DOI:
10.1039/C9AN01346F
(Paper)
Analyst, 2020,
145, 97-106
Identifying fates of cancer cells exposed to mitotic inhibitors by quantitative phase imaging†
Received
17th July 2019
, Accepted 12th November 2019
First published on 14th November 2019
Abstract
Cell cycle deregulation is a cancer hallmark that has stimulated the development of mitotic inhibitors with differing mechanisms of action. Quantitative phase imaging (QPI) is an emerging approach for determining cancer cell sensitivities to chemotherapies in vitro. Cancer cell fates in response to mitotic inhibitors are agent- and dose-dependent. Fates that lead to chromosomal instabilities may result in a survival advantage and drug resistance. Conventional techniques for quantifying cell fates are incompatible with growth inhibition assays that produce binary live/dead results. Therefore, we used QPI to quantify post-mitotic fates of G0/G1 synchronized HeLa cervical adenocarcinoma and M202 melanoma cells during 24 h of escalating-dose exposures to mitotic inhibitors, including microtubule inhibitors paclitaxel and colchicine, and an Aurora kinase A inhibitor, VX-680. QPI determined cell fates by measuring changes in cell biomass, morphology, and mean phase-shift. Cell fates fell into three groups: (1) bipolar division from drug failure; (2) cell death or sustained mitotic arrest; and (3) aberrant endocycling or multipolar division. In this proof-of-concept study, colchicine was most effective in producing desirable outcomes of sustained mitotic arrest or death throughout its dosing range, whereas both paclitaxel and VX-680 yielded dose-dependent multipolar divisions or endocycling, respectively. Furthermore, rapid completion of mitosis associated with bipolar divisions whereas prolonged mitosis associated with multipolar divisions or cell death. Overall, QPI measurement of drug-induced cancer cell fates provides a tool to inform the development of candidate agents by quantifying the dosing ranges over which suboptimal inhibitor choices lead to undesirable, aberrant cancer cell fates.
Introduction
Developing effective anti-cancer treatment regimens remains a significant therapeutic challenge. Treatment selection based on available diagnostic data including assessment of histologic tumor subtype, clinical grade and stage, molecular biomarkers, and genome-profiling studies can still lead to variable patient outcomes. This indicates a pressing need to continue developing new agents and regimens.1 The prediction of treatment outcomes and selection of therapeutic agents typically relies upon drug performance studies from preclinical research and clinical trials. In these settings, drug performance assessments are most commonly by multi-day growth inhibition assays in vitro and tumor shrinkage in vivo. However, data from these binary analyses may fail to uncover processes within cancer cells that further increase drug resistance and tumor aggressiveness. Cancer cells that persist after therapy may acquire additional genetic or epigenetic changes that make future treatments progressively more difficult.2 Therefore, a method that captures the full range of cell fates after a specific treatment, for example with a mitotic inhibitor, could reveal unsuspected suboptimal drug regimens with an elevated risk of promoting more aggressive tumors.
Specific mitotic inhibitors have frequent use to treat specific cancers, such as paclitaxel for breast and ovarian cancers. As a group they target the microtubule system or associated cell division kinases with the goal of activating growth checkpoints to induce mitotic arrest and apoptosis of cancer cells.3 Despite widespread use, most mitotic inhibitors show neurotoxicity, poor in vivo efficacy, and are difficult to dose adequately, thereby limiting applications.4 Preclinical studies also reveal that post-treatment surviving cancer cells may aberrantly exit from mitosis with multipolar cell divisions or endocycling from a weakened mitotic checkpoint caused by suboptimal mitotic inhibitor dosing.5,6 These mitotic aberrations may cause aneuploidy, chromosome instability, and increased tumor aggression.7–9 A rapid method to detect and classify aberrant mitotic outcomes for mitotic inhibitor treated cancers could improve drug development and selection.
Flow cytometry that uses DNA intercalating dyes, confocal microscopy, fluorescence time-lapse microscopy, and multi-day growth inhibition assays are current methods for assessing cellular responses to mitotic inhibitors.5,6,10 Unfortunately these approaches are often laborious, can be cell destructive, are limited to discrete measurement time points that can miss emerging therapy resistance, or require labeling that may interfere with cell behavior. For example, the most commonly practiced multi-day growth inhibition assays only provide total numbers of viable or dead cancer cells in tissue culture at specific treatment time points. EC50 values generated from this type of counting assay only shows population trends and overlooks phenotypic outcomes of individual cancer cells that survive treatment. This approach therefore yields limited insight into drug response kinetics and potential aberrant outcomes.
To overcome limitations in current screening methods and to increase throughput, we deployed a version of quantitative phase imaging (QPI) we refer to as live cell interferometry (LCI) to measure single cell responses to three mitotic inhibitors with different mechanisms of action using dose-escalating drug concentrations. Current state-of-the-art QPI techniques, including digital holographic microcopy and spatial light interference microscopy, accurately quantify optical path length delays caused by cellular contents at submicron resolution, free of labeling agents and phototoxicity effects.11,12 Measurements of phase shifts are then processed to produce biophysical cell parameters, such as dry mass and mass transport.11,12 These relevant biophysical properties can be analyzed in a wide range of QPI applications, such as measurements of cytotoxicity.13,14 Our approach uses quadriwave lateral shearing interferometry (QWLSI) to precisely quantify the phase-shift of incident light interacting with the non-aqueous mass, or biomass, of individual cells.15 The QWLSI approach has been shown to be accurate over a range of different specimen thickness and insensitive to imaging location, magnification, or degree of spatial light coherence.16 Conversion of measured phase-shifts in light into biomass uses an experimentally determined cell average specific refractive index, which enables quantifying changes in cell biomass over time.17,18 Prior LCI studies revealed breast cancer cell line sensitivities to trastuzumab (Herceptin) within 6 h, a speed compatible for studies of patient biopsy materials, with results replicating multi-day growth inhibition assays.19,20 More recently, LCI successfully dissected tumor heterogeneity and drug resistance for melanoma cells in a mixture21 and could replicate known tumor sensitivities to cisplatin in mouse patient-derived xenograft (PDX) models of breast cancer.22 These prior LCI studies validated QPI utility in cancer, but did not evaluate cancer cell outcomes beyond binary growth inhibition results. The use of multi-parametric QPI response profiling data that could further inform preclinical drug development and clinical drug selection is an exciting possibility explored here.
