DOI:
10.1039/C9RA01561B
(Paper)
RSC Adv., 2019,
9, 18713-18719
Analysis of serum metabolomics among biopsy-proven diabetic nephropathy, type 2 diabetes mellitus and healthy controls†
Received
1st March 2019
, Accepted 10th June 2019
First published on 26th June 2019
Abstract
Type 2 diabetes mellitus (T2DM) has a rising prevalence and diabetic nephropathy (DN) is a major complication of T2DM. Metabolomics could provide novel insights into the pathogenesis, so we aimed to explore serum metabolomic profiles from DN to T2DM. Serum samples were collected from 14 biopsy-proven DNs, 14 age/gender-matched T2DMs without renal diseases (DM), 14 age/gender-matched healthy controls (CTRL) and household contacts of DM group (HH). Serum metabolomics was analyzed by untargeted liquid chromatography-tandem mass spectrometry (LC/MS) assays. There were a total of 1470 metabolites identified from all serum samples. 45 metabolites with significantly different intensity were found between DN and DM, e.g., biliverdin and taurine were reduced while L-arginine was increased in DN comparing to DM. DN could be distinguished from age/gender matched DM patients by L-arginine (AUC = 0.824) or taurine levels (AUC = 0.789). The metabolic pathways affected by metabolite distinctions between DN and DM also existed, among which taurine and hypotaurine metabolism exhibited the highest pathway impact. L-Methionine, deethylatrazine, L-tryptophan and fumaric acid were reduced in DM comparing with those of CTRL, but had no different intensity in DM and HH groups. The changes were demonstrated in the metabolomic profiles of biopsy-proven DN compared to DM. Biopsy-proven DN patients could be distinguished from age/gender matched DM by L-arginine or taurine levels in serum metabolomic profiles. Taurine and hypotaurine metabolism pathway had the highest impact in pathway set enrichment analysis, which potentially affected the pathogenesis of DN from T2DM.
1. Introduction
Type 2 diabetes mellitus (T2DM), with a rising global prevalence ranging from 11.6% to 14%,1–3 is a disorder of elevated glucose levels primarily due to insulin resistance, inadequate insulin resistance or inadequate insulin secretion. Genetic, metabolic and environmental risk factors are known, including diets and lifestyles.4 Metabolomics enable the concomitant measurement of metabolites and further provide novel insights into the pathogenesis of T2DM.5,6 Catabolism of branched-chain amino acids played an important role in T2DM and the branched-chain keto-acid metabolite 3-methyl-2-oxovalerate was a strong predictive biomarker for impaired fasting glucose.5 Additionally, five branched-chain and aromatic amino acids had highly significant associations with diabetes and a combination of three amino acids predicted diabetes.6 These findings underscored the potential role of metabolomics early in the pathogenesis and detection of T2DM.
With the development of experimental instruments and gradual amplification of relative databases, mass spectrometry (MS) coupled with liquid chromatography is increasingly becoming a prevalent and powerful approach for the identification and quantification of metabolites in diseases.7 In particular, the untargeted metabolite profiling, an approach that allows the hypothesis-free assessment of a wide spectrum of metabolites resulting from endogenous metabolism, dietary intake and gut microbial activity,8 has the potential to broaden new discoveries related to the pathogenesis of diseases. Using untargeted liquid chromatography-tandem mass spectrometry (LC/MS), several metabolites were found to be associated with lower likelihood of developing T2DM while indolepropionic acid was associated with reduced likelihood of T2DM.9
Diabetic nephropathy (DN), a major microvascular complication of diabetes mellitus, is the most common cause of end-stage renal diseases (ESRD) worldwide.10–12 Kidney diseases developed in almost 35% of patients with T2DM13 and is associated with the increased mortality.14 The definition of DN is generally based on the changes in albuminuria and glomerular filtration rate (GFR), which does not exclude non-diabetes causes of renal disease in patients with diabetes mellitus, unless pathologically confirmed.15 Using capillary electrophoresis-mass spectrometry (CE-MS), identified metabolites could distinguish between DN with macroalbuminuria and diabetic patients without albuminuria.16 However, no study has investigated serum metabolomic differences biopsy-proven DN between T2DM, and the metabolic basis together with early molecular events related to the onset of DN are still poorly understood. Therefore, there is a need to utilize novel technologies to explore the understanding and improve the potential for early detection.
In the study, our aims were to explore serum metabolomics related to the difference between biopsy-proven DN and T2DM, and to assess potential impacts of diets and lifestyles on serum metabolites by applying untargeted LC/MS assay.
