DOI:
10.1039/C9RA05060D
(Paper)
RSC Adv., 2019,
9, 26552-26558
Identification of donkey meat in foods using species-specific PCR combined with lateral flow immunoassay
Received
4th July 2019
, Accepted 19th August 2019
First published on 23rd August 2019
Abstract
Food authenticity is a global issue and has raised increasing concerns in the past decades. DNA-based methods are more favourable than the conventional protein-based techniques and have been applied to species identification and meat fraud detection. To effectively identify donkey meat for meat product authentication, a highly specific and robust method that coupled polymerase chain reaction (PCR) with lateral flow immunoassay (LFI) was developed. Donkey-specific PCR primers were designed by targeting at the mitochondrial D-loop gene and the specificity was verified in silico and in vitro against 22 species involved in meat authentication. A limit of detection of 0.0013 ng μL−1 DNA extract was achieved and as low as 0.001% w/w (raw) and 0.01% w/w (cooked) donkey meat in beef were successfully detected using the developed PCR-LFI. LFI strip-based visualization of PCR products allowed for a 10-fold higher sensitivity than conventional gel electrophoresis and significantly reduced the analysis time for the post-PCR analysis. This PCR-LFI is highly suitable for rapid identification of donkey or incorporating into multiplex screening protocol for other meat authentication in the laboratories of both regulatory agencies and commercial services.
1. Introduction
The issue of food authenticity has a long history and has raised increasing concerns internationally in the past decades.1 Generally being considered as the intentional or unintentional misrepresentation or adulteration of food products,2 food fraud can cause adverse health effects on consumers, including nutrition defects, food allergy, poisoning, and even lethal symptoms.1 Food fraud costs the global food industry $10 to $15 billion annually2 and compromises the public trust in government agencies and the food industry as a whole.3 One of the biggest food fraud outrages in the recent years was from the meat industry – the 2013's horse meat scandal in Europe that was featured in the headlines of most news media. Horse meat was identified as high as 60–100% in the tested beef products4 and the adulterants could be extended to donkey meat.5 Not only culturally and legally unaccepted by many English-speaking countries, it is also a religious taboo in Kosher and Islamic cultures to slaughter horses or donkeys for consumption.6 On the contrary, donkey meat is regarded as a delicacy in a few countries (e.g., Italy, China) and donkey skins are exceedingly demanded by Asian market for producing Chinese traditional supplement (e.g., Colla Corii Asini), leading to the necessity of testing their integrity and authenticity for the manufacturers, retailers, and end-users.7 Due to the unusually high demand as aforementioned, some African countries require technical measures to identify donkey components for controlling illegal exportation8 and high incidence of donkey-involved meat adulteration.9 Therefore, highly accurate analytical methods are needed for the identification of donkey species from meat and processed meat products.
Various modern technologies have been developed and applied to food authentication with customization, including spectroscopic, chromatographic, DNA-based, immunoanalytical techniques.10 Each technique has its own merits and drawbacks, depending on the particular analytical objective (e.g., geographical origins and substitution) and food commodities (e.g., dairy, juice). For the species identification in meat authentication, favourable analytical methods are typically based upon the detection of either protein/peptide biomarkers or unique conservative DNA sequences.11
Traditionally, detection of protein had been the most suitable method to determine animal species,12 and enzyme-linked immunosorbent assay (ELISA) was a very popular tool in food industry for being easy to perform, robust, and low-cost.13 However, the best compromise between false positive and false negative rates had to be determined in each laboratory according to the particular objective (e.g., for religious certification requiring lower false negative rate) and the performance of ELISA kits.13 Chromatographic methods are typically coupled with mass spectrometry to build a data library for protein profiling of each species,14 including capillary electrophoresis.15 Moreover, the emerging mass spectrometry-based proteomics has been applied to meat authentication, targeting species-specific peptide biomarkers.16,17 The primary structure of proteins is reasonably stable against processing, which allows one to seek peptide biomarkers that are of less risk in fragmentation than the risk of DNA degradation during amplification.16 Nevertheless, tedious sample preparation, massive data generated, and the high cost in both equipment investment and personnel training limit the expansion of mass spectrometry-based methods to a great extent. Besides, another group of techniques being able to differentiate species in meat mixtures are based upon the signals generated corresponding to the chemical structure of the samples, including visible and near-infrared spectroscopy,18 mid-infrared spectroscopy,19 and nuclear magnetic resonance spectroscopy.20 However, relatively complex chemometric models need to be developed to interpret the spectra and make useful prediction.
