DOI:
10.1039/C9RA02186H
(Paper)
RSC Adv., 2019,
9, 12162-12173
Exploring metabolic biomarkers and regulation pathways of acute pancreatitis using ultra-performance liquid chromatography combined with a mass spectrometry-based metabolomics strategy
Received
21st March 2019
, Accepted 8th April 2019
First published on 17th April 2019
Abstract
Acute pancreatitis (AP), as a common kind of pancreas-based inflammatory disease, is accompanied by a serious and abnormal metabolism. However, the specific metabolic process of AP is still unclear. Novel and effective drugs against acute pancreatitis are urgently required. To explore the metabolic biomarkers and regulation pathways of acute pancreatitis, ultra-performance liquid chromatography (UPLC) combined with a mass spectrometry (MS)-based metabolomics strategy was used. Sixteen male adult Sprague-Dawley rats were divided into two groups: a sham operation group (SO) and an AP model group. The AP animal model was induced via the retrograde ductal infusion of 3.5% sodium taurocholate, and rats in the SO group were infused with 0.9% saline. After serum sample collection and sacrifice, a metabolomics strategy based on UPLC-MS was used to detect serum metabolites and metabolic pathways by comparing the SO and AP model groups through full-scan analysis. A total of 19 metabolites were detected in the serum for highlighting the differences between the two groups: L-arabitol, citric acid, isocitric acid, L-phenylalanine, L-tyrosine, dihydroxyacetone, L-valine, succinic acid, 3-hydroxybutyric acid, uric acid, acetylglycine, palmitic amide, homocysteine, D-glutamine, L-arginine, arachidonic acid, N-acetylserotonin, (R)-3-hydroxy-hexadecanoic acid, and D-mannose. Six crucial metabolic pathways, phenylalanine, tyrosine and tryptophan biosynthesis, arachidonic acid metabolism, glyoxylate and dicarboxylate metabolism and the citrate cycle, were involved; these have potential to become novel targets for the treatment of AP. The ingenuity pathway analysis (IPA) platform is used to gain insights into the metabolic targets in the system, referring to development disorders, cell-to-cell signaling and interactions, cellular assembly and organization, cell compromise, cell growth and proliferation, carbohydrate metabolism and others. It is suggested that UPLC-MS-based metabolomics is capable of accurately depicting the pathological mechanisms of acute pancreatitis, which can drive new drug development.
1. Introduction
As one of main culprits behind autodigestive disorders threatening human health in recent years, acute pancreatitis (AP) is induced by the inflammation of the exocrine part of the pancreas, where digestive enzymes are secreted into the duodenum.1,2 Some of the main symptoms in patients are abdominal pain, back pain, a tender abdomen, increased heart rate, nausea and vomiting, fever and weight loss.3 Recent studies from the United States report that approximately 15–40 cases of AP are confirmed per 100
000 inhabitants, which is an increase over recent years.4,5 It can lead to multiple organ failure, such as renal and cardiovascular failure, as well as respiratory failure.6 According to the severity of the symptoms, the pathogenetic conditions of AP can either improve or aggravate within several days. Almost four-fifths of AP patients without serious morbidity can be treated. If undiagnosed or misdiagnosed, it causes the death of the patient due to multiple organ failure.7 It can be seen that early diagnosis and early prevention are of great significance in the control of AP.8 However, the pathogenesis of AP is still unclear, in relation to premature intracellular protease activation and other developments, such as oxidative stress.9,10 Currently, AP diagnosis depends on standard laboratory tests and radiological imaging, such as ultrasound, MRI and CT scans, and then fluid and nutrient replenishment, and pancreatic protease inhibitors are usually adopted in clinical practice.11–13 Owing to the invasive, risky, and time-consuming nature of these procedures, many individuals at risk for AP have not been effectively screened in time. The metabolic environment of patients can be affected by pathological damage, leading to difficulties in early diagnosis and treatment.14 It is necessary to gain insight into the pathomechanism of AP at a metabolic level before seeking out novel drugs against AP and associated systemic conditions.
Metabolomics is devoted to unscrambling the characteristics and interactions of low molecular weight endogenous metabolites in a biological sample, such as tissue, or a bodily fluid, such as blood.15 In the human body, there are far fewer metabolites (about 4000) than genes or proteins. The metabolome represents a magnifying glass highlighting biological processes of cells occurring in the body, which are involved in anabolism, catabolism, absorption, distribution, the detoxification of natural and xenobiotic materials, and energy utilization.16 Genomic and protein-related information is not completely dependent on endogenous and exogenous factors, such as enzymatic, pathological, environmental, and lifestyle factors, but alterations in metabolite levels have the ability to better reflect the functional status of cells because they occur downstream of DNA, RNA, and protein alterations.17 Compared with other omics, metabolomics, as a high-resolution and high-throughput research method, is considered to be an accessible approach for exploring diagnostic biomarkers at this stage; this is ascribed to the evolution of analytical technologies and software tools for data processing and statistics. In recent years, NMR, LC-MS and gas chromatography-mass spectrometry (GC-MS) metabolic techniques have attained widespread use as analysis methods.18 MS-based metabolomics possesses higher sensitivity, which means that there is the chance to survey a generally large number of small molecules. However, it adds the risk of generating technical artifacts and uncertainty in measurements. A combination of different metabolomics techniques could lead to the obtaining of comprehensive and sufficient metabolite information from intricate biological samples.19 It has been suggested that metabolite profiling is a promising tool for AP diagnosis, disease staging, the detection of phenotypes, prognosis prediction, predicting the onset of disease, and developing new drugs.20–23 In this study, a serum metabolomics strategy based on UPLC-MS combined with multivariate data methods and pathway analysis was used to seek therapeutic targets for AP after the retrograde ductal infusion of 3.5% sodium taurocholate to rats. In addition, histological examinations and biochemical indices assist in elucidating the establishment of an AP model.
2. Methods
2.1 Ethics statement
The study protocol was approved by the Ethics Committee for Animal Experiments of BeiJing University. All rats were handled in light of the University Guidelines and the Animal Care Committee Guidelines of Qiqihar Medical University. All operations were performed under chloral hydrate anesthesia, and all efforts were made to minimize suffering.