2. Materials and methods
2.1. Study participants and blood sample collection
At West China Hospital, Chengdu, China, we enrolled T2DM patients with DN confirmed by renal biopsy from March 2017 to January 2018, with estimated glomerular filtration rate (eGFR) > 60 mL min−1/1.73 m2 and urine albumin creatinine ratio (UACR) ≥ 30 mg g−1 as group DN.17 We also enrolled T2DM patients without renal disease (UACR < 30 mg g−1 and eGFR > 60 mL min−1/1.73 m2),17,18 matched for gender and age as DM group. We included two control groups composed of gender and age-matched healthy subjects (CTRL), as well as household contacts on the same diet of each patient on DM group (HH). Each group consisted of 14 subjects. Blood samples were collected from each participant using empty tubes. The tubes were then immediately centrifuged at 3000 rpm for 15 minutes and the serum obtained was stored at −80 °C until analysis.19 All the participants gave their written informed consent for participation in the study. The study protocol was approved by Biomedical Ethics Committee of West China Hospital of Sichuan University (no. 2016-273). All the procedures followed the Declaration of Helsinki principles.
2.2. Metabolites extraction
Thawed at 4 °C, 200 μL of each sample and 800 μL of methanol was transferred into centrifuge tubes and mixed by vertexing for 60 s. After centrifuging at 12
000 rpm at 4 °C for 10 min, all supernatant in each tube was transferred into another tubes. Blow-dried by vacuum concentration, samples were dissolved with 300 μL methanol aqueous solution (4
:
1, 4 °C) and filtered through a 0.22 μm membrane.20,21 The prepared sample extracts for LC/MS were then obtained. 20 μL of each prepared sample extraction was taken for the quality control (QC) samples and the rest were for LC/MS test.
2.3. LC/MS conditions
Chromatographic separation was accomplished in an ACQUITY UPLC system equipped with an ACQUITY UPLC BEH C18 column (100 × 2.1 mm, 1.7 μm, Waters, USA) maintained at 4 °C. The temperature of the autosampler was 4 °C. Gradient elution of analytes was carried out with 0.1% formic acid in water (A) and 0.1% formic acid in acetonitrile (B) at a flow rate of 0.25 mL min−1. Injection of 5 μL of each sample was done after equilibration. An increasing linear gradient of solvent B (v/v) was used as follows: 0–1 min, 2% B; 1–9.5 min, 2%∼50% B; 9.5–14 min, 50%∼98% B; 14–15 min 98% B; 15–15.5 min, 98%∼2% B; 15.5–17 min, 2%.22 The ESI-MS experiments were executed on the Thermo LTQ-Orbitrap XL mass spectrometer with the spray voltage of 4.8 kV and −4.5 kV in positive and negative modes, respectively. Sheath gas and auxiliary gas were set at 45 and 15 arbitrary units, respectively. The capillary temperature was 325 °C. The voltages of capillary and tube were 35 V and 50 V, −15 V and −50 V in positive and negative modes, respectively. The Orbitrap analyzer scanned over a mass range of m/z 89–1000 for full scan at a mass resolution of 60
000. Data dependent acquisition (DDA) MS/MS experiments were performed with CID scan. The normalized collision energy was 30 eV. Dynamic exclusion was implemented with a repeat count of 2, and exclusion duration of 15 s.23
2.4. Data processing
The raw data is converted to mzXML format using Proteowizard software (v 3.0.8789).24 All statistical analyses were made in the R software (v 3.3.2). The relative standard deviation (RSD) of potential characteristic peaks in QC samples, i.e. the coefficient of variation (CV) should not exceed 30%, if exceeded, the relevant characteristic peaks should be deleted. For samples with missing values for a metabolite, a cutoff point of 50 for both negative and positive ion modes was applied to the untargeted measurements. For QC samples, metabolites with over 50% missing values were cut off. Missing values were supplemented using k-nearest neighbor algrorithm.25 The data was normalized by the median values. Significant differences in metabolite levels were assessed by Wilcox test. The correction for multiple comparisons in the metabolic analysis was performed using the Benjamin and Hochberg method. The metabolomic measurement was considered to be significantly different if P < 0.05.
3. Results
3.1. General characteristics of all participants
We included 14 DN patients confirmed by renal biopsy with a median age of 52.9 years. All biopsy-proven DN patients were with eGFR > 60 mL min−1/1.73 m2 and UACR ≥ 30 mg g−1. Baseline characteristics of DN, DM and two control groups (CTRL and HH groups) are summarized in Table 1.
