DOI:
10.1039/C9RA05836B
(Paper)
RSC Adv., 2019,
9, 35364-35371
Rapid lipidomics analysis for sepsis-induced liver injury in rats and insights into lipid metabolic pathways using ultra-performance liquid chromatography/mass spectrometry†
Received
27th July 2019
, Accepted 19th October 2019
First published on 1st November 2019
Abstract
Lipidomics has been applied in the identification and quantification of molecular lipids within an organism, and to provide insights into mechanisms in clinical medicine. Sepsis is a major systemic inflammatory syndrome and the liver here is a potential target organ for dysfunctional response. However, the study of alterations in global lipid profiles associated with sepsis-induced liver injury is still limited. In this work, we set out to determine alterations of lipidomics profiles in a rat model of sepsis-induced liver injury using an untargeted lipidomics strategy. Liquid chromatography coupled with mass spectrometry in conjunction with multivariate data analysis and pathway analysis were used to acquire a global lipid metabolite profile. Meanwhile, biochemistry index and histopathological examinations of the liver were performed to obtain auxiliary measurements for determining the pathological changes associated with sepsis-induced liver injury. Eleven lipid metabolites and two metabolic pathways were discovered and associated with sepsis-induced liver injury. The results indicated that various biomarkers and pathways may provide evidence for and insight into lipid profile alterations associated with sepsis-induced liver injury, and hence pointed to potential strategic targets for clinical diagnosis and therapy in the future.
1. Introduction
Lipids play a pivotal role in many biological functions such as regulation and disease prevention. Some studies have shown that lipid metabolisms are associated with the occurrence and development of diseases.1,2 Identification and quantification of lipids in living organisms offers new opportunities for understanding the functional role of various lipids in disease development.3 With a comprehensive data sets of mass spectrometry (MS) and MS/MS spectra, lipidomics can be used to quickly and sensitively monitor the composition and abundance of specific lipid molecules.4 Currently, the combination of high-performance liquid chromatography and MS greatly broadens the range of assays used to directly identify and precisely quantify hundreds of lipids from total lipid extract in a relatively short analysis time.5,6
Sepsis, which has a high mortality rate, is a serious systemic inflammation caused by infection or trauma, and usually results in multiple organ dysfunction syndrome (MODS) in patients in clinical practice.7,8 During the course of sepsis, the liver is one of the most critical potential targets of inflammatory disorders.9 Liver damage is a serious threat to human health, and leads to many painful hepatic diseases such as liver fibrosis, cirrhosis and cancer, bringing about a serious impact on the organism.10,11 It has been reported that sepsis-related liver injury is involved in the pathogenesis of MODS and contributes to its poor prognosis.12,13 Therefore, it is imperative to clarify the mechanism of pathological sepsis-induced liver injury and provide a safe and effective treatment strategy. Bacterial lipopolysaccharide (LPS) is a polysaccharide found in the outer membranes of all Gram-negative bacteria, and can give rise to inflammatory response syndrome, septic shock, and even organ dysfunction.14 It mainly results in liver damage, together with severe liver injury.15 In modern research, LPS has been applied to establish experimental models for dissecting endotoxin-induced liver injury mechanisms.
Previous studies have indicated the detrimental effects of lipid metabolism disorders on liver damage.16,17 However, there has not been much research on changes in the “global” lipid profile of sepsis-induced liver injury. Here, our aim was to use LC/MS to detect and investigate the changes of lipidomics in rats with sepsis-induced liver injury caused by LPS. This study provided new insights into lipid metabolism related to sepsis-induced liver damage, which may give us a better comprehension of the pathological mechanism and metabolic disturbance, and open up a new platform for drug discovery and development.
2. Materials and methods
2.1 Chemicals and reagents
Methanol and acetonitrile (chromatographic grade) were purchased from Tedia (Fairfield, CA, USA), formic acid was purchased from Honeywell (Morristown, New Jersey, USA), and distilled water was produced using a Milli-Q ultrapure water system (Millipore, Bedford, MA, USA). Lipid standards with purity > 99% were purchased from Sigma-Aldrich (St Louis, MO). LPS (E. coli, serotype O127:B8; Sigma-Aldrich, MO, USA) was diluted in 0.9% saline. Isofluorane and physiological saline solution were purchased from a drugstore (Harbin, China). Assay kits for alanine aminotransferase (ALT), aspartate aminotransferase (AST), alkaline phosphatase (ALP), and γ-glutamyl transpeptidase (γ-GT) were purchased from Sigma-Aldrich (Munich, Germany); those for tumor necrosis factor-α (TNF-α), interleukin-1β (IL-1β), interleukin-6 (IL-6) and interleukin-4 (IL-4) were purchased from Zhongsheng Beikong Biological Technology Limited Corporation (Beijing, China); and those for maleic dialdehyde (MDA) and superoxide dismutase (SOD) were purchased from Thermo Scientific (Waltham, MA, USA).