Not like proteins and peptides that are often denatured or degraded during food processing (e.g., heating), DNA is more stable under harsh physical and chemical environment, such as high temperature and extreme acidity.21 In general, DNA-based techniques are more favourable and more often practised in real-world applications towards meat species identification. Fundamentally, in each method, polymerase chain reaction (PCR) or one variation thereof is used to exponentially amplify particular DNA segments and subsequently coupled with a detecting or tracing technique to determine the existence of the DNA target. For meat authentication, DNA-based methods have been extensively studied, including but not limited to PCR-restriction fragment length polymorphism (RFLP),22 quantitative PCR (qPCR, or known as real-time PCR),23 multiplex PCR,24 random amplified polymorphic DNA (RAPD)-PCR,25 (semi-)nested PCR,26 and PCR-DNA sequencing.9
Several attempts have been reported to identify donkey meat among other food matrices. A 10-base primer has been used in a PCR-RAPD method to differentiate the meat of 11 common food animals and high frequent adulterants, including donkey meat.27 Although distinguishable DNA fragment patterns were generated for each species, the performance in analyzing binary mixtures varied unsatisfactorily.27 A PCR-RFLP method was also reported to identify meat from cattle, sheep, swine, chicken, and donkey & horse using five pairs of primers.28 However, the DNA of horse and donkey could only be differentiated by the enzyme restriction step.28 Furthermore, a species-specific PCR method was reported to identify pork, horse meat, and donkey meat, achieving a limit of detection (LOD) as low as 0.1% w/w donkey meat in beef.29 Besides, real-time PCR,23 multiplex PCR,30,31 PCR-DNA pyrosequencing,32 DNA microarray33 and others have also been reported for species-specific detection of donkey meat. Nevertheless, their methods bear some limitations, such as a limited number of species for specificity test,29 relatively labour intensive bench work (e.g., electrophoresis),28 and the use of expensive equipment.30–32 Thus, a highly specific, robust, cost-effective, time-saving and user-friendly method is required for the identification of donkey meat by food industry and regulatory agencies.
In this study, a species-specific PCR coupled with lateral flow immunoassay method was developed and validated. We designed a pair of highly specific primers targeting the non-coding mitochondrial D-loop region of donkey origin, considering many advantages of mitochondrion DNA, such as higher abundance than the nuclear genome and more sequence diversity among genetically closed species. The gold nanoparticle-labelled antibody was used to recognize the PCR products to generate red bands visible to naked eyes for rapid visualization. The high specificity (against 22 other species) and satisfying detection sensitivity in various raw and cooked products were achieved, leading to its adoption in the routine analysis in government laboratories.
2. Material and methods
2.1. Collection of meat samples
Authentic meat samples were collected as follows. Donkey meat was purchased directly from a local farm in Tianjin. Meat cuts from whole carcasses were morphologically identified and then purchased from local butcher shops, including beef, pork, lamb, deer meat, and horse meat. Chicken, duck, goose, quail, and rabbit were purchased as whole carcasses from local groceries. Camel meat and buffalo meat were purchased from verified suppliers in Inner Mongolia and Jiangxi Province, both morphologically checked. Muscle samples of dog, cat, mouse and rat were collected from the carcasses provided by Tianjin University of Traditional Chinese Medicine. Meat samples of mink and fox were provided by Tianjin Animal Husbandry and Veterinary Research Institute. Four vegetables commonly found in processed donkey meat products (i.e., carrot, cabbage, green onion, pepper) were also collected. All the samples were kept under 4 °C during transportation and then stored at −20 °C until further processing and DNA extraction.
2.2. Sample preparation
The muscle part was separated from each meat sample, placed into a new disposable blender container to avoid cross-contamination, and then homogenized thoroughly. Binary mixtures of donkey meat in beef (i.e., 100%, 50%, 25%, 10%, 5%, 1%, 0.1%, 0.01%, and 0.001%, w/w) were prepared by mixing the homogenized pure beef and donkey meat, considering that beef had been the main target of adulteration using donkey meat. To further confirm the robustness of the method, binary mixtures were deep fried for 5 min, water-boiled for 30 min, or autoclaved under 103.4 kPa at 121 °C for 20 min, separately. Samples with each type of treatment were at least triplicated, followed by a thorough homogenization. The four vegetable samples were cut and blended into slurry as well.