Table 1 Baseline characteristics of participantsa,b
|
DN (n = 14) |
DM (n = 14) |
CTRL (n = 14) |
HH (n = 14) |
P value |
DN, diabetic nephropathy; DM, type 2 diabetes mellitus without renal diseases; CTRL, healthy controls; HH, households; T2DM, type 2 diabetes mellitus; HbA1c, glycosylated hemoglobin; UACR, urine albumin creatinine ratio; eGFR, estimated glomerular filtration rate. One-way ANOVA was used to compare continuous variables (age, body mass index, fasting blood glucose, HbA1c, serum creatinine, UACR, eGFR) and chi-square was used to compare categorical variables (gender, smoking/stroke/heart diseases history, hypertension). |
Age, years |
52.93 ± 9.98 |
53.29 ± 9.00 |
52.86 ± 9.91 |
44.29 ± 17.31 |
0.149 |
Gender, female/male |
5/9 |
5/9 |
5/9 |
10/4 |
0.144 |
Body mass index, kg m−2 |
27.47 ± 3.63 |
25.01 ± 2.00 |
24.47 ± 2.47 |
25.33 ± 4.19 |
0.08 |
Course of T2DM, years |
10.29 ± 5.36 |
3 (3, 7.25) |
— |
— |
— |
Smoking history, yes/no |
6/8 |
7/7 |
5/9 |
4/10 |
0.683 |
Hypertension, yes/no |
14/0 |
6/8 |
2/12 |
1/13 |
<0.001 |
Stroke history, yes/no |
3/11 |
1/13 |
0/14 |
0/14 |
0.091 |
Heart diseases history, yes/no |
2/12 |
3/11 |
0/14 |
0/14 |
0.115 |
Fasting blood glucose, mmol L−1 |
8.86 ± 4.63 |
8.18 ± 3.89 |
4.9 ± 0.81 |
5.95 ± 2.15 |
0.006 |
HbA1c, % |
7.73 ± 1.47 |
7.36 ± 1.89 |
5.86 ± 0.26 |
5.91 ± 1.24 |
<0.001 |
Serum creatinine, μmol L−1 |
73.93 ± 20.2 |
73.71 ± 14.08 |
73.07 ± 10 |
60.14 ± 8.75 |
0.03 |
UACR, mg g−1 |
2280.84 ± 2116.68 |
12.09 ± 8.56 |
10.49 ± 8.65 |
19.50 ± 6.90 |
<0.001 |
eGFR, mL min−1/1.73 m2 |
93.26 ± 17.01 |
93.48 ± 13.02 |
96.01 ± 9.29 |
106.92 ± 16.05 |
0.173 |
3.2. Global metabolite profiling
The PCA score plots and quality assurance (QA) results in the negative and positive ion modes were showed in ESI Fig. 1.† A total of 1470 kinds of metabolites (17 in negative ion mode, 1453 in positive ion mode) were detected. To investigate the global metabolite profiles, we used FDR-corrected P value < 0.01 as the threshold values of statistical significance in the study.
Compared with CTRL, 9 metabolites were present in higher levels in DM samples, whereas 8 metabolites were lower in DM samples in negative ion mode. The numbers of metabolites detected at higher and lower levels in the DM group were 179 and 1285 in positive ion mode, respectively. Compared with DM, 9 metabolites were found at higher levels in the DN sera, while 7 metabolites were lower in DN samples in negative ion mode. The numbers of metabolites at higher and lower levels in the DN group were 1315 and 148 in positive ion mode, respectively (Fig. 1). Orthogonal Projections to Latent Structures Discriminant Analysis (OPLS-DA) in both negative and positive ion modes were performed (Fig. 2).
