DOI:
10.1039/C9RA05569J
(Paper)
RSC Adv., 2019,
9, 29973-29979
The fecal metabolome is associated with gestational diabetes mellitus†
Received
19th July 2019
, Accepted 11th September 2019
First published on 23rd September 2019
Abstract
Dysbiosis of gut microbiota has been linked to gestational diabetes mellitus (GDM), and grows as a resource for GDM biomarkers. However, the contributions of gut microbiota to GDM remain incompletely understood. Metabolites are key messengers in the interactions between gut microbiota and the host. Metabolomics is emerging as an essential tool in exploring the contributions of gut microbiota to diseases. In this study, we performed 1H-NMR based metabolomics on the feces of 62 pregnant women, including 31 women with GDM, and 31 women as the non-diabetes (NDM) control. Using Principle Component Analysis (PCA) and Orthogonal Projection to Latent Structures Discrimination Analysis (OPLS-DA), we observed clear cluster separation of the fecal metabolome between women with GDM and the NDM control. We further applied several feature selection methods to find five fecal metabolites contributing to the cluster separation of the fecal metabolome. These five metabolites, namely dibutyl decanedioate, N-acetylgalactosamine-4-sulphate, homocysteine, L-malic acid, and butanone, were significantly correlated with the clinical indices of GDM. Metabolite enrichment and pathway analysis on the five metabolites suggested that the fecal citrate cycle and sulfur metabolism were correlated with GDM. The results of this study demonstrated that disorders in the fecal metabolome are associated with GDM.
Introduction
Gestational diabetes mellitus (GDM) is a glucose intolerance with onset or first recognition during pregnancy.1 Diverse adverse outcomes are associated with GDM, including higher odds of macrosomia, respiratory distress, trauma, and cardiac complications, and increased risk of type 2 diabetes (T2DM) for the pregnant women and their offspring in the future.2,3 Although GDM shares similar symptoms with T2DM, onset only during pregnancy is the exclusive feature of GDM.4 GDM has attracted intensive studies due to its poor outcomes, however, the pathogenesis of GDM remains incompletely understood.5
Gut microbiota has been recently correlated with GDM.6,7 GDM is featured with dysbiosis in gut microbiota. The ratio of control-enriched bacteria to GDM enriched bacteria is negatively correlated with hyperglycemia.6 Metabolome and microbiota has been emerging as a source of potential biomarkers for GDM.8 A panel of four lipids (triglyceride [51:1], triglyceride [48:1], phosphatidylcholine [32:1], and choline ether) was reported to improve the predictive power of GDM by age and BMI alone from AUC (area under curve) 0.69 to AUC 0.74.9 N-Acetylaspartic acid and C16:1 in serum could discriminate GDM from the non-diabetic control with a good predictive power (AUC 0.75).10 Microbiota is also a biomarker resource for GDM. Kuang Y. S. et al.11 reported that 20 MLGs (metagenome linkage groups) from gut microbiota provide a discriminatory power of AUC 0.91. Biomarkers from feces support the development of non-invasive diagnostic approaches for GDM.12 While the associations between GDM and gut microbiota have been confirmed, the interaction mechanisms between gut microbiota and the host remain incompletely understood.13
Metabolites are key messengers in the interactions between gut microbiota and the host.14,15 Gut microbiota produced metabolites play essential roles in modulating host physiology and pathology,16,17 including short chain fatty acids (SCFAs) and trimethylamine oxide (TMAO). Metabolomics is emerging as a powerful tool in elucidating the interactions between gut microbiota and the host.18
In the present study, we performed 1H-NMR based metabolomics on fecal metabolome of GDM to observe that hyperglycemia is a discriminating factor of GDM fecal metabolome. GDM associated fecal metabolites were explored, and the related metabolic pathways were discussed.
Materials and methods
Study participants
A cohort of 62 pregnant women entered the Second Hospital of Shanxi Medical University (Shanxi province, PR China) between March and October of 2018 were enrolled in this study, including 31 women with GDM, and 31 non-diabetes (NDM) control (diagnosed as described below). Women with known pre-existing impaired fasting glucose, cardiovascular hematological diseases, abnormal kidney or liver function, chronic or severe acute infections were excluded. Fasting plasma was collected, and a 3 hour oral glucose tolerance test (OGTT) was performed during the 24th–28th week of gestation. GDM was diagnosed with the criteria of the International Association of Diabetes and Pregnancy Study Group (IADPSG), with at least one plasma glucose level no less than the following thresholds:19 fasting, 5.1 mmol L−1, OGTT-1 hour, 10.0 mmol L−1, OGTT-2 hour, 8.5 mmol L−1. The study was conducted according to the guidelines in the Declaration of Helsinki, and approved by the Ethics Committee of the Second Hospital of Shanxi Medical University.