2.2 Chemicals and reagents
Chromatographic grade chemicals including methanol, acetonitrile and formic acid were purchased from Sigma-Aldrich (St. Louis, MO, USA) and Honeywell Company (Morristown, New Jersey, USA). The distilled water used for preparing all the aqueous solutions and mobile phases was obtained from Watson's Food & Beverage Co. Ltd (Guangzhou, China). Leucine enkephalin with a purity >99% was obtained from Sigma-Aldrich (St. Louis, MO, USA). Isoflurane and physiologic saline solution were purchased from a drugstore (Harbin, China). The assay kits for serum amylase, tumor necrosis factor-alpha (TNF-alpha), interleukin-6 (IL-6), myeloperoxidase (MPO), malondialdehyde (MDA) and superoxide dismutase (SOD) activities were all purchased from Wako Pure Chemical Industries Ltd (Osaka, Japan).
2.3 Animal model of acute pancreatitis
Six weeks old healthy male adult Sprague-Dawley (SD) rats weighing 200 ± 20 g were purchased from the Animals Experimentation Center of the BeiJing University of Chinese Medicine. All rats were maintained in an air-conditioned animal room at an appropriate temperature of 22 ± 2 °C and a relative humidity of 60 ± 5%, which provides 12 h diurnal cycles. Before the experiments, the animals are acclimated for one week with special feed and tap water. Sixteen rats were randomly allocated to two groups, with 8 rats in each group as follows: a sham operation (SO) group and an AP model group. It was reported that the retrograde ductal infusion of sodium taurocholate is a common way to induce AP. Animals fasted for 12 h and were offered free access to tap water before the operation. All rats were drugged via the intraperitoneal injection of 3 mL kg−1 10% chloral hydrate. Firstly, the hepatic duct was closed using a small bulldog clamp, and then the biliopancreatic duct was cannulated through the offside of the front opening of the duodenum papilla. 1 mL kg−1 3.5% sodium taurocholate was used for the retrograde pancreaticobiliary duct injection of rats in the AP model group using an infusion pump, whereas rats in the SO group were subject to the same operation with 1 mL kg−1 0.9% saline. After operation, the animals were also infused with physiologic saline solution for reviviscence.
2.4 Histological examinations and biochemical indices
Twelve hours after model establishment, the two groups of rats were injected with 4% pentobarbital sodium (0.3 mL/100 g body weight). 5 mL heparinized blood samples were obtained from the angular vein and centrifuged at 3500 rpm for 15 min at 4 °C. The serum supernatant was collected and stored immediately at −80 °C. The serum amylase, tumor necrosis factor-alpha (TNF-alpha), interleukin-6 (IL-6), myeloperoxidase (MPO), malondialdehyde (MDA) and superoxide dismutase (SOD) activities were determined according to the manufacturer instructions using assay kits. Pancreatic tissue was immediately removed and immersed in 10% formalin neutral buffer solution for H&E analysis after fixation, embedding in paraffin, and sectioning. After the experiments, the rats were sacrificed and cremated.
2.5 Metabolomics studies
2.5.1 Sample preparation. The serum samples were thawed at 4 °C and further processed for metabolomics analysis. 150 μL of serum was mixed with 700 μL of methanol for deproteinization, and this mixture was then vortexed for 30 s. The mixed serum sample was centrifuged at 12
000 rpm and 4 °C for 15 min. The supernatant was collected and evaporated to dryness under a nitrogen gas stream at 45 °C. After reconstitution using an extraction buffer including 20% acetonitrile, 40% methanol and 40% water, the dried sample was centrifuged again at 12
000 rpm and 4 °C for 15 min to remove fine particulate matter. 2 μL of supernatant was transferred to a glass vial for injection for UPLC-MS analysis.
2.5.2 LC-MS conditions. Chromatographic analysis was performed using a Waters Acquity TM Ultra Performance LC system (Waters, Milford, USA), which was equipped with a pump, an autosampler and a 0.17 μm ACQUITY BEH C 18 chromatography column. Mass spectrometry was performed using a Waters Synapt TM HDMS (Waters, Milford, USA), equipped with positive ionization mode (ESI+) and negative ionization mode (ESI−) sources for sample analysis. A quality control (QC) sample, characterized by its ability to check basic information related to the chromatography column, was used; it seemed a salient sample, as it involved all of the compounds of the sample to be analyzed.The optimizing mobile chromatography phase consisted of (A) acetonitrile with 0.15% formic acid and (B) water with 0.15% formic acid. The elution gradient conditions were set to the following: 0–1 min, 2–10% A; 1–2.5 min, 10–20% A; 2.5–5 min, 20–45% A; 5–6 min, 45–60% A; 6–9 min, 60–83% A; 9–11 min, 83–100%A; 11–13 min, 100% A; and 13–15 min, 100–2% A. 2 μL of sample solution was injected for separation and the column temperature was held at 30 °C with a flow rate of 0.4 mL min−1. Then, the obtained elution was promptly transferred to the MS system before splitting. The cone voltage was 45 V and 35 V in positive and negative mode, respectively. 100–2000 m/z scan data were acquired with a 0.2 s scan time and a 0.1 s inter-scan delay over a 15 min run-time. In positive mode, the capillary voltage was set to 3.5 kV, and the capillary voltage was set to 2.8 kV in negative mode. The MS source temperature was set to 110 °C, and the desolvation gas temperature was set to 350 °C under a desolvation gas flow of 50 L h−1. 0.1 ng mL−1 of leucine enkephalin infused under a flow rate of 120 μL min−1 as a reference ion for positive ion mode ([M + H]+ = 556.2771) and negative ion mode ([M − H]− = 554.2615) was used to ensure exact data measurements.
2.5.3 Data processing and analysis. For data dimension reduction and matrix acquisition, the raw UPLC-MS data was processed using Progenesis QI software v1.0 (Nonlinear Dynamics, Newcastle, U.K.). The retention time and m/z data pair for each peak was collected, and then each ion intensity from each detected peak was normalized to the sum of the peak intensities, which provides the peak code, m/z value and normalized intensity. Multivariate analysis was performed using EZinfo 2.0 software (Waters Corporation, Manchester, UK), i.e., unsupervised principal component analysis (PCA), partial least squares-discriminant analysis (PLS-DA), orthogonal projections to latent structures-discriminant analysis (OPLS-DA) and variable importance in projection (VIP) score plots. Via t-test analysis, differential ions with VIP values of more than 1 and p values of less than 0.05 were screened and deemed as making a great contribution to the discrepancies between the SO and AP model groups. According to preliminary information, including retention time (Rt), precise molecular mass, and MS/MS fragment data, the selected potential biomarkers were investigated and identified using online databases, such as LIPID MAPS (http://www.lipidmaps.org/tools/index.html), HMDB (http://www.hmdb.ca/), MassBank (http://www.massbank.jp), ChemSpider (http://www.chemspider.com) and METLIN (http://metlin.scripps.edu). Potential pathways closely associated with biomarkers were established using MetaboAnalyst 4.0 (http://www.metaboanalyst.ca/). All quantitative data were expressed as mean ± SD. In the Student's t-tests, p values less than 0.05 were considered as statistically significant and p values less than 0.01 were considered as extremely statistically significant.
