Carbonyl standard |
Monoisotopic mass |
Conjugated m/z |
Retention time/min |
Standard |
Fecal sample |
1 |
Formaldehyde |
30.0105 |
309.1556 |
5.70 |
5.68 |
2 |
Acetaldehyde |
44.0262 |
323.1713 |
6.34 |
6.42 |
3 |
Acetone |
58.0418 |
337.1869 |
7.07 |
7.12 |
4 |
Propanal |
58.0418 |
337.1869 |
7.16 |
7.16 |
5 |
Glycolaldehyde |
60.0211 |
339.1662 |
5.87 |
5.87 |
6 |
Butanone |
72.0575 |
351.2026 |
7.83 |
7.84 |
7 |
Butanal |
72.0575 |
351.2026 |
8.06 |
8.06 |
8 |
Hydroxyacetone |
74.0367 |
353.1818 |
6.32 |
6.25 |
9 |
DL-Lactaldehyde |
74.0367 |
353.1818 |
6.56 |
6.63 |
10 |
Cyclopentanone |
84.0575 |
363.2026 |
8.10 |
8.00 |
11 |
Diacetyl |
86.0367 |
365.1818 |
7.28 |
7.26 |
12 |
Valeraldehyde |
86.0731 |
365.2183 |
9.28 |
9.27 |
13 |
Pyruvic acid |
88.0160 |
367.1611 |
6.63 |
6.62 |
14 |
Acetoin |
88.0524 |
367.1975 |
6.64 |
6.62 |
15 |
Cyclohexanone |
98.0731 |
377.2182 |
8.98 |
9.00 |
16 |
β-Hydroxypyruvic acid |
104.0109 |
383.1560 |
5.13 |
5.12 |
17 |
Erythrose |
120.0422 |
399.1873 |
5.10 |
5.08 |
18 |
Acetophenone |
120.0575 |
399.2026 |
9.47 |
9.53 |
19 |
4-Hydroxybenzaldehyde |
122.0367 |
401.1818 |
8.00 |
7.99 |
20 |
trans-Cinnamaldehyde |
132.0575 |
411.2026 |
10.18 |
10.20 |
21 |
L-Arabinose/ribose |
150.0528 |
429.1979 |
5.21 |
5.19 |
22 |
(1R)-Myrtenal |
150.1044 |
429.2495 |
11.44 |
11.45 |
23 |
Rhamnose |
164.0684 |
443.2135 |
5.42 |
5.39 |
24 |
Menadione |
172.0524 |
451.1975 |
5.16 |
5.17 |
25 |
4-Hydroxyphenylpyruvic acid |
180.0422 |
459.1873 |
9.24 |
9.23 |
26 |
D-Fructose/D-glucose |
180.0633 |
459.2084 |
5.08 |
5.09 |
27 |
D-Allose |
180.0633 |
459.2084 |
5.17 |
5.19 |
29 |
Daidzein |
254.0579 |
533.2030 |
8.46 |
8.51 |
30 |
D-Glucose 6-phosphate |
258.0151 |
537.1602 |
5.22 |
5.23 |
31 |
Retinal |
284.2140 |
563.3591 |
14.35 |
14.38 |
32 |
Luteolin |
286.0477 |
565.1928 |
7.58 |
7.54 |
33 |
Cortexolone |
346.2144 |
625.3595 |
11.38 |
11.41 |
Conclusions
In summary, our chemoselective modification method was applied for the first time to the analysis of carbonyl compounds in urine samples and led to the discovery of five previously undetected metabolites. We also report the construction of a metabolite conjugation library of 94 ketone- or aldehyde-containing metabolites for unambiguous structure validation, and demonstrated its utility by applying it to the analysis of a pooled fecal sample in which we discovered five metabolites not previously known to be found in feces. These results demonstrate that our bipartite approach using our chemoselective probe combined with either sophisticated bioinformatic analysis or a unique conjugation library allows for the discovery of unknown as well as the validation of known metabolites in complex human samples. This study lays the foundation for selective metabolomics analysis of carbonyl-containing compounds at low concentrations in any sample type and the discovery of biomarkers of disease.
Conflicts of interest
There are no conflicts to declare.
Acknowledgements
This study was funded by the Swedish Research Council (VR 2016-04423), Carl Tryggers Foundation (CTS 2016:155/CTS 2018:820) and a generous start-up grant from the Science for Life Laboratory (to D.G.). We thank the staff at Karolinska University Hospital for collecting the samples.
