DOI:
10.1039/C9RA07071K
(Paper)
RSC Adv., 2019,
9, 41419-41430
Lipidomics as a tool of predicting progression from non-alcoholic fatty pancreas disease to type 2 diabetes mellitus†
Received
4th September 2019
, Accepted 9th December 2019
First published on 16th December 2019
Abstract
The lipid metabolism relationship between non-alcoholic fatty pancreas disease (NAFPD) and type 2 diabetes mellitus (T2DM) is poorly defined. We aim to identify novel T2DM-related lipid biomarkers in addition to previous studies and provide the evidence for elucidating the relationship between NAFPD and T2DM in a lipid perspective. In this study, multi-dimensional mass spectrometry-based shotgun lipidomics (MDMS-SL) was used to investigate the potential discriminating lipid profile of the fasting plasma of 105 Chinese individuals (39 NAFPD patients, 38 T2DM patients and 30 healthy controls). Then multivariate statistical analysis combined with pathway analysis was performed to identify the lipid biomarker and explore the potential relationship of these two important diseases. The results described a marked reduction of plasmalogen and a significant 4-hydroxynonenal increase in the two diagnostic group, which indicated increased oxidative stress and peroxisomal dysfunction in patients. 60 discriminating metabolites were identified by multivariate statistical analysis of the lipidomics data. In addition, ingenuity pathway analysis (IPA) and a metabolic network constructed by prediction of IPA indicated that lipid metabolism, molecular transport, carbohydrate metabolism and small molecule biochemistry were correlated with disease progression. Our results revealed that the profile of plasma lipid alteration characteristic of NAFPD was similar to that of T2DM, especially during the period prior to the onset of T2DM.
1. Introduction
Type 2 diabetes mellitus (T2DM) is a heterogeneous metabolic disease characterized by dysregulation of glucose and lipid metabolism.1 It is predicted that 642 million people worldwide will live with diabetes by 2040.2 Non-alcoholic fatty pancreas disease (NAFPD) is a sub-type of pancreatic steatosis, which has gained increasing attention with the emergence of epidemic obesity and metabolic syndrome. A large body of research in the last two decades has demonstrated that NAFPD is associated with insulin resistance (IR), β-cell dysfunction and risk of diabetes.3,4 However, the prevalence and development mechanisms of NAFPD and T2DM in patients still remain elusive. IR is a pathophysiological factor and precursor for diabetes in all ethnic populations. However, previous study showed that South Asians have increased propensity for IR even at lower levels of BMI, which might be attributed to greater deposition of visceral fat in South Asians as compared to Caucasians.5 In addition, Asian diabetic patients such as in China are more susceptible to ectopic fat deposition than Westerns in a given BMI.6 Hence, study on the lipid profile of NAFPD and T2DM of Chinese populations may help better understand the inter-ethnic differences.
As both NAFPD and T2DM patients are closely associated with lipid metabolic disorder, we hypothesize that molecular lipid aberration in plasma may help disclose their underlying mechanisms and facilitate development of strategies against the two diseases. Lipidomics has provided a new strategy to globally survey metabolites associated with prediabetes and metabolic syndrome, which may shed new light on the ongoing biochemical processes and help identify biomarkers to predict the occurrence and progression of metabolic diseases.
The aim of the present study was to better understand the underlying pathogenesis and relationship of these two important diseases. Analyses included lipid profile and biomarker in patients and pathways affected by these metabolic candidates. A metabolic network including the discriminating lipids was constructed for further elucidating the pathogenesis of diseases progression.
2. Results
2.1 Baseline characteristics
The study included 30 healthy controls and 77 NAFPD patients including 38 with co-existing T2DM, who ranged in age from 29 to 63 years (Table 1). The patient demographic data, including gender, age, body mass index (BMI), FPG, 2hPG, fasting insulin (FINS), 2 h postprandial glucose (2h-INS), homeostasis model assessment-insulin residence (HOMA-IR), glycosylated hemoglobin (HbA1c), triacylglycerol (TG), total cholesterol (TC), high-density lipoprotein-cholesterol (HDL), low-density lipoprotein-cholesterol (LDL), adiponectin (APN), leptin (LP), glucagon-like peptide 1 (GLP-1), tumor necrosis factor-α (TNF-α) are summarized. There was no significant difference in these characteristics between the patients and the healthy controls. There was no significant difference in these characteristics between the patients and the healthy controls.
Table 1 Baseline characteristicsa
Characteristics |
Group |
NC (n = 30) |
NDM (n = 39) |
T2DM (n = 38) |
Except for sex, data are given as medians and 25–75% range. |
Male gender (%) |
56.7 (17/30) |
48.7 (19/39) |
68.4 (26/38) |
Age (years) |
40.9 (29–62) |
49.1 (35–69) |
48.3 (34–63) |
BMI |
23.8 (19.2–26.6) |
24.7 (20.6–28.5) |
25.8 (22.0–30.1) |
FPG (mM) |
4.8 (4.2–5.8) |
5.8 (4.9–7.2) |
8.6 (4.2–10.3) |
2hPG (mM) |
6.5 (5.1–7.3) |
8.7 (7.5–10.4) |
13.4 (10.1–16.7) |
FINS (mU L) |
5.9 (4.9–7.8) |
9.2 (5.9–12.8) |
13.7 (10.8–19.4) |
2h-INS (mU L−1) |
31.5 (24.9–37.6) |
42.8 (28.1–59.5) |
53.1 (33.2–70.6) |
HOMA-IR |
1.05 (0.1–1.2) |
1.7 (1.1–3.9) |
2.0 (0.9–4.0) |
HbA1c |
4.8 (4.2–5.6) |
6.8 (4.9–11.1) |
6.4 (4.6–10.1) |
TG (mmol L−1) |
1.2 (0.7–2.1) |
1.2 (0.7–2.65) |
2.1 (0.4–3.4) |
TC (mmol L−1) |
4.1 (2.6–5.6) |
4.5 (4.3–5.2) |
4.8 (3.5–6.7) |
HDL (mmol L−1) |
1.2 (0.8–1.7) |
1.3 (0.8–2.1) |
1.1 (0.7–1.8) |
LDL (mmol L−1) |
2.9 (1.6–3.9) |
2.9 (1.3–3.9) |
2.7 (0.9–3.9) |
APN (μg mL−1) |
12.1 (3.8–23.4) |
11.9 (2.1–34.1) |
10.1 (1.4–33.8) |
LP (ng mL−1) |
20.4 (1.4–53.9) |
24.5 (2.9–63.6) |
19.9 (3.1–47.5) |
GLP-1 (pg mL−1) |
105.3 (26.8–323.7) |
74.4 (15.5–255.7) |
76.3 (13.4–297.4) |
TNF-α (pg mL−1) |
16.3 (8.5–25.9) |
13.7 (4.2–37.8) |
15.1 (6.1–37.5) |
2.2 Lipidomics analysis revealed marked reduction of plasmalogen content in patients
Cohort studies have shown that low plasma phospholipid content is associated with the onset of T2DM.7,8 PC and PE represent the largest amount of glycerophospholipid in mammals. Thus, MDMS-SL was first employed to quantify individual species of PC and PE classes in plasma. Our result is in line with previous study. In addition, we found a significant reduction in their plasmalogen (i.e., plasmenylcholine, pPC; plasmenylethanolamine, pPE) in the two disease groups.
