DOI:
10.1039/C8RA10243K
(Paper)
RSC Adv., 2019,
9, 3004-3011
Molecular simulation of CO2/CH4/H2O competitive adsorption and diffusion in brown coal
Received
13th December 2018
, Accepted 17th January 2019
First published on 22nd January 2019
Abstract
Carbon dioxide enhanced coalbed methane recovery (CO2-ECBM) has been proposed as a promising technology for the natural gas recovery enhancement as well as mitigation of CO2 emissions into the atmosphere. Adsorption and diffusion of CO2/CH4 mixture play key roles in predicting the performance of CO2-ECBM project, i.e., the production of coalbed methane as well as the geological sequestration potential of carbon dioxide. In the present work, the mechanism of competitive adsorption and diffusion of CO2/CH4/H2O mixture in brown coal were investigated by employing grand canonical Monte Carlo and molecular dynamics simulation. The effects of temperature and pressure on competitive adsorption and diffusion behaviours were explored. It is found that CO2 has much stronger adsorption ability on brown coal than CH4. The adsorption amounts of CO2/CH4 increase with pressure but have a decreasing trend with temperature. High adsorption selectivity of CO2/CH4 is observed with pressure lower than 0.1 MPa. In addition, the effects of moisture content in brown coal on the adsorption characteristics have been examined. Simulation results show that the adsorption capacities of CO2/CH4 are significantly suppressed in moist brown coal. The competitive adsorption of CO2/CH4/H2O follows the trend of H2O ≫ CO2 > CH4. Moreover, the results reveal that moisture content has great effects on the self-coefficients of CO2/CH4. Compared with dry coal, the self-diffusion coefficients of CO2 and CH4 reduce by 78.7% and 75.4% in brown coal with moisture content of 7.59 wt%, respectively. The microscopic insights provided in this study will be helpful to understand the competitive adsorption and diffusion mechanism of CO2/CH4/H2O in brown coal and offer some fundamental data for CO2-ECBM project.
Introduction
In recent years coalbed methane (CBM) has attracted enormous attention as a high efficient, environmentally friendly and abundant source of energy.1–3 CBM, which consists of methane, a small amount of heavy hydrocarbons, CO2, H2O, and other gases, is mainly absorbed on the matrix of coal particle surface. The optimal estimation of worldwide CBM resource would exceed 200 trillion cubic meters (Tcm), as much as convectional natural gas.4 The CBM resource in China has been estimated to exceed 36 Tcm, following Russia and Canada.5 In November 2016, China's National Energy Administration released the exploration and production activity plan for coalbed methane (coal mine methane).6 It stated that the annual production of ground coalbed methane should exceed 10 Bcm (billion cubic meters) by the year 2020, increasing from 4.4 Bcm in 2015, which offers big opportunity for coalbed methane development. Furthermore, extracting methane before exploiting coal can effectively avoid the risk of gas outburst and explosion.7,8 In addition to hydraulic fracturing, injection of CO2 to enhance coalbed methane recovery (CO2-ECBM) has recently received considerable interest all over the world.9–13 Large amount of CO2 can be injected into unminable coal seams and displace the pre-adsorbed methane due to the higher preferential adsorption for CO2 over CH4 in coal seams. This could not only enhance recovery of CBM but also effectively reduce greenhouse gas (GHG) emissions by trapping CO2 in coalbed. CO2-ECBM is an effective technology of CO2 capture, utilization and sequestration (CCUS), which has been considered as one of the most important technological alternatives to contribute to the global CO2 emission reduction. Previous studies have shown that the majority of total pore volume is dominated by micropores (i.e., <2 nm) and mesopores (i.e., 2–50 nm) in various coals.11 Therefore, the in-depth understanding of the adsorption and diffusion mechanisms of CO2 and CH4 at the microscale in coalbeds is essential to develop effective and reliable strategies for CO2-ECBM project. Extensive research work has been carried out in this field.
