DOI:
10.1039/C8RA07572G
(Paper)
RSC Adv., 2019,
9, 3351-3358
High-throughput metabolic profiling, combined with chemometrics and bioinformatic analysis reveals functional alterations in myocardial dysfunction†
Received
11th September 2018
, Accepted 15th January 2019
First published on 24th January 2019
Abstract
High-throughput metabolic profiling technology has been used for biomarker discovery and to reveal underlying metabolic mechanisms. Sepsis-induced myocardial dysfunction (SMD) is a common complication in sepsis patients, and severely affects their quality of life. However, the pathogenesis of SMD is currently unclear, and there has been inadequate basic research. In this study, metabolic profiling was explored by liquid chromatography/mass spectrometry (LC/MS) combined with chemometrics and bioinformatic analysis. The global metabolome data were analyzed using chemometrics analysis including principal component analysis and partial least squares discriminant analysis for significant metabolites. Variable importance for projection values obtained utilizing a pattern recognition method were used to identify potential biomarkers. The differential metabolites were putatively identified using the metabolome database and bioinformatics analysis was conducted via Ingenuity Pathway Analysis (IPA) to predict the likely functional alterations. In total, 21 differential metabolites were found in SMD and these were involved in phenylalanine, tyrosine and tryptophan biosynthesis, arachidonic acid metabolism, glycine, serine and threonine metabolism, and so on. The analysis revealed that the metabolites were strongly related to molecular transport, and small molecule biochemistry metabolic pathways. The present study indicates that high-throughput metabolic profiling, combined with chemometrics and a bioinformatic platform, can reveal the likely functional alterations in disease and could provide more precise and credible information in the basic research of disease pathogenesis.
Introduction
Sepsis-induced myocardial dysfunction (SMD) is a leading cause of death in intensive care units (ICU) with high morbidity and mortality worldwide.1,2 Numerous studies have indicated that SMD exists in the high output stage of sepsis. Indeed, septic patients with SMD have a 70% chance of mortality compared to a 20% mortality rate for patients without myocardial dysfunction.3,4 Multiple mechanisms have been suggested to underlie myocardial dysfunction, including inflammatory responses, myocardial and mitochondrial disorders, and apoptosis.5 SMD contributes to a high risk of mortality and is linked to a detrimental prognosis of sepsis.6 The molecular mechanism of SMD has been studied with regard to cytokines, apoptosis, immune regulation, mitochondria, and so on.7–9 However, the precise metabolic mechanisms and their role in the pathogenesis of SMD remain incompletely understood.
Clinical studies confirmed that myocardial dysfunction is the main clinical manifestation in sepsis.10 Therefore, it is critical that we clarify the mechanisms of SMD and explore effective treatments. Lipopolysaccharide (LPS)-induced myocardial injury is an animal model that is commonly used to assess sepsis-induced cardiac dysfunction.11 Experimentally, the administration of LPS to laboratory animals, especially mice, has been widely used to study the mechanisms of SMD.12 Metabolomics, the study of a biologic process involving all of the metabolites in a whole organism, has been integrated into multidisciplinary methods to profile changes in small molecules associated with disease.13–15 Metabolomics has been applied to identify metabolic biomarkers in several human diseases.16–18 Ultra-performance liquid chromatography (UPLC) coupled with mass spectrometry (MS) has higher resolution, high sensitivity and rapid separation, and has been widely used to investigate metabolite alterations in disease.19,20
For the reasons given above, we hypothesized that metabolomics is a reliable method to assess LPS-induced SMD in a mouse model. Accordingly, metabolic profiling was explored by integrating a UPLC/MS platform with bioinformatics analysis and chemometrics analysis. Variable importance for projection values obtained using a chemometrics method were used to identify potential biomarkers. The differential metabolites were putatively identified and bioinformatics analysis was conducted via Ingenuity Pathway Analysis (IPA) to identify the predicted pathways and biological functions in a well-established mouse model of SMD.