In this proof-of-concept study, we provide a new multi-parametric analytical method to identify different cell fate outcomes to mitotic inhibitors using QPI measurements of cell biomass, morphology, and mean phase-shift of light. Our study provides dynamic data on mitotic inhibitor activities and the frequencies of abnormal and undesirable outcomes during early exposure time points that may make tumors more difficult to treat.7 Our cell fate identification strategy may also be useful for developing and testing other anticancer agents and regimens.
Materials and methods
Cells and cell culture
HeLa human cervical adenocarcinoma cells were from the American Type Culture Collection (ATCC) and M202 human melanoma cells were a gift from Dr Owen Witte (UCLA). HeLa cells were maintained in 1
:
1 DME/F-12 media (Thermo Fisher Scientific) and M202 cells were maintained in RPMI 1640 media (Thermo Fisher Scientific), with each media supplemented by 10% FBS (Omega Scientific), 100 U mL−1 penicillin (Corning), 100 μg mL−1 streptomycin (Corning) and 2 mmol/L-glutamine (Thermo Fisher Scientific).
Growth inhibition assay
Twelve-well flat bottom plates (Thermo Fisher Scientific) received 5 × 104 cells per well. Paclitaxel (Sigma-Aldrich), colchicine (Sigma-Aldrich), or VX-680 (Selleckchem) small molecule mitotic inhibitors, or DMSO (Sigma-Aldrich) carrier-control, were added to cell culture media at the indicated doses and durations (Fig. S1 and S2†). Cells from three replicate wells per treatment condition were harvested each day, stained with trypan blue, and counted using an automated cell counter (Countess; Invitrogen).
Cell preparation for QPI
ibidi 4-well Ph+ μ-slides received 1.5 × 104 cells per mL that were then grown for 7–10 h to homeostasis. Media containing 2 mM thymidine (Sigma-Aldrich) was added to arrest HeLa cells in G0/G1 phase for 18 h and M202 cells for 20 h. Synchronized cells were released from cell cycle block by media washing three times. Fresh media with the indicated doses of paclitaxel, colchicine, VX-680, or DMSO were then added to the 4-well μ-slide and then sealed with anti-evaporation oil (ibidi) before QPI on the microscope stage.
Live cell interferometry
QPI of HeLa and M202 cells was performed on an Axio Observer A1 inverted microscope (Zeiss) with a SID4Bio quadriwave lateral shearing interferometry (QWLSI) camera (Phasics). A temperature and CO2 regulated stage-top cell incubation chamber (ibidi) was fit to a motorized xy stage (Thorlabs) to maintain environmental homeostasis and enable QPI at multiple locations. A Zeiss LD Plan Neofluar 20× NA 0.4 objective was heated and maintained at 37 °C with a custom built copper objective heater driven by a heat controller (Thorlabs). Trans-illumination was by a 660 nm center wavelength collimated LED (Thorlabs). Image collection occurred every 10 min for 24 h at 15 randomly selected imaging locations per well containing cells plated with sufficient spacing to enable automated image processing and biomass segmentation. Imaging locations were selected within the central part of each well, where the highest quality quantitative phase information can be obtained without aberrations caused by the cell culture apparatus or optical hardware. The selected imaging fields cover a range of locations within each imaged well, to obtain a sample that is representative of the cell population (Fig. S3†).
QPI data analysis
Interferograms captured by the SID4Bio QWLSI camera were converted to phase-shift and intensity images using the Phasics MATLAB software development kit. These images were analyzed using custom MATLAB (MathWorks) scripts that eliminated background aberrations by fitting a 4th order Zernike surface to cell-free regions and subtracting the fitted surface from the image. Cell biomass data was extracted from background corrected images by integrating light phase-shift in segmented cell projected areas and multiplying by the experimentally determined specific refractive increment of 0.0018.17,23,24 Data analysis of cell fates and time spent in mitosis are performed using morphology as discussed in the following section. The entire procedure requires 3–4 hours for image processing and data analyses, depending on the number of locations imaged in each experiment.
Morphology metrics
Mean phase shift: The mean phase-shift of a cell is obtained by dividing the total integrated phase-shift for that particular cell by its projected area. Interphase flat and spread-out versus round and mitotic phase HeLa cells were sampled to establish average mean phase-shift values for both morphologies. Since HeLa and M202 cells share comparable sizes, morphologies, optical densities and the same tracking criteria for the MATLAB tracking algorithm, the same average mean phase-shift values are representative for both cell types (Fig. S4†). Shape factor: The shape factor of a cell, also called circularity or isoperimetric quotient, is calculated by dividing the projected area, A, of a cell by its circumference or the length of its perimeter, P, (4πA/P2). Random flat HeLa and M202 cells and mitotic HeLa cells were sampled to establish average shape factor values for both morphologies (Fig. S4†). A perfect circle has a shape factor of 1.0 and an irregular shape, such as an interphase adherent cell, has a shape factor of approximately 0.5 (Fig. S4†).
Flow cytometry
Cells were collected from T25 flasks after 24 and 48 h of drug exposure, washed once with 1× PBS, pH 7.4, and then re-suspended in 500 μL of FxCycle™ PI/RNase Staining Solution (Thermo Fisher Scientific). Flow cytometry was performed on FACS BD LSRII and FACS BD Fortessa flow cytometers (BD Biosciences). DNA content analysis was by FlowJo software.
Statistical analysis
Chi-square tests of independence were performed on contingency tables assessing the observed cell fate counts per inhibitor dosage for significant association relative to expected counts in each drug treatment panel with a 95% confidence interval (Prism 6, GraphPad, Inc.). Dose response curve-fitting was performed using the curve fitting toolbox in MATLAB (MathWorks). Two-sample Kolmogorov–Smirnov (KS) tests were performed between bipolar cell division versus prolonged mitotic arrest and cell death fate distributions for each drug treatment panel in MATLAB with a 95% confidence interval. One-way ANOVA with unbalanced sample groups was performed on duration of mitosis datasets between cell fate groups in MATLAB with a 95% confidence interval. Statistical significance required p < 0.05.
