DOI:
10.1039/C9RA04841C
(Paper)
RSC Adv., 2019,
9, 27105-27116
Exploring the chemical space and the bioactivity profile of lactams: a chemoinformatic study†
Received
26th June 2019
, Accepted 17th August 2019
First published on 28th August 2019
Abstract
Lactams are a class of compounds important for drug design, due to their great variety of potential therapeutic applications, spanning cancer, diabetes, and infectious diseases. So far, the biological profile and chemical diversity of lactams have not been characterized in a systematic and detailed manner. In this work, we report the chemoinformatic analysis of beta-, gamma-, delta- and epsilon-lactams present in databases of approved drugs, natural products, and bioactive compounds from the large public database ChEMBL. We identified the main biological targets in which the lactams have been evaluated according to their chemical classification. We also identified the most frequent scaffolds and those that can be prioritized in chemical synthesis, since they are scaffolds with potential biological activity but with few reported analogs. Results of the biological and chemoinformatic analysis of lactams indicate that spiro- and bridged-lactams belong to classes with the lowest number of compounds and unique scaffolds, and some showing activity against specific targets. Information obtained from this analysis allows focusing the design of new chemical structures in less explored spaces and with increased possibilities of success.
Introduction
Lactams can be considered as some of the most significant heterocycles in medicinal chemistry and drug discovery.1–4 Their importance is not only related to being spread over a large variety of natural products and drugs, but also to their value as building blocks for the design and synthesis of novel amino acids, alkaloids or peptidomimetic compounds. Structurally, lactams are cyclic amides that can be classified according to the size of the ring. The most common lactams are four-membered (β-lactams, also known as 2-azetidinones, Fig. 1a),1,5–8 five-membered (γ-lactams, also known as 2-pyrrolidinones, Fig. 1b),2,9–11 six-membered (δ-lactams, also known as 2-piperidinones, Fig. 1c)3,12 and seven-membered rings (ε-lactams, also known as 2-azepanones, Fig. 1d).4,13–15 Besides their well-known antibiotic activity,16–18 lactams show a large spectra of biological outcomes, with potential therapeutic applications in different fields, spanning from cancer, diabetes and infectious diseases. In fact, a number of lactam-containing compounds are reported to act as HIV-1 integrase inhibitor,19,20 opioid receptor agonist,21,22 as well as antitumoral,23–25 anti-inflammatory,26,27 and antidepressant agents28–30 (Fig. 1).
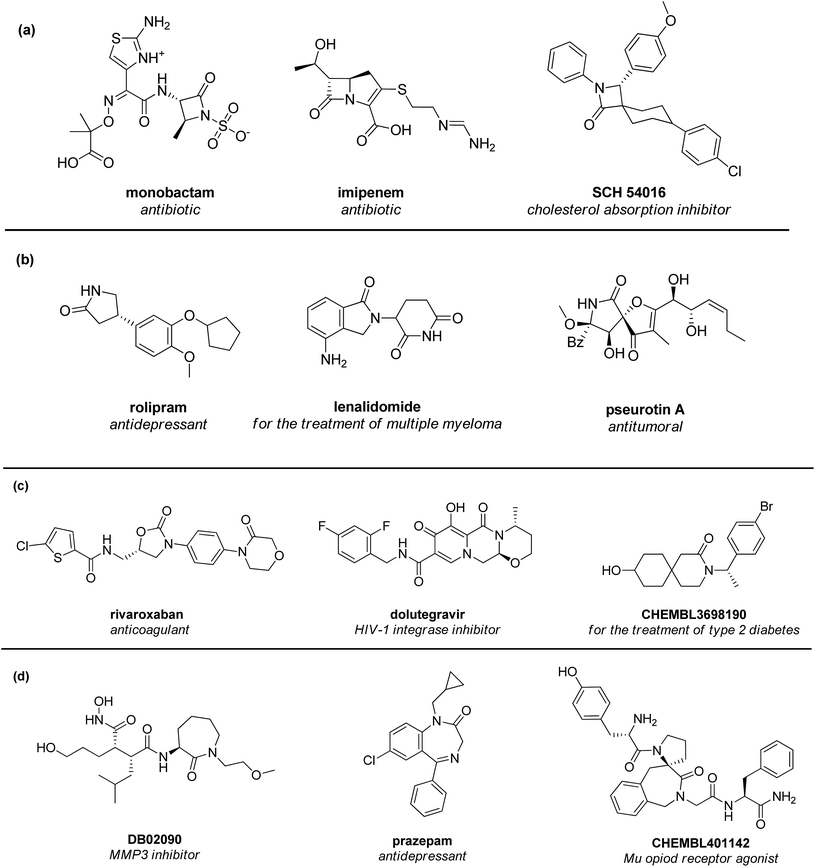 |
| Fig. 1 Selected examples of biologically active β- (a), γ- (b), δ- (c) and ε-lactam (d) containing molecules. | |
Not last in importance, lactams are also conformationally restricted scaffolds with peptidomimetic features, used to improve the potency, the selectivity, and the metabolic stability of peptide-based drugs.31 In particular, spiro-β-lactams have been used as β-turn mimetics in peptidomimetic chemistry, for studying the active conformation of peptides in biochemistry.32–34
Despite the relevance of lactams in drug discovery, the contents and diversity of these heterocycles in natural products, drugs and biologically active compounds has not been characterized so far. For this reason, the goal of this study was to identify, quantify, and classify lactam compounds that are present in compound data sets of broad interest in drug discovery, similarly to what we have previously reported for bicyclic acetals.35 To this end, we analyzed three major types of databases: approved drugs for clinical use, compounds with annotated biological activity available in a large public collection, and a comprehensive collection of natural products. The chemical diversity and coverage in chemical space of lactam compounds was analyzed based on physicochemical properties and molecular scaffolds. The lactam compounds annotated with biological activity were further analyzed according to their chemical classification and chemotype enrichment analysis to identify the most frequent scaffolds and those that have a promising biological activity profile but have not been largely explored so far.