Many scholars have conducted experiments to study gas adsorption and diffusion in coal. Busch et al.14,15 performed CH4/CO2 mixture adsorption-diffusion experiments on coal and found CO2 sorption rates are consistently higher by a factor of 2–3 than those for CH4, when comparing single-gas sorption experiments. A laboratory experiment on coalbed methane displacement with CO2 injection was performed in the work.16 Their results indicated that the transport rate of CH4 is dependent on its adsorption on coals. More recently, core flooding experiments of CO2-ECBM was performed by Sander et al.17 They reported that the displacement efficiency of CO2-ECBM was up to 100% of the initial methane. Ranathunga et al.18 conducted experiments to investigate applicability of CO2 enhanced coal bed methane recovery to low rank coal. They concluded that compared with natural recovery, CO2 flooding enhanced CH4 production and the superior competence of supercritical CO2 in CH4 recovery is independent of coal rank or maturity. One can refer to a recent review for more experimental studies on CO2-ECBM.19
In addition to experimental work, much simulation work has also recently been carried out. Zeng et al.20 used a coupled adsorption-strain model and Transport of Unsaturated Groundwater and Heat Simulator (TOUGH2) to simulate the enhanced coalbed methane recovery process. Their results suggested that it takes about 1800 days to displace 90% of methane considering the coal deformation and permeability change. However, it is difficult to explore the microscopic interactions using such macroscale experiments or simulator, which is essential to understand the mechanism of adsorption and diffusion behaviours for CO2-ECBM project. Therefore, microscopic methods are very suitable for investigating the adsorption and diffusion behaviours which occur in nano- and micro-sized pores in coal. Liu and Wilcox21 used defective and defect-free graphene surfaces to represent the structural heterogeneity and related chemical nature of the organic matrix in coal and shale. By employing density functional theory (DFT), they observed that CO2 bonds stronger to the defective graphene surface than perfect graphene. Dang et al.22 and Song et al.23 applied DFT and molecular simulations to investigate CO2/CH4 adsorption behaviours in low rank coal. They claimed that the adsorption of at the oxygen- and nitrogen-containing functional groups is strengthened by their basicity and high temperature is not conducive to the vitrinite's adsorption of CH4/CO2/H2O. Carbon dioxide adsorption-assisted CH4 desorption in coal was studied in the work.24 Besides adsorption characteristics, Zhao et al.25,26 adopted molecular simulations to study CO2/CH4 binary adsorption and transport diffusion in bituminous coal. Their results have suggested an optimal injection depth for CO2-ECBM project. The interaction between sub-bituminous coal and water was examined by molecular dynamics simulations.27 They claimed that the water molecules prefer to absorb with carboxylic groups. Although much work has been carried out, there are only a few studies focusing on the adsorption and diffusion of CO2/CH4 mixture in brown coal, which account for around 47% of total coal reserve. However, brown coal only made up 21.20% global coal supply in 2011,28 indicating that there is huge potential of coalbeds methane recovery and CO2 sequestration for brown coal in the future. As is well known, moisture content is the key factor that hinders the efficient use of brown coal. Thus, it is necessary to investigate the moisture effect on competitive adsorption and diffusion performance in brown coal reservoir.
In the present work, the grand canonical Monte Carlo (GCMC) and molecular dynamics (MD) are employed to investigate CO2/CH4 competitive adsorption and diffusion behaviour in brown coal. The effects of temperature, pressure and moisture content on competitive adsorption and diffusion are examined and discussed. The objectives of this study is to provide a molecular-level insight into CO2/CH4 competitive adsorption and diffusion mechanism in brown coal and also offer some fundamental data for further investigation on CO2-ECBM project.
Models and methodology
Brown coal structure construction
The nature of coal is a heterogeneous porous solid material with complex physical and chemical structures. The weight of carbon content ranges from 60% to 95%, depending on their ranks.29,30 Thus, there are a large number of coal structures and so far over 130 molecular level representations have been proposed.31 As one kind of low-rank coals, brown coal has abundant resource in China as well as in the rest of world. In the present study, the molecular model of brown coal was built based on the model in the literature,32 as shown in Fig. 1(a). This model has captured a few of essential features of brown coal, including single aromatic rings linked and cross-linked by aliphatic side chains, which is suitable for the investigations of gas adsorption and diffusion behaviour in brown coal.22
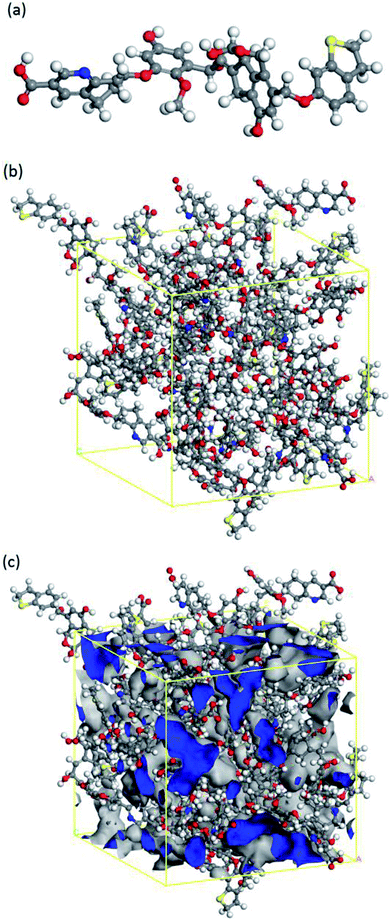 |
| Fig. 1 The structure of brown coal: (a) molecular model, C39H37O10NS. Colour scheme: C, grey; H, white; O, red; N, blue; S, yellow. (b) Optimized configuration of brown coal. (c) Simulation cell of coal with pores (blue zones). | |
To perform adsorption and diffusion simulations in porous coal structure, an amorphous cell containing 20 optimized coal molecules was then built. By applying annealing dynamics with a temperature cycle from 300 K to 600 K, the system was equilibrated and the optimized brown coal structure (26.93 × 26.93 × 26.93 Å3) was obtained, as shown in Fig. 1(b). In order to achieve an appropriate brown coal structure for further simulations, the micro-porosity was calculated by using fractional free volume definition33 and Connolly surface method.34 The obtained porosity of 24.62% and equilibrium density of 1.21 g cm−3 were consistent with the experimental results 21% and 1.18–1.43 g cm−3, respectively.35,36 It should be mentioned that the chemical composition and physical properties may vary between different brown coals. Nevertheless, the constructed molecular model is capable of studying CO2/CH4 competitive adsorption and diffusion mechanism in brown coal.
