DOI:
10.1039/C8SM02133C
(Paper)
Soft Matter, 2019,
15, 792-802
Curvature sensing by cardiolipin in simulated buckled membranes†
Received
19th October 2018
, Accepted 21st December 2018
First published on 2nd January 2019
Abstract
Cardiolipin is a non-bilayer phospholipid with a unique dimeric structure. It localizes to negative curvature regions in bacteria and is believed to stabilize respiratory chain complexes in the highly curved mitochondrial membrane. Cardiolipin's localization mechanism remains unresolved, because important aspects such as the structural basis and strength for lipid curvature preferences are difficult to determine, partly due to the lack of efficient simulation methods. Here, we report a computational approach to study curvature preferences of cardiolipin by simulated membrane buckling and quantitative modeling. We combine coarse-grained molecular dynamics with simulated buckling to determine the curvature preferences in three-component bilayer membranes with varying concentrations of cardiolipin, and extract curvature-dependent concentrations and lipid acyl chain order parameter profiles. Cardiolipin shows a strong preference for negative curvatures, with a highly asymmetric chain order parameter profile. The concentration profiles are consistent with an elastic model for lipid curvature sensing that relates lipid segregation to local curvature via the material constants of the bilayers. These computations constitute new steps to unravel the molecular mechanism by which cardiolipin senses curvature in lipid membranes, and the method can be generalized to other lipids and membrane components as well.
I. Introduction
Biological membranes are subject to large deformations in cellular organelles, which can involve membrane remodeling. The interplay between lipids, proteins, and membrane curvature is now recognized as a key factor for cellular organization and membrane protein function.1–6 Recent experimental work has revealed curvature sensing and generation by many membrane associated molecules such as peripheral7–13 and transmembrane14–18 proteins, amphipathic and antimicrobial peptides,19–23 and lipids.24–26 However, the small sizes of individual lipid molecules tend to produce weak effects on the curvature scales of organelles or cells, unless amplified by cooperative effects or lipid–protein association.25–27
Cardiolipin (CL) is a lipid that constitutes 10–20% of mitochondrial membrane phospholipids,28 and is a major constituent in many bacterial membranes,29e.g., about 5% of E. coli phospholipids.30 CL deficiency is associated with numerous diseases,31–34 and CL is believed to stabilize respiratory chain complexes in mitochondrial membranes.35,36 CL is also involved in proton transport along the mitochondrial membrane surface.37 The unique structure of CL, which resembles two phospholipids joined together via the head groups, yields a conical shape that is the origin of its curvature sensing abilities. For example, CL localizes to the curved poles and septa of rod-shaped bacteria,26,38 and promotes polar localization of certain membrane proteins.39,40 Further, ATP synthase (which exploits energy released via transmembrane proton transport) associates with the highly curved edges of the mitochondrial membrane cristae,18 where the high curvature might also promote local enrichment of CL.
The majority of experimental studies of lipid and protein curvature sensing are based on fluorescence methods that monitor how sensor molecules partition between regions of different curvatures.8–11,16,20,24,25 There are several challenges with these experimental methods. One is the difficulty to ensure that the curvature sensor and the surrounding membrane are not influenced by dyes or other additives.41 For supported lipid bilayers,10,42 unwanted interactions with the solid surface must be avoided. Finally, fluorescence microscopy only provides the position and/or local density of the fluorescent labels,43 and therefore gives only indirect information about the structural basis of curvature sensing.
Fortunately, computer simulations have potential to provide additional details on the microscopic level, but face methodological and technological challenges of their own. Simulating curvature sensing and deformation in a flat bilayer patch relies on induced local membrane deformations which can be difficult to interpret.18,23,44–46 Curved membrane surfaces can be generated by simulating spheres47,48 or cylinders,49 but require equilibration of lipids and solute in different leaflets and compartments, which is only feasible for strongly coarse-grained membrane models. In this work, we use simulated membrane buckling50–60 and develop an elastic theory to overcome these difficulties. Our buckling method59 is based on a reaction coordinate (the arc-length) along the membrane profile, which presents a continuous range of curvatures in a single simulation, and is amenable for comparison to elastic membrane theories. In our simulations, the buckled membrane patch (Fig. 1a) consists of two equivalently deformed monolayers, which due to periodic boundary conditions does not divide the solutes into multiple closed compartments. Thus, the distributions of various species along the buckled membrane reflect their respective curvature preferences,53,54,56–60 and equilibration is limited only by in-plane lipid diffusion. We use the coarse-grained Martini model61–63 (Fig. 1b) to sample curvature dependent distributions and structural variations of CL mixed with the two other major E. coli lipids,64 1-palmitoyl-2-oleoyl phosphatidylethanolamine (POPE), and 1-palmitoyl-2-oleoyl phosphatidylglycerol (POPG). We find a strong curvature dependence on the lipid structure. The curvature dependent distributions are well explained by different intrinsic preferences for positive, zero, and negative curvature by POPG, POPE, and CL, respectively. The strength of these curvature effects is relevant to membrane structures such as mitochondrial cristae,18 and inclusion bodies resulting by over-expression of bacterial membrane proteins.12
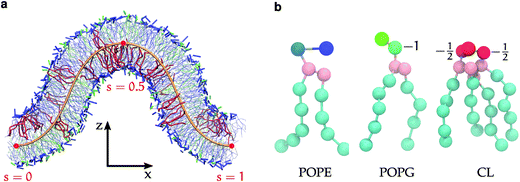 |
| Fig. 1 (a) Snapshot of a simulated trajectory fitted to the theoretical buckled shape in orange, POPE in blue, POPG in green and CL in red. The bilayer composition is 384 POPE, 104 POPG and 24 CL, and the compression factor is γ = 0.3. (b) Martini representations of POPE, POPG(−1) and CL(−1) lipids annotated by head group charges. | |
II. Methods
A. Coarse-grained molecular dynamics simulations
All molecular dynamics (MD) simulations were run with the Gromacs 4.6 program65 employing the MARTINI force field for lipids,61,66–68 and coarse-grained polarizable water,63 with relative permittivity εr = 2.5. The production trajectories were simulated at 300 K with the Bussi velocity rescaling thermostat69 (coupling time constant of 1 ps), semiisotropic pressure coupling with the Parrinello–Rahman barostat at 1 bar (12 ps time constant), and constant area in the xy-plane. van der Waals interactions were modeled with a Lennard-Jones potential, shifted to zero between 0.9 and 1.2 nm. Electrostatics were handled using the particle-mesh Ewald method,70 with a real-space cutoff of 1.4 nm. These were the parameters used in the original Martini parameterization.61 We used a time-step of 25 fs and VMD71 was employed to visualize simulated trajectories.
