Overexpression of a serine hydroxymethyltransferase increases biomass production and reduces recalcitrance in the bioenergy crop Populus†
Received
24th September 2018
, Accepted 5th October 2018
First published on 2nd November 2018
Abstract
Cell wall recalcitrance is the major obstacle for plant biomass conversion to biofuels. In this study, we functionally characterized a serine hydroxymethyltransferase (SHMT) from Populus and evaluated its potential for developing lignocellulosic feedstocks. SHMT is an enzyme that plays an important role in cellular one-carbon pathways. However, little is known about its function in plant cell wall-related processes. Among nine SHMT genes in the Populus genome, PtSHMT2 was highly expressed in the developing xylem and was co-expressed with secondary cell wall biosynthetic genes. In Populus transgenic plants overexpressing PdSHMT2, the biomass yield and sugar (glucose and xylose) release were increased whereas the lignin content was decreased. Transcriptomics and metabolomics analyses revealed that genes and metabolites related to secondary cell wall biosynthesis were affected by PdSHMT2 overexpression. Based on the transcription factor binding sites of differentially expressed genes in PdSHMT2 overexpression lines, a total of 27 hub transcription factors were identified. We proposed a regulatory model of action of PdSHMT2 with transcriptional master switches of secondary cell wall biosynthesis. Collectively, these results suggest that PdSHMT2 is a promising candidate for genetic engineering to improve feedstock characteristics to enhance biofuel conversion and reduce the cost of lignocellulosic biofuel production.
Introduction
Serine hydroxymethyltransferase (SHMT, EC 2.1.2.1) is a pyridoxal phosphate-dependent enzyme that plays an important role in cellular one-carbon (C1) pathways by catalyzing the reversible conversions of L-serine to glycine and simultaneously tetrahydrofolate (THF) to 5,10-methylene THF.1 Prokaryotes have single gene encoding SHMT. In animals and fungi, two SHMT isoforms (cytosolic and mitochondrial) are encoded by two distinct genes.2 The structure of the SHMT monomer is similar across prokaryotes and eukaryotes, whereas its functional form is a dimer in prokaryotes and a tetramer in eukaryotes.1 The structure of SHMT directly affects its function. For example, for sheep liver cytosolic SHMT (scSHMT), the mutation of D227 leads to formation of inactive dimers, E74 controls the conversion between “open” and “close” forms, and K256 plays a crucial role in maintaining the tetrameric structure.1 In addition, the function of SHMT is affected by post-translational modification, such as ubiquitination and sumoylation. Anderson et al. (2012) reported that mammalian SHMT1 interacts with an E2 conjugase, Ubc13, which mediates competitional ubiquitination or sumoylation. The ubiquitination is required for SHMT1 nuclear export and increases its stability within the nucleus, whereas sumoylation of SHMT1 is involved in nuclear degradation.3 Moreover, SHMT plays a role in transcriptional regulation. For example, human cytoplasmic SHMT (hcSHMT) protein can bind mRNA and displays increased affinity for the 5′ untranslated region of its mRNA.4
To date, SHMT in plants has been reported to function in the photorespiratory pathway and stress responses. In the photorespiratory pathway, SHMT is associated with the glycine decarboxylase complex (GDC),5 which catalyzes the decarboxylation of photorespiratory Gly to yield NH3, CO2 and a C1 unit that is transferred to THF.6 Mutants of mitochondrial SHMT or GDC exhibit symptoms of chlorosis.7 In Arabidopsis, the circadian clock regulates the expression of genes encoding mitochondrial components of the photorespiratory pathway, including AtSHMT1 and AtSHMT4.8 Potato plants with antisensed SHMT have lower photosynthetic capacity and accumulate glycine in light.9 In addition, SHMT is involved in biotic and abiotic stress responses. The Arabidopsis shmt1-1 mutant is more susceptible to infection with necrotrophic and biotrophic pathogens, and salicylic acid-induced genes and H2O2 detoxification-related genes are constitutively activated in shmt1-1 plants. Under abiotic stresses, shmt1-1 plants exhibit hypersensitivity to salt stress.10 SHMT is degraded by 26S proteasome, but it can be stabilized by a ubiquitin-specific protease, UBP16, through deubiquitination of SHMT1 in Arabidopsis.11 So far, the function of SHMT in cell wall-related processes has not been reported. Recently, several other enzymes in C1 metabolism have been reported to play important roles in cell wall-related processes. In C1 metabolism, methylenetetrahydrofolate reductase (MTHFR) catalyzes the conversion of 5,10-methylenetetrahydrofolate to 5-methyltetrahydrofolate, while folylpolyglutamate synthase (FPGS) catalyzes the addition of glutamate moieties to folate and folate derivatives. Both MTHFR and FPGS can affect lignin biosynthesis in maize.12,13 Cystathionine γ-synthase (CGS) catalyzes the formation of cystathionine from cysteine and an activated derivative of homoserine whereas S-adenosylhomocysteine hydrolase (SAHH) is responsible for the reversible hydration of S-adenosyl-L-homocysteine into adenosine and homocysteine. In switchgrass, down-regulation of CGS enhanced lignin biosynthesis whereas down-regulation of SAHH reduced the lignin biosynthesis and increased cell wall saccharification efficiency.14
In vascular plants, secondary cell walls (SCWs) are the most abundant renewable plant biomass, and are widely used for many applications including energy, textiles, pulping and paper-making. SCWs are composed of lignin, cellulose and hemicelluloses. The deposition of SCWs allows cells to function as mechanical tissues for structural support and protection.15 Many transcription factors (TFs) have been identified controlling SCW biosynthesis in a hierarchical manner. In the first layer of the SCW regulatory network, SND1 and NST1 are master regulators of secondary wall biosynthesis in fibers.16 The second-layer master switches including two functional redundant MYB genes, MYB46 and MBY83, which activate the expression of the third-layer TFs and enzymes which are directly involved in SCW biosynthesis.17 MYB4 is a transcriptional repressor that negatively regulates C4H and itself in plants.18 The Arabidopsis myb4 mutant accumulates sinapate esters in leaves.19 In switchgrass, overexpressing its orthologous PvMYB4 reduced the lignin content and ester-linked p-CA
:
FA ratio and increased sugar release.20 It has been reported that the expression of MYB4 can be induced by glucose.18 However, whether other metabolites or enzymes in metabolic pathways are involved in the SCW regulatory pathway is largely unknown.
In this study, we characterized an SHMT gene in Populus encoded by the locus Potri.001G320400 (PtSHMT2), which has strong expression in the developing xylem during poplar wood formation. We overexpressed this gene in P. deltoides ‘WV94’ and found that it enhanced plant growth and altered cell wall composition and sugar release in transgenic plants. By analyzing the transcriptomics and metabolomics data using PdSHMT2 overexpression lines, we examined the potential molecular mechanisms of action of PdSHMT2 in the secondary cell wall biosynthesis and revealed the association of PdSHMT2 with the transcriptional master regulators of secondary cell wall biosynthesis. Collectively, these findings provide new insights into the design of genetic engineering leading to cost-effective biomass conversion into biofuels.
Results and discussion
SHMT family in Populus
To study the function of SHMT in Populus, we first analyzed the whole gene family of SHMT in Populus and other plant species. The SHMT genes were queried using BLAST in Populus trichocarpa genome and 11 other plant species, including three other woody species Salix purpurea, Eucalyptus grandis and Vitis vinifera, three annual dicots Arabidopsis thaliana, Medicago truncatula, and Glycine max; four monocots Oryza sativa, Zea mays, Brachypodium distachyon and Sorghum bicolor, and a moss Physcomitrella patens. A total of 95 SHMT genes were identified in these 12 species, and the SHMT family in dicots (7–15 members) is larger than that in monocots (4–7 members) (Fig. 1 and Table S1 in the ESI†). Among those nine SHMTs identified in Populus, namely PtSHMT1–9, three paralogous pairs (PtSHMT2/9, PtSHMT3/5 and PtSHMT7/8) were likely generated by a Salicoid genome duplication and rearrangement event (Fig. S1 in the ESI†).