2.3. DNA extraction
Genomic DNA was extracted from each sample (i.e., pure meats, vegetables, and binary mixtures) using a modified cetyltrimethylammonium bromide (CTAB) method. In brief, 500 mg of each homogenized sample was added into 3 mL of Nuclei Lysis Solution (Promega Corporation, Madison, USA), followed by adding 35 μL of 20 mg mL−1 Proteinase K (Promega Corporation, Madison, USA). The mixture was then incubated at 65 °C for 60 min with inversion for a few times every 15 min, followed by centrifugation at 8000 × g for 10 min. After that, 600 μL of supernatant was transferred to a new 1.5 mL microcentrifuge tube, and 600 μL of phenol
:
chloroform
:
isoamyl alcohol (25
:
24
:
1, v/v) was quickly added with vigorous shaking, followed by centrifugation at 12
000 × g for 10 min. The upper layer (600 μL, aqueous) was transferred to a new tube for another round of phenol–chloroform–isoamyl alcohol wash and centrifugation. Then, 300 μL of the aqueous phase was transferred to a 1.5 mL tube, and 750 μL of pre-cooled absolute ethanol was added with a few times of gentle inversion. After resting at room temperature for 10 min, the tube was centrifuged at 12
000 × g for 20 min. The precipitated DNA pellet was washed with cold 70% ethanol and re-dissolved in 50 μL of distilled water. The purity and yield of the extracted DNA were determined by using a UV mini-1240 spectrophotometer (Shimadzu Corporation, Kyoto, Japan).
2.4. Primer design and modification
A pair of donkey-specific primers was designed by targeting at mitochondria D-loop sequences. The conservative regions were recognized based upon eight donkey (Equus asinus) sequences (i.e., X97337.1, NC_001788.1, KT182635, KM881681, KM234997, KM234999, KM234998, and AF220938) from the Genbank database. A highly specific region of 287 bp was identified via alignment analysis of the mitochondria D-loop region of donkey along with that of the 18 animal species as aforementioned, using “Pairwise/Multiple align” function of Geneious 6.0 software (Biomatters Ltd., New Zealand). The specificity of the designed primers was confirmed by performing an in silico analysis using the Primer-BLAST tool (https://www.ncbi.nlm.nih.gov/tools/primer-blast/). Primers were also examined for their GC content, primer-dimer and hairpin formation using the “Primer Design” function of the Geneious software. The designed primers were synthesized by Sangon Biotech Co. Ltd. (Shanghai, China), 5′-end modified with fluorescein isothiocyanate (FITC) for the forward sequence (5′-FITC-CCATATCAGCTCAACATACAATACTC-3′) and with biotin for the reverse sequence (5′-biotin-CCGTAGAAACCCCCACGTTTAG-3′).
2.5. PCR amplification of the extracted DNA
PCR amplification was performed in a 20 μL reaction system, containing 1 μL (20 pg to 100 ng) of template DNA, 2 μL of 10 × PCR buffer (TaKaRa Bio Inc., Shiga, Japan), 2 μL of 10 mM dNTP mix (TaKaRa Bio Inc., Shiga, Japan), 0.8 μL of each primer (5 μM) and 1 U of HS Ex Taq DNA polymerase (TaKaRa Bio Inc., Shiga, Japan). Using a PTC-200 thermal cycler (MJ Research, Quebec, Canada), the following program was executed for amplification: an initial denaturation at 94 °C for 5 min; 30 cycles of denaturation at 94 °C for 30 s, annealing at 56 °C for 30 s and elongation at 72 °C for 30 s; and a final extension at 72 °C for 5 min. The PCR products were characterized by agarose gel electrophoresis using Gel Doc XR system (Bio-Rad Laboratories, California, USA) and by sequencing from commercial service (Sangon Biotech Co. Ltd, Shanghai, China).
