DOI:
10.1039/C9NR03040A
(Review Article)
Nanoscale, 2019,
11, 22152-22171
Sensing of circulating cancer biomarkers with metal nanoparticles
Received
9th April 2019
, Accepted 24th August 2019
First published on 27th August 2019
Abstract
The analysis of circulating cancer biomarkers, including cell-free and circulating tumor DNA, circulating tumor cells, microRNA and exosomes, holds promise in revolutionizing cancer diagnosis and prognosis using body fluid analysis, also known as liquid biopsy. To enable clinical application of these biomarkers, new analytical tools capable of detecting them in very low concentrations in complex sample matrixes are needed. Metal nanoparticles have emerged as extraordinary analytical scaffolds because of their unique optoelectronic properties and ease of functionalization. Hence, multiple analytical techniques have been developed based on these nanoparticles and their plasmonic properties. The aim of this review is to summarize and discuss the present development on the use of metal nanoparticles for the analysis of circulating cancer biomarkers. We examine how metal nanoparticles can be used as (1) analytical transducers in various sensing principles, such as aggregation induced colorimetric assays, plasmon resonance energy transfer, surface enhanced Raman spectroscopy, and refractive index sensing, and (2) signal amplification elements in surface plasmon resonance spectroscopy and electrochemical detection. We critically discuss the clinical relevance of each category of circulating biomarkers, followed by a thorough analysis of how these nanoparticle-based designs have overcome some of the main challenges that gold standard analytical techniques currently face, and what new directions the field may take in the future.
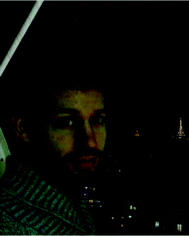 Roger M. Pallares | Roger M. Pallares: After working on the growth of 2D nanomaterials at NTT Basic Research Laboratories (Japan), he started a joint doctoral program between University College London (UCL, UK) and the Agency for Science, Technology and Research (Singapore), obtaining a PhD in materials science from UCL in 2016. He has worked as a postdoctoral scholar at Northwestern University (IL, USA), and is currently a postdoctoral fellow at Lawrence Berkeley National Laboratory (CA, USA). His research interests focus on the use of nanomaterials for biomedical applications. |
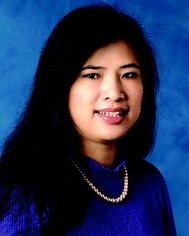 Nguyen Thi Kim Thanh | Professor Nguyen T. K. Thanh, FRSC, FIMMM, FInstP, FRSB (http://www.ntk-thanh.co.uk), held a prestigious Royal Society University Research Fellowship (2005–2014) and was promoted to full professor of nanomaterials in 2013 at University College London. She conducts cutting edge interdisciplinary and innovative research on the design, chemical synthesis, and physical characterization of magnetic and plasmonic nanomaterials for biomedical applications. In collaboration with physicists, material scientists, and chemical engineers she has produced the next generation of nanoparticles with very high magnetic moment, fine tuning Au nanorods, and novel hybrid and multifunctional nanostructures. Detailed mechanistic studies of their formation by sophisticated and advanced analysis of the nanostructure allow tuning of the physical properties at the nanoscale; these can subsequently be exploited for diagnosis and treatment of various diseases such as cancers in collaboration with biologists, biochemists and clinicians. She has published over 100 peer reviewed journal articles and book chapters, books and theme issues. Among them 10 papers were featured as cover pages. Her research has accrued over 9000 citations, with an average of 65 citations per item, giving her an i10 index of over 50, and notably she has 12 papers with over 100 citations, with 1 research paper attracting an exceptional over 2300 citations. |