3. Results
3.1 Histological and biochemical analysis
The movement of rats in the model group was slower than that of the control rats. In a histopathological examination with H&E staining, it was shown that the pancreatic tissue of model rats was observed to show severe edema, acinar cell degeneration, and moderate inflammation compared with the control mice. The serum amylase activity and levels of TNF-α, IL-6, MPO and MDA in rats were significantly increased in the model group (p < 0.01). Compared with the control rats, the levels of SOD in the serum of the AP mice were lower (p < 0.05). The results suggested that the condition of the pancreatic tissue became worse and worse as time went on, which led to reduced immunity in the model rats (Fig. 1).
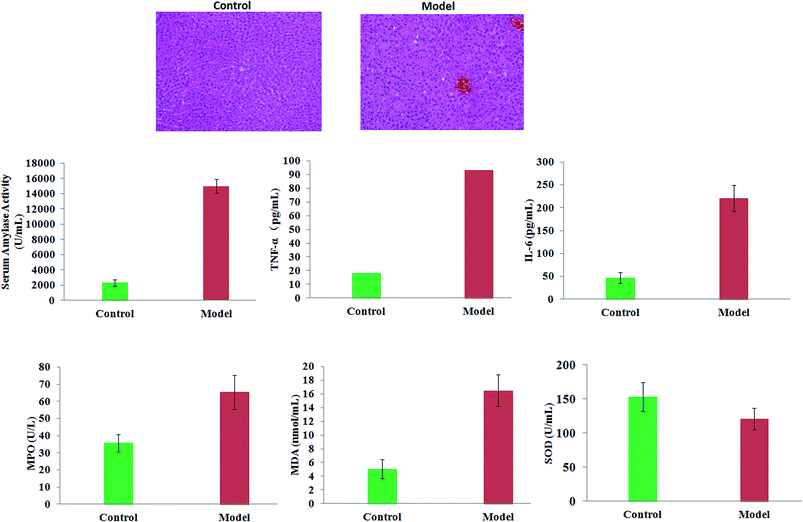 |
| Fig. 1 A comparison of the extent of AP lesions in pancreas tissue and clinical biochemistry analysis between the control and model groups. | |
3.2 Multivariate statistical analysis
Every group of serum samples was collected and analyzed under the optimal UPLC-MS conditions mentioned above. After Progenesis QI manipulation, 9892 peaks and 8042 peaks were discerned as positive ion (ESI+) peaks and negative ion (ESI−) peaks, respectively. As shown in Fig. 2, each point stands for an individual sample in the PCA score plot. The metabolic profiles of the model group were separated evidently from those of the control group, which revealed that serum metabolites in the AP rats do not demonstrate normal levels. Then, more sophisticated OPLS-DA was performed to explore whether the differentially expressed metabolites were responsible for the separation between the control group and the model group. In Fig. 3, the ions furthest away from the origin may be considered as the differentiating metabolites in the corresponding S-plot. The acquired VIP-plots closely relate to potential biomarker screening using the variation and correlation contributions, through which differentially expressed metabolites ions were selected using VIP values of more than 1 and p values of less than 0.05 from Student's t-test analysis.
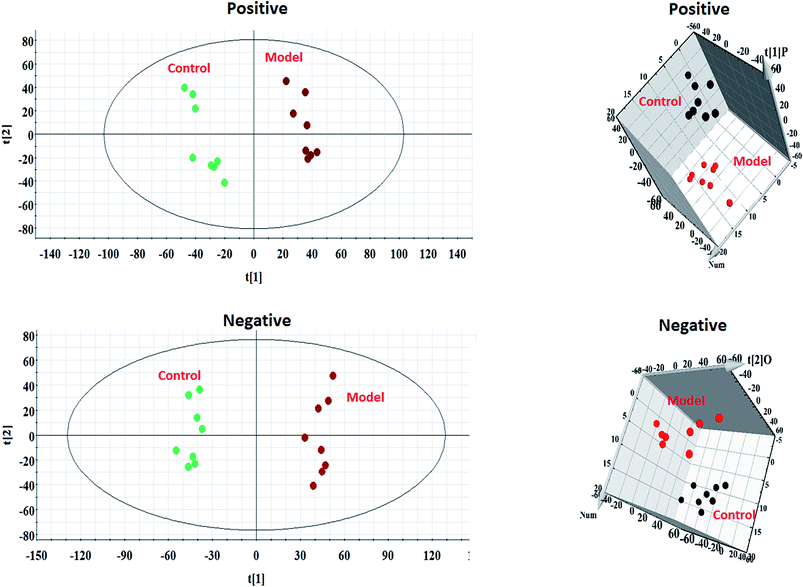 |
| Fig. 2 PCA score plots and 3D OPLS-DA score plots from serum sample UPLC-MS spectral data from the control and model groups in positive and negative mode. | |
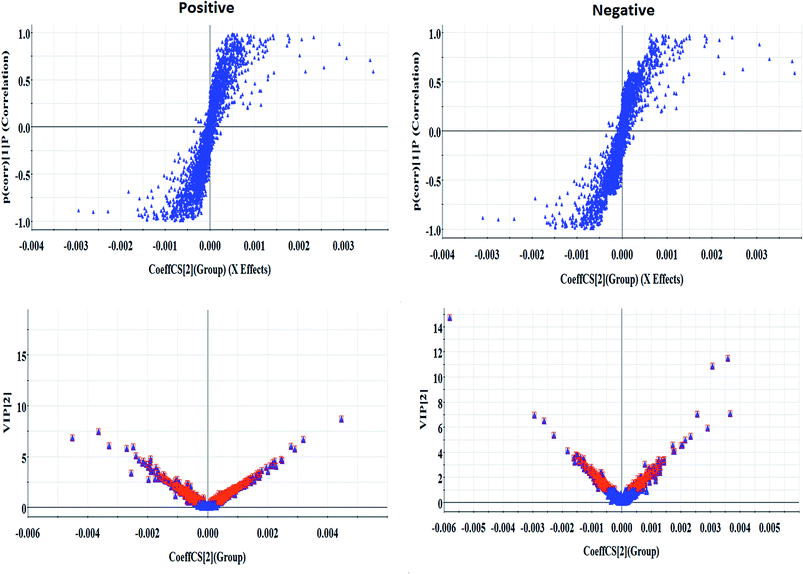 |
| Fig. 3 S-plots and VIP-plots of the OPLS-DA model data from the control and model groups in positive and negative mode. | |
3.3 Potential biomarker analysis
MS/MS information from differentially expressed metabolites ions was collected. With the help of biochemical online databases, such as MetaboAnalyst, LIPID MAPS, HMDB, MassBank and METLIN, possible chemical compositions and presumed molecular formulas were collected. As shown in Table 1, a total of 19 metabolites were tentatively proposed as potential biomarkers to interpret the separation between the control and model groups: includes L-arabitol, citric acid, isocitric acid, L-phenylalanine, L-tyrosine, dihydroxyacetone, L-valine, succinic acid, 3-hydroxybutyric acid, uric acid, acetylglycine, palmitic amide, homocysteine, D-glutamine, L-arginine, arachidonic acid, N-acetylserotonin, (R)-3-hydroxy-hexadecanoic acid, and D-mannose. As shown in Fig. 4, five metabolites were decreased in the AP rats compared with the control rats, and fourteen metabolites were increased.