Results
Identifying post-mitotic cell fate outcomes using QPI
We deployed QPI to measure synchronized HeLa and M202 single cancer cell biomass and morphology responses after mitotic entry, in the presence of escalating doses of several mitotic inhibitors (Fig. 1 and Table 1). We chose paclitaxel, a microtubule stabilizing agent, colchicine, a microtubule destabilizing drug, and VX-680, an Aurora kinase A inhibitor that represent a range of mitotic inhibitor modes of action. Cells were treated with each drug for 5 h before QPI of randomly selected locations at 10 min imaging intervals over the next 24 h (Fig. 1A). Changes in biophysical and morphological parameters over the imaging period provided data to determine cell fate outcomes of single cancer cells in each condition (Fig. 1B and C). Two EC50 values, one for successful bipolar divisions representing drug failure and one for cell death/arrest, were calculated based on the distributions of cell fate outcomes in the sampled cell populations (Fig. 1D). ΔEC50, the difference between these two EC50 values, therefore describes the concentration range over which cancer cells display aberrant mitotic exits at suboptimal dosages for each type of drug treatment (Fig. 1D).
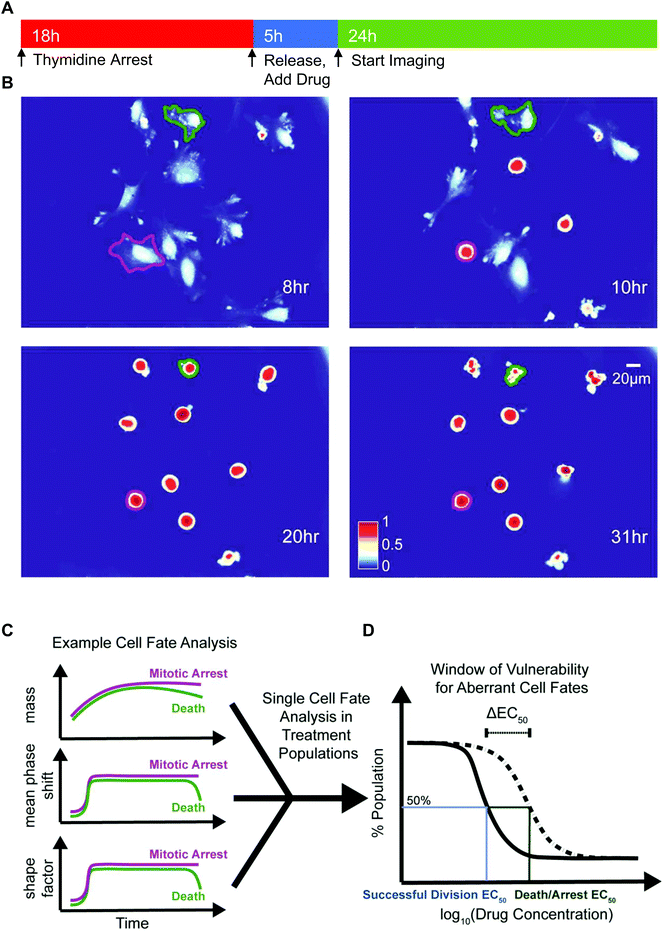 |
| Fig. 1 Experimental design and analysis schematics. (A) Timeline of the experiment, showing cell synchronization and period of QPI. (B) Example QPI images of single HeLa cells under 50 nM of colchicine treatment. The same cells outlined in green and purple were tracked continuously over time to determine their post-mitotic entry fates, in this case are death and prolonged mitotic arrest. Color bar indicates phase shift in nm. Time stamps indicate time since start of drug exposure. (C) Representative schematic of biomass, mean phase-shift and shape factor data over time for two cell fates shown in (B): death and mitotic arrest. (D) Representative schematic of two EC50 curves generated based on normalized cell-fate outcomes distributions from randomly sampled single cells over a range of drug concentrations. The difference between the two EC50 values, ΔEC50, provides a dosing range in which the drug can potentially cause aberrant mitotic exits. | |
Table 1 Mitotic inhibitors drug concentrations administered to M202 and HeLa cell lines in live cell interferometer study
Cell lines |
Paclitaxel (nM) |
Colchicine (nM) |
VX-680 (nM) |
HeLa |
10, 50, 100, 500 |
50, 150, 500, 1500, 2500 |
100, 300, 600 |
M202 |
1, 3, 9, 50, 100, 500 |
1, 5, 10, 20, 50 |
30, 90, 150, 300, 600 |
We classified the mitotic fate of each tracked cancer cell into one of five categories: (1) successful bipolar division, (2) multipolar division, (3) endocycling, (4) cell death, and (5) prolonged mitotic arrest (Fig. 2A). We then subjectively divided these five outcomes into three groups. Group 1 is successful bipolar divisions, representing the failure of a drug to block mitosis at the surveyed doses. Group 2 includes prolonged mitotic arrest and cell death, which are desirable anticancer cell fates. Group 3 fates are hidden in growth inhibition assays and include multipolar divisions and endocycling. Group 3 fates can yield chromosomal aberrations and make tumor cells increasingly aggressive and difficult to eradicate.7–9 Importantly, conventional multi-day growth inhibition counting assays combine group 1, group 2 mitotic arrest, and group 3 outcomes to report on the cumulative number of live cancer cells at specific time points during and post-drug treatment without discriminating group 3 unfavorable and potentially dangerous outcomes.