A buckled bilayer is produced by assembling a flat bilayer in the xy-plane with a specified Lx/Ly ratio (Lx > Ly). After the bilayer is equilibrated, the bilayer is compressed along the x-direction, keeping the length of the simulation box in the y-direction fixed. The compression is performed by rescaling the simulation box instantly by a certain compression factor Lx/L = 1 − γ (where γ = 0 indicates a flat bilayer). The curved bilayer is energy minimized, re-equilibrated, and then simulated in production runs of 20–30 μs. We computed lipid autocorrelations to estimate sampling times, and found that single lipids are correlated for 120 ns in a pure POPE bilayer (details are given in Section S1 and Fig. S1 in the ESI†). Since the autocorrelation time is closely linked to lipid diffusion, we expect this result to approximately hold for all the simulated lipid systems. The first 1 μs was then discarded as equilibration in all simulations.
The simulated bilayers contained 512 lipid molecules, but with varying lipid composition. The charged systems were neutralized with counterions, and the amount of water in the systems corresponds to fully hydrated bilayers.72 The ratio between the lateral box lengths was Lx/Ly ≈ 4.5. Two single-component systems with 512 POPG and 512 POPE were included. The other simulations were multicomponent systems, with 384 POPE lipids, 0, 8 or 24 CLs, and the rest being POPG lipids. We used a CL model developed for Martini68 that corresponds to di-[1-palmitoyl-2-oleoyl-phosphatidylglycerol], corresponding to the most common type of cardiolipin found in E. coli membranes.73 The phosphate head groups (Fig. 1b) of POPG and CL are charged (−1). We used a symmetric head charge distribution for CL, with −0.5 charge units on each phosphate group, conforming to recent experimental data.74–76 We simulated two extra replicas of the system with 24 CL molecules (CL12): one with added salt (at 0.15 M), and one with a higher degree of buckling (γ = 0.4 compared to the other simulations at γ = 0.3). Since the presence of divalent cations can decrease the molecular surface area77 so that CL undergoes a lamellar-to-hexagonal (La-HII) phase transition,78 we used only monovalent ions (Na+ and Cl−) to neutralize and/or change the salt concentrations in the simulated systems. Table 1 summarizes the simulations carried out in this work. Note that all reported times are actual simulation times and have not been scaled with the semi-universal factor of 4 sometimes employed for the Martini model.79
Table 1 Molecular dynamics simulations, with varying lipid compositions, carried out in this work. The systems are listed by lipid composition, ion concentrations, compression factors γ, simulation box sizes and simulation times. All systems contain ∼20
000 polarizable water beads, except allPG and CL4, which are smaller in the z-direction and contain ∼11
000 polarizable water beads. The reported times are actual simulation times (i.e., they have not been multiplied by any speed-up factor)
|
POPG |
POPE |
CL |
Na+ |
Cl− |
γ
|
L
x
·Ly [nm2] |
L
z
[nm] |
Sim. time [μs] |
allPG |
512 |
0 |
0 |
512 |
0 |
0.3 |
113.4 |
18.7 |
20 |
allPE |
0 |
512 |
0 |
0 |
0 |
0.3 |
114.0 |
30.0 |
20 |
CL0 |
128 |
384 |
0 |
128 |
0 |
0.3 |
115.2 |
27.7 |
30 |
CL4 |
120 |
384 |
8 |
128 |
0 |
0.3 |
115.3 |
17.2 |
30 |
CL12 |
104 |
384 |
24 |
128 |
0 |
0.3 |
120.3 |
28.4 |
30 |
CL12s |
104 |
384 |
24 |
335 |
207 |
0.3 |
119.7 |
25.1 |
30 |
CL12b |
104 |
384 |
24 |
128 |
0 |
0.4 |
105.4 |
32.1 |
30 |
B. Trajectory analysis
A flat bilayer that is compressed along its long axis adopts a buckled profile (Fig. 1a). The buckled bilayer is subject to random fluctuations, in particular in the phase of the buckled shape. To extract accurate lipid position data from the simulation, we therefore align each trajectory frame to a reference shape. This is carried out by fitting the membrane surface defined by the innermost lipid tail-beads to a theoretical buckled shape, indicated by a orange line in Fig. 1a. The procedure is described in detail in ref. 59, and briefly summarized here for convenience. We have also applied the method to study curvature sensing by amphipathic peptides.56,57
The membrane shape obtained with this buckling procedure is the two-dimensional analogue to the compressed elastic rod known as the Euler elastica.51,52 A theoretical description of the bilayer membrane as a thin elastic sheet leads, via a Helfrich functional model,80 to a parameterization of the elastica in terms of the arc-length parameter 0 ≤ s ≤ 1 (Fig. 1(a)). The free energy is56
| 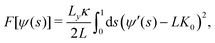 | (1) |
where
L is the length of the uncompressed bilayer,
Ly is the projected length in the long box dimension,
κ is the mean curvature modulus,
K0 is the spontaneous curvature of the bilayer, and
ψ(
s) is the angle between the
x-axis and the local tangential vector on the membrane midplane.
F is minimized with the projected length
Lx kept fixed. The differential equation obtained from this minimizing procedure is solved with periodic boundary conditions, which leads to a closed expression for the angle as function of the arc-length,
ψ(
s). This solution, however, is given in terms of Jacobi elliptic functions,
51,52 and the Cartesian coordinates of the buckled shape, (
X(
s),
Z(
s)), need to be obtained by integration of the sine and cosine of
ψ(
s).
As an alternative, we have developed a numerical method which avoids special functions and efficiently extracts accurate values for X(s) and Z(s) from eqn (1).59 This method relies on Fourier expansions of X(s) and Z(s), with Fourier coefficients stored in lookup-tables for fast evaluation. The heart of the algorithm is to minimize the sum-of-square distances from the innermost lipid tail-beads (xj,zj) to their corresponding positions along buckled shape X(sj,γ), Z(sj,γ). The parameters in the minimization procedure are the offsets in the x and z directions, the sj (j = 1,…,N where N is the number of innermost tail-beads) which are the projected positions of the innermost tail-beads along the buckle in terms of s, and the compression factor γ (to prevent area fluctuations from biasing the fit). In practice, γ-fluctuations are only a few percent.56 Implementation details and speed and accuracy tests of this method can be found in ref. 59. An open source Matlab implementation of the Euler elastica is available within the mxdrfile package.81
The local curvature at position s is directly evaluated by the standard formula
| 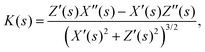 | (2) |
since
X(
s) and
Z(
s) and all their derivatives are well-defined within the numerical method. We use the reference shape fitted to the bilayer midplane, in the same way for both monolayers, in all of our analysis. This means that our curvatures are measured with respect to the bilayer midplane. The sign convention is such that positive curvature is when the membrane bends away from the probe. This means that maximum positive curvature in the upper monolayer occurs at
s = 0.5, while the curvature in the lower monolayer is given by −
K(
s), and takes its maximum positive value at
s = 0, 1. The buckled shape is symmetric around its maximum at
s = 0.5, and periodic with period
s = 1. A simulation box contains one period of the buckled shape because of the periodic boundary conditions.
Fig. 1 shows a snapshot of a buckled bilayer, annotated with the fitted shape along the bilayer midplane. Position data for membrane components relative to the buckled profile can easily be extracted from an aligned trajectory. The projected s-positions of the innermost lipid tail-beads serve as the reaction coordinate to measure the curvature preference of each lipid component.