2.2.1 The PC amount undergoes change in NDM and DM patients. There are three subclasses of PC (phosphatidylcholine, dPC; pPC; and plasmanylcholine, aPC). Several species of pPC decreased significantly, while dPC and aPC showed no significant change. The results are shown in Fig. 1A and Table S1.†
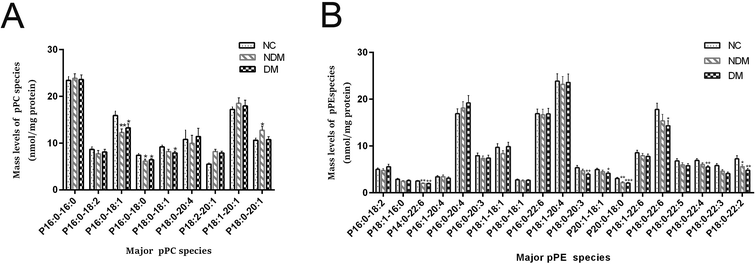 |
| Fig. 1 Comparison of the amount of altered pPC (a) and pPE (b). Only the major species of plasma pPC and pPE content from different experimental groups were displayed. *P < 0.05, **P < 0.01, and ***P < 0.001 compared with the control group. | |
2.2.2 The PE amount undergoes change in NDM and DM patients. The three subclasses of PE (phosphatidylethanolamine, dPE; pPE; and plasmanylethanolamine, aPE) were also quantified. The total amount of pPE in plasma decreased from 184.44 ± 13.45 nmol mL−1 in NC group to 170.05 ± 13.04 nmol mL−1 in NDM group and 170.16 ± 13.44 nmol mL−1 in DM group, with a mean decrease of about 10%. Another subtype of PE, aPE, showed a significant decline in the two groups of NDM (9.24 ± 0.72 nmol mL−1, P < 0.05)and DM (8.26 ± 0.64 nmol mL−1, P < 0.01) groups relative to that in the control group (11.62 ± 0.9 nmol per mg protein), with the decline degree reaching 20% and 29% respectively. The total content of dPE did not change significantly. The results are detailed in Fig. 1B and Table S2.†
2.3 Lipid peroxidation is increased in NDM and DM patients as shown by lipidomics analysis
Most plasmalogens and aPE contents in NDM group and the DM group were significantly decreased as shown in above analysis. Knowing that plasmalogens and aPE have important antioxidant properties, so we inferred an increasing oxidative stress in patients preliminarily. In order to further verify this inference, MDMS-SL was further employed to determine the levels of a sensitive indicator of lipid peroxidase 4-hydroxyalkenal, knowing that it is one of the important products generated in lipid peroxidation and its content can directly reflect the body level of oxidative stress. It was found in our study that the concentration of 4-hydroxynonenal was increased in NDM and DM groups as compared with that in NC group (1.73 ± 0.14 nmol mL−1 and 2.06 ± 0.21 nmol mL−1 vs. 1.52 ± 0.13 nmol mL−1, P < 0.05). Fig. 2 and Table S3.†
 |
| Fig. 2 Comparison of the amount of altered 4-hydroxynonenals. 4-Hydroxyalkenals including the content of 4-hydroxy-2(E)-hexenal(4-HHE), 4-hydroxy-2(E)-nondinenal (4-HNDE) and 4-hydroxy-2(E)-nonenal (4-HNE) from different experimental groups were displayed. *P < 0.05 and **P < 0.01 compared with the control group. | |
2.4 LysoPC and LysoPE species are decreased in NDM and DM patients as shown by lipidomics
Many previous study showed IR was characterized by lower levels of LysoPE and LysoPC,9,10 which is consistent with our finding. The content of most plasma lysoPC species was reduced substantially in NDM and DM groups as compared with that in NC group, which is consistent with the trend of PC (Fig. 3A), although the difference was not statistically significant.
 |
| Fig. 3 Comparison of the amount of altered LysoPC (A) and LysoPE (B). Only the major species of plasma LysoPC and LysoPE content from different experimental groups were displayed. *P < 0.05, **P < 0.01, and ***P < 0.001 compared with the control group. | |
MDMS-SL analysis showed that lysoPE (a metabolite of PE) reduced by 17.5% and 18.1% in NDM and DM groups respectively as compared with that in NC group (19.44 ± 0.94 nmol mL−1 and 18.34 ± 1.14 nmol mL−1 vs. 23.55 ± 1.25 nmol mL−1, P < 0.05) (Fig. 3B).
2.5 Multivariate statistical analysis of the lipidomics data
OPLS analysis in positive and negative ion modes (Fig. 4A and B) were performed with the data obtained by MDMS-SL to compare the lipid profiles of different subjects. According to the OPLS score plot, the metabolic pattern behaved differently in different experimental groups. Discriminating metabolites were identified according to the criteria of a variable importance for projection (VIP) > 1 and a P-value < 0.05. A total of 27 discriminating metabolites were then identified in NDM vs. NC group, including 5 PIs, 9 PEs, 2 SMs, 2 LysoPEs,7 PCs and 2 LysoPCs (Table 2). There were 33 discriminating metabolites in DM vs. NC group, including 2 PIs, 12 PEs, 2 LysoPEs, 3 SMs, 9 PCs, 3 LysoPCs, 1 4-hydroxy hexenal – carnosine (HHE) and 1 Car (Table 3). To further understand the metabolic differences between experimental groups, the identified lipid data were analysed using clustering heat map (Fig. 4C). Even though several lipid clusters overlapped slightly, most lipids were grouped into two differentiated clusters clearly, which is in line with the OPLS analysis.