2.6. Lateral flow immunoassay
The lateral flow immunoassay was fabricated in a classic design (Fig. 1). Gold nanoparticle conjugated anti-FITC mouse monoclonal antibody was deposited on the conjugated pad for recognizing PCR products. Streptavidin and goat anti-mouse polyclonal antibody were deposited on the cellulose membrane as the test line and control line, respectively. In a typical test, 5 μL of PCR product was transferred on the sample pad and then the strip was dipped into about 100 μL of assay buffer (10 mM Tris–HCl, pH 7.2, 1% BSA, and 1% Tween-20) for 3–5 min. As the liquid moved along the strips, the FITC-labelled DNA was bound to the gold-labelled anti-FITC antibody and then captured by streptavidin (test line) and the secondary antibody (control line), forming two red lines. If no amplified DNA was present in the PCR product, gold-labelled antibody bound to FITC-labelled primer would be captured by the secondary antibody (control line) but not streptavidin (test line).
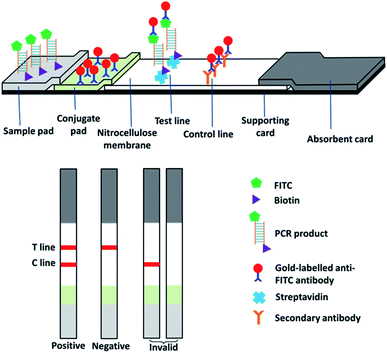 |
| Fig. 1 Scheme of PCR-coupled lateral flow immunoassay. | |
2.7. Tests for specificity, sensitivity, and robustness
The developed species-specific PCR-LFI method was tested for the specificity against individual samples of 18 animal species and 4 vegetables. A series of 5-fold sequential dilutions of DNA extract (i.e., 100, 20, 4, 0.8, 0.16, 0.032, 0.0064, 0.0013, 0.00025, 0.00005 and 0.00001 ng μL−1) from donkey meat were tested to determine the sensitivity of the method. Binary mixtures of donkey meat and beef, raw or processed in three different ways (Section 2.2), were tested using the developed method. All tests were conducted for at least three times.
2.8. Test of commercial products for meat authentication
The developed PCR-LFI method was adopted in the routine analysis at government laboratories of Tianjin and the following meat products on the market were tested: frozen pork dumplings (uncooked), frozen beef dumplings (uncooked), frozen lamb dumplings (uncooked), duck roll (cooked), frozen pork skewers (uncooked), beef in brown sauce (cooked), lamb skewers (cooked), beef jerky (vacuum sealed), beef jerky cubes (vacuum sealed), and donkey burger. All products were tested following the procedures as aforementioned with slightly modification. For example, only the filling of dumplings was collected for test, and the sauce of beef in brown sauce was washed off to reduce the possible interference. To further reduce the variation among different laboratories and for administration ease, anti-FITC universal lateral flow dipstick (Ustar Biotechnologies, Hangzhou, China) and LFI assay buffer (BGI Beijing Genomics Institute) were used for analysing commercial products.
3. Result and discussion
3.1. Specificity
As confirmed by the in silico analysis via the Primer-BLAST tool, the designed primers only amplify a 287-bp sequence from the mitochondrial DNA of a donkey with no cross-reactivity to the other 22 species. This was verified by the sequencing data of the PCR product from the commercial service where the primers were synthesized (data not shown). At the same time, agarose gel electrophoresis was conducted to visualize the specificity against the other 22 species (Fig. 2A), followed by the actual tests using the lateral flow immunoassay (Fig. 2B). Only the PCR product from donkey meat generated the signals as the 287-bp band (i.e., first sample beside the DNA ladder “M”) while all the other 22 species and the negative control (i.e., “NC” on the very right) had no band (Fig. 2A). Similarly, only the PCR product from donkey meat generated signals on both the test and control lines while all the other 22 species and the negative control led to the presence of solely control line (Fig. 2B). Thus, the results from all prediction and experiments demonstrate the high specificity of the designed primer against the common meats (beef, pork, etc.) and vegetables (carrot, green pepper, etc.) as well as some meats of uncommon animal found in previous records as adulterants (fox, mouse, cat, etc.) involved in the meat authentic issue related to donkey meat. Compared to the scope of the previous studies in which mainly focused on major meats available in common grocery stores in developed countries,6,7,23,27,28,31,33 our current study also included many species that are poorly monitored but do exist in meat products produced in Africa9 and Asia (internal data). This provides a more powerful tool for regulatory agencies with high confidence in reducing the false positive issue during the routine tests.