Experimental methods
Reagents and materials
Acetonitrile, HPLC grade, was provided by Merck (Germany). Leucine enkephalin was supplied from Sigma-Aldrich (St. Louis, MO, USA). Water was supplied from the Milli-Q ultra-pure water system (Millipore, Billerica, USA). Formic acid, HPLC grade, was provided by Tedia (USA). LPS was supplied by Sigma (St. Louis, MO, USA).
Animals
Male C57BL/6J mice (8–10 weeks old, weighing 20–24 g) were obtained from the Animal Research Center of Heilongjiang University of Chinese Medicine. The animals were kept with a 12 h/12 h light/dark cycle at 21–23 °C and fed a standard chow diet ad libitum before experiments.
Ethical statement
The experimental procedures were approved by the Animal Care and Ethics Committee at Heilongjiang University of Chinese Medicine and all experiments were performed in accordance with the Declaration of Helsinki.
Lipopolysaccharide injection
LPS administration was used to induce sepsis as previously described.21 E. coli LPS was dissolved in sterile physiological saline solution (0.9% NaCl) at a concentration of 1 mg mL−1. Forty mice were randomized into the following two groups: the control group (n = 20), the treatment group (n = 20). The treatment and control animals were injected with either 0.5 mL normal saline solution containing LPS (10 mg kg−1 body weight, i.p.) or 0.5 mL normal saline only. This dose of LPS was based on previous observations of overt myocardial dysfunction.22 At the end of the experiments, blood collection was performed, and the hearts were removed for histological analyses.
Histopathological examination
At the end of the experiment, the whole heart was removed. Whole hearts were fixed in 10% formalin, embedded in paraffin, and sectioned onto slides. Serial sections of 4 μm were cut for Haematoxylin and Eosin staining (H&E) analysis.
Cytokine measurements
Blood was collected from the experimental and control mice to determine the serum levels of cytokines. Samples were allowed to clot for 2 h at room temperature before centrifugation for 10 min at 2000 g. The serum was then removed and stored at −80 °C for subsequent enzyme-linked immunosorbent assay (ELISA) analysis. The levels of cytokines (tumor necrosis factor-α (TNF-α), interleukin-4 (IL-4)) were examined using a Quantikine Mouse ELISA kit (R&D Systems, MN).
Sample preparation
Sample preparation for the serum metabolomics analysis was conducted as follows: prior to analysis, 100 μL serum samples were thawed at 4 °C, followed by vigorous vortexing for 2 min, centrifugation for 10 min at a speed of 5000 rpm at 4 °C, and the supernatant was then filtered through a 0.22 μm membrane. The supernatant was placed into an auto sampler vial for UPLC/QTOF-MS/MS analysis in positive and negative electrospray ionization (ESI) mode. A mixed sample containing aliquots of all the serum samples was prepared as the quality control (QC) sample. The QC sample was analyzed once for every 10 test samples.
UPLC/QTOF-MS/MS analysis
We transferred a pretreated sample (2 μL) into a 2.1 μm × 100 μm × 1.7 μm C8 column using a UPLC system (Waters Corp., Milford, USA). The column temperature was maintained at 45 °C. The gradient consisted of 0.1% formic acid in water as mobile phase A and 0.1% formic acid in acetonitrile as mobile phase B. The positive ion and negative ion mode involved 1–15% acetonitrile for 0–3.0 min, 15–50% for 3.0–5 min and 50–98% for 5–10 min; the concentration was held at 98% for 2 min. The injection volume was 3 μL and the flow rate was 0.4 mL min−1. MS analysis was performed using a Waters Micromass Q-TOF/MS system (Waters Corp., Milford, USA). The parameters were set up as follows: desolvation temperature of 250 °C, source temperature of 100 °C, capillary voltage of 3000 V, cone voltage of 25 V, extraction cone voltage of 3 V, desolvation gas flow of 600 L h−1, collision energy of 5 eV. MS data were collected from 50 to 1000 m/z in full scan mode.