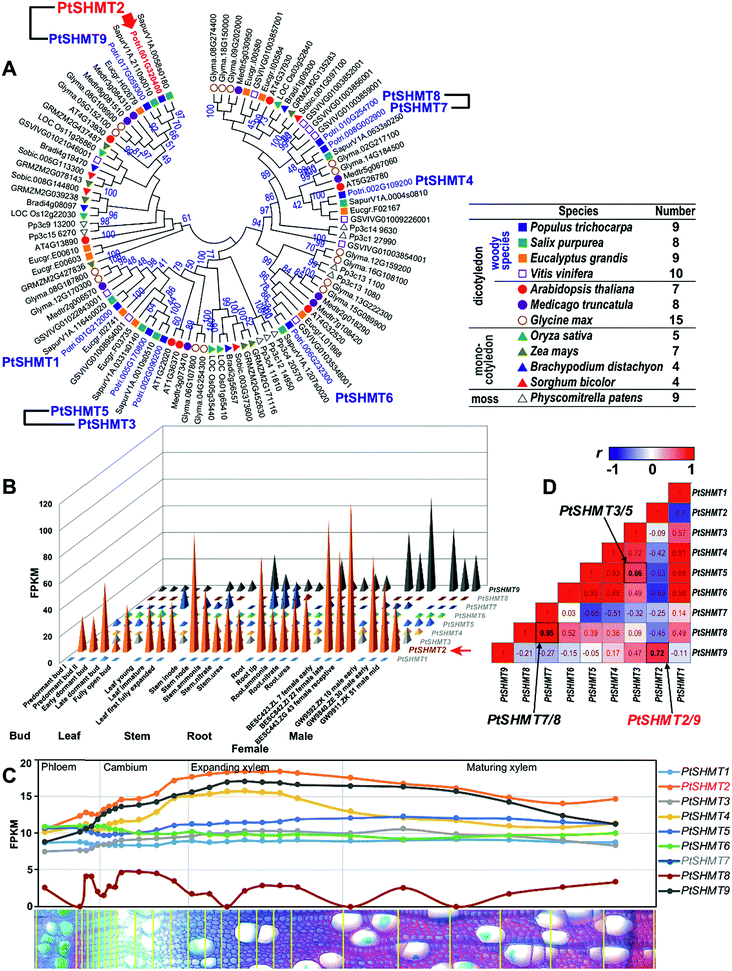 |
| Fig. 1 Phylogenetic analysis and expression patterns of SHMT genes. (A) Phylogenetic analysis. The phylogenetic tree was constructed using the full-length amino acid sequences of SHMT proteins from 12 plant species by the neighbor-joining (NJ) method with 1000 bootstrap replicates. Three PtSHMT paralogous pairs generated by a whole-genome duplication (WGD) event (Fig. S1 in the ESI†) are shown by linked black lines. (B) Expression patterns of PtSHMT genes across various tissues. (C) Expression patterns of PtSHMT genes during wood formation. The nm-scale expression of PtSHMT genes was obtained from the AspWood gene expression database.21 (D) Pairwise comparison of the Pearson correlation coefficient (r) of expression in the PtSHMT family based on results in (B) and (C). The three paralogous pairs are labelled by arrows. | |
On the basis of the expression data from different tissues of P. trichocarpa (Populus Gene Atlas) (https://phytozome.jgi.doe.gov), we compared the expression patterns of the nine PtSHMT genes. Noticeably, only PtSHMT2 and its paralog PtSHMT9 showed high abundance across poplar tissues, and PtSHMT2 was strongly expressed in the stem (Fig. 1B). Phylogenetic (Fig. 1A) and sequence similarity (Fig. S2 and S3 in the ESI†) analyses indicated that PtSHMT2 is closely clustered with AtSHMT4 (At4g13930). The pairwise correlation of the nine PtSHMT expression patterns indicated that two PtSHMT paralogous pairs (PtSHMT2/9 and PtSHMT7/8) have highly positive correlation coefficients (r = 0.72 and 0.95) compared to pair PtSHMT3/5 (r = 0.66) (Fig. 1D). In contrast, the promoter similarity of pair PtSHMT3/5 is higher than that of the other two pairs (Fig. S4 in the ESI†). The divergence between the expression and promoter sequence implies that the expression of PtSHMT paralogous pairs may be controlled by different mechanisms. Furthermore, the expression patterns of the nine PtSHMTs developed from the AspWood gene expression database were compared in nm-scale stem tissues of P. tremula.21 Among the nine PtSHMTs, PtSHMT2 has the highest abundance and was induced in expanding xylem (Fig. 1C), implying that it may play an important role in SCW-related processes.
To explore the possible regulatory mechanism of PtSHMT2 expression, we analyzed the cis-acting elements of the promoter region (3000 bp upstream of the translation initiation site). Based on the functional annotation, the identified cis-acting elements were classified into four groups – development, hormone, stress and others. Noticeably, seven circadian elements (involved in circadian control), three ABREs (involved in ABA responsiveness), two CAT-box elements (related to meristem expression) and two MYB binding sites (MBS and MRE) were identified in the promoter of PtSHMT2 (Fig. S5 in the ESI†). In Arabidopsis, the shmt1-1 mutant is defective in mitochondrial SHMT activity and displays a lethal photorespiratory phenotype when grown at the ambient CO2 level.22 The expression of genes of the photorespiratory pathway has been shown to be regulated by a circadian clock. Arabidopsis SHMT1 and SHMT4 exhibit circadian oscillation in mRNA abundance.8 This is consistent with the highest abundance of circadian elements in the promoter of PtSHMT2.
We then constructed a co-expression network of PtSHMT2 based on the global gene expression patterns across various tissues and under different stresses in poplar. According to the functional classification, a number of cell wall-related genes were identified in the co-expression network (Fig. S6 and S7 in the ESI†). These include several key transcription factors (TFs) such as NST1, SND3, MYB46, MYB103, NAC073, NAC075, KNAT7 and WOX14, and important enzymes such as LAC10, LAC17, PRX44, PRX53, MAN6, IRX2, XTHs and UGT80A2 which are known regulators of SCW biosynthesis.15,23 These results supported the role of PtSHMT2 in cell wall-related processes.
Overexpression of PdSHMT2 increases plant growth
To directly examine the potential function of SHMT2 in Populus, we cloned the full-length open reading frame of SHMT2 from P. deltoides ‘WV94’ (PdSHMT2) and overexpressed it in ‘WV94’ using the UBIQUITIN3 constitutive promoter. Eight independent transgenic lines were generated and two independent lines (#1 and #2) with high expression of PdSHMT2 were selected for further analysis (Fig. S8 in the ESI†). Compared to the control plants, the two transgenic lines showed increases in above-ground biomass; the diameter increased by ∼25%, height increased by ∼46%, leaf area increased by 64% and diameter2 × height (D2H) increased by ∼133% (Fig. 2A–E). Increasing the yield of bioenergy crops is one of the major goals of bioenergy engineering.24 This results demonstrated that overexpression of PdSHMT2 can effectively increase the feedstock yield in Populus.