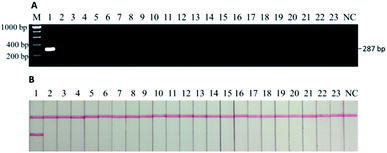 |
| Fig. 2 (A) Electrophoretic gel of PCR products and (B) lateral flow immunoassay results of specificity tests. M: DNA ladder, 1: donkey, 2–23: chicken, duck, goose, quail, cat, dog, rat, mouse, pig, horse, cattle, buffalo, sheep, deer, camel, rabbit, mink, fox, carrot, cabbage, green onion, and pepper, NC: negative control. Only donkey sample generates the band at 287 bp as well as both test and control lines in LFI. | |
3.2. Sensitivity
The tests for sensitivity and associated robustness were carried out in two stages (Section 3.2 and 3.3). The first stage was to determine the limit of detection of PCR-LFI using DNA extract of pure donkey meat. DNA extract was sequentially diluted (i.e., 100, 20, 4, 0.8, 0.16, 0.032, 0.0064, 0.0013, 0.00025, 0.00005, and 0.00001 ng μL−1) and then PCR amplified, and both gel electrophoresis and LFI test were separately conducted. While gel electrophoresis revealed PCR product at the initial concentration between 0.0064 and 0.0013 ng μL−1 (Fig. 3A), the PCR-LFI method clearly showed a better sensitivity below 0.0013 ng μL−1 (Fig. 3B). This LOD was comparable with or better than 0.01 ng,29 0.005–0.05 ng,31 0.001 ng (ref. 23) reported in previous studies involving PCR-based methods. Though a better LOD of 0.00034 ng was achieved with the multiplex-PCR technique,30 an expensive genetic analyzer and internal standards were required for post-PCR analysis in which LFI had huge advantages due to the simplicity and extremely low cost.
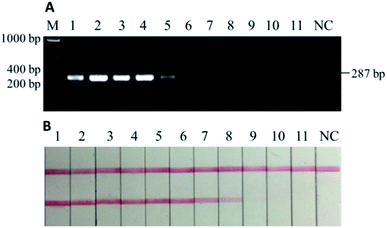 |
| Fig. 3 (A) Electrophoretic gel of PCR products and (B) lateral flow immunoassay results of sensitivity tests using DNA extracts from pure donkey meat. M: DNA ladder, 1–11: 100, 20, 4, 0.8, 0.16, 0.032, 0.0064, 0.0013, 0.00025, 0.00005, and 0.00001 ng μL−1, NC: negative control. The LOD of PCR-LFI equals to 0.0013 ng μL−1 or less (B: sample 8). | |
3.3. Robustness in testing binary mixtures with heat processing
To further test the robustness, or the second stage of sensitivity, beef and donkey meat mixtures with four different states (i.e., raw, 5 min of deep frying, 30 min of water-boiling, and 20 min autoclaving under 103.4 kPa at 121 °C) were tested. The weight percentage of “adulterated” donkey meat in beef were 100%, 50%, 25%, 10%, 5%, 1%, 0.1%, 0.01%, and 0.001%. In the raw meat mixture, as low as 0.001% w/w donkey meat could be detected in beef by using PCR-LFI (Fig. 4B).
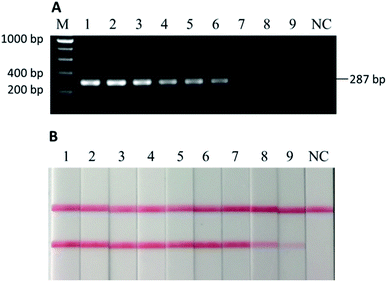 |
| Fig. 4 (A) Electrophoretic gel of PCR products and (B) lateral flow immunoassay results of binary mixtures of donkey meat in beef, raw. M: DNA ladder, 1–9: 100%, 50%, 25%, 10%, 5%, 1%, 0.1%, 0.01%, and 0.001% of donkey meat in beef, NC: negative control. As low as 0.001% of donkey meat could be detected (B: sample 9). | |
However, the performance was weakened to various extents for the heat-processed samples. The lowest amount of donkey meat that could be detected in beef was 0.01%, 0.01%, and 1% (w/w), for 5 min of deep frying, 30 min of water-boiling, and 20 min of autoclaving under 103.4 kPa at 121 °C, respectively (Fig. 5B, D and F). The LODs for deep fried and water-boiled samples were better than the 0.1% (w/w) reported in a previous study using the same binary mixture model,29 and the LOD for autoclaved samples was comparable with the 1–5% (w/w) with a donkey/horse model.34 The internal temperature of meat during typical home-cooking, mimicked by deep frying and water-boiling, is between 60–80 °C (ref. 35) and occasionally reaches the boiling point of water or higher. This temperature range falls in the working condition of PCR thermocycles where DNA is mainly denatured rather than degraded, which may explain the slight impairment of the sensitivity for deep fried and water-boiled samples (i.e., from 0.001% to 0.01%). On the other hand, the laboratory autoclaving condition raises the internal temperature to a sufficiently high level at which the targeted DNA may degrade massively. The comparison with the parallel gel electrophoresis revealed >10 times better sensitivity by using LFI for the detection in home cooking conditions (Fig. 5A–D). Note that a sufficient homogenization is critical and good practice for testing samples with very low content of donkey meat in order to reduce false negatives. Overall, the developed PCR-LFI method is highly robust in testing processed meat for the identification of donkey species, exempting the long time in gel electrophoresis as the typical visualization technique of the PCR products.