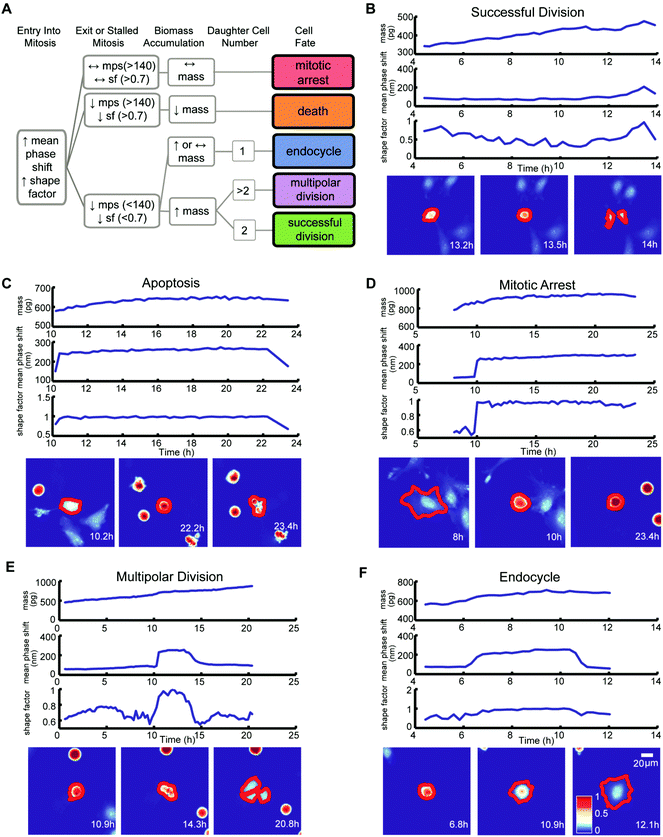 |
| Fig. 2 Cell fate algorithm with examples. (A) A decision tree diagram showing the MATLAB algorithm for determining cell fates based on dynamic changes in cell biomass, mean phase-shift, and shape factor measured by QPI. (B) Example of a HeLa cell undergoing a bipolar division. (C) Example of a HeLa cell dying. (D) Example of a HeLa cell in prolonged mitotic arrest. (E) Example of a HeLa cell undergoing a multipolar division. (F) Example of a HeLa cell undergoing endocycling. | |
Criteria for classifying cell fates relies upon specific biophysical and morphological QPI measurements. A sharp surge in mean phase-shift above 140 nm and shape factor, a measurement of roundness, above 0.7 defines the time point of mitotic entry as cells ‘round up’ (Fig. S4†).25 During a successful bipolar division, a single cell separates into two cells and then flattens to resume growth during interphase. The mitotic interval with cytokinesis is complete when two cells emerge at or near the former parent cell location with mean phase-shift and shape factor below 140 nm and 0.7 thresholds (Fig. 2A and B).25 A cell death fate occurs when cell biomass shows either a sudden or a slow decline following mitotic entry (Fig. 2A and C).26 During cell death the mean phase-shift through the cell decreases but stays above the 140 nm threshold due to pyknosis, karyorrhexis, and cytoplasm content condensation.27 The shape factor value also decreases when severe membrane blebbing or cell disintegration occurs (Fig. 2C). A prolonged mitotic arrest is identified by stagnation of changes in cell biomass, with mean phase-shift and shape factor remaining above 140 nm and 0.7 thresholds, and no cell division or other morphological changes occurring (Fig. 2A and D). A multipolar cell division fate shows the same time-dependent tracing pattern in mean phase-shift, shape factor, and biomass accumulation as bipolar divisions with three or more cells arising at or near the previous parent cell location (Fig. 2A and E). Following multipolar divisions, not all daughter cells grow or thrive, likely due to aberrant chromosome partitioning.28 Finally, QPI identifies endocycling when a cancer cell shows a sudden surge in mean phase-shift and shape factor, indicative of mitotic ‘rounding’, but returns to a G0/G1 flat morphology, mean phase-shift under 140 nm, and shape factor less than 0.7 without cell division (Fig. 2A and F).29 In cases when single cells are hard to dissect from neighbors or are difficult to track over time, we apply manual screening corrections to the results of the cell fate algorithm.
Calculating EC50,growth and EC50,death using QPI quantified cell fate outcomes
In growth inhibition assays, cell fate outcomes are binary, meaning that a drug does or does not alter live cell numbers compared to control conditions over time from the sum of cell divisions, arrests, and deaths. Thus, conventional preclinical drug development assays generate one EC50 curve to fit a binary outcome. By contrast, QPI yields three cell fate groups (groups 1–3) with qualitatively distinct outcomes available for quantification. Therefore, instead of analyzing total live cell numbers, we generated two dose–response curves from QPI data. One dose–response curve corresponds to successful bipolar divisions from drug insensitivity (Fig. 3, solid line). The second dose–response curve describes desirable outcomes with prolonged mitotic arrest or cell death (Fig. 3, dotted line). We characterized these curves based on a standard log-scale normalized response equation: | 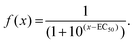 | (1) |
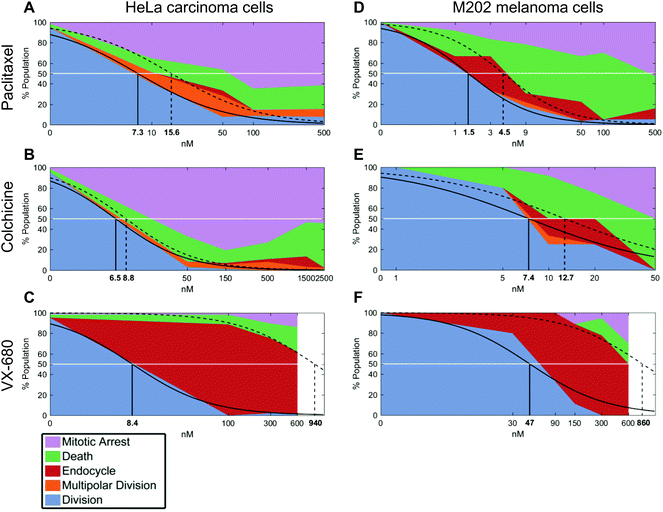 |
| Fig. 3 Cell fate distribution analysis. (A) Cell fate distribution of HeLa cells with paclitaxel exposure (n = 187). (B) Cell fate distribution of HeLa cells with colchicine exposure (n = 380). (C) Cell fate distribution of HeLa cells with VX-680 exposure (n = 223). (D) Cell fate distribution of M202 cells with paclitaxel exposure (n = 128). (E) Cell fate distribution of M202 cells with colchicine exposure (n = 42). (F) Cell fate distribution of M202 cells with VX-680 exposure (n = 63). A solid line in each panel represents a dose response curve fit to the bipolar division distribution. A dotted line in each panel represents a dose response curve fit to the prolonged mitotic arrest or cell death distribution. Numbers represent EC50 values of for each curve. Legend shows color code for cell fate outcomes. | |
We next compared calculated EC50 values between drug treatment panels (Table S1†). In particular, the ability of QPI to identify multiple cancer cell fates enables the characterization of mitotic inhibitors for both sensitivity, represented by a low average EC50 value, as well as avoidance of undesirable fates. The concentration window in which undesirable fates occur is described by ΔEC50 = EC50,death − EC50,growth. Therefore, QPI also enables the identification of mitotic inhibitors with a low ΔEC50, indicating a small concentration window that results in undesirable cell fates. When comparing ΔEC50 between inhibitors and cell lines, we used normalized value of ΔEC50/EC50,growth because relative ΔEC50 shows the impact of concentration variance relative to the target dose of a specific inhibitor (Table S1†). Colchicine had the smallest relative ΔEC50, for both HeLa cells (0.35) and M202 cells (0.72) (Fig. 3B and E). This result is consistent with previous results showing that colchicine induces negligible or low amounts of aneuploidy.6 Paclitaxel showed a slightly larger relative ΔEC50 for both HeLa cells (1.1) and M202 cells (2.0), due to the increase in multipolar divisions and endocycling events compared to colchicine (Fig. 3A and D). VX-680 presented by far the largest relative ΔEC50 for HeLa cells (110) and M202 cells (17), due to a large proportion of endocycling events (Fig. 3C and F). Lastly, Kolmogorov–Smirnov test results show that bipolar divisions and prolonged mitotic arrest or cell death dose response distributions statistically differ (Table S1†), with the exception of M202 cells under increasing paclitaxel doses (p = 0.052).