Notes and references
- E. Y. Hsiao, S. W. McBride, S. Hsien, G. Sharon, E. R. Hyde, T. McCue, J. A. Codelli, J. Chow, S. E. Reisman, J. F. Petrosino, P. H. Patterson and S. K. Mazmanian, Cell, 2013, 155, 1451–1463 CrossRef CAS PubMed.
-
V. D. Appanna, in Human Microbes - The Power Within: Health, Healing and Beyond, ed. V. D. Appanna, Springer Singapore, Singapore, 2018, DOI:10.1007/978-981-10-7684-8_1, pp. 1–36.
- J. K. Nicholson, E. Holmes, J. Kinross, R. Burcelin, G. Gibson, W. Jia and S. Pettersson, Science, 2012, 336, 1262–1267 CrossRef CAS PubMed.
- M. S. Donia and M. A. Fischbach, Science, 2015, 349, 1254766 CrossRef PubMed.
- I. Rowland, G. Gibson, A. Heinken, K. Scott, J. Swann, I. Thiele and K. Tuohy, Eur. J. Nutr., 2018, 57, 1–24 CrossRef CAS PubMed.
- M. Singh, A. Kapoor and A. Bhatnagar, Chem.-Biol. Interact., 2015, 234, 261–273 CrossRef CAS PubMed.
- W. Jumpathong, W. Chan, K. Taghizadeh, I. R. Babu and P. C. Dedon, Proc. Natl. Acad. Sci. U. S. A., 2015, 112, E4845–E4853 CrossRef CAS PubMed.
- K. Dettmer, P. A. Aronov and B. D. Hammock, Mass Spectrom. Rev., 2007, 26, 51–78 CrossRef CAS PubMed.
- R. R. da Silva, P. C. Dorrestein and R. A. Quinn, Proc. Natl. Acad. Sci. U. S. A., 2015, 112, 12549–12550 CrossRef CAS PubMed.
- C. H. Johnson, J. Ivanisevic and G. Siuzdak, Nat. Rev. Mol. Cell Biol., 2016, 17, 451–459 CrossRef CAS PubMed.
- N. L. Kuehnbaum and P. Britz-McKibbin, Chem. Rev., 2013, 113, 2437–2468 CrossRef CAS PubMed.
- Z. J. Zhu, A. W. Schultz, J. Wang, C. H. Johnson, S. M. Yannone, G. J. Patti and G. Siuzdak, Nat. Protoc., 2013, 8, 451–460 CrossRef CAS PubMed.
- K. S. Smirnov, T. V. Maier, A. Walker, S. S. Heinzmann, S. Forcisi, I. Martinez, J. Walter and P. Schmitt-Kopplin, Int. J. Med. Microbiol., 2016, 306, 266–279 CrossRef CAS PubMed.
- S. Yan, J. Huang, Z. Chen, Z. Jiang, X. Li and Z. Chen, Sci. Bull., 2016, 61, 1151–1153 CrossRef CAS.
- D. Globisch, L. M. Eubanks, R. J. Shirey, K. M. Pfarr, S. Wanji, A. Y. Debrah, A. Hoerauf and K. D. Janda, Bioorg. Med. Chem. Lett., 2017, 27, 3436–3440 CrossRef CAS PubMed.
- D. Globisch, A. Y. Moreno, M. S. Hixon, A. A. K. Nunes, J. R. Denery, S. Specht, A. Hoerauf and K. D. Janda, Proc. Natl. Acad. Sci. U. S. A., 2013, 110, 4218–4223 CrossRef CAS PubMed.
- R. J. Shirey, D. Globisch, L. M. Eubanks, M. S. Hixon and K. D. Janda, ACS Infect. Dis., 2018, 4, 1423–1431 CrossRef CAS PubMed.
- E. J. Saude, D. Adamko, B. H. Rowe, T. Marrie and B. D. Sykes, Metabolomics, 2007, 3, 439–451 CrossRef CAS.
- W. Zou, J. She and V. V. Tolstikov, Metabolites, 2013, 3, 787–819 CrossRef PubMed.
- M. M. Khamis, D. J. Adamko and A. El-Aneed, Mass Spectrom. Rev., 2017, 36, 115–134 CrossRef CAS PubMed.