Table 1 The differentially expressed metabolites in AP rats using a non-targeted serum metabolomics strategy
No. |
Rt |
m/z |
|
Proposed compound |
Formula |
Ion form |
Trend |
1 |
2.63 |
153.07 |
HMDB01851 |
L-Arabitol |
C5H12O5 |
M + H |
↑ |
2 |
0.55 |
191.01 |
HMDB00094 |
Citric acid |
C6H8O7 |
M − H |
↑ |
3 |
0.66 |
215.01 |
HMDB00193 |
Isocitric acid |
C6H8O7 |
M + H |
↓ |
4 |
0.92 |
164.07 |
HMDB00159 |
L-Phenylalanine |
C9H11NO2 |
M − H |
↑ |
5 |
0.24 |
182.08 |
HMDB00158 |
L-Tyrosine |
C9H11NO3 |
M + H |
↑ |
6 |
8.3 |
89.02 |
HMDB01882 |
Dihydroxyacetone |
C3H6O3 |
M − H |
↑ |
7 |
0.55 |
116.07 |
HMDB00883 |
L-Valine |
C5H11NO2 |
M − H |
↑ |
8 |
0.71 |
117.01 |
HMDB00254 |
Succinic acid |
C4H6O4 |
M − H |
↑ |
9 |
1.98 |
127.03 |
HMDB00357 |
3-Hydroxybutyric acid |
C4H8O3 |
M + H |
↑ |
10 |
0.69 |
169.04 |
HMDB00289 |
Uric acid |
C5H4N4O3 |
M + H |
↑ |
11 |
1.89 |
118.05 |
HMDB00532 |
Acetylglycine |
C4H7NO3 |
M + H |
↓ |
12 |
8.52 |
256.26 |
HMDB12273 |
Palmitic amide |
C16H33NO |
M + H |
↑ |
13 |
2.46 |
136.04 |
HMDB00742 |
Homocysteine |
C4H9NO2S |
M + H |
↓ |
14 |
3.03 |
145.06 |
HMDB03423 |
D-Glutamine |
C5H10N2O3 |
M − H |
↓ |
15 |
0.60 |
175.12 |
HMDB00517 |
L-Arginine |
C6H14N4O2 |
M + H |
↑ |
16 |
7.90 |
327.23 |
HMDB01043 |
Arachidonic acid |
C20H32O2 |
M + H |
↑ |
17 |
1.09 |
217.09 |
HMDB01238 |
N-Acetylserotonin |
C12H14N2O2 |
M − H |
↓ |
18 |
5.91 |
271.22 |
HMDB10734 |
(R)-3-Hydroxy-hexadecanoic acid |
C16H32O3 |
M − H |
↑ |
19 |
1.00 |
181.07 |
HMDB00169 |
D-Mannose |
C6H12O6 |
M + H |
↑ |
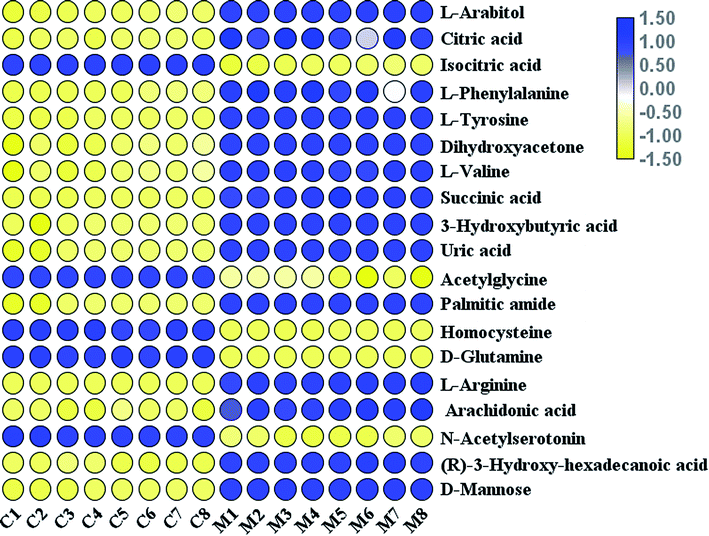 |
| Fig. 4 A heatmap visualisation of the serum samples from the control and model groups. | |
3.4 Potential metabolic pathway analysis
MetPA is a free web-based tool with pathway topology analysis based on the MetaboAnalyst platform. The pathogenesis of AP mainly involves the following pathways: phenylalanine, tyrosine and tryptophan biosynthesis, phenylalanine metabolism, valine, leucine and isoleucine biosynthesis, arachidonic acid metabolism, glyoxylate and dicarboxylate metabolism and the citrate cycle (TCA cycle), whose p values are 1.000, 0.4071, 0.3330, 0.3260, 0.2960 and 0.1205, respectively (Fig. 5). The KEGG global metabolic network, which portrays metabolites and enzymes/KEGG orthologs, is shown in Fig. 6 and is associated with 9 metabolites and 10 pathways. The metabolite–metabolite interaction network involved citric acid, L-tyrosine, L-phenylalanine, isocitric acid, L-valine, succinic acid, L-arginine, homocysteine, arachidonic acid, uric acid, and D-mannose, and is extruded in line with reactions involving similar chemical structures and molecular activities, as shown in Fig. 7. Then, IPA was utilized to further decipher ingenuity pathway data involving detailed changes in complex data; this indicated that AP pathogenesis is mainly associated with development disorder, cell-to-cell signaling and interactions, cellular assembly and organization, cell compromise, cell growth and proliferation, carbohydrate metabolism and others (Fig. 8).