Two recent simulation studies58,60 of lipid curvature sensing in buckled membranes used a different analysis approach. Those studies employed an analysis method based on spline-fitting the lipid head beads and then extracting the local curvature by differentiation (i.e., by eqn (2)). Our analysis of the average bead densities, shown in Fig. 2a and Fig. S2 (ESI†), strongly suggests that the distance between the planes defined by the head groups vs. the bilayer midplane is independent of curvature, so that translating between these planes is only a matter of geometry. While the spline-fitting analysis is more flexible in terms of bilayer shape, our approach makes explicit use of elasticity to parameterize possible bilayer shapes. Further, since we have a theoretical reference for the buckled shape, we can align many frames to compute averages such as Fig. 2. More importantly, the well-defined particle positions sj along the buckled shape enables direct comparison to physical models developed using the tools of statistical physics.56,59 Thus, with our approach we can move beyond a descriptive analysis and directly validate or refute different model hypotheses. Since the reference shape of our membrane buckle is based on a model of solid physical merit (the Euler elastica52), our results furnish an enduring starting platform for future refinements.
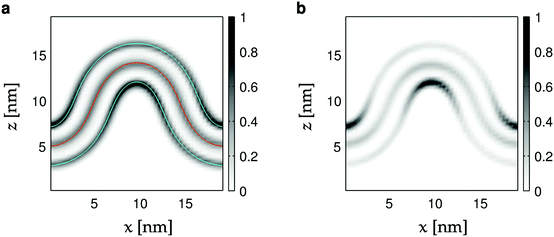 |
| Fig. 2 Heat maps of head- and tail bead densities in the xz-plane. (a) All lipid components for the CL12 simulation (Table 1). The red curve corresponds to the average fit (X(s; 〈γ〉), Z(s; 〈γ〉)). The blue curves are parallel to the first one, but displaced by 2.1 nm. (b) Same data as in panel (a) but for the CL component alone. Head bead densities have been weighted by factors 4 (POPG, POPE) and 8 (CL) to compensate for the number of tails per lipid, and the fact that the midplane contains tail beads from both monolayers. | |
C. Error analysis
We performed a thorough error analysis with bootstrapping82 to quantify the statistical errors of all calculated quantities (densities, tail order parameters, and enhancement ratios) in this work. We report all errors as standard errors of the mean (SEMs), calculated with 10
000 bootstrap realizations, and sampled from data points separated by 150 ns to ensure independence (as described in Section S1 in the ESI†). For clarity, most figures show the standard errors as shaded areas in the background, but only for the CL0, CL12 and CL12b systems. In the other systems, the errors were smaller than the symbols. In addition, Fig. S5 (ESI†) shows the error analysis for all the bilayer densities.
III. Results and discussion
Curvature sensing of lipids in buckled bilayers with varying compositions was investigated with coarse-grained molecular dynamics (MD) simulations. The lipid compositions were chosen to mimic the inner membrane of E. coli,30 and the CL concentration was varied from 0% to 5% with fixed POPE concentration. We expect the strongest curvature localization for CL, since the shape of CL is significantly more conical than that of POPE, while the shape of POPG is cylindrical. We describe the buckling effects with respect to packing in terms of the lipid components and acyl chain order parameters. Then, we measure the relative enrichment of lipid components in curved bilayer regions. Finally, we develop an analytic theory that relates local midplane curvature to lipid redistribution, and use it to extract information about membrane elastic constants.
A. Buckled shape
First, we compare the simulated buckled bilayers to their corresponding theoretical shapes. Fig. 2a shows the distribution of the innermost tail-beads and the head-beads of each leaflet (in the xz-plane) in the simulated systems. Since there are two innermost tail-beads (four for cardiolipin) for every head-bead in each of the two leaflets, and since both leaflets contribute to the tail-bead distribution, the bin counts for the head-beads are multiplied by 4 for POPG and POPE, and by 8 for cardiolipin. The middle solid curve corresponds to the theoretical buckled shape, with the average fitted value of γ, and shows excellent agreement to the simulated buckle. The outer curves are parallel to the middle one, but displaced by 2.1 nm to the planes of the head-beads. Since they follow the headgroup densities well along the whole profile (see also Fig. S3, ESI†), we conclude that the average bilayer thickness does not change with curvature.
Geometrical effects of bilayer curvature with respect to lipid packing are apparent in Fig. 2a. While the bilayer innermost tail-bead density is almost constant, the head-bead regions corresponding to negative curvature are enriched, while the opposite is true for positive curvature. This effect is much more pronounced for cardiolipin as shown in Fig. 2b.
B. Geometric effects of curvature on lipid packing
The leaflets are equivalent and symmetric since the overall buckled shape is symmetric. In particular, the local midplane curvature at a point s0 in the upper leaflet is the same at points 1 − s0 in the upper, and point s0 + 0.5 in the lower leaflet, respectively. Also, the same point s0 in the lower leaflet is subject to the same magnitude in midplane curvature, but with opposing curvature sign.
However, our simulations show asymmetric curvature dependence of the lipid distributions. This asymmetry is observed in Fig. 3a and c, which show the monolayer lipid density ρ(s) defined by the innermost tail-beads. The density function ρ(s), takes the perspective of the upper monolayer, with s = 0.5 corresponding to the region of maximal positive curvature, and s = {0, 1} corresponding to largest negative curvature. Contributions to ρ(s) from lower monolayer lipids are constructed by shifting their s-values by half a period. Fig. 3a and c show that the depletion around the region of minimal curvature is larger than the enrichment at maximal curvature. Also, the profile ρ(s) is flat around maximum curvature (s = 0.5). The bilayer midplane density
(s) is depicted in Fig. 3d. This alternate function corresponds to the distribution of the innermost tail-beads of all lipids, without regard to which monolayer they occupy. Fig. 3d shows that this density does not deviate more than a few percent from
(s) = 1, yet the deviations are systematic and consistent with the monolayer asymmetry.
