DOI:
10.1039/C9RA03525G
(Paper)
RSC Adv., 2019,
9, 20796-20805
Exploring the pharmacological effects and potential targets of paeoniflorin on the endometriosis of cold coagulation and blood stasis model rats by ultra-performance liquid chromatography tandem mass spectrometry with a pattern recognition approach†
Received
10th May 2019
, Accepted 19th June 2019
First published on 3rd July 2019
Abstract
This study was employed to explore the potential biomarkers of endometriosis of cold coagulation and blood stasis (ECB) model rats and the effective mechanism of action of paeoniflorin (PF). The serum metabolomics approach was carried out using the UPLC-MS technique with a pattern recognition approach to prove the possible biomarkers of the ECB model rats and the perturbed pathways. Subsequently, the mechanism of PF treatment of this disease model was elucidated. The results revealed that the serum metabolism profiles in two groups were also separated significantly. Moreover, 8 biomarkers were found in the positive mode, and 5 biomarkers were found in the negative mode. Totally, 13 biomarkers participated in the metabolism of phenylalanine, arachidonic acid, etc. After treatment with PF, 10 biomarkers were regulated. Among the 10 biomarkers, 4 were statistically significant: L-phenylalanine, L-tryptophan, LysoPC (18:4(6Z,9Z,12Z,15Z)), and LysoPC (16:1(9Z)). We initially confirmed that PF could significantly regulate the metabolic expression of multiple metabolic pathways in the ECB model rats. For the first time, this study explored the mechanism of action of PF treatment based on the metabolic pathways of the organism and demonstrated the potential of the metabolomics techniques for the study of drug action mechanisms.
Introduction
Traditional Chinese Medicine (TCM) has been guided by its basic theories and has made significant contributions to the development of medicine and healthcare. In TCM theories, there are several different disharmony patterns (named ZHENG) such as the syndrome of blood stasis, syndrome of Qi stagnation, and Yin/Yang deficiency syndrome.1 As one of the syndromes of blood stasis, cold coagulation is caused by cold-evil. In traditional Chinese medicine theories, cold leads to blood clotting, and blood clotting leads to blood stasis; therefore, cold-evil easily leads to the syndrome of blood stasis,2,3 which has a serious influence on females during the menstrual period; moreover, it can cause various problems in women in the reproductive age. In addition, endometriosis is a grim challenge that may lead to female infertility. Generally, endometrium covers the luminal surface of the uterus. However, the endometrium can grow in other parts of the body due to certain factors, which can further develop into endometriosis. Thus, this disease is defined as the presence of endometrial stroma and glands;4 approximately 10–15 women of reproductive age per 100 people suffer from this disease.5–9 Pelvic pain, secondary dysmenorrhea and infertility10 are the main clinical manifestations of endometriosis. Regardless of any clinical manifestation, it is a severe threat to the physical and mental health of females of childbearing age. Therefore, the study of the etiology, pathogenesis, and treatment of endometriosis has become an important issue in the medical profession.
As the emerging “omic” technologies, metabolomics involves the integrated analysis of low-molecular-weight metabolites of a particular biological sample or an organism.11,12 The concept of metabolomics originated from the analysis of metabolic profiles proposed in the 1970s.13 With the development of genomics, transcriptomics and proteomics, Fiehn14 proposed the concept of metabolomics in 1997,15 and in 1999, Nicholson16 et al. also proposed the concept of metabolomics. The emergence of metabolomics provides many valuable research methods and strategies for the development of traditional Chinese medicine. The worth of metabolomics originates from the wide diversity of low-molecular-weight endogenous metabolites, which independently or in synergies provide characteristic profiles for different human diseases such as cardiovascular, neurological and inflammatory diseases;17 moreover, studies associated with metabolomics can be beneficial to strengthen our insights into disease mechanisms and drug effects, heightening our ability to forecast individual differences in drug reaction phenotypes.18,19 On the basis of previous studies and after years of constant exploration and research, in 2001, Wang20 proposed a novel strategy to address key points, for example, the property of syndromes, the evaluation of clinical efficacy of prescriptions, and the discovery of the basis of pharmacodynamics-chinmedomics (Chinese medicine metabolomics); the purpose of this study was to discover and determine the material basis for the efficacy of Chinese medicine and solve the quality problems, such as the effectiveness and safety of traditional Chinese medicines, related to the material basis of the efficacy.21,22 Therefore, high-throughput and high-resolution technologies, multivariate data analysis software and robust tools are necessary to support the large-scale data analysis. Currently, hyphenated techniques, such as the LC-MS (liquid chromatography-mass spectrometry) technology, are widely used for the determination and characterization of potential biomarkers in the metabolomics system owing to not only the high reproducibility and good linear range of the LC, but also the high definition of MS.23,24 Among these, ultra-performance liquid chromatography-tandem mass spectrometry (UPLC-MS) is one of the most commonly used approaches in assessing the efficacy of herbal medicine due to its high sensitivity and rapid analysis speed.25,26 However, the amount of raw data obtained through the UPLC-MS analysis is very large, and conventional statistical methods make it difficult to find differences among the samples or groups and the molecules that cause these differences. Thus, it is indispensable to apply special methods to analyze metabolomics data. Pattern recognition methods have been used to deal with this issue, which mainly include unsupervised methods and supervised methods. Principal components analysis (PCA) belongs to the former methods, whereas the orthogonal single correction partial least squares-discriminant analysis (OPLS-DA) and partial least squares-discriminant analysis (PLS-DA)27 belong to the latter methods, which are commonly used to filter out irrelevant information that interferes with metabolite analysis results during the metabolomics research.
The ingredients of traditional Chinese medicine are complicated, and the mechanism via which a substance exerts its efficacy remains unclear; Radix Paeoniae, the dried root of Paeonia lactiflora Pallas, is a Chinese herb. Paeoniflorin is an important active monomer of Radix Paeoniae. However, the pharmacological effect of paeoniflorin on ECB remains unclear. Therefore, in this study, ultra-performance liquid chromatography-electrospray ionization/quadrupole-time-of-flight high-definition mass spectrometry with the pattern recognition approach was used to characterize the metabolites present in the serum of ECB rats after oral administration of paeoniflorin, and the therapeutic mechanisms of paeoniflorin towards ECB were elucidated.