Demographic data and sample collection
Demographic data of the enrolled pregnant women were obtained by interview at the same day with OGTT, including age, height, body weight, nationality, and blood pressure. Overnight fasting stool samples were collected during the fourth trimester of pregnancy and stored in a deep freezer at −80 °C.
1H-NMR based metabolic profiling
Stool sample preparation for 1H-NMR spectral profiling was performed based on a previously reported procedure with slight modifications.20 Briefly, 100 mg feces was resolved with 1 mL D2O (containing 0.05% TSP (3-trimethylsilyl-[2,2,3,3-D4]-propionate) as internal standard), homogenized in an ice-water bath with IKA T10 Basic ULTRA-TURRAX disperser (T10 Basic, IKA, Germany), and then centrifuged at 4 °C, 15
000 rpm for 15 min. Five hundred and fifty microliter of the supernatant was transferred into a 5 mm NMR tube for analysis. 1H-NMR spectrometry was profiled by a Bruker 600 MHz AVANCE III NMR spectrometer (Bruker BioSpin, Germany). The spectrum was acquired using noesygppr1d pulse sequence with the following parameters: spectral size 65
536 points, spectral width 12
345.7 Hz, pulse width 40.5 μs, relaxation delay 1.0 s, 64 scans. MestReNova (v8.0.1, Mestrelab Research, Santiago de Compostela, Spain) was used for spectra processing. The phase and baseline were corrected manually and the chemical shift of TSP was calibrated at 0.00 ppm. The spectra region of δ 0.16 to δ 9.58 were segmented at 0.01 ppm width after exclusion of the region of residual water (δ 4.60–5.15). The obtained NMR data was normalized to the total sum of spectra before further analysis.
Multivariate statistical analysis
MetaboAnalyst (version 4.0, http://www.metaboanalyst.ca) was applied for multivariate statistical analysis of the metabolomic data.21 An overview of the metabolomic data was obtained by principal component analysis (PCA). A supervised method named partial-least squares discrimination analysis (PLS-DA) was applied to identify the important variables with discriminative power, and to exclude outliers. Orthogonal projection to latent structures discrimination analysis (OPLS-DA) was employed to investigate the between-group differences by incorporating known classification information. The best-fitted OPLS-DA model was selected by a cross-validation of all models using a 200-cycle permutation test. The fitting validity and predictive ability of the selected OPLS-DA model were assessed by the parameters of multiple correlation coefficient (R2) and the cross-validated R2 (Q2). Metabolite was identified by searching the HMDB database (http://www.hmdb.ca) with the chemical shift and the coupling constant of the NMR features. Metabolic pathway enrichment was performed by the embedded module of MetaboAnalyst 4.0.
Results
Data pre-processing and quality control of the untargeted metabolome
A data integrity check was performed before subsequent analysis, with no missing value detected. The metabolomics data were then subjected to a normalization procedure including normalization by sum, log transformation, and Pareto scaling. A normal distribution of the metabolomics data was observed after the normalization (Fig. S1†). Quality control (QC) samples were used to monitor the robustness of sample preparation and the reproducibility of instrument analysis. QC sample was prepared by pooling equal aliquots of each fecal sample, and was analyzed after every ten test samples. Quality control of the metabolomic data was performed by PCA on the test samples and the QC samples. All of the QC samples were tightly clustered in the sample space of PCA (Fig. S2†), suggesting a good reproducibility of the metabolomic experiments.
Clear cluster separation in the fecal metabolome between GDM and the NDM control
To initially evaluate the natural separation of the metabolomic data and to select the principle features responsible for the separation, PCA was performed on the fecal metabolome of all the enrolled pregnant women (Fig. 1a). Two principle components were selected, which explained 39.2% of the overall variance (24.7% and 14.5% for PC1 and PC2, respectively). The fecal metabolome of GDM clustered and showed a separation trend with those of the NDM control.