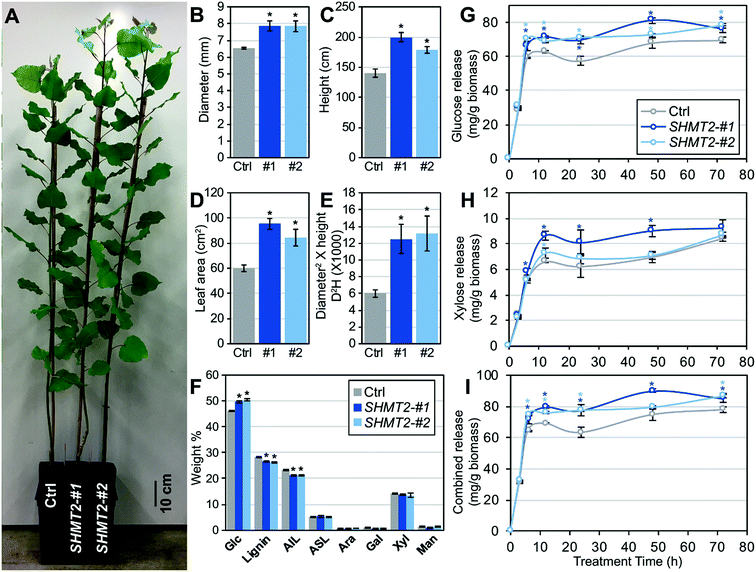 |
| Fig. 2 Plant growth characteristics and sugar release of PtSHMT2 overexpression plants. (A) PtSHMT2 overexpression transgenic plants grown under greenhouse conditions. The diameter of basal stem (B), height (C) and leaf area (D) were compared between transgenic lines and control plants. The above-ground biomass (E) of transgenic poplar samples was estimated using the formula diameter2 × height (D2H, mm3). (F) Chemical composition analysis by means of ion chromatography after two-step acid treatment. Glc, glucose; AIL, acid insoluble lignin; ASL, acid soluble lignin; Ara, arabinose; Gal, galactose; Xyl, xylose; Man, mannose; ash content (%) is not included. (G–I) Saccharification efficiency of PtSHMT2 overexpression poplars. Glucose (G), xylose (H) and combined sugar (I) release with enzymatic hydrolysis times (0, 3, 6, 12, 24, 48 and 72 h). Shown are mean values of three biological replicates ± S.E. | |
Cell wall chemical composition and sugar release
The cell wall composition is directly associated with the efficiency of biomass conversion to biofuels. To explore whether cell wall composition is affected by PdSHMT2, we investigated the carbohydrate composition in stem tissues by means of ion chromatography after two-step sulfuric acid hydrolysis procedures. Compared to control plants, the two transgenic lines (#1 and #2) had significantly higher glucose content (7.37% and 8.72% increase, respectively) and lower lignin content (6.05% and 7.57% decrease, respectively), whereas there were no significant changes in the contents of arabinose, galactose, xylose or mannose (Fig. 2F). The reduced lignin content in transgenic lines was mainly due to the reduction of acid insoluble lignin (AIL) (Fig. 2F).
To assess the sugar release performance of the PdSHMT2 overexpression lines, glucose and xylose releases during the enzymatic hydrolysis were monitored. At 6 h hydrolysis, the two transgenic lines already showed higher glucose release than the control plants. At the final time point of 72 h hydrolysis, the glucose release increased by 9.38% and 12.4% in #1 and #2, compared to the control, respectively (Fig. 2G). More xylose release was detected in #1 at 6 h and increased by 31.4% at 12 h (Fig. 2H). The total sugar release in the two transgenic lines was constantly higher than in the control plants from 6 h and increased by 9.4% and 11.4% at 72 h (Fig. 2I) for lines #1 and #2, respectively.
The structural information on lignin, such as lignin S/G ratio, level of lignin subunit content and lignin interunit linkages, was analyzed through nuclear magnetic resonance (NMR) to examine the biomass characteristics. As shown in Fig. 3, lignin syringyl (S) and guaiacyl (G) subunits, p-hydroxybenzoate (PB), and dominant lignin inter-unit linkages β-aryl ether (β-O-4′), phenylcoumaran (β-5′), and resinols (β–β′) were identified in the aromatic and aliphatic regions of PtSHMT2 overexpression and control lines. The lignin S/G ratio is viewed as one of the factors affecting recalcitrance.25,26 The HSQC analysis revealed that the two PdSHMT2 overexpression lines had a similar lignin S/G ratio to the control plants (Fig. 3), suggesting that the higher sugar release observed in the transgenic lines without pretreatment in this study was not due to lignin S/G ratios (Fig. 2). In the two PdSHMT2 overexpression lines, the β-5′ content was relative higher but the β–β′ content was relative lower than those of the control plants (Fig. 3). These small variations revealed from lignin 2D NMR spectra suggested that the lignin structures in the transgenic lines are similar to those in the control plant and that overexpression of PdSHMT2 in Populus had little effect on the lignin compositional units and side-chain linkages. Sugar release has been related to both the lignin content and lignin S/G ratio as well as cell wall structure-related factors.27 Collectively, the reduced lignin content (and increased glucose content) rather than lignin structural variations likely contributed to the enhanced sugar release observed in the PdSHMT2 transgenics plants.
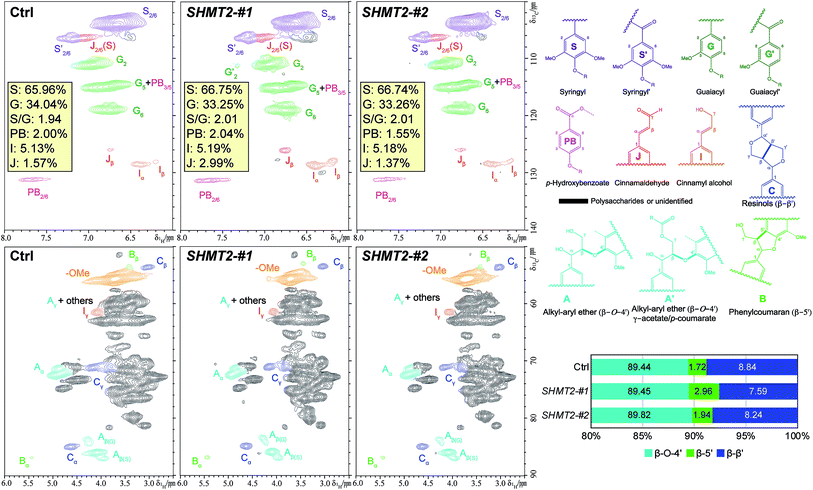 |
| Fig. 3 Structural characterization of lignin by 2D NMR. 2D HSQC spectra are shown for the aromatic regions (top) and the aliphatic regions (bottom) of the whole cell walls from stems of two PtSHMT2 overexpression lines and control poplars. The colors of the substructures shown match those of the corresponding cross peaks in the 2D HSQC spectra. | |
Overexpression of PdSHMT2 leads to changes in the transcriptome
To further reveal the molecular mechanism of SHMT2 involvement in poplar cell wall modification, we performed RNA-Seq using mature leaves of two PdSHMT2 overexpression lines and control plants. Compared to control plants, a total of 483 (230 up and 253 down) and 1159 (467 up and 692 down) differentially expressed genes (DEGs) were identified in line #1 and line #2, respectively (Table S2 in the ESI†). To explore the potential function of DEGs affected by SHMT2, MapMan analysis was conducted to classify these DEGs combined from the two transgenic lines (Fig. S9 in the ESI†). According to the MapMan classification system, a total of 23 up- and 20 down-DEGs belong to cell wall-related processes, 3 up- and 20 down-DEGs belong to secondary metabolism, 7 up- and 13 down-DEGs are involved in development, and 13 up- and 18 down-DEGs play roles in signaling (Fig. 4A). In the metabolic pathway, cell wall component biosynthetic genes (cellulose, hemicellulose and lignin biosynthesis) were down-regulated, whereas degradation-related genes (cellulose and beta-1,4-glucanases, mannan-xylose-arabinose-fucoses, pectate lyases and polygalacturonases) were up-regulated. In addition, cell wall precursor synthesis-related genes, lignin biosynthesis-related genes, simple phenol-related genes, and raffinose-related genes were down-regulated (Fig. 4B). We further classified the genes on the basis of their description and their Arabidopsis orthologs' functional description. In total, 132 up- and 163 down-DEGs related to the cell wall, secondary metabolism, cell cycle or cell growth, development, hormone, proteolysis, TFs, etc. were selected as “core-DEGs” for further analysis (Fig. 4C and Table S3 in the ESI†). In the two transgenic lines, eight genes encoding glycosyl hydrolases (GHs) were significantly up-regulated, whereas nine lignin biosynthesis related genes (one C3H, two C4H, two F5H, one MAX1, one CCoAMT and two CCoAOMT) and eight cellulose biosynthesis related genes (CesA4, CesA7, CesA8, CSLG2 and four RIC4 homologs) were down-regulated. Moreover, six cell cycle related genes (CYCA2;1, two CYCD3;1, CYCD3;2, CYCD6;1 and CNGC15) and eight cell growth related genes (DWARF1, GRF7, PRX33 and five EXPA members) were up-regulated in the PdSHMT2 overexpression lines (Fig. 4C). GHs comprise a large family of enzymes with a broad range of structures and substrate specificities, are ubiquitous in plants and play essential roles in various biological processes.28 GHs are known to be involved in the degradation of biomass such as cellulose, hemicellulose, and starch.29 For instance, KOR1, a GH9 family endo-1,4-β-glucanase, can degrade β-glucan with an unbranched β-(1→4)-linked backbone which is the basic structure of cellulose.30 The reduced cell wall recalcitrance in PdSHMT2 overexpression lines might be partially explained by the induced GH expressions. The decreased lignin content and accelerated growth rates in PdSHMT2 overexpression lines are consistent with the down-regulated lignin biosynthesis genes and up-regulated cell cycle related genes, respectively.