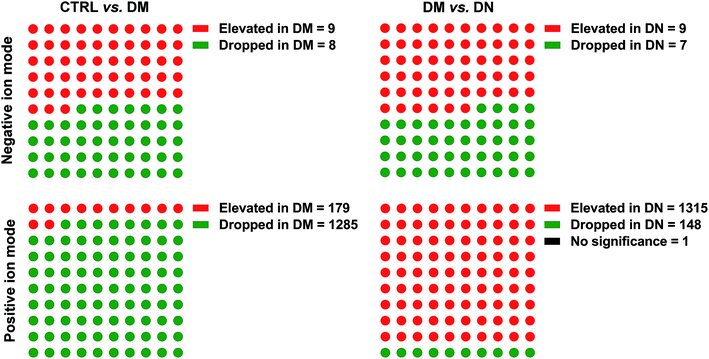 |
| Fig. 1 Metabolites between CTRL/DM, and DM/DN groups. DN, diabetic nephropathy; DM, type 2 diabetes mellitus without renal diseases; CTRL, healthy controls. | |
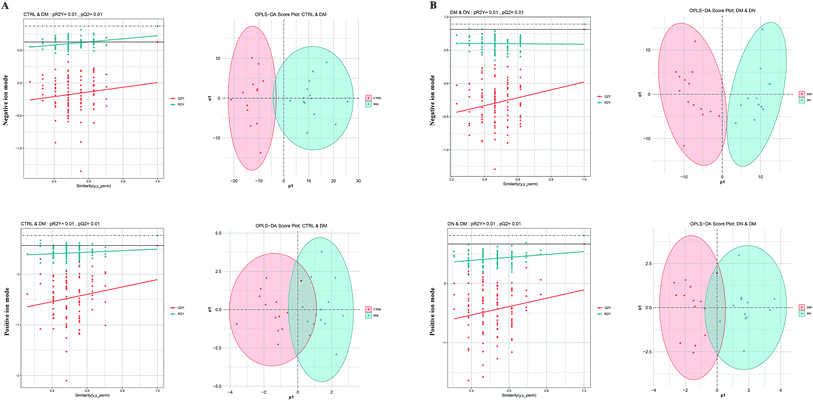 |
| Fig. 2 OPLS-DA between CTRL/DM and DM/DN groups in both negative and positive ion modes. (A) OPLS-DA between CTRL and DM in both negative and positive ion modes; (B) OPLS-DA between DM and DN in both negative and positive ion modes. OPLS-DA, Orthogonal Projections to Latent Structures Discriminant Analysis; DN, diabetic nephropathy; DM, type 2 diabetes mellitus without renal diseases; CTRL, healthy controls. | |
3.3. The metabolite difference between DN and DM
A total of 45 metabolites with significantly different intensity between DN and DM were found. The heatmap revealed the differential metabolites between DN and DM (Fig. 3A). As shown in the heatmaps and box plots (Fig. 3B–D), the contents of biliverdin and taurine were reduced in the DN samples, comparing with those of DM samples. However, L-arginine level was increased in the DN group comparing with DM group. The identification graphs for above-mentioned three metabolites were presented in ESI Fig. 2.†
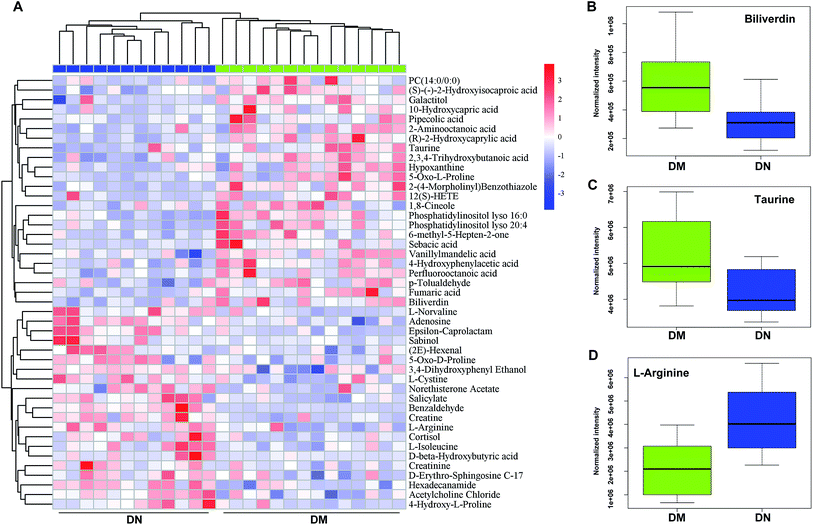 |
| Fig. 3 The differential metabolites between DN and DM. (A) Heatmap showing differential metabolites between DN and DM. (B) Biliverdin levels in DN and DM; (C) taurine levels in DN and DM; (D) L-arginine levels in DN and DM. DN, diabetic nephropathy; DM, type 2 diabetes mellitus without renal diseases. | |
To illustrate the metabolomic signature of DN and further explore the potential of serum metabolomics in DN identification, the receiver operating characteristics (ROC) curve for classifying DN from DM patients was developed. DN individuals could be accurately detected by L-arginine levels (AUC = 0.824) or taurine levels (AUC = 0.789) (Fig. 4A and B). The pathway set enrichment analyses were performed using Metabolanalyst (www.metabolanalyst.ca) to elucidate the metabolic pathways affected by metabolite distinctions between DN and DM. The analysis between DN and DM groups revealed the perturbation of 25 networks, among which taurine and hypotaurine metabolism exhibited the highest pathway impact (Fig. 4C, ESI Table 1†).
