DOI:
10.1039/C9RA00252A
(Paper)
RSC Adv., 2019,
9, 11707-11717
iTRAQ based proteomic analysis of PM2.5 induced lung damage
Received
11th January 2019
, Accepted 29th March 2019
First published on 15th April 2019
Abstract
Haze pollution has become a global environmental problem, subsequently affecting air quality, climate, economy and human health. Notably, PM2.5 (particulate matter with an aerodynamic diameter less than 2.5 micrometers) significantly accounts for a variety of adverse health effects, in particular pulmonary diseases such as asthma and lung cancer. Clinical diagnosis and medical treatment of the lung damage caused by PM2.5 still remain significant challenges due to the lack of specific biomarkers and pathways. Here, we established a rat model of nonsurgical intratracheal instillation to investigate PM2.5 exposure and employed iTRAQ based analytical technique and bioinformatics tools to identify putative biomarkers and pathways. We identified 163 differentially expressed proteins (DEPs). Among these proteins, we screened six DEPs (HMOX1, MP2K5, XRCC1 E9PTZ7, KNT2 and A1AG) as the putative biomarkers, with significant differentially expressed levels (percentage increment > 140%). Pathway analysis indicated that calcium signaling, MAPK and PI3K/AKT might be involved in the process of PM2.5-induced lung damage. Western-blotting was used to verify DEPs in the AEC-II cell model for early diagnosis. In summary, our data can serve as fundamental research clues for further studies of PM2.5-induced toxicity in the lungs.
1. Introduction
In recent years, haze pollution has caused an extremely severe environmental crisis in developing countries, especially in China.1 Among all kinds of carcinogens, particulate matter with an aerodynamic diameter of less than 2.5 μm (PM2.5) shows the most adverse effects on health, attracting an increasing number of global concerns.2,3 More than 800 million people are exposed to haze pollution, and approximately 7 million premature deaths happened in 2012.4,5 A growing number of investigations demonstrated that the morbidity and mortality of lung cancer was significantly increased due to haze pollution.6,7 In detail, an increase of 10 μg m−3 of PM2.5 produced an increment of 4–6% overall mortality, 10% cardiovascular disease prevalence and 22% lung cancer prevalence.8,9 In particular, in North China cities such as Beijing and Tianjin haze pollution is much more serious than that in South China cities such as Shanghai and Guangzhou, resulting in life expectancy in North China being about 5.5 years lower than in South China.10
Although all parties have made a lot of efforts, for example, the Chinese State Council set the goal to reduce the concentration of PM2.5 to 60 μg m−3 by 2017,11 billions of people still suffer from a series of lung diseases caused by haze pollution. Therefore, the diagnostic and therapeutic solutions for PM2.5-induced lung damage in the early stage are urgently needed. However, traditional diagnostic methods such as multiple CT scans and lung biopsies are risky and only available for advanced stage.12 On the other hand, clinical treatments for lung cancer at advanced stage including surgery, radiation and chemotherapy are less effective and have side effects.13 To address these challenges, this paper combines a set of novel analytical methods and bioinformatics tools to screen differentially expressed proteins (DEPs), analyze these DEPs and construct pathways. We established a rat model of nonsurgical intratracheal instillation and employed Isobaric Tags for Relative and Absolute Quantitation (iTRAQ) coupled with LC-MS/MS to analyze DEPs. iTRAQ based proteomics has proved to be one of the state-of-the-art quantitative techniques for protein profiling.14–16 We profiled DEPs of PM2.5 exposed lung tissue, identified significant DEPs as biomarkers and further analyzed biological maps of pathways. Western-blot method was used to determine the relative expression of DEPs such as HMOX1, MP2K5, E9PTZ7, XRCC1, A1AG and KNT2.
2. Materials and methods
2.1 PM2.5 collection
PM2.5 samples were collected by using three air samplers simultaneously in Tianjin, China, from June 2014 to January 2015. PM2.5 samples were dispersed in MQ water from Millipore Sigma (St. Louis, MO, USA), sonicated for 20 minutes, then filtered through six layers of sterile gauze, centrifuged and lyophilized. 300 samples were collected. Prior to use, PM2.5 was dispersed in sterilized 0.9% physiological saline.
2.2 PM2.5 exposure
Healthy adult, clean-grade male SD rats, weighing 180–200 g, were purchased from the Animal Center of Tianjin and were acclimated for one week. The rats were housed in metallic cages under standard conditions (24 ± 2 °C and 50 ± 5% humidity). We randomly divided rats into two groups, control group and PM2.5 group, and each group contained 10 rats. We employed a nonsurgical intratracheal instillation method to investigate PM2.5 exposure.17,18 The rats in PM2.5 group were intratracheally instilled with 15.0 mg per kg b.w. PM2.5, while rats in control group were treated with the same does of saline by intratracheal instillation. The instillation was performed once every 5 days. All rats received regular food and water during the whole period of the experiment. Rats were fasted 24 hours after the last instillation and then killed with aortic blood method.19,20 After the chest cavity was opened, lung tissues were harvested, then quick-frozen and stored at −80 °C. All animal procedures were performed in accordance with the Guidelines for Care and Use of Laboratory Animals of Tianjin University and approved by the Animal Ethics Committee of China Research Institute of Medical Biology.
2.3 Histological evaluation
Harvested lung tissues were excised into small pieces and immediately fixed in 4% paraformaldehyde in phosphate-buffered saline (pH 7.4) for hematoxylin/eosin (H&E) staining.21 After paraffin embedding, sections were H and E stained, and pathomorphological lung changes were photographed using a digital camera mounted on a light microscope (BX 51; Olympus, Tokyo, Japan). Pathology scores were based on pulmonary interstitial edema, alveolar edema, inflammatory cell infiltration, alveolar haemorrhage, hyaline membrane formation, pulmonary atelectasis etc.