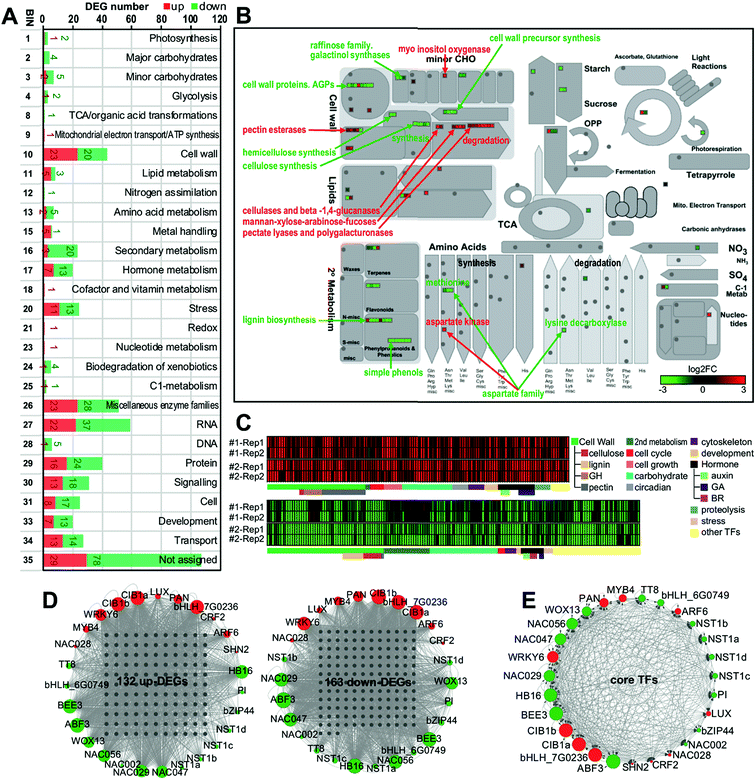 |
| Fig. 4 Transcriptomic changes in the PdSHMT2 overexpression transgenic plants. (A) Functional classification of DEGs in two PdSHMT2 overexpression lines using MapMan Bin. (B) Involvement of DEGs in the metabolism processes. (C) Expression patterns of DEGs involved in cell wall, secondary metabolism and other growth-related processes in the two PdSHMT2 transgenic lines. A total of 132 up-DEGs and 163 down-DEGs were identified. Red and green colors indicate log2 transformed fold changes (transgenic/control) in each replicate. (D) Regulatory network of the 27 hub TFs with 132 up-regulated (left) and 163 down-regulated core-DEGs (right). (E) The regulatory relationships among the 27 core TFs. A larger node indicates a larger number of genes regulated by a TF. Red and green nodes indicate up- or down-regulated TFs, respectively. | |
To seek potential hub TFs which play key roles in controlling gene expression in the PdSHMT2 overexpression lines, we analyzed the TF binding sites (TFBSs) in the promoter regions of core-DEGs. Based on the TFBS enrichment in the promoter of core-DEGs, a total of 27 TFs were identified as the hub TFs including four NST1 homologs (NST1a, NST1b, NST1c, and NST1d), two CIB1 homologs (CIB1a and CIB1b), one MYB4, and one TT8 (Fig. 4D and E).
To validate the RNA-Seq results, we selected eight hub TFs (MYB4, CIB1b, WRKY6, NST1a, NST1b, NST1c, NST1d and TT8) and seven TFs (MYB46, MYB83, MYB63, MYB7, NAC056, GT2 and HBI1) from the DEG list for expression analysis using qRT-PCR. Most of these 15 selected TFs are known to be involved in the transcriptional regulation of secondary cell wall biosynthesis. NST1 is a primary regulator of the SCW formation,31 which directly regulates the SCW biosynthetic master switches MYB46 and MYB83.23MYB63 is a direct target of MYB46/83 and directly regulates lignin biosynthesis.32 In contrast, MYB4 is a repressor and it can bind to the C4H promoter and its own promoter.33 As shown in Fig. 5, the expression of activator genes such as NST1s, MYB46, MYB83, and MYB63 was repressed, whereas the expression of the repressor gene MYB4 was enhanced in PdSHMT2 overexpression lines. CIB1 is a cryptochrome-interacting basic-helix-loop-helix type TF and interacts with CRY2 in a blue light-specific manner in Arabidopsis.34 The up-regulated CIB1 in PdSHMT2 overexpression lines is consistent with the potential involvement of PdSHMT2 in the circadian related processes. Taken together, these results suggested that overexpression of PdSHMT2 leads to changes in the expression of these TFs which in turn regulate the expression of cell wall structural genes, resulting in altered cell wall chemistry.
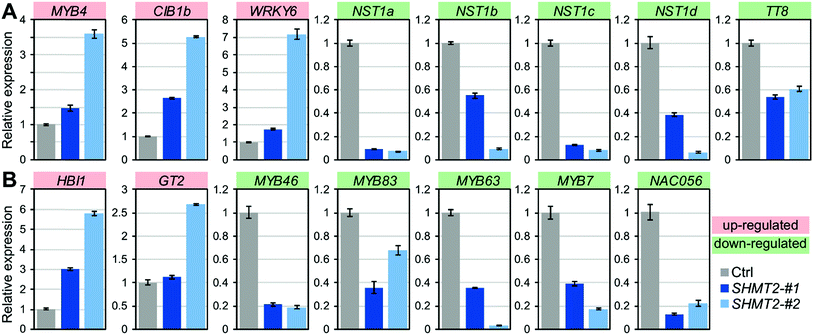 |
| Fig. 5 qRT-PCR validation of expression of hub TFs (A) and other known cell wall-related TFs (B). Shown are mean values of three biological replicates ± S.E. | |
Overexpression of PdSHMT2 leads to changes in metabolite profiling
To investigate the impact of PdSHMT2 overexpression at the metabolic level, we performed metabolomic analysis using the PdSHMT2 transgenic lines. Compared to the control plants, a total of 33 up-regulated and 17 down-regulated metabolites were identified in the two transgenic lines (Fig. 6A). Based on the systematic pathway analysis of the metabolome, the most relevant pathway of these 50 differential accumulated metabolites is “Galactose metabolism” (Fig. 6B). In the galactose metabolic pathway, the content of six metabolites was significantly changed in the two PdSHMT2 overexpression lines. Sucrose, glucose, galactose and fructose contents were increased, whereas galactinol and raffinose contents were decreased in the PdSHMT2 overexpression lines (Fig. 6C). These results suggest that PdSHMT2 mainly affects the galactose metabolic pathway at the metabolic level.