(s) is maximal around s = {0.25, 0.75}, where the bilayer is flat and lipid packing is tightest (densities are normalized as probability densities). Interestingly, we have previously seen the opposite trend for an even more coarse-grained three-bead lipid model.59
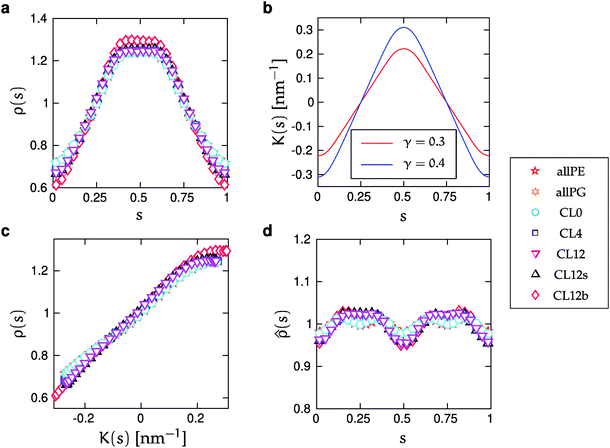 |
| Fig. 3 The lipid distribution along the curved bilayers. (a) The monolayer density ρ(s) for all lipids as a function of s. (b) Curvature, K(s), (defined in eqn (2)) as function of s for the two γ-values used in this work. (c) Monolayer density ρ(s) for all lipids as a function of curvature K(s). Symbols correspond to different lipid compositions, degrees of buckling and salt concentrations (Table 1). The s-values correspond to the upper monolayer. The s-positions of the lower monolayer have been translated by half a period, according to ρ(s) = (ρupper(s) + ρlower(s + 0.5))/2. (d) Bilayer midplane density (s), which includes both monolayers. The sizes of the standard errors of the mean (SEMs) is smaller than the symbols in panels (a) and (c). In panel (d), SEMs are slightly larger than the symbols but are omitted for clarity. Fig. S5 in the ESI† shows a scaled-up version of this figures with SEMs. | |
C. Curvature-dependent lipid structure
We calculated lipid acyl chain order parameters,
, for the tails83 as functions of local midplane curvature (eqn (2)) to investigate how lipid structure is affected by curvature. The lipids are binned according to their s-positions, and averaged over all lipids in each bin for the entire trajectory. Fig. 4 shows the order parameter 〈P2〉, which is an average over the 4 tail bead-to-tail bead bonds, for saturated and unsaturated tails. The 〈P2〉-parameters for both kinds of tails follow similar trends as functions of curvature, but differ in magnitude and in the larger slope for the saturated tails in the positive curvature region. The chain order parameters for POPG and POPE are very similar, as expected due to their identical lipid tails. The 〈P2〉-value is slightly larger for POPG in all cases except for the single component simulations. The likely explanation is that the charged headgroup keeps POPG molecules more elongated (Fig. S3 in the ESI† shows that, for the CL12 system, the planes formed by POPG head beads are farther away from the midplane compared to POPE and CL, which are at indistinguishable distances). The chain order parameter is maximized at close-to-flat regions (K ≈ 0), and minimized in the most negative curvature region for CL, and at the most positive curvatures for POPG and POPE. Taking both kinds of tails into account, this variation is considerably stronger for CL, indicating that a possible mechanism for CL curvature sensing is its ability to spread its tails at negative curvatures. In all lipids, the chain order parameter profiles for saturated tails decrease more rapidly as positive curvature increases than their unsaturated counterparts. This difference is larger for POPG and POPE compared to CL, reinforcing CL to avoid positive curvature regions. These two observations suggest that the curvature sensing properties of CL can be tuned by tweaking its tail composition.60
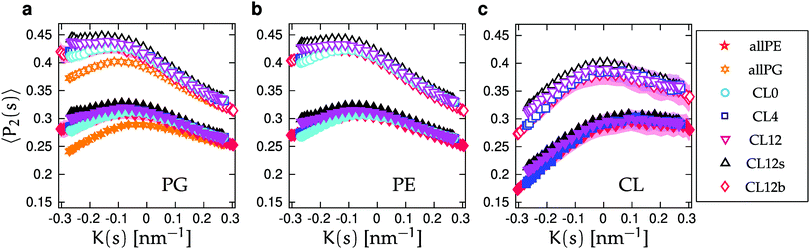 |
| Fig. 4 Average order parameter 〈P2〉 as function of K(s) for saturated (open symbols) and unsaturated (solid symbols) tails. Panels correspond to (a) POPG, (b) POPE, and (c) CL, and symbols to lipid bilayers with different compositions, degrees of buckling, and salt concentrations (Table 1). Shaded areas show the standard errors of the mean (SEMs), as described in Section IIC. SEMs are only shown for CL0, CL12 and CL12b for clarity. SEMs are only noticeable in the regions of largest negative curvature in (a), where POPG is depleted, and are smaller than the symbols in (b). | |
The presence of CL leads to a small increase in 〈P2〉 for the remaining lipids. We believe that this is due to the tails of CLs filling the larger available volume in regions of negative curvature. This mechanism is similar to how cholesterol can “plug the holes” in fluid bilayers that are rich in unsaturated lipids.84 Analogously, the ability for CL to stabilize protein–lipid domains has been ascribed to its ability to fill gaps in protein interfaces.85
D. Curvature-dependent lipid distributions
The different lipids sense curvature with varying strengths. In this section, we show that cardiolipin strongly prefers negative curvature regions, and outcompetes POPE for the region with most negative curvature. POPG is conversely enriched in regions with positive curvature.
Fig. 5 shows the normalized relative densities Φj of each lipid component j as function of local midplane curvature K(s). These densities are calculated as follows: From an aligned trajectory, the s-positions of the innermost tail-beads are collected to form the monolayer distributions ρj(s) for each lipid species j (Fig. S2 in the ESI†). ρj(s) is normalized to
, where fj is the fraction of lipids of species j in the monolayer. The relative densities of each lipid component are computed by dividing ρj(s) by the corresponding all-lipid density ρ(s) (shown in Fig. 3a), i.e.,
| 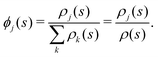 | (3) |
The relative densities are finally normalized as
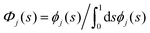
. For the two-component bilayer,
Fig. 5 shows that POPE is enriched around the region of negative curvature (
s = {0, 1}), while POPG is conversely depleted in the same region. When cardiolipin is increasingly added to the mixture, the CL density is also enriched around negative curvature, but more strongly than POPE. Thus, at large CL concentrations, it outcompetes POPE for the region of maximum negative curvature, and POPE is partially displaced.
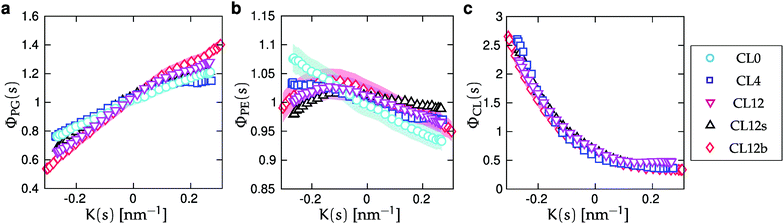 |
| Fig. 5 Normalized relative densities Φj(s) as functions of curvature K(s) for each lipid component. Φj(s) is calculated as the density ρj(s) (Fig. S2, ESI†), divided by the corresponding all-component density ρ(s) (Fig. 3), and normalized as a probability density. The panels correspond to (a) POPG, (b) POPE, and (c) CL, and symbols correspond to the simulated systems as reported in Table 1. The shaded areas show the standard errors of the mean (SEMs), as described in Section IIC. SEMs are only shown for CL0, CL12 and CL12b for clarity, and are smaller than the symbols for all values of K(s) in panels (a) and (c). | |
CL localization to negative curvature depends not only on its shape,60 but can also be affected by its headgroup charge.67,86 However, the ionization state of the CL headgroup has been heatedly debated in the literature (see ref. 87 and the references therein), partly because of its potential role in the molecular pathways for Barth syndrome33 and/or as a proton reservoir for proton-pumping respiratory enzymes.37 Recent FT-IR measurements,76 titration experiments,75 and electrokinetic/spectroscopic methods74 lend convincing support to CL being fully ionized at physiological conditions, with a symmetric charge distribution (−1 on each phosphate head group). We used a reduced-charge model of CL with −0.5 charge units on each phosphate group, to partially balance the overestimated electrostatic interactions in the Martini model (which uses relative permittivity εr = 2.5 with the polarizable water model).