Methods
All calculations reported in this work were done with KNIME36 and R.37
Compound data sets
The compound data sets used in this study are summarized in Table 1. The drugs approved for clinical use were retrieved from DrugBank;38 the compounds annotated with biological activity were obtained from ChEMBL v. 23,39 and the natural products were obtained from the Universal Natural Product Database (UNPD).40 Prior to the analysis, each molecule was prepared using the node Wash provided by function included in the program Molecular Operating Environment (MOE).41 This node disconnects salts of metals, removes simple components, and recalculates protonation states. With this program, inorganic compounds were eliminated, as well as duplicated compounds.
Table 1 Data sets analyzed in this work
Data base |
No. unique compounds |
Approved drugs |
1812 |
ChEMBL version 23 |
1 727 112 |
Universal natural product database (UNPD) |
109 579 |
Identification and classification of lactams
The identification and classification of the components of scaffolds with lactams was carried out using SMARTS, a useful language to describe molecular patterns and perform a search for substructures.42 Fig. 2 shows the substructures defined for the identification and classification of lactams in the approved drugs, UNPD, and ChEMBL databases. The full list of SMARTS can be found in the ESI.† The possible frameworks of lactams were defined and classified according to the size (number of atoms) of the lactam ring as β (four-membered lactam), γ (five-membered lactam), δ (six-membered lactam), and ε (seven-membered lactam). Each class was further classified into isolated, fused, spiro, and bridged lactams. The number of lactams present in each database was quantified.
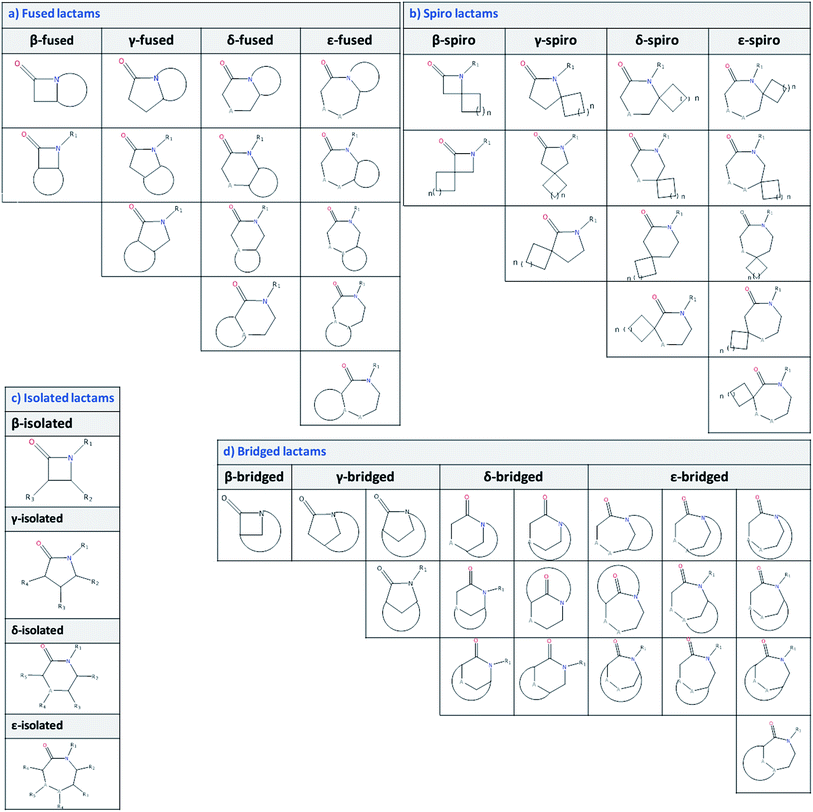 |
| Fig. 2 Substructures defined for the identification and classification of lactams in β-, γ-, δ- and ε-lactams, which are further classified as fused- (a), spiro- (b), isolated- (c) and bridged- (d) lactams. | |
Analysis of the chemical space
The chemical space of the lactams was analyzed by molecular properties. Principal component analysis (PCA)43 was used to generate a visual representation of the chemical space based on six molecular properties of pharmacological interest, namely; hydrogen bond donors (HBD), hydrogen bond acceptors (HBA), octanol/water partition coefficient (log
P), molecular weight (MW), number of rotatable bonds (RB), and topological polar surface area (TPSA). The molecular shape of the lactam compounds was evaluated using the Principal Moments of Inertia (PMI) graph.44
Calculation of the biological profile
Compounds in ChEMBL were associated with their bioactivity data. For this work, only compounds with activity reported as IC50 and percentage of inhibition were included. Those compounds with a pIC50 greater than 6 (<1000 nM) and percentage of inhibition greater than 60% were considered as active compounds.
Enrichment analysis
Based on the activity values reported for lactam compounds in ChEMBL, chemotype enrichment plots were constructed. A chemotype enrichment plot is a two-dimensional graph that shows the association between the enrichment factor (EF) of each chemotype vs. the frequency of that chemotype.45 The enrichment factor measures the proportion of active compounds for a given cyclic system or scaffold in a certain biological target and the frequency gives us an idea of the amount of analogues that there is for a particular scaffold. The chemotype enrichment plots are useful because they provide a general but quantitative information of the number of analogs reported for each scaffold, as well as their average activity with respect to the other scaffolds present. In addition, this plot allows for the selection of novel scaffolds where the information of their analogs is not yet explored.