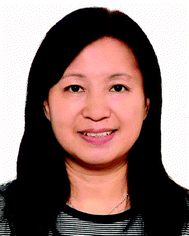 Xiaodi Su | Professor Xiaodi Su received her PhD in analytical chemistry from Nankai University (China) in 1995. Currently she is a senior scientist and strategic research office councillor in the Institute of Materials Research and Engineering (IMRE), A*STAR, Singapore. She is also an adjunct associate professor in the Department of Chemistry at the National University of Singapore. Her research interests include developing advanced analytical technologies and nanomaterial-based biosensors for medical diagnosis, environmental monitoring, and food analysis. |
1. Introduction
Tissue biopsy is a gold standard technique for the investigation and diagnosis of cancer. Nevertheless, the removal of patient tissue presents important limitations in terms of sample acquisition and information obtained. As an example, one of the main challenges in tissue biopsy is cancer heterogeneity,1 which can exist within the same tumor (intra-tumoral heterogeneity) and between metastases in the same patient (inter-metastatic heterogeneity).2 Therefore, the extraction of tissue from a specific solitary tumor may not provide the whole information of the patient condition. However, multiple tissue extractions are not recommended to overcome cancer heterogeneity because every extraction increases the risk of spreading the disease to other parts of the body,3 and tissue biopsies present clinical complications (e.g. 17.1% of thoracic biopsies have been reported to result in adverse events4). Furthermore, the location of some tumors makes the sample extraction very challenging or impossible.5
Liquid biopsy, as an alternative to tissue/solid biopsy, is less invasive and more robust against cancer heterogeneity. The analysis and quantification of cancer-related biomarkers from various body fluids, including blood,6,7 urine,8 saliva9 and cerebrospinal fluid,10 present significant advantages: (1) body fluids are a fresh source of biomarkers; (2) the samples are obtained through non-invasive or minimally-invasive procedures; and (3) liquid samples can be collected at any point throughout the course of the therapy, providing dynamic information regarding the tumor evolution. Nevertheless, all these benefits are hampered by the lack of sensitivity or specificity of most protein cancer biomarkers, such as prostate specific antigen (PSA)11,12 or carcinoembryonic antigen (CEA).13 Thus, liquid biopsies for these proteins are only used as supplementary diagnostic tools. In addition, the analysis of cancer biomarkers has been mostly focused on disease diagnosis, rather than prognosis, which could have the potential to improve the treatment and disease management.
During the last few years, increasing amount of research has been published regarding new kinds of circulating biomarkers, such as cell-free and circulating tumor DNA,14 microRNA,15 circulating tumor cells16 and exosomes.17 They have been identified as potential biomarkers with unique clinical opportunities for diagnosis and prognosis of cancer because of (1) their high stability in body fluids; (2) concentration-dependence of disease states; and (3) rapid clearance from the bloodstream, providing real-time information of the patient condition.18 However, their applications in the clinic have been limited by low concentration and high heterogeneity (in some cases). New sensing methodologies with high sensitivity, tolerance to complex matrix backgrounds and multiplex detection are required to promote the clinical applications of circulating biomarkers.