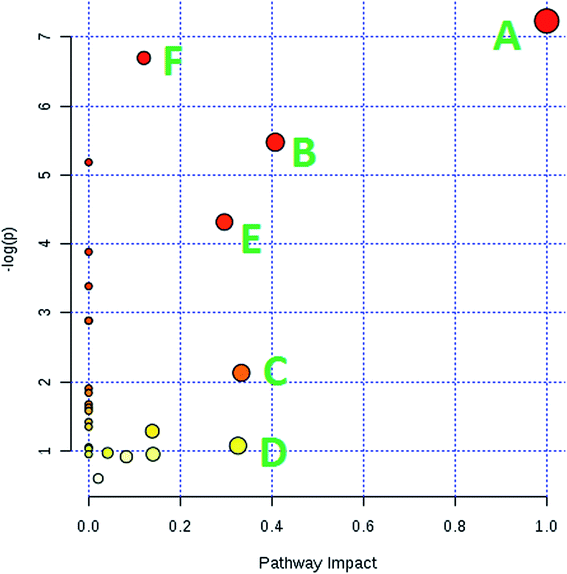 |
| Fig. 5 Pathway analysis of the metabolic variations in AP rats. (A) phenylalanine, tyrosine and tryptophan biosynthesis; (B) phenylalanine metabolism; (C) valine, leucine and isoleucine biosynthesis; (D) arachidonic acid metabolism; (E) glyoxylate and dicarboxylate metabolism; and (F) the citrate cycle (TCA cycle). | |
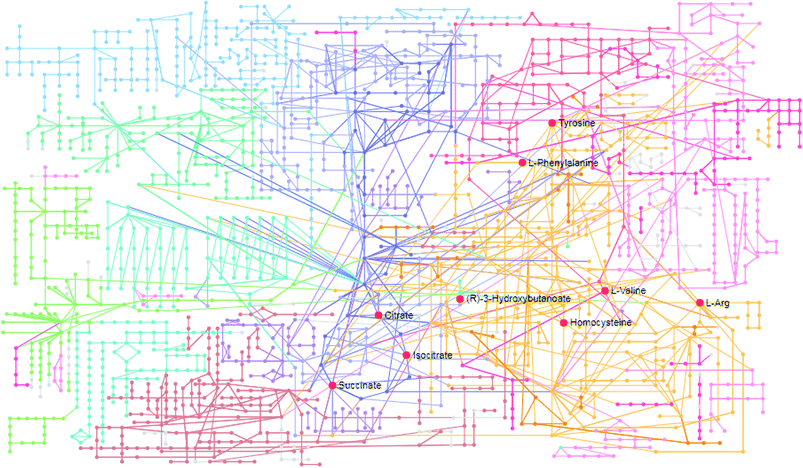 |
| Fig. 6 KEGG global metabolic network related to AP pathogenesis. | |
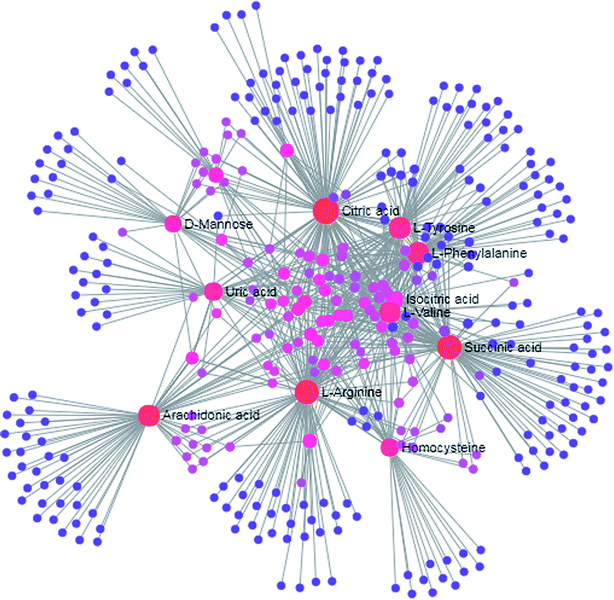 |
| Fig. 7 Metabolite–metabolite interaction network related to AP pathogenesis. | |
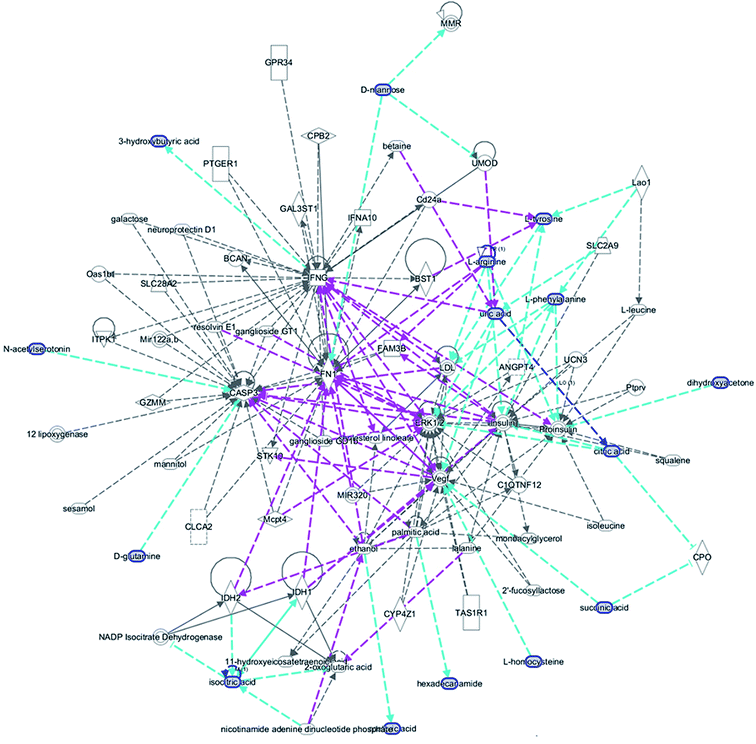 |
| Fig. 8 IPA prediction networks related to an AP pathomechanism induced by the retrograde ductal infusion of sodium taurocholate in rats in this study. | |
4. Discussion
Pancreatic duct reflex caused by pancreaticobiliary reflux is one of the main causes of AP, which does harm to the pancreatic duct. A large amount of pancreatic enzymes in the pancreas are activated and released in advance to cause inflammation. At the same time, activated various inflammatory cytokines, tumor necrosis factors, and oxidative stress species enter the pancreas, leading to the activation of proinflammatory mediators, which sequentially damage pancreatic cells and surrounding tissues, even leading to multiple organ failure and death.24 At present, the release of inflammatory factors promotes the apoptosis of pancreatic cells, and the reduction in the apoptosis of pancreatic cells after AP has become one of the current research hotspots in many pathogenesis analyses of AP.25,26
The selection of animal species and the method of model preparation play important roles in this type of research. A drug-induced AP rat model is superior to a mouse model in terms of stability and the degree of pancreatic lesion homogeneity, and rats are also more convenient when it comes to monitoring hemodynamics and organ function.27 In recent years, it has become easier to prepare animal models of transgenic and knockout mice with the rapid development of genetic modification technology, which makes it more conducive to verify the regulation of key genes in terms of AP, but the sensitivity of different strains of mice to AP remains different.28 At present, the retrograde injection of sodium taurocholate (ST) into the pancreatic bile tract, invented by Aho HJ, is the most commonly used AP induction method.29 Sodium taurocholate is one of the main components of two kinds of bile acids in the body. The retrograde injection of sodium taurocholate mainly simulates acute pancreatitis caused by bile reflux in the lower obstruction of the common bile duct; it is required to implant the plastic tube of the pancreaticobiliary tube through the duodenal papilla opening. ST is injected through the plastic tube, and the pancreatic cells immediately demonstrate edema, hemorrhage and necrosis within 24 hours. This is complex and traumatic, and the duodenum is then cut into a puncture tube to reduce the risk of intestinal damage and bleeding over time. It has been reported that the oxidative stress and NO synthase regulation patterns in the pancreatic tissue of rats subject to direct pancreatic duct ligation (PDL) are similar to those seen during human pancreatitis, suggesting that the PDL-induced rat pancreatitis model is a promising model for studying the development of human acute pancreatitis.30 The retrograde injection AP model is reliable, but the severity and repeatability of AP is highly dependent on the perfusion concentration, perfusion pressure, perfusion volume, and time. It is more suitable for etiology studies than for therapeutic studies. In addition, there are pancreatic subcapsular injection, L-arginine injection, cerulein injection, and bile-free ethionine feeding approaches.31