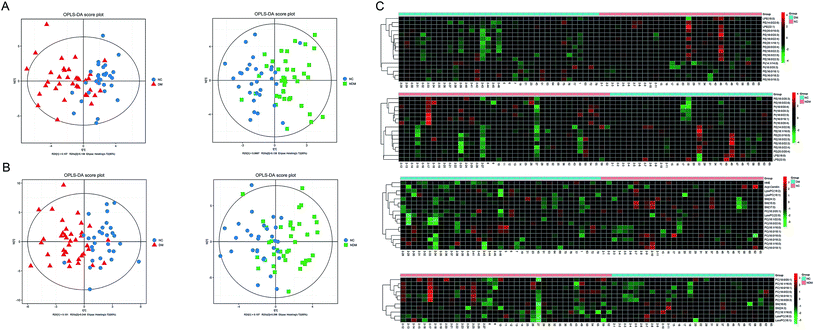 |
| Fig. 4 OPLS score plot of the experimental groups. Box (green), NDM group; circle (blue), NC group; triangle (red), DM group. (A) ESI+, NDM group vs. NC group, R2X(cum) = 0.205, R2Y(cum) = 0.461, Q2(cum) = 0.14; DM group vs. NC group, R2X(cum) = 0.245, R2Y(cum) = 0.448, Q2(cum) = 0.0629. (B) ESI−, NDM group vs. NC group, R2X(cum) = 0.403, R2Y(cum) = 0.399, Q2(cum) = −0.0344; DM group vs. NC group, R2X(cum) = 0.349, R2Y(cum) = 0.513, Q2(cum) = 0.223. Heat map for identified lipids in experimental groups (C). The colour of each section is proportional to the significance of lipid change (red, up-regulated; green, down-regulated). Rows: samples; columns: metabolites. | |
Table 2 List of statistically significant metabolites in NDM vs. NC group
Name |
Lipid ion |
Monoisotopicmass |
VIP |
P-value |
Fold change |
MDMS-SL (EIC−) |
Higher in NDM |
PI(16:0/18:1) |
[PI − H]− |
835.5336542 |
1.375 |
0.007 |
1.445 |
PI(16:0/20:4) |
[PI − H]− |
857.5180041 |
1.240 |
0.024 |
1.431 |
PI(18:0/20:4) |
[PI − H]− |
885.5493042 |
1.614 |
0.004 |
1.293 |
PI(18:0/20:3) |
[PI − H]− |
887.5649543 |
1.630 |
0.000 |
1.532 |
PI(18:0/22:5) |
[PI − H]− |
911.5649543 |
1.466 |
0.024 |
1.327 |
Lower in NDM |
PE(18:1/16:0) |
[PE − Fmoc]− |
922.5281153 |
1.277 |
0.032 |
0.855 |
PE(14:0/22:6) |
[PE − Fmoc]− |
940.53868 |
1.882 |
0.004 |
0.802 |
PE(20:0/18:0) |
[PE − Fmoc]− |
980.4760797 |
2.372 |
0.000 |
0.724 |
PE(18:0/20:3) |
[PE − Fmoc]− |
990.55433 |
1.173 |
0.013 |
1.196 |
PE(18:0/22:5) |
[PE − Fmoc]− |
998.5594154 |
1.075 |
0.028 |
0.878 |
PE(18:0/22:4) |
[PE − Fmoc]− |
1000.575065 |
1.161 |
0.033 |
0.880 |
PE(20:0/20:4) |
[PE − Fmoc]− |
1002.590716 |
1.458 |
0.007 |
0.807 |
PE(18:0/22:2) |
[PE − Fmoc]− |
1004.606366 |
1.680 |
0.009 |
0.791 |
PE(18:0/22:6) |
[PE − Fmoc]− |
1012.53868 |
1.692 |
0.016 |
1.220 |
LPE(18:0) |
[LPE − Fmoc]− |
686.3140998 |
1.029 |
0.000 |
0.614 |
LPE(22:0) |
[LPE − Fmoc]− |
742.3767 |
1.021 |
0.029 |
0.743 |
![[thin space (1/6-em)]](https://www.rsc.org/images/entities/char_2009.gif) |
MDMS-SL (EIC+) |
Lower in NDM |
SM(16:0) |
[SM + Li]+ |
709.5835748 |
1.029 |
0.016 |
0.921 |
SM(24:3) |
[SM + Li]+ |
815.6618251 |
1.139 |
0.008 |
0.822 |
PC(16:1/16:0) |
[PC + Li]+ |
738.562505 |
2.262 |
0.002 |
1.396 |
PC(16:0/18:1) |
[PC + Li]+ |
750.5988905 |
1.810 |
0.002 |
0.792 |
PC(16:0/18:1) |
[PC + Li]+ |
766.5938051 |
2.115 |
0.002 |
1.193 |
PC(18:0/18:1) |
[PC + Li]+ |
794.6251053 |
1.610 |
0.013 |
1.137 |
PC(18:0/20:1) |
[PC + Li]+ |
806.6614908 |
1.418 |
0.014 |
1.197 |
PC(18:0/20:3) |
[PC + Li]+ |
818.6251053 |
2.4587 |
0.000 |
1.330 |
PC(18:0/22:5) |
[PC + Li]+ |
842.6251053 |
1.997 |
0.026 |
1.152 |
LysoPC(18:2) |
[LPC + Na]+ |
542.322 |
2.746 |
0.001 |
0.791 |
LysoPC(18:1) |
[LPC + Na]+ |
544.337 |
1.655 |
0.036 |
0.864 |
Table 3 List of statistically significant metabolites in DM vs. NC group
Name |
Lipid ion |
Monoisotopicmass |
VIP |
P-value |
Fold change |
MDMS-SL (EIC−) |
Higher in DM |
PI(14:1/14:0) |
[PI − H]− |
751.4397538 |
1.183 |
0.031 |
1.297 |
PI(18:0/20:3) |
[PI − H]− |
887.5649543 |
1.129 |
0.002 |
1.404 |
Lower in DM |
PE(16:0/18:2) |
[PE − Fmoc]− |
936.5073798 |
1.572 |
0.001 |
1.337 |
PE(16:0/18:1) |
[PE − Fmoc]− |
938.5230299 |
1.285 |
0.014 |
1.287 |
PE(14:0/22:6) |
[PE − Fmoc]− |
940.53868 |
1.707 |
0.012 |
0.816 |
PE(18:0/18:2) |
[PE − Fmoc]− |
964.53868 |
1.378 |
0.008 |
1.23 |
PE(18:0/20:3) |
[PE − Fmoc]− |
974.5594154 |
1.722 |
0.000 |
0.755 |
PE(20:1/18:1) |
[PE − Fmoc]− |
976.5750655 |
1.817 |
0.008 |
0.836 |
PE(20:0/18:0) |
[PE − Fmoc]− |
980.4760797 |
2.110 |
0.000 |
0.713 |
PE(18:0/22:6) |
[PE − Fmoc]− |
996.5437653 |
1.154 |
0.005 |
0.824 |
PE(18:0/22:5) |
[PE − Fmoc]− |
998.5594154 |
1.106 |
0.018 |
0.863 |
PE(18:0/22:4) |
[PE − Fmoc]− |
1000.575065 |
2.037 |
0.000 |
0.809 |
PE(20:0/20:4) |
[PE − Fmoc]− |
1002.590716 |
2.244 |
0.000 |
0.729 |
PE(18:0/22:2) |
[PE − Fmoc]− |
1004.606366 |
2.375 |
0.000 |
0.671 |
LPE(18:0) |
[LPE − Fmoc]− |
686.3140998 |
1.167 |
0.000 |
0.625 |
LPE(22:1) |
[LPE − Fmoc]− |
740.3610499 |
1.650 |
0.019 |
0.633 |
![[thin space (1/6-em)]](https://www.rsc.org/images/entities/char_2009.gif) |
MDMS-SL (EIC+) |
Lower in DM |
SM(15:0) |
[SM + Li]+ |
695.5679247 |
1.630 |
0.001 |
0.837 |
SM(17:0) |
[SM + Li]+ |
723.5992249 |
1.518 |
0.000 |
0.804 |
SM(24:2) |
[SM + Li]+ |
817.6774752 |
1.162 |
0.046 |
0.876 |
PC(16:1/16:0) |
[PC + Li]+ |
738.562505 |
1.625 |
0.007 |
1.355 |
PC(16:0/18:1) |
[PC + Li]+ |
750.5988905 |
1.547 |
0.011 |
0.816 |
PC(16:0/18:0) |
[PC + Li]+ |
752.6145406 |
1.456 |
0.009 |
0.845 |
PC(16:0/18:2) |
[PC + Li]+ |
764.5781551 |
1.112 |
0.029 |
1.110 |
PC(16:0/18:1) |
[PC + Li]+ |
766.5938051 |
1.848 |
0.004 |
1.166 |
PC(18:0/18:1) |
[PC + Li]+ |
778.6301906 |