- M. Monteiro, N. Moreira, J. Pinto, A. S. Pires-Luis, R. Henrique, C. Jeronimo, M. L. Bastos, A. M. Gil, M. Carvalho and P. Guedes de Pinho, J. Cell. Mol. Med., 2017, 21, 2092–2105 CrossRef CAS PubMed.
- D. Siegel, A. C. Meinema, H. Permentier, G. Hopfgartner and R. Bischoff, Anal. Chem., 2014, 86, 5089–5100 CrossRef CAS PubMed.
- C. Ballet, M. S. P. Correia, L. P. Conway, T. L. Locher, L. C. Lehmann, N. Garg, M. Vujasinovic, S. Deindl, J. M. Lohr and D. Globisch, Chem. Sci., 2018, 9, 6233–6239 RSC.
- M. S. P. Correia, M. Rao, C. Ballet and D. Globisch, ChemBioChem, 2019, 20, 1678–1683 CrossRef CAS PubMed.
- N. Garg, L. P. Conway, C. Ballet, M. S. P. Correia, F. K. S. Olsson, M. Vujasinovic, J. M. Lohr and D. Globisch, Angew. Chem., Int. Ed., 2018, 57, 13805–13809 CrossRef CAS PubMed.
- S. L. Capehart and E. E. Carlson, Chem. Commun., 2016, 52, 13229–13232 RSC.
- E. E. Carlson and B. F. Cravatt, Nat. Methods, 2007, 4, 429–435 CrossRef CAS PubMed.
- P. Deng, R. M. Higashi, A. N. Lane, R. C. Bruntz, R. C. Sun, M. V. Ramakrishnam Raju, M. H. Nantz, Z. Qi and T. W. Fan, Analyst, 2017, 143, 311–322 RSC.
- S. Zhao, M. Dawe, K. Guo and L. Li, Anal. Chem., 2017, 89, 6758–6765 CrossRef CAS PubMed.
- E. E. Carlson and B. F. Cravatt, J. Am. Chem. Soc., 2007, 129, 15780–15782 CrossRef CAS PubMed.
- E. F. Holmquist, U. B. Keiding, R. Kold-Christensen, T. Salomon, K. A. Jorgensen, P. Kristensen, T. B. Poulsen and M. Johannsen, Anal. Chem., 2017, 89, 5066–5071 CrossRef CAS PubMed.
- R. Kold-Christensen, K. K. Jensen, E. Smedegard-Holmquist, L. K. Sorensen, J. Hansen, K. A. Jorgensen, P. Kristensen and M. Johannsen, Redox Biol., 2019, 26, 101252 CrossRef CAS PubMed.
- L. P. Conway, N. Garg, W. Lin, M. Vujasinovic, J. M. Lohr and D. Globisch, Chem. Commun., 2019, 55, 9080–9083 RSC.
- R. Tautenhahn, G. J. Patti, D. Rinehart and G. Siuzdak, Anal. Chem., 2012, 84, 5035–5039 CrossRef CAS PubMed.
- X. Domingo-Almenara, J. R. Montenegro-Burke, J. Ivanisevic, A. Thomas, J. Sidibe, T. Teav, C. Guijas, A. E. Aisporna, D. Rinehart, L. Hoang, A. Nordstrom, M. Gomez-Romero, L. Whiley, M. R. Lewis, J. K. Nicholson, H. P. Benton and G. Siuzdak, Nat. Methods, 2018, 15, 681–684 CrossRef CAS PubMed.
- H. Kunz and J. März, Angew. Chem., Int. Ed. Engl., 1988, 27, 1375–1377 CrossRef.
- G. Leriche, L. Chisholm and A. Wagner, Bioorg. Med. Chem., 2012, 20, 571–582 CrossRef CAS PubMed.
- A. Phaniendra, D. B. Jestadi and L. Periyasamy, Indian J. Clin. Biochem., 2015, 30, 11–26 CrossRef CAS PubMed.
- K. Fukuhara, A. Ohno, Y. Ota, Y. Senoo, K. Maekawa, H. Okuda, M. Kurihara, A. Okuno, S. Niida, Y. Saito and O. Takikawa, J. Clin. Biochem. Nutr., 2013, 52, 133–138 CrossRef CAS PubMed.
- M. An and Y. Gao, Genomics, Proteomics Bioinf., 2015, 13, 345–354 CrossRef PubMed.
- S. S. Hecht, P. Upadhyaya and M. Wang, Chem. Res. Toxicol., 2011, 24, 781–790 Search PubMed.