2.4 Protein extraction and digestion
The lung tissues were powdered with liquid nitrogen and sonicated in urea lysis buffer (50 mM Tris 8.0, 8 M urea, 1% NP40, 1% sodium deoxycholate, 2 mM EDTA, 5 mM DTT and 1/100 protease inhibitor cocktail, Solarbio, Beijing, China). After centrifugation, the protein concentration in the supernatant was measured using the 2-D Quant Kit from GE Healthcare (Little Chalfont, Buckinghamshire, UK). The protein was reduced with 5 mM DTT, alkylated with 30 mM IAM and precipitated with ice-cold acetone. Protein precipitate was redissolved in 0.1 M TEAB from Sigma-Aldrich (St. Louis, MO, USA) and digested with 1/25 trypsin at 37 °C overnight. The ultimate peptide was desalted with Strata X C18 SPE column (Los Angeles, California, USA) and vacuum-dried.
2.5 iTRAQ labeling
The dried peptide was reconstituted in 20 μL 0.5 M TEAB and processed by following the manufacturer's protocol (iTRAQ 8plex kit, AB Sciex). Briefly, iTRAQ reagent was thawed and reconstituted in 70 μL acetonitrile. Peptide and reagent were mixed and incubated at room temperature for 1 hour and then dried by vacuum centrifugation.
2.6 Pre-separation of tryptic peptides
The labeled peptide was mixed and fractionated with LC20AD HPLC. Then, peptide was reconstituted in buffer A (100% H2O, pH 10.0) and loaded into a column (4.6 × 250 mm, XBridge Shield C18 RP, Waters). The peptide was eluted at a flow rate of 1 mL min−1 with a gradient of 5–12% buffer B (80% ACN, pH 10.0) for 20 min, 12–35% buffer B for 45 min, 35–80% buffer B for 5 min. Subsequently, the system was maintained in 80% buffer B for 5 min before equilibrating with 5% buffer B. The elution was monitored by measuring absorbance at 214 nm, and fractions were collected every 1 min. The eluted peptide was combined into 20 fractions and dried under vacuum.
2.7 LC/MS analysis
The lyophilized peptide was resuspended in buffer A (2% ACN, 0.1% FA), loaded onto an Acclaim PepMap 100 C18 trap column (75 μm × 2 cm, Dionex, Waltham, MA, USA) with Ultimate 3000 nanoUPLC (Dionex) and eluted onto an Acclaim PepMap RSLC C18 analytical column (Dionex, 75 μm × 25 cm). Buffer A linear gradient was run at 300 nL min−1 for 45 min, starting from 11% to 20% buffer B, followed by 2 min gradient to 80% buffer B, and maintained at 80% buffer B for 3 min. The peptide was subjected to NSI source in mass spectrometry Q Exactive plus (Waltham, MA, USA) coupled online to the UPLC. MS1 spectra were acquired at the resolution of 70
000 (at 200 m/z) with an AGC (automatic gain control) of 3
000
000 and a max IT of 250 ms. MS/MS data were acquired at the resolution of 17
500 through isolation windows of 2 Da, and the AGC and max IT were set to 100
000 and 100 ms, the NCE (normalized collision energy) was set to 28%. And the loop count was set to 15 which means 15 MS/MS scans would be acquired between each full scan.
2.8 Protein identification
Protein identification and quantification were performed using the Paragon Algorithm and further process using the Pro Group™ algorithm were implemented in Protein Pilot™ v4.0 software (ABI-MDS Sciex). The raw data were converted to mascot generic file using Proteome Discoverer (Waltham, MA, USA) and processed with Mascot search engine (Matrix Science, v.2.3.02). Tandem mass spectra were searched against UniProt rat reference proteome database (32
983 sequences). The mass error was set to 10 ppm for precursor ions and 0.02 Da for fragment ions. Carbamidomethyl (C), iTRAQ 8plex (K and N-term) was specified as fixed modification, oxidation (M) and iTRAQ 8plex (Y) was specified as variable modification. The decoy (reverse) database was searched to estimate the false discovery rate (FDR). The results were revalued by algorithm percolator, and PSMs (peptide-spectrum match) with unused score of >1.3 corresponded to a peptide confidence level of >95%, and a p-value < 0.05. Notably particularly, for quantitation, a protein must have at least two unique peptides above identity. The protein ratio type was weighted, the normalization method was median.
2.9 Bioinformatics analysis
Identified proteins were assigned as biological processes based on Gene Ontology (GO). Protein classification and analysis were performed with evolutionary relationships (PANTHER), a classification system v 9.0 (http://www.pantherdb.org/). Functional interaction networks were identified with the STRING program v 9.1 STRING (http://string-db.org/). On the other hand, we searched for all differentially expressed proteins and used the recommended and alternative names assigned to each protein in the UniProtKB database, and detected biological maps of pathway with the Kyoto Encyclopedia of Genes and Genomes (KEGG) (http://www.genome.jp/kegg/).
2.10 Western-blot
Differentially expressed proteins such as HMOX1, MP2K5, XRCC1 and A1AG were measured by Western blot. Human lung AEC-II cells (Lanmeng Biotechnology, Hebei, China) were harvested after the treatments. After lysis for 30 min on ice with Cell Protein Extraction Reagent, the total protein concentration was determined by BCA assay according to the manufacturer's instructions. Each sample containing approximately 50 μg of proteins was electrophoresed on 12% SDS-PAGE and transferred onto PVDF membranes. The membranes were blocked with 5% non-fat milk for 2 h at room temperature. Primary antibodies (DF6623, AF5393, AF0296, DF7667, Affinity Biosciences, OH, USA) were incubated at 4 °C overnight. After washing, the membranes were incubated with horseradish peroxidase-conjugated secondary antibodies (ZB-2306, ZB-2305, ZSGB-BIO, Beijing, China). The immunoreactive bands were detected with an enhanced chemiluminescent (ECL) reagent kit according to the manufacturer's protocol and a chemiluminescence detection system. The obtained images were analyzed by Image J software to analyze the gray value of the target bands for relative quantitative information analysis of the proteins.
3. Results and discussion
3.1 Effects of PM2.5 on lung histology and damage
Pulmonary histological evaluation and pathological scores are shown in Fig. 1. The lung of control group showed a clear structure and normal histopathology, and few inflammatory cells could be found in bronchus and bronchiolitis. In contrast, there were a large number of inflammatory cells around bronchus in PM2.5 group (as shown in the black arrow); the structure of bronchial columnar epithelial cells was filled with inflammatory cells (as shown in the red arrow); the monolayer structure of the local alveolar epithelial cell wall disappeared. Epithelial cells thickened, and they aggregated into clusters of three to four layers (as shown in the yellow arrow); partial alveolar atrophied and collapsed (as shown in the brick red arrow); partial were compensatory hypertrophy (as shown in the green arrow). According to the pulmonary histological evaluation, we knew that PM2.5 could cause lung damage.