To construct the chemotype-enrichment plot, the molecular scaffolds of each compound were calculated under the definition of Bemis and Murcko46 and the compounds were grouped by the targets they were active against. EF were calculated using the expression:
|
 | (1) |
where
|
 | (2) |
and
|
 | (3) |
where,
C is the total number of compounds tested,
C+ the number of compounds defined as active,
Cl the number of total compounds with a scaffold l tested, and
C+l the number of compounds with a scaffold l active against the target. Scaffolds with high frequency and an EF greater than 1 are of special interest in the drug design, since they have more structure–activity relationship (SAR) information and are enriched in active compounds for the targets in which they have tested.
Results and discussion
Initially, the chemical diversity and coverage in chemical space of lactam compounds was analyzed based on physicochemical properties and molecular scaffolds. Then, lactam compounds annotated with biological activity were further analyzed according to their chemical classification and chemotype enrichment analysis to identify the most frequent scaffolds and those that have a promising biological activity profile but have not been largely explored so far.
a) Identification and classification of lactams
Initially, the topological diversity of lactam compounds in the three different databases of general interest (Fig. 3) was analyzed. Specifically, the relative frequency of isolated-, bridged-, fused- and spiro-lactams in the databases of approved drugs, natural products, and ChEMBL are reported in Fig. 3. In total, 42
228 lactams were identified, of which the majority is present in ChEMBL, and for the three databases a larger fraction of fused lactams was found. For approved drugs there is a greater fraction of β-fused, while in ChEMBL and UNPD there are abundant γ-fused and δ-fused lactams, respectively. No bridged and spiro lactams were found in natural products or in approved drugs; in ChEMBL, bridged and spiro lactams have been poorly explored. As for the lactams defined as “isolated” (Fig. 2), the most explored have been the γ ones, and are those that are found more frequently in nature.
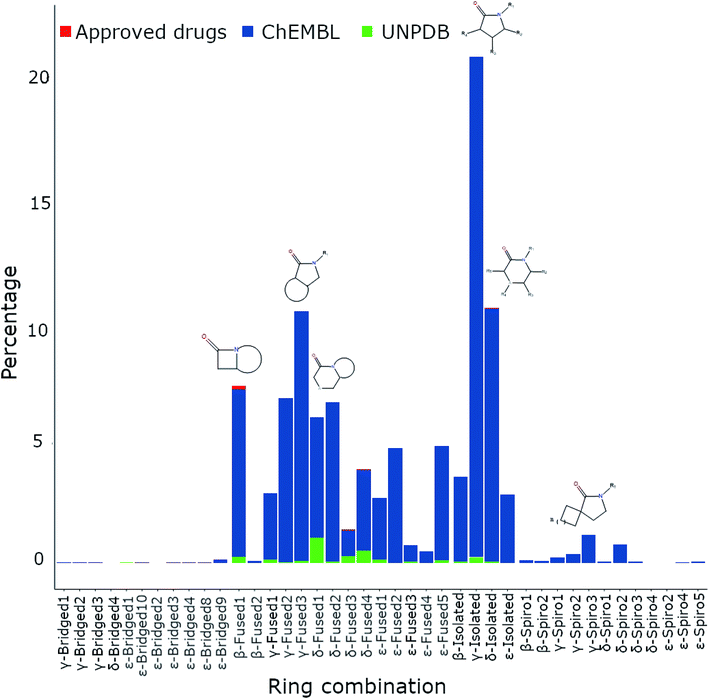 |
| Fig. 3 Percentage of β-, γ-, δ- and ε-lactams, which are further classified as isolated-, bridged-, fused-, and spiro-lactams in the databases of approved drugs, natural products, and ChEMBL. | |
b) Chemical space
The chemical space of lactams contained in three databases is shown in Fig. 4. The figure shows that ChEMBL (data points in blue) covers most of the chemical space and is also the database with the largest physicochemical and shape diversity. In contrast, natural products (data points in green) and approved drugs (data points in red) occupy a more focused region of the property space, which in turn is contained within the space of ChEMBL.
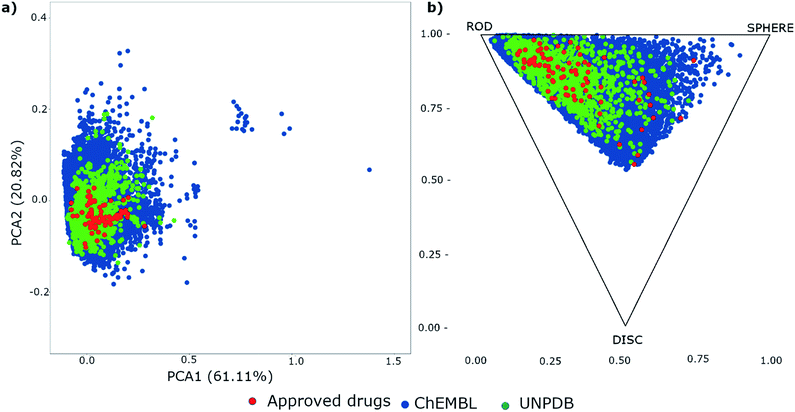 |
| Fig. 4 Visual representation of the chemical space: (a) principal component analysis of six physicochemical properties of pharmaceutical interest: molecular weight, hydrogen bond donors, hydrogen bond acceptors, the octanol and/or water partition coefficient, topological polar surface area, and number of rotatable bonds. (b) Principal Moment of Inertia (PMI) plot. The top left-hand corner of the PMI represents compounds with rod-like features (e.g., acetylene), the top right corner represents compounds with spherical features (e.g., adamantane), and the bottom corner represents compounds with disc-like features (e.g., benzene). | |
c) Biological activity profile
To identify the biological targets in which the lactams have been evaluated, compounds in ChEMBL were associated with their bioactivity data, as indicated in the Methods section. Table 2 shows the number of targets in which the lactams have been tested according to their chemical classification, the number of unique compounds, and scaffolds and the percentage of active compounds. From this classification and analysis of biological information of lactams it can be concluded that compounds with a γ-fused, γ-isolated, and δ-fused lactams have a more extensive activity profile compared to compounds with a spiro- or bridged-lactam that have been tested in a lower number of biological targets. Likewise, γ-fused, γ-isolated, and δ-fused have reported a larger number of active compounds, that is relevant, given the high number of compounds and unique scaffolds. Compounds as β- and ε-spiro, stand out for being the classes of lactams with a higher percentage of active compounds, and with the least amount of compounds and unique scaffolds.