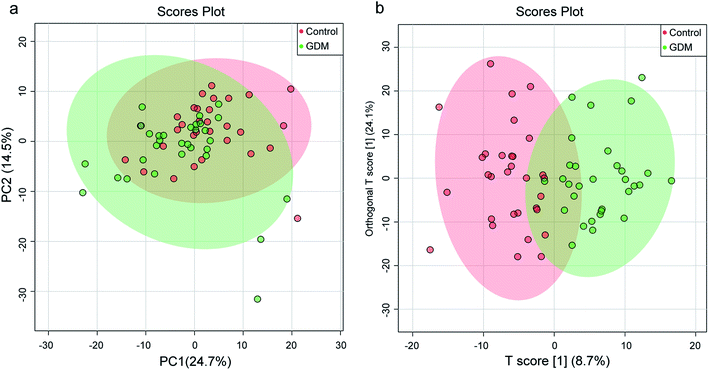 |
| Fig. 1 Principle component analysis (PCA) and orthogonal projection to latent structures discrimination analysis (OPLS-DA) on the fecal metabolome between women with gestational diabetes mellitus (GDM) and the non-diabetes mellitus (NDM) control. (a) Scores plot of PCA based on the first two principle components (PCs), which explain 39.2% of the variance (24.7% and 14.5% for PC1 and PC2, respectively). (b) Scores plot of OPLS-DA based on the first predictive component (T scores [1]) and the first orthogonal component (orthogonal T scores [1]), which explain 32.9% of the variance. | |
To better discriminate the fecal metabolome of GDM and the NDM control, a supervised OPLS-DA incorporating known classification information was performed (Fig. 1b). The best-fitted OPLS-DA model was selected by a cross-validation of all models using a 1000-cycle permutation test (Fig. S3†). The goodness of fit (R2) and prediction ability (Q2) of the selected model were 0.703 (P < 0.001) and 0.412 (P < 0.001), respectively. A clear separation of GDM and the NDM control was observed in the selected OPLS-DA model (Fig. 1b), suggesting that changes occurred in the metabolic profiles of GDM comparing to the NDM control.
Identification of differential fecal metabolites between GDM and the NDM control
The between-group differential metabolites were selected from the NMR features which simultaneously met all of the following criteria: significant changed relative abundances with adjusted P < 0.05 in a student's t-test (Fig. 2a), between group fold change of the relative abundance > 2 (Fig. 2b), adjusted P < 0.05 in significance analysis of microarrays (and metabolites) (Fig. 2c), Variable Importance for the Projection (VIP) values greater than 1 in the selected PLS-DA model (Fig. 2d). A total of 11 differential NMR features were selected, among which 5 metabolites were structurally identified (Table 1), including dibutyl decanedioate, N-acetylgalactosamine-4-sulphate, homocysteine, L-malic acid, and butanone. The relative abundances of the differential metabolites (Fig. 3) demonstrated that homocysteine and butanone were elevated, and the other three metabolites (dibutyl decanedioate, L-malic acid, and N-acetylgalactosamine-4-sulphate) were decreased in the feces of GDM.
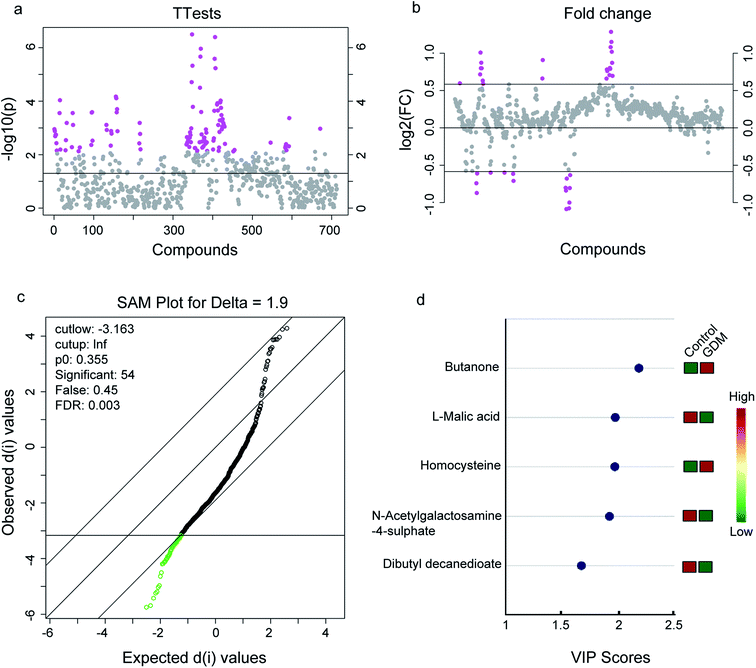 |
| Fig. 2 Differential metabolites in the fecal metabolome between women with gestational diabetes mellitus (GDM) and the non-diabetes mellitus (NDM) control. (a) T-Test on the whole 713 compounds extracted from the 1H-NMR spectrometry on the feces of the enrolled pregnant women. A total of 110 compounds with P-value < 0.01 were selected (marked with pink, see details in Table S2†). (b) Fold change analysis on the whole 713 compounds. A total of 35 compounds with fold change >2 were selected (marked with pink, see details in Table S3†). (c) Significant analysis of metabolites (SAM) on the whole 713 compounds. A total of 54 compounds with adjusted-P value < 0.05 were selected (marked with green, see details in Table S4†). (d) Variables importance in the projection (VIP) analysis of the structurally identified metabolites. Only metabolites with VIP score > 1 were exhibited (see details in Table 1). | |