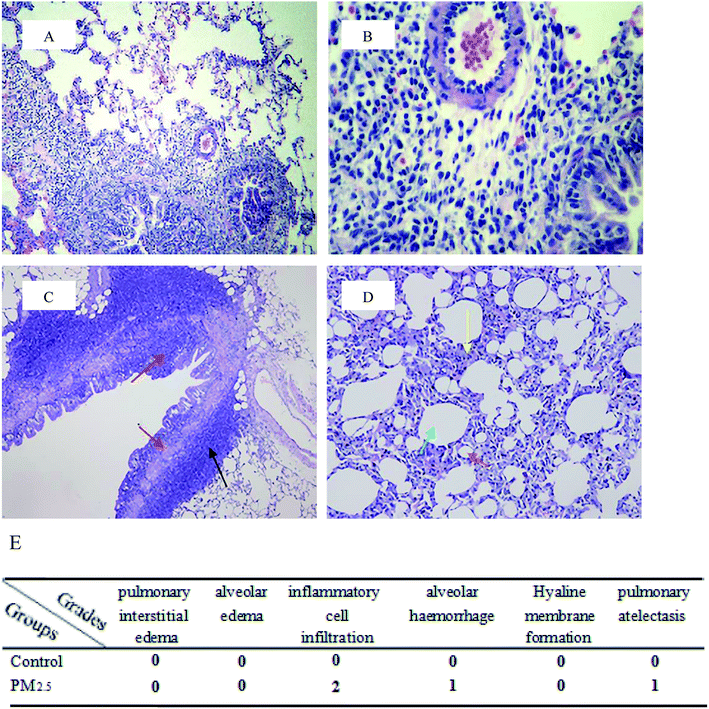 |
| Fig. 1 Pulmonary histological evaluation and pathological scores. The lung tissues of control group ((A and B) 10× magnification and 40× magnification, respectively) and PM2.5 group ((C and D) 10× magnification and 40× magnification, respectively) were stained with haematoxylin–eosin (H&E). Compared with the control group, there were a large number of inflammatory cells around bronchus in PM2.5 group (as shown in the black arrow); the structure of bronchial columnar epithelial cells was filled with inflammatory cells (as shown in the red arrow); the monolayer structure of the local alveolar epithelial cell wall disappeared. Epithelial cells thickened, and they aggregated into clusters of three to four layers (as shown in the yellow arrow); partial alveolar atrophied and collapsed (as shown in the brick red arrow); partial were compensatory hypertrophy (as shown in the green arrow). (E) Pathological scores. | |
3.2 Construction of experimental model and iTRAQ based proteomics system
We established a rat model of nonsurgical intratracheal instillation to study the lung damage caused by PM2.5. The process mainly included four clusters: PM2.5 exposure, sample preparation, iTRAQ/LC-MS analysis and bio-information analysis (Fig. 2). PM2.5 was collected using air sampler in Tianjin (one of the cities in North China which was suffered severe haze pollution) from June 2014 to January 2015, a typical haze season with PM2.5 index ranging from 60–300 μg m−3. PM2.5 samples were dispersed in 0.9% physiological saline. Intratracheal instillation of PM2.5 solution was performed 3 times in 15 days on rats. After instillation, rats were anesthetized and sacrificed. Afterwards, lung tissue was harvested, followed with protein extraction. iTRAQ labeling was conducted after peptide digestion. HPLC and LC-MS were used to pre-separate and separate peptides, respectively. It was noted that iTRAQ labeling, HPLC and LC-MS were performed by an analysis specialist in a double-blind manner. In detail, after we prepared the analysis samples, we transferred the samples to the analysis specialist without exposing sample information. Afterwards, the analysis specialist carried out iTRAQ labeling, HPLC and LC-MS. We next employed the paragon algorithm to quantify proteins, following with bio-information analysis. We categorized DEPs into biological processes with the Gene Ontology (GO) program. Furthermore, we identified functional interaction networks with the STRING program and discovered the pathways related to PM2.5-induced lung damage using the Kyoto Encyclopedia of Genes and Genomes (KEGG).
 |
| Fig. 2 Construction of experimental model and iTRAQ based proteomics system. The process included five clusters: PM2.5 exposure, peptide fractions from lung protein, iTRAQ/LC-MS analysis, data analysis and bioinformation analysis, and Western blotting. | |
3.3 Protein identification and biomarker screening
At first, we identified DEPs of PM2.5-induced lung damage as candidates for biomarkers. We set the healthy rats without PM2.5 exposure as the control group. The ratio of protein expression of PM2.5 exposure group/control group was utilized to evaluate the protein expression level. We defined the cut-off ratio values of >1.3 and <0.77 (with more than 95% in confidence) as up-regulation and down-regulation, respectively. We discovered 163 DEPs in response to intratracheal instillation of PM2.5, including 83 up-regulated proteins and 80 down-regulated proteins (Fig. 3). Increased percentage of DEPs (PI) was defined to evaluate the protein expression level by using the following equation: PI = (A1 − A0)/A0 × 100%, where A1 was the expression level of PM2.5 exposure group and A0 was the expression level of control group. The protein with a difference of more than 2 times the expression level (PI > 100%) was a minority compared to the overall data. A differential protein with a growth rate of PI > 140% was used as a DEP for lung injury induced by PM2.5 exposure. Among these 163 DEPs, it was apparent that six proteins, heme oxygenase 1 (HMOX1), MAP kinase kinase 5 (MP2K5), DNA repair protein (XRCC1), protein RT1-CE12 (E9PTZ7), alpha-1-acid glycoprotein (A1AG) and T-kininogen 2 (KNT2) were significant DEPs with PI > 140% (see detailed information in Table 1).