Methods and materials
Chemicals and reagents
Paeoniflorin was obtained from Chengdu PUSH Bio. Technology Co., Ltd (batch number PS160803-06). Estradiol benzoate injection was purchased from Animal Pharmaceutical Hangzhou, China (batch number 110202511). Acetonitrile (HPLC grade) was supplied by Fisher (USA). Methanol was obtained from Fisher (USA). Formic acid (HPLC grade) was purchased from Kermel (Tianjin, China). Leucine enkephalin was supplied by Sigma-Aldrich (MO, USA). Deionized water was supplied by Watson's Food & Beverage Co., Ltd. (Guangzhou, China). All chemicals and reagents were of analytical purity.
Animals and groups
Female Wistar rats, weighing 200–240 g, were obtained from the GLP Center of Heilongjiang University of Chinese Medicine. The room temperature was set at 24–26 °C with 40 ± 5% relative humidity under 12 h light/12 h dark cycle. Before the experiment, all rats in the metabolism cages were acclimatized for a week. During this period, rats were provided with water and normal food freely. We divided the rats into 5 groups stochastically: sham groups 1 and 2 (control group), ECB groups 1 and 2 (model group), and paeoniflorin group (therapy group), with 10 rats in each group. The experimental procedures were approved by the Animal Care and Ethics Committee at Heilongjiang University of Chinese Medicine, and all experiments were performed in accordance with the declaration of Helsinki.
Animal experiments and preparation of the PF oral solution
The method endometrial auto-transplantation combined with ice water immersion was applied to prepare the ECB model.28 The animal experimental procedures were executed during estrus. Moreover, the entire surgical procedure was executed under sterile environments. The rats in the five groups were anesthetized with 0.2 mL/100 g sodium pentobarbital, which placed in a supine posture and fastened them; then, routine disinfection of the skin was conducted using medical cotton dipped in an appropriate quantity of 10% povidone-iodine solution. To reach the abdominal cavity, 2–3 cm midabdominal incision was made. Then, by ligating the left uterine horn in both sides, about 1 cm tissue was cut and longitudinally opened. The sample freshly cut was put into a sterile container prefilled with 0.9% sodium chloride solution and cut into 2 pieces. The pieces were about 4 mm × 4 mm large. Moreover, two part organizations were respectively sutured into the uterine and abdominal wall of the same rat with USP 6/0 polyglactin. After 2 mL of 0.9% sodium chloride solution was administered into the abdominal cavity, the abdominal wall was sutured. Subsequently, the midline incision was closed by a 3/0 suture line. Following this, the rats were injected with 0.5 mL gentamicin each day by intraperitoneal injection for 3 consecutive days. Subsequently, 0.1 mL estradiol was administered to rats by subcutaneous injection once every 3 days, for 3 times totally. The control group rats were merely performed an abdominal incision without tissue transplantation. Except the sham group rats, other rats were immersed in 0–1 °C ice water for 8–10 min for about 14 consecutive days. Prior to this experiment, the rats were recovered for two weeks owing to the surgery.
After ice water immersion, the treatment group was intragastrically administrated with paeoniflorin of 27.6 mg kg−1 body weight. An aliquot of distilled water was given to all the rats except the paeoniflorin group. The administration process lasted for 28 days. The paeoniflorin standard powder was dissolved in distilled water and then ultrasonically mixed for 30 min to prepare an oral solution.
Calculation of the ectopic tissues volume
The rats were anesthetized with sodium pentobarbital (0.2 mL 100 g−1). The ectopic tissues were separated, and the length, width and height of the grafts were measured using the vernier caliper. The graft volume was calculated using the formula V (mm3) = 0.52 × A × B × C.
Hematoxylin–eosin staining (H&E staining)
The ectopic endometrium approximately 1 mm × 1 mm was removed and fixed in 10% formaldehyde immediately, then, it was embedded in paraffin and sectioned. The paraffin sections were fixed with the dye (hematoxylin and eosin) for histopathological analysis. The characteristics of tissue morphology in each group were observed using a microscope.
Collection of blood and preparation of serum samples
Blood was collected from the hepatic portal vein in the model group 2 and control group 2 at the last day of treatment. Subsequently, the samples were centrifuged at 4000 rpm for 15 min, and the supernatant was transferred into a clean tube for use as a serum sample. The sample was stored at −80 °C. Prior to analysis, the serum samples were thawed and vortexed. The 100 μL serum sample was carefully aspirated, and 300 μL methanol was added to the sample in order to remove proteins. After vortexing, the liquid was centrifuged at 13
000 rpm at 4 °C for 10 min. The supernatant was added to an appropriate amount of methanol in the tube and dried with nitrogen in the water bath at 40 °C. Then, 200 μL methanol was used to reconstitute the residue, followed by centrifugation at 13
000 rpm at 4 °C for 10 min again and filtered using a 0.22 μm membrane. Finally, the contents were transferred to vials for UPLC-MS analysis.
Analysis conditions of serum samples
Chromatography conditions. An Acquity™ UPLC (Waters, USA) and the Masslynx software were used for the process of chromatographic analysis. The isolation of section was implemented using an ACQUITY UPLC™ phenomenex column (2.1 nm × 50 mm, 1.7 μm). The column temperature was set at 40 °C, and the optimal mobile phase contained (A) acetonitrile with 0.1% formic acid and (B) water with 0.1% formic acid in the mobile phase. The gradient elution program for UPLC analysis started from: 0–4 min, 2–40% A; 4–10 min, 40–70% A; 10–13 min, 70–100% A; 13–16 min, 100% A; 16–17 min, 100–2% A; The elution velocity was 0.4 mL min−1. Then 3 μL of serum sample in the positive and negative modes was injected into the instrument, respectively.
MS conditions. The electrospray ionization source was conducted in the positive and negative ion mode in order to acquire data. The analysis parameters are as follows: ion source temperature, 110 °C; gas temperature, 350 °C; and desolvation gas flow, 750 L h−1. The capillary voltage was 1.5 kV in the negative mode and 1.3 kV in the positive mode. The sample cone voltage was set at 50 V and 45 V in positive and negative ion modes, respectively. The setting of the data acquisition rate was 0.2 s per scan. Data were gathered from 50 to 1500 Da in the centroid mode. A lock-mass of 0.2 ng mL−1 leucine enkephalin was used as a reference compound to monitor ion for [M − H]−= 554.2615 (negative ion mode) and [M + H]+= 556.2771 (positive ion mode) to obtain an accurate mass.