Table 1 Fecal metabolites with differential abundances between GDM and the NDM controla
Name |
Chemical shift |
VIP |
p[1] |
p(corr)[1] |
p-value |
FDR |
s: single-peak, d: doublet-peak t: triplet-peak, q: quartet-peak; m: multiplet-peak. VIP: variable importance in the projection. FDR: false discovery rate, p(corr)[1]: correlation coefficient calculated with principle component 1 of the selected OPLSDA model. p[1]: covariance coefficient calculated with principle component 1 of the selected OPLSDA model. |
Dibutyl decanedioate |
2.206 s, 2.363 d, 4.062 s, 4.113 s |
1.6756 |
−2.6395 |
−0.5885 |
1.94 × 10−5 |
1.73 × 10−3 |
N-Acetylgalactosamine-4-sulphate |
4.06 s, 5.26 s |
1.9259 |
−3.3358 |
−0.5873 |
1.34 × 10−4 |
5.95 × 10−3 |
Homocysteine |
2.15 m, 2.66 m |
1.9739 |
3.4692 |
0.5821 |
1.99 × 10−4 |
7.36 × 10−3 |
L-Malic acid |
2.35 dd, 4.30 dd |
1.9745 |
−3.1200 |
−0.6590 |
1.10 × 10−6 |
2.61 × 10−4 |
Butanone |
1.06 t, 2.14 s, 2.46 q |
2.1865 |
4.0019 |
0.5938 |
2.78 × 10−4 |
7.36 × 10−3 |
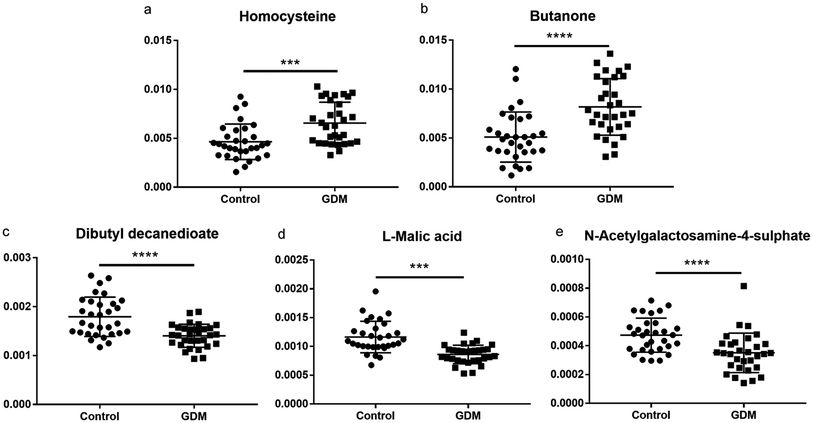 |
| Fig. 3 Relative abundance of the differential fecal metabolites between women with GDM and the NDM control. (a) homocysteine; (b) butanone; (c) dibutyl decanedioate; (d) L-malic acid; (e) N-acetylgalactosamine-4-sulphate. The relative abundance of each metabolite was calculated with the relative peak area (each peak area divided by the total peak area of a sample). The between-group statistic significance was calculated with Student's t-test. ***, P < 0.001, ****, P < 0.0001. | |
The differential fecal metabolites were correlated with the clinical indices of GDM
To investigate the relationship between the fecal metabolome and GDM associated clinical variables, spearman rank correlation was performed (Fig. 4a and Table S5†). Among the GDM related clinical indices, fasting glucose level was positively correlated with butanone and homocysteine (P < 0.01), and negatively correlated with L-malic acid, dibutyl decanedioate, and N-acetylgalactosamine-4-sulphate (P < 0.01). DeltaBMI (the BMI increase from before pregnancy to the third trimester of pregnancy) was positively correlated with L-malic acid (P < 0.01). While no metabolite was correlated with blood pressure, total plasma triglyceride, and plasma triglyceride (P > 0.05). Among the five differential metabolites between GDM group and the NDM control group, butanone was positively correlated with homocysteine (P < 0.01), and L-malic acid was positively correlated with dibutyl decanedioate (P < 0.01). Members of the positive-correlated metabolite-pairs (butanone vs. homocysteine and L-malic acid vs. dibutyl decanedioate) were negatively correlated with L-malic acid, dibutyl decanedioate and N-acetylgalactosamine-4-sulphate, respectively. These results suggested that gut metabolites may contribute to the changes in GDM related clinical indices.