2.2 Animal treatments
Male Wistar rats weighing 180 ± 20 g were purchased from the Animals Experimentation Center of Heilongjiang University of Chinese Medicine and used after 7 days of acclimatization. All rats were fed under a controlled temperature (22–26 °C) and relative humidity (5 ± 5%) in pathogen-free cages, and a 12 h diurnal cycle was provided. For refraining from any external interference factors, the experiments met the requirements for the rats and cages being kept the same during the whole process. During the study, the rats were provided access to normal rodent pellet diet and water. After acclimatizing to these conditions for one week, 16 rats were randomly assigned into control saline-treated or LPS-treated model groups, with 8 animals for each group. Rats in the model group were deprived of food and water 6 h before the experiment, and then were injected with LPS at 5 mg kg−1 (isotonic NaCl solution diluted to 1 mL) in the tail vein to give rise to an LPS-induced liver injury model of sepsis.18,19 The experimental procedures were approved by the Animal Care and Ethics Committee at Heilongjiang University of Chinese Medicine and all experiments were performed in accordance to the Declaration of Helsinki.
2.3 Collection of biological samples
At 24 h after modeling, 10% chloral hydrate (5 mL kg−1) serving as an anesthetic was intraperitoneally injected into the two groups of rats. Blood samples from the ventral vena cava of each rat were delivered into Eppendorf tubes, and then centrifuged at 3000 rpm at 4 °C for 15 min, and the obtained serum samples were collected and stored at −80 °C until kit detection and lipidomics analysis was carried out. Tissue from the right lobe of the liver was promptly removed after obtaining the blood and fixed with a 10% neutral solution of paraformaldehyde to prepare paraffin sections for hematoxylin and eosin (H&E) staining analysis. All animals were euthanized by being injected with 3% pentobarbital sodium (0.1 mL/100 g weight) to induce enterotoxemia.
2.4 Lipid extraction
Serum was extracted for lipidomics analysis using the following method. Serum samples were thawed at 4 °C, and a volume of 50 μL of each sample was administered into a 2 mL microcentrifuge tube. On ice, 350 μL of methanol solution containing lipid internal standards were then added into the microcentrifuge tube. Each tube was then subjected to vortexing for 30 s, followed by adding 1 mL of methyl tert-butyl ether to the tube and then vibrating it for 1 h. A volume of 400 μL of the resulting supernatant was lyophilized in a 1.5 mL centrifuge tube, to which a volume of 250 μL of water was then added. The tube was then vortexed for 30 s and centrifuged at 12
000 rpm for 10 min at 4 °C. When the lyophilized material dissolved again, a volume of 40 μL dichloromethane–methanol (2
:
1, v/v) was added to the tube followed by 120 μL of acetonitrile–isopropanol–water (65
:
30
:
5, v/v) containing 5 mmol L−1 ammonium acetate, and the tube was then vortexed for 30 s. After centrifugation, the supernatant was placed in a glass vial for injection.
2.5 Clinical chemistry and histopathological examinations
The clinical biochemistry kits associated with liver function, inflammatory response and oxidation–reduction reactions, including those involving alanine amino-transferase (ALT), aspartate aminotransferase (AST), alkaline phosphatase (ALP) and γ-glutamyl transpeptidase (γ-GT), tumor necrosis factor-α (TNF-α), interleukin-1β (IL-1β), interleukin-4 (IL-4), interleukin-6 (IL-6), maleic dialdehyde (MDA) and superoxide dismutase (SOD), were used according to standard methods requirements of the kits. The right lobe of the liver was fixed by a 10% neutral solution of paraformaldehyde and then embedded in paraffin. Then 5 μm thick tissue sections of the liver were sliced from paraffin blocks for analysis using hematoxylin and eosin dye. Image-Pro Plus 5.0 software (Media Cybernetics, Bethesda, MD, USA) was used to observe H&E staining of tissue sections of control and model groups.
2.6 LC/MS analysis
A liquid chromatograph (LC) coupled to a mass spectrometer (Waters Corporation) was used for sample analysis, and consisted of a pump, autosampler and an ACQUITY BEH C 18 chromatography column with a 0.17 μm stationary phase. The column temperature was kept at 40 °C. The mobile phase gradient was produced using acetonitrile containing 0.1% formic acid as phase A and water with 0.1% formic acid as phase B, and the scheme 0–1 min, 1–40% A; 1–6 min, 40–100% A; 6–7 min, 100% A isocratic; 7–8 min, 100–1% A; 8–10 min, 1% A isocratic. The injection volume was 2 μL and flow rate was 0.3 mL min−1. A quality control (QC) sample containing all of the substances to be analyzed was used to inspect the column before analysis.