Table 2 Summary of biological information of lactams classified according to their chemical class
|
β-Fused |
β-Isolated |
β-Spiro |
γ-Fused |
γ-Isolated |
γ-Spiro |
δ-Fused |
δ-Isolated |
δ-Spiro |
ε-Fused |
ε-Spiro |
ε-Bridged |
No. human targets |
52 |
88 |
4 |
501 |
352 |
15 |
351 |
179 |
9 |
190 |
5 |
5 |
No. non-human targets |
94 |
52 |
2 |
172 |
184 |
11 |
153 |
71 |
8 |
76 |
2 |
6 |
% active compounds |
37.18 |
43.58 |
62.74 |
46.76 |
43.39 |
7.87 |
39.93 |
48.76 |
6.32 |
50.69 |
62.16 |
17.20 |
No. unique compounds |
627 |
892 |
41 |
4062 |
3297 |
198 |
2406 |
2042 |
255 |
1861 |
25 |
78 |
No. unique scaffolds |
280 |
376 |
19 |
1638 |
1658 |
138 |
1259 |
1031 |
206 |
926 |
12 |
58 |
Fig. 5 shows the biological targets in which the lactams show activity, the biological targets were grouped according to the type of target reported in ChEMBL. As can be seen, lactams show activity in a wide variety of both human and non-human targets, suggesting that lactams have a privileged character in the development of bioactive compounds.
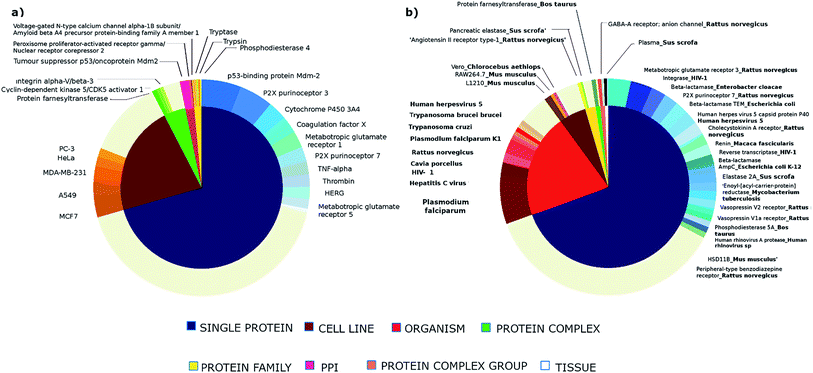 |
| Fig. 5 Pie charts summarizing the biological targets with the highest number of active lactam compounds. (a) Human targets, (b) non-human targets. The targets are grouped according to the type of target reported in ChEMBL. Compounds with a pIC50 greater than 6 (<1000 nM) and percentage of inhibition greater than 60% were considered as active compounds. | |
The human targets with the highest number of active lactam compounds are p53-binding protein Mdm-2, P2X purinoceptor 3 and 7, cytochrome P450 3A4 coagulation factor X, metabotropic glutamate receptor 1, TNF-alpha, thrombin and HERG. The non-human targets with the highest number of active lactam compounds are metabotropic glutamate receptor 3, P2X purinoceptor 7 and cholecystokinin a receptor of Rattus norvegicus, beta-lactamase of Enterobacter cloacae, Escherichia coli and Staphylococcus aureus. Others non-human targets are reverse transcriptase and integrase of the human immunodeficiency virus type 1 (HIV-1) and the human herpes virus 5 capsid protein P40 in the human herpesvirus 5. Also, lactams have been shown to be active against Plasmodium falciparum, hepatitis C virus, and Trypanosoma cruzi.
Structure multiple-activity relationships (SmART) of lactams. To analyze more in detail the biological profile of lactams, the results of the biological activity in human targets of sets of lactams with more than 50 compounds were clustered and analyzed, as shown in Fig. 6. The biological targets are grouped according to the type of target as reported in ChEMBL, and lactams are grouped according to their chemical classification (Fig. 2). From this visualization it is possible to identify biological characteristics according to the grouping of scaffolds. For example, given a class of compounds, the biological profile can be characterized according to the databases that provide both structural and biological characteristics of the compounds. Subsequently, this information could be used to predict the possible targets of a small molecule for the similarity with known bioactive compounds, or for the optimization of active compounds.