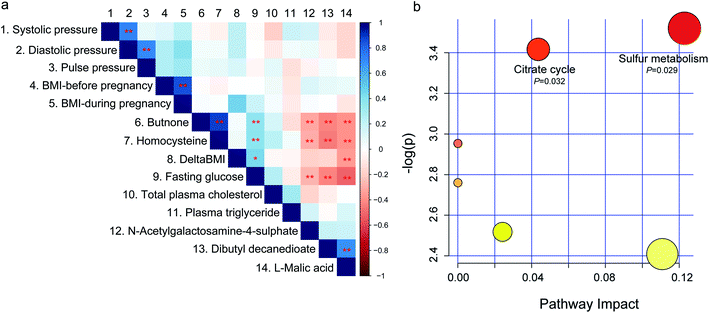 |
| Fig. 4 Spearman correlation and pathway analysis of the differential fecal metabolites. (a) Spearman rank correlation between the differential fecal metabolites and the clinical indices of GDM. A number was endowed to each metabolite or clinical parameter, which is corresponding to the number on the top of figure. *, P < 0.05, **, P < 0.01. (b) Metabolite enrichment and pathway analysis of the differential fecal metabolites between women with GDM and the NDM control using the MetaboAnalyst web portal. Only pathways with impact scores higher than 0.05 and P-Values lower than 0.05 were labelled. | |
Fecal citrate cycle and sulfur metabolism were correlated with GDM
Because the GDM associated differential fecal metabolites showed significant correlations among each other, we wondered if there are shared metabolic pathways existed among them. For further biological interpretation, metabolite enrichment and pathway analysis were performed on the five differential metabolites by MetaboAnalyst web portal using the integrated pathway tool. Citrate cycle (P = 0.032) and sulfur metabolism (P = 0.029) were significantly enriched (Fig. 4b). Sulfur metabolism is essential in the maintain of cell redox homeostasis.22 Citrate cycle is the central metabolic hub of the cell,23 and is suppressed in diabetes mellitus.24 Because no correlation network among the five differential metabolites were observed (data not shown), we speculated that these metabolites may independently act on the host. The results of this study, together with those of previous reports,25,26 suggested that energy metabolism and redox homeostasis were disturbed in the fecal metabolome of GDM.
Discussion
While alternations in the composition of gut microbiome are recognized as potential biomarkers of GDM,27 little is known how gut metabolome affect GDM. As fecal metabolome has been proved to be a functional readout of the gut microbiome,28 we performed 1H-NMR based fecal metabolomics to find GDM associated fecal metabolites and their related metabolic pathways. The results of this study suggested that gut metabolome may contribute to GDM through citrate cycle and sulfur metabolism associated metabolites.
Metabolomics has been recognized as a powerful approach in the study of gestational diabetes.18,29 GDM associated metabolic disorders have been reported in maternal blood,30 maternal urine,31,32 cord blood33 and fetal serum.34 In addition to the previous reports, this study provides evidences to support metabolic disorder in feces of women with GDM. Changes in metabolic pathways during pregnancy were revealed by previous metabolomics studies. Enhancement in citrate cycle was reported previously in maternal urine of fetal malformations, suggesting increased fetal energy requirement.35 Sulfur metabolism in the serum of pregnant women was associated with serum glucose at OGTT-1 h in a cohort of northern European mothers,36 which is similar to the observation of this study.
Homocysteine is an essential marker of cardiovascular risk, while women with GDM or a history of GDM have a significantly elevated serum level of homocysteine.37,38 Excess homocysteine production during pregnancy in animal experiments results in the impairment of reproductive and developmental processes.39 However, the sources of homocysteine have not been comprehensively elucidated. Gut microbiome is the sources of several key metabolites with essential functions on host physiology including short chain fatty acids,40 TMAO,41 etc. In the present study, we observed that fecal homocysteine of women with GDM was significantly elevated. And the elevated homocysteine is associated with fasting glucose and three other fecal metabolites including N-acetylgalactosamine-4-sulphate, dibutyl decanedioate, and L-malic acid (Fig. 4a). These results suggested that fecal homocysteine may contribute to GDM.
L-Malic acid is an intermediate of the citric acid cycle that is essential in energy metabolism.42 L-Malic acid could directly inactivate the enzyme activity of alpha-glucosidase.43 Alpha glucosidase is involved in the final step of the carbohydrate digestion, which is directly related to the levels of plasma glucose.43 The abundance of L-malic acid was significantly reduced in liver of the diabetic rats44 and in peripheral nerves of type I diabetes.45 The fecal abundance of L-malic acid was significantly reduced in GDM women in this study, suggesting an opposite effect of fecal metabolites on hyperglycemia.