MS spectra of lipids were obtained by using an MS spectrometer equipped with an electrostatic ionization source including positive ionization mode (ESI+) and negative ionization mode (ESI−) operating at 100–2000 m/z in the full scan mode. The source parameters were set as follows: cone voltage of 30 V, capillary voltage of 3500 V, source temperature of 120 °C, desolvation temperature of 380 °C, desolvation gas flow of 500 L h−1, and cone gas flow of 40 L h−1 0.1 ng mL−1. Also, leucine enkephalin was applied as the reference ingredient under a flow rate of 120 μL min−1 in order to produce as precise measurements as possible. The analysis parameters were set as follows: fragment or voltage maintained at 70 V, collision energy of 20 V, and cell accelerator voltage of 15 V. The scan time and inter-scan delay were set to 0.2 s and 0.1 s, respectively. Nitrogen was applied for drying and collision.
2.7 Statistical analysis and data processing
All of the LC-MS raw data were initially analyzed using the MarkerLynx applications manager, and were subjected here to analytical performance inclusion criteria such as mass accuracy, precision, and retention time reproducibility. The MarkerLynx software was used to monitor each peak expressed by the retention time and m/z data pair. In each sample, the ion intensities of each peak detected were normalized to the sum of the peak intensities in that sample, which were subsequently multiplied by 10
000. Finally, the procedure was used to produce a table of mass and retention time with all relevant relative intensities of the detected peaks.
The normalized data reprocessed using MarkerLynx were imported to EZinfo 2.0 software (Waters Corporation, Manchester, UK) for multivariate analysis and constructing models; unsupervised principal components analysis (PCA) and supervised partial latent structures-discriminant analysis (PLS-DA) as well as orthogonal projection to latent structure-discriminant analysis (OPLS-DA) were carried out. S-plots and a VIP-plot were produced following OPLS analysis according to their contribution to the variation and correlation, and then the potential markers were extracted from the VIP-plot (>1). Based on querying databases such as HMDB (http://www.hmdb.ca/), MetaboAnalyst (http://www.metaboanalyst.ca/), and LIPID MAPS (http://www.Lipidmaps.org/tools/index.html) together with the highly precise mass measurement (mass error < 5 ppm) and MS/MS pattern, the selected lipid potential biomarkers were identified. The MetaboAnalyst 3.0 database was used to understand the pathway analysis and inner link in this study. All quantitative data were expressed as means ± SD and analyzed using SPSS Statistics 19.0 (SPSS, Chicago, IL, USA) for statistical comparisons of different groups. p values of less than 0.05 and less than 0.01 were considered significant and extremely significant, respectively. Correlation studies using the Pearson correlation test were done when appropriate (Fig. 1).
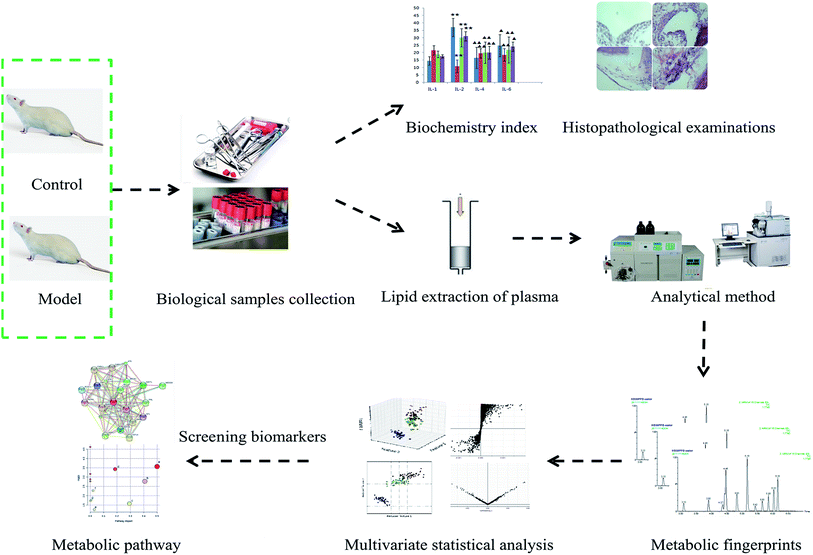 |
| Fig. 1 Overall flowchart for the experimental lipidomics approach analysis performed in the study. | |
3. Results and discussion
3.1 Results
Almost all of the model rats showed obviously slowed actions, slow reactions and anorexia after being injected with LPS. To verify the success of the animal model, the plasma levels of ALT, AST, ALP, γ-GT, TNF-α, IL-1β, IL-6, IL-4, MDA and SOD, widely considered to be biochemical criteria related to sepsis-induced liver injury, were measured in the control and model groups of rats. The serum concentrations of these biochemical items were within the normal ranges in the saline-treated control group, but were all distinctly altered in the model group. The levels of ALT, AST, ALP, γ-GT, TNF-α, IL-1β, IL-6 and MDA in the sera of the model group were evidently increased, and those of IL-4 and SOD were slightly decreased, compared with those of the control group. These biochemical results indicated that the model group rats were in an abnormal state, having displayed hepatic damage, immune reaction lipid peroxidation, and other related conditions; these results provided evidence for the success of the sepsis-induced liver injury animal model (Fig. 2A). In addition, disease severity was further verified by performing H&E staining of the liver (Fig. 2B). In contrast to the normal liver tissue of rats in the control group, the liver tissue in the model group showed an abnormal structure, lipid droplets, swollen cells, inflammatory cell infiltration, balloon-like changes in structures, and severe liver congestion. Both the biochemical and histopathological results thus indicated that the sepsis-induced liver injury model was successfully reproduced.