2.086 |
0.001 |
0.840 |
PC(18:2/20:1) |
[PC + Li]+ |
802.6301906 |
1.439 |
0.038 |
0.852 |
PC(18:1/22:6) |
[PC + Li]+ |
838.5938051 |
2.304 |
0.000 |
0.759 |
PC(18:0/22:6) |
[PC + Li]+ |
840.6094552 |
1.305 |
0.017 |
0.843 |
LysoPC(18:2) |
[LPC + Na]+ |
542.322 |
1.914 |
0.014 |
0.846 |
LysoPC(18:1) |
[LPC + Na]+ |
544.337 |
1.932 |
0.010 |
0.825 |
LysoPC(22:6) |
[LPC + Na]+ |
590.322 |
1.815 |
0.001 |
0.725 |
HNE |
[HNE + H]+ |
341.1824949 |
1.613 |
0.005 |
0.701 |
Acyl-carnitine |
[Acyl-carnitine + H]+ |
424.342684 |
1.187 |
0.040 |
0.834 |
2.6 Receiver operating characteristic (ROC) curve analysis
Discriminating metabolites appeared in both NDM vs. NC and DM vs. NC is of significance for disclosing the relationship between lipid metabolism with NAFPD and T2DM. Venn analysis was additionally applied to learn the possible marker that participates in NAFPD and T2DM pathological process (Fig. 5). Further selection of potential indicators with those metabolites fold change > 1 was conducted by ROC analysis. As shown in Fig. 1A, PC 16:1-16:0, PI 18:0-20:3 is the metabolite with area under the ROC curve (AUC)
≥ 0.6 (Fig. S1†).
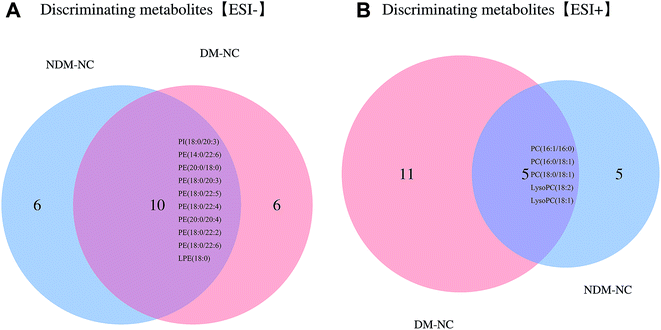 |
| Fig. 5 Venn diagrams showing overlaps of discriminating metabolics between the experimental groups at EIC− (a) and EIC+ (b). | |
2.7 Metabolic pathway analysis
Potential target metabolic pathway analysis revealed that the metabolites identified by multivariate statistical analysis in the negative mode were responsible for the metabolism of glycosylphosphatidylinositol (GPI)-anchor biosynthesis and glycerophospholipid metabolism (P-value < 0.05, Fig. 6A). Metabolites in the positive mode are involved pathways of in glycerophospholipid metabolism, linoleic acid metabolism, alpha-linolenic acid metabolism and arachidonic acid metabolism (P-value < 0.05, Fig. 6B). The pathways of glycerophospholipid metabolism, ether lipid metabolism and glycerolipid metabolism are closely linked to each other (http://www.kegg.jp/).
 |
| Fig. 6 Pathway analysis for identified metabolites. All matched pathways are plotted according to P-value from pathway enrichment analysis and pathway impact score from pathway topology analysis. Each bubble in the bubble diagram represents a metabolic pathway. The horizontal axis and bubble size represent impact score from pathway topology analysis (the larger the circle the higher the impact score); the vertical coordinate where the bubble is and the bubble color indicate the P value (take the negative natural log, i.e. −log P-value) of the enrichment analysis (the darker the bubble the lower P-value). (A) Pathway analysis of the 32 identified metabolites at EIC−. (a) Glycosylphosphatidylinositol (GPI)-anchor biosynthesis; (b) glycerophospholipid metabolism (B) pathway analysis of the 26 identified metabolites at EIC+. (a) Glycerophospholipid metabolism; (b) linoleic acid metabolism; (c) alpha-linolenic acid metabolism; (d) arachidonic acid metabolism. | |
2.8 Network construction
Eight species and 137 kinds of lipid components were identified in the plasma, including PC, LPC, PI, PE, LPE, SM, HNE and Car. Knowing that the interaction model among them can be constructed with the support of IPA (an integrated biological pathway analysis software) and help predict diseases and functions by analyzing experimental data of metabolic groups, we imported the discriminating metabolites in plasma (both positive and negative modes) into IPA to establish an interactive network for the sake of enhancing our understanding about the most relevant and potential candidate biomarkers and canonical pathways of medications associated with NAFPD and T2DM. The path designer network in negative mode showed 9 CPs were involved with 37 chemicals, 6 of which are discriminating metabolites (Fig. 7A). Moreover, lipid metabolism, molecular transport and carbohydrate metabolism were presumed to be related with predictive analysis of disease and function. The path designer network in positive mode shows that 20 CPs were involved with 36 chemicals, 7 of them are discriminating metabolites (Fig. 7B), its main biological functions are lipid metabolism, molecular transport and small molecule biochemistry. In general, multitargets and multipathways were included in NAFPD and T2DM, and representative metabolites, such as PI, PE, PC and SM may be the targeting regulatory molecular biomarkers. Phosphatidylethanolamine biosynthesis, 3-phosphoinositide degradation, 3-phosphoinositide biosynthesis, type 2 diabetes mellitus, sphingomyelin metabolism, phosphatidylcholines biosynthesis, sphingomyelin-1-phosphate signaling, ceramide signaling, phospholipase, triacylglycerol biosynthesis could be the major interfering signaling pathways.