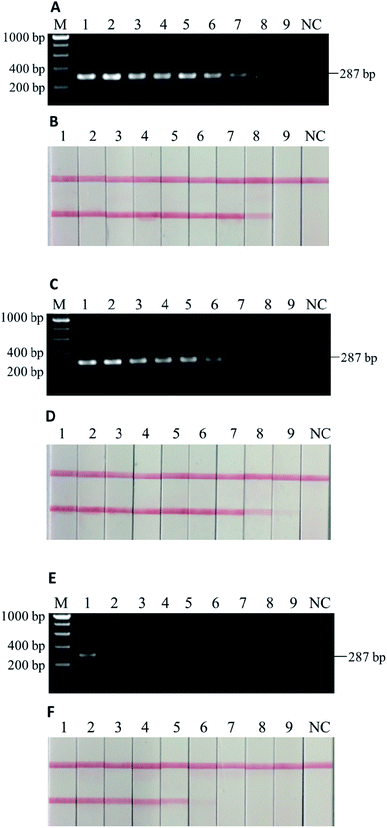 |
| Fig. 5 Electrophoretic gel of PCR products and lateral flow immunoassay results of binary mixtures of donkey meat in beef, with (A & B) 5 min of deep frying, (C & D) 30 min of water-boiling, and (E & F) 20 min of autoclaving under 103.4 kPa at 121 °C. M: DNA ladder, 1–9: 100%, 50%, 25%, 10%, 5%, 1%, 0.1%, 0.01%, and 0.001% (w/w) of donkey meat in beef, NC: negative control. As low as 0.01% (B: sample 8), 0.01% (D: sample 8), and 1–5% (F: sample 5 and 6) of donkey meat could be detected with heat processing. | |
3.4. Test of commercial products for meat authentication
As a part of food authentication surveillance, the result of tests of commercial meat products of various brands using the developed PCR-LFI method is summarized in Table 1. Each sample was tested for three times and the results of triplicates were consistent.
Table 1 Tests for donkey meat in commercial products using PCR-LFI method
Commercial food products |
Number of samples |
Number of positive |
Frozen pork dumplings (uncooked) |
5 |
0 |
Frozen beef dumplings (uncooked) |
5 |
0 |
Frozen lamb dumplings (uncooked) |
5 |
0 |
Duck roll (cooked) |
5 |
0 |
Frozen pork skewers (uncooked) |
5 |
0 |
Beef in brown sauce (cooked) |
5 |
0 |
Lamb skewers (cooked) |
5 |
0 |
Beef jerky (vacuum sealed) |
10 |
0 |
Beef jerky cubes (vacuum sealed) |
10 |
0 |
Donkey burger |
46 |
42 |
No donkey meat was identified as adulterant in any tested product except for donkey burger. However, 4 out of 46 donkey burger samples were found free of donkey meat. Different from beef burgers in which raw ground meat was used to cook and serve, donkey burgers are prepared by sandwiching chopped meat freshly cut from whole chunks of cooked donkey meat. This eliminated the possibility of the typical way of meat adulteration like in ground beef patty. All of the 4 negative samples were identified as horse meat using a pair of universal 16S ribosomal RNA gene primers for PCR followed by sequencing (data not shown), demonstrating the accuracy of the developed species-specific PCR-LFI method.