Data processing. All of the raw data were imported into the Progenesis QI software (V 2.1, Waters Corporation, MA) for achieving proper alignment, peak-picking and normalization. Then, resultant data matrices of previous step were imported to the Ezinfo software (V 3.0) for multivariate statistical analysis with pattern recognition methods. Then, the data matrix was generated to obtain information such as retention time, m/z, and peak area. The cluster profile of groups was analyzed by principal components analysis (PCA). Using Ezinfo, we acquired the variable importance in projection (VIP) values and the relative intensity of markers by orthogonal single correction partial least squares-discriminant analysis (OPLS-DA) score. We explored the characteristics across groups by the t-test.
Biomarkers identification. Via extracting the serum samples by the UPLC-MS system, we obtained metabolic profiles of the rats. The raw data were input into the Progenesis QI software. Then, a serial workflow containing vector editing, ion intensity detection, and base peaks ion intensity chromatogram was run to detect the small molecular species existing in ECB markers. A sequential components' statistical analysis was complied, and the .usp profile was obtained for the following data processing. Metabolic data matrices between sham group and ECB group were analyzed by OPLS-DA. A preliminary VIP value was selected for preliminary screening of potential biomarkers. The changes in the content of these potential markers were counted, and the secondary screening was carried out according to the principle that each metabolite in the control group and the model group had significant difference. The metabolites satisfying both the abovementioned conditions could be considered the possible markers. Then the candidate ions were identified by the serum biomarkers identification method, match mol file and secondary mass spectrometry information. Finally, potential biomarkers of the ECB rat model were obtained.
Metabolic pathway analysis. Metabolomics Pathway Analysis (MetPA) was mainly used to find the metabolomic pathways associated with metabolites according to the analysis of pathway topological characteristics. The name of the compound, KEGG or HMDB number of the detected biomarkers were introduced into the website http://www.metaboanalyst.ca for metabolic pathway analysis. The metabolic pathways relevant to the ECB model were obtained and plotted, and then, schematic drawing of the respective metabolic pathways was obtained. The impact threshold was set to 0.10. Any pathway above this threshold was classified as a potential target pathway.
Statistical analysis. Data were conveyed by SPSS (V 21.0) for statistical analysis and expressed as means ± SD. By two-sample Student's t-test, differences in average value were calculated to be statistically significant; p < 0.05 was considered statistically significant, and p < 0.01 indicated an extremely significant difference.
Results
Assessment of endometriosis of the cold coagulation and blood stasis rat model
Control group 1 and model group 1 were used to assess this model. The ectopic lesion volume of the control group 1 and model group 1 showed a significant difference, as shown in Fig. 1. Obviously, we observed that the ectopic tissue of the model group 2 showed a cystic vesicle with a transparent or translucent liquid inside. The results of histopathological observation (Fig. 2) in the control group 2 showed that the endometrial structure was intact; the gland was rich, and the structure was clear; the glandular epithelial cells were high columnar, arranged neatly and tightly, and the cytoplasm was rich; the interstitial cells were fusiform, arranged neatly and evenly distributed; the blood vessels were rich. In the model group 2, the epithelial cells of ectopic endometrium were short columnar and partially pseudostratified, and the cell morphology was incomplete. Compared with the case of the intimal tissue of the control group 2, the number of interstitial cells and glands increased significantly, and the blood vessels were abundant. Thus, this model was successfully established.
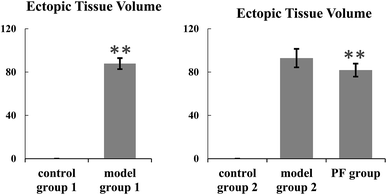 |
| Fig. 1 Changes in endometrium graft volume. | |
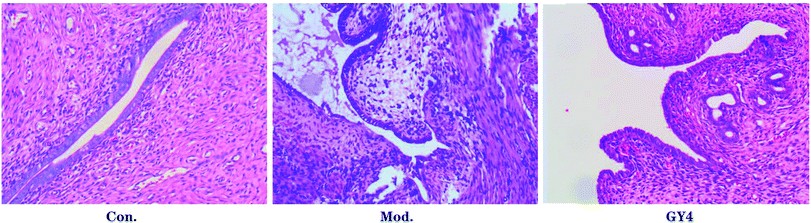 |
| Fig. 2 Endometriosis effect of shape and structure of ectopic endometrial cells in the different groups after oral administration of PF for 28 days (100× magnification). Con.: control group; Mod.: model group; GY4.: PF group. | |
The therapeutic effects of PF on the endometriosis of the cold coagulation and blood stasis model rats
The control group 2, model group 2 and PF group were used to explore the therapeutic effects for this model. Fortunately, after treatment with PF, the volumes of the ectopic endometrial tissue reduced or the tissue disappeared significantly when compared with the case of the model group 2. The data of the ectopic lesion volume are shown in Table 1. The results of the H&E staining (Fig. 2) for the PF group show that the ectopic endometrial tissue has different degrees of atrophy: the cystic cavity shrinks, the capsule wall becomes thinner, and the glandular epithelial cells become short columnar or flat, partially degenerated, necrotic or shedding, and loosely arranged with irregular nuclei and uncertain position; the number of glands decreases or the glands even disappear, showing atrophy, and the inflammatory cells appear to ooze; the number of mesenchymal cells is reduced, and these cells are small, sparse, and even absent, with reduced blood vessels and fibrosis in some areas.
Table 1 Effect of endometrium grafts volume in serum of GFW (
± SD)a
Group |
Rats/n |
Ectopic tissue volume/mm3 |
Data are expressed as means ± SD. Compared with normal control group 1, ΔP < 0.05; compared with model group 2, *P < 0.05, **P < 0.01. |
Control group 1 |
10 |
0.00 ± 0.00 |
Model group 1 |
8 |
87.85 ± 5.09ΔΔ |
Control group 2 |
10 |
0.00 ± 0.00 |
Model group 2 |
9 |
92.87 ± 8.54 |
PF group |
9 |
80.07 ± 7.12** |
Metabolic profiling analysis
Metabolomics analysis was executed on the metabolic profile data of the serum samples obtained from normal rats and ECB model rats on the 28th day of modeling (Fig. 3). The serum profiles of the BPI chromatograms of the abovementioned two groups were roughly similar. However, only several peaks were adjusted. Using the unsupervised pattern recognition method-PCA, the score plots that reflected the trend between groups were obtained (Fig. 4). It can be seen from the analysis results that the normal groups in the positive (Fig. 4A) and negative ion mode (Fig. 4B) cluster in the same region, and the rats in the model group are clustered, whereas the rats in the normal group are distinctly distinguished. This is due to the effect of external factors on modeling. The metabolic profile has changed.