Butanone, also known as methyl ethyl ketone, is a colorless liquid that has a sweet or sharp and fragrant acetone-like odour.46 Exposure to butanone results in cognitive rehabilitation and brain injury.47 Dibutyl decanedioate as a fatty acid ester, is used as a flavoring agent with no safety concern.48 To our knowledge, the variations in the levels of butanone and dibutyl decanedioate in the fecal metabolome of GDM were firstly reported in the present study. Their exact contributions to GDM need further explorations.
In summary, a clear separation was observed in OPLS-DA of the fecal metabolome between women with GDM and the NDM control. Five fecal metabolites with differential abundance were identified, among which two metabolites (homocysteine and butanone) were elevated and the other three (L-malic acid, dibutyl decanedioate, and N-acetylgalactosamine-4-sulphate) were decreased in women with GDM. The five differential fecal metabolites and their related metabolic pathways (citrate cycle and sulfur metabolism) were significantly correlated with the clinical indices of GDM. The results of this study suggested that fecal metabolome is associated with GDM.
Conflicts of interest
The authors declare that they have no conflict of interest in this work.
Acknowledgements
This study was supported by Shanxi Soft Science Research Program (No. 2018041031-2 and No. 2017041038-3) and Shanxi Undergraduate Training Program for Innovation and Entrepreneurship (No. 2018185).
References
- R. Agha-Jaffar, N. Oliver, D. Johnston and S. Robinson, Nat. Rev. Endocrinol., 2016, 12, 533–546 CrossRef PubMed.
- T. A. Buchanan, A. H. Xiang and K. A. Page, Nat. Rev. Endocrinol., 2012, 8, 639–649 CrossRef CAS PubMed.
- R. F. Goldstein, S. K. Abell, S. Ranasinha, M. Misso, J. A. Boyle, M. H. Black, N. Li, G. Hu, F. Corrado, L. Rode, Y. J. Kim, M. Haugen, W. O. Song, M. H. Kim, A. Bogaerts, R. Devlieger, J. H. Chung and H. J. Teede, JAMA, 2017, 317, 2207–2225 CrossRef PubMed.
- L. Zajdenverg and C. A. Negrato, Archives of Endocrinology and Metabolism, 2017, 61, 208–210 CrossRef PubMed.
- K. P. Law and H. Zhang, Clin. Chim. Acta, 2017, 468, 60–70 CrossRef CAS PubMed.
- J. Wang, J. Zheng, W. Shi, N. Du, X. Xu, Y. Zhang, P. Ji, F. Zhang, Z. Jia, Y. Wang, Z. Zheng, H. Zhang and F. Zhao, Gut, 2018, 67, 1614–1625 CrossRef CAS PubMed.
- I. Ferrocino, V. Ponzo, R. Gambino, A. Zarovska, F. Leone, C. Monzeglio, I. Goitre, R. Rosato, A. Romano, G. Grassi, F. Broglio, M. Cassader, L. Cocolin and S. Bo, Sci. Rep., 2018, 8, 12216 CrossRef PubMed.
- K. Mokkala, N. Houttu, T. Vahlberg, E. Munukka, T. Ronnemaa and K. Laitinen, Acta Diabetol., 2017, 54, 1147–1149 CrossRef PubMed.
- L. Lu, A. Koulman, C. J. Petry, B. Jenkins, L. Matthews, I. A. Hughes, C. L. Acerini, K. K. Ong and D. B. Dunger, Diabetes Care, 2016, 39, 2232–2239 CrossRef CAS PubMed.
- W. Hou, X. Meng, A. Zhao, W. Zhao, J. Pan, J. Tang, Y. Huang, H. Li, W. Jia, F. Liu and W. Jia, Mol. Cell. Proteomics, 2018, 17, 431–441 CrossRef CAS PubMed.
- Y. S. Kuang, J. H. Lu, S. H. Li, J. H. Li, M. Y. Yuan, J. R. He, N. N. Chen, W. Q. Xiao, S. Y. Shen, L. Qiu, Y. F. Wu, C. Y. Hu, Y. Y. Wu, W. D. Li, Q. Z. Chen, H. W. Deng, C. J. Papasian, H. M. Xia and X. Qiu, GigaScience, 2017, 6, 1–12 CrossRef PubMed.
- Q. Liang, J. Chiu, Y. Chen, Y. Huang, A. Higashimori, J. Fang, H. Brim, H. Ashktorab, S. C. Ng, S. S. M. Ng, S. Zheng, F. K. L. Chan, J. J. Y. Sung and J. Yu, Clin. Cancer Res., 2017, 23, 2061–2070 CrossRef CAS PubMed.