We tested if increased charge screening can enhance CL curvature sensing in a simulation with 0.15 M salt concentration (CL12s), which is equivalent to that in blood plasma at physiological conditions. We found only minor changes to all calculated quantities (lipid distributions, chain order parameters, and enhancement ratios, as shown in Fig. 3–6) compared to their salt-free counterparts. The differences are most pronounced, but within 1–2%, at high positive and negative curvatures, and negligible at intermediate curvatures. These observations are consistent with earlier simulations58 that also showed a small effect on the degree of CL localization when the head group charge changed from −1 e to −2 e.
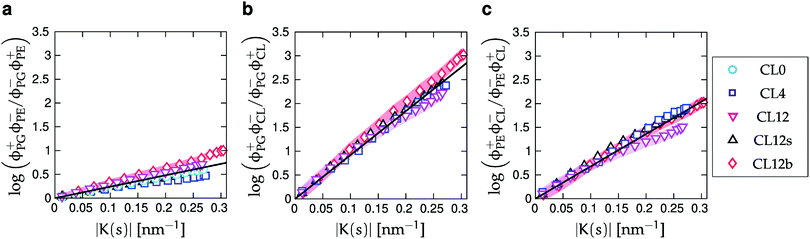 |
| Fig. 6 Enhancement ratios for the two- and three-component simulations. ϕ+j(s) is the local relative density of lipid species j in regions of positive curvature (an innermost lipid tail bead with s ∈ (0.25, 0.75) corresponds to positive curvature if it resides in the upper monolayer), and ϕ−j(s) denotes the relative density of lipids in negative curvature regions. Black lines are least-squares fits to eqn (10). Shaded areas show the standard errors of the mean (SEMs), as described in Section IIC, for the CL0, CL12 and CL12b systems. | |
Chen et al. have shown that 1,2-dioleoyl-sn-glycero-3-phosphoethanolamine (DOPE) is more prone than CL to assemble into highly negative curvature structures in the Hexagonal II (HII) phase.88 Furthermore, CL curvature sensing depended on the presence of divalent calcium ions. This is most likely a characteristic of the HII phase, where CL curvature sensing is strongly suppressed by electrostatic repulsion between headgroup charges. The dominating factor in our simulations of the buckled lamellar phase is lipid packing frustration.
E. Quantitative analytical model of relative densities
We now develop an analytical theory for three-component lipid mixtures to provide a quantitative analysis of the competition between lipid components for the most favorable curvature region. We validate the theory by deriving a linear relationship between curvature and the logarithm of the relative mole fractions of different lipids in opposing monolayers, the so-called enhancement ratios. As in our previous work,56,57,59 we consider the buckled bilayer shape to be fixed, as enforced by the simulation geometry, and model the lipid species distribution along the buckled profile.
Following ref. 3 and 47, we model the curvature dependent energy per lipid molecule of species j in a monolayer with the harmonic expression
| 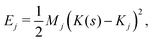 | (4) |
where
K(
s) is the midplane curvature,
Kj is the lipid's preferred curvature, and
Mj is the bending modulus per molecule,
i.e., the bending modulus times the area per lipid. The mixing entropy in the monolayer per molecule is given by the ideal gas expression
| S = −kB(log ϕj(s) − 1), | (5) |
where
ϕj(
s) denotes the local mole fraction of lipid species
j, defined in
eqn (3). The free energy per leaflet becomes
| 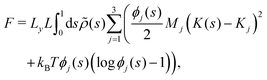 | (6) |
which is subject to the constraints
| 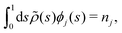 | (7) |
and
|  | (8) |
where
![[small rho, Greek, tilde]](https://www.rsc.org/images/entities/i_char_e0e4.gif)
(
s) is the all-lipid number density, and
nj is the number of lipids of species
j in the monolayer. Minimizing with respect to
ϕj(
s) yields
|  | (9) |
where
μj are Lagrange multipliers from the condition in
eqn (7), and
α(
s) enforces the point-wise constraint in
eqn (8) (see the ESI
† for details of these calculations). Since we use the midplane curvature, opposing monolayers have the same curvatures but with opposing signs at any
s value. Moreover, the terms
α(
s) and
μj can be made to cancel if we compute ratios of relative fractions in opposing leaflets. Such considerations lead to quite simple relations of the form
|  | (10) |
where
ϕ+i and
ϕ−i denote the molar fractions in opposing leaflets of positive and negative curvature, respectively. As lipids partition between regions of positive and negative curvature,
eqn (10) characterizes the competition between lipid species with different curvature preferences. Analyzing our simulation results in this way in
Fig. 6, we see excellent agreement with the predicted linear relationship. From the slopes in
Fig. 6, we find weak competition between POPG and POPE, (
KPGMPG −
KPEMPE ≈ 1.2
kBT nm), and strong competition between POPG and CL (
KPGMPG −
KCLMCL ≈ 4.6
kBT nm), which makes sense since these lipids prefer curvatures of opposing sign. The slope of the line of the remaining pair, POPE and CL (
KPEMPE −
KCLMCL ≈ 3.4
kBT nm), quantifies the extent to which CL outcompetes POPE for negative curvature, as seen qualitatively in
Fig. 5, and in a previous study of lipid distribution in buckled Martini membranes.
58
A direct comparison of our simulations with experimental elastic constants is not possible, since we only extract combinations of products of the bending moduli and intrinsic curvatures for the lipid species. However, it is possible to do a consistency check on the product of elastic constants by assuming typical literature values for the bending moduli89 (25kBT) and area per lipids90 (0.7 nm2). With these numbers for all lipid species, the extracted products of bending modulus and intrinsic curvatures can be solved to yield the intrinsic curvature differences KPG − KPE ≈ 0.06 nm−1, KPG − KCL ≈ 0.26 nm−1, and KPE − KCL ≈ 0.17 nm−1. These differences are similar in magnitude to the experimentally estimated intrinsic curvatures of CL (−1 nm−1 to −0.2 nm−1)91 as well as other lipids.24,92
Finally, we emphasize that although Fig. 6 shows enhancement ratios versus curvature, the comparison is based on number densities along the buckled profile. Our analysis therefore relies on our ability to extract lipid arc-length positions sj from the simulations, which is also the reason for the unequal spacing of points along the K(s)-axes in Fig. 6.56,59
IV. Conclusions
In this work, we used a computational approach to investigate curvature sensing in coarse-grained models of three-component lipid bilayers with varying concentrations of cardiolipin (CL), which is known experimentally to aggregate in membrane regions of negative curvature.85
The shapes of the simulated buckled bilayers were in excellent agreement with predictions from elastic theory,51,52 and we observed no curvature dependent bilayer thinning for any of the lipid mixtures. We found that the curvature dependence of the lipid packing was asymmetric, with the density depletion being largest at the minimal curvatures. Our analysis shows that CL lipids strongly prefer membrane regions with negative curvatures. POPE lipids also prefer negative curvatures, but more weakly, and are outcompeted for the most negative curvatures by CL. POPG lipids show opposite behavior and are enriched in the regions on positive curvature. The relative preferences of CL and PE for negative curvature agree qualitatively with the observations of Boyd et al.58 They studied mixtures of POPE, POPC, and CL in a buckled geometry using the Martini model and a simulation setup similar to the one used here, but with a different analysis method.