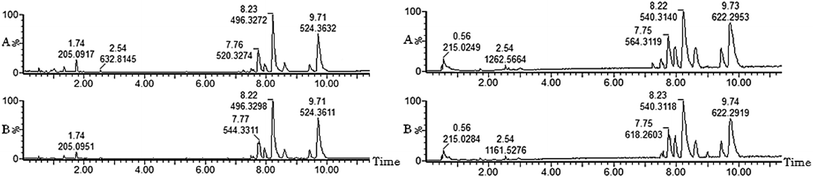 |
| Fig. 3 UPLC-MS base peaks ion (BPI) intensity chromatograms of ECB model rats in control group (A) and model group (B) by positive and negative ion modes. | |
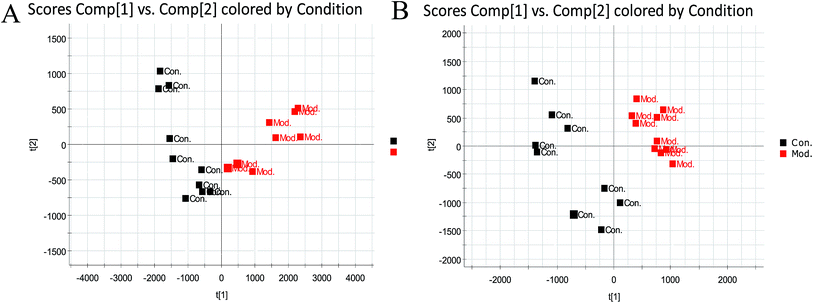 |
| Fig. 4 Score plot of serum profile of sham group and model group scanned by positive and negative ion modes. (Data were analyzed by PCA.) Note: A: In the positive ion mode, B: in the negative ion mode; Con: the sham group, Mod: the model group. | |
Determination of serum biomarkers for the ECB model
To further distinguish between the control group and the model group, the supervised OPLS-DA was employed to divide samples into two clusters in the positive mode (Fig. 5A) and negative mode (Fig. 5B). In our study, the OPLS-DA score plot analysis separated the serum samples from the control group and the model group completely based on the difference in their metabolic profiles. The significant variables were founded through the VIP plot. If the VIP value of the variable is greater than 1, the variable can contribute significantly to classification. The intensity of ions with higher VIP values was selected from the S-plot and the VIP-plot in the positive (ESI Fig. 1A and 2A†) and negative mode (ESI Fig. 1B and 2B†). Then, we selected ions with a VIP value greater than 1 and a t-test p value less than 0.05 as biomarker candidate ions after statistical analysis of the data. Finally, the metabolites with high-resolution MS and MS/MS fragments were further analyzed using the databases HMDB, ChemSpider and KEGG.
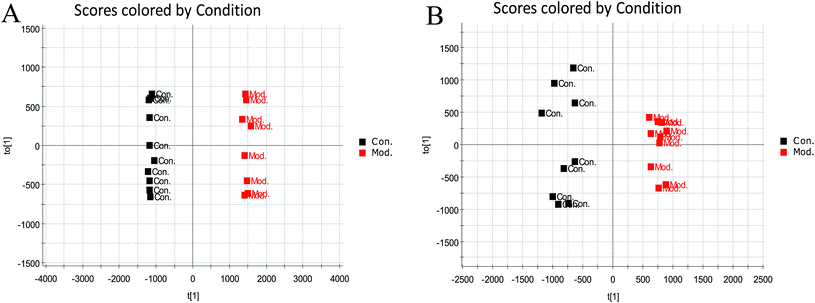 |
| Fig. 5 Score plot of serum profile of sham group and model group scanned by positive and negative ion modes. (Data were analyzed by OPLS-DA.) Note: A: in the positive ion mode, B: in the negative ion mode; Con: the sham group, Mod: the model group. | |
Using the abovementioned methods, free metabolites, such as related metabolites, and mass spectra were searched, and a total of 13 blood biomarkers associated with the ECB rat model were identified, among which eight were positive ions in the positive ion mode, namely, L-benzene L-phenylalanine, cervonoyl ethanolamide, LysoPC (16:1(9Z)), LysoPC (18:2(9Z,12Z)), LysoPE (16:0/0:0), LysoPC (16:0), LysoPC (18:1(9Z)), and LysoPC (15:0), and five were negative ions in the negative ion mode: L-tryptophan, hippuric acid, 5-HETE, etc. The specific information is shown in Table 2 and ESI Table 1.† The statistical results of the changes in the microscopic content of latent biomarkers in the model group and sham group are shown in the ESI Fig. 3.†
Table 2 Serum potential biomarkers related to ECB identified in positive and negative ion modea
No. |
Rt min |
Determined mass |
Calc mass |
Actual mass |
[M − H]−/[M + H]+ |
mDa |
ppm |
Proposed composition |
Postulated identity |
HMDB number |
Trend |
↑↓ indicates that the level of the marker is increased or decreased in the urine of rats in the ECB model group; compared with the control group, ΔP < 0.05, ΔΔP < 0.01. |
1 |
1.36 |
166.0869 |
166.0868 |
165.0789 |
[M + H]+ |
0.1 |
0.6 |
C9H11NO2 |
L-Phenylalanine |
HMDB00159 |
↑ΔΔ |
2 |
1.73 |
203.0827 |
203.0821 |
204.0898 |
[M − H]- |
0.6 |
3 |
C11H12N2O2 |
L-Tryptophan |
HMDB00929 |
↓Δ |
3 |
2.20 |
178.0504 |
178.0504 |
179.0582 |
[M − H]− |
0 |
0 |
C9H9NO3 |
Hippuric acid |
HMDB00714 |
↑ΔΔ |
4 |
4.79 |
514.293 |
514.2934 |
515.3011 |
[M − H]− |
−0.4 |
−1 |
C26H46NO7P |
LysoPC (18:4(6Z,9Z,12Z,15Z)) |
HMDB10389 |
↓ΔΔ |
5 |
5.51 |
373.2745 |
373.2473 |
372.2664 |
[M + H]+ |
0.2 |
0.5 |
C24H36O3 |
Cervonoyl ethanolamide |
HMDB13627 |
↓ΔΔ |
6 |
7.25 |
494.3242 |
494.3247 |
493.3168 |
[M + H]+ |
−0.5 |
−1 |
C24H48NO7P |
LysoPC (16:1(9Z)) |
HMDB10383 |
↓ΔΔ |
7 |
7.54 |
520.34 |
520.3403 |
519.3324 |
[M + H]+ |
−0.3 |
−0.6 |
C26H50NO7P |
LysoPC (18:2(9Z,12Z)) |
HMDB10386 |
↓Δ |
8 |
7.71 |
500.279 |
500.2777 |
501.2855 |
[M − H]− |
1.2 |
2.4 |
C25H44NO7P |
LysoPE (0:0/20:4 (8Z,11Z,14Z,17Z)) |
HMDB11488 |
↓ΔΔ |
9 |
8.13 |
454.2939 |
454.2934 |
453.2855 |
[M + H]+ |
0.5 |
1.1 |
C21H44NO7P |
LysoPE (16:0/0:0) |
HMDB11503 |
↓ΔΔ |
10 |
8.23 |
496.3404 |
496.3403 |
495.3324 |
[M + H]+ |
0.1 |
0.2 |
C24H50NO7P |
LysoPC (16:0) |
HMDB10382 |
↓ΔΔ |
11 |
8.64 |
522.3563 |
522.356 |
521.3481 |
[M + H]+ |
0.3 |
0.6 |
C26H52NO7P |
LysoPC (18:1(9Z)) |
HMDB02815 |
↓Δ |
12 |
8.99 |
319.2278 |
319.2273 |
320.2351 |
[M − H]− |
0.5 |
1.6 |
C20H32O3 |
5-HETE |
HMDB11134 |
↑ΔΔ |
13 |
9.61 |
482.0325 |
482.3247 |
481.3168 |
[M + H]+ |
0.3 |
0.6 |
C23H48NO7P |
LysoPC (15:0) |
HMDB10381 |
↓ΔΔ |
Metabolic pathway analysis
The identification of 13 blood biomarkers by chemical name, KEGG or HMDB number in the website http://www.metaboanalyst.ca for the Met-PA analysis indicated that six metabolic pathways were disordered: phenylalanine, tyrosine and tryptophan biosynthesis, glycerophospholipid metabolism, phenylalanine metabolism, tryptophan metabolism, arachidonic acid metabolism, and aminoacyl-tRNA biosynthesis, as shown in the ESI Fig. 4.† According to the Met-PA analysis results, the impact threshold of phenylalanine, tyrosine and tryptophan biosynthesis, phenylalanine metabolism and tryptophan metabolism was above 0.1. Therefore, they were the target pathways. The specific data results are shown in the ESI Table 2.† The results revealed that these endogenous compounds produced strong perturbations in the entire metabolic trace and were closely related to the ECB model.
Therapeutic effect of PF on the ECB model rats