Noble metals have been used in medicine throughout the history of civilization.19,20 For instance, Egyptians were already using gold in dentistry around 4500 years ago,21 Persians used to stock clean water in silver containers in order to avoid contamination22 and the Hippocratic Corpus, an ancient medical Greek book written in the 5th century B.C., described the use of gold wires in jaw fractures.23 During the past couple of decades, significant progress in colloidal and surface chemistry has resulted in a significant volume of basic and applied research of noble metals at the nanoscale, such as metal nanoparticles (mNPs).24–26 The size-dependent physicochemical properties of mNPs have allowed them to be used as sensitive transductors for bioassays and sensors to detect various biological events through color change, fluorescence modulation and spectroscopy enhancement.27–29 These extended applications are based on mNPs being easily functionalized by many recognition elements, such as DNA, proteins and antibodies, that provide selectivity towards different biological targets.27,30,31 The combination of highly versatile nanoparticle sensing principles with recognition elements has resulted in bioassays with fast responses and visual outcome, suitable for use in resource limited environments (Scheme 1).32
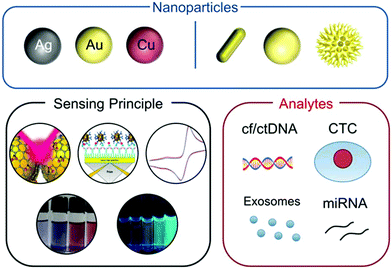 |
| Scheme 1 Schematic representation of the main components on the sensing of circulating cancer biomarkers by mNPs covered in this review. Adapted with permission from ref. 33 (Copyright 2011 Elsevier), ref. 34 (Copyright 2010 Royal Society of Chemistry), ref. 35 (Copyright 2018 Materials Research Society), ref. 36 (Copyright 2010 American Chemical Society), and ref. 37 (Copyright 2012 American Chemical Society). | |
The great interest surrounding circulating cancer biomarkers for cancer diagnosis has been shown in the publication of numerous reviews focusing on the biology17,38–40 and clinical aspects of these biomarkers14,16,41,42 as well as the different techniques used to analyze them,43–46 including some based on nanomaterials and nanoparticles.47,48 There is not yet, however, a critical review that (1) critically evaluates the status of circulating cancer biomarker based diagnosis, such as the common techniques used in the analysis and their current limitations, (2) describes how methods based on mNPs can overcome these limitations and (3) discusses what possibilities nanosensing may offer in the coming years. In this review we bridge this gap by analyzing the recent progress in using mNPs for circulating cancer biomarker sensing. We introduce the topic by summarizing the fundamentals of mNPs and their sensing principles; we also describe the clinical opportunities that each circulating cancer biomarker offers. We then evaluate the analytical challenges that MNP-based assays have overcome and further comment on the great potential that mNP-based biosensors hold for the future.
2. Metal nanoparticles as sensing materials
2.1. Localized surface plasmon resonance
The unique optical and electronic properties of mNPs are the result of the collective oscillation of the conduction band electrons when perturbed by an external electromagnetic radiation.49 This electromagnetic force pushes away the electron cloud from the equilibrium position, inducing a surface polarization that promotes the oscillating movement and restores the system's equilibrium (Fig. 1a). This phenomenon occurs at very specific light frequencies and is called localized surface plasmon resonance (LSPR).50 The first quantitative explanation of LSPR appeared in 1908, when Gustav Mie solved Maxwell's electromagnetic equations for small spherical gold particles.51 Despite that pioneering work, the unusual optical properties of mNPs did not achieve widespread popularity until two decades ago, when different morphologies were finally accessible through newly developed synthetic protocols.52,53 The new colloidal chemistry combined with improvement in computing power provided a deeper understanding and refreshed the interest on the interaction between mNPs and light.
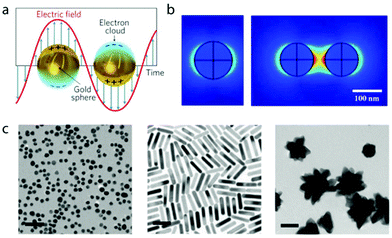 |
| Fig. 1 (a) Schematic representation of electron collective oscillation with the incident electromagnetic field in gold nanoparticles. Adapted with permission from ref. 61 (Copyright 2011 Springer Nature). mNPs of other materials, such as silver or copper, also exhibit LSPR. (b) Simulation of the electromagnetic field (V m−1) for a single 100 nm gold nanoparticle and a dimer with incident 633 nm wavelength light. Adapted with permission from ref. 62 (Copyright 2011 MDPI). (c) Polymer-coated AuNPs with spherical, rod and star morphologies. Adapted with permission from ref. 63 (Copyright 2010 Royal Society of Chemistry). | |
LSPR is sensitive to many parameters, such as size and shape of the nanoparticle,54 refractive index of the surrounding medium,55 ligands on the surface,56 temperature,57 and interparticle distance.58,59 Depending on the nanoparticle size and shape, the energy of excited plasmons is released by radiative (i.e. scattering of light) or non-radiative pathways.60 The non-radiative paths include the production of heat (if the nanoparticle is isolated) or electron transfer to adsorbed entities, such as in catalysis or semiconductor doping.