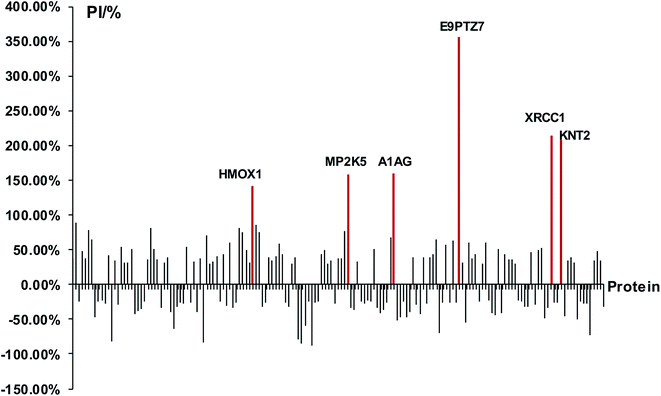 |
| Fig. 3 Analysis of DEPs and screening of biomarkers. The red lines represented significant DEPs with PI > 140%. | |
Table 1 Significant DEPs of lung damage induced by PM2.5 exposure
Entry name |
Protein names |
Gene names |
Percentage increase (I) |
Expressed pattern |
Molecular function |
HMOX1-RAT |
Heme oxygenase 1 |
Hmox1 |
141.30% |
↑ |
Heme oxygenase cleaves the heme ring at the alpha methene bridge to form biliverdin |
XRCC1_RAT |
DNA repair protein XRCC1 |
Xrcc1 |
214.30% |
↑ |
Repair DNA from oxidative damage and genetic variation |
MP2K5_RAT |
MAP kinase kinase 5 |
Map2k5 |
158.10% |
↑ |
Protecting cells from stress-induced apoptosis |
E9PTZ7_RAT |
Protein RT1-CE12 |
RT1-CE1 |
356.40% |
↑ |
Antigen processing and presentation of peptide antigen via MHC class I |
A1AG_RAT |
Alpha-1-acid glycoprotein |
Orm1 |
159.50% |
↑ |
Functions as transport protein in the blood stream. Appears to function in modulating the activity of the immune system during the acute-phase reaction |
KNT2_RAT |
T-kininogen 2 |
N/A |
207.20% |
↑ |
They effect smooth muscle contraction, induction of hypotension |
3.4 Classification and networking of functional DEPs
In order to obtain a fundamental understanding of pathways, we next performed GO program to classify DEPs to achieve a global view of protein functions.22,23 In the analysis of the GO program, proteins were attributed to involve in specific biological processes. We categorized these biological processes into six significant clusters based on functions including metabolic process, cellular process, biological regulation, response to stimulus, localization and immune system process (Fig. 4a). In general, when suffering exogenous damage, the organisms usually response to the stimulus and immune system process significantly.24 GO analysis showed that eighteen proteins were enriched in response to the stimulus, fifteen proteins in the immune system process, and twelve proteins in both processes (Fig. 4b, and see detailed information in Table 2). These results indicated that response to stimulus was closely related to immune system process. Previous studies provided implications on this relevance. For example, it has been reported that oxidative stress stimulus induced by PM2.5 exposure stimulated inflammatory responses, coupled with oxidative stress stimulus, further leading to cell apoptosis and immune response.25,26 We further analyzed the network of protein–protein interactions between DEPs through STRING program. We obtained 16 protein clusters (Fig. 5, and see detailed functions of protein clusters in Table 3).
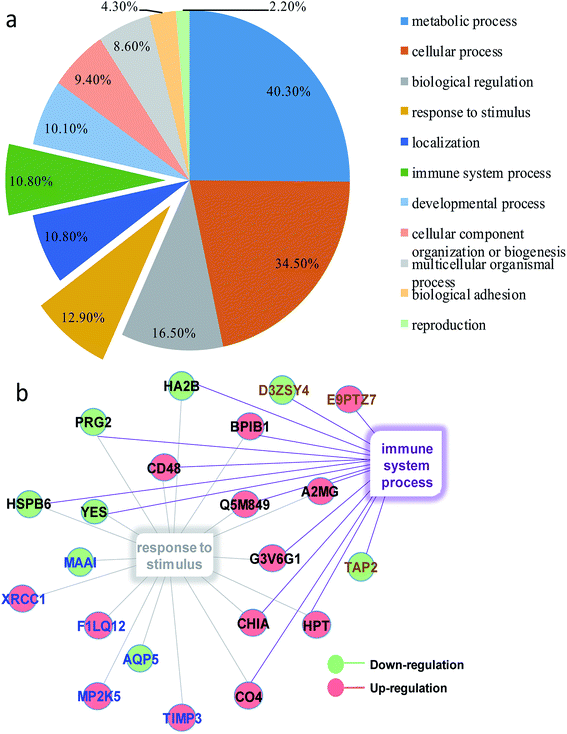 |
| Fig. 4 (a) Classification of molecular functions of DEPs. (b) DEPs enriched in response to stimulus and immune system process. Red balls represented down-regulated proteins and green balls referred to up-regulated proteins; blue words meant proteins exclusively enriched in response to stimulus, brown words were proteins exclusively enriched in immune system response, and black words denoted proteins enriched in both biology processes. | |
Table 2 DEPs enriched in the biological functions of stimulus and immune
Entry name |
Protein names |
Gene names |
PM2.5/control |
Expressed pattern |
Molecular function |
HA2B_RAT |
RT1 class II antigen, Ba chain |
RT1-Ba |
0.736 |
↓ |
Antigen processing and presentation |
PRG2_RAT |
Proteoglycan 2 |
Prg2 |
0.714 |
↓ |
Defense response to bacterium |
XRCC1_RAT |
DNA repair protein XRCC1 |
Xrcc1 |
3.143 |
↑ |
Repair DNA from oxidative damage and genetic variation |
CD48_RAT |
CD48 antigen |
Cd48 |
1.32 |
↑ |
Mast cell and T cell activation |
YES_RAT |
Tyrosine-protein kinase Yes |
Yes1 |
0.742 |
↓ |
Regulation of cell growth and survival, apoptosis, cell–cell adhesion, cytoskeleton remodeling, and differentiation |
MAAI_RAT |
Maleylacetoacetate isomerase |
Gstz1 |
0.738 |
↓ |
Glutathione transferase activity, L-phenylalanine catabolic process |
HSPB6_RAT |
Heat shock protein beta-6 |
Hspb6 |
0.676 |
↓ |
Regulation of muscle contraction |
CHIA_RAT |
Acidic mammalian chitinase |
Chia |
1.507 |
↑ |
Defense against nematodes, fungi and other pathogens |
CO4_RAT |
Complement C4 |
C4 |
1.345 |
↑ |
Complement activation; inflammatory response |
HPT_RAT |
Haptoglobin |
Hp |
1.757 |
↑ |
Acute inflammatory response |
MP2K5_RAT |
MAP kinase kinase 5 |
Map2k5 |
2.581 |
↑ |
Protecting cells from stress-induced apoptosis |
F1LQ12_RAT |
Protein Vom2r46 |
Vom2r46 |
1.496 |
↑ |