 |
| Fig. 7 The path designer network of discriminating metabolites (yellow mark) in positive (A) and negative modes (B). There are 7 discriminating metabolites in positive mode such as PI, PE and LPE, in which PI and PE tend to be central of the network; there are 6 discriminating metabolites in positive mode such PC, LPC, SM, carnosine and car, in which PC and SM tend to be central of the network. | |
3. Discussion
T2DM is a progressive process and patients can stay in a complex prodromal phase for many years. Thus, it is of great importance to find markers to track and evaluate the risk of T2DM at its early state. NAFPD is one of the high-risk conditions that precedes onset of T2DM, which has dramatically attracted attention for T2DM prevention and pathogenesis research. However, the underlying associations and molecular mechanisms between NAFPD and T2DM still remain poorly understood. Lipids represent an essential group of organic molecules, which can be further divided into diverse classes and subclasses. They are important energy storage molecules and the main constituents of cellular membranes.11,12 What's more, lipids are critical messenger molecules.13 Previous studies suggested that lipids may serve as mediators of IR.14 Since both NAFPD and T2DM are characterized by dysregulated lipid metabolism and closely associated with IR, we believe lipid metabolism is critical to unraveling the underlying mechanisms and possible relationship of these two important diseases. Lipid profile alteration is not only a consequence of diseases, but can also precede the onset of diseases, which allows it to be a biomarker of specific diseases and a predictor of disease progression in the future.15 So far, lipidomics analysis of human plasma has indeed discovered lipid signatures such as PC, PE, Cer, PI and TAG as potential disease biomarkers of ectopic fat deposition and T2DM over the past years.16–18 However, study on the complexity of lipid metabolism has been hampered by the analysis of defined lipid species and difficulty in separating lipid molecules. Thus, we integrated lipidomics with clinical research by virtue of the analytical power of MDMS-DL,19 which can separate various kinds of lipids effectively and make qualitative and quantitative analysis of individual lipids possible.
It is noteworthy that we revealed marked reduction of PC and PE plasmalogen in patients. The relationship between plasmalogen levels and metabolic diseases such as T2DM, cardiovascular disease, and some cancers has been found in identified lipidomic profiling of cohort studies. It was reported that plasmalogens had a negative associations with obesity,20 prediabetes and T2DM.21 In this study, we provided evidence of plasmalogen as a biomarker in NAFPD. The primarily existent forms of plasmalogen are pPE and pPC. Although the functions of plasmalogens have not yet been fully elucidated, it has been demonstrated that they can protect mammalian cells against the damaging effects of reactive oxygen species (ROS).22,23 MDMS-SL revealed that total pPE content was significantly reduced in NDM and DM patients compared with that in NC (P < 0.05), and DM group had more significantly changed pPE species than NDM group. Virtually, all pPE species showed varying degrees of reduction. As synthesis of pPE occurs in peroxisome because it generates the critical precursor for plasmalogen, we inferred a peroxidase dysfunction in disease groups, which can be supported by the decreased aPE content. As peroxisomes plays an important role in lipid metabolism and ROS modulation,24 its dysfunction can worsen dyslipidemia. The analysis of pPC classes in plasma showed a similar trend. Plasmalogens are well known to be endogenous ROS scavengers.25 Changes in plasmalogen homeostasis can exacerbate free fatty acid accumulation and mitochondrial dysfunction, which may increase the ROS level.26 Oxidative stress would result in IR through various stress sensitive intracellular pathways.27 The vinyl ether bond at the sn-1 position of plasmalogens is accessible to be attacked and cleavaged by ROS, leading to generation a large number of lipid peroxidation products including 4-hydroxyalkenals. 4-Hydroxyalkenals is a sensitive indicator of lipid peroxidation that can reflect oxidative stress level of the body. The increased HNE content in our study indicated that the patients are under enhanced oxidative stress and subsequent lipid peroxidation tend to be the underlying mechanism of marked reduction of pPE levels presented NDM and DM patients. In other words, low plasmalogens can lead to a vicious cycle of oxidative stress and IR, which may be underlying mechanism in the process of NAFPD and T2DM. In addition, we also detected a reduction in lysoPE and lysoPC in the plasma of these patients, which provides more evidence for the association between decreased lysoPE and lysoPC levels and metabolic diseases.28
OPLS analysis was performed to substantiate the alterations of lipid profiles between the NDM and DM patients and healthy controls. We applied strict inclusion and exclusion criteria for NDM and DM patients and controls to minimize potential influence from systemic confounding factors. Finally, 60 discriminating metabolites in patients, including 7 PI, 21 PE, 5 SM, 4 LysoPEs, 16 PC, 5 LysoPCs, 1 HHE and 1 Car were identified (VIP value > 1). Venn analysis showed that 15 of them tend to be metabolic biomarkers in progression to T2DM in NAFPD patients, which include 8 PEs, 3 PCs, 1 lysoPE, 2 lysoPC and 1 PI. These results further validate that phospholipids as represented by PC and PE are of great importance as predictor for T2DM in NAFPD patients. Among them, PC16:1-16:0 and PI 18:0-20:3 has the AUC ≥ 0.6 and is therefore considered to be the key metabolite for progression of T2DM in NAFPD patients. Obviously, these lipid candidates require further validation with rigorous clinical standard before clinical application.
Pathway analysis results revealed that the discriminating metabolites of NAFPD and T2DM was mainly related to metabolism of GPI-anchor biosynthesis, glycerophospholipid metabolism, linoleic acid metabolism, alpha-linolenic acid metabolism and arachidonic acid metabolism. Finally, the metabolic network constructed by correlating the disturbed metabolic pathways suggests that lipid metabolism, molecular transport, small molecule biochemistry and carbohydrate metabolism were also presumed related with disease progression according to IPA predictive analysis of disease and function. Glycerophospholipid metabolism pathway is related to disease progression in both positive and negative mode and glycerolphospholipids such as PI, PC and PE are in the central of the network. They can influence lipid and carbohydrate metabolism, which exhibits considerable evidence for their pathogenic role in NAFPD and T2DM.
Since the risk of diabetes begins to increase at comparatively normal BMI in Asian populations, ectopic fat deposition may be a better measure of actual diabetic risk. Our study not only provided evidence for NAFPD as a risk factor of T2DM, but also suggest that the NAFPD and T2DM patients are under increasing ROS level and enhanced oxidative stress. ROS and oxidative stress has been associated with an increased risk for IR and diabetes. However, to our knowledge, there have been no studies assessing levels of oxidative stress in Asian T2DM populations. Our findings may help to further explain the greater the deposition of visceral fat in Asians compared to Caucasians and other populations.29–31 We also validate the key phospholipid metabolites for progression of T2DM in NAFPD patients, which is closely related to IR. However, phospholipid metabolism can be influenced by diet. For example, PE and PI were characterized as high-fat diet-induced lipids in muscle in previous study.32 Higher intakes of refined carbohydrates, saturated fats, and trans fats could adversely affect lipid metabolism and IR, whereas a higher intake of polyunsaturated fat and possibly long-chain n-3 fatty acids could be beneficial.33 So these lipid candidates require further validation with consideration of different nutritional statuses. This association between NAFPD and T2DM needs to be further validated with the combination of various “omics”, i.e., metabolomics, genomics and proteomics and a larger number of patients. In addition, there was no statistically significant difference among the groups in our study and the study might not be adequately powered to be stratified by sex, which may due to the insufficient sample size. Further study by expanding the sample size to explore the correlation between gender and disease incidence as well as lipid profile characteristics is needed.