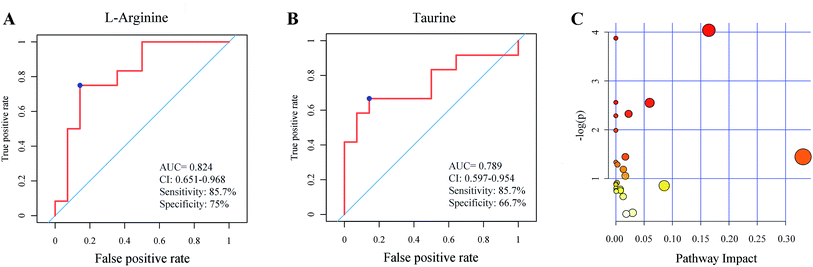 |
| Fig. 4 Classifications to identify DN and DM. (A) ROC curve classifying DN from DM, based on L-arginine levels; (B) ROC curve classifying DN from DM, based on taurine levels; (C) metabolic pathways affected by metabolite distinctions between DN and DM. DN, diabetic nephropathy; DM, type 2 diabetes mellitus without renal diseases. | |
3.4. The metabolomics among DM, households (HH) and age/gender matched healthy controls (CTRL)
Seen from the heatmap showing the differential metabolites among DM, HH and CTRL groups (Fig. 5), L-methionine, deethylatrazine, L-tryptophan and fumaric acid were significantly reduced in DM samples comparing with those of CTRL. However, these metabolites had no different intensity in the DM group and their healthy households (HH), who shared the dietary habits and lifestyles.
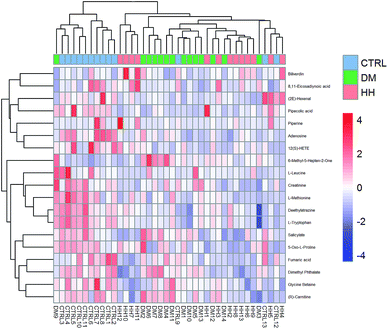 |
| Fig. 5 Heatmap showing differential metabolites among DM, HH, CTRL groups. DM, type 2 diabetes mellitus without renal diseases; CTRL, healthy controls; HH, households. | |
4. Discussion
In the study, we demonstrated the significant changes in the serum metabolomic profiles between renal biopsy-proven DN and DM patients. Importantly, DN could be distinguished from age and gender matched DM patients by L-arginine levels (AUC = 0.824) or taurine levels (AUC = 0.789). The metabolic pathways affected by metabolite distinctions between renal biopsy-proven DN and DM also existed, among which taurine and hypotaurine metabolism had the highest pathway impact. As DM and DN not only imply the disease itself, but goes along with dietary habits and lifestyles, metabolites from sera of DM patients were compared with both age/gender-matched healthy controls and households with the same diet and lifestyles. Interestingly, metabolites like L-methionine, deethylatrazine, L-tryptophan and fumaric acid were significantly reduced in DM comparing with CTRL, but had no different intensity in DM patients and their healthy households.
Previously, metabolic alterations in serum including sugar metabolites, amino acids and choline-containing phospholipids, were proved to be associated with higher risks of T2DM.26 Niewczas et al.27 found that abnormal plasma concentrations of putative uremic solutes and essential amino acids might contribute to the progression to ESRD in T2DM. Compared with DM-CKD cohort, multiple urine metabolites were significantly changed in the DM + CKD cohort.28 Considering dietary habits, certain dietary modification was reported to alter serum metabolic profiles.29 Floegel et al.30 once suggested that special dietary patterns were found related to T2DM-relevant metabolites.