Strong far- and near-field effects are produced when the plasmon is excited. The former defines the extinction, scattering and absorption cross section of the nanoparticles. The latter affects the space near the surface, changing the interaction with nearby molecules or other particles. These near-field effects are used in several analytical techniques, such as surface enhanced Raman spectroscopy64 or fluorescence enhancement.65 The plasmon fields in nanoparticles are more sensitive to distance (i.e. they scale as 1/r3, where r is the distance from the metal surface) than in bulk metal (i.e. they scale as 1/r).66 This strong distance-dependency confines the electromagnetic field around the nanoparticle, becoming a very localized and high-density phenomenon (Fig. 1b). When two nanoparticles are placed in close proximity (i.e. distances below half nanoparticle diameter), their LSPR couples, changing the behavior of the plasmon and producing hot spots in the nanoparticle gap.67 The stronger electromagnetic field in the gap can be used in different ways, such as enhancing Raman scattering signals, which can achieve single-molecule sensitivity.68
2.2. Different classes of mNPs
Gold (Au), silver (Ag) and copper (Cu) are attractive candidates for optical technologies because their LSPR bands are in the visible region, in contrast to transition metals, whose plasmon bands lie in the UV region.69 Nevertheless, since Cu is easily oxidized,70 most mNP studies have been focused on Au and Ag.
The most common strategy in the synthesis of mNPs is salt-reduction. In this approach, a soluble metal salt is reduced by a reducing agent in the presence of a stabilizing component, which tailors the growth of the crystal in different morphologies, including nanospheres, nanorods, and nanostars (Fig. 1c), and prevents aggregation and precipitation.
Spherical gold nanoparticles (AuNPs) are the most commonly used mNPs and are frequently synthesized through the Turkevich method, where Au3+ salts are reduced to Au0 by citrate at 100 °C.71 Then, the metallic Au nucleates yielding small AuNPs (∼2–4 nm), which aggregate, rendering larger particles with diameters around 20 nm. Interestingly, no further stabilization is required since citrate acts as both reducing and capping agents. Further studies proved that the nanoparticle diameter could be adjusted from 20 to 60 nm by changing the molar ratio between Au3+ and citrate.72,73 Although the original Turkevich method dates back to 1951, the growth mechanism of the particles is still not fully understood, being the subject of several recent studies.74–76 Another popular synthesis of AuNPs is the Brust–Schiffrin method, where Au3+ cations (from HAuCl4) are reduced by NaBH4 in a two-phase (water/toluene) system, in the presence of alkanethiol. The nanoparticles grow in the toluene phase with diameters ranging from 1 to 3 nm.77 Finally, monodisperse AuNPs have been recently obtained with fast reaction times by taking advantage of microfluidic flow reactors.78,79
Gold nanorods (AuNRs) are frequently used for biological applications because their tunable longitudinal LSPR band can be easily shifted within the near-infrared biological window by changing the rod aspect ratio. In addition, AuNRs show stronger near-infrared absorption than other morphologies and scatter light at smaller sizes.54 AuNRs are synthesized through a seed-mediated method where hexadecyltrimethylammonium bromide is used as a directing agent.80–84
Gold nanostars (AuNSs) are composed of a spherical core and several protruding tips.85 The optical behavior of AuNSs is due to the combination of the two components, with a small plasmon band originating from the core and a big one from the tips. Interestingly, the position of the main plasmon band strongly depends on the aspect ratio, aperture angle and roundness of the tips but little on their number.86 AuNSs are synthesized through both seedless and seed-mediated methods31,85–87 and their main LSPRs induce strong field enhancements near the tip ends.88 This strong field has been used to enhance the SERS signal and decrease the detection levels down to the zeptomolar level.89