Detect massive pheromones |
G3V6G1_RAT |
Immunoglobulin joining chain |
Jchain |
1.433 |
↑ |
Synthesize and transport Igs |
A2MG_RAT |
Alpha-2-macroglobulin |
A2m |
1.886 |
↑ |
Acute inflammatory response to antigenic stimulus |
Q5M849_RAT |
Interferon-induced protein 35 |
Ifi35 |
1.39 |
↑ |
Trans located to the nucleus via the stimulation of interferons |
AQP5_RAT |
Aquaporin-5 |
Aqp5 |
0.525 |
↓ |
Generation of pulmonary secretions |
BPIB1_RAT |
BPI fold-containing family B member 1 |
Bpifb1 |
1.419 |
↑ |
Innate immunity in nose and lungs |
TIMP3_RAT |
Metalloproteinase inhibitor 3 |
Timp3 |
1.356 |
↑ |
Response to organic substance and tissue regeneration |
E9PTZ7_RAT |
Protein RT1-CE12 |
RT1-CE1 |
4.564 |
↑ |
Antigen processing and presentation of peptide antigen via MHC class I |
D3ZSY4_RAT |
Eosinophil peroxidase |
Epx |
0.738 |
↓ |
Eosinophil migration and oxidative stress |
TAP2_RAT |
Antigen peptide transporter 2 |
Tap2 |
0.582 |
↓ |
Adaptive immune response, antigen processing and presentation via MHC class I |
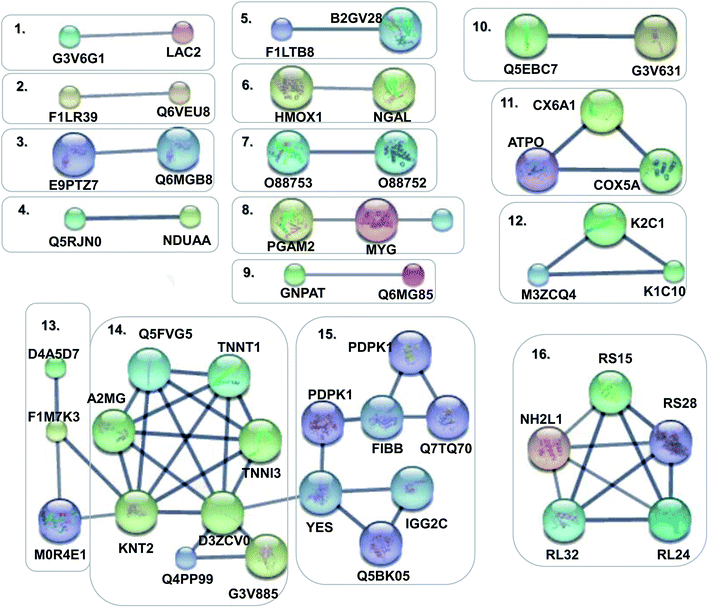 |
| Fig. 5 Protein–protein interaction networks of DEPs. Disconnected nodes were hided in the network. The small notes represented proteins whose 3D structure were unknown, while large notes represented proteins whose 3D structure were known or predicted. Edges represented protein–protein associations in the high confidence of 0.7. | |
Table 3 Interaction networks of DEPs
Cluster number |
Entry name |
Protein name |
Number |
Function of protein cluster |
1 |
G3V6G1_RAT, LAC2_RAT |
Ig lambda-2 chain C region, protein Jchain |
2 |
Adaptive immune response |
2 |
F1LR39_RAT, Q6VEU8_RAT |
WD repeat-containing protein 18, protein Ddx24 |
2 |
Major pathway of rRNA processing in the nucleolus |
3 |
E9PTZ7_RAT, Q6MGB8_RAT |
Protein RT1-CE12, protein RT1-A2 |
2 |
Involved in the presentation of foreign antigens to the immune system |
4 |
Q5RJN0_RAT, NDUAA_RAT |
Protein Ndufs7, NADH–ubiquinone oxidoreductase 42 kDa subunit |
2 |
Mitochondrial electron transport, NADH to ubiquinone |
5 |
F1LTB8_RAT, B2GV28_RAT |
UDP-glucuronosyltransferase, cytochrome P450 2B1 |
2 |
Involved in an NADPH-dependent electron transport pathway |
6 |
HMOX1_RAT, NGAL_RAT |
Heme oxygenase 1, neutrophil gelatinase-associated lipocalin |
2 |
Sensitive indicator of inflammatory damage |
7 |
O88753_RAT, O88752_RAT |
Epsilon 2 globin, epsilon 1 globin |
2 |
Hemoglobin subunit epsilon |
8 |
PGAM2_RAT, MYG_RAT, HPT_RAT |
Phosphoglycerate mutase 2, myoglobin, haptoglobin |
3 |
Reduced activity or antioxidant activity, serves as a reserve supply of oxygen |
9 |
GNPAT_RAT, Q6MG85_RAT |
Dihydroxyacetone phosphate acyltransferase, 1-acyl-sn-glycerol-3-phosphate acyltransferase |
2 |
Synthesis of PA, CDP-diacylglycerol biosynthesis |
10 |
Q5EBC7_RAT, G3V631_RAT |
Rab GTPase-binding effector protein 2, protein Rabgef1 |
2 |
Regulation of inflammatory response |
11 |
CX6A1_RAT, ATPO_RAT, COX5A_RAT |
Cytochrome c oxidase subunit 6A1, mitochondrial, ATP synthase subunit O, mitochondrial, cytochrome c oxidase subunit 5A, mitochondrial |
3 |
The oxidase in mitochondrial electron transport |
12 |
K2C1_RAT, M3ZCQ4_RAT, K1C10_RAT |
Keratin, type II cytoskeletal 1, 2, 10 |
3 |
Regulate the activity of kinases |
13 |
D4A5D7_RAT, F1M7K3_RAT, M0R4E1_RAT |
Protein phosphatase 1 regulatory subunit 12C, protein Myl7, myosin light chain 4 |
3 |
Myosin regulatory |
14 |
Q5FVG5_RAT, TNNT1_RAT, TNNI3_RAT, D3ZCV0_RAT, G3V885_RAT, Q4PP99_RAT, KNT2_RAT, A2MG_RAT |
Tropomyosin 2, slow skeletal muscle troponin T, cardiac troponin I, protein Actn2, myosin-6, cardiac troponin C, T-kininogen 2, alpha-2-macroglobulin |
8 |
Regulation of muscle contraction |
15 |
PDPK1_RAT, Q7TQ70_RAT, FIBB_RAT, PDPK1_RAT, YES_RAT, Q5BK05_RAT, IGG2C_RAT |
Protein kinase B, kinase, Ac1873, fibrinogen beta chain, protein kinase B kinase, tyrosine-protein kinase Yes, LOC367586 protein, Ig gamma-2C chain C region |
7 |
Immunological regulation |
16 |
RS15_RAT, RS28_RAT, RL24_RAT, RL32_RAT, NH2L1_RAT |
40S ribosomal protein S15, S28, 60S ribosomal protein L24, L32, NHP2-like protein 1 |
5 |
Ribosomal protein, translation proteins |
The analysis results implied that cluster 4, 5, 8, 11 were associated with antioxidant activity and cluster 1, 2, 3, 6, 9, 12, 15 were relevant with inflammatory response and immune regulation (Table 4). The above analysis showed that oxidative stress stimulus, inflammatory response and immune regulation were the most responsible factors for PM2.5-induced lung damage.