4. Materials and methods
The study was conducted in accordance with the Declaration of Helsinki (Finland, 1964). It also has followed all applicable institutional and government regulations concerning ethics in China and was approved by the Ethics Committee of Ningbo Chinese Medical Hospital. All subjects signed the informed consent form. The inclusion and exclusion criteria were same as we reported before.34
4.1 Participant recruitment and grouping
A total of 110 participants were enrolled for the MDMS-SL analysis in this study, including 80 patients with NAFPD and 30 healthy individuals. All participants were unrelated Chinese Han individuals who were recruited from the Ningbo Chinese Hospital from October 2015 to April 2017. Finally, a total of 107 subjects were divided into the 3 groups, namely the normal control group (NC group, n = 30), the NAFPD with no T2DM group (NDM group, n = 39, 1 patient taking statins is excluded), and NAFPD with T2DM (DM group, n = 38, 1 cancer patient and 1 pregnant woman is excluded).
4.2 Diagnostic criteria for NAFPD
All the ultrasonic data were collected by 2 licensed ultrasonic physicians, using high resolution ultrasound (LOGIQ7, GE, US) and 3.5 MHz linear sensor. An objective pancreas echo contrast was obtained by comparing the different echogenicity between the pancreas and spleen.35 The criteria for NAFPD diagnosis were as follows: (1) the increase in echogenicity of the pancreatic parenchyma was greater than that of the spleen and the echo enhancement area was larger than 80%; (2) the pancreas had a plump morphology, enlarged volume and fuzzy edge; and (3) the three values of the head, body and tail of the pancreas were close. Patients with pancreatic fibrosis were excluded.
4.3 Criteria for T2DM diagnosis
The diagnosis of T2DM was based on the 2010 American Diabetes Association (ADA) criteria,36 including (1) fasting blood glucose (FPG) ≥ 7.0 mmol L−1; (2) plasma glucose of 2 hours post glucose-load (2hPG) ≥11.1 mmol L−1 during an oral glucose tolerance test (OGTT)
4.4 Lipid profiling using MDMS-SL analysis
4.4.1 Lipid standards and chemicals. Lipid internal standards including choline glycerophospholipid (PC), ethanolamine glycerophospholipid (PE), lysophosphatidylethanolamine (LysoPE), lysophosphatidylcholine (LysoPC), acyl-carnitine (Car), ceramide (Cer), sphingomyelin (SM), 4-hydroxy-2(E)-nonenal (HNE) were purchased from Avanti Polar lipids, Inc. (Alabaster, AL, USA), Matreya, Inc. (Pleasant Gap, PA, USA), or Nu Chek, Inc. (Elysian, MN, USA). All the solvents were obtained from Burdick and Jackson (Honeywell International Inc., Muskegon, MI). All other reagents were at least analytical grade and purchased from Fisher (USA). The internal standard mixture was prepared according to literature and preliminary experimental results37 (Table S4†).
4.4.2 Plasma sample preparation. Human blood samples (5 mL) obtained from volunteers who met the above criteria were packed in a coagulation tube and stored at low temperature during the whole transportation process. Each blood sample was centrifuged at 376g for 10 min at room temperature. The supernatant was collected as the plasma sample. The samples were numbered and stored at −80 °C for subsequent experiment.
4.4.3 Lipid extraction. Lipids from each plasma sample (100 μL) were extracted with CHCl3/MeOH (1
:
1, v/v) according to the protocol of Bligh and Dyer38 in the presence of internal standards as previously described39 for global lipid analysis. The internal standard was added into each plasma sample based on its protein content. Specifically, 4 mL CHCl3/MeOH (1
:
1, v/v), 2 mL LiCl solution (50 mM), and internal standard (36 μL after calculation) were added to each plasma sample in a 10 mL centrifuge glass tube. The extraction mixtures were vortexed and centrifuged at 1150g for 5 min. The CHCl3 layer of each extract mixture was removed to another 10 mL centrifuge glass tube. An additional 2 mL of CHCl3 was added to the MeOH layer of each test tube, and the organic media from each individual sample was combined and dried under a nitrogen stream after centrifugation. Finally, each individual lipid extracts was resuspended in CHCl3/MeOH (1
:
1, v/v) corresponding to 2 mL mL−1 serum and flushed with nitrogen, capped, and stored at −20° for ESI/MS.
4.4.4 MDMS-SL analysis. The lipid extracts were further diluted with 1:2 CHCl3/MeOH/isopropanol (1
:
2
:
4, v/v) to ensure that the lipid concentration was below 50 pmol mL−1 to avoid lipid aggregation. Next, the diluted lipid extract was directly infused into the ESI ion source of a TSQ Quantum Ultra mass spectrometer (Thermo Fisher Scientific, San Jose, CA) equipped with an automated NanosPray apparatus (TriVersa NanoMate, Advion Bioscience Ltd, Ithaca, NY). A potential difference of 1.2 keV was applied between the electrospray needle and the interior of the ion source (320 °C). The customized sequence subroutine of the Xcalibur software was employed for automatic switch between different scanning modes (1 min for full MS scan mode, 2–5 min for tandem MS analysis) corresponding to all potentially occurring lipid classes of the serum sample to give rise to a final neutral loss scan. The first and third quadrupole detectors were set with a mass resolution of peak width 0.7 Th, while the second quadrupole serves as collision cell and argon was used as the collision gas. A collision gas pressure of 1.0 mTorr and a collision energy of 35 eV were employed.
4.5 Data processing
4.5.1 Data processing and statistical analysis. MS data processing, including noise filter, baseline correction, peak intensity identification, and quantitation was performed by Microsoft Office Excel 2010 (Microsoft, Redmond, WA) macros program. Data are presented as mean ± SEM. Dunnet t-tests were conducted to elucidate the altering lipid species by SPSS (23.0) to determine significant differences (P < 0.05) between the groups.
4.5.2 Multivariate statistical analysis. The raw MDMS-SL data for all samples were initially processed using Thermo Lipid Search v4.0.20 Software (Thermo Scientific, USA). The data from each sample were then normalized to total area and then imported into the software SIMCA-P (V13.0 Umetrics AB, Umea, Sweden) to perform multivariate statistical analysis in the form of supervised orthogonal partial least square (OPLS) analysis. Knowing that OPLS is beneficial to refocus the analysis towards discriminating lipids that contribute to the study objectives and removal of a systematic variation does not necessarily align with the scientific question of interest, we used the OPLS score plots and VIP statistics to select significant variables responsible for group separation. Then, these features were further subjected to a two-tailed t-test. Candidates with a OPLS-DA VIP > 1 and t-test p values < 0.5 was preferred for further analysis. Subsequent clustering heatmaps for discriminating candidates were performed by heatmap v1.0.12.
4.5.3 Network and pathway analysis. IPA is an integrated biological pathway analysis software based on cloud computing, which is supported by a highly structured biological information platform named Ingenuity Knowledge Base. Discriminating lipids were further analyzed by IPA to visualize the metabolomic data and identify potential metabolic pathways.