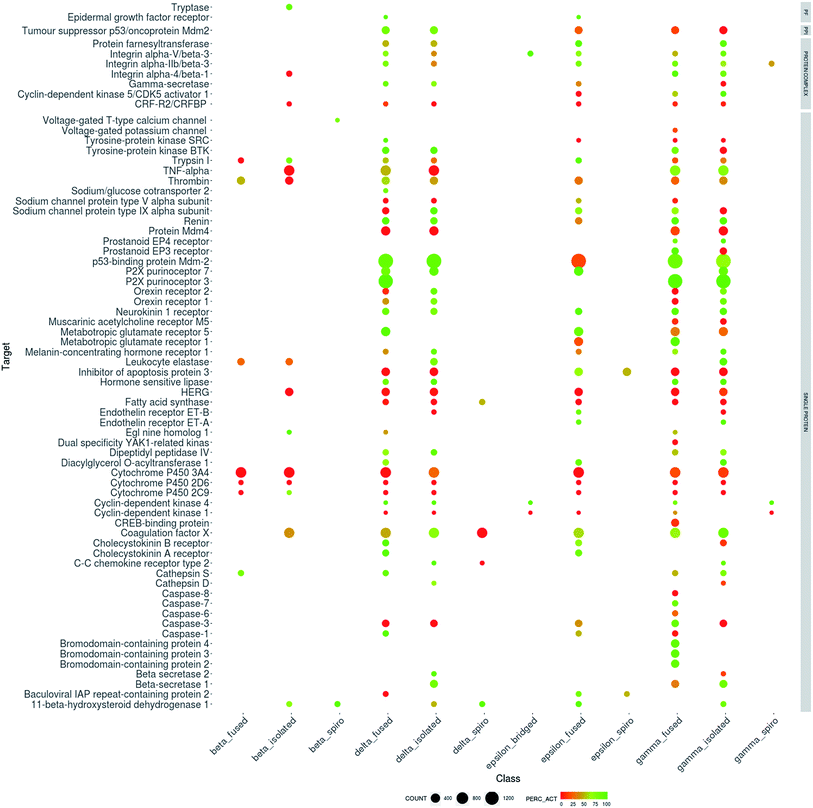 |
| Fig. 6 Biological profile of data sets of lactams with more than 50 compounds tested in human targets. The lactams are grouped according to their chemical classification (Fig. 2) and the targets are grouped according to their classification in ChEMBL. The size of each dot indicates the relative number of compounds. The colors of the data points denote the percentage of active compounds using a continuous scale (red, a lower percentage and green, a higher percentage). PF: protein family, PPI: protein–protein interaction. | |
It can be ascertained that for certain biological targets there is a greater diversity of classes of compounds that have been tested to. For example, δ-fused, δ-single, ε-fused, γ-fused and γ-single compounds have shown activity with the P2X purinoceptor 7. Another example is thrombin, in which β-fused, β-single, δ-fused, δ-single, γ-fused, γ-single and ε-fused compounds have been tested and where the majority of active compounds belong to the β-fused and δ-fused classes.
Nevertheless, some molecular targets in which only one specific class has been explored were also identified from this analysis. For example, for bromodomains 4, 3 and 2 only gamma-fused compounds have been tested shown promising activity, and examples of compounds tested against bromodomain 4 are shown in Fig. 7. In this example, the function of the appendices is highlighted, which can give rise to different hits from a similar scaffold. The compounds are grouped according to their chemical classification, in color the value of −log
IC50 is indicated (in green the least active and blue the most active compounds). Molecule pairs that show a very large and unexpected change in activity despite having a rather similar structure are called activity cliffs.47 These pairs are particularly interesting since they point to specific and relevant structure–activity relationships helpful to design new structural motives with improved activities.
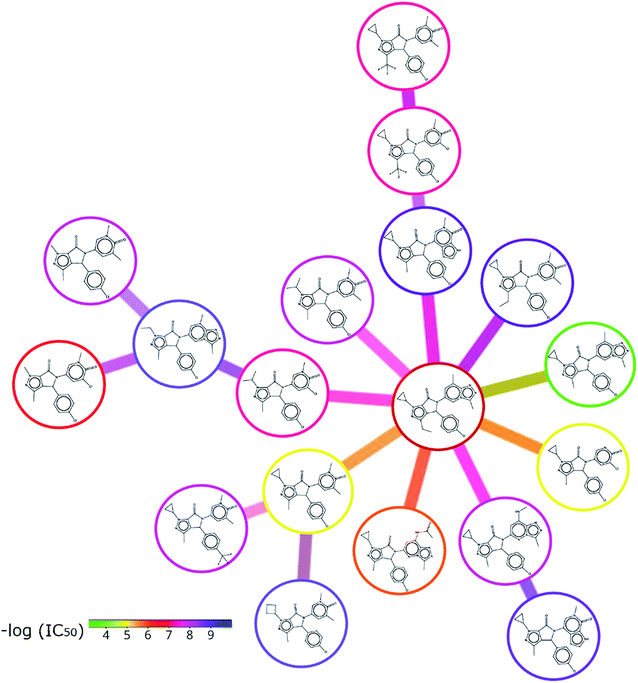 |
| Fig. 7 SAR of lactams tested against bromodomain 4. The compounds are grouped according to their chemical classification, and the value of −log IC50 is shown with colors (in green the least active and in blue the most active compounds). The most similar neighbors to consider for each molecule were calculated in DataWarrior by a rubberbanding forcefield approach.48 | |
d) Chemotype enrichment analysis
As an additional chemoinformatic tool to characterize the biological properties of lactam classes, the chemotype enrichment plot (chemotype frequency vs. EF) of lactams according to their chemical classification was developed, as shown in Fig. 8. This graphic allows the quickly identify of the scaffolds that have a high EF. In addition, with the information of the frequency is possible to identify those that have a larger number of analogues and those that have been little explored.