- O. Koren, J. K. Goodrich, T. C. Cullender, A. Spor, K. Laitinen, H. K. Backhed, A. Gonzalez, J. J. Werner, L. T. Angenent, R. Knight, F. Backhed, E. Isolauri, S. Salminen and R. E. Ley, Cell, 2012, 150, 470–480 CrossRef CAS PubMed.
- D. Much, A. Beyerlein, A. Kindt, J. Krumsiek, F. Stuckler, M. Rossbauer, A. Hofelich, D. Wiesenacker, S. Hivner, M. Herbst, W. Romisch-Margl, C. Prehn, J. Adamski, G. Kastenmuller, F. Theis, A. Ziegler and S. Hummel, Diabetologia, 2016, 59, 2193–2202 CrossRef CAS PubMed.
- M. S. Donia and M. A. Fischbach, Science, 2015, 349, 1254766 CrossRef PubMed.
- M. Priyadarshini, A. Thomas, A. C. Reisetter, D. M. Scholtens, T. M. S. Wolever, J. L. Josefson and B. T. Layden, Transl. Res., 2014, 164, 153–157 CrossRef CAS PubMed.
- Z. Wang, E. Klipfell, B. J. Bennett, R. Koeth, B. S. Levison, B. Dugar, A. E. Feldstein, E. B. Britt, X. Fu, Y. M. Chung, Y. Wu, P. Schauer, J. D. Smith, H. Allayee, W. H. Tang, J. A. DiDonato, A. J. Lusis and S. L. Hazen, Nature, 2011, 472, 57–63 CrossRef CAS PubMed.
- X. Mao, X. Chen, C. Chen, H. Zhang and K. P. Law, Clin. Chim. Acta, 2017, 475, 116–127 CrossRef CAS PubMed.
- L. S. Weinert, Diabetes Care, 2010, 33, e97–e98 CrossRef PubMed.
- S. Lamichhane, C. C. Yde, M. S. Schmedes, H. M. Jensen, S. Meier and H. C. Bertram, Anal. Chem., 2015, 87, 5930–5937 CrossRef CAS PubMed.
- J. Chong, O. Soufan, C. Li, I. Caraus, S. Li, G. Bourque, D. S. Wishart and J. Xia, Nucleic Acids Res., 2018, 46, W486–W494 CrossRef CAS PubMed.
- F. Carbonero, A. C. Benefiel, A. H. Alizadeh-Ghamsari and H. R. Gaskins, Front. Physiol., 2012, 3, 448 CAS.
- M. P. Murphy and L. A. J. O'Neill, Cell, 2018, 174, 780–784 CrossRef CAS PubMed.
- D. Sachse, L. Sletner, K. Mørkrid, A. K. Jenum, K. I. Birkeland, F. Rise, A. P. Piehler and J. P. Berg, PLoS One, 2012, 7, e52399 CrossRef CAS PubMed.
- G. Boden, Obstet. Gynecol. Clin. N. Am., 1996, 23, 1–10 CrossRef CAS PubMed.
- K. Yanar, S. Aydin, B. Simsek, I. Yaylim, S. Turan, M. E. Sitar, C. Cacina, O. Kucukhuseyin, A. Tuten, U. Cakatay and A. Benian, Can. J. Diabetes, 2019, 43, 173–178 CrossRef PubMed.
- M. K. W. Crusell, T. H. Hansen, T. Nielsen, K. H. Allin, M. C. Ruhlemann, P. Damm, H. Vestergaard, C. Rorbye, N. R. Jorgensen, O. B. Christiansen, F. A. Heinsen, A. Franke, T. Hansen, J. Lauenborg and O. Pedersen, Microbiome, 2018, 6, 89 CrossRef PubMed.
- J. Zierer, M. A. Jackson, G. Kastenmuller, M. Mangino, T. Long, A. Telenti, R. P. Mohney, K. S. Small, J. T. Bell, C. J. Steves, A. M. Valdes, T. D. Spector and C. Menni, Nat. Genet., 2018, 50, 790–795 CrossRef CAS PubMed.
- C. F. McCabe and W. Perng, Curr. Diabetes Rep., 2017, 17, 57 CrossRef PubMed.
- B. Gelaye, C. B. Clish, M. Denis, G. Larrabure, M. G. Tadesse, A. Deik, K. Pierce, K. Bullock, C. Dennis, D. A. Enquobahrie and M. A. Williams, Diabetes Metab., 2019, 45, 39–46 CrossRef CAS PubMed.