The ESI-MS metabolic profile of the control group, model group, and PF group serum on the 28th day of administration was input into the Progenesis QI software for processing. Subsequently, these ions were processed by supervised PLS-DA to obtain score plots (ESI Fig. 5†) that could reflect the trend between groups. The control group and the ECB model group showed obvious clustering and separation. The results indicated that the serum metabolic profile of rats was significantly different from that of the normal group after the model was established. After administration, the model rats had a tendency similar to that of the control group; after 28 days of drug administration, by analyzing the trend of the ECB model serum biomarkers, we found that PF could affect the microbiological changes of potential biomarkers in the ECB model rats, and the content of these biomarkers tended to approach that of the blank control group. Altogether, 13 biomarkers were associated with the ECB model; among these, 10 were aminoglycoside, namely L-phenylalanine, L-tryptophan, hippuric acid, cervonoyl ethanolamide, 5-HETE, etc; moreover, 4 biomarkers were statistically significant. The detailed results are shown in the ESI Fig. 6 and Table 3.†
Discussion
Metabolism is a general term for a series of ordered chemical reactions that occur in living organisms to sustain life, which produces a series of metabolites. Metabolites are generally excreted through various body fluids. Hence, some clinically readily available biological fluids, such as urine, blood, and saliva29 extracts, have become the common samples for metabolomics research. After the introduction of metabolomics, it has developed very rapidly. It has been extensively applied in disease diagnosis30 and animal models, Traditional Chinese Medicine (TCM) research,31 toxicology,32,33 nutrition science34 and other research fields. Metabolomics methods and technical systems have matured and formed a complete set of research systems and strategies. A wide range of applications, its methods and technical systems are becoming more mature and form the complete research system and strategy. Metabolomics mainly analyzes the changes of small-molecular-weight metabolites of a specific biological sample or an organism35,36 caused by changes in the body's physiological and pathological phenomena. It uses some high-precision analytical methods and chemometrics methods to identify patterns in the diseased and normal groups to help understand the process of disease. We explored the changes of metabolites and their metabolic pathways in the body and searched for biomarkers related to diseases, which can be used for the early diagnosis and treatment of diseases, pathogenesis and prognosis. Generally, when a biological system is subjected to an exogenous stimulus or an endogenous disease, the small-molecule metabolites may change, or they may change with time, and the metabolomics techniques investigate the metabolic pathways in biological systems by this change. The specific research content is roughly divided into the following four aspects: sample processing, sample testing, data analysis, and retrieval of commonly used databases. It has been reported that paeoniflorin ameliorates acute renal injury37 and alpha-naphthylisothiocyanate (ANIT)-induced cholestasis,38 suppressing inflammation.39 Moreover, paeoniflorin was identified as a quality marker (Q-makers) of Tangzhiqing tablet40,41 and Buyanghuanwu injection.42 These studies prove that it is of great significance to carry out some pharmacological studies on paeoniflorin.
In this study, a rapid, high-sensitivity and high-throughput technology, i.e. UPLC-Q/TOF-MS, was used to clearly discriminate rats with ECB from matched controls and confirm the potential biomarkers. Herein, 13 biomarkers associated with the ECB disease model (5 in the negative ion mode and 8 in the positive ion mode) were identified. The biomarkers, such as LysoPC (15:0), LysoPC (16:0), LysoPC (16:1(9Z)), LysoPC (18:1(9Z)) and LysoPC (18:2(9Z,12Z)), found herein were derived from phosphatidylcholine (PC).43 LysoPE (0:0/20:4(8Z,11Z,14Z,17Z)) and LysoPE (16:0/0:0) are the metabolic products of phosphatidylethanolamine (PE).44 Both PC and PE are structural components of cell membranes,43,44 and they can be generated by phospholipase A2.44,45 Another pathway for LysoPC formation occurs by the transfer of one fatty acid of PC to cholesterol by lecithin-cholesterol acyltransferase (LCAT), which is an enzyme that converts free cholesterol into cholesteryl ester. Cholesteryl ester is a more hydrophobic form of cholesterol that is sequestered in the liver.46 LysoPC (15:0), LysoPC (16:0), LysoPC (16:1(9Z)), LysoPC (18:1(9Z)), LysoPC (18:2(9Z,12Z)), LysoPE (0:0/20:4(8Z,11Z,14Z,17Z)) and LysoPE (16:0/0:0) were discovered in the ECB disease model involved in the glycerophospholipid metabolism, which were in accordance with previous studies.47,48 5-HETE was found in the serum of ECB rats, which participated in the arachidonic acid metabolism. In the cases of lipoxygenases and cyclooxygenases, arachidonic acid49 is first converted to 5-hydroperoxyeicosatetraenoic acid (5-HPETE).50 It can be reduced to 5-hydroxyeicosatetraenoic acid (5-HETE) by ubiquitous glutathione peroxidase (GPx).50 It is one of the eicosanoids. Eicosanoids have been shown to be involved in the inflammation reaction51,52 in previous studies. Due to ectopic endometrial tissue, it is accompanied by an inflammatory reaction. Therefore, we speculated that PF may inhibit the inflammatory response by a certain concentration of 5-HETE in serum through the arachidonic acid metabolic pathway. In previous reports,39 PF was also proved to have the effect of suppressing inflammation. The results indicated that PF probably regulated the level of 5-HETE through the arachidonic acid metabolism to exert its anti-inflammation effect for ECB.