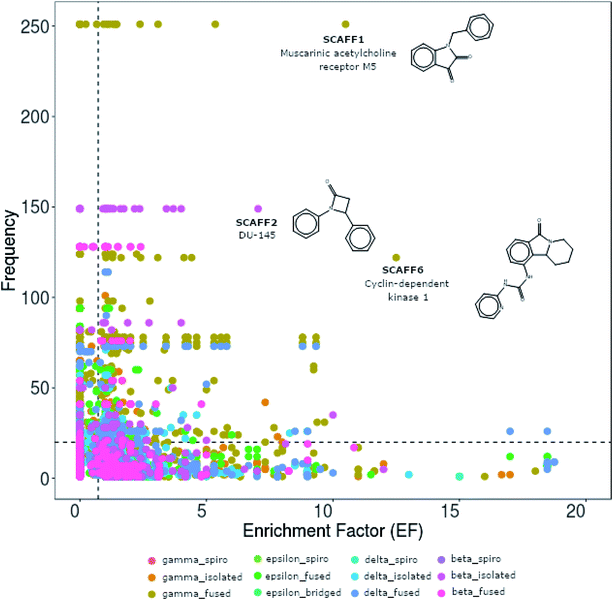 |
| Fig. 8 Chemotype enrichment plot. The abscissa indicate the enrichment factor (EF) as defined by eqn (1). Background activity is indicated by the vertical dashed line at EF = 1. The ordinate indicates the chemotype frequency in the database. Background frequency is indicated by the horizontal dashed line at frequency = 20. To facilitate the view, only scaffolds with EF up to 20 are displayed. Each of the data points in the figure corresponds to a chemotype that are further distinguished in color by class. The structure of the highlighted scaffolds can be seen in Fig. 9a. | |
In total, there are 131 scaffolds containing a lactam ring with a frequency greater than 20 and an EF greater than 1. Of these, 45 scaffolds contain a gamma-fused ring, 21 delta-fused, 18 epsilon-fused, 19 gamma-single, 16 delta-single, 10 beta-single, and 5 a beta-fused ring. Examples of these scaffolds are reported in Fig. 9a. For example, SCAFF1 and SCAFF2 have been among the most explored scaffolds, with 265 and 149 analogs, respectively, and have been shown to be active, mainly in cell lines. SCAFF3 (128 analogues) and SCAFF71 (41 analogues), both classified as beta-fused have been shown to be active against the beta lactamase of Bacillus licheniformis, Staphylococcus aureus and Escherichia coli. Scaffolds such as SCAFF25 and SCAFF53, although possessing low frequency, show selectivity against Plasmodium falciparum and the estrogen receptor alpha, respectively. In contrast, scaffolds such as SCAFF4 are active against bromodomains 2, 3 and 4.
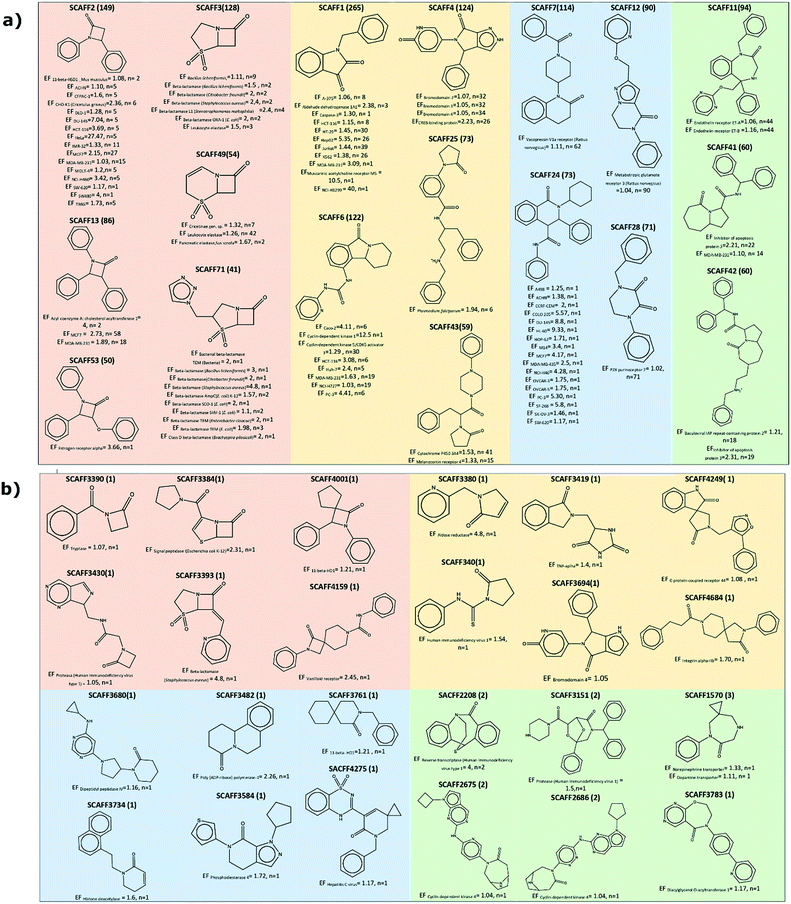 |
| Fig. 9 (a) Scaffolds most frequents with EF greater than 1. (b) Less frequent scaffolds with EF greater than 1. The scaffold ID is indicated and in brackets the frequency of that scaffold in the database. Enrichment factors (EF) are shown and the number (n) of total compounds with the scaffold that have been tested against the particular target. | |
On the other hand, 2934 scaffolds with an EF higher than 1 but with frequency equal or lower than 20 were found. Most of these scaffolds are gamma-fused and gamma-single with 652 and 492 compounds, respectively. A large amount of epsilon-fused (494), delta single (473) and delta fused (471) compounds were also found. Scaffolds of spiro compounds (β-, γ-, δ-, and ε-) and bridged-ε-lactams stand out since as mentioned above, they have been less explored, however, some of these scaffolds show activity against certain biological targets. For example, compounds with β-spiro scaffolds have shown activity for the Vallinoid receptor and only 11 scaffolds with less than 5 compounds each have been reported. Within the group of spiro lactams, δ-spiro and γ-spiro have been the most tested, however, only 17 δ-spiro and 10 γ-spiro scaffolds with EF greater than 1 have been identified. Potential targets for scaffolds δ-spiro are C–C Chemokine receptor −5, human immunodeficiency virus 1, Angiotensin II type 1a receptor, Hepatitis C virus and 11-β-HD1, and for the γ-spiro scaffolds G protein coupled receptor 44, Angiotensin II type 1a, Integrin II type 1a, Integrin alpha-11b/beta-3, cyclin dependent kinase 4, voltage-gated T type calcium channel and aldose reductase. For the ε-spiro class, only one active scaffold was identified against the norepinephrine transporter. Compounds with ε-bridged scaffolds have shown activity in targets such as cyclin-dependent kinase 4, human immunodeficiency virus type 1, and the protein complex integrin alpha-V/beta-3, for this class of compounds only 11 scaffolds have been identified, which also they have less than five analogues each. Examples of scaffolds with a smaller number of analogs reported but with activity against specific targets are described in Fig. 9b. The complete list of scaffolds can be found in the ESI.†
Conclusions
Lactams are a class of compounds important for drug design and can be found in chemical structures in different topologies (isolated-, bridged-, fused-, and spiro-). As shown in this work, this class of compounds is present in drugs approved for clinical use and in large collections of natural products (UNPDB) and bioactive compounds of pharmaceutical importance (ChEMBL). Although the use of lactams has been focused mainly on antibiotics, with this work it was possible to identify all the biological targets in which the lactams have been evaluated and reported in the public domain and those in which it has been given the highest priority. It was also possible to identify the most frequent scaffolds that contain a lactam ring and those that represent a promising opportunity in synthesis, since they are scaffolds with potential biological activity but with few analogues. In this view, the results of the biological and chemoinformatic analysis of the lactams indicate that the spiro- and bridged-lactams are the classes of lactams with the lowest number of compounds and unique scaffolds. It is expected to apply the results of this work to the design of new compounds. Also, as the public databases are updated, it is expected to follow up on the analysis of lactams in the drug design.