For practical application of rapid screening methods in the food industry and regulatory inspections, the average cost per test of food sample is one of the decisive factors in choosing appropriate assay and tools. For instance, though conventional qPCR allows direct reading without post-PCR visualization, about 10 times more expensive equipment, special dye/probes for visualization and high-quality consumable for reducing variations add up to the cost of ca. $8–10 per test. In comparison, the cost of developed species-specific PCR-LFI method (ca. $0.3–0.5 per test) is much more budget-friendly to both the regulatory agency and the low-profit food industry considering the massive number of samples.
4. Conclusions
A novel species-specific PCR-LFI was developed to identify donkey meat for meat authentication. The high specificity of this assay was confirmed by both in silico and in vitro tests against 22 other species, including common food-type meats (e.g., beef), accompanied vegetables (e.g., carrot, green pepper), and uncommon animal meats found in previous records as adulterants (horse meat, fox, mouse, cat, etc.). A LOD of 0.0013 ng μL−1 DNA extract was achieved using the developed PCR-LFI. This method also successfully detected as low as 0.001% w/w (raw) and 0.01% w/w (cooked) donkey meat in beef. Compared with gel electrophoresis, LFI strip-based visualization of PCR products allowed for a 10-fold higher sensitivity and significantly reduced the time required during post-PCR analysis. This PCR-LFI is highly suitable for rapid identification of donkey meat or incorporating into multiplex screening protocol for meat authentication in the laboratories of both regulatory agencies and commercial services.
Conflicts of interest
There are no conflicts to declare.
Acknowledgements
This work was supported to WZ by Tianjin Normal University (Project ZX0471800627) and to XL by Natural Sciences and Engineering Research Council of Canada (NSERC CRDPJ 486586-15).
References
- J. Spink and D. C. Moyer, J. Food Sci., 2011, 76, R157–R163, DOI:10.1111/j.1750-3841.2011.02417.x.
- The CFIA Chronicle, http://www.inspection.gc.ca/about-the-cfia/the-cfia-chronicle-fall-2017/food-fraud/eng/1508953954414/1508953954796, accessed May 20th, 2019.
- A. Krystallis, in Food Authentication: Management, Analysis and Regulation, ed. C. A. Georgiou and G. P. Danezis, John Wiley & Sons Ltd, Chichester, 2017, part b, ch. 3, pp. 25–82. DOI:10.1002/9781118810224.ch3.
- BBC News, https://www.bbc.com/news/uk-21375594, accessed May 16th, 2019.
- The Independent, https://www.independent.co.uk/news/uk/home-news/horsemeat-found-in-british-supermarkets-may-be-donkey-8489030.html, accessed May 15th, 2019.
- A. E.-A. A. Ahmed, E. El-Saied, A. H. Mahmoud, M. I. A. El-Hamied and A. M. Abd-Elmoteleb, Global Vet., 2016, 16, 126–132, DOI:10.5829/idosi.gv.2016.16.01.16316.
- P. Lv, Y. Zhao, F. Qi, X. Zhou, J. You, Y. Qin and Y. Zhang, J. Food Drug Anal., 2011, 19, 123–130 CAS.
- TRT World, https://www.trtworld.com/life/nigeria-bans-export-of-donkeys-as-chinese-demand-for-skins-lifts-prices-14115, accessed May 16th, 2019.
- D.-M. Cawthorn, H. A. Steinman and L. C. Hoffman, Food Control, 2013, 32, 440–449, DOI:10.1016/j.foodcont.2013.01.008.
- A. Schieber, in Modern Techniques for Food Authentication, ed. D.-W. Sun, Academic Press, London, 2nd edn, 2018, ch. 1, pp. 1–21. DOI:10.1016/b978-0-12-814264-6.00001-3.
- M. Alikord, H. Momtaz, J. keramat, M. Kadivar and A. H. Rad, J Food Meas Charact., 2018, 12, 145–155, DOI:10.1007/s11694-017-9625-z.
- N. Z. Ballin, Meat Sci., 2010, 86, 577–587, DOI:10.1016/j.meatsci.2010.06.001.
- I. Giovannacci, C. Guizard, M. Carlier, V. Duval, J.-L. Martin and C. Demeulemester, Int. J. Food Sci. Technol., 2004, 39, 863–867, DOI:10.1111/j.1365-2621.2004.00859.x.
- C.-C. Chou, S.-P. Lin, K.-M. Lee, C.-T. Hsu, T. W. Vickroy and J.-M. Zen, J. Chromatogr. B: Anal. Technol. Biomed. Life Sci., 2007, 846, 230–239, DOI:10.1016/j.jchromb.2006.09.006.