To confirm that a wide range of VX-680 dosing generates QPI detected endocycling, as predicted by a large ΔEC50, we analyzed the DNA content of HeLa and M202 cells exposed to VX-680 using flow cytometry (Fig. S5†). As an example, there was a large increase in the number of cells with 4n DNA compared to those with 2n DNA in cells exposed to 300 nM and 600 nM VX-680. A small population of HeLa cells with 8n DNA content emerged at 300 nM VX-680 that dramatically increased with 600 nM VX-680 (Fig. S5C–F†), in agreement with the features of endocycling.29
Comparing EC50,growth and EC50,death to conventional EC50 from live cell counting assays
In parallel, we performed live cell counting assays for HeLa and M202 cancer cells exposed to paclitaxel, colchicine, and VX-680 at 24 hours and multi-day timepoints (Fig. S1 and S2†). As anticipated, these agents markedly reduced the accumulation of cancer cells at 24 hours and over 6 days (Fig. S1 and S2†). Growth inhibition studies are the standard for drug development, although drug effects beyond changes in total live cell numbers were indiscernible. It is important to note that EC50 values from live cell counting assays are defined by concentrations at which the total number of live cells present in the treatment well is 50% of the total number of live cells present in the control well. Whereas, EC50 values from QPI analysis are defined by concentrations at which 50% of the sampled cells in a specific treatment undergo a certain cell fate within the first division after treatment. When compared to QPI measured EC50 values, live cell counting EC50 values are therefore on the same or nearest log scale, but greater than both EC50,growth and EC50,death for paclitaxel and colchicine treatments. However, in the case of VX-680, many endocycling events occur that do not lead to immediate cell deaths. This leads to large differences between EC50,growth and EC50,death values measured by QPI. The EC50 measured by live cell counting assay falls in the middle of the two on the log scale. This result therefore indicates that the QPI EC50,growth and EC50,death correspond differently to the standard live cell counting EC50 for different mitotic inhibition mechanisms.
We also assessed whether inhibitor dosage statistically associates with outcome by applying chi-square tests of independence for each cancer cell type and drug combination (Fig. 3). Our analyses show that within each of the six cancer cell-drug treatment pairings, cell fate outcomes were dose-dependent. Increasing dose shifts cell fate distributions from mostly successful bipolar divisions to mostly mitotic arrests and deaths (p < 0.0001 for each condition) (Table S2†). For example, HeLa cells showed a range of outcomes at lower doses of paclitaxel and VX-680 (Fig. 3A and C). At 10 nM of paclitaxel, a dose between the two observed QPI EC50 values, all outcomes except endocycling occurred, with only a small amount of endocycling appearing at slightly higher drug concentrations (Fig. 3A). By contrast, the majority of HeLa cells endocycle at all VX-680 concentrations within the first 24 hours of treatment, whereas prolonged mitotic arrest or cell death increased in outcome frequency only at 600 nM VX-680. This concentration is 4–12 times higher than the recommended effective dose (50–150 nM) in vitro against thyroid and blood-cancer cell lines (Fig. 3C),30,31 yet well within the range of mean plasma concentration of the maximum-tolerated dose (64 mg m−2 h−1) in patients, determined in phase I clinical trial.32
Analyzing correlation between mitosis durations and cell fate outcomes
Finally, there has been debate over whether the duration of mitosis during anticancer drug exposure affects cancer cell outcomes.10,33 This data is quantifiable from QPI data showing the period from onset of mitosis to scored cell outcomes.3,10 Single HeLa cell tracking data show that time spent in mitosis statistically differ between cell fate outcomes (p = 1.8 × 10−54). Multipolar divisions, cell death, or arrest are frequent outcomes of extended mitosis time, whereas endocycling usually results from shorter periods of mitosis (Fig. 4). For M202 cells, even though the mean values of time spent in mitosis for cell fate outcomes are statistically different (p = 2.3 × 10−4), there is no significant trend for mitosis durations between outcomes categories (Fig. S6†). Mitosis durations less than 100 minutes more frequently (45% for HeLa and 34% for M202) resolve as drug insensitivity with successful bipolar cell divisions compared to other fates in both cell lines (Fig. 4D and S6D†). These data clearly reveal variability in responses to the same mitotic inhibitor treatments from different cancer cell lines. Thus, QPI-derived outcomes classifications reinforce the importance of studying responses in multiple cancer cell lines and types during preclinical drug development that may correspond to differences in personalized responses to different treatment agents and regimens for individuals with cancer.