Conflicts of interest
The authors declare that they do not have any conflict of interest related to this manuscript.
Acknowledgements
This work was supported by the National Council of Science and Technology (CONACyT, Mexico) grant number 282785. FIS-G is thankful to CONACyT for the granted scholarship number 629458. AT and EL acknowledge MIUR (PRIN2015, cod. 20157WW5EH) for financial support.
References
- L. I. Llarrull, S. A. Testero, J. F. Fisher and S. Mobashery, Curr. Opin. Microbiol., 2010, 13, 551–557 CrossRef CAS PubMed.
- J. Caruano, G. G. Muccioli and R. Robiette, Org. Biomol. Chem., 2016, 14, 10134–10156 RSC.
- A. Lepikhina, O. Bakulina, D. Dar’in and M. Krasavin, RSC Adv., 2016, 6, 83808–83813 RSC.
- P. Gross and J. A. Zapp, CRC Crit. Rev. Toxicol., 1984, 13, 205–216 CrossRef CAS PubMed.
- J. Bhalla, S. S. Bari, A. Rathee, A. Kumar and A. Bhalla, J. Heterocycl. Chem., 2017, 54, 2297–2306 CrossRef CAS.
- S. Hosseyni and A. Jarrahpour, Org. Biomol. Chem., 2018, 16, 6840–6852 RSC.
- G. S. Singh and S. Sudheesh, ARKIVOC, 2014, 1, 337–385 Search PubMed.
- M. Alborz, A. Jarrahpour, R. Pournejati, H. R. Karbalaei-Heidari, V. Sinou, C. Latour, J. M. Brunel, H. Sharghi, M. Aberi, E. Turos and L. Wojtas, Eur. J. Med. Chem., 2018, 143, 283–291 CrossRef CAS PubMed.
- L.-W. Ye, C. Shu and F. Gagosz, Org. Biomol. Chem., 2014, 12, 1833–1845 RSC.
- S. Dong, M. Frings, D. Zhang, Q. Guo, C. G. Daniliuc, H. Cheng and C. Bolm, Chemistry, 2017, 23, 13888–13892 CrossRef CAS PubMed.
- T. Matsumoto, S. Nakamura, S. Nakashima, T. Ohta, M. Yano, J. Tsujihata, J. Tsukioka, K. Ogawa, M. Fukaya, M. Yoshikawa and H. Matsuda, J. Nat. Med., 2016, 70, 376–383 CrossRef CAS PubMed.
- J. Seibel, D. Brown, A. Amour, S. J. Macdonald, N. J. Oldham and C. J. Schofield, Bioorg. Med. Chem. Lett., 2003, 13, 387–389 CrossRef CAS.
- F. E. Dutton and B. H. Lee, Tetrahedron Lett., 1998, 39, 5313–5316 CrossRef CAS.
- C.-C. Wang and C.-M. Lee, J. Hazard. Mater., 2007, 145, 136–141 CrossRef CAS PubMed.
- X. Zhi, Y. Zhang, J. Huang and H. Xu, Sci. Rep., 2017, 7, 3917 CrossRef PubMed.
- M. Shahid, F. Sobia, A. Singh, A. Malik, H. M. Khan, D. Jonas and P. M. Hawkey, Crit. Rev. Microbiol., 2009, 35, 81–108 CrossRef CAS PubMed.
- K.-F. Kong, L. Schneper and K. Mathee, APMIS, 2010, 118, 1–36 CrossRef CAS PubMed.
- G. Kapoor, S. Saigal and A. Elongavan, J. Anaesthesiol., Clin. Pharmacol., 2017, 33, 300–305 CrossRef CAS.
- E. J. Velthuisen, B. A. Johns, D. P. Temelkoff, K. W. Brown and S. C. Danehower, Eur. J. Med. Chem., 2016, 117, 99–112 CrossRef CAS.
- M. Métifiot, K. Maddali, B. C. Johnson, S. Hare, S. J. Smith, X. Z. Zhao, C. Marchand, T. R. Burke Jr, S. H. Hughes, P. Cherepanov and Others, ACS Chem. Biol., 2012, 8, 209–217 CrossRef PubMed.
- R. D. Marco, R. De Marco, A. Bedini, S. Spampinato, L. Comellini, J. Zhao, R. Artali and L. Gentilucci, J. Med. Chem., 2018, 61, 5751–5757 CrossRef PubMed.