Competitive adsorption and GCMC simulation
In this work, the grand canonical Monte Carlo (GCMC) method was firstly employed to study the adsorption behaviour of CO2/CH4 in brown coal. The condensed-phase optimized molecular potential for the atomistic simulation studies (COMPASS) force field was applied in the simulations.37 The Ewald sum method was used to describe the electrostatic interactions with an accuracy of 10−3 kcal mol−1. As for van der Waals (vdW) interactions, they were calculated by atom-based approach with a cutoff distance of 12.5 Å. While interactions between organic matter and gas molecules were described using the Lennard-Jones (LJ) 12–6 potential.38 In addition, the classical Metropolis rule was adopted to accept or reject the generation, disappearance, translation, and rotation of small gas molecules to ensure the lowest energy state of the system.39 The accept probabilities of the exchange, conformer, rotation, translation and regrowth were 39%, 20%, 20%, 20% and 2%, respectively. Simulation cases were performed with 1 × 107 Monte Carlo steps. The equilibrium steps were 5 × 106 and the production steps were 5 × 106 for the calculation of required physical parameters. More details regarding GCMC simulation can be referred to our previous study of competitive adsorption in shale matrix.40
In GCMC simulation, the chemical potential of the gas, the volume and temperature of the system are fixed. The chemical potential or equivalently the fugacity is imposed when carrying out GCMC simulations. In this study, the fugacity of CH4 and CO2 mixture (i.e., ‘corrected’ pressure) was determined by using the Peng–Robinson (P–R) equation of state.41 The adsorption amount obtained in simulations is absolute amount, while excess amount is usually applied in experiments. The relationship between absolute amount and excess amount of adsorption can be written as:
where
nex and
nabs represent the excess and absolute amounts adsorbed, respectively.
ρb denotes the density of the bulk phase at the conditions of interest, which can be calculated using the P–R equation of state.
Vads is the pore volume.
To further investigate the competitive adsorption behaviour, the adsorption selectivity of CO2 over CH4 is defined:
|
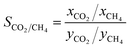 | (2) |
where
xi is the mole fraction of component i in the adsorption phase, and
yi is the mole fraction of component i in the bulk phase. Higher adsorption selectivity (>1) indicates a stronger adsorption capacity of CO
2 over CH
4.
In order to study the effect of moisture content on absorption, the average water density was introduced to quantify the moisture content:
|
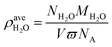 | (3) |
where
NH2O represents the number of H
2O molecules,
MH2O is the water molar weigh,
V denotes the volume of the slit nanopore,
NA is Avogadro's number and
ϖ is the moisture.
Diffusion and MD simulation
Gas diffusion in coalbeds, which plays a key role in predicting the potential of methane extraction and carbon sequestration in CO2-ECBM project, is of great importance. Self-diffusion, which indicates random motions or mixing of particles in the thermodynamic equilibrium, can be determined by curves of mean square displacement (MSD) and Einstein method. The formula of the Einstein method can be written as follows: |
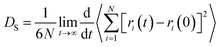 | (4) |
where DS is the self-diffusion coefficient, N is the number of adsorbates, t is the simulation time and ri(t) and ri(0) are position vectors at t and the initial time, respectively. The angular bracket denotes an ensemble average property. Based on MSD curves, linear regression is carried out and the slope k can be obtained. Thus, the diffusion coefficient can be simplified as DS = k/6.
Based on the adsorption model of brown coal, the molecular dynamics (MD) simulation was applied to calculate the diffusion coefficients of species in brown coal. In the simulations, the NVT (canonical) and NPT (constant-pressure, constant-temperature) ensemble were successively adopted to obtain optimized diffusion model and calculate data for further analysis. Each MD simulation time was set to 2 ns with a time step of 0.001 ps. It should be mentioned that the mole fractions of CO2 and CH4 in the bulk phase are kept to be 0.5 and the pressure is the total pressure when competitive adsorption and diffusion of CO2/CH4 are concerned in this study. All GCMC and MD simulations were conducted in Accelrys Materials Studio software.