In addition, we also discovered that amino acid metabolism (phenylalanine metabolism, tryptophan metabolism) was obviously disturbed by endometriosis of cold coagulation and blood stasis. This may be due to the fact that endometriosis results from physiological mechanism of tissue injury and repair.53 The catabolic state induced in response to injury in endometriosis leads to increased breakdown of endogenous protein and release of free amino acids in circulation. It has been reported that several amino acids in tissues of women with endometriosis are found to be dysregulated. The levels of phenylalanine in serum were higher, which further confirms our experimental results.54 After treatment, phenylalanine and tryptophan were reversed from the ECB group to the control group. Thus, we concluded that paeoniflorin may be used to treat the disease by regulating the catabolic state of the amino acid and changing the concentration of phenylalanine and tryptophan in the serum.
Biomarkers have become an integral part of the research on diseases.54–59 In this study, it was found that PF regulated 10 biomarkers by the metabolic pathways of phenylalanine, tyrosine and tryptophan biosynthesis, phenylalanine metabolism, and tryptophan metabolism, to achieve a certain therapeutic effect. Although the systematic metabolic analysis was used in our study, we can integrate the physiological indicators and pathological observations to improve this study.
Conclusions
On the basis of metabolomics technology coupled with a pattern recognition approach, we identified small-molecule metabolites in rat serum. Characterization of the profiles and pattern recognition analysis were carried out. It has been proved that PF influences the metabolic profile changes of the ECB model rats. Using the OPLS-DA pattern recognition method combined with multivariate statistical analysis, the results obtained indicated changes in 13 metabolites, which might be involved in 3 target metabolic pathways: phenylalanine, tyrosine and tryptophan biosynthesis, phenylalanine metabolism, and tryptophan metabolism. After treatment with PF, 10 biomarkers had been regulated: L-phenylalanine, L-tryptophan, hippuric acid, LysoPC (18:4(6Z,9Z,12Z,15Z)), cervonoyl ethanolamide, LysoPE (0:0/20:4(8Z,11Z,14Z,17Z)), LysoPC (16:0), LysoPC (18:1(9Z)), LysoPC (16:1(9Z)), and 5-HETE. Our study has elucidated the mechanism of action of PF treatment from the metabolic pathways of the organism and demonstrated the potential of the metabolomics techniques for the study of drug action mechanisms, which provides a better understanding of ECB pathogenesis and effective metabolic pathways for the PF treatment of ECB.
Conflicts of interest
There are no conflicts to declare.
Acknowledgements
This research was supported by the grants received from the National Natural Science Foundation of China (Grant No. 81373931, 81573877), Innovative Talent Supporting Plan of Heilongjiang University of Chinese Medicine (Grant No. 2012jc01), Young Talent Lift Engineering Project of China Association of Traditional Chinese Medicine (QNRC2-B06), and Natural Science Foundation of Heilongjiang Province (YQ2019H030).
References
- X. Wang, A. Zhang and H. Sun, et al., Systems biology technologies enable personalized traditional Chinese medicine: a systematic review, Am. J. Chin. Med., 2012, 40(06), 1109–1122 CrossRef PubMed.
- S. Su, J. Duan and W. Cui, et al., Network-Based Biomarkers for Cold Coagulation Blood Stasis Syndrome and the Therapeutic Effects of Shaofu Zhuyu Decoction in Rats, Evid.-Based Complementary Altern. Med., 2013, 2013(2–4), 1–15 Search PubMed.
- J. X. Zhang, Y. Feng and Y. Zhang, et al., Hemorheology Index Changes In A Rat Acute Blood Stasis Model: A Systematic Review And Meta-Analysis, Afr. J. Tradit., Complementary Altern. Med., 2017, 14(4), 96 CrossRef CAS PubMed.
- K. Y. Hsiao, M. H. Wu and S. J. Tsai, Epigenetic regulation of the pathological process in endometriosis, Reprod. Med. Biol., 2017, 16(4), 314–319 CrossRef CAS PubMed.
- F. Facchin, G. Barbara and E. Saita, et al., Impact of endometriosis on quality of life and mental health: pelvic pain makes the difference, J. Psychosom. Obstet. Gynecol., 2015, 36(4), 135 CrossRef PubMed.
- J. Li, L. Guan and H. Zhang, et al., Endometrium metabolomic profiling reveals potential biomarkers for diagnosis of endometriosis at minimal-mild stages, Reprod. Biol. Endocrinol., 2018, 16(1), 42 CrossRef PubMed.
- Y. Dai, X. Y. Li and J. Shi, et al., A review of the risk factors, genetics and treatment of endometriosis in Chinese women: a comparative update, Reprod. Health, 2018, 82(15), 1–12 Search PubMed.
- V. H. Eisenberg, C. Weil and G. Chodick, et al., Epidemiology of endometriosis: a large population-based database study from a healthcare provider with 2 million members, BJOG, 2017, 125(1), 55–62 CrossRef PubMed.
- X. T. Han, H. Y. Guo and D. L. Kong, et al., Analysis of characteristics and influence factors of diagnostic delay of endometriosis, Zhonghua Fuchanke Zazhi, 2018, 53(2), 92–98 CAS.
- P. V. Foti, R. Farina and S. Palmucci, et al., Endometriosis: clinical features, MR imaging findings and pathologic correlation, Insights Into Imaging, 2018, 9(7), 1–24 Search PubMed.