5. Conclusions
In this study, we for the first time utilized MDMS-SL to determine plasma lipid profile signature of NAFPD and T2DM patients, in an attempt to enhance the current understanding about NAFPD and explore potential lipid biomarkers for predicting prognosis from NAFPD to T2DM. The results demonstrate that pPC and pPE were decreased and 4-hydroxyalkenal level was increased remarkably in NDM and DM patients, probably due to lipid peroxidation resulting from increased oxidative stress and peroxisomal dysfunction. There was also a reduction in LysoPC and LysoPE, which is in line with previous studies. Our multivariate statistical analysis and ROC analysis demonstrated that PC 16:1-16:0 and PI 18:0-20:3 are key indicator in the lipid metabolism in NAFPD and T2DM patients in our study. Metabolic pathway analysis and functional analyses showed that the discriminating lipids were primarily involved in glycerophospholipids metabolism and glycosylphosphatidylinositol-anchored protein biosynthesis. Furthermore, lipid metabolism, molecular transport, small molecule biochemistry, and carbohydrate metabolism were also presumably related to NAFPD and T2DM according to the metabolic network constructed by IPA. Altogether, our study showed that the profile of plasma lipid alteration in NAFPD patients is similar to that in T2DM patients. Also, we identified several novel mutual lipid candidates in both NAFPD and T2DM groups. All these results demonstrate that NAFPD is not a silent spectator but a pathogenetic predictor of T2DM, and that lipid biomarkers have predictive power in predicting insidious progression to T2DM.
Conflicts of interest
We wish to confirm that there are no known conflicts of interest associated with this publication and there has been no significant financial support for this work that could have influenced the outcome.
Acknowledgements
This research was funded by National Natural Science Foundation of China (No. 81774177, No. 81603351).
Notes and references
- D. J. Porte and S. E. Kahn, Beta-cell dysfunction and failure in type 2 diabetes: potential mechanisms, Diabetes, 2001, 50(suppl. 1), S160–S163 CrossRef CAS PubMed.
- K. Ogurtsova, J. D. da Rocha Fernandes, Y. Huang, U. Linnenkamp, L. Guariguata, N. H. Cho, D. Cavan, J. E. Shaw and L. E. Makaroff, IDF Diabetes Atlas: Global estimates for the prevalence of diabetes for 2015 and 2040, Diabetes Res. Clin. Pract., 2017, 128, 40–50 CrossRef CAS PubMed.
- B. S. Romana, H. Chela, F. E. Dailey, F. Nassir and V. Tahan, Non-Alcoholic Fatty Pancreas Disease (NAFPD): A Silent Spectator or the Fifth Component of Metabolic Syndrome? A Literature Review, Endocr., Metab. Immune Disord.: Drug Targets, 2018, 18(6), 547–554 CrossRef CAS PubMed.
- C.-Y. Wang, H.-Y. Ou, M.-F. Chen, T.-C. Chang and C.-J. Chang, Enigmatic ectopic fat: prevalence of nonalcoholic fatty pancreas disease and its associated factors in a Chinese population, J. Am. Heart Assoc., 2014, 3, e000297 Search PubMed.
- U. P. Gujral, R. Pradeepa, M. B. Weber, K. M. V. Narayan and V. Mohan, Type 2 diabetes in South Asians: similarities and differences with white Caucasian and other populations, Ann. N. Y. Acad. Sci., 2013, 1281, 51–63 CrossRef PubMed.
- R. C. W. Ma and J. C. N. Chan, Type 2 diabetes in East Asians: similarities and differences with populations in Europe and the United States, Ann. N. Y. Acad. Sci., 2013, 1281, 64–91 CrossRef PubMed.
- M. J. Takkunen, U. S. Schwab, V. D. F. de Mello, J. G. Eriksson, J. Lindstrom, J. Tuomilehto and M. I. J. Uusitupa, Longitudinal associations of serum fatty acid composition with type 2 diabetes risk and markers of insulin secretion and sensitivity in the Finnish Diabetes Prevention Study, Eur. J. Nutr., 2016, 55, 967–979 CrossRef CAS PubMed.
- D. F. Markgraf, H. Al-Hasani and S. Lehr, Lipidomics—Reshaping the Analysis and Perception of Type 2 Diabetes, Int. J. Mol. Sci., 2016, 17(11), 1841 CrossRef PubMed.
- A. Floegel, N. Stefan, Z. Yu, K. Muhlenbruch, D. Drogan, H.-G. Joost, A. Fritsche, H.-U. Haring, M. Hrabe de Angelis and A. Peters, et al. Identification of serum metabolites associated with risk of type 2 diabetes using a targeted metabolomic approach, Diabetes, 2013, 62, 639–648 CrossRef CAS PubMed.
- K. T. Tonks, A. C. Coster, M. J. Christopher, R. Chaudhuri, A. Xu, J. Gagnon-Bartsch, D. J. Chisholm, D. E. James, P. J. Meikle and J. R. Greenfield, et al. Skeletal muscle and plasma lipidomic signatures of insulin resistance and overweight/obesity in humans, Obesity, 2016, 24, 908–916 CrossRef CAS PubMed.
- A. D. Barbosa, K. Lim and M. Mari, et al. Compartmentalized Synthesis of Triacylglycerol at the Inner Nuclear Membrane Regulates Nuclear Organization, Dev. Cell, 2019, 50(6), 755–766.e6 CrossRef CAS PubMed.
- T. C. Walther and R. V. J. Farese, Lipid droplets and cellular lipid metabolism, Annu. Rev. Biochem., 2012, 81, 687–714 CrossRef CAS PubMed.
- P. Hammerschmidt, D. Ostkotte, H. Nolte, M. J. Gerl, A. Jais, H. L. Brunner, H.-G. Sprenger, M. Awazawa, H. T. Nicholls and S. M. Turpin-Nolan, et al. CerS6-Derived Sphingolipids Interact with Mff and Promote Mitochondrial Fragmentation in Obesity, Cell, 2019, 177, 1536–1552 CrossRef CAS PubMed.
- S. Jacob, J. Machann, K. Rett, K. Brechtel, A. Volk, W. Renn, E. Maerker, S. Matthaei, F. Schick and C. D. Claussen, et al. Association of increased intramyocellular lipid content with insulin resistance in lean nondiabetic offspring of type 2 diabetic subjects, Diabetes, 1999, 48, 1113–1119 CrossRef CAS PubMed.
- B. Knebel, K. Strassburger, J. Szendroedi, J. Kotzka, M. Scheer, B. Nowotny, K. Mussig, S. Lehr, G. Pacini and H. Finner, et al. Specific Metabolic Profiles and Their Relationship to Insulin Resistance in Recent-Onset Type 1 and Type 2 Diabetes, J. Clin. Endocrinol. Metab., 2016, 101, 2130–2140 CrossRef CAS PubMed.
- Y. Wang, C. Zhao, J. Mao, J. Ai, M. Shenwu, T. Shi, D. Zhang, X. Wang and Y. Deng, Integrated lipidomics and transcriptomic analysis of peripheral blood reveals significantly enriched pathways in type 2 diabetes mellitus, BMC Med. Genomics, 2013, 6, S12 CrossRef PubMed.