Results and discussion
Pure and mixture gas adsorption isotherms
GCMC simulations were firstly carried out for the absolute adsorption isotherms of pure CH4 and CO2 in the pressure range of 0–10 MPa at different temperatures, as shown in Fig. 2. It is found that the adsorption capacities for CH4 and CO2 increase with the increasing pressure, but with different scales. The adsorption capacity for CO2 increases more rapidly at low pressure and tends to be constant at high pressure, as can be seen in Fig. 2(b). The results show the adsorption isotherms for both CH4 and CO2 fit very well with Langmuir equation, which is widely used to describe the gas adsorption in coal/shale matrix. The results are consistent with previous work.22,42,43 From Fig. 2(a) and (b), it can be seen that CO2 adsorb on coal matrix more easily than CH4. The adsorption capacity of CO2 is 1.916 mmol g−1 at the pressure of 8 MPa and temperature of 298.15 K, while the value of CH4 is 0.901 mmol g−1. Similar results have also been obtained in previous experimental work.19
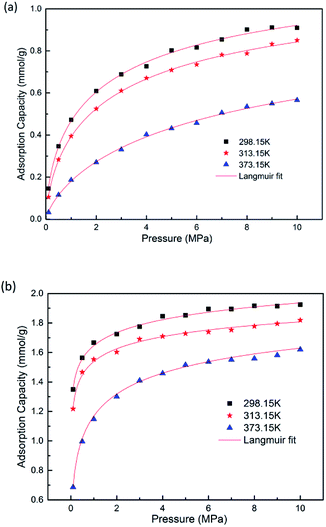 |
| Fig. 2 Absolute adsorption isotherms of pure component at different temperatures: (a) CH4; (b) CO2. | |
Fig. 3 demonstrates the competitive adsorption isotherms of CO2/CH4 binary mixture in brown coal. It is observed that the adsorption capacity of CH4 is significantly suppressed at the presence of CO2. The adsorption amount of CO2 is 1.809 mmol g−1, slightly lower than that of pure component under the condition of 8 MPa/298.15 K. However, the adsorption amount of CH4 falls to 0.0586 mmol g−1, nearly 93.5% reduction compared to that of the pure CH4 capacity in brown coal. This could be attributed to the much stronger interaction between coal–CO2, relative to coal–CH4 and CO2–CH4.44,45 Actually, this unique characteristic makes carbon dioxide superior working fluid in ECBM project.
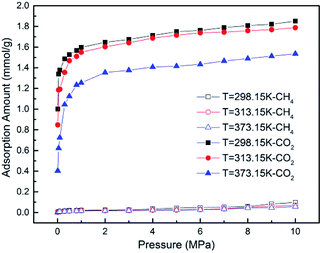 |
| Fig. 3 Competitive adsorption amount of CO2 and CH4 in the binary mixture at different temperatures. | |
Effects of temperature and pressure on competitive adsorption behaviours
To further reveal the effects of pressure and temperature on the competitive adsorption performance of CO2/CH4 binary mixture, a series of GCMC simulations were carried out. Fig. 4(a) and (b) illustrate the adsorption amount of CO2 and CH4 in binary mixture adsorption, respectively. It is found that as pressure increases, the adsorption amounts of both CO2 and CH4 increase gradually. For CH4 component, the adsorption amount increases from 0.028 to 0.038 mmol g−1 as pressure grows from 4 to 7 MPa at the temperature of 313.15 K. As for CO2, the value increases from 1.687 to 1.745 mmol g−1. The results also show that the adsorption amounts of CO2/CH4 decrease with the increasing temperature of 298.15–373.15 K. This is due to the fact that as temperature goes up, the irregular thermal motion of CO2/CH4 molecules become more intense, making it more difficult for the molecules trapped in the nanopores of coal matrix. It can be seen from the simulation results that a lower temperature would be favorable for the adsorption of CO2/CH4 binary mixture in brown coal.
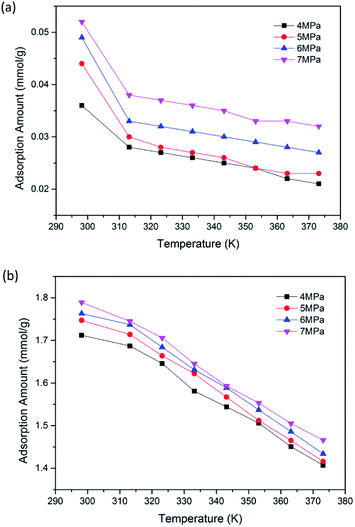 |
| Fig. 4 Adsorption amount of (a) CH4; (b) CO2 in the binary mixture as a function of temperature at different pressures. | |
To evaluate the efficiency of CO2 sequestration in CO2-ECBM project, the adsorption selectivity of CO2/CH4 binary mixture was calculated, as plotted in Fig. 5. It is observed that super high selectivities larger than 200 were obtained at low pressure 0.01 MPa for a wide range of temperature. As pressure increases, the adsorption selectivity declines remarkably and the decrease tends to be gradual at higher pressure >2 MPa. Similar results have also be found in brown coal22 and in shale clay minerals.46 From the simulation results, it can be concluded that a lower pressure is helpful to enhance the adsorption selectivity of CO2/CH4 but higher pressure is beneficial to the adsorption amount. Therefore, an appropriate pressure should be concerned in CO2-ECBM project.