Metabolite identification
MS/MS experiments were conducted to obtain fragmentation patterns of selected metabolites for determining their structures, and accurate molecular weights were also calculated. Accurate mass data and isotopic distributions for the precursor and product ions were compared to the spectral data. The mass tolerance between measured m/z values and exact mass was set to within 5 ppm. The metabolite product ion spectrum with its structure information was confirmed using MassFragment software. The relevant pathways of potential endogenous metabolites were assessed using the online website MetaboAnalyst 3.0.23
Metabolic pathway analysis
Ingenuity Pathway Analysis (IPA, Ingenuity® Systems, http://www.ingenuity.com, Redwood City, CA, USA) enables bioinformaticians to identify the biological functions which are most relevant to their work. The “core analysis” module in the IPA analysis system was used to analyze the differential metabolites and build networks of the differentially regulated metabolites. Canonical pathway analysis of those metabolites was also confirmed, with the criterion that a score of higher than 1 was used to obtain the most enriched network.
Statistical analysis
MS data were imported to MarkerLynx (Waters Corporation) for peak detection and alignment. We used the Pareto scaling method for data transformation before multivariate statistical analysis. Chemometrics analyses including principal component analysis (PCA) and orthogonal partial least squares discriminant analysis (OPLS-DA) were performed using EZinfo software (V2, Waters Corp., Milford, USA). VIP values (variable importance for projection) provided by OPLS-DA were used to evaluate the variable contribution and find potential biomarkers. All statistical tests were two-sided, with all statistical analyses performed using SPSS software (Version 17.0, SPSS Inc., Chicago, Illinois). Differences with p < 0.05 were considered statistically significant.
Results and discussion
Histological sections of the hearts treated with LPS were analyzed (Fig. S1†). In the control animals, the myocardiocytes were in healthy shape and were well aligned. The sections demonstrated the occurrence of extravasation of red blood cells, and hyperemia of blood vessels. The treatment group showed more severe damage than the control group (Fig. S1A†). Fig. S1B† shows the levels of TNF-α and IL-4 in serum from mice subjected to LPS-induced sepsis. The treatment group showed significantly increased concentrations of TNF-α and IL-4 in the serum when compared with control mice. In our study, we applied UPLC/MS to collect