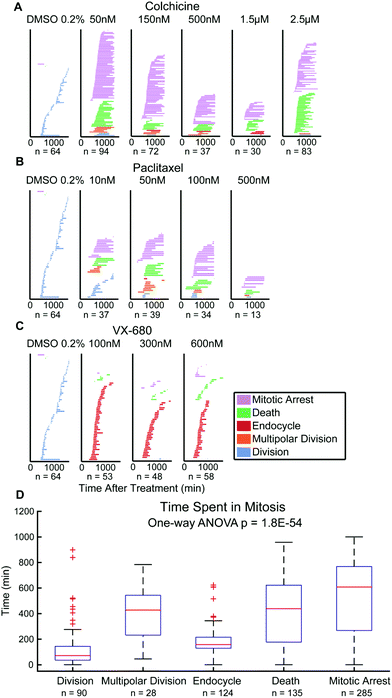 |
| Fig. 4 Cell fate responses and mitosis durations. (A) Time spent in mitosis for HeLa single cells with colchicine exposure. The length of each color bar indicates the amount of time spent in mitosis for that individual cell. The color of the bar corresponds to its cell fate outcome. (B) Time spent in mitosis for HeLa single cells with palitaxel exposure. (C) Time spent in mitosis for HeLa single cells with VX-680 exposure. (D) Box plot of time spent in mitosis for five cell fate outcomes. One-way ANOVA with unbalanced sample groups was performed comparing all samples. There are statistically significant differences between the mean values of mitosis durations for different cell fate outcomes (p = 1.8 × 10−54). | |
Discussion
Changes in cell biomass as a response indicator for screening cancer drugs has gained traction in recent years because of multiple technological breakthroughs.19,21,22,34–36 A common thread in this emerging area is the increasing linkage between biomass accumulation rates with traditional measures of drug efficacy and preclinical outcomes of growth inhibitors in many types of modeled malignancies. QPI methods in particular are providing additional biophysical insights based on changes in cell morphology during studies of cell division and cancer-immune cell interactions.25,37 Here, we expanded the application spectrum for quantitative phase methods by combining biomass and morphological analyses in studies of the pharmacodynamic characteristics of small molecule mitotic inhibitors against two types of cancer.
We showed that during exposure to mitotic inhibitors QPI classification into three outcome groups yields useful concentration windows in which undesirable fates that include multipolar cell divisions and endocycling occur, as described by ΔEC50. Cancer cells exposed to inadequate mitotic inhibitor concentrations resolve transient cell cycle arrest by apoptosis or ‘mitotic slippage’ to resume growth and cycling,38 which can result in chromosome abnormalities such as aneuploidy and tetraploidy.39 Numerical and structural chromosome aberrations, which often occur with multipolar cell divisions and endocycling, may contribute to increased therapy resistance40 through a range of molecular mechanisms.7,8 Aneuploidy paradoxically can promote or suppress tumor growth and may cause an elevated rate of tumor recurrence by generating drug-resistant heterogeneity with evolving growth and survival advantages.40,41 Tetraploid cells and cells with certain chromosomal rearrangements act as intermediates to further chromosome instability and the development of aneuploidy.7 Unfortunately, tetraploidy and chromosomal aberrations that can contribute to therapy resistance have also been linked to mitotic inhibitor exposure at sub-lethal concentrations.6 It has been shown that mitotic slippage occurs far more frequently in vivo than in vitro, suggesting possible underestimation of group 3 outcomes with QPI compared to inefficacious agents and malignancy augmentation that may occur in patients.42 Interestingly, proteotoxic and metabolic stress are reported for aneuploid cells,43 which may result from multipolar divisions and endocycling events identified using our QPI methods.
Inhibitor mechanisms, concentrations, and mitotic arrest duration are major determinants of cancer cell outcomes following escape from mitotic arrest.33,44,45 Our QPI approach analyzed these key characteristics for different mitotic inhibitors. Because of off-target cytotoxicity, mitotic inhibitors typically have low therapeutic indices that can limit clinical dosages43 and cause abnormal exits with viable cells containing chromosomal abnormalities.6,44In vivo, this undesirable outcome can occur in under-dosed or poorly perfused tumors and potentially jeopardizes clinical outcomes by adding to therapy resistance and tumor aggressiveness.
In vitro multi-day growth inhibition binary assays yield a single EC50 curve that overlooks a range of potential mitotic slippage events that add to chromosomal aberrations. Advances in non-QPI techniques such as in vivo microscopy and real time FUCCI imaging enable quantifying mitotic exits for a small number of cells.46,47 Additional methods, such as microchannel resonators, have limitations in measurement longevity and are low throughput. Recently, QPI methods were used to quantify cytotoxicity using area and morphological information.13,14 Although previous QPI applications showed results comparable to automatic cell profilers, the analysis did not fully leverage the wealth of biophysical information captured by QPI techniques. In contrast, the QPI analysis method presented here exposed differences in outcomes between a microtubule-destabilizing agent, paclitaxel, a microtubule-stabilizing agent, colchicine, and a cell division inhibitor, VX-680. Our decision tree algorithm provided five cancer cell outcome categories and three subjective groups to enable determination of dual EC50 curves for each drug and tumor cell type examined. For a particular drug and tumor type, the extent of EC50 value separation, ΔEC50, predicts the likelihood of undesirable multipolar divisions or endocycling that can result in aneuploidy and increased therapy resistance, providing useful concentration ranges to avoid in which aberrant mitotic exits prevail.
We note that there is a difference between the dual EC50s determined via QPI and the single EC50 determined via live cell counting, especially for paclitaxel and colchicine. Paclitaxel and colchicine induced large numbers of mitotic arrests in the treated cell populations. While live cell counting classifies arrested cells as live cells, the QPI data analysis method described here does not account for arrested cells in EC50,growth. Criteria used for a cell to be included in EC50,growth requires that cell to successfully divide during the imaging period. Therefore, live cell counting identified a higher proportion of cells as live cells than QPI analysis did as growing cells, producing a larger EC50 value than EC50,growth for paclitaxel and colchicine treatments.