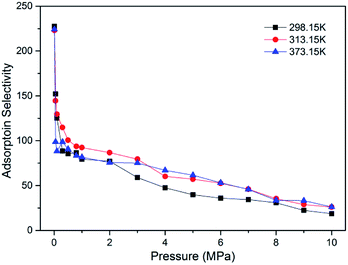 |
| Fig. 5 Adsorption selectivity of CO2/CH4 in brown coal as a function of pressure at different temperatures. | |
Effects of moisture content on competitive adsorption behaviours
Previous literature has suggested that water content could affect the gas adsorption in coal matrix.19 To quantify the effect of moisture content on CO2/CH4 adsorption in CO2-ECBM project, CO2/CH4/H2O ternary mixture competitive adsorption behaviours in brown coal were studied in this section. A certain number of H2O molecules were pre-loaded on the optimized brown coal structure, as shown in Fig. 1(b). According to eqn (3), moisture contents of 2.53 wt%, 5.06 wt% and 7.59 wt% were obtained by pre-loading 20, 40 and 60H2O molecules in the simulation cell, respectively. Fig. 6 demonstrates the competitive adsorption performance of CO2/CH4/H2O ternary mixture at different temperatures. It is found that the adsorption capacities of all three components decrease with the rise of temperature. In addition, it is observed that compared with single component or binary mixture of CO2/CH4, the adsorption capacities of CO2 and CH4 are significantly suppressed at the presence of moisture content. The adsorption capacity of H2O molecules is 7.552 mmol g−1 under the condition of 8 MPa/298.15 K, while they are 0.241 mmol g−1 and 0.001 mmol g−1 for CO2 and CH4, respectively. It can be seen that the adsorption capacity of the ternary mixture in brown coal follows the trend of H2O ≫ CO2 > CH4. The reasons might be as follows. The quadrupole nature of CO2 makes it easier to adsorb on the coal matrix compared to the nonpolar CH4 but harder compared to the polar H2O.40,47 Also water molecules preferentially adsorb on the oxygen and nitrogen functional group in coal structures, resulting in significant decrease of the adsorption sites for CO2 and CH4 molecules.11,48 Moreover, water molecules could adsorb each other with hydrogen bond to form clusters, which will further interfere with the adsorption of CO2 and CH4 molecules. The simulated results are consistent with the conclusions from previous experimental work by Day et al.49
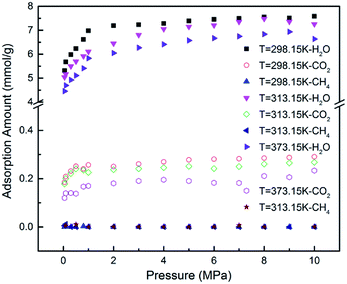 |
| Fig. 6 Competitive adsorption amount of CO2/CH4/H2O ternary mixture at different temperatures. | |
Fig. 7(a) presents the comparison of the adsorption amount of CO2/CH4 mixture in brown coal under dry and moist condition at the temperature of 298.15 K. From the simulation results, it can be seen that the adsorption amounts of CO2/CH4 are much larger than that under moist condition. The results also show that the adsorption amount would decline with the increase of moisture content. Specifically, the adsorption amount of CO2 decreases from 1.809 mmol g−1 (0H2O, dry condition) to 0.122 mmol g−1 (60H2O, 7.59 wt%) at the pressure of 8 MPa. For CH4, the value decreases from 0.0585 mmol g−1 to 0.003 mmol g−1. That is to say, the CO2 sequestration capacity would be reduced with the rise moisture content in brown coal, but the displacement efficiency of CH4 by injected CO2 could be potentially enhanced. In addition, it is found that as pressure increase, the adsorption amount of CO2/CH4 would go up either in dry or moist condition in brown coal. The adsorption selectivity of CO2/CH4 is displayed in Fig. 7(b). It is observed that the adsorption selectivities decrease with the increasing pressure. The moisture contents lower than 5.06 wt% is unfavourable to adsorption selectivity. It should be mentioned that when moisture content further increases, the adsorption selectivity would be fluctuated because the adsorption capacity of CH4 decreases to an extremely small amount under high moisture contents. The results would be helpful to determine the CO2 injection amount into the brown coal reservoir according to its specific moisture condition.