- B. Vallejo-Córdoba and M. Cota-Rivas, J. Capillary Electrophor., 1998, 5, 171–175 Search PubMed.
- C. von Bargen, J. Brockmeyer and H.-U. Humpf, J. Agric. Food Chem., 2014, 62, 9428–9435, DOI:10.1021/jf503468t.
- M. Montowska and E. Pospiech, Food Chem., 2013, 136, 1461–1469, DOI:10.1016/j.foodchem.2012.09.072.
- L. W. Mamani-Linares, C. Gallo and D. Alomar, Meat Sci., 2012, 90, 378–385, DOI:10.1016/j.meatsci.2011.08.002.
- O. G. Meza-Márquez, T. Gallardo-Velázquez and G. Osorio-Revilla, Meat Sci., 2010, 86, 511–519, DOI:10.1016/j.meatsci.2010.05.044.
- W. Jakes, A. Gerdova, M. Defernez, A. D. Watson, C. McCallum, E. Limer, I. J. Colquhoun, D. C. Williamson and E. K. Kemsley, Food Chem., 2015, 175, 1–9, DOI:10.1016/j.foodchem.2014.11.110.
- D. A. Jonas, I. Elmadfa, K. H. Engel, K. J. Heller, G. Kozianowski, A. König, D. Müller, J. F. Narbonne, W. Wackernagel and J. Kleiner, Ann. Nutr. Metab., 2001, 45, 235–254, DOI:10.1159/000046734.
- P. S. Girish, A. S. R. Anjaneyulu, K. N. Viswas, B. M. Shivakumar, M. Anand, M. Patel and B. Sharma, Meat Sci., 2005, 70, 107–112, DOI:10.1016/j.meatsci.2004.12.004.
- J. Chisholm, C. Conyers, C. Booth, W. Lawley and H. Hird, Meat Sci., 2005, 70, 727–732, DOI:10.1016/j.meatsci.2005.03.009.
- S. Ghovvati, M. R. Nassiri, S. Z. Mirhoseini, A. H. Moussavi and A. Javadmanesh, Food Control, 2009, 20, 696–699, DOI:10.1016/j.foodcont.2008.09.002.
- M. C. Koh, C. H. Lim, S. B. Chua, S. T. Chew and S. T. W. Phang, Meat Sci., 1998, 48, 275–285, DOI:10.1016/s0309-1740(97)00104-6.
- C. Zhang, Food Control, 2013, 31, 326–330, DOI:10.1016/j.foodcont.2012.11.002.
- A. Arslan, I. Ilhak, M. Calicioglu and M. Karahan, J. Muscle Foods, 2005, 16, 37–45, DOI:10.1111/j.1745-4573.2004.07504.x..
- A. Doosti, P. Ghasemi Dehkordi and E. Rahimi, J. Food Sci. Technol., 2014, 51, 148–152, DOI:10.1007/s13197-011-0456-3.
- Z. Kesmen, F. Sahin and H. Yetim, Meat Sci., 2007, 77, 649–653, DOI:10.1016/j.meatsci.2007.05.018.
- S. S. Tobe and A. M. T. Linacre, Electrophoresis, 2008, 29, 340–347, DOI:10.1002/elps.200700706.
- F. Parchami Nejad, F. Tafvizi, M. Tajabadi Ebrahimi and S. E. Hosseni, Eur. Food Res. Technol., 2014, 239, 533–541, DOI:10.1007/s00217-014-2249-1.
- Z. Hu, Z. Wang, S. Zhang, Y. Bian and C. Li, Forensic Sci. Int.: Genet., 2015, 5, e561–e563, DOI:10.1016/j.fsigss.2015.09.222.
- G. Cottenet, V. Sonnard, C. Blancpain, H. Z. Ho, H. L. Leong and P. F. Chuah, Food Control, 2016, 67, 135–143, DOI:10.1016/j.foodcont.2016.02.042.
- A. Chen, C. Wei, G. Chen, Y. Zhao and S. Yang, Int. J. Food Sci. Technol., 2015, 50, 834–839, DOI:10.1111/ijfs.12720.
- U.S. Department of Health & Human Services, https://www.foodsafety.gov/keep/charts/mintemp.html, accessed May 31st, 2019.
Footnote |
† These authors contribute equally to this work. |
|
This journal is © The Royal Society of Chemistry 2019 |