Spherical silver nanoparticles (AgNPs) are also grown through the Turkevich method,90–92 where citrate acts as both reducing agent and ligand.93 While the Turkevich protocol yields small and spherical AuNPs, this method produces more heterogeneous Ag particles with larger sizes (i.e. diameters around 60 nm). Recent advances in their synthesis include the use of microfluidic reactors for continuous growth of the particles.79,94 AgNPs have bluer LSPR bands than AuNPs and stronger field enhancements.95 Thus, since the publication of the early synthetic protocols, AgNPs were successfully used as a substrate for SERS.90,92
Metal nanoclusters (mNCs) are a subclass of mNPs that show luminescence properties. mNCs are made of a few to hundreds of atoms with particle sizes smaller than 2 nm.96,97 They present discrete electronic states as a result of strong quantum confinement effects98 (due to the sub-2 nm sizes) and strong interaction with ligands.99 Thus, mNCs present molecule-like behavior, such as HOMO–LUMO transitions,100 strong fluorescence101,102 and quantized charging.103 These properties make them ideal candidates for catalysis104–106 and optical technologies.107,108 Ligand selection in the growth of mNCs is essential to stabilize them and tune the final emission wavelength.109–114
2.3. Sensing principles for mNP assays
mNPs have been used in many sensing applications because of their ability to absorb and scatter light in the LSPR frequencies, which can be customized in the visible and near infrared regions of the spectrum.32 The sensing principles are versatile,115 including colorimetry based on particle aggregation, LSPR exploiting refractive index changes, fluorescence enhancement or quenching caused by nanoparticle energy transfer, and surface enhanced spectroscopy. The nanoparticles with LSPR found in the visible range are ideal probes for rapid on-site sensing application, including point-of-care diagnostics, since the changes of absorption can be detected by the naked eye or using inexpensive instruments.116 Nanoparticles that absorb or scatter light in the near IR region are more suitable to detect analytes in biological samples because their LSPR is located within the biological optical window, where sample matrix interferences are minimized.117
2.3.1. Colorimetric detection.
Colorimetric assays are based on the inter-particle plasmonic coupling between particles that renders a change in the solution color (i.e. from red to blue in the case of AuNPs) as the LSPR band red-shifts because of particle aggregation (Fig. 2).118 The color change can be induced by any analyte binding that directly or indirectly aggregates (or disaggregates) the nanoparticles, and thus serves as a measure of the presence of specific analytes.119–121
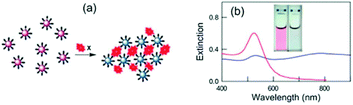 |
| Fig. 2 (a) Scheme of analyte-triggered AuNP aggregation and (b) the corresponding change in the nanoparticle optical properties. Reproduced with permission from ref. 132 (Copyright 2012 Royal Society of Chemistry). | |
Early aggregation-based designs date back to the 1990s and were initially focused on the detection of single-stranded oligonucleotides. Two sets of AuNPs were functionalized with two probe strands, and the hybridization between the probes and a target that contained complementary sequences to the two probes triggered the nanoparticle aggregation.122 Mirkin et al. were able to obtain sensitivities down to the femtomolar level with these designs.122 Functionalization of AuNPs with antibodies allowed the detection of other antigens such as proteins. This was the principle behind the pregnancy tests commercialized by Carter-Wallace in the early/mid-1990s.123
Since the early reports of Mirkin et al.,122 aggregation-based assays have become one of the most studied mNP-based designs used to characterize multiple analytes through cross-linking aggregation, such as on-particle DNA hybridization and/or antigen–antibody recognition.124,125 Later, non-crosslinking ones, which are based on electrostatic interactions, were also developed.126–129 These aggregation based assays present several key advantages over cross-linking and other homogeneous assays (such as fluorescence-based ones130,131), including label-free protocols, fast homogeneous solution interactions and allowing detection by the naked eye or using low cost instruments.32 Nevertheless, there are still a few issues that hinder the aggregation-based assay applications in a complex matrix, such as variable nanoparticle stability under changes of pH, temperature or ionic strength.32 The upcoming improvements on particle colloidal stability will define the future applications of mNP aggregation-based designs.