Since lipid redistribution can stabilize curved membranes,93 we calculated the chain order parameters of the lipid tails along the curved surface to assess the curvature dependence of the lipid structures. We found the largest decrease in the chain order parameter in regions of maximal negative curvature for CL, where it is most concentrated. This is consistent with a geometric mechanism for curvature sensing, with cardiolipin “repairing” local membrane deformations in the curved structure.
Finally, we developed and validated a Helfrich-type elastic model that quantifies the competition among lipid components for the most favorable curvature region. The theory provides a simple relation between enhancement ratios47 for multicomponent membranes and the local curvature, and can be used to determine some of the membrane's material constants.
CL has theoretically been predicted to form clusters that localize to the poles of E. coli membranes once a critical concentration threshold is reached, partly driven by lipid–lipid interactions.27 We see no significant concentration dependence of our CL enhancement ratios (Fig. 6b and c), as expected for a model without lipid–lipid interactions (eqn (6)). However, while the nominal CL concentration in our simulations reach well above the predicted threshold of 1%,85 the absolute number of CL molecules may be too small to form clusters. We also probe curvatures more than an order of magnitude larger than those of bacterial cell poles, so it is perhaps not surprising that the curvature mismatch energy strongly dominates in our results. However, this regime is still of biological relevance for the extreme curvatures present for example in the mitochondrial inner membrane.18
CL is the signature lipid of mitochondria. Several proteins bind cardiolipin with high affinity, including respiratory complexes I, III, IV and V.94 The efficiency of ATP synthase is linked to high membrane curvature in mitochondrial cristae,95,96 and specific interactions between CL and the rotary mechanism of ATP synthase.97 CL segregation in the highly curved mitochondrial inner membrane may also enhance ATP synthase function by acting as a proton sink.98 The computations and analysis reported in this work are stepping stones for the theoretical explanation of curvature sensing by cardiolipin in lipid membranes at a molecular level, and can be generalized to study the general interplay between shape and composition in biological membranes.
Conflicts of interest
There are no conflicts to declare.
Acknowledgements
This work has been supported by the Swedish Research Council (Vetenskapsrådet), the EU Horizon 2020 “SmartNanoTox” project, and the Swedish Foundation for Strategic Research via the Center for Biomembrane Research. The simulations were performed on resources provided by the Swedish National Infrastructure for Computing (SNIC) at the PDC Center for High Performance Computing at the KTH Royal Institute of Technology, the National Supercomputer Center at Linköping University, and the High Performance Computing Center North at Umeå University.
References
- Y. Shibata, J. Hu, M. M. Kozlov and T. A. Rapoport, Annu. Rev. Cell Dev. Biol., 2009, 25, 329–354 CrossRef CAS PubMed.
- T. R. Graham and M. M. Kozlov, Curr. Opin. Cell Biol., 2010, 22, 430–436 CrossRef CAS PubMed.
- T. Baumgart,
et al.
, Annu. Rev. Phys. Chem., 2011, 62, 483–506 CrossRef CAS PubMed.
- H. T. McMahon and J. L. Gallop, Nature, 2005, 438, 590–596 CrossRef CAS PubMed.
- J. Zimmerberg and M. M. Kozlov, Nat. Rev. Mol. Cell Biol., 2006, 7, 9–19 CrossRef CAS PubMed.
- R. Phillips, T. Ursell, P. Wiggins and P. Sens, Nature, 2009, 459, 379–385 CrossRef CAS PubMed.
- B. J. Peter, H. M. Kent, I. G. Mills, Y. Vallis, P. J. G. Butler, P. R. Evans and H. T. McMahon, Science, 2004, 303, 495–499 CrossRef CAS PubMed.
- B. Sorre, A. Callan-Jones, J. Manzi, B. Goud, J. Prost, P. Bassereau and A. Roux, Proc. Natl. Acad. Sci. U. S. A., 2012, 109, 173–178 CrossRef CAS PubMed.
- P. Ramesh, Y. F. Baroji, S. N. S. Reihani, D. Stamou, L. B. Oddershede and P. M. Bendix, Sci. Rep., 2013, 3, 1565 CrossRef PubMed.
- W.-T. Hsieh, C.-J. Hsu, B. R. Capraro, T. Wu, C.-M. Chen, S. Yang and T. Baumgart, Langmuir, 2012, 28, 12838–12843 CrossRef CAS PubMed.
- C. Zhu, S. L. Das and T. Baumgart, Biophys. J., 2012, 102, 1837–1845 CrossRef CAS PubMed.
- H. M. Eriksson, P. Wessman, C. Ge, K. Edwards and Å. Wieslander, J. Biol. Chem., 2009, 284, 33904–33914 CrossRef CAS PubMed.
- J. C. Stachowiak, E. M. Schmid, C. J. Ryan, H. S. Ann, D. Y. Sasaki, M. B. Sherman, P. L. Geissler, D. A. Fletcher and C. C. Hayden, Nat. Cell Biol., 2012, 14, 944–949 CrossRef CAS PubMed.
- A. Tonnesen, S. M. Christensen, V. Tkach and D. Stamou, Biophys. J., 2014, 106, 201–209 CrossRef CAS PubMed.
- N. W. Schmidt, A. Mishra, J. Wang, W. F. DeGrado and G. C. Wong, J. Am. Chem. Soc., 2013, 135, 13710–13719 CrossRef CAS PubMed.
- S. Aimon, A. Callan-Jones, A. Berthaud, M. Pinot, G. E. Toombes and P. Bassereau, Dev. Cell, 2014, 28, 212–218 CrossRef CAS PubMed.
- I. Arechaga, B. Miroux, S. Karrasch, R. Huijbregts, B. de Kruijff, M. J. Runswick and J. E. Walker, FEBS Lett., 2000, 482, 215–219 CrossRef CAS PubMed.
- K. M. Davies, C. Anselmi, I. Wittig, J. D. Faraldo-Gómez and W. Kühlbrandt, Proc. Natl. Acad. Sci. U. S. A., 2012, 109, 13602–13607 CrossRef CAS PubMed.
- G. Drin, J.-F. Casella, R. Gautier, T. Boehmer, T. U. Schwartz and B. Antonny, Nat. Struct. Mol. Biol., 2007, 14, 138–146 CrossRef CAS PubMed.
- N. S. Hatzakis, V. K. Bhatia, J. Larsen, K. L. Madsen, P.-Y. Bolinger, A. H. Kunding, J. Castillo, U. Gether, P. Hedegård and D. Stamou, Nat. Chem. Biol., 2009, 5, 835–841 CrossRef CAS PubMed.