QPI analyses revealed that HeLa and M202 bipolar divisions from drug failure show the shortest mitosis periods, consistent with previous studies in which prolonged mitotic arrest resulted in hypersensitivity to additional death cues.33 Additional studies showed several existing chemotherapeutics that induce apoptosis at normal dosages can trigger mitotic catastrophe that directly lead to apoptosis at very low dosages in aneuploid and polyploid cells.48 This can dramatically increase chemotherapeutic tolerance in patients and hint at effective combinatorial therapies in cancer treatments using mitotic inhibitors. Therefore, future QPI studies include screens for cell fate outcomes under combined exposure of energy stressors or low dose chemotherapeutics and mitotic inhibitors that induce aneuploidy.
Conclusions
In this study, we demonstrate a novel application of QPI in screening and identifying aberrant cell fate outcomes as a result of suboptimal mitotic inhibitor doses. Conventional growth inhibition assays rely on live cell counting to generate EC50 values that infer pharmacokinetics of mitotic inhibitors, and fail to reveal vital information on negative effects of the inhibitors. QPI analyzes cell fate outcome profiles, EC50,growth, and EC50,death values that provide in-depth insights into mechanism of action and risky dosing windows of mitotic inhibitors. Importantly, this QPI technique is compatible with patient derived organoids that resemble heterogeneous patient tumors better than in vitro single cell type cultures. Screening mitotic inhibitor cocktails on tumor organoids with QPI can facilitate the development of next generation cancer therapies.
Conflicts of interest
M. A. T. is a co-founder, board member, shareholder, and consultant for NanoCav, LLC, a private start-up company working on quantitative phase microscopy techniques and applications. T. A. Z. is consultant for NanoCav, LLC. The other authors do not have any conflicting interests to declare.
Acknowledgements
D. H. conducted the experiments, analyzed the data, prepared the figures and wrote the manuscript. I. J. R. helped perform experiments and analyzed data under supervision of D. H. G. M. and J. R. analyzed results and edited the manuscript. T. A. Z. supervised the study along with M. A. T., who initiated, supervised and obtained funding for the study. We thank Fasih Ahsan for advice on statistical analysis. Funded by National Institutes of Health grant R21CA227480 to M. A. T. and R01CA185189 to M. A. T. and J. R., and in part, by NCI Cancer Center Support Grant P30CA016042 to the UCLA Jonsson Comprehensive Cancer Center and P30CA016059 to the VCU Massey Cancer Center.
References
- E. Shtivelman, M. Q. Davies, P. Hwu, J. Yang, M. Lotem, M. Oren, K. T. Flaherty and D. E. Fisher, Oncotarget, 2014, 5, 1701–1752 Search PubMed.
- H. Shi, W. Hugo, X. Kong, A. Hong, R. C. Koya, G. Moriceau, T. Chodon, R. Guo, D. B. Johnson, K. B. Dahlman, M. C. Kelley, R. F. Kefford, B. Chmielowski, J. A. Glaspy, J. A. Sosman, N. van Baren, G. V. Long, A. Ribas and R. S. Lo, Cancer Discovery, 2014, 4, 80–93 CrossRef CAS.
- B. A. Weaver and D. W. Cleveland, Cancer Cell, 2005, 8, 7–12 CrossRef CAS.
- E. Manchado, M. Guillamot and M. Malumbres, Cell Death Differ., 2012, 19, 369–377 CrossRef CAS PubMed.
- D. A. Brito and C. L. Rieder, Curr. Biol., 2006, 16, 1194–1200 CrossRef CAS.
- J. G. Chen and S. B. Horwitz, Cancer Res., 2002, 62, 1935–1938 CAS.
- T. Fujiwara, M. Bandi, M. Nitta, E. V. Ivanova, R. T. Bronson and D. Pellman, Nature, 2005, 437, 1043–1047 CrossRef CAS.
- N. J. Ganem, Z. Storchova and D. Pellman, Curr. Opin. Genet. Dev., 2007, 17, 157–162 CrossRef CAS PubMed.
- J. M. Schvartzman, R. Sotillo and R. Benezra, Nat. Rev. Cancer, 2010, 10, 102–115 CrossRef CAS PubMed.
- K. E. Gascoigne and S. S. Taylor, Cancer Cell, 2008, 14, 111–122 CrossRef CAS PubMed.
- Y. Park, C. Depeursinge and G. Popescu, Nat. Photonics, 2018, 12, 578–589 CrossRef CAS.
- P. Marquet, C. Depeursinge and P. Magistretti, Neurophotonics, 2014, 1, 020901 CrossRef PubMed.
- B. Greve, F. Sheikh-Mounessi, B. Kemper, I. Ernst, M. Gotte and H. Eich, Strahlenther. Onkol., 2012, 188, 1038–1047 CrossRef CAS.
- J. Kühn, E. Shaffer, J. Mena, B. Breton, J. Parent, B. Rappaz, M. Chambon, Y. Emery, P. Magistretti, C. Depeursinge, P. Marquet and G. Turcatti, Assay Drug Dev. Technol., 2013, 11, 101–107 CrossRef.
- P. Bon, G. Maucort, B. Wattellier and S. Monneret, Opt. Express, 2009, 17, 13080–13094 CrossRef CAS.
- S. Aknoun, J. Savatier, P. Bon, F. Galland, L. Abdeladim, B. Wattellier and S. Monneret, J. Biomed. Opt., 2015, 20, 126009 CrossRef.
- J. Reed, J. Chun, T. A. Zangle, S. Kalim, J. S. Hong, S. E. Pefley, X. Zheng, J. K. Gimzewski and M. A. Teitell, Biophys. J., 2011, 101, 1025–1031 CrossRef CAS.
- T. A. Zangle, J. Chun, J. Zhang, J. Reed and M. A. Teitell, Biophys. J., 2013, 105, 593–601 CrossRef CAS.
- J. Chun, T. A. Zangle, T. Kolarova, R. S. Finn, M. A. Teitell and J. Reed, Analyst, 2012, 137, 5495–5498 RSC.