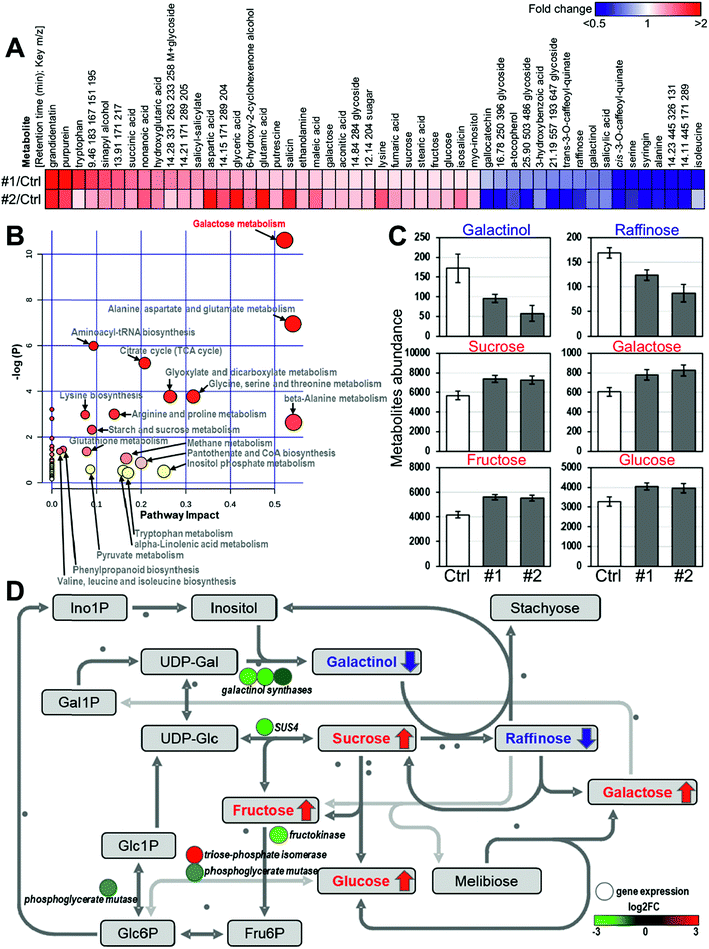 |
| Fig. 6 Effects of PtSHMT2 overexpression on the poplar metabolome. (A) Metabolite profile of two PdSHMT2 overexpression lines (#1 and #2) compared to control plants. (B) Pathway analysis of differentially accumulated metabolites between PtSHMT2 overexpression lines and control poplars. Global metabolic alterations of the most relevant pathways induced by PtSHMT2 overexpression were revealed using MetaboAnalyst; a small p value and large pathway impact factor indicate that the pathway is greatly influenced. (C) Metabolite abundance. In the most affected galactose metabolism pathway, two metabolites (galactinol and raffinose) were inhibited while four metabolites (sucrose, galactose, fructose, and glucose) were induced. (D) Integrated analysis of the transcriptomic and metabolomic data in the galactose metabolism pathway. Red and green circles indicate DEGs identified from the transcriptomics analysis. Red or blue arrows indicate the differential metabolites identified from the metabolomics analysis. The map of galactose metabolism was downloaded from MapMan. | |
We then combined the transcriptomic and metabolomic results to explain the metabolite changes in the galactose metabolic pathway. As shown in Fig. 6D, the decrease in galactinol content was caused by the down-regulation of three galactinol synthase genes, which directly catalyze the biosynthesis of galactinols. Reduced galactinol content restricted conversion from sucrose to raffinose, so sucrose was accumulated and raffinose was inhibited. SUS4 controls reversible catalysis between UDP-Glc and sucrose, and the Arabidopsis sus4 mutant has high leaf sucrose content in the dark and high root sucrose content under both light and dark conditions.35 With the increase of sucrose content, its downstream fructose and glucose were also accumulated. It has been reported that sugars such as sucrose and glucose can function as signaling molecules to trigger gene expression in plants. In Arabidopsis, 82 TFs from 22 families are responsive to the glucose signal with greater than three-fold changes, including bHLH, MYB, AP2, etc.36 The expression of MYB4 can be activated by 3% glucose after dark adaptation.37 The up-regulation of MYB4 in PdSHMT2 overexpression lines is consistent with the increased glucose content. Taken together, the results from transcriptomic and metabolomic analyses support the role of PdSHMT2 in cell wall-related processes.
Conclusions
We describe an alternative strategy for increasing biomass production and reducing recalcitrance in the bioenergy crop Populus by manipulating the expression of a serine hydroxymethyltransferase. On the basis of our findings, we propose a regulatory model in which PdSHMT2 is associated with the repression of activators including the first-layer master switches (NST1/2/3) and the second-layer master switches (MYB46/83) in the regulatory network of SCW biosynthesis. On the other hand, PdSHMT2 affects metabolism to enhance the accumulation of sucrose and glucose, which triggers the expression of repressor MYB4 to inhibit the expression of lignin biosynthetic genes (Fig. 7). A field trial on agronomic performance demonstrated that cell wall modifications via PdSHMT2 overexpression did not compromise first-year performance under field conditions.38 Collectively, genetically engineered poplar plants with increased glucose content, reduced lignin content and increased sugar release resulting from PdSHMT2 overexpression have the potential to serve as a promising biomass feedstock for efficient conversion into biofuels.
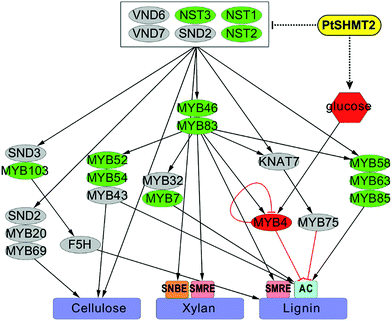 |
| Fig. 7 Proposed model of the involvement of PtSHMT2 in cell wall biosynthesis. The secondary cell wall biosynthetic pathway was modified from Poovaiah et al.57 Red nodes indicate up-regulated TFs or metabolites. Green nodes indicate down-regulated TFs. The differentially expressed NAC or MYB genes are shown in Fig. S10 in the ESI.† | |
Experimental
Bioinformatics analysis
To identify Populus SHMT proteins, the full-length amino acid sequences of Arabidopsis SHMT proteins were subjected to a BLASTp search in the Populus trichocarpa genome from Phytozome (https://phytozome.jgi.doe.gov). In addition, the SHMT proteins were searched in 11 other plant species including three woody species Salix purpurea, Eucalyptus grandis and Vitis vinifera, three dicots Arabidopsis thaliana, Medicago truncatula and Glycine max; four monocots Oryza sativa, Zea mays, Brachypodium distachyon and Sorghum bicolor, and a moss Physcomitrella patens for phylogenetic analysis. The SHMT candidates were searched in the Pfam database to validate the presence of the SHMT motif (PF00464). Multiple sequence alignment of the full-length amino acid sequences was performed using Clustal X2.39 The phylogenetic trees were constructed using the neighbor-joining method in the MEGA package V5.1
40 with bootstrap values from 1000 replicates indicated at each node. Three-dimensional structure prediction was performed by means of the I-TASSER (iterative threading assembly refinement, v4.4) toolkit.41
Normalized expression values of the PtSHMT genes from various tissues and organs were obtained from the Populus Gene Atlas database (https://phytozome.jgi.doe.gov/phytomine/aspect.do?name=Expression). The nm-scale expression of PtSHMTs was obtained from the AspWood gene expression database.21 The co-expression network of PtSHMT2 was created using data obtained from the co-expressed biological process database for poplar.42 Cytoscape43 was used to visualize the resulting network.