Metabolomics tools play an important role in researching metabolic disorders and metabolism-related diseases,32–35 in the discovery and identification of biomarkers36–39 and in investigating responses to treatment.40–48 A significant increase in serum amylase for 12 hours in the rats, revealed by serological examination, demonstrated that serum amylase is strongly activated. At the same time, the release of cytokines into circulation during acute severe pancreatitis was detected. The results showed that the levels of inflammatory factors, such as tumor necrosis factor-α (TNF-α) and interleukin-6 (IL-6), are significantly elevated in the AP group of rats. In the oxidative stress system analysis, an increase in the cytokines MPO and MDA, which promote oxidative stress in AP rats, and a decrease in the cytokine SOD, which attenuates oxidative stress, indicated that the model rats were in an oxidative stress-enhancing state.49,50 This is one of the causes of secondary tissue damage. The above results were similarly verified from pathological analysis of sections of pancreas. The stress state can change the free amino acid content in the body, and an enhancement in skeletal muscle protein degradation is one of the important factors leading to the redistribution of amino acids in the blood. The Phe/Try ratio is a sensitive indicator of skeletal muscle protein degradation. Phe and Try levels in the model group were significantly higher than in the control group, suggesting that the plasma amino acid profile changes and protein breakdown occurs in skeletal muscle. The study found that for a higher Phe/Try ratio, the skeletal muscle protein breakdown may be faster, and skeletal muscle consumption is most pronounced. From the perspective of energy metabolism, it should be noted that energy supply to the body to prevent the excessive decomposition of protein should be paid attention to in the early treatment of AP.51,52 Proline, leucine and isoleucine contain large hydrophobic side chains, and their rigid structural features and hydrophobic interactions have an important influence on protein folding. In this study, it was found that the synthesis of proline, leucine and isoleucine was impeded, indicating that the protein production pathway was hampered, which is similar to the pathological mechanism of pancreatitis. Arachidonic acid metabolites include prostaglandins and leukotrienes, both of which are cleavable by arachidonic acid under the action of PLA2. Arachidonic acid is converted to prostacyclin (PGI2) and hemagglutinin A2 (TXA2) under the catalysis of cyclooxygenase. PGI2 is a potent platelet aggregation inhibitor and vascular relaxant, and TXA2 is the strongest platelet aggregation agent and vasoconstrictor. The opposite effects of PGI2 and TXA2 maintain an equilibrium, which is an important physiological function of the body that can control the normal hemostasis mechanism. The content of arachidonic acid in the model group increased, leading to the imbalance between PGI2 and TXA2. Leukotriene produced by another metabolic pathway of arachidonic acid has biological effects, such as increased capillary permeability, contracted blood vessels, and neutrophil chemotaxis. It is also the main cause of pancreatic pain in AP patients. Amino acid metabolism and arachidonic acid metabolism have the potential to be seen as novel targets for the treatment of AP.53
5. Conclusions
The characteristics of the serum metabolomic profile discrepancies between AP and SO rats were found. L-Arabitol, citric acid, isocitric acid, L-phenylalanine, L-tyrosine, dihydroxyacetone, L-valine, succinic acid, 3-hydroxybutyric acid, uric acid, acetylglycine, palmitic amide, homocysteine, D-glutamine, L-arginine, arachidonic acid, N-acetylserotonin, (R)-3-hydroxy-hexadecanoic acid, and D-mannose may be seen as potential biomarkers of AP morbidity, which contribute further understanding to the pathophysiology and metabolic state. Amino acid metabolism and arachidonic acid metabolism contribute to the evaluation of a holistic mechanism and the search for new drugs for AP. The UPLC-MS based metabolomics method was able to offer novel and non-invasive methodology for AP research in the medical world.
Conflicts of interest
There are no conflicts to declare.
References
- M. E. Krenzer, Understanding acute pancreatitis, Nursing, 2016, 46(8), 34–40 CrossRef PubMed.
- P. A. Banks, D. L. Conwell and P. P. Toskes, The management of acute and chronic pancreatitis, Gastroenterol. Hepatol., 2010, 6(2), 1–16 Search PubMed.
- A. Sato, Acute pancreatitis. 1. Epidemiology and symptoms, Nihon Naika Gakkai Zasshi, 2010, 99(1), 4–8 CrossRef PubMed.