Table 4 Key pathways responsible to mechanisms of PM2.5
KEGG code |
Pathway |
DEP number |
Proteins |
Pathway description |
rno05204 |
Chemical carcinogenesis |
1 |
UDP-glucuronosyltransferase |
Chemical carcinogenesis can alter signal-transduction pathways |
rno05203 |
Viral carcinogenesis |
1 |
Protein Actn2 |
Viruses can contribute to initiation as well as progression of human cancers |
rno04612 |
Antigen processing and presentation |
1 |
Protein LOC103689996 |
Process and present antigen |
rno04152 |
AMPK signaling pathway |
1 |
PkB kinase |
It is caused by metabolic stresses that either interfere with ATP production or that accelerate ATP consumption |
rno04146 |
Peroxisome |
2 |
Sterol carrier protein 2, glycerone-phosphate O-acyltransferase |
Peroxisomes are essential organelles that play a key role in redox signalling and lipid homeostasis |
rno04010 |
MAPK signaling pathway |
2 |
MAPK kinase 5, evolutionarily conserved signaling intermediate in Toll pathway, mitochondrial |
Involved in various cellular functions, including cell proliferation, differentiation and migration |
rno04151 |
PI3K–AKT signaling pathway |
1 |
Protein kinase B kinase |
PI3K–AKT is activated by cellular stimuli or toxic insults and regulates fundamental cellular functions. PI3kinase AKT also regulates F-actin |
rno04150 |
mTOR signaling pathway |
1 |
Protein kinase B kinase |
mTOR is activated by the presence of growth factors, amino acids, energy status, stress and oxygen levels to regulate biological processes, including autophagy and protein synthesis |
rno04660 |
T cell receptor signaling pathway |
1 |
Protein kinase B kinase |
A key event for an efficient response of the immune system, lead to T-cell proliferation activation, cytokine production and differentiation into effector cells |
rno04020 |
Calcium signaling pathway |
1 |
Protein Tnnc1 |
The influx of Ca2+ from the environment or release from internal stores causes a very rapid and dramatic increase in cytoplasmic calcium concentration, which has been widely exploited for signal transduction |
rno04145 |
Phagosome |
1 |
Tap2 protein |
Phagocytosis is the process of taking in relatively large particles by a cell, and is a central mechanism in the tissue remodeling, inflammation, and defense against infectious agents |
rno04650 |
Natural killer cell mediated cytotoxicity |
1 |
CD48 antigen |
NK cells are lymphocytes of the innate immune system that are involved in early defenses against both allogeneic (nonself) cells and autologous cells |
rno04670 |
Leukocyte transendothelial migration |
3 |
Protein Actn2, claudin, protein Myl7 |
Leukocyte migration from the blood into tissues is vital for immune surveillance and inflammation |
rno05310 |
Asthma |
1 |
Eosinophil peroxidase |
Asthma is a complex syndrome with many clinical phenotypes in both adults and children |
rno05223 |
Non-small cell lung cancer |
1 |
Protein kinase B kinase |
Non-small-cell lung cancer (NSCLC) accounts for approximately 85% of lung cancer |
3.5 Establishment of pathways
Pathway analysis can provide implications for clinical prevention and therapeutic strategies. Therefore, we analyzed the pathways of DEPs using the KEGG database. We discovered that a total of 101 pathways were involved in PM2.5-induced lung damage. In particular, 15 pathways were found to be involved in responses to stimuli, inflammatory and immune responses such as calcium signaling, mitogen-activated protein kinase (MAPK) signaling, and AKT/phosphoinositide 3-kinase (AKT/PI3K) signaling.
Activations of calcium signaling, MAPK and AKT/PI3K pathways regulated inflammatory response and activated immune response system, including antigen processing and presentation,27,28 T cell and B cell proliferation, natural killer cell (NK cell) mediated cytotoxicity, leukocyte migration and phagocytosis. Based on KEGG analysis and previous literature, we achieved an integral pathway to explain the mechanisms of PM2.5-induced lung damage (Fig. 6). Chemical carcinogenesis and viral carcinogenesis of PM2.5 induced pulmonary disease, such as asthma and lung cancer through the stimulus, inflammatory response and immune response.29,30 The first stage of lung damage was the processing and presentation of antigens. Reactive oxygen species (ROS) and microorganisms coated on PM2.5 functioned as antigens activating immune response. CD8+ and CD4+ T cells participated in the processing and presentation of antigens through MHC-I and MHC-II, respectively.31 The second stage was the activation of inflammation and immunity, such as activation of T lymphocytes cell, B lymphocytes cell and NK cell. Calcium signaling pathway participated in the proliferation of immune cells.32 Subsequently, the immune cells released the active mediators, i.e., the formation of antibody by B cells, chemotactic factors by mast cell and secretory factor killing by T cell. The last stage was the formation of lung injury. Toxic particles induced by eosinophils led to tissue damage and promoted the development of chronic inflammation. On the other hand, NK cells had potent cytotoxic activity against infectious or neoplastic cells and induced programmed death of lung cells, eventually leading to pulmonary diseases, such as asthma and even lung cancer.33 The proteins, Tnnc1, MP2K5 and PkB kinase, were involved in calcium signaling, MAPK signaling and PI3K/AKT pathway, respectively.