- A. Zhang, Z. Ma and H. Sun, et al., High-Throughput Metabolomics Evaluate the Efficacy of Total Lignans From Acanthophanax Senticosus Stem Against Ovariectomized Osteoporosis Rat, Front. Pharmacol., 2019 DOI:10.3389/fphar.2019.00553.
- S. Qiu, A. H. Zhang and H. Sun, et al., Overview on metabolomics in traditional Chinese medicine, World J. Pharmacol., 20143,(3), 33–38 Search PubMed.
- P. G. Devaux, M. G. Horning and E. C. Horning, O-benzyloximes: derivatives for the study of ketosteroids by gas chromatography. Application to urinary steroids of the newborn human, Anal. Biochem., 1971, 41(1), 70–82 CrossRef CAS PubMed.
- O. Fiehn, Metabolomics the link between genotypes and phenotypes, Plant Mol. Biol., 2002, 48(1–2), 155–171 CrossRef CAS.
- O. Fiehn, Metabolic networks of Cucurbitamaxima phloem, Phytochemistry, 2003, 62(6), 875–886 CrossRef CAS.
- J. K. Nicholson, J. C. Lindon and E. Holmes, Metabonomics: understanding the metabolic responses of living systems to pathophysiological stimuli via multivariate statistical analysis of biological NMR spectroscopic data, Xenobiotica, 1999, 29(11), 1181–1189 CrossRef CAS PubMed.
- A. Zhang, H. Sun and X. Wang, Urinary metabolic profiling of rat models revealed protective function of scoparone against alcohol induced hepatotoxicity, Sci. Rep., 2014, 4, 6768 CrossRef PubMed.
- X. J. Wang, J. L. Ren and A. H. Zhang, et al., Novel applications of mass spectrometry-based metabolomics in herbal medicines and its active ingredients: current evidence, Mass Spectrom. Rev., 2019 DOI:10.1002/mas.21589.
- H. Fang, A. H. Zhang and H. Sun, et al., High-throughput metabolomics screen coupled with multivariate statistical analysis identifies therapeutic targets in alcoholic liver disease rats using liquid chromatography-mass spectrometry, J. Chromatogr. B: Anal. Technol. Biomed. Life Sci., 2019, 1109, 112–120 CrossRef CAS PubMed.
- X. Wang, A. Zhang and H. Sun, Future perspectives of Chinese medical formulae: chinmedomics as an effector, OMICS, 2012, 16(7–8), 414–421 CrossRef CAS PubMed.
- X. Wang, A. Zhang and X. Zhou, et al., An integrated chinmedomics strategy for discovery of effective constituents from traditional herbal medicine, Sci. Rep., 2016, 6, 18997 CrossRef CAS PubMed.
- A. H. Zhang, H. Sun and G. L. Yan, et al., Chinmedomics: A Powerful Approach Integrating Metabolomics with Serum Pharmacochemistry to Evaluate the Efficacy of Traditional Chinese Medicine, Engineering, 2019,(5), 60–68 CrossRef.
- A. Zhang, H. Sun and X. Wang, Mass spectrometry-driven drug discovery for development of herbal medicine, Mass Spectrom. Rev., 2016, 37(3), 307–320 CrossRef.
- A. Zhang, X. Zhou and H. Zhao, et al., Metabolomics and proteomics technologies to explore the herbal preparation affecting metabolic disorders using high resolution mass spectrometry, Mol. BioSyst., 2017, 13(2), 320–329 RSC.
- X. Wang, A. Zhang and G. Yan, et al., UHPLC-MS for the analytical characterization of traditional Chinese medicines, Trends Anal. Chem., 2014, 63, 180–187 CrossRef CAS.
- W. Dong, P. Wang and X. Meng, et al., Ultra-performance Liquid Chromatography-High-definition Mass Spectrometry Analysis of Constituents in the Root of Radix Stemonae and those Absorbed in Blood after Oral Administration of the Extract of the Crude Drug, Phytochem. Anal., 2012, 23(6), 657–667 CrossRef CAS PubMed.
- X. Wang, Q. Wang and A. Zhang, et al., Metabolomics study of intervention effects of Wen-Xin-Formula using ultra high-performance liquid chromatography/mass spectrometry coupled with pattern recognition approach, J. Pharm. Biomed. Anal., 2013, 74, 22–30 CrossRef CAS PubMed.
- M. W. Vernon and E. A. Wilson, Studies on the surgical induction of endometriosis in the rat, Fertil. Steril., 1985, 44(5), 684–694 CrossRef CAS PubMed.
- X. Wang, A. Zhang and H. Sun, et al., Discovery and development of innovative drug from traditional medicine by integrated chinmedomics strategies in the post-genomic era, TrAC, Trends Anal. Chem., 2016, 76, 86–94 CrossRef CAS.
- D. F. Gomez-Casati, M. I. Zanor and M. V. Busi, Metabolomics in plants and humans: applications in the prevention and diagnosis of diseases, BioMed Res. Int., 2013, 2013(7), 792527 Search PubMed.
- H. Sun, M. Wang and A. Zhang, et al., UPLC–Q-TOF–HDMS Analysis of Constituents in the Root of Two Kinds of Aconitum Using a Metabolomics Approach, Phytochem. Anal., 2013, 24(3), 263–276 CrossRef CAS PubMed.
- H. Sun, F. Wu and A. Zhang, et al., Profiling and identification of the absorbed constituents and metabolites of schisandra lignans by ultra-performance liquid chromatography coupled to mass spectrometry, Biomed. Chromatogr., 2013, 27(11), 1511–1519 CrossRef CAS PubMed.
- A. Zhang, S. Qiu and H. Xu, et al., Metabolomics in diabetes, Clin. Chim. Acta, 2014, 429, 106–110 CrossRef CAS PubMed.
- A. Zhang, H. Sun and S. Qiu, et al., Advancing drug discovery and development from active constituents of yinchenhao tang, a famous traditional chinese medicine formula, Evid.-Based Complementary Altern. Med., 2013, 2013, 257909 Search PubMed.
- H. Sun, A. Zhang and G. Yan, et al., Proteomics study on the hepatoprotective effects of traditional Chinese medicine formulae Yin-Chen-Hao-Tang by a combination of two-dimensional polyacrylamide gel electrophoresis and matrix-assisted laser desorption/ionization-time of flight mass spectrometry, J. Pharm. Biomed. Anal., 2013, 75, 173–179 CrossRef CAS PubMed.