- S. G. Krishna, A. K. Kamboj and P. A. Hart, et al., The Changing Epidemiology of Acute Pancreatitis Hospitalizations: A Decade of Trends and the Impact of Chronic Pancreatitis, Pancreas, 2017, 46(4), 482–488 CrossRef PubMed.
- J. Fan, L. Ding and Y. Lu, et al., Epidemiology and Etiology of Acute Pancreatitis in Urban and Suburban Areas in Shanghai: A Retrospective Study, Gastroentero.l Res. Pract., 2018, 2018, 1420590 Search PubMed.
- G. Weitz, J. Woitalla and P. Wellhöner, et al., Comorbidity in acute pancreatitis relates to organ failure but not to local complications, Z. Gastroenterol., 2016, 54(3), 226–230 CrossRef CAS PubMed.
- S. Mayor, A fifth of acute pancreatitis cases are not diagnosed promptly, inquiry warns, BMJ, 2016, 354, i3746 CrossRef PubMed.
- N. He, Y. Y. Yan and Y. Q. Ying, et al., Individualized vancomycin dosing for a patient diagnosed as severe acute pancreatitis with concurrent extracorporeal membrane oxygenation and continuous veno-venous hemofiltration therapy: a case report, Beijing Daxue Xuebao, Yixueban, 2018, 50(5), 915–920 CAS.
- S. Regnér, J. Manjer and S. Appelros, et al., Protease activation, pancreatic leakage, and inflammation in acute pancreatitis: differences between mild and severe cases and changes over the first three days, Pancreatology, 2008, 8(6), 600–607 CrossRef PubMed.
- S. Bopanna, B. Nayak and S. Prakash, et al., Increased oxidative stress and deficient antioxidant levels may be involved in the pathogenesis of idiopathic recurrent acute pancreatitis, Pancreatology, 2017, 17(4), 529–533 CrossRef CAS PubMed.
- T. Seta, Y. Noguchi and S. Shikata, et al., Treatment of acute pancreatitis with protease inhibitors administered through intravenous infusion: an updated systematic review and meta-analysis, BMC Gastroenterol., 2014, 14, 102 CrossRef PubMed.
- E. Zerem, Treatment of severe acute pancreatitis and its complications, World J. Gastroenterol., 2014, 20(38), 13879–13892 CrossRef PubMed.
- R. Restrepo, H. E. Hagerott and S. Kulkarni, et al., Acute Pancreatitis in Pediatric Patients: Demographics, Etiology, and Diagnostic Imaging, AJR, Am. J. Roentgenol., 2016, 206(3), 632–644 CrossRef.
- I. Mikolasevic, S. Milic and L. Orlic, et al., Metabolic syndrome and acute pancreatitis, Eur. J. Intern. Med., 2016, 32, 79–83 CrossRef CAS PubMed.
- A. H. Zhang, H. Sun, S. Qiu and X. J. Wang, Recent highlights of metabolomics in chinese medicine syndrome research, Evid.-Based Complementary Altern. Med., 2013, 2013, 402159 Search PubMed.
- A. Zhang, H. Sun, G. Yan, P. Wang and X. Wang, Mass spectrometry-based metabolomics: applications to biomarker and metabolic pathway research, Biomed. Chromatogr., 2016, 30(1), 7–12 CrossRef PubMed.
- T. Zhang, A. Zhang, S. Qiu, H. Sun, Y. Han, Y. Guan and X. Wang, High-throughput metabolomics approach reveals new mechanistic insights for drug response of phenotypes of geniposide towards alcohol-induced liver injury by using liquid chromatography coupled to high resolution mass spectrometry, Mol. BioSyst., 2016, 13(1), 73–82 RSC.
- T. Zhang, A. Zhang, S. Qiu, S. Yang and X. Wang, Current Trends and Innovations in Bioanalytical Techniques of Metabolomics, Crit. Rev. Anal. Chem., 2016, 46(4), 342–351 CrossRef CAS PubMed.
- A. H. Zhang, J. B. Yu and H. Sun, et al., Identifying quality-markers from Shengmai San protects against transgenic mouse model of Alzheimer's disease using chinmedomics approach, Phytomedicine, 2018, 45, 84–92 CrossRef CAS PubMed.
- H. Sun, A. Zhang and Q. Song, et al., Functional metabolomics discover
pentose and glucuronate interconversion pathways as promising targets for Yang Huang syndrome treatment with Yinchenhao Tang, RSC Adv., 2018, 8(64), 36831–36839 RSC.
- A. Zhang, H. Sun and G. Yan, et al., Metabolomics Analysis of Marker Metabolites for Patients with Pancreatic Cancer, Med. Chem., 2014, 4, 506–510 Search PubMed.
- A. Zhang, H. Sun and G. Yan, et al., Chinmedomics: A Powerful Approach Integrating Metabolomics with Serum Pharmacochemistry to Evaluate the Efficacy of Traditional Chinese Medicine, Engineering, 2019, 5, 60–68 CrossRef.
- X. Wang, H. Lv and A. Zhang, et al., Metabolite profiling and pathway analysis of acute hepatitis rats by UPLC-ESI MS combined with pattern recognition methods, Liver Int., 2014, 34(5), 759–770 CrossRef CAS PubMed.
- S. Majidi, A. Golembioski and S. L. Wilson, et al., Acute Pancreatitis: Etiology, Pathology, Diagnosis, and Treatment, South. Med. J., 2017, 110(11), 727–732 CrossRef CAS PubMed.
- S. Q. Ding, Y. Li and Z. G. Zhou, et al., Toll-like receptor 4-mediated apoptosis of pancreatic cells in cerulein-induced acute pancreatitisin mice, Hepatobiliary Pancreatic Dis. Int., 2010, 9(6), 645–650 CAS.
- T. Hu, Y. Chen and Q. Jiang, et al., Overexpressed eNOS upregulates SIRT1 expression and protects mouse pancreatic β cells from apoptosis, Exp. Ther. Med., 2017, 14(2), 1727–1731 CrossRef CAS.
- M. Li, J. Yu and L. Zhao, et al., Inhibition of macrophage migration inhibitory factor attenuates inflammation and fetal kidney injury in a rat model of acute pancreatitis in pregnancy, Int. Immunopharmacol., 2019, 68, 106–114 CrossRef CAS PubMed.
- X. Hong, J. Zhang and Q. Wu, et al., Challenges in detecting pre-malignant pancreatic lesions during acute pancreatitis using a serum microRNA assay: a study based on KrasG12D transgenic mice, Oncotarget, 2016, 7(16), 22700–22710 Search PubMed.