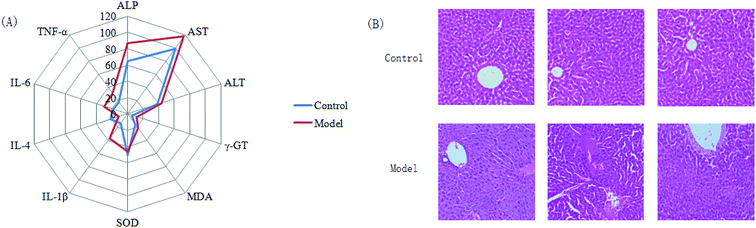 |
| Fig. 2 (A) Display of the pathological changes associated with sepsis-induced liver injury according to a clinical biochemistry analysis. In the model group, sepsis-induced liver injury resulted in significant increases in the serum levels of ALP (p < 0.05), AST (p < 0.05), ALT (p < 0.05), γ-GT (p < 0.01), MDA (p < 0.01), IL-1β (p < 0.05), IL-6 (p < 0.05), and TNF-α (p < 0.05) together with small decreases for SOD (p < 0.01) and IL-4 (p < 0.05) compared with the control groups. (B) H&E staining of liver tissue from the control and model groups. The model groups showed disordered hepatocyte cords, severe fatty degeneration, spotty or focal necrosis and infiltration of inflammatory cells; hemorrhagic necrosis with foci of lymphomonocytic infiltration around fibrotic tissue was also observed. | |
An untargeted approach for lipidomics research based on an LC/MS system was applied to acquire the lipid metabolic profiles of the control and model rats in ESI+ and ESI− modes. The total ion chromatograms in positive and negative ion modes are shown in Fig. S1.† Due to the complexity of the spectra, visual inspection of these LC/MS spectra showed less of a difference between the groups than would have been expected; hence, all of the resulting datasets were subsequently analyzed to explore the clustering of each group using multivariate data analysis for revealing any potential variables contributing to distinguishing the control from the sepsis-induced liver injury model. Original data from LC-MS were monitored and analyzed using Marker Lynx with the definite acquisition method and then imported into EZinfo 2.0 software. For obtaining a clear and comprehensive metabolic profile of sepsis-induced liver injury, PCA scores showing the original data (weight-averaged) and OPLS-DA score plots showing accurate calculations of the discrepancy between the control and model rats were applied in the subsequent LC/MS data analysis. In both positive ion mode (Fig. 3A) and negative ion mode (Fig. 4A), PCA score plots exhibited noticeable separation between the control and model groups, which indicated distinct biochemical alterations for the rats injected with LPS injection. As a more sophisticated analysis, small-molecule metabolites of sepsis-induced liver injury were used in OPLS-DA score plots in both positive ion mode (Fig. 3B) and negative ion mode (Fig. 4B). An S-plot from OPLS-DA score plots was used as a loading plot for exploring the covariance and correlation between the endogenous metabolites and causative factors, which usually identify atypical metabolites in the organism (Fig. 3C and 4C). The ions furthest away from the origin may be considered as the differentiating lipids. To optimize potential markers, the VIP score of the OPLS/DA data (Fig. 3D in positive ion mode and Fig. 4D in negative ion mode) expressed the contribution of the variables to the model. When exceeding a threshold of the VIP values, specifically VIP > 1.0, a variable was regarded as making a significant contribution to the clustering and discrimination.