- A. H. Zhang, J. B. Yu, H. Sun, L. Kong, X. Q. Wang, Q. Y. Zhang and X. J. Wang, Identifying quality-markers from Shengmai San protects against transgenic mouse model of Alzheimer's disease using chinmedomics approach, Phytomedicine, 2018, 45, 84–92 CrossRef CAS PubMed.
- P. Wang, W. Wang and Q. Shi, et al., Paeoniflorin ameliorates acute necrotizing pancreatitis and pancreatitis induced acute renal injury, Mol. Med. Rep., 2016, 14(2), 1123–1131 CrossRef CAS PubMed.
- H. Q. Zhou, W. Liu and J. Wang, et al., Paeoniflorin attenuates ANIT-induced cholestasis by inhibiting apoptosis, in vivo, via mitochondria-dependent pathway, Biomed. Pharmacother., 2017, 89, 696–704 CrossRef CAS.
- Y. Zhao, X. He and X. Ma, et al., Paeoniflorin ameliorates cholestasis via regulating hepatic transporters
and suppressing inflammation in ANIT-fed rats, Biomed. Pharmacother., 2017, 89, 61–68 CrossRef CAS.
- Z. Li, J. Liu and Y. Li, et al., Identify super quality markers from prototype-based pharmacokinetic markers of Tangzhiqing tablet (TZQ) based on, in vitro, dissolution/permeation and, in vivo, absorption correlations, Phytomedicine, 2018, 45, 59–67 CrossRef CAS PubMed.
- Y. T. Zhang, M. F. Xiao and Q. Liao, et al., Application of TQSM polypharmacokinetics and its similarity approach to ascertain Q-marker by analyses of transitivity, in vivo, of five candidates in Buyanghuanwu injection, Phytomedicine, 2018, 45, 18–25 CrossRef CAS PubMed.
- Z. Li, J. Liu and D. Zhang, et al., Nuciferine and paeoniflorin can be quality markers of Tangzhiqing tablet, a Chinese traditional patent medicine, based on the qualitative, quantitative and dose-exposure-response analysis, Phytomedicine, 2018, 44, 155–163 CrossRef CAS PubMed.
- A. Zhang, H. Sun and G. Yan, et al., Serum proteomics in biomedical research: a systematic review, Appl. Biochem. Biotechnol., 2013, 170(4), 774–786 CrossRef CAS PubMed.
- S. K. P. Lau, K.-C. Lee and G. C. S. Lo, et al., Metabolomic Profiling of Plasma from Melioidosis Patients Using UHPLC-QTOF MS Reveals Novel Biomarkers for Diagnosis, Int. J. Mol. Sci., 2016, 17(307), 1–21 Search PubMed.
- A. Zhang, H. Sun and S. Qiu, et al., Metabolomics in noninvasive breast cancer, Clin. Chim. Acta, 2013, 424, 3–7 CrossRef CAS PubMed.
- T. Matsumoto, T. Kobayashi and K. Kamata, Role of Lysophosphatidylcholine (LPC) in Atherosclerosis, Curr. Med. Chem., 2007, 14(30), 3209–3220 CrossRef CAS PubMed.
- A. Zhang, H. Sun and X. Wang, Recent advances in metabolomics in neurological disease, and future perspectives, Anal. Bioanal. Chem., 2013, 405(25), 8143–8150 CrossRef CAS PubMed.
- X. S. Meng and M. Jiang, Study on the mechanism of Chuanxiong of Chinese Medicine based on metabolomics on rats with cold blood stasis syndrome, Liaoning J. Tradit. Chin. Med., 2012, 39, 218–221 Search PubMed.
- W. S. Powell and J. Rokach, Biosynthesis, biological effects, and receptors of hydroxyeicosatetraenoic acids (HETEs) and oxoeicosatetraenoic acids (oxo-ETEs) derived from arachidonic acid, Biochim. Biophys. Acta, Mol. Cell Biol. Lipids, 2015, 1851(4), 340–355 CrossRef CAS PubMed.
- M. Gillian and P. Graham, Cross-Talk between Cancer Cells and the Tumour Microenvironment: The Role of the 5-Lipoxygenase Pathway, Int. J. Mol. Sci., 2017, 18(2), 236–262 CrossRef PubMed.
- Y. B. Joshi and P. Domenico, The 5-lipoxygenase pathway: oxidative and inflammatory contributions to the Alzheimera, Front. Cell. Neurosci., 2015, 8, 1–7 Search PubMed.
- R. Khan, V. Spagnoli and J. C. Tardif, et al., Novel anti-inflammatory therapies for the treatment of atherosclerosis, Atherosclerosis, 2015, 240(2), 497–509 CrossRef CAS.
- G. Leyendecker, L. Wildt and G. Mall, The pathophysiology of endometriosis and adenomyosis: tissue injury and repair, Arch. Gynecol. Obstet., 2009, 280(4), 529–538 CrossRef CAS PubMed.
- A. Zhang, H. Sun and G. Yan, et al., Metabolomics study of type 2 diabetes using ultra-performance LC-ESI/quadrupole-TOF high-definition MS coupled with pattern recognition methods, J. Physiol. Biochem., 2014, 70(1), 117–128 CrossRef CAS PubMed.
- H. Sun, X. Li and A. Zhang, et al., Exploring potential biomarkers of coronary heart disease treated by Jing Zhi Guan Xin Pian using high-throughput metabolomics, RSC Adv., 2019, 9(20), 11420–11432 RSC.
- J. Ren, H. Sun and H. Dong, et al., A UPLC-MS-based metabolomics approach to reveal the attenuation mechanism of Caowu compatibility with Yunnan Baiyao, RSC Adv., 2019, 9(16), 8926–8933 RSC.
- H. Sun, A. Zhang and Q. Song, et al., Functional metabolomics discover pentose and glucuronate interconversion pathways as promising targets for Yang Huang syndrome treatment with Yinchenhao Tang, RSC Adv., 2018, 8(64), 36831–36839 RSC.
- H. Zhang, A. Zhang and J. Miao, et al., Targeting regulation of tryptophan metabolism for colorectal cancer therapy: a systematic review, RSC Adv., 2019, 9(6), 3072–3080 RSC.
- H. Sun, H. L. Zhang and A. H. Zhang, et al., Network pharmacology combined with functional metabolomics discover bile acid metabolism as a promising target for mirabilite against colorectal cancer, RSC Adv., 2018, 8(53), 30061–30070 RSC.
Footnote |
† Electronic supplementary information (ESI) available. See DOI: 10.1039/c9ra03525g |
|
This journal is © The Royal Society of Chemistry 2019 |