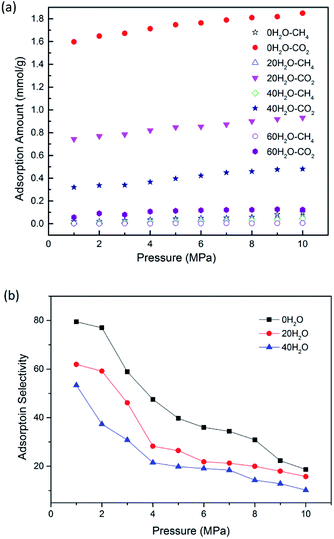 |
| Fig. 7 The adsorption amount (a); adsorption selectivity (b) of CO2/CH4 as a function of pressure at different moisture contents. | |
Self-diffusion behaviours of CO2/CH4 binary mixture
In ECBM project, the desorbed gas from the coal matrix surface together with free gas in micropores diffuse into the coal cleat-fracture system. Therefore, diffusion plays an important role of gas transport in the ECBM process. As discussed before, self-diffusion coefficient can be determined by using mean square displacement (MSD) and Einstein method. Fig. 8 shows the MSD curves of CO2 at different pressures at the temperature of 298.15 K. It is found that the slope for the pressure of 5 MPa is higher than that of 7 MPa, indicating that the self-diffusion coefficient of CO2 decreases with the rise of pressure. In another word, high pressure is disadvantageous to the diffusion of gas in brown coal, which is consistent of previous experimental research.50 The trend of self-diffusion coefficient for CO2/CH4 binary mixture with pressure in dry coal is displayed in Fig. 9. The results illustrate that the self-diffusion coefficient of both CO2 and CH4 declines with pressure. Moreover, the self-diffusion coefficient of CO2 is much higher than that of CH4 under the same condition. The reason might be that CO2 has relatively smaller kinetic diameter (0.33 nm) than that of CH4 (0.38 nm).51 Therefore, the ultramicropores in coal matrix that allow CO2 molecules to diffuse may obstruct CH4 molecules. The results are consistent with the conclusions obtained in previous research.52
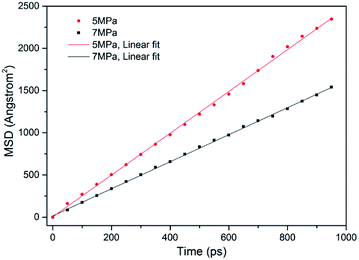 |
| Fig. 8 Mean square displacement curves of CO2 at different pressures. | |
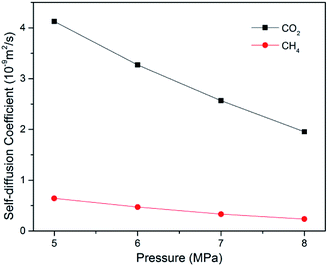 |
| Fig. 9 Self-diffusion coefficient of CO2 and CH4 as a function of pressure. | |
Fig. 10 presents the comparison of self-diffusion coefficients of CO2/CH4 in dry and moist brown coal. It is observed that the self-diffusion coefficients of CO2/CH4 in dry brown coal are higher than that in moist condition. It can be attributed to the formed water clusters in moist coal which could narrow the micropores and create energy barriers for gas diffusions. This phenomenon is consistent with the experimental work in shale and coal matrix.53,54 In addition, the self-diffusion coefficients of CO2/CH4 decrease greatly with the rise of moisture content. Compared with brown coal in dry condition, the self-diffusion coefficients of CO2 and CH4 exhibit up to 78.7% and 75.4% reductions in brown coal with 7.59 wt% moisture content, respectively. Similar results have also been reported in coal matrix and shale nanopores.53,55
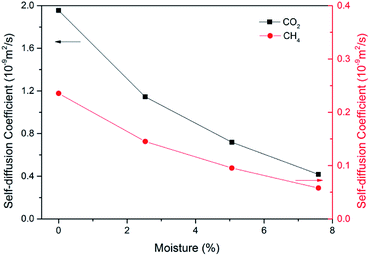 |
| Fig. 10 Self-diffusion coefficient of CO2 and CH4 as a function of moisture content. | |
Conclusions
In the present work, the competitive adsorption and diffusion characteristics of CO2/CH4/H2O mixture in brown coal were investigated by using GCMC and MD simulations. The effects of temperature, pressure and moisture content on competitive adsorption and diffusion behaviours of CO2/CH4 have been explored and discussed in detail. The following conclusions were drawn from the investigations.
(1) Simulation results show that CO2 is preferentially adsorbed over CH4 in brown coal matrix. The adsorption of CH4 is significantly suppressed at the presence of CO2. Up to 93.5% reduction in adsorption amount of CH4 has been observed in CO2/CH4 mixture compared with pure CH4.
(2) A lower temperature is favourable for the adsorption amount of binary mixture. With the rise of pressure, the adsorption amount of CO2/CH4 is enhanced but adsorption selectivity declines. Therefore, an appropriate pressure should be concerned in CO2-ECBM project.