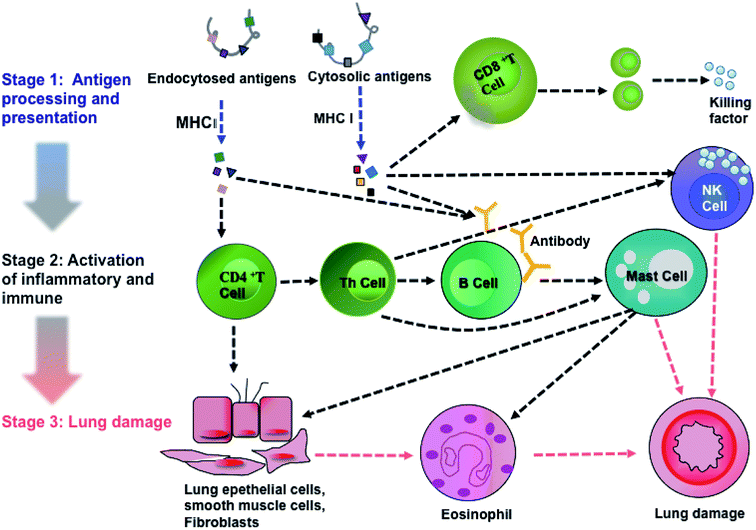 |
| Fig. 6 An integrated pathway of PM2.5-induced lung damage. | |
3.6 Western-blot analysis and biomarker verification
Human lung AEC-II cells were treated with 100 μg mL−1 PM2.5 for 72 h, and four differentially significant protein expressions of HMOX1, MP2K5, XRCC1 and A1AG were determined by Western-blot assay (E9PTZ7, KNT2 corresponding antibody were not found). As shown in Fig. 7, PM2.5 exposure increased the expression of XRCC1 protein by 1.13 times (P > 0.05, not statistically significant), MP2K5 by 1.33 times (P < 0.05), HMOX1 by 1.74 times (P < 0.01), and A1AG by 1.22 times (P < 0.05). Quantitative results were consistent with proteomic analysis. Combined with statistical analysis, MP2K5, HMOX1 and A1AG were used as biomarkers for detecting PM2.5-induced lung injury.
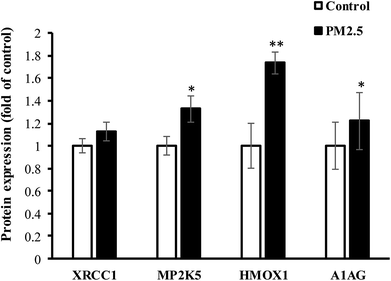 |
| Fig. 7 The expression of significant DEPs in AEC-II cells exposed to PM2.5. | |
4. Conclusion
In conclusion, we developed a novel integrative system combining a set of experimental models, analytical techniques and bioinformatics analysis tools to investigate the proteomics of PM2.5-induced lung damage. Six significant DEPs were screened as biomarkers, including HMOX1, MP2K5, XRCC1, E9PTZ7, A1AG and KNT2. The results of the GO and STRING program suggested that oxidative stress stimulus, inflammatory response and immune regulation were the most significant processes in lung injury. KEGG analysis revealed that calcium signaling, MAPK and PI3K/AKT might be involved in the process of PM2.5-induced lung damage. The proteins, Tnnc1, MP2K5 and PkB kinase, were involved in calcium signaling, MAPK signaling and PI3K/AKT pathway, respectively, which provided implications for preventive and therapeutic strategies of PM2.5 associated pulmonary diseases such as asthma and lung cancer. Further evidences like gene knockout are also needed. Our present study provide primary information on biomarkers and pathways of PM2.5-induced toxicity in lung tissue and can serve as fundamental research clues for future studies.
Conflicts of interest
The authors declare no competing financial interests.
Acknowledgements
This work was supported by grants from the National Natural Science Foundation of China (No. 31571825, No. 31271979) and Key Experiment Research Foundation for Water Resources and Water Environment of Tianjin (No. YF117100102).
References
- R. J. Huang, Y. Zhang, C. Bozzetti, K. F. Ho, J. J. Cao, Y. Han, K. R. Daellenbach, J. G. Slowik, S. M. Platt, F. Canonaco, P. Zotter, R. Wolf, S. M. Pieber, E. A. Bruns, M. Crippa, G. Ciarelli, A. Piazzalunga, M. Schwikowski, G. Abbaszade, J. Schnelle-Kreis, R. Zimmermann, Z. An, S. Szidat, U. Baltensperger, I. El Haddad and A. S. Prevot, Nature, 2014, 514, 218–222 CrossRef CAS PubMed.
- C. M. Wong, H. Tsang, H. K. Lai, G. N. Thomas, K. B. Lam, K. P. Chan, Q. S. Zheng, J. G. Ayres, S. Y. Lee, T. H. Lam and T. Q. Thach, Cancer Epidemiol., Biomarkers Prev., 2016, 25, 839–845 CrossRef CAS PubMed.
- G. Sancini, F. Farina, C. Battaglia, I. Cifola, E. Mangano, P. Mantecca, M. Camatini and P. Palestini, PLoS One, 2014, 9, e109685 CrossRef PubMed.
- R. Chen, Z. Zhao and H. Kan, Am. J. Respir. Crit. Care Med., 2013, 188, 1170–1171 CrossRef.
- J. O. Anderson, J. G. Thundiyil and A. Stolbach, J. Med. Toxicol., 2012, 8, 166–175 CrossRef CAS.