metabolite information with respect to SMD. Based on the PCA, none of the samples were identified as outliers and excluded from further statistical analysis (Fig. 1 and S2†). The OPLS-DA approach was established to reveal the specific metabolic changes in the treatment and control groups and to improve their separation. The treatment and control mice could be separated into two distinct clusters, which indicated that the metabolic states were different between the treatment and control groups, and as displayed by the score plots. The loading ion plots of the OPLS-DA model built from treatment and control samples are presented in Fig. 2 and S3.†
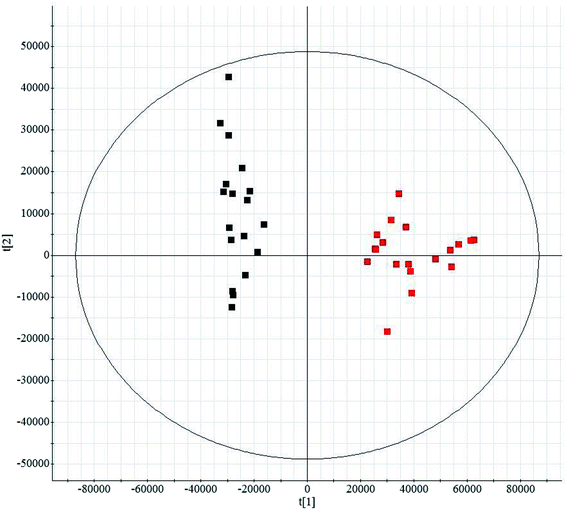 |
| Fig. 1 Principal component analysis score plot of the UPLC/MS serum metabolite data obtained in positive ion mode by liquid chromatography/mass spectrometry. Note: black, control group; red, treatment group. | |
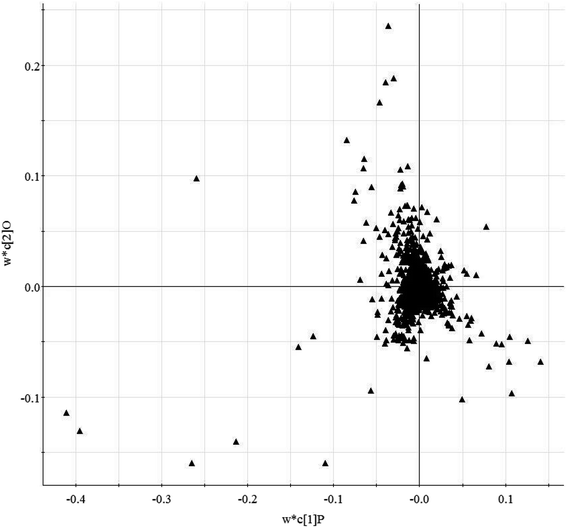 |
| Fig. 2 Loading-plot of the serum metabolite data obtained in positive ion mode by liquid chromatography/mass spectrometry (R2X = 0.91, R2Y = 0.77, Q2Y = 0.82). | |
In this study, an untargeted UPLC/MS technique was performed to investigate the metabolic signatures in serum samples from SMD mice. In total, 3601 variables were selected in the final data table for subsequent analyses. To screen the putative biomarkers for SMD using multivariate analysis, VIP values, which are based on weighted coefficients, were obtained from the OPLS-DA model. The key metabolites, which were essential for distinguishing between the treatment and control cases, were selected from the VIP plot (Fig. 3 and S4†). A total of 21 potential metabolites such as pyroglutamic acid, 5-aminopentanoic acid, p-aminobenzoic acid, citric acid, L-tyrosine, pipecolic acid and L-methionine were selected, on the basis of the VIP (VIP > 10) and Student's t test (p < 0.05), and significant differences between treatment and control groups were also shown (Table S1†). This suggests that metabolic profiling might be helpful in determining the metabolic phenotype of SMD disease. The change in metabolite concentrations is shown in a heatmap (Fig. 4).
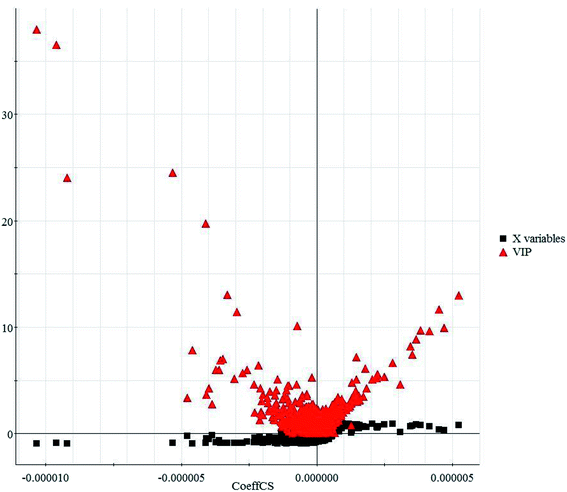 |
| Fig. 3 VIP-plot based on the matched partial least squares discriminant analysis model for the interesting variables in the positive ion mode. | |
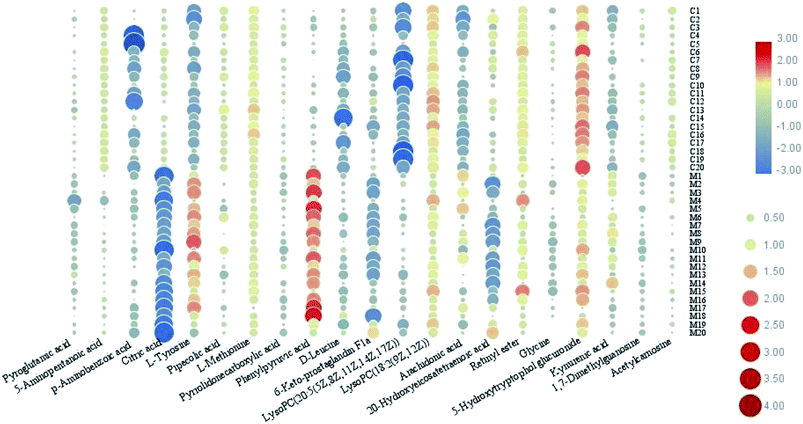 |
| Fig. 4 The relative concentration changes in serum biomarkers in SMD mice. | |