Generation of transgenic poplar
The full-length open-reading frame of PtSHMT2 was amplified from Populus deltoides genotype WV94 and was cloned into the pAGW560 binary vector, in which expression was driven by the Arabidopsis UBIQUITIN3 promoter. Agrobacterium-mediated transformation into WV94 was conducted at ArborGen Inc. (Ridgeville, SC) as described previously.44 These plants were transferred and grown in greenhouses at Oak Ridge National Laboratory (Oak Ridge, TN) at a constant temperature of 25 °C and 16/8 h light/dark photoperiod. To estimate stem cylinder volume, plant height and stem base diameter of six-month-old plants were measured. We measured the primary stem length from the stem base (6 cm above the soil surface) to the shoot apex for plant height and measured the diameter of the stem base using calipers.
Chemical composition analysis
The size of poplar samples was reduced to 40 mesh using a Wiley mill (Thomas Scientific, Swedesboro, NJ) and Soxhlet-extracted with ethanol/toluene (1
:
2, v/v) for 24 h. The extractives-free sample was analyzed by a method consisting of two-step sulfuric acid (H2SO4) hydrolysis according to published literature.45 In the first step, the extractives-free sample was hydrolyzed with 72% (w/w) H2SO4 at 30 °C for 1 h. In the second step, the hydrolyzed sample was diluted to a final concentration of 4% H2SO4 (w/w), followed by autoclaving at 121 °C for 1 h. The hydrolysate was filtered from the solid residue. The filtered liquid fraction was examined using a Dionex ICS-3000 ion chromatography system (Thermo Fisher Scientific, Sunnyvale, CA) for quantifying the sugar content. The total lignin content was quantified via acid-soluble and acid-insoluble lignin separation from the hydrolysate and solid residue, respectively. Acid-soluble lignin was measured with the liquid fraction at 240 nm wavelength using UV/Vis spectroscopy. Acid insoluble lignin was quantified with the filtered solid residue as described in the NREL procedure. All analyses were technically duplicated for reported values and statistical analysis.
Sugar release test through enzymatic hydrolysis
The released glucose and xylose were measured according to published literature.46 Dried and Wiley-milled (40 mesh) stems of the Populus control and transgenic plants were used for sugar release measurement. The dried sample (250 mg) was loaded into 50 mM citrate buffer solution (pH 4.8) with Novozymes CTec2 (gift product with 70 mg protein per gram of biomass from Novozymes, Franklinton, NC). The enzymatic hydrolysis was carried out at 50 °C and 200 rpm in an incubator shaker. Liquid hydrolysate was periodically collected at 0, 3, 6, 12, 24, 48, and 72 h, and enzymes in the hydrolysate were deactivated in boiling water for 10 min before carbohydrate analysis. The released sugars in each hydrolysate were measured using a Dionex ICS-3000 ion chromatography system. Each analysis was conducted in duplicate.
Heteronuclear single quantum coherence (HSQC) NMR analysis
NMR spectra were recorded at 30 °C on a Bruker Avance III HD 500 spectrometer and spectral processing was carried out using Bruker Topspin 3.5 (Mac) software. The whole cell NMR samples were prepared according to the literature.47 Poplar stem powders (40 meshes) were extracted with an ethanol/toluene mixture (1
:
2, v/v) for 24 h. The extractives-free samples (air-dried) were loaded into a 50 mL ZrO2 grinding jar (including 10 × 10 ball bearings) in a Retsch Ball Mill PM 100. The biomass was then ball milled at 600 rpm with a frequency of 5 min with 5 min pauses in-between for a total of 2 h. The ball-milled whole cell wall sample (∼60 mg) and an NMR solvent mixture of 4/1 (v/v) DMSO-d6/HMPA-d18 (∼0.4 mL) were loaded in a 5 mm NMR tube. The biomass and NMR solvents were mixed by vortexing for forming uniform gel-state samples, and then placed in a sonicator for 1–2 h before the analysis. The 2D heteronuclear single quantum coherence (HSQC) NMR experiments were carried out with a standard Bruker pulse sequence (hsqcetgpspsi2.2) with the following acquisition parameters: a spectral width of 12 ppm in the F2 (1H) dimension with 1024 data points (acquisition time 85.2 ms) and 166 ppm in the F1 (13C) dimension with 256 increments (acquisition time 6.1 ms), a 1.0 s delay, a 1JC–H of 145 Hz, and 128 scans. The relative abundance of lignin compositional subunits and interunit linkage were estimated using volume integration of contours in HSQC spectra.47,48 For monolignol measurements of S, G, H, and p-hydroxybenzoate (PB), the S2/6, G2, H2/6, and PB2/6 contours were used for relative quantitation. The contours of Cα signals were integrated for interunit linkage estimation.
RNA sequencing and data analysis
For RNA-Seq, mature leaves from PtSHMT2 overexpression and control poplars were collected for RNA extraction. For each line, two biological replicates were used for library construction and sequencing. A total of 4 μg of total RNA was used to generate a cDNA library for Illumina sequencing. The RNA-Seq library was generated by a standard method as described in the Illumina sequencing sample preparation protocol (San Diego, CA, USA). After filtering out low-quality reads, RNA-Seq reads were aligned to the P. trichocarpa genome (version 3.0, https://phytozome.jgi.doe.gov/pz/portal.html#!info?alias=Org_Ptrichocarpa) using TopHat2.49 Differentially expressed genes (DEGs) were identified using the R package DESeq2.50 Raw P-values were adjusted for multiple comparison effects using the q-value (false discovery rate) method.51 The cutoff for significant DEGs was set as >2 absolute fold change (FC) and q-value < 0.05. MapMan52 and Gene Ontology (GO) were used for functional classification of DEGs. GO enrichment analysis was performed using agriGO.53 For transcription factor binding site (TFBS) prediction, a 2 kb sequence upstream of the translation start site of identified DEGs was analyzed using PlantPAN.54
Metabolomics analysis
For metabolite profiling, 25 mg of leaf tissues lyophilized and ground with a Wiley mill were twice extracted from each transgenic line and controls with 2.5 mL 80% ethanol overnight and then the extracts combined prior to drying a 0.50 mL aliquot in a nitrogen stream. As an internal standard, 75 μL of sorbitol at 1.0 mg mL−1 was added to the first extract. Dried extracts were dissolved in acetonitrile, followed by TMS derivatization and analyzed by GC-MS according to Li et al.55 Metabolite peaks were extracted using a characteristic mass-to-charge (m/z) ratio and quantified by area integration, and the concentrations were normalized to the quantity of the internal standard (sorbitol) recovered and the amount of sample extracted, derivatized and injected. A large user-defined database of mass spectral electron impact ionization fragmentation patterns of TMS-derivatized compounds (∼2300 signatures) was used to identify the metabolites of interest. Unidentified metabolites were represented by their retention time and key m/z ratios. The metabolite data were presented as fold changes of the transgenic line vs. the average of the control lines from three biological replicates. Student's t-tests were used to determine whether differences were statistically significant (P ≤ 0.05).