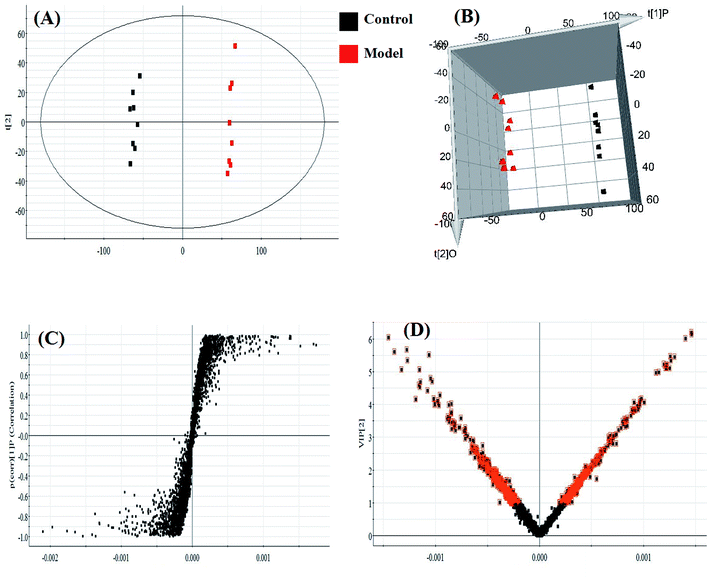 |
| Fig. 3 Multivariate statistical analysis of LC/MS data in positive ion mode. (A) PCA score plot of the lipid profile sorting the control and model groups in positive ion mode. (B) 3D PLS-DA model score plot of the LC-MS spectra data in positive ion mode. (C) S-plot and (D) VIP-plot of the OPLS-DA model of the LC-MS spectra data in positive ion mode. | |
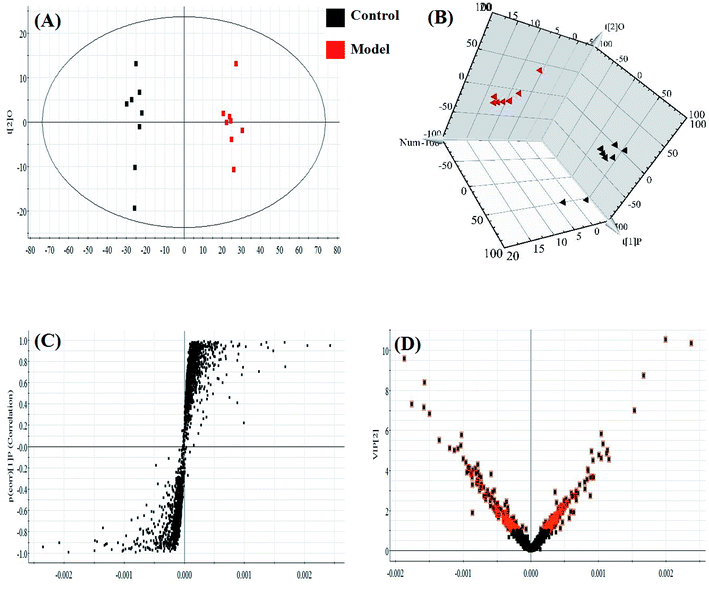 |
| Fig. 4 Multivariate statistical analysis of LC/MS data in negative ion mode. (A) PCA score plot of the lipid profile sorting the control and model groups in negative ion mode. (B) 3D PLS-DA model score plot of the LC-MS spectra data in negative ion mode. (C) S-plot and (D) VIP-plot of the OPLS-DA model of the LC-MS spectra data in negative ion mode. | |
In light of the criterion, markedly changed lipids closely related to sepsis-induced liver injury and that showed differences between the groups (t test, p < 0.05) were initially identified using HMDB, MetaboAnalyst 3.0 software, LIPID MAPS. Then, 11 potential biomarkers were identified after MS/MS analysis (Table S1†). Compared with control rats, the levels of PC(20:4(5Z,8Z,11Z,14Z)/15:0), PC(18:3(6Z,9Z,12Z)/20:2(11Z,14Z)), PC(18:2(9Z,12Z)/20:4 (8Z,11Z,14Z,17Z)), PG(18:3(6Z,9Z,12Z)/22:5(4Z,7Z,10Z,13Z,16Z)) and PE(14:1(9Z)/24:1(15Z)) were determined to be significantly up-regulated, and the levels of PG(18:2(9Z,12Z)/18:3(6Z,9Z,12Z)), PE(20:4(8Z,11Z,14Z,17Z)/15:0), PS(16:1(9Z)/20:3(8Z,11Z,14Z)), Cer(d18:0/18:1(9Z)), Cer(d18:0/18:0), SM(d18:1/22:0) were determined to be significantly down-regulated, from a hierarchical clustering heatmap (Fig. 5), with these biomarkers associated with glycerophospholipid metabolism and glycosylphosphatidylinositol-anchor biosynthesis (Fig. 6 and Table S2†).