Myocardial dysfunction is a common clinical manifestation in sepsis.24 SMD is being studied extensively. However, its pathogenesis is still unknown. Metabolomics is the non-targeted analysis of global changes in metabolites and a potential tool for discovering new biomarkers for disease.25–27 The identified biomarkers of SMD were imported into the MetPA website to analyze the relevant metabolic pathways. A total of 20 metabolic pathways were involved in disturbances relating to phenylalanine, tyrosine and tryptophan biosynthesis, arachidonic acid metabolism, glycine, serine and threonine metabolism, glyoxylate and dicarboxylate metabolism, phenylalanine metabolism, retinol metabolism, and tyrosine metabolism (Impact > 0.1, Fig. S5, Table S2†). These results indicate that endogenous metabolites demonstrate a strong disturbance in the whole metabolic profile which is closely connected with the SMD model.
In the present study, IPA was used to analyze the differentiated metabolites and associated network relationships, to examine the metabolic pathways that were most changed. The most significantly altered network was ‘amino acid metabolism, molecular transport and small molecule biochemistry’ (Fig. 5), with a score above 3. Based on IPA analysis, certain metabolites (1-18:2(9Z,12Z)lysophosphatidylcholine, 20-hydroxyeicosatetraenoic acid, arachidonic acid, citric acid, kynurenic acid, L-methionine, L-tyrosine, and phenylpyruvic acid) are involved in and play a central role in this network. These data strongly suggest that disturbance within this metabolism is characteristic for SMD.
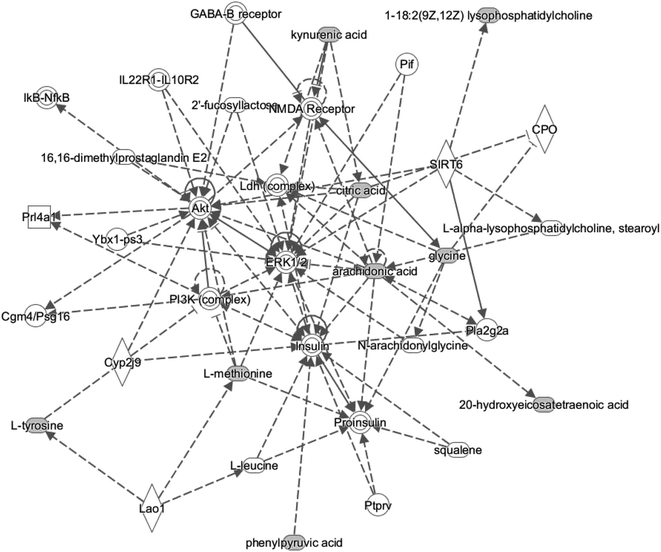 |
| Fig. 5 Network analysis of the differential metabolites using the Ingenuity Pathway Analysis tool. | |
SMD increases mortality in sepsis, and myocardial dysfunction is the leading cause of death in patients with sepsis, but the underlying pathophysiology of myocardial dysfunction is not completely understood, which limits effective therapy for this disorder. Experimentally, the administration of LPS to mice has been widely used to study the mechanisms of SMD. Despite more than three decades of clinical and basic research on SMD, there is no gold standard for evaluating cardiac function in sepsis. Recent research demonstrated that TNF-α and IL-4 served as indicators of damaged cardiac myocytes in SMD.28 In our study, we observed that two indexes (TNF-α and IL-4) increased in mice after LPS administration when compared with controls. We provide evidence that increased expression of TNF-α and IL-4 in serum from mice may be involved in the pathogenesis of SMD. Taken together, histological and serum results confirmed that myocardial injury occurred after LPS administration in the mouse model.