2.3.2. Refractive index sensing.
Refractive index sensors are based on the change of the dielectric constant of the metal vicinity by the analyte. Because this effect is distance dependent, the analyte has to be located in close proximity to the metal surface to effectively change the refractive index of the surroundings and shift the position of the plasmon band.133 The first designs of refractive index sensors were developed in the early 1980s and used Ag films as substrates.134,135 Nowadays, however, most common designs employ a thin Au surface as a plasmonic material and are known as surface plasmon resonance (SPR) sensors.136 In recent years, several alternatives using AuNPs,137 Au nanocrosses,138 Au nanoholes139 or Ag triangles140 have been developed in order to improve the technique sensitivity compared to Au films.
2.3.3. Surface enhanced Raman spectroscopy.
Raman scattering is the inelastic scattering of electromagnetic radiation by a target molecule.141 Since this phenomenon depends on the vibrational modes of the molecule, the spectrum is characteristic for each target.142 Nevertheless, the efficiency of inelastic scattering is low.143 The intensity of Raman scattering can be enhanced by placing the analyte near a metal surface, whose plasmon field is excited.143 This approach is called surface enhanced Raman spectroscopy (SERS). Isolated AuNPs have been reported to enhance the signal up to 103–104 and aggregated nanoparticles up to 1015,144 which allows single-molecule detection.145,146 The formation of hot spots between AuNP gaps, where their plasmon fields are coupled, is accounted for by more significant signal enhancements. Interestingly, theoretical studies indicate that field effects on hot spots can only enhance the SERS signal up to 1010.147 Therefore, an additional factor besides the electromagnetic field has to play a role in the SERS signal generation. Otto and Persson proposed a chemical factor, where the ballistic electrons in the metal interact with a strongly chemisorbed molecule.148,149 Because direct Raman measurement of analytes within complex samples may yield very complicated SERS spectra, active dyes known as Raman tags are used. Thus, the analytes are detected through indirect assays, where the Raman signals of the tags bound to the analytes are measured.150
2.3.4. Fluorescence-based detection.
Although fluorescence is one of the most well-established techniques in sensing and biomedical diagnostics,151 there is still a need for improving the sensitivity.152 There are several factors that limit fluorescence applications, such as photobleaching of fluorophores153 and autofluorescence of luminescent samples.154 Modifying the emission behavior of fluorophores by coupling them with a metal surface has been applied to overcome these limitations.152
Plasmon-resonance energy transfer (PRET) sensing.
When a donor (e.g. organic dye or quantum dot) is placed near a metal surface, a resonant energy transfer occurs in a similar way to Förster resonance energy transfer (FRET) between organic dyes or quantum dots.155 In addition to the energy transfer, the plasmon also affects the donor radiative lifetime.155 Both effects contribute to the strong fluorescence quenching, which can be described by the Gersten–Nitzan model.156 Even though FRET and PRET have similarities, they also present significant differences: PRET shows stronger quenching efficiency, due to the greater molar extinction coefficient of the plasmonic nanoparticles in comparison with organic dyes.60 FRET occurs in a distance range from 1 to 10 nm between the donor and acceptor, while nanoparticle-based PRET can double that distance.157 Photoluminescence and luminescence lifetime experiments have proved that the fluorescence quenching in PRET decreases at a metal–dye separation of 1/d4, while traditional FRET decreases at a donor–acceptor distance of 1/d6.158
A wide group of PRET sensors have been designed by combining mNPs with different kinds of donors, such as organic dyes,159,160 quantum dots,161–163 metal nanoclusters164,165 and conjugated polyelectrolytes.166,167 These have been applied for the sensing of metal ions,159,168 small molecules,169,170 proteins,171 and bacteria172 and for tracking molecular events, such as protein–DNA binding.166 Interestingly, PRET has been used in molecular beacons for DNA sensing.173 In this, the extremes of a self-complementary probe with a hairpin structure are functionalized with a donor and a mNP. The hairpin structure locates the donor near the nanoparticle, leading to strong fluorescence quenching. Upon probe hybridization with the target, the hairpin structure opens in a rod-like conformation and separates the donor and the acceptor (Fig. 3). The fluorescence is then restored and the intensity depends on the target concentration. This principle can also be used to monitor the cleavage of nucleotides by nucleases.174
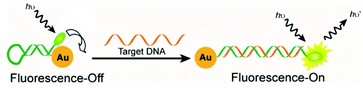 |
| Fig. 3 Scheme of a molecular beacon exploiting the PRET principle for DNA sensing. Reproduced with permission from ref. 27 (Copyright 2012 American Chemical Society). | |
Metal-enhanced fluorescence sensing.