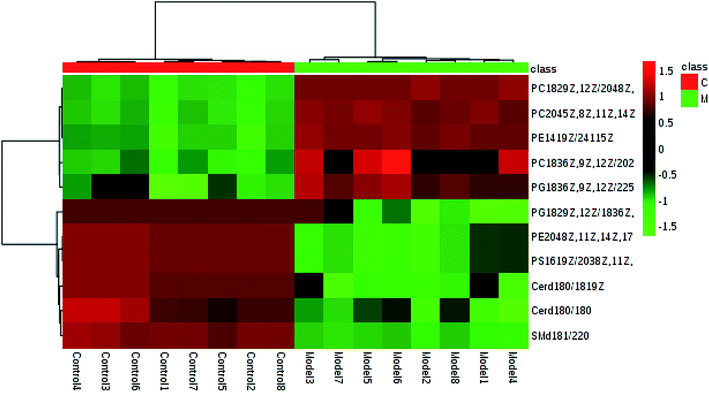 |
| Fig. 5 Hierarchical clustering heatmap of the 12 tested metabolites with up-regulation indicated in red and down-regulation in green. | |
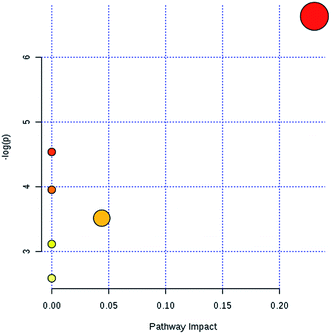 |
| Fig. 6 The metabolic pathway analysis results of lipid profile alterations in sepsis-induced liver injury. | |
3.2 Discussion
The LPS-induced sepsis rat model characterized by severe liver injury has been widely applied to study sepsis-induced liver injury. LPS has been recently shown, using biochemical and histological studies, to produce metabolic abnormalities resembling sepsis-induced liver injury in humans. Sepsis is a systemic inflammatory reaction brought about by the infection of specific pathogenic organisms such as bacteria, viruses, fungi and parasites, which lead to severe sepsis and septic shock. Severe sepsis is an acute and secondary organ dysfunction that is also named multiple organ dysfunction syndrome. Septic shock is a hypotension that cannot be reversed when severe sepsis is complicated with fluid resuscitation.20 LPS is capable of activating macrophages and other target cells to stimulate the production of various active factors in vivo. LPS extracted from bacteria displays a variety of physiological activities: it not only can cause pathological changes, but also improve the body's defense against tumors and infections. The mechanism of LPS toxicity resulting in sepsis mainly involves the production of a protective barrier around bacteria reducing the effectiveness of antibiotics; in addition, LPS in the host cells accelerates the release of TNF, IL and other cytokines to give rise to disorders in the body environment including a large number of reactive oxygen species, fever, diffuse intravascular coagulation and other clinical syndromes.21 The liver is the primary organ for endotoxin attack and is also a common target organ for immune damage. Some studies have shown that LPS is not only associated with aggravated cirrhosis and alcoholic liver injury, but also directly causes liver damage. The apoptosis of hepatocytes induced by LPS is related to the changed expression of bax, bcl-2, Fas and Fas.
Glycerophospholipids are an abundant type of phospholipids in cells, and constitute bile and membrane surface-active substances as well as biological membranes, and participate in the recognition and signal transduction of proteins in cell membranes.22 In the current work, the levels of PC(20:4(5Z,8Z,11Z,14Z)/15:0), PC(18:3(6Z,9Z,12Z)/20:2(11Z,14Z)), PC(18:2(9Z,12Z)/20:4(8Z,11Z,14Z,17Z)) in the model group of rats was significantly increased relative to those in the control group, which indicated that glycerophospholipid metabolism was abnormal in the model group. Many receptors, differentiated antigens, and some active proteins can be attached to the cell membrane through a glycolipid structure called glycosylphosphatidylinositol (GPI) anchored, which is the only way that proteins bind to the cell membrane. Compared with the control group, PE(20:4 (8Z,11Z,14Z,17Z)/15:0) was lower and PE(14:1(9Z)/24:1(15Z)) was increased in the model group, indicating that abnormal material identification and transport occurred in the cell, which has been shown to cause metabolic disorders.23 Some studies have shown that for cholesterol to function properly in the body and to show proper metabolism, it must be combined with linoleic acid. Body lipid metabolism disorders caused by insufficient amounts of linoleic acid have been shown to result in reduced immunity, forgetfulness, fatigue, vision loss and other symptoms. In addition, the lack of α-linoleum acid has been shown to be associated with deficient absorption and utilization of vitamins, minerals, proteins and other nutrients.24 Sphingolipids are associated with numerous important signal transduction processes involved in cell growth, differentiation, senescence, and programmed cell death. Hydrolysis of intracellular sphingomyelin is catalyzed by neurolipases in lysosomes.25 In patients with sepsis, abnormal metabolism of sphingolipid leads to phospholipase defects, which cause the accumulation of sphingomyelin in tissues. Such accumulation can seriously affect the liver, spleen, and central nervous system and even be fatal.26–30 In the state of sepsis-induced liver injury, inflammation in the body stimulates arachidonic acid metabolism and release of its metabolites, leading to fever, pain, vasodilatation, increased permeability and leukocyte exudation. A large number of lipids have been observed to play an important role in energy homeostasis, membrane structure and signaling.