Previous research has identified amino acids measured using mass spectrometry in the plasma of septic patients.29–33 The differential metabolite expression may provide more reliable biomarkers for sepsis. A study explored the dynamic concentration profiles of amino acids and found that the metabolic spectrum of the amino acids changed dramatically in sepsis patients.34 In this study, high-throughput metabolic phenotyping was used to characterize sensitive and economical peripheral biomarker(s) associated with SMD. The PCA score plot showed significant discrimination between the treatment and control cases. Serum metabolite profiling showed SMD to be associated with a profound abnormality in the metabolic phenotype. Metabolic pathway analysis indicated that endogenous metabolites such as pyroglutamic acid, 5-aminopentanoic acid, p-aminobenzoic acid, citric acid, L-tyrosine, pipecolic acid and L-methionine, which are closely connected with SMD, demonstrate a highly disturbed metabolism. Altogether, our results represent a good foundation for the full understanding of the pathogenesis of SMD and will provide beneficial effects for septic patients in the near future.
Conclusions
SMD increases mortality in sepsis, yet the underlying mechanism is unclear. Early recognition of intrinsic myocardial dysfunction is critical for the administration of the most appropriate therapy for septic patients. New approaches to allow a deeper understanding of the underlying mechanism should help to improve the prognosis of patients with myocardial dysfunction in the near future. In this work, high-throughput UPLC/MS-based metabolic phenotyping was used to characterize serum biomarkers associated with the SMD metabolome. We found increased levels of pyroglutamic acid, 5-aminopentanoic acid, p-aminobenzoic acid, citric acid, L-tyrosine, pipecolic acid and L-methionine as compared to the control group. Further analysis of metabolic pathway variation indicated that many of the changed metabolites were involved in phenylalanine, tyrosine and tryptophan biosynthesis, arachidonic acid metabolism, glycine, serine and threonine metabolism, glyoxylate and dicarboxylate metabolism, phenylalanine metabolism, retinol metabolism, and tyrosine metabolism. The elucidation of these mechanisms should help identify new and specific therapeutic targets for septic patients. Our results demonstrate that integrating a metabolomics platform with chemometrics and bioinformatics is a promising approach for systematically dissecting the underlying metabolic mechanism of disease.
Conflicts of interests
The authors declare no competing interests.
Acknowledgements
This work was supported by grants from the Key Program of Natural Science Foundation of State (Grant No. 81774276, 81470196).
References
- T. Suzuki, Y. Suzuki and J. Okuda, et al., Sepsis-induced cardiac dysfunction and β-adrenergic blockade therapy for sepsis, Journal of Intensive Care, 2017, 5(1), 22 CrossRef PubMed.
- R. Neviere, F. Delguste and A. Durand, et al., Abnormal Mitochondrial cAMP/PKA Signaling Is Involved in Sepsis-Induced Mitochondrial and Myocardial Dysfunction, Int. J. Mol. Sci., 2016, 17(12), 2075 CrossRef PubMed.
- A. C. S. Freitas, M. J. Figueiredo and E. C. Campos, et al., Activation of Both the Calpain and Ubiquitin-Proteasome Systems Contributes to Septic Cardiomyopathy through Dystrophin Loss/Disruption and mTOR Inhibition, PLoS One, 2016, 11(11), e0166839 CrossRef PubMed.
- K. R. Walley, New Approaches to Modifying Inotropy in Sepsis-Induced Myocardial Dysfunction, Crit. Care Med., 2017, 45(4), 754–756 CrossRef PubMed.