- S. M. Rawls, W. Robinson, S. Patel and A. Baron, Neuropharmacology, 2008, 55, 865–870 CrossRef CAS PubMed.
- M. Baiula, P. Galletti, G. Martelli, R. Soldati, L. Belvisi, M. Civera, S. D. Dattoli, S. M. Spampinato and D. Giacomini, J. Med. Chem., 2016, 59, 9721–9742 CrossRef CAS PubMed.
- D. Kuhn, C. Coates, K. Daniel, D. Chen, M. Bhuiyan, A. Kazi, E. Turos and Q. P. Dou, Front. Biosci., 2004, 9, 2605–2617 CrossRef.
- B. Xing, J. Rao and R. Liu, Mini-Rev. Med. Chem., 2008, 8, 455–471 CrossRef CAS.
- C. Saturnino, B. Fusco, P. Saturnino, G. D. E. Martino, F. Rocco and J.-C. Lancelot, Biol. Pharm. Bull., 2000, 23, 654–656 CrossRef CAS.
- J. Wei, X. Pan, Z. Pei, W. Wang, W. Qiu, Z. Shi and G. Xiao, J. Trauma Acute Care Surg., 2012, 73, 654–660 CrossRef CAS.
- D. J. Greenblatt and R. I. Shader, N. Engl. J. Med., 1978, 299, 1342–1344 CrossRef CAS.
- I. A. Volchegorskii and E. A. Trenina, Bull. Exp. Biol. Med., 2006, 142, 73–75 CrossRef CAS PubMed.
- K. Gillard, H. B. Miller and M. S. Blackledge, Chem. Biol. Drug Des., 2018, 92, 1822–1829 CrossRef CAS PubMed.
- E. M. Khalil, A. Pradhan, W. H. Ojala, W. B. Gleason, R. K. Mishra and R. L. Johnson, J. Med. Chem., 1999, 42, 2977–2987 CrossRef CAS PubMed.
- S. S. Bari and A. Bhalla, in Heterocyclic Scaffolds I: β-Lactams, ed. B. K. Banik, Springer Berlin Heidelberg, Berlin, Heidelberg, 2010, pp. 49–99 Search PubMed.
- H. Bittermann and P. Gmeiner, J. Org. Chem., 2006, 71, 97–102 CrossRef CAS PubMed.
- E. Alonso, F. López-Ortiz, C. del Pozo, E. Peralta, A. Macías and J. González, J. Org. Chem., 2001, 66, 6333–6338 CrossRef CAS PubMed.
- E. Lenci, G. Menchi, F. I. Saldívar-Gonzalez, J. L. Medina-Franco and A. Trabocchi, Org. Biomol. Chem., 2019, 17, 1037–1052 RSC.
- KNIME – Open for Innovation, https://www.knime.com/, accessed 23 May 2019 Search PubMed.
- B. D. Ripley, MSOR Connections. The newsletter of the LTSN Maths, Stats & OR Network, 2001, vol. 1, pp. 23–25 Search PubMed.
- D. S. Wishart, Y. D. Feunang, A. C. Guo, E. J. Lo, A. Marcu, J. R. Grant, T. Sajed, D. Johnson, C. Li, Z. Sayeeda, N. Assempour, I. Iynkkaran, Y. Liu, A. Maciejewski, N. Gale, A. Wilson, L. Chin, R. Cummings, D. Le, A. Pon, C. Knox and M. Wilson, Nucleic Acids Res., 2018, 46, D1074–D1082 CrossRef CAS.
- A. Gaulton, A. Hersey, M. Nowotka, A. P. Bento, J. Chambers, D. Mendez, P. Mutowo, F. Atkinson, L. J. Bellis, E. Cibrián-Uhalte, M. Davies, N. Dedman, A. Karlsson, M. P. Magariños, J. P. Overington, G. Papadatos, I. Smit and A. R. Leach, Nucleic Acids Res., 2017, 45, D945–D954 CrossRef CAS PubMed.
- J. Gu, Y. Gui, L. Chen, G. Yuan, H.-Z. Lu and X. Xu, PLoS One, 2013, 8, e62839 CrossRef CAS PubMed.
- Chemical Computing Group (CCG), Montreal, Canada, https://www.chemcomp.com/, accessed 23 May 2019.
- Daylight Theory: SMARTS – A Language for Describing Molecular Patterns, https://www.daylight.com/dayhtml/doc/theory/theory.smarts.html, accessed 23 May 2019.
- D. I. Osolodkin, E. V. Radchenko, A. A. Orlov, A. E. Voronkov, V. A. Palyulin and N. S. Zefirov, Expert Opin. Drug Discovery, 2015, 10, 959–973 CrossRef PubMed.
- W. H. B. Sauer and M. K. Schwarz, J. Chem. Inf. Comput. Sci., 2003, 43, 987–1003 CrossRef CAS PubMed.
- J. L. Medina-Franco, J. Petit and G. M. Maggiora, Chem. Biol. Drug Des., 2006, 67, 395–408 CrossRef CAS PubMed.
- G. W. Bemis and M. A. Murcko, J. Med. Chem., 1996, 39, 2887–2893 CrossRef CAS PubMed.
- J. Bajorath, Expert Opin. Drug Discovery, 2017, 12, 879–883 CrossRef PubMed.
- T. Sander, J. Freyss, M. von Korff and C. Rufener, J. Chem. Inf. Model., 2015, 55, 460–473 CrossRef CAS PubMed.
Footnote |
† Electronic supplementary information (ESI) available. See DOI: 10.1039/c9ra04841c |
|
This journal is © The Royal Society of Chemistry 2019 |
Click here to see how this site uses Cookies. View our privacy policy here.