Metabolite peaks were assigned using MS data, MSE fragments, molecular weights and elemental compositions or interpreted using available biochemical databases, such as HMDB (https://www.hmdb.ca/), KEGG (https://www.kegg.com/) and Chemspider (https://www.chemspider.com). Fold changes from each group/control group or each group/CRF group and ROC curves were recorded using Metaboanalysis 3.0.
qRT-PCR analysis
One μg of total RNA was used to generate cDNA by using the Rite aid reverse transcriptase following the manufacturer's instructions (Thermo Fisher Scientific, Hudson, NH). Gene-specific primers were designed using Primer3 software (http://frodo.wi.mit.edu/primer3/input.htm) with an annealing temperature of 58–60 °C and an amplicon of 150–250 bp. qRT-PCR was performed using a Maxima SYBR Green/ROX qPCR master mix (Thermo Fisher Scientific) according to the manufacturer's instructions. The relative gene expression was calculated by the 2−ΔΔCt method56 using PtUBQ10b as an internal control. All experiments were performed by using three biological replicates and three technical replicates. The primers used in this study are listed in Table S4 in the ESI.†
Statistical analysis
Statistical analysis to determine statistical significance was performed by Student's t tests of paired samples. The asterisk in each figure indicates a significant difference compared to control samples (P < 0.05).
Authors' contributions
JZ performed experiments, analyzed data and wrote the manuscript. ML, CGY, YP and AJR performed chemical compositional analysis, lignin NMR analysis, and sugar release determination. WR, KAW and CMC generated Populus transgenic lines. VS, EAL, KB and JS generated and analyzed RNA-Seq data. ACB, SSJ and LEG measured biomass production. NLE and TJT generated and analyzed metabolomics data. XY designed the construct for Populus transformation. GAT, WM and JGC conceived the study, coordinated research and contributed to experimental design and data interpretation. All authors read and approved the final manuscript.
Conflicts of interest
There is no conflict of interest.
Acknowledgements
A special thanks to Clark M. Mindy and Zach L. Zeigler for growing and maintaining plants in ORNL greenhouses. This research was supported by the BioEnergy Science Center (BESC) and the Center for Bioenergy Innovation (CBI). BESC and CBI are supported by the Office of Biological and Environmental Research (BER) in the U.S. Department of Energy Office of Science. Oak Ridge National Laboratory is managed by UT-Battelle, LLC for the U.S. Department of Energy under Contract Number DE-AC05-00OR22725. The work conducted by the U.S. Department of Energy Joint Genome Institute is supported by the Office of Science of the U.S. Department of Energy under Contract No. DE-AC02-05CH11231. This manuscript has been authored by UT-Battelle, LLC under Contract No. DE-AC05-00OR22725 with the U.S. Department of Energy. The United States Government retains and the publisher, by accepting the article for publication, acknowledges that the United States Government retains a non-exclusive, paid-up, irrevocable, world-wide license to publish or reproduce the published form of this manuscript, or allow others to do so, for United States Government purposes. The Department of Energy will provide public access to these results of federally sponsored research in accordance with the DOE Public Access Plan (http://energy.gov/downloads/doe-public-access-plan).
References
- N. A. Rao, M. Ambili, V. R. Jala, H. S. Subramanya and H. S. Savithri, Biochim. Biophys. Acta, Proteins Proteomics, 2003, 1647, 24–29 CrossRef.
-
E. A. Cossins, in Biochemistry of Metabolism, Elsevier, 1987, pp. 317–353 Search PubMed.
- D. D. Anderson, J. Y. Eom and P. J. Stover, J. Biol. Chem., 2012, 287, 4790–4799 CrossRef CAS PubMed.
- X. W. Liu, B. Reig, I. M. Nasrallah and P. J. Stover, Biochemistry, 2000, 39, 11523–11531 CrossRef CAS PubMed.
- R. Douce and M. Neuburger, Curr. Opin. Plant Biol., 1999, 2, 214–222 CrossRef CAS PubMed.
-
D. Oliver and C. McIntosh, Molecular Biology of Plant Mitochondria, 1995 Search PubMed.
- R. C. Leegood, P. J. Lea, M. D. Adcock and R. E. Häusler, J. Exp. Bot., 1995, 1397–1414 CrossRef CAS.
- C. R. McClung, M. Hsu, J. E. Painter, J. M. Gagne, S. D. Karlsberg and P. A. Salomé, Plant Physiol., 2000, 123, 381–392 CrossRef CAS PubMed.
- J. K. Schjoerring, G. Mack, K. H. Nielsen, S. Husted, A. Suzuki, S. Driscoll, R. Boldt and H. Bauwe, Plant J., 2006, 45, 71–82 CrossRef CAS PubMed.
- J. I. Moreno, R. Martin and C. Castresana, Plant J., 2005, 41, 451–463 CrossRef CAS PubMed.
- H. P. Zhou, J. F. Zhao, Y. Q. Yang, C. X. Chen, Y. F. Liu, X. H. Jin, L. M. Chen, X. Y. Li, X. W. Deng, K. S. Schumaker and Y. Guo, Plant Cell, 2012, 24, 5106–5122 CrossRef CAS PubMed.
- L. Li, S. Hill-Skinner, S. Liu, D. Beuchle, H. M. Tang, C. T. Yeh, D. Nettleton and P. S. Schnable, Plant J., 2015, 81, 493–504 CrossRef CAS PubMed.
- H. M. Tang, S. Liu, S. Hill-Skinner, W. Wu, D. Reed, C. T. Yeh, D. Nettleton and P. S. Schnable, Plant J., 2014, 77, 380–392 CrossRef CAS PubMed.
- Z. Bai, T. Qi, Y. Liu, Z. Wu, L. Ma, W. Liu, Y. Cao, Y. Bao and C. Fu, Plant Biotechnol. J., 2018 DOI:10.1111/pbi.12935.
- R. Q. Zhong, C. H. Lee and Z. H. Ye, Trends Plant Sci., 2010, 15, 625–632 CrossRef CAS PubMed.
- J. Zhang, K. Nieminen, J. A. A. Serra and Y. Helariutta, Curr. Opin. Plant Biol., 2014, 17, 56–63 CrossRef CAS PubMed.
- Y. Nakano, M. Yamaguchiz, H. Endo, N. A. Rejab and M. Ohtani, Front. Plant Sci., 2015, 6 Search PubMed.
- H. L. Jin, E. Cominelli, P. Bailey, A. Parr, F. Mehrtens, J. Jones, C. Tonelli, B. Weisshaar and C. Martin, EMBO J., 2000, 19, 6150–6161 CrossRef CAS PubMed.
- D. Schenke, C. Boettcher and D. Scheel, Plant, Cell Environ., 2011, 34, 1849–1864 CrossRef CAS PubMed.
- H. Shen, X. Z. He, C. R. Poovaiah, W. A. Wuddineh, J. Y. Ma, D. G. J. Mann, H. Z. Wang, L. Jackson, Y. H. Tang, C. N. Stewart, F. Chen and R. A. Dixon, New Phytol., 2012, 193, 121–136 CrossRef CAS PubMed.
- D. Sundell, N. R. Street, M. Kumar, E. J. Mellerowicz, M. Kucukoglu, C. Johnsson, V. Kumar, C. Mannapperuma, N. Delhomme and O. Nilsson, Plant Cell, 2017, 29, 1585–1604 CrossRef CAS PubMed.
- L. M. Voll, A. Jamai, P. Renné, H. Voll, C. R. McClung and A. P. Weber, Plant Physiol., 2006, 140, 59–66 CrossRef CAS PubMed.