- T. Janušonis and R. Paškevičiūtė, Clinical Case: Sepsis-Induced Cardiac Dysfunction, Health Sciences, 2016, 26(5), 26–28 CrossRef.
- Y. Zhou, Y. Song, Z. Shaikh, H. Li, H. Zhang, Y. Caudle, S. Zheng, H. Yan, D. Hu, C. Stuart and D. Yin, MicroRNA-155 attenuates late sepsis-induced cardiac dysfunction through JNK and β-arrestin 2, Oncotarget, 2017, 8(29), 47317–47329 CrossRef PubMed.
- Y. Okuhara, S. Yokoe and T. Iwasaku, et al., Interleukin-18 gene deletion protects against sepsis-induced cardiac dysfunction by inhibiting PP2A activity, Int. J. Cardiol., 2017, 243, 396–403 CrossRef PubMed.
- Z. Zheng, H. Ma and X. Zhang, et al., Enhanced Glycolytic Metabolism Contributes to Cardiac Dysfunction in Polymicrobial Sepsis, J. Infect. Dis., 2017, 215(9), 1396–1406 CrossRef CAS PubMed.
- Q. Liang, H. Liu, H. Xing, Y. Jiang and A. Zhang, Urinary UPLC-MS metabolomics dissecting the underlying mechanisms of huaxian capsule protects against sepsis, RSC Adv., 2016, 6, 40436–40441 RSC.
- R. L. Wilson, V. Selvaraju and R. Lakshmanan, et al., Thioredoxin-1 attenuates sepsis-induced cardiomyopathy after cecal ligation and puncture in mice, J. Surg. Res., 2017, 220, 68–78 CrossRef CAS PubMed.
- E. Delile, R. Nevière and P. A. Thiébaut, et al., Reduced insulin resistance contributes to the beneficial effect of protein tyrosine phosphatase-1B deletion in a mouse model of sepsis, Shock, 2017, 48(3), 355–363 CrossRef CAS PubMed.
- T. Fan, X. Feng and Y. Yang, et al., Downregulation of PI3K-γ in a mouse model of sepsis-induced myocardial dysfunction, Cytokines, 2017, 96, 208–216 CrossRef CAS PubMed.
- Q. Liang, Y. Zhu and H. Liu, et al., High-throughput lipidomics enables discovery of the mode of action of huaxian capsule impacting the metabolism of sepsis, RSC Adv., 2017, 7(71), 44990–44996 RSC.
- Y. Zhao, H. Lv and S. Qiu, et al., Plasma metabolic profiling and novel metabolite biomarkers for diagnosing prostate cancer, RSC Adv., 2017, 7(48), 30060–30069 RSC.
- Q. Liang, H. Liu and L. Xie, et al., High-throughput and multi-dimensional omics approach uncovers a huaxian capsule to ameliorate the dysregulated expression profiling of severe sepsis rats, RSC Adv., 2017, 7(32), 19894–19903 RSC.
- Q. Liang, H. Liu, L. Xie, X. Xue Li and A. H. Zhang, High-throughput metabolomics enables biomarker discovery in prostate cancer, RSC Adv., 2017, 7, 2587–2593 RSC.
- Q. Liang, H. Liu and L. Xie, et al., High-throughput metabolomics enables biomarker discovery in prostate cancer, RSC Adv., 2017, 7(5), 2587–2593 RSC.
- X. Wang, J. Li and A. H. Zhang, Urine metabolic phenotypes analysis of extrahepatic cholangiocarcinoma disease using ultra-high performance liquid chromatography-mass spectrometry, RSC Adv., 2016, 6(67), 63049–63057 RSC.
- Q. Liang, H. Liu and Y. Jiang, et al., Novel liquid chromatography-mass spectrometry for metabolite biomarkers
of acute lung injury disease, Anal. Methods, 2016, 8, 6017 RSC.