Under some conditions, the electromagnetic coupling between a mNP and a fluorophore yields additional de-excitation pathways, which may enhance the fluorophore's excitation rates and/or the radiative decay rates that in turn result in fluorescence enhancement.175–177 Both plasmon-induced quenching and fluorescence enhancement compete and are distance dependent phenomena. At short distances, the energy transfer between the fluorophore and plasmon dominates.178 However, at specific distances farther from the metal surface, the energy transfer is highly reduced, while the electromagnetic field is still strong enough to enhance the fluorescence.179,180 A distance range between 10 and 20 nm from the metal surface has been reported to present the strongest fluorescence enhancement.178 Additionally, the plasmon band overlapping the fluorophore's emission and absorbance bands is also required for maximum enhancement.175 Regarding the role of the size and morphology of nanoparticles, the particles with a larger scattering cross-section present higher fluorescence enhancement.181,182 Even though metal-enhanced fluorescence sensors are not as common as PRET sensors, some homogeneous assays using this mechanism have been developed for the detection of biomolecules, such as DNA183 or proteins.184
2.3.5. Electrochemical detection.
The biocompatibility, electronic and catalytic properties of mNPs make them attractive substrates for electrochemical sensing.185 In these, the nanoparticles play a role in recognizing the analytes as well as generating the electronic signal for sensing.186 Although mNPs can be used in a wide range of electrochemical schemes, most of them can be classified into two groups: (1) nanoparticle-based enzyme electrodes, where mNPs enhance the electron transfer between the redox centers of proteins, which usually are insulated by the protein shell, and the electrodes187 and (2) nanoparticle-based bioaffinity electrodes, where mNPs amplify the transduction of the analyte–electrode interaction through a sandwich immunoassay or another labeling protocol.187 In addition to these two schemes, mNPs can also be used to immobilize biomolecules and to catalyze electrochemical reactions on the electrodes.188
3. Analysis of circulating cancer biomarkers with metal nanoparticles
3.1. Cell-free DNA and circulating tumor DNA
The discovery of DNA in blood plasma by Mandel et al. dates back to 1948.189 These extracellular DNA molecules are adsorbed on proteins (histones) and are predominantly around 180 base pair long.190 Although the exact mechanism that promotes the release of cell-free DNA (cfDNA) into the bloodstream is not fully understood,191 sequencing analysis indicates that cfDNA originates from apoptotic cells in healthy individuals.190 In patients with high cell turnover disorders, such as cancer, myocardial infraction and autoimmune conditions, higher levels of cfDNA with both apoptotic and necrotic origins are detected.193 A meta-analysis on the diagnostic accuracy of cfDNA showed similar results compared to conventional biomarkers and not enough differentiation capabilities to be used as a single cancer indicator.191 Multiple reports for different types of cancer, however, highlighted the cfDNA prognostic value, where the oligonucleotide concentration levels could be correlated to relapse probability and overall survival.191 Therefore, cfDNA has been highlighted as a promising biomarker to predict the patient outcome and forecast relapse probability rather than to diagnose.