- F. Ito, Y. Sono and T. Ito, Antioxidants, 2019, 8, 72 Search PubMed.
- D. S. Wishart, Y. D. Feunang, A. Marcu, A. C. Guo, K. Liang, R. Vázquez-Fresno, T. Sajed, D. Johnson, C. Li, N. Karu, Z. Sayeeda, E. Lo, N. Assempour, M. Berjanskii, S. Singhal, D. Arndt, Y. Liang, H. Badran, J. Grant, A. Serra-Cayuela, Y. Liu, R. Mandal, V. Neveu, A. Pon, C. Knox, M. Wilson, C. Manach and A. Scalbert, Nucleic Acids Res., 2018, 46, D608–D617 CrossRef CAS PubMed.
- K. Rigdova, Y. Wang, M. Ward and W. J. Griffiths, Biochem. Biophys. Res. Commun., 2014, 446, 762–767 CrossRef CAS PubMed.
- Y. Lu, D. Yao and C. Chen, Metabolites, 2013, 3, 993–1010 CrossRef PubMed.
- L. Yu, P. Liu, Y. L. Wang, Q. W. Yu, B. F. Yuan and Y. Q. Feng, Analyst, 2015, 140, 5276–5286 RSC.
- D. J. Trader and E. E. Carlson, Org. Lett., 2011, 13, 5652–5655 CrossRef CAS PubMed.
- A. Y. Odendaal, D. J. Trader and E. E. Carlson, Chem. Sci., 2011, 2, 760–764 RSC.
- E. L. Schymanski, J. Jeon, R. Gulde, K. Fenner, M. Ruff, H. P. Singer and J. Hollender, Environ. Sci. Technol., 2014, 48, 2097–2098 CrossRef CAS PubMed.
- N. Garg, A. Hansson, H. K. Knych, S. D. Stanley, M. Thevis, U. Bondesson, M. Hedeland and D. Globisch, Org. Biomol. Chem., 2018, 16, 698–702 RSC.
- M. Katajamaa, J. Miettinen and M. Oresic, Bioinformatics, 2006, 22, 634–636 CrossRef CAS PubMed.
- F. Olivon, G. Grelier, F. Roussi, M. Litaudon and D. Touboul, Anal. Chem., 2017, 89, 7836–7840 CrossRef CAS PubMed.
- T. Pluskal, S. Castillo, A. Villar-Briones and M. Oresic, BMC Bioinf., 2010, 11, 395 CrossRef PubMed.
- K. Duhrkop, K. Scheubert and S. Bocker, Metabolites, 2013, 3, 506–516 CrossRef PubMed.
- M. Dowling, J. Wenskovitch, J. T. Fry, S. Leman, L. House and C. North, IEEE Trans. Vis. Comput. Graph, 2018, 25, 172–182 Search PubMed.
- C. Guijas, J. R. Montenegro-Burke, X. Domingo-Almenara, A. Palermo, B. Warth, G. Hermann, G. Koellensperger, T. Huan, W. Uritboonthai, A. E. Aisporna, D. W. Wolan, M. E. Spilker, H. P. Benton and G. Siuzdak, Anal. Chem., 2018, 90, 3156–3164 CrossRef CAS PubMed.
- C. P. Zambirinis, S. Pushalkar, D. Saxena and G. Miller, Cancer J., 2014, 20, 195–202 CrossRef CAS PubMed.
- I. Dickson, Nat. Rev. Gastroenterol. Hepatol., 2018, 15, 328 CrossRef PubMed.
- V. S. Akshintala, R. Talukdar, V. K. Singh and M. Goggins, Clin. Gastroenterol. Hepatol., 2019, 17, 290–295 CrossRef CAS PubMed.
- A. Milshteyn, D. A. Colosimo and S. F. Brady, Cell Host Microbe, 2018, 23, 725–736 CrossRef CAS PubMed.
- J. F. Martin and P. Liras, Curr. Opin. Pharmacol., 2019, 48, 8–16 CrossRef CAS PubMed.
- M. M. Rinschen, J. Ivanisevic, M. Giera and G. Siuzdak, Nat. Rev. Mol. Cell Biol., 2019, 20, 353–367 CrossRef CAS PubMed.
Footnote |
† Electronic supplementary information (ESI) available. See DOI: 10.1039/d0an00150c |
|
This journal is © The Royal Society of Chemistry 2020 |