(3) Although the adsorption of both CH4 and CO2 are remarkably reduced, the displacement efficiency of CH4 by injected CO2 could be potentially enhanced at the presence of moisture content in brown coal. A proper CO2 injection amount can be determined by the moisture condition in CO2-ECBM project.
(4) The self-diffusion coefficient of CO2 is higher than that of CH4 in either dry or moist brown coal within the studied parameters. Up to 78.7% and 75.4% reductions in self-diffusion coefficient have been observed for CO2 and CH4 in brown coal with 7.59 wt% moisture content.
Conflicts of interest
There are no conflicts to declare.
Acknowledgements
This work was supported by the National Natural Science Foundation of China (Grant No. 51706018, 51576063) and the Fundamental Research Funds for the Central Universities (Grant No. FRF-TP-18-018A2).
References
- C. McGlade, J. Speirs and S. Sorrell, Energy, 2013, 55, 571–584 CrossRef.
- A. Stephen, A. Adebusuyi, A. Baldygin, J. Shuster, G. Southam, K. Budwill, J. Foght, D. S. Nobes and S. K. Mitra, RSC Adv., 2014, 4, 22779–22791 RSC.
- T. A. Moore, Int. J. Coal Geol., 2012, 101, 36–81 CrossRef CAS.
- T. Wang and B. Lin, Renewable Sustainable Energy Rev., 2014, 39, 546–554 CrossRef.
- H. C. Lau, H. Li and S. Huang, Energy Fuels, 2017, 31, 4588–4602 CrossRef CAS.
- National Development and Reform Commission (NDRC), A strategy of Coal Bed Methane (Coal Mine Methane) Development and Utilization During the 13th Five Year Plan, 2016, http://www.ndrc.gov.cn/fzgggz/fzgh/ghwb/gjjgh/201708/W020170809596452050477.docx, (in Chinese) Search PubMed.
- B. Nie, X. Liu, L. Yang, J. Meng and X. Li, Fuel, 2015, 158, 908–917 CrossRef CAS.
- H. Li, Y. Ogawa and S. Shimada, Fuel, 2003, 82, 1271–1279 CrossRef CAS.
- Y. Huang, Q. P. Zheng, N. Fan and K. Aminian, Appl. Energy, 2014, 113, 1475–1483 CrossRef CAS.
- F. van Bergen, J. Gale, K. J. Damen and A. F. B. Wildenborg, Energy, 2004, 29, 1611–1621 CrossRef CAS.
- K. Mosher, J. He, Y. Liu, E. Rupp and J. Wilcox, Int. J. Coal Geol., 2013, 109–110, 36–44 CrossRef CAS.
- V. Prabu and N. Mallick, Renewable Sustainable Energy Rev., 2015, 50, 229–244 CrossRef CAS.
- M. S. A. Perera, Energy Fuels, 2017, 31, 10324–10334 CrossRef CAS.
- A. Busch, Y. Gensterblum, B. M. Krooss and R. Littke, Int. J. Coal Geol., 2004, 60, 151–168 CrossRef CAS.
- A. Busch, Y. Gensterblum, B. M. Krooss and N. Siemons, Int. J. Coal Geol., 2006, 66, 53–68 CrossRef CAS.
- H. Yu, J. Yuan, W. Guo, J. Cheng and Q. Hu, Int. J. Coal Geol., 2008, 73, 156–166 CrossRef CAS.
- R. Sander, L. D. Connell, Z. Pan, M. Camilleri, D. Heryanto and N. Lupton, Int. J. Coal Geol., 2014, 131, 113–125 CrossRef CAS.
- A. S. Ranathunga, M. S. A. Perera, P. G. Ranjith and C. H. Wei, Fuel, 2017, 189, 391–399 CrossRef CAS.
- M. Mukherjee and S. Misra, Earth-Sci. Rev., 2018, 179, 392–410 CrossRef CAS.
- Q. Zeng, Z. Wang, L. Liu, J. Ye, B. J. McPherson and J. D. McLennan, Energy Fuels, 2018, 32, 1942–1955 CrossRef CAS.
- Y. Liu and J. Wilcox, Environ. Sci. Technol., 2011, 45, 809–814 CrossRef CAS PubMed.
- Y. Dang, L. Zhao, X. Lu, J. Xu, P. Sang, S. Guo, H. Zhu and W. Guo, Appl. Surf. Sci., 2017, 423, 33–42 CrossRef CAS.
- Y. Song, B. Jiang and W. Li, Phys. Chem. Chem. Phys., 2017, 19, 17773–17788 RSC.
- H. Xu, W. Chu, X. Huang, W. Sun, C. Jiang and Z. Liu, Appl. Surf. Sci., 2016, 375, 196–206 CrossRef CAS.