4. Conclusions
In this study, we used a non-targeted lipidomics strategy to explore alterations in lipids associated with sepsis-induced liver injury in rats. LC/MS analysis data showed alterations of some potential lipid biomarkers in the development of sepsis-induced liver injury, including up-regulation of PC(20:4(5Z,8Z,11Z,14Z)/15:0), PC(18:3(6Z,9Z,12Z)/20:2(11Z,14Z)), PC(18:2(9Z,12Z)/20:4(8Z,11Z,14Z,17Z)), PG(18:3(6Z,9Z,12Z)/22:5(4Z,7Z,10Z,13Z,16Z)) and PE(14:1(9Z)/24:1(15Z)), and down-regulation of PG(18:2(9Z,12Z)/18:3(6Z,9Z,12Z)), PE(20:4(8Z,11Z,14Z,17Z)/15:0), PS(16:1(9Z)/20:3(8Z,11Z,14Z)), Cer(d18:0/18:1(9Z)), Cer(d18:0/18:0), SM(d18:1/22:0) and TG(22:0/24:1(15Z)/18:2(9Z,12Z)), biomarkers associated with glycerophospholipid metabolism and glycosylphosphatidylinositol-anchor biosynthesis. Such an improved understanding of the metabolite profile characteristics of sepsis-induced liver injury may find use in the in-depth study of the pathogenesis of this type of injury, and may also be regarded as a critical breakpoint for the diagnosis and treatment of sepsis-induced liver injury in the future.
Conflicts of interest
There are no conflicts to declare.
Acknowledgements
This work was supported by grants from the Key Program of Natural Science Foundation of State (Grant No. 81974557, 81774276, 81470196).
References
- Q. Liang, H. Liu and Y. Jiang, et al., Discovering lipid phenotypic changes of sepsis-induced lung injury using high-throughput lipidomic analysis, RSC Adv., 2016, 6, 38233–38237 RSC.
- S. B. Nayak and B. Stephanie, et al., Controlling Lipids AIDS in the Prevention of Type 2 Diabetes, Hypertension, and Cardiovascular Diseases, Int. J. Prev. Med., 2017, 8, 39 CrossRef.
- Q. Liang, H. Liu and X. Li, et al., High-throughput metabolomics analysis discovers salivary biomarkers for predicting mild cognitive impairment and Alzheimer's disease, RSC Adv., 2016, 6, 75499–75504 RSC.
- F. Yan, Z. Wen and R. Wang, et al., Identification of the lipid biomarkers from plasma in idiopathic pulmonary fibrosis by lipidomics, BMC Pulm. Med., 2017, 17, 174 CrossRef.
- X. Wang, J. Li and A. H. Zhang, Urine metabolic phenotypes analysis of extrahepatic cholangiocarcinoma disease using ultra-high performance liquid chromatography-mass spectrometry, RSC Adv., 2016, 6(67), 63049–63057 RSC.
- Q. Liang, H. Liu and Y. Jiang, et al., Novel liquid chromatography-mass spectrometry for metabolite biomarkers of acute lung injury disease, Anal. Methods, 2016, 8(31), 6017–6022 RSC.
- T. Tarui, Y. Yamaguchi and K. Suzuki, et al., Early evaluation of severity in patients with severe sepsis: a comparison with “septic shock” - subgroup analysis of the Japanese Association for Acute Medicine Sepsis Registry (JAAM-SR), Acute Medicine & Surgery, 2017, 4(4), 426–431 Search PubMed.
- D. B. Knox, M. J. Lanspa and K. G. Kuttler, et al., Phenotypic clusters within sepsis-associated multiple organ dysfunction syndrome, Intensive Care Med., 2015, 41(5), 814–822 CrossRef.
- D. Wang, Y. Yin and Y. Yao, Advances in sepsis-associated liver dysfunction, Burns & Trauma, 2014, 2(3), 97–105 Search PubMed.
- R. W. Walker, H. Allayee and A. Inserra, Macrophage accumulation and fibrosis in adipose tissue is linked to liver damage and metabolic risk in obese children, Obesity, 2014, 22(6), 1512–1519 CrossRef.
- Q. Liang, H. Liu and L. Xie, et al., High-throughput metabolomics enables biomarker discovery in prostate cancer, RSC Adv., 2017, 7(5), 2587–2593 RSC.