In the diabetic state, high glucose and activation of local renin–angiotensin systems may induce the rapid activation of NADPH oxidase in renal tissues of diabetic patients and animals.31 Administration of biliverdin was demonstrated to protect against both albuminuria and renal mesangial expansion in db/db mice,32 and the protection against diabetic nephropathy was through the inhibition of oxidative stress.33–35 Treating diabetic rats with taurine could further suppress the progression of nephropathy, reduce urinary protein excretion, mesangial extracellular matrix expansion and transforming growth factor-α (TGF-α) expression in renal glomerulus.36 Lin et al.37 followed the same conclusion that by decreasing blood glucose level, improving lipid metabolism, glomerular basement membrane (GBM) metabolism and kidney function, taurine could prevent the development of nephropathy. Furthermore, taurine was demonstrated to decrease UACR and ameliorate the glomerular volume, GBM thickness and the numbers of open slit pores38 in type 2 diabetic rats, whose nephroprotective effect is due to its antioxidant property, the recovery in nephron and reduction in renal vascular endothelial growth factor (VEGF) expression. Taurine was previously proved to reduce renal NAPDH oxidase activity39 and suppress xanthine oxidase activity.40 Moreover, Han et al.'s study41 strongly suggested that the malfunction of the taurine transporter found in diabetes patients was a key risk factor for developing diabetic nephropathy. Studies also showed that taurine could prevent both high glucose-induced hypertrophic growth in renal tubular epithelial cells42,43 and reduce apoptosis in human tubular cells.44 All above studies possibly explained the reduction of biliverdin and taurine levels in the DN samples. Additionally, hypotaurine, an intermediate in the biosynthesis of taurine,45 was found to possess protective effects against membrane damage and normalize morphology of erythrocytes in type 2 diabetic rats.46 Taken together, taurine and hypotaurine metabolism may play a vital role in the progression to DN.
Arginine is metabolized through two pathways: the arginase pathway and the nitric oxide synthase (NOS) pathway.47 Overactivity of arginase causes a shortfall of arginine for the NOS pathway.48 Increased renal arginase activity and reduced NOS expression was confirmed in diabetes.49 Endothelial dysfunction associated with reduced NO bioavailability has been strongly indicated as a critical factor in DN.50 You et al.51 proved that L-arginine supplementation failed to have any beneficial effect on DN model. Their results indicated that uptake of L-arginine in affected renal cells may be impaired. They also speculated the production of downstream metabolites, rather than arginine depletion is important in DN pathology. It raised a concern that the increase in L-arginine could be detrimental, which is consistent with our findings.
It was demonstrated that the serum changes of methionine were a consequence of changing diets or lifestyles.52 Our results indicated that the changes of deethylatrazine, L-tryptophan and fumaric acid in serum may be also due to the different diets or lifestyles.
To our knowledge, this is the first study indicating the relationship between serum metabolomics and the difference to DN from T2DM. All the enrolled DN patients with eGFR > 60 mL min−1/1.73 m2 were diagnosed by renal biopsy, aiming to exclude the impact of renal function.28 Age and gender-matched DM groups were enrolled to minimize confounding factors. Additionally, we compared DM patients with both age and gender-matched healthy controls and households, to evaluate if diets or lifestyles exerted impact on certain metabolites. However, there are limitations to our study. Although we tried to minimize multiple confounding factors, serum metabolites can still be affected unavoidably by many factors. Our study population consist of patients of Chinese origin and sample size was limited due to strict inclusion criteria. Care should be taken to extrapolate our results. Additionally, the sample size in our study was limited, further studies with larger sample sizes are required. Moreover, we inferred that metabolite profiling may reveal underlying physiology of DN, mainly based on speculations. Thus, the role of metabolites in DN could possibly be overestimated. Further verifications are needed.
5. Conclusions
We demonstrated the changes in the metabolomic profiles in biopsy-proven DN compared to DM. Biliverdin and taurine were reduced while L-arginine was increased in DN comparing to DM. Importantly, DN could be accurately distinguished from age and gender matched DM patients by L-arginine levels (AUC = 0.824) or taurine levels (AUC = 0.789) in serum. The metabolic pathways affected by metabolite distinctions between DN and DM also existed, among which taurine and hypotaurine metabolism had the highest pathway impact. It implied that taurine and hypotaurine metabolism may affect the pathogenesis of DN from T2DM. L-Methionine, deethylatrazine, L-tryptophan and fumaric acid were significantly reduced in DM samples comparing with those of CTRL but had no different intensity in their healthy households (HH), which means those metabolites might be affected by dietary habits and lifestyles.
Conflicts of interest
There are no conflicts to declare.
Acknowledgements
This study is supported by National Key Research & Development Program of China (2016YFC1305403), National Natural Science Foundation of China (81700634) and Program of State Key Laboratory of Kidney Diseases in PLA General Hospital (KF-01-141).