- Q. Zhao and R. A. Dixon, Trends Plant Sci., 2011, 16, 227–233 CrossRef CAS PubMed.
- Y. Wang, C. Fan, H. Hu, Y. Li, D. Sun, Y. Wang and L. Peng, Biotechnol. Adv., 2016, 34, 997–1017 CrossRef CAS PubMed.
- M. Li, Y. Pu and A. J. Ragauskas, Front. Chem., 2016, 4, 45 Search PubMed.
- M. H. Studer, J. D. DeMartini, M. F. Davis, R. W. Sykes, B. Davison, M. Keller, G. A. Tuskan and C. E. Wyman, Proc. Natl. Acad. Sci., 2011, 108, 6300–6305 CrossRef CAS PubMed.
- M. E. Himmel, S. Y. Ding, D. K. Johnson, W. S. Adney, M. R. Nimlos, J. W. Brady and T. D. Foust, Science, 2007, 315, 804–807 CrossRef CAS PubMed.
- B. Wu, B. Zhang, Y. Dai, L. Zhang, K. Shang-Guan, Y. Peng, Y. Zhou and Z. Zhu, Plant Physiol., 2012, 159, 1440–1452 CrossRef CAS PubMed.
- L. L. Li, S. R. McCorkle, S. Monchy, S. Taghavi and D. van der Lelie, Biotechnol. Biofuels, 2009, 2, 10 CrossRef PubMed.
- M. Mølhøj, P. Ulvskov and F. Dal Degan, Plant Physiol., 2001, 127, 674–684 CrossRef.
- N. Mitsuda, A. Iwase, H. Yamamoto, M. Yoshida, M. Seki, K. Shinozaki and M. Ohme-Takagi, Plant Cell, 2007, 19, 270–280 CrossRef CAS PubMed.
- J. H. Ko, W. C. Kim, J. Y. Kim, S. J. Ahn and K. H. Han, Mol. Plant, 2012, 5, 961–963 CrossRef CAS PubMed.
- B. Berger, R. Stracke, R. Yatusevich, B. Weisshaar, U. I. Flügge and T. Gigolashvili, Plant J., 2007, 50, 911–916 CrossRef CAS PubMed.
- X. Li, Q. Wang, X. H. Yu, H. T. Liu, H. Yang, C. X. Zhao, X. M. Liu, C. Tan, J. Klejnot, D. P. Zhong and C. T. Lin, Proc. Natl. Acad. Sci. U. S. A., 2011, 108, 20844–20849 CrossRef CAS PubMed.
- Z. Bieniawska, D. H. P. Barratt, A. P. Garlick, V. Thole, N. J. Kruger, C. Martin, R. Zrenner and A. M. Smith, Plant J., 2007, 49, 810–828 CrossRef CAS PubMed.
- J. Price, A. Laxmi, S. K. St Martin and J. C. Jang, Plant Cell, 2004, 16, 2128–2150 CrossRef CAS PubMed.
- A. K. Banas and H. Gabrys, Plant Signaling Behav., 2007, 2, 221–230 CrossRef.
- D. Macaya-Sanz, J. G. Chen, U. C. Kalluri, W. Muchero, T. J. Tschaplinski, L. E. Gunter, S. J. Simon, A. K. Biswal, A. C. Bryan, R. Payyavula, M. Xie, Y. Yang, J. Zhang, D. Mohnen, G. A. Tuskan and S. P. DiFazio, Biotechnol. Biofuels, 2017, 10, 253 CrossRef PubMed.
- J. D. Thompson, T. Gibson and D. G. Higgins, Curr. Protoc. Bioinf., 2002, 2.3. 1–2.3. 22 Search PubMed.
- K. Tamura, D. Peterson, N. Peterson, G. Stecher, M. Nei and S. Kumar, Mol. Biol. Evol., 2011, 28, 2731–2739 CrossRef CAS PubMed.
- J. Yang, R. Yan, A. Roy, D. Xu, J. Poisson and Y. Zhang, Nat. Methods, 2015, 12, 7–8 CrossRef CAS PubMed.
- Y. Ogata, H. Suzuki and D. Shibata, J. Wood Sci., 2009, 55, 395 CrossRef CAS.
- M. E. Smoot, K. Ono, J. Ruscheinski, P.-L. Wang and T. Ideker, Bioinformatics, 2010, 27, 431–432 CrossRef PubMed.
- A. A. Karve, S. S. Jawdy, L. E. Gunter, S. M. Allen, X. Yang, G. A. Tuskan, S. D. Wullschleger and D. J. Weston, New Phytol., 2012, 196, 726–737 CrossRef CAS PubMed.
- A. Sluiter, B. Hames, R. Ruiz, C. Scarlata, J. Sluiter, D. Templeton and D. Crocker, Laboratory Analytical Procedures, 2008, 1617, 1–16 Search PubMed.
- Y. Yang, C. G. Yoo, H.-B. Guo, W. Rottmann, K. A. Winkeler, C. M. Collins, L. E. Gunter, S. S. Jawdy, X. Yang and H. Guo, Biotechnol. Biofuels, 2017, 10, 74 CrossRef PubMed.
- C. G. Yoo, Y. Pu, M. Li and A. J. Ragauskas, ChemSusChem, 2016, 9, 1090–1095 CrossRef CAS PubMed.
- C. José, A. G. Lino, J. L. Colodette, C. F. Lima, A. Gutiérrez, Á. T. Martínez, F. Lu, J. Ralph and J. Rencoret, Biomass Bioenergy, 2015, 81, 322–338 CrossRef.
- D. Kim, G. Pertea, C. Trapnell, H. Pimentel, R. Kelley and S. L. Salzberg, Genome Biol., 2013, 14, R36 CrossRef PubMed.
- M. I. Love, W. Huber and S. Anders, Genome Biol., 2014, 15, 550 CrossRef PubMed.
- J. D. Storey and R. Tibshirani, Proc. Natl. Acad. Sci., 2003, 100, 9440–9445 CrossRef CAS PubMed.
- O. Thimm, O. Bläsing, Y. Gibon, A. Nagel, S. Meyer, P. Krüger, J. Selbig, L. A. Müller, S. Y. Rhee and M. Stitt, Plant J., 2004, 37, 914–939 CrossRef CAS PubMed.
- T. Tian, Y. Liu, H. Yan, Q. You, X. Yi, Z. Du, W. Xu and Z. Su, Nucleic Acids Res., 2017, 45, W122–W129 CrossRef CAS PubMed.
- C.-N. Chow, H.-Q. Zheng, N.-Y. Wu, C.-H. Chien, H.-D. Huang, T.-Y. Lee, Y.-F. Chiang-Hsieh, P.-F. Hou, T.-Y. Yang and W.-C. Chang, Nucleic Acids Res., 2016, 44, D1154–D1160 CrossRef CAS PubMed.
- Y. Li, T. J. Tschaplinski, N. L. Engle, C. Y. Hamilton, M. Rodriguez, J. C. Liao, C. W. Schadt, A. M. Guss, Y. Yang and D. E. Graham, Biotechnol. Biofuels, 2012, 5, 2 CrossRef CAS PubMed.
- K. J. Livak and T. D. Schmittgen, methods, 2001, 25, 402–408 CrossRef CAS PubMed.
- C. R. Poovaiah, M. Nageswara-Rao, J. R. Soneji, H. L. Baxter and C. N. Stewart, Plant Biotechnol. J., 2014, 12, 1163–1173 CrossRef CAS PubMed.
Footnote |
† Electronic supplementary information (ESI) available. See DOI: 10.1039/c8se00471d |
|
This journal is © The Royal Society of Chemistry 2019 |
Click here to see how this site uses Cookies. View our privacy policy here.