- N. Nesseler, Y. Launey and C. Aninat, et al., Clinical review: the liver in sepsis, Crit. Care, 2012, 16, 235 CrossRef.
- G. Albuszies and U. B. Brückner, Antioxidant therapy in sepsis, Intensive Care Med., 2003, 29, 1632–1636 CrossRef.
- C. Bandoro and J. A. Runstadler, Bacterial Lipopolysaccharide Destabilizes Influenza Viruses, mSphere, 2017, 2(5), e00267-17 CrossRef.
- Q. Liang, H. Liu and H. Xing, et al., Urinary UPLC-MS metabolomics dissecting the underlying mechanisms of huaxian capsule protects against sepsis, RSC Adv., 2016, 6, 40436–40441 RSC.
- Q. Liang, H. Liu and H. Xing, et al., UPLC-QTOF/MS based metabolomics reveals metabolic alterations associated with severe sepsis, RSC Adv., 2016, 6, 43293–43298 RSC.
- Q. Liang, C. Wang and B. Li, et al., Metabolic footprinting to understand therapeutic effects and mechanisms of silybin on acute liver damage in rat, Pharmacogn. Mag., 2015, 11(43), 586–593 CrossRef CAS.
- Y. Lu, W. Chen and K. Song, et al., 15-hydroxyprostaglandin dehydrogenase (15-PGDH) prevents lipopolysaccharide (LPS)-induced acute liver injury, PLoS One, 2017, 12(4), e0176106 CrossRef.
- K. M. Moon, B. Lee and Ji W. Jeong, et al., Thio-barbiturate-derived compounds are novel antioxidants to prevent LPS-induced inflammation in the liver, Oncotarget, 2017, 8(53), 91662–91673 CrossRef.
- J. D. Ruiz-Mesa, I. Marquez-Gomez and G. Sena, et al., Factors associated with severe sepsis or septic shock in complicated pyelonephritis, Medicine, 2017, 96(43), e8371 CrossRef.
- Y. Liu, B. Luer and X. Liying, et al., Chebulagic acid inhibits the LPS-induced expression of TNF-α and IL-1β in endothelial cells by suppressing MAPK activation, Exp. Ther. Med., 2015, 10(1), 263–268 CrossRef CAS.
- Q. Liang, H. Liu and T. Zhang, et al., Discovery of serum metabolites for diagnosis of mild cognitive impairment to Alzheimer's disease progression using an optimized metabolomics method, RSC Adv., 2016, 6, 3586–3591 RSC.
- H. Du, X. Zhao and A. Zhang, Identifying potential therapeutic targets of a natural product Jujuboside B for insomnia through network pharmacology, Plant Sci. Today, 2014, 1(2), 69–79 CrossRef.
- V. Lakshmanan, K. Y. Rhee and W. Wang, et al., Metabolomic Analysis of Patient Plasma Yields Evidence of Plant-Like α-Linolenic Acid Metabolism in Plasmodium falciparum, J. Infect. Dis., 2012, 206(2), 238–248 CrossRef CAS.
- M. Aslan, F. Özcan and H. Tuzcu, et al., Inhibition of neutral sphingomyelinase decreases arachidonic acid mediated inflammation in liver ischemia-reperfusion injury, Int. J. Clin. Exp. Pathol., 2014, 7(11), 7814–7823 Search PubMed.
- Y. Li, S. Qiu and A. Zhang, High-throughput metabolomics to identify metabolites serve as diagnostic biomarkers of prostate cancer, Anal. Methods, 2016, 8, 3284–3290 RSC.
- Q. Liang, H. Liu and H. Xing, et al., High-resolution mass spectrometry for exploring metabolic signatures of sepsis-induced acute kidney injury, RSC Adv., 2016, 6, 29863–29868 RSC.
- Q. Liang, H. Liu and Y. Jiang, et al., High-throughput metabolic profiling for discovering metabolic biomarkers of sepsis-induced acute lung injury, RSC Adv., 2016, 6, 11008–11013 RSC.
- Q. Liang, C. Wang and B. Li, et al., Lipidomics Analysis Based on Liquid Chromatography Mass Spectrometry for Hepatocellular Carcinoma and Intrahepatic Cholangiocarcinoma, RSC Adv., 2015, 5, 63711–63718 RSC.
- Q. Liang, H. Liu and T. Zhang, et al., Potential urine biomarkers from a high throughput metabolomics study of severe sepsis in a large Asian cohort, RSC Adv., 2015, 5, 102204–102209 RSC.
Footnote |
† Electronic supplementary information (ESI) available. See DOI: 10.1039/c9ra05836b |
|
This journal is © The Royal Society of Chemistry 2019 |
Click here to see how this site uses Cookies. View our privacy policy here.