- V. K. Bhatia, K. L. Madsen, P.-Y. Bolinger, A. Kunding, P. Hedegård, U. Gether and D. Stamou, EMBO J., 2009, 28, 3303–3314 CrossRef CAS PubMed.
- N. Mizuno, J. Varkey, N. C. Kegulian, B. G. Hegde, N. Cheng, R. Langen and A. C. Steven, J. Biol. Chem., 2012, 287, 29301–29311 CrossRef CAS PubMed.
- A. R. Braun, E. Sevcsik, P. Chin, E. Rhoades, S. Tristram-Nagle and J. N. Sachs, J. Am. Chem. Soc., 2012, 134, 2613–2620 CrossRef CAS PubMed.
- M. M. Kamal, D. Mills, M. Grzybek and J. Howard, Proc. Natl. Acad. Sci. U. S. A., 2009, 106, 22245–22250 CrossRef CAS PubMed.
- B. Sorre, A. Callan-Jones, J.-B. Manneville, P. Nassoy, J.-F. Joanny, J. Prost, B. Goud and P. Bassereau, Proc. Natl. Acad. Sci. U. S. A., 2009, 106, 5622–5626 CrossRef CAS PubMed.
- L. D. Renner and D. B. Weibel, Proc. Natl. Acad. Sci. U. S. A., 2011, 108, 6264–6269 CrossRef CAS PubMed.
- R. Mukhopadhyay, K. C. Huang and N. S. Wingreen, Biophys. J., 2008, 95, 1034–1049 CrossRef CAS PubMed.
- G. Daum, Biochim. Biophys. Acta, Rev. Biomembr., 1985, 822, 1–42 CrossRef CAS.
- C. Sohlenkamp and O. Geiger, FEMS Microbiol. Rev., 2016, 40, 133–159 CrossRef CAS PubMed.
- S. Sundararaj, A. Guo, B. Habibi-Nazhad, M. Rouani, P. Stothard, M. Ellison and D. S. Wishart, Nucleic Acids Res., 2004, 32, D293–D295 CrossRef CAS PubMed.
- A. J. Chicco and G. C. Sparagna, Am. J. Physiol.: Cell Physiol., 2007, 292, C33–C44 CrossRef CAS PubMed.
- H. K. Saini-Chohan, M. G. Holmes, A. J. Chicco, W. A. Taylor, R. L. Moore, S. A. McCune, D. L. Hickson-Bick, G. M. Hatch and G. C. Sparagna, J. Lipid Res., 2009, 50, 1600–1608 CrossRef CAS PubMed.
- M. Schlame and M. Ren, FEBS Lett., 2006, 580, 5450–5455 CrossRef CAS PubMed.
- S. M. Claypool and C. M. Koehler, Trends Biochem. Sci., 2012, 37, 32–41 CrossRef CAS PubMed.
- S. M. Claypool, Y. Oktay, P. Boontheung, J. A. Loo and C. M. Koehler, J. Cell. Biol., 2008, 182, 937–950 CrossRef CAS PubMed.
- D. Acehan, A. Malhotra, Y. Xu, M. Ren, D. L. Stokes and M. Schlame, Biophys. J., 2011, 100, 2184–2192 CrossRef CAS PubMed.
- T. H. Haines and N. A. Dencher, FEBS Lett., 2002, 528, 35–39 CrossRef CAS PubMed.
- F. Kawai, M. Shoda, R. Harashima, Y. Sadaie, H. Hara and K. Matsumoto, J. Bacteriol., 2004, 186, 1475–1483 CrossRef CAS PubMed.
- T. Romantsov, A. R. Battle, J. L. Hendel, B. Martinac and J. M. Wood, J. Bacteriol., 2010, 192, 912–924 CrossRef CAS PubMed.
- T. Romantsov, S. Helbig, D. E. Culham, C. Gill, L. Stalker and J. M. Wood, Mol. Microbiol., 2007, 64, 1455–1465 CrossRef CAS PubMed.
- J. L. Alejo, S. C. Blanchard and O. S. Andersen, Biophys. J., 2013, 104, 2410–2418 CrossRef CAS PubMed.
- J. C. Black, P. P. Cheney, T. Campbell and M. K. Knowles, Soft Matter, 2014, 10, 2016 RSC.
- P. Ferrand, P. Gasecka, A. Kress, X. Wang, F.-Z. Bioud, J. Duboisset and S. Brasselet, Biophys. J., 2014, 106, 2330–2339 CrossRef CAS PubMed.
- C. Ge, J. Gómez-Llobregat, M. J. Skwark, J.-M. Ruysschaert, Å. Wieslander and M. Lindén, FEBS J., 2014, 281, 3667–3684 CrossRef CAS PubMed.
- P. D. Blood and G. A. Voth, Proc. Natl. Acad. Sci. U. S. A., 2006, 103, 15068–15072 CrossRef CAS PubMed.
- B. J. Reynwar, G. Illya, V. A. Harmandaris, M. M. Muller, K. Kremer and M. Deserno, Nature, 2007, 447, 461–464 CrossRef CAS PubMed.
- I. R. Cooke and M. Deserno, Biophys. J., 2006, 91, 487–495 CrossRef CAS PubMed.
- H. J. Risselada and S. J. Marrink, Phys. Chem. Chem. Phys., 2009, 11, 2056–2067 RSC.
- V. A. Harmandaris and M. Deserno, J. Chem. Phys., 2006, 125, 204905 CrossRef PubMed.
- W. K. d. Otter, J. Chem. Phys., 2005, 123, 214906 CrossRef PubMed.
- H. Noguchi, Phys. Rev. E: Stat., Nonlinear, Soft Matter Phys., 2011, 83, 061919 CrossRef PubMed.
- M. Hu, P. Diggins IV and M. Deserno, J. Chem. Phys., 2013, 138, 214110 CrossRef PubMed.
- H. Wang, J. de Joannis, Y. Jiang, J. C. Gaulding, B. Albrecht, F. Yin, K. Khanna and J. T. Kindt, Biophys. J., 2008, 95, 2647–2657 CrossRef CAS PubMed.
- H. Cui, E. Lyman and G. A. Voth, Biophys. J., 2011, 100, 1271–1279 CrossRef CAS PubMed.
- Q.-Y. Wu and Q. Liang, Langmuir, 2014, 30, 1116–1122 CrossRef CAS PubMed.
- J. Gómez-Llobregat, F. Elías-Wolff and M. Lindén, Biophys. J., 2016, 110, 197–204 CrossRef PubMed.
- A. Martyna, J. Gomez-Llobregat, M. Lindén and J. S. Rossman, Biochemistry, 2016, 55, 3493–3496 CrossRef CAS PubMed.
- K. J. Boyd, N. N. Alder and E. R. May, Langmuir, 2017, 33, 6937–6946 CrossRef CAS PubMed.
- F. Elías-Wolff, M. Lindén, A. P. Lyubartsev and E. G. Brandt, J. Chem. Theory Comput., 2018, 14, 1643–1655 CrossRef PubMed.