- L. A. Torre, F. Bray, R. L. Siegel, J. Ferlay, J. Lortet-Tieulent and A. Jemal, Ca-Cancer J. Clin., 2015, 65, 87–108 CrossRef PubMed.
- A. J. Badyda, J. Grellier and P. Dabrowiecki, Adv. Exp. Med. Biol., 2017, 944, 9–17 CrossRef PubMed.
- A. Colao, G. Muscogiuri and P. Piscitelli, Int. J. Environ. Res. Public Health, 2016, 13, 1–9 CrossRef PubMed.
- X. Chen, L. W. Zhang, J. J. Huang, F. J. Song, L. P. Zhang, Z. M. Qian, E. Trevathan, H. J. Mao, B. Han, M. Vaughn, K. X. Chen, Y. M. Liu, J. Chen, B. X. Zhao, G. H. Jiang, Q. Gu, Z. P. Bai, G. H. Dong and N. J. Tang, Sci. Total Environ., 2016, 571, 855–861 CrossRef CAS PubMed.
- Y. Chen, A. Ebenstein, M. Greenstone and H. Li, Proc. Natl. Acad. Sci. U. S. A., 2013, 110, 12936–12941 CrossRef CAS PubMed.
- L. Curtis, W. Rea, P. Smith-Willis, E. Fenyves and Y. Pan, Environ. Int., 2006, 32, 815–830 CrossRef CAS PubMed.
- K. Hackner, P. Errhalt, M. R. Mueller, M. Speiser, B. A. Marzluf, A. Schulheim, P. Schenk and J. Bilek, J. Breath Res., 2016, 10, 046003 CrossRef PubMed.
- A. Hirai, S. Shinohara, T. Kuwata, M. Takenaka, Y. Chikaishi, S. Oka, K. Kuroda, N. Imanishi and F. Tanaka, Surgical Case Reports, 2016, 2, 46 CrossRef PubMed.
- S. L. Shirran and C. H. Botting, J. Proteomics, 2010, 73, 1391–1403 CrossRef CAS PubMed.
- Y. S. Zhou and W. N. Chen, J. Proteomics, 2011, 75, 511–516 CrossRef CAS PubMed.
- X. T. Zhong, Z. Q. Wang, R. Y. Xiao, Y. Q. Wang, Y. Xie and X. P. Zhou, J. Proteomics, 2017, 152, 88–101 CrossRef CAS PubMed.
- P. Mantecca, F. Farina, E. Moschini, D. Gallinotti, M. Gualtieri, A. Rohr, G. Sancini, P. Palestini and M. Camatini, Toxicol. Lett., 2010, 198, 244–254 CrossRef CAS PubMed.
- X. Deng, W. Rui, F. Zhang and W. Ding, Cell Biol. Toxicol., 2013, 29, 143–157 CrossRef CAS PubMed.
- A. E. Quaglio, A. C. Castilho and L. C. Di Stasi, Life Sci., 2015, 136, 60–66 CrossRef CAS PubMed.
- Z. Zhou, Y. Liu, F. Duan, M. Qin, F. Wu, W. Sheng, L. Yang, J. Liu and K. He, PLoS One, 2015, 10, e0138267 CrossRef PubMed.
- J. K. Horton, D. F. Stefanick, N. R. Gassman, J. G. Williams, S. A. Gabel, M. J. Cuneo, R. Prasad, P. S. Kedar, E. F. Derose, E. W. Hou, R. E. London and S. H. Wilson, DNA Repair, 2013, 12, 774–785 CrossRef CAS PubMed.
- Y. Li, O. Bai, J. Cui and W. Li, Eur. J. Med. Genet., 2016, 59, 91–103 CrossRef PubMed.
- S. Diaz-Moran, M. Palencia, C. Mont-Cardona, T. Canete, G. Blazquez, E. Martinez-Membrives, R. Lopez-Aumatell, M. Sabariego, R. Donaire, I. Moron, C. Torres, J. A. Martinez-Conejero, A. Tobena, F. J. Esteban and A. Fernandez-Teruel, Behav. Brain Res., 2013, 257, 129–139 CrossRef CAS PubMed.
- G. X. Ren, C. E. Wu, C. Teng and Y. Yao, Food Agric. Immunol., 2018, 29(1), 953–963 CrossRef CAS.
- S. Michael, M. Montag and W. Dott, Environ. Pollut., 2013, 183, 19–29 CrossRef CAS PubMed.
- U. S. Akhtar, R. D. McWhinney, N. Rastogi, J. P. Abbatt, G. J. Evans and J. A. Scott, Inhalation Toxicol., 2010, 22(suppl. 2), 37–47 CrossRef CAS PubMed.
- J. Cao, G. Qin, R. Shi, F. Bai, G. Yang, M. Zhang and J. Lv, J. Appl. Toxicol., 2016, 36, 609–617 CrossRef CAS PubMed.
- R. Su, X. Jin, W. Zhang, Z. Li, X. Liu and J. Ren, Chemosphere, 2016, 167, 444–453 CrossRef PubMed.
- R. Ghosh, P. Rossner, K. Honkova, M. Dostal, R. J. Sram and I. Hertz-Picciotto, Environ. Int., 2016, 87, 94–100 CrossRef CAS PubMed.
- L. Yang, G. Liu, Z. Lin, Y. Wang, H. He, T. Liu and D. W. Kamp, Environ. Toxicol., 2016, 31, 923–936 CrossRef CAS PubMed.
- R. D. F. E. Silva, L. F. G. R. Ferreira, M. Z. Hernandes, M. E. F. De Brito, B. C. De Oliveira and A. A. Da Silva, Front. Immunol., 2016, 7, 327 Search PubMed.
- P. S. Lakey, T. Berkemeier, H. Tong, A. M. Arangio, K. Lucas, U. Poschl and M. Shiraiwa, Sci. Rep., 2016, 6, 32916 CrossRef CAS PubMed.
- M. Voss and Y. T. Bryceson, Clin. Immunol., 2015, 15, 1–14 Search PubMed.
Footnote |
† These authors contributed equally to this paper. |
|
This journal is © The Royal Society of Chemistry 2019 |
Click here to see how this site uses Cookies. View our privacy policy here.