- E. P. Rhee, S. Cheng, M. G. Larson, G. A. Walford, G. D. Lewis, E. McCabe, E. Yang, L. Farrell, C. S. Fox and C. J. O'Donnell, et al. Lipid profiling identifies a triacylglycerol signature of insulin resistance and improves diabetes prediction in humans, J. Clin. Invest., 2011, 121, 1402–1411 CrossRef CAS PubMed.
- K. T. Tonks, A. C. Coster, M. J. Christopher, R. Chaudhuri, A. Xu, J. Gagnon-Bartsch, D. J. Chisholm, D. E. James, P. J. Meikle and J. R. Greenfield, et al. Skeletal muscle and plasma lipidomic signatures of insulin resistance and overweight/obesity in humans, Obesity, 2016, 24, 908–916 CrossRef CAS PubMed.
- X. Han and R. W. Gross, Shotgun lipidomics: electrospray ionization mass spectrometric analysis and quantitation of cellular lipidomes directly from crude extracts of biological samples, Mass Spectrom. Rev., 2005, 24, 367–412 CrossRef CAS PubMed.
- J. M. Weir, G. Wong, C. K. Barlow, M. A. Greeve, A. Kowalczyk, L. Almasy, A. G. Comuzzie, M. C. Mahaney, J. B. M. Jowett and J. Shaw, et al. Plasma lipid profiling in a large population-based cohort, J. Lipid Res., 2013, 54, 2898–2908 CrossRef CAS PubMed.
- P. J. Meikle, G. Wong, C. K. Barlow, J. M. Weir, M. A. Greeve, G. L. MacIntosh, L. Almasy, A. G. Comuzzie, M. C. Mahaney and A. Kowalczyk, et al. Plasma lipid profiling shows similar associations with prediabetes and type 2 diabetes, PLoS One, 2013, 8, e74341 CrossRef CAS PubMed.
- S. Paul, G. I. Lancaster and P. J. Meikle, Plasmalogens: A potential therapeutic target for neurodegenerative and cardiometabolic disease, Prog. Lipid Res., 2019, 74, 186–195 CrossRef CAS PubMed.
- K. Gorgas, A. Teigler, D. Komljenovic and W. W. Just, The ether lipid-deficient mouse: tracking down plasmalogen functions, Biochim. Biophys. Acta, 2006, 1763, 1511–1526 CrossRef CAS PubMed.
- M. S. Dahabieh, E. Di Pietro, M. Jangal, C. Goncalves, M. Witcher, N. E. Braverman and S. V. Del Rincon, Peroxisomes and cancer: The role of a metabolic specialist in a disease of aberrant metabolism, Biochim. Biophys. Acta, Rev. Cancer, 2018, 1870, 103–121 CrossRef CAS PubMed.
- R. Maeba and N. Ueta, Ethanolamine plasmalogens prevent the oxidation of cholesterol by reducing the oxidizability of cholesterol in phospholipid bilayers, J. Lipid Res., 2003, 44, 164–171 CrossRef CAS PubMed.
- A. Selathurai, G. M. Kowalski, M. L. Burch, P. Sepulveda, S. Risis, R. S. Lee-Young, S. Lamon, P. J. Meikle, A. J. Genders and S. L. McGee, et al. The CDP-Ethanolamine Pathway Regulates Skeletal Muscle Diacylglycerol Content and Mitochondrial Biogenesis without Altering Insulin Sensitivity, Cell Metab., 2015, 21, 718–730 CrossRef CAS PubMed.
- J. E. Klaunig, Z. Wang, X. Pu and S. Zhou, Oxidative stress and oxidative damage in chemical carcinogenesis, Toxicol. Appl. Pharmacol., 2011, 254, 86–99 CrossRef CAS PubMed.
- K. T. Tonks, A. C. Coster, M. J. Christopher, R. Chaudhuri, A. Xu, J. Gagnon-Bartsch, D. J. Chisholm, D. E. James, P. J. Meikle and J. R. Greenfield, et al. Skeletal muscle and plasma lipidomic signatures of insulin resistance and overweight/obesity in humans, Obesity, 2016, 24, 908–916 CrossRef CAS PubMed.
- M. B. Weber, R. Oza-Frank, L. R. Staimez, M. K. Ali and K. M. Narayan, Type 2 diabetes in Asians: prevalence, risk factors, and effectiveness of behavioral intervention at individual and population levels, Annu. Rev. Nutr., 2012, 32, 417–439 CrossRef CAS PubMed.
- A. Misra, A. Ramchandran, R. Jayawardena, U. Shrivastava and C. Snehalatha, Diabetes in South Asians, Diabetic Med., 2014, 31(10), 1153–1162 CrossRef CAS PubMed.
- R. C. Ma and J. C. Chan, Type 2 diabetes in East Asians: similarities and differences with populations in Europe and the United States, Ann. N. Y. Acad. Sci., 2013, 1281, 64–91 CrossRef PubMed.
- N. Goto-Inoue, K. Yamada and A. Inagaki, et al. Lipidomics analysis revealed the phospholipid compositional changes in muscle by chronic exercise and high-fat diet, Sci. Rep., 2013, 3, 3267 CrossRef PubMed.
- F. B. Hu, R. M. van Dam and S. Liu, Diet and risk of Type II diabetes: the role of types of fat and carbohydrate, Diabetologia, 2001, 44(7), 805–817 CrossRef CAS PubMed.
- S. Weng, J. Zhou, X. Chen, Y. Sun, Z. Mao and K. Chai, Prevalence and factors associated with nonalcoholic fatty pancreas disease and its severity in China, Medicine, 2018, 97, e11293 CrossRef PubMed.
- D. M. Yang, H. C. Kim, J. K. Ryu, K. R. Joo and K. J. Ahn, Sonographic appearance of focal fatty infiltration of the pancreas, J. Clin. Ultrasound, 2010, 38, 45–47 Search PubMed.
- American Diabetes Association, Executive summary: Standards of medical care in diabetes–2010, Diabetes Care, 2010, 33(suppl. 1), S4–S10 Search PubMed.
- X. Han, S. Rozen and S. H. Boyle, et al. Metabolomics in early Alzheimer's disease: identification of altered plasma sphingolipidome using shotgun lipidomics, PLoS One, 2011, 6(7), e21643 CrossRef CAS PubMed.
- E. G. Bligh and W. J. Dyer, A rapid method of total lipid extraction and purification, Can. J. Biochem. Physiol., 1959, 37, 911–917 CrossRef CAS PubMed.
- X. Han, S. Rozen, S. H. Boyle, C. Hellegers, H. Cheng, J. R. Burke, K. A. Welsh-Bohmer, P. M. Doraiswamy and R. Kaddurah-Daouk, Metabolomics in early Alzheimer's disease: identification of altered plasma sphingolipidome using shotgun lipidomics, PLoS One, 2011, 6, e21643 CrossRef CAS PubMed.
Footnote |
† Electronic supplementary information (ESI) available. See DOI: 10.1039/c9ra07071k |
|
This journal is © The Royal Society of Chemistry 2019 |
Click here to see how this site uses Cookies. View our privacy policy here.