- K. J. Boyd, N. N. Alder and E. R. May, Biophys. J., 2018, 114, 2116–2127 CrossRef CAS PubMed.
- S. J. Marrink, H. J. Risselada, S. Yefimov, D. P. Tieleman and A. H. De Vries, J. Phys. Chem. B, 2007, 111, 7812–7824 CrossRef CAS PubMed.
- D. H. de Jong, G. Singh, W. D. Bennett, C. Arnarez, T. A. Wassenaar, L. V. Schäfer, X. Periole, D. P. Tieleman and S. J. Marrink, J. Chem. Theory Comput., 2012, 9, 687–697 CrossRef PubMed.
- S. O. Yesylevskyy, L. V. Schäfer, D. Sengupta and S. J. Marrink, PLoS Comput. Biol., 2010, 6, e1000810 CrossRef PubMed.
- C. Raetz and W. Dowhan, J. Biol. Chem., 1990, 265, 1235–1238 CAS.
- B. Hess, C. Kutzner, D. van der Spoel and E. Lindahl, J. Chem. Theory Comput., 2008, 4, 435–447 CrossRef CAS PubMed.
- S. J. Marrink, A. H. De Vries and A. E. Mark, J. Phys. Chem. B, 2004, 108, 750–760 CrossRef CAS.
- M. Dahlberg, J. Phys. Chem. B, 2007, 111, 7194–7200 CrossRef CAS PubMed.
- M. Dahlberg and A. Maliniak, J. Chem. Theory Comput., 2010, 6, 1638–1649 CrossRef CAS PubMed.
- G. Bussi, D. Donadio and M. Parrinello, J. Chem. Phys., 2007, 126, 014101 CrossRef PubMed.
- U. Essmann, L. Perera, M. L. Berkowitz, T. Darden, H. Lee and L. G. Pedersen, J. Chem. Phys., 1995, 103, 8577–8593 CrossRef CAS.
- W. Humphrey, A. Dalke and K. Schulten, J. Mol. Graphics, 1996, 14, 33–38 CrossRef CAS PubMed.
-
S. Tristram-Nagle, Membrane Hydration: The Role of Water in the Structure and Function of Biological Membranes, Springer International Publishing, Cham, 2015, ch. 2, pp. 17–43 Search PubMed.
- M. Batley, N. H. Packer and J. W. Redmond, Biochim. Biophys. Acta, Lipids Lipid Metab., 1982, 710, 400–405 CrossRef CAS.
- M. Sathappa and N. N. Alder, Biochim. Biophys. Acta, Biomembr., 2016, 1858, 1362–1372 CrossRef CAS PubMed.
- G. Olofsson and E. Sparr, PLoS One, 2013, 8, 1–6 Search PubMed.
- D. Malyshka, L. A. Pandiscia and R. Schweitzer-Stenner, Vib. Spectrosc., 2014, 75, 86–92 CrossRef CAS.
- J. A. Killian, M. C. Koorengevel, J. A. Bouwstra, G. Gooris, W. Dowhan and B. de Kruijff, Biochim. Biophys. Acta, Biomembr., 1994, 1189, 225–232 CrossRef CAS.
- A. Ortiz, J. A. Killian, A. J. Verkleij and J. Wilschut, Biophys. J., 1999, 77, 2003–2014 CrossRef CAS PubMed.
- S. J. Marrink and D. P. Tieleman, Chem. Soc. Rev., 2013, 42, 6801–6822 RSC.
- W. Helfrich, Z. Naturforsch., C: Biochem., Biophys., Biol., Virol., 1973, 28, 693–703 CAS.
-
J. Kapla and M. Lindén, 2018, arXiv:1811.03012.
-
M. E. J. Newman and G. T. Barkema, Monte Carlo Methods in Statistical Physics, Oxford University Press, USA, 1999 Search PubMed.
- A. Botan, F. Favela-Rosales, P. F. J. Fuchs, M. Javanainen, M. Kanduč, W. Kulig, A. Lamberg, C. Loison, A. Lyubartsev, M. S. Miettinen, L. Monticelli, J. Määttä, O. H. S. Ollila, M. Retegan, T. Róg, H. Santuz and J. Tynkkynen, J. Phys. Chem. B, 2015, 119, 15075–15088 CrossRef CAS PubMed.
- S. Bhattacharya and S. Haldar, Biochim. Biophys. Acta, Biomembr., 2000, 1467, 39–53 CrossRef CAS.
- E. Mileykovskaya and W. Dowhan, Biochim. Biophys. Acta, Biomembr., 2009, 1788, 2084–2091 CrossRef CAS PubMed.
- G. L. Powell and D. Marsh, Biochemistry, 1985, 24, 2902–2908 CrossRef CAS PubMed.
- E. E. Kooijman, L. A. Swim, Z. T. Graber, Y. Y. Tyurina, H. Bayir and V. E. Kagan, Biochim. Biophys. Acta, Biomembr., 2017, 1859, 61–68 CrossRef CAS PubMed.
- Y.-F. Chen, K.-Y. Tsang, W.-F. Chang and Z.-A. Fan, Soft Matter, 2015, 11, 4041–4053 RSC.
- J. F. Nagle, M. S. Jablin, S. Tristram-Nagle and K. Akabori, Chem. Phys. Lipids, 2015, 185, 3–10 CrossRef CAS PubMed.
- N. Kučerka, M.-P. Nieh and J. Katsaras, Biochim. Biophys. Acta,
Biomembr., 2011, 1808, 2761–2771 CrossRef PubMed.
- K. C. Huang and K. S. Ramamurthi, Mol. Microbiol., 2010, 76, 822–832 CrossRef CAS PubMed.
- J. Zimmerberg and M. M. Kozlov, Nat. Rev. Mol. Cell Biol., 2006, 7, 9–19 CrossRef CAS PubMed.
- S. Leibler, J. Phys., 1986, 47, 507–516 CrossRef CAS.
- M. Schlame, D. Rua and M. L. Greenberg, Prog. Lipid Res., 2000, 39, 257–288 CrossRef CAS PubMed.
- M. Schlame and M. Ren, Biochim. Biophys. Acta, Biomembr., 2009, 1788, 2080–2083 CrossRef CAS PubMed.
- M. Strauss, G. Hofhaus, R. R. Schröder and W. Kühlbrandt, EMBO J., 2008, 27, 1154–1160 CrossRef CAS PubMed.
- A. L. Duncan, A. J. Robinson and J. E. Walker, Proc. Natl. Acad. Sci. U. S. A., 2016, 113, 8687–8692 CrossRef CAS PubMed.
-
M. Bogdanov, E. Mileykovskaya and W. Dowhan, Lipids in Health and Disease, Springer, Dordrecht, 2008, pp. 197–239 Search PubMed.
Footnotes |
† Electronic supplementary information (ESI) available. See DOI: 10.1039/c8sm02133c |
‡ Present address: Scania CV AB, Södertälje, Sweden. |
|
This journal is © The Royal Society of Chemistry 2019 |
Click here to see how this site uses Cookies. View our privacy policy here.