References
- H. Caspard, S. Jabbour and N. Hammar, et al., Diabetes, Obes. Metab., 2018, 20(3), 667–671 CrossRef PubMed
. - R. C. Ma, X. Lin and W. Jia, Lancet Diabetes Endocrinol., 2014, 2(12), 980–991 CrossRef PubMed
. - T. Pitts-Tucker, Br. Med. J., 2012, 345, e6135 CrossRef PubMed
. - E. S. Schellenberg, D. M. Dryden, B. Vandermeer, C. Ha and C. Korownyk, Ann. Intern. Med., 2013, 159(8), 543–551 CrossRef PubMed
. - C. Menni, E. Fauman and I. Erte, et al., Diabetes, 2013, 62(12), 4270–4276 CrossRef CAS PubMed
. - T. J. Wang, M. G. Larson and R. S. Vasan, et al., Nat. Med., 2011, 17(4), 448–453 CrossRef CAS PubMed
. - X. Zhao, Z. Zeng and A. Chen, et al., Anal. Chem., 2018, 90(12), 7635–7643 CrossRef CAS PubMed
. - K. Hanhineva, M. A. Lankinen and A. Pedret, et al., J. Nutr., 2015, 145(1), 7–17 CrossRef CAS PubMed
. - V. D. de Mello, J. Paananen and J. Lindstrom, et al., Sci. Rep., 2017, 7, 46337 CrossRef CAS PubMed
. - R. S. Barsoum, N. Engl. J. Med., 2006, 354(10), 997–999 CrossRef CAS PubMed
. - P. Du, B. Fan and H. Han, et al., Kidney Int., 2013, 84(2), 265–276 CrossRef CAS PubMed
. - L. Zhang, J. Long and W. Jiang, et al., N. Engl. J. Med., 2016, 375(9), 905–906 CrossRef PubMed
. - I. H. de Boer, T. C. Rue, Y. N. Hall, P. J. Heagerty, N. S. Weiss and J. Himmelfarb, J. Am. Med. Assoc., 2011, 305(24), 2532–2539 CrossRef CAS PubMed
. - M. Afkarian, M. C. Sachs and B. Kestenbaum, et al., J. Am. Soc. Nephrol., 2013, 24(2), 302–308 CrossRef CAS PubMed
. - D. Fineberg, K. A. Jandeleit-Dahm and M. E. Cooper, Nat. Rev. Endocrinol., 2013, 9(12), 713–723 CrossRef CAS PubMed
. - A. Hirayama, E. Nakashima and M. Sugimoto, et al., Anal. Bioanal. Chem., 2012, 404(10), 3101–3109 CrossRef CAS PubMed
. - A. S. Levey, P. E. de Jong and J. Coresh, et al., Kidney Int., 2011, 80(1), 17–28 CrossRef PubMed
. - G. L. Bakris, R. Agarwal and J. C. Chan, et al., J. Am. Med. Assoc., 2015, 314(9), 884–894 CrossRef CAS PubMed
. - M. A. Fernandez-Peralbo, F. Priego-Capote, M. D. Luque de Castro, A. Casado-Adam, A. Arjona-Sanchez and F. C. Munoz-Casares, J. Pharm. Biomed. Anal., 2014, 91, 131–137 CrossRef CAS PubMed
. - W. B. Dunn, D. Broadhurst and P. Begley, et al., Nat. Protoc., 2011, 6(7), 1060–1083 CrossRef CAS PubMed
. - E. Zelena, W. B. Dunn and D. Broadhurst, et al., Anal. Chem., 2009, 81(4), 1357–1364 CrossRef CAS PubMed
. - T. Sangster, H. Major, R. Plumb, A. J. Wilson and I. D. Wilson, Analyst, 2006, 131(10), 1075–1078 RSC
. - E. J. Want, P. Masson and F. Michopoulos, et al., Nat. Protoc., 2013, 8(1), 17–32 CrossRef CAS PubMed
. - C. A. Smith, E. J. Want, G. O'Maille, R. Abagyan and G. Siuzdak, Anal. Chem., 2006, 78(3), 779–787 CrossRef CAS PubMed
. - D. Li, H. Gu and L. Zhang, Expert Syst. Appl., 2010, 37(10), 6942–6947 CrossRef
. - A. Floegel, N. Stefan and Z. Yu, et al., Diabetes, 2013, 62(2), 639–648 CrossRef CAS PubMed
. - M. A. Niewczas, T. L. Sirich and A. V. Mathew, et al., Kidney Int., 2014, 85(5), 1214–1224 CrossRef CAS PubMed