- M. Leitner, L. Fragner, S. Danner, N. Holeschofsky, K. Leitner, S. Tischler, H. Doerfler, G. Bachmann, X. Sun, W. Jaeger, A. Kautzky-Willer and W. Weckwerth, Front. Mol. Biosci., 2017, 4, 84 CrossRef PubMed.
- X. Chen, J. V. de Seymour, T. L. Han, Y. Xia, C. Chen, T. Zhang, H. Zhang and P. N. Baker, Metabolomics, 2018, 14, 149 CrossRef PubMed.
- E. Shokry, L. Marchioro, O. Uhl, M. G. Bermudez, J. A. Garcia-Santos, M. T. Segura, C. Campoy and B. Koletzko, Acta Diabetol., 2019, 56, 421–430 CrossRef PubMed.
- Y. P. Lu, C. Reichetzeder, C. Prehn, K. von Websky, T. Slowinski, Y. P. Chen, L. H. Yin, B. Kleuser, X. S. Yang, J. Adamski and B. Hocher, Cell. Physiol. Biochem., 2018, 45, 625–638 CrossRef CAS PubMed.
- S. O. Diaz, J. Pinto, G. Graca, I. F. Duarte, A. S. Barros, E. Galhano, C. Pita, C. Almeida Mdo, B. J. Goodfellow, I. M. Carreira and A. M. Gil, J. Proteome Res., 2011, 10, 3732–3742 CrossRef CAS PubMed.
- D. M. Scholtens, J. R. Bain, A. C. Reisetter, M. J. Muehlbauer, M. Nodzenski, R. D. Stevens, O. Ilkayeva, L. P. Lowe, B. E. Metzger, C. B. Newgard, W. L. Lowe and H. S. C. R. Grp, Diabetes, 2016, 65, 2039–2050 CrossRef CAS PubMed.
- M. Mascarenhas, S. Habeebullah and M. G. Sridhar, J. Pregnancy, 2014, 2014, 123024 Search PubMed.
- S. Alatab, H. Fakhrzadeh, F. Sharifi, M. Mirarefin, Z. Badamchizadeh, M. Ghaderpanahi, A. Hossein-Nezhad and B. Larijani, J. Diabetes Metab. Disord., 2013, 12, 34 CrossRef PubMed.
- M. M. Murphy and J. D. Fernandez-Ballart, Adv. Clin. Chem., 2011, 53, 105–137 CAS.
- D. J. Morrison and T. Preston, Gut Microbes, 2016, 7, 189–200 CrossRef PubMed.
- L. Hoyles, M. L. Jimenez-Pranteda, J. Chilloux, F. Brial, A. Myridakis, T. Aranias, C. Magnan, G. R. Gibson, J. D. Sanderson, J. K. Nicholson, D. Gauguier, A. L. McCartney and M. E. Dumas, Microbiome, 2018, 6, 73 CrossRef PubMed.
- Z. Chi, Z. P. Wang, G. Y. Wang, I. Khan and Z. M. Chi, Crit. Rev. Biotechnol., 2016, 36, 99–107 CrossRef CAS PubMed.
- L. Gou, Y. Zhan, J. Lee, X. Li, Z. R. Lu, H. M. Zhou, H. Lu, X. Y. Wang, Y.
D. Park and J. M. Yang, Appl. Biochem. Biotechnol., 2015, 175, 2232–2245 CrossRef CAS PubMed.
- H. Xia, H. Tang, F. Wang, X. Yang, Z. Wang, H. Liu, D. Pan, C. Yang, S. Wang and G. Sun, Food Res. Int., 2019, 116, 20–29 CrossRef CAS PubMed.
- D. R. Rojas, R. Kuner and N. Agarwal, J. Mol. Med., 2019, 97, 845–854 CrossRef CAS PubMed.
- P. H. Dalton, D. D. Dilks and M. I. Banton, AIHAJ, 2000, 61, 340–350 CAS.
- I. K. Liyanage, M. R. Navinan, A. C. Pathirana, H. R. Herath, J. Yudhishdran, N. Fernandopulle and A. Kulatunga, J. Occup. Med. Toxicol., 2015, 10, 26 CrossRef PubMed.
- J. K. Sethi and A. J. Vidal-Puig, J. Lipid Res., 2007, 48, 1253–1262 CrossRef CAS PubMed.
Footnotes |
† Electronic supplementary information (ESI) available. See DOI: 10.1039/c9ra05569j |
‡ These authors contributed equally to this work. |
|
This journal is © The Royal Society of Chemistry 2019 |
Click here to see how this site uses Cookies. View our privacy policy here.