- N. A. O'Brien, B. C. Browne, L. Chow, Y. Wang, C. Ginther, J. Arboleda, M. J. Duffy, J. Crown, N. O'Donovan and D. J. Slamon, Mol. Cancer Ther., 2010, 9, 1489–1502 CrossRef.
- D. Huang, K. A. Leslie, D. Guest, O. Yeshcheulova, I. J. Roy, M. Piva, G. Moriceau, T. A. Zangle, R. S. Lo, M. A. Teitell and J. Reed, Anal. Chem., 2018, 90, 3299–3306 CrossRef CAS.
- G. F. Murray, T. H. Turner, K. A. Leslie, M. A. Alzubi, D. Guest, S. S. Sohal, M. A. Teitell, J. C. Harrell and J. Reed, ACS Omega, 2018, 3, 17687–17692 CrossRef CAS.
- R. Barer, Nature, 1952, 169, 366–367 CrossRef CAS.
-
K. F. A. Ross, Phase contrast and interference microscopy for cell biologists, St Martin's Press, New York, 1967 Search PubMed.
- T. A. Zangle, M. A. Teitell and J. Reed, PLoS One, 2014, 9, e115726 CrossRef.
- I. Chowdhury, B. Tharakan and G. K. Bhat, Comp. Biochem. Physiol., Part B: Biochem. Mol. Biol., 2008, 151, 10–27 CrossRef.
-
R. S. Cotran, V. Kumar, T. Collins and S. L. Robbins, Robbins pathologic basis of disease, Saunders, Philadelphia, 6th edn, 1999 Search PubMed.
- N. J. Ganem, S. A. Godinho and D. Pellman, Nature, 2009, 460, 278–282 CrossRef CAS.
- B. A. Edgar, N. Zielke and C. Gutierrez, Nat. Rev. Mol. Cell Biol., 2014, 15, 197–210 CrossRef CAS PubMed.
- Y. Arlot-Bonnemains, E. Baldini, B. Martin, J. G. Delcros, M. Toller, F. Curcio, F. S. Ambesi-Impiombato, M. D'Armiento and S. Ulisse, Endocr.-Relat. Cancer, 2008, 15, 559–568 CrossRef CAS.
- X. F. Huang, S. K. Luo, J. Xu, J. Li, D. R. Xu, L. H. Wang, M. Yan, X. R. Wang, X. B. Wan, F. M. Zheng, Y. X. Zeng and Q. Liu, Blood, 2008, 111, 2854–2865 CrossRef CAS.
- A. M. Traynor, M. Hewitt, G. Liu, K. T. Flaherty, J. Clark, S. J. Freedman, B. B. Scott, A. M. Leighton, P. A. Watson, B. Zhao, P. J. O'Dwyer and G. Wilding, Cancer Chemother. Pharmacol., 2011, 67, 305–314 CrossRef CAS.
- M. E. Bekier, R. Fischbach, J. Lee and W. R. Taylor, Mol. Cancer Ther., 2009, 8, 1646–1654 CrossRef CAS PubMed.
- A. E. Cetin, M. M. Stevens, N. L. Calistri, M. Fulciniti, S. Olcum, R. J. Kimmerling, N. C. Munshi and S. R. Manalis, Nat. Commun., 2017, 8, 1613 CrossRef.
- Y. Li, M. J. Fanous, K. A. Kilian and G. Popescu, Sci. Rep., 2019, 9, 248 CrossRef.
- J. H. Kang, T. P. Miettinen, L. Chen, S. Olcum, G. Katsikis, P. S. Doyle and S. R. Manalis, Nat. Methods, 2019, 16, 263–269 CrossRef CAS.
- T. A. Zangle, D. Burnes, C. Mathis, O. N. Witte and M. A. Teitell, PLoS One, 2013, 8, e68916 CrossRef CAS.
- N. Sakurikar, J. M. Eichhorn and T. C. Chambers, J. Biol. Chem., 2012, 287, 39193–39204 CrossRef CAS.
- Z. Storchova and C. Kuffer, J. Cell Sci., 2008, 121, 3859–3866 CrossRef CAS.
- B. A. Weaver and D. W. Cleveland, J. Cell Biol., 2009, 185, 935–937 CrossRef CAS.
- R. Sotillo, J. M. Schvartzman, N. D. Socci and R. Benezra, Nature, 2010, 464, 436–440 CrossRef CAS.
- J. D. Orth, R. H. Kohler, F. Foijer, P. K. Sorger, R. Weissleder and T. J. Mitchison, Cancer Res., 2011, 71, 4608–4616 CrossRef CAS.
- C. Dominguez-Brauer, K. L. Thu, J. M. Mason, H. Blaser, M. R. Bray and T. W. Mak, Mol. Cell, 2015, 60, 524–536 CrossRef CAS PubMed.
- M. A. Jordan, K. Wendell, S. Gardiner, W. B. Derry, H. Copp and L. Wilson, Cancer Res., 1996, 56, 816–825 CAS.
- K. Torres and S. B. Horwitz, Cancer Res., 1998, 58, 3620–3626 CAS.
- S. Miwa, S. Yano, H. Kimura, M. Yamamoto, M. Toneri, Y. Matsumoto, F. Uehara, Y. Hiroshima, T. Murakami, K. Hayashi, N. Yamamoto, M. Bouvet, T. Fujiwara, H. Tsuchiya and R. M. Hoffman, Cell Cycle, 2015, 14, 621–629 CrossRef CAS.
- J. D. Orth, Y. Tang, J. Shi, C. T. Loy, C. Amendt, C. Wilm, F. T. Zenke and T. J. Mitchison, Mol. Cancer Ther., 2008, 7, 3480–3489 CrossRef CAS.
- T. V. Denisenko, I. V. Sorokina, V. Gogvadze and B. Zhivotovsky, Drug Resist. Updates, 2016, 24, 1–12 CrossRef.
Footnote |
† Electronic supplementary information (ESI) available. See DOI: 10.1039/c9an01346f |
|
This journal is © The Royal Society of Chemistry 2020 |