. - K. Sharma, B. Karl and A. V. Mathew, et al., J. Am. Soc. Nephrol., 2013, 24(11), 1901–1912 CrossRef CAS PubMed
. - M. Lankinen, U. Schwab and P. V. Gopalacharyulu, et al., Nutr. Metab. Cardiovasc. Dis., 2010, 20(4), 249–257 CrossRef CAS PubMed
. - A. Floegel, A. von Ruesten and D. Drogan, et al., Eur. J. Clin. Nutr., 2013, 67(10), 1100–1108 CrossRef CAS PubMed
. - M. Kitada, D. Koya and T. Sugimoto, et al., Diabetes, 2003, 52(10), 2603–2614 CrossRef CAS PubMed
. - M. Fujii, T. Inoguchi and S. Sasaki, et al., Kidney Int., 2010, 78(9), 905–919 CrossRef CAS PubMed
. - L. Li, L. Ma and P. Fu, Drug Des., Dev. Ther., 2017, 11, 3531–3542 CrossRef CAS PubMed
. - S. Tao, L. Li and L. Li, et al., Acta Diabetol., 2019, 56(5), 581–592 CrossRef PubMed
. - L. Li, S. Tao and L. Ma, et al., Chin. Med. J., 2019, 132(10), 1228–1232 CrossRef PubMed
. - S. Higo, S. Miyata, Q. Y. Jiang, R. Kitazawa, S. Kitazawa and M. Kasuga, Kobe J. Med. Sci., 2008, 54(1), E35–E45 Search PubMed
. - S. Lin, J. Yang and G. Wu, et al., J. Biomed. Sci., 2010, 17(1), S46 CrossRef PubMed
. - J. H. Koh, E. S. Lee and M. Hyun, et al., Int. J. Endocrinol., 2014, 2014, 397307 Search PubMed
. - K. Winiarska, K. Szymanski, P. Gorniak, M. Dudziak and J. Bryla, Biochimie, 2009, 91(2), 261–270 CrossRef CAS PubMed
. - J. Das, V. Vasan and P. C. Sil, Toxicol. Appl. Pharmacol., 2012, 258(2), 296–308 CrossRef CAS PubMed
. - X. Han, T. Ito, J. Azuma, S. W. Schaffer and R. W. Chesney, Adv. Exp. Med. Biol., 2015, 803, 217–226 CrossRef CAS PubMed
. - J. S. Huang, L. Y. Chuang, J. Y. Guh and Y. J. Huang, Toxicol. Sci., 2009, 111(1), 109–119 CrossRef CAS PubMed
. - J. S. Huang, L. Y. Chuang, J. Y. Guh, Y. L. Yang and M. S. Hsu, Toxicol. Appl. Pharmacol., 2008, 233(2), 220–226 CrossRef CAS PubMed
. - D. Verzola, M. B. Bertolotto and B. Villaggio, et al., J. Invest. Med., 2002, 50(6), 443–451 CrossRef CAS PubMed
. - P. Sarkar, P. Basak, S. Ghosh, M. Kundu and P. C. Sil, Food Chem. Toxicol., 2017, 110, 109–121 CrossRef CAS PubMed
. - D. Gossai and C. A. Lau-Cam, Adv. Exp. Med. Biol., 2009, 643, 359–368 CrossRef CAS PubMed
. - S. P. Narayanan, Z. Xu and N. Putluri, et al., Cell Death Dis., 2014, 5, e1075 CrossRef CAS PubMed
. - G. Liew, Z. Lei and G. Tan, et al., Curr. Diab. Rep., 2017, 17(11), 102 CrossRef PubMed
. - S. M. Morris. Jr., T. Gao, T. K. Cooper, D. Kepka-Lenhart and A. S. Awad, Diabetes, 2011, 60(11), 3015–3022 CrossRef PubMed
. - M. A. Creager, T. F. Luscher, F. Cosentino and J. A. Beckman, Circulation, 2003, 108(12), 1527–1532 CrossRef PubMed
. - H. You, T. Gao, T. K. Cooper, S. M. Morris. Jr. and A. S. Awad, Am. J. Physiol.: Renal, Fluid Electrolyte Physiol., 2014, 307(11), F1292–F1301 CAS
. - T. Reinehr, B. Wolters and C. Knop, et al., Eur. J. Nutr., 2015, 54(2), 173–181 CrossRef CAS PubMed
.
Footnotes |
† Electronic supplementary information (ESI) available. See DOI: 10.1039/c9ra01561b |
‡ The authors contributed equally to the work. |
|
This journal is © The Royal Society of Chemistry 2019 |