- H. J. Aho, T. J. Nevalainen and A. J. Aho, Experimental pancreatitis in the rat. Development of pancreatic necrosis, ischemia and edema after intraductal sodium taurocholate injection, Eur. Surg. Res., 1983, 15(1), 28–36 CrossRef CAS PubMed.
- I. Buchwalow, J. Schnekenburger and D. Atiakshin, et al., Oxidative stress and NO generation in the rat pancreatitis induced by pancreatic duct ligation, Acta Histochem., 2017, 119(3), 252–256 CrossRef CAS PubMed.
- L. Peng, L. G. Wu and B. Li, et al., Early enteral nutrition improves intestinal immune barrier in a rat model of severe acute pancreatitis, J. Hepatobiliary Pancreat. Sci., 2016, 23(11), 681–687 CrossRef PubMed.
- A. Zhang, H. Sun and H. Xu, et al., Cell metabolomics, OMICS, 2013, 17(10), 495–501 CrossRef CAS PubMed.
- H. Sun, A. H. Zhang and S. B. Liu, et al., Cell metabolomics identify regulatory pathways and targets of magnoline against prostate cancer, J. Chromatogr. B: Anal. Technol. Biomed. Life Sci., 2018, 1102–1103, 143–151 CrossRef CAS PubMed.
- A. Zhang, H. Sun and X. Wang, Mass spectrometry-driven drug discovery for development of herbal medicine, Mass Spectrom. Rev., 2018, 37(3), 307–320 CrossRef CAS PubMed.
- H. Sun, M. Wang and A. Zhang, et al., UPLC–Q-TOF–HDMS Analysis of Constituents in the Root of Two Kinds of Aconitum Using a Metabolomics Approach, Phytochem. Anal., 2013, 24(3), 263–276 CrossRef CAS PubMed.
- F. Wu, H. Sun and W. Wei, et al., Rapid and global detection and characterization of the constituents in ShengMai San by ultra-performance liquid chromatography–high-definition mass spectrometry, J. Sep. Sci., 2011, 34(22), 3194–3199 CrossRef CAS PubMed.
- X. Wang, A. Zhang and H. Sun, et al., Discovery and development of innovative drug from traditional medicine by integrated chinmedomics strategies in the post-genomic era, TrAC, Trends Anal. Chem., 2016, 76, 86–94 CrossRef CAS.
- A. Zhang, H. Sun and X. Wang, Urinary metabolic profiling of rat models revealed protective function of scoparone against alcohol induced hepatotoxicity, Sci. Rep., 2014, 4, 6768 CrossRef PubMed.
- X. Liu, A. Zhang and H. Fang, et al., Serum metabolomics strategy for understanding the therapeutic effects of Yin-Chen-Hao-Tang against Yanghuang syndrome, RSC Adv., 2018, 8(14), 7403–7413 RSC.
- A. Zhang, H. Sun and G. Yan, et al., Metabolomics study of type 2 diabetes using ultra-performance LC-ESI/quadrupole-TOF high-definition MS coupled with pattern recognition methods, J. Physiol. Biochem., 2014, 70(1), 117–128 CrossRef CAS PubMed.
- Q. Song, A. Zhang and G. Yan, et al., Technological advances in current metabolomics and its application in tradition Chinese medicine, RSC Adv., 2017, 7(84), 53516–53524 RSC.
- Q. Zhao, A. Zhang and W. Zong, et al., Chemometrics strategy coupled with high resolution mass spectrometry for analyzing and interpreting comprehensive metabolomic characterization of hyperlipemia, RSC Adv., 2016, 6(113), 112534–112543 RSC.
- X. Wang, Y. Han, A. Zhang, et al., Metabolic profiling provides a system for the understanding of Alzheimer's disease in rats post-treatment with Kaixin San, Chinmedomics, Academic Press, 2015, pp. 347–362 Search PubMed.
- A. Zhang, H. Wang and H. Sun, et al., Metabolomics strategy reveals therapeutical assessment of limonin on nonbacterial prostatitis, Food Funct., 2015, 6(11), 3540–3549 RSC.
- J. L. Ren, A. H. Zhang and L. Kong, et al., Advances in mass spectrometry-based metabolomics for investigation of metabolites, RSC Adv., 2018, 8(40), 22335–22350 RSC.
- A. Zhang, H. Sun and S. Qiu, et al., Advancing drug discovery and development from active constituents of yinchenhao tang, a famous traditional chinese medicine formula, Evid.-Based Complementary Altern. Med., 2013, 2013, 257909 Search PubMed.
- X. Wang, Q. Wang and A. Zhang, et al., Metabolomics study of intervention effects of Wen-Xin-Formula using ultra high-performance liquid chromatography/mass spectrometry coupled with pattern recognition approach, J. Pharm. Biomed. Anal., 2013, 74, 22–30 CrossRef CAS PubMed.
- W. Dong, P. Wang and X. Meng, et al., Ultra-performance Liquid Chromatography–High-definition Mass Spectrometry Analysis of Constituents in the Root of Radix Stemonae and those Absorbed in Blood after Oral Administration of the Extract of the Crude Drug, Phytochem. Anal., 2012, 23(6), 657–667 CrossRef CAS PubMed.
- A. Zhang, H. Sun, W. Sun, et al., Metabolomics and Proteomics Annotate Therapeutic Mechanisms of Geniposide, Chinmedomics, Academic Press, 2015, pp. 157–173 Search PubMed.
- S. A. Mirmalek and A. Gholamrezaei Boushehrinejad, et al., Antioxidant and Anti-Inflammatory Effects of Coenzyme Q10 on L-Arginine-Induced Acute Pancreatitis in Rat, Oxid. Med. Cell. Longevity, 2016, 2016, 5818479 Search PubMed.
- P. Sandstrom, L. Trulsson and T. Gasslander, et al., Serum amino acid profile in patients with acute pancreatitis, Amino Acids, 2008, 35(1), 225–231 CrossRef CAS PubMed.
- L. Castillo, Y. M. Yu and J. S. Marchini, et al., Phenylalanine and tyrosine kinetics in critically ill children with sepsis, Pediatr. Res., 1994, 35(5), 580–588 CrossRef CAS PubMed.
- R. Pezzilli, A. M. Morselli-Labate and R. Corinaldesi, et al., NSAIDs and Acute Pancreatitis: A Systematic Review, Pharmaceuticals, 2010, 3(3), 558–571 CrossRef CAS PubMed.
|
This journal is © The Royal Society of Chemistry 2019 |
Click here to see how this site uses Cookies. View our privacy policy here.