- Q. Liang, H. Liu and H. Xing, et al., UPLC-QTOF/MS based metabolomics reveals metabolic alterations associated with severe sepsis, RSC Adv., 2016, 6, 43293–43298 RSC.
- H. Y. Chung, A. S. Kollmey and A. Schrepper, et al., Adjustment of Dysregulated Ceramide Metabolism in a Murine Model of Sepsis-Induced Cardiac Dysfunction, Int. J. Mol. Sci., 2017, 18(4), 839 CrossRef PubMed.
- R. L. Wilson, V. Selvaraju and R. Lakshmanan, et al., Thioredoxin-1 attenuates sepsis-induced cardiomyopathy after cecal ligation and puncture in mice, J. Surg. Res., 2017, 220, 68–78 CrossRef CAS PubMed.
- J. Xia, I. V. Sinelnikov, B. Han and D. S. Wishart, MetaboAnalyst 3.0--making metabolomics more meaningful, Nucleic Acids Res., 2015 Jul 1, 43(W1), W251–W257 Search PubMed.
- R. L. Wilson, V. Selvaraju and R. Lakshmanan, et al., Thioredoxin-1 attenuates sepsis-induced cardiomyopathy after cecal ligation and puncture in mice, J. Surg. Res., 2017, 220, 68–78 CrossRef CAS PubMed.
- Q. Liang, T. Zhang, Y. Jiang, H. Xing, C. Wang, B. Li and A. Zhang, Metabolomics of Alcoholic Liver Disease: A Clinical Discovery Study, RSC Adv., 2015, 5, 80381–80387 RSC.
- Q. Liang, H. Liu, H. Xing, Y. Jiang, T. Zhang and A. Zhang, High-resolution mass spectrometry for exploring metabolic signatures of sepsis-induced acute kidney injury, RSC Adv., 2016, 6, 29863–29868 RSC.
- Q. Liang, H. Liu, T. Zhang, Y. Jiang, H. Xing and A. Zhang, High-throughput metabolic profiling for discovering metabolic biomarkers of sepsis-induced acute lung injury, RSC Adv., 2016, 6, 11008–11013 RSC.
- O. Nduka and J. E. Parrillo, The pathophysiology of septic shock, Crit. Care Nurs. Clin. North Am., 2011, 23(1), 41–66 CrossRef PubMed.
- A. Tesfai, N. MacCallum and N. S. Kirkby, et al., Metabolomic profiling of amines in sepsis predicts changes in NOS canonical pathways, PLoS One, 2017, 12(8), e0183025 CrossRef PubMed.
- Y. Zhang, P. Liu, Y. Li and A. H. Zhang, Exploration of metabolite signatures using high-throughput mass spectrometry coupled with multivariate data analysis, RSC Adv., 2017, 7(11), 6780–6787 RSC.
- Y. F. Li, S. Qiu and L. J. Gao, et al., Metabolomic estimation of the diagnosis of hepatocellular carcinoma based on ultrahigh performance liquid chromatography coupled with time-of-flight mass spectrometry, RSC Adv., 2018, 8(17), 9375–9382 RSC.
- Y. Zhao, H. Lv and S. Qiu, et al., Plasma metabolic profiling and novel metabolite biomarkers for diagnosing prostate cancer, RSC Adv., 2017, 7(48), 30060–30069 RSC.
- Y. Zhao, H. Lv and S. Qiu, et al., Plasma metabolic profiling and novel metabolite biomarkers for diagnosing prostate cancer, RSC Adv., 2017, 7(48), 30060–30069 RSC.
- L. Su, H. Li and A. Xie, et al., Dynamic changes in amino acid concentration profiles in patients with sepsis, PLoS One, 2015, 10(4), e0121933 CrossRef PubMed.
Footnote |
† Electronic supplementary information (ESI) available. See DOI: 10.1039/c8ra07572g |
|
This journal is © The Royal Society of Chemistry 2019 |