- Y. Zhao, Y. Feng and X. Zhang, Environ. Sci. Technol., 2016, 50, 9380–9389 CrossRef CAS PubMed.
- Y. Zhao, Y. Feng and X. Zhang, Fuel, 2016, 165, 19–27 CrossRef CAS.
- W. Zhang, M. He, H. Wei, X. Zhu, X. You, X. Lyu and L. Li, Mol. Simul., 2018, 44, 769–773 CrossRef CAS.
- X. H. Xia, B. Chen, X. D. Wu, Y. Hu, D. H. Liu and C. Y. Hu, J. Cleaner Prod., 2017, 143, 125–144 CrossRef.
- M. Firouzi, E. C. Rupp, C. W. Liu and J. Wilcox, Int. J. Coal Geol., 2014, 121, 123–128 CrossRef CAS.
- J.-S. Bae and S. K. Bhatia, Energy Fuels, 2006, 20, 2599–2607 CrossRef CAS.
- J. P. Mathews and A. L. Chaffee, Fuel, 2012, 96, 1–14 CrossRef CAS.
- S. Bhoi, T. Banerjee and K. Mohanty, Fuel, 2014, 136, 326–333 CrossRef CAS.
- K. Golzar, S. Amjad-Iranagh, M. Amani and H. Modarress, J. Membr. Sci., 2014, 451, 117–134 CrossRef CAS.
- M. L. Connolly, Science, 1983, 221, 709–713 CrossRef CAS PubMed.
- B. Dai, A. Hoadley and L. Zhang, Fuel, 2017, 202, 352–365 CrossRef CAS.
- M. Rieder, J. C. Crelling, O. Šustai, M. Drábek, Z. Weiss and M. Klementová, Int. J. Coal Geol., 2007, 71, 115–121 CrossRef CAS.
- H. Sun, J. Phys. Chem. B, 1998, 102, 7338–7364 CrossRef CAS.
- J. E. Jones, Proc. R. Soc. London, Ser. A, 1924, 106, 463–477 CrossRef CAS.
- N. Metropolis, A. W. Rosenbluth, M. N. Rosenbluth, A. H. Teller and E. Teller, J. Chem. Phys., 1953, 21, 1087–1092 CrossRef CAS.
- W. Zhou, Z. Zhang, H. Wang, Y. Yan and X. Liu, RSC Adv., 2018, 8, 33939–33946 RSC.
- P. M. Mathias and T. W. Copeman, Fluid Phase Equilib., 1983, 13, 91–108 CrossRef CAS.
- J. Zhang, M. B. Clennell, K. Liu, D. N. Dewhurst, M. Pervukhina and N. Sherwood, Fuel, 2016, 177, 53–62 CrossRef CAS.
- J. Zhang, M. B. Clennell, D. N. Dewhurst and K. Liu, Fuel, 2014, 122, 186–197 CrossRef CAS.
- J. Zhang, K. Liu, M. B. Clennell, D. N. Dewhurst and M. Pervukhina, Fuel, 2015, 160, 309–317 CrossRef CAS.
- H. J. Kim, Y. Shi, J. He, H.-H. Lee and C.-H. Lee, Chem. Eng. J., 2011, 171, 45–53 CrossRef CAS.
- Q. Wang and L. Huang, Fuel, 2019, 239, 32–43 CrossRef CAS.
- S. Gautam, T. Liu and D. Cole, Molecules, 2018, 24, 99 CrossRef PubMed.
- Y. Gensterblum, A. Busch and B. M. Krooss, Fuel, 2014, 115, 581–588 CrossRef CAS.
- S. Day, R. Sakurovs and S. Weir, Int. J. Coal Geol., 2008, 74, 203–214 CrossRef CAS.
- P. Naveen, M. Asif, K. Ojha, D. C. Panigrahi and H. B. Vuthaluru, Energy Fuels, 2017, 31, 6825–6837 CrossRef CAS.
- X. G. Zhang, P. G. Ranjith, M. S. A. Perera, A. S. Ranathunga and A. Haque, Energy Fuels, 2016, 30, 8832–8849 CrossRef CAS.
- J. Han, A. K. Bogomolov, E. Y. Makarova, Z. Yang, Y. Lu and X. Li, Energy Fuels, 2017, 31, 13528–13535 CrossRef CAS.
- Z. Pan, L. D. Connell, M. Camilleri and L. Connelly, Fuel, 2010, 89, 3207–3217 CrossRef CAS.
- W. Yuan, Z. Pan, X. Li, Y. Yang, C. Zhao, L. D. Connell, S. Li and J. He, Fuel, 2014, 117, 509–519 CrossRef CAS.
- S. Wang, Q. Feng, M. Zha, F. Javadpour and Q. Hu, Energy Fuels, 2018, 32, 169–180 CrossRef CAS.
|
This journal is © The Royal Society of Chemistry 2019 |
Click here to see how this site uses Cookies. View our privacy policy here.