Between 3% and 93% of all DNA in the bloodstream originate from tumor cells in cancer patients, depending on the stage and size of the tumor.193,194 This DNA with tumor origin is known as circulating tumor DNA (ctDNA) and contains characteristic genetic alterations identical to those from the tumors that can be targeted for non-invasive diagnosis. For instance, detectable levels of ctDNA were present in more than 75% of pancreatic, ovarian, colorectal, melanoma, breast, gastroesophageal, melanoma, head and neck cancer patients.195 Furthermore, ctDNA could be observed in patients without other measurable biomarkers, such as circulating tumor cells.195 Regarding the clinical value, KRAS mutation analysis of ctDNA showed a sensitivity and a specificity of 96 and 95%, respectively, in the diagnosis of thoracic malignancies.39 ctDNA has also shown greater correlation with changes in tumor burden compared to conventional markers, such as CA 15-3 in metastatic breast cancer patients.196
Hence, cfDNA (overall amount of DNA in the bloodstream) is a good biomarker for patient prognosis (i.e. forecast of disease outcome), while the levels of ctDNA have shown promising results in diagnosis (i.e. identification of type of disease and stage).
Both cfDNA and ctDNA are extracellular oligonucleotides but are not in a completely free form. Because blood is rich in DNases, i.e. enzymes that cleave the DNA backbone, only DNA adsorbed on proteins or complexed in lipid structures remains stable in the bloodstream.40 In order to extract the DNA from these complexes, several commercial kits based on silica membranes have been developed that yield the isolated oligonucleotides in buffer solution.44
There are two main strategies to analyze plasma DNA for cancer diagnosis, targeting either cfDNA or ctDNA.14 For cfDNA, whose total concentration (not the specific sequence) is correlated to the patient prognosis, techniques such as UV-Vis spectroscopy, fluorescent intercalating dyes and quantitative real-time polymerase chain reaction (qPCR) are used.14 Although these techniques are commercially available, they have limits in their accuracy of detection because the limit of detection is too close to the clinically relevant concentrations, or require complex and time consuming enzymatic amplification. For ctDNA, genetic mutations from the primary tumor are identified and quantified in the plasma DNA. Because there are frequently occurring mutations that drive tumor formation, such as point mutations and deletion mutations in KRAS or EGFR, the ctDNA analysis can target these genetic alterations through digital PCR or next generation sequencing techniques.197,198 Alternatively, untargeted methods including genome-wide detection of single nucleotide mutations, as well as mutations of larger genome sections, such as rearrangements and chromosomal copy-number, have been developed for general genetic analysis without focusing on specific known mutations.199,200
3.1.1. Quantification of cfDNA.
The majority of mNP-based sensing techniques target ctDNA with specific cancer-related sequences (more discussion in the next section). The demand towards cfDNA quantification, however, has led to the development of several mNP sensors and assays. The detection of cfDNA with clinical impact is challenging because of the wide physiological concentration range (from low ng ml−1 to high μg ml−1) for different cancer types. Hence, an effective sensor must be able to cover as wide as possible concentration ranges. Two strategies have been developed to address this challenge: (1) mNP-based inverse sensitivity response assays127 and (2) designs based on SERS.201,202 The first approach exploits the electrostatic interaction between hexadecyltrimethylammonium bromide coated AuNRs and DNA, which results in an unusual DNA concentration-dependent aggregation of the particles.127,203 The colorimetric assay provides inverse sensitivity, where the lower the analyte concentration, the higher the sensing response, and covers a wide range of cfDNA concentrations (from 20 ng ml−1 to 10 μg ml−1), which are associated with different types of cancer.127 The second strategy records the SERS spectra of serum cfDNA and identifies characteristic peaks.201 For instance, Ito et al. were able to distinguish with Ag nanoscale column chips individuals with gastric and colorectal cancer from healthy individuals and patients with benign disease by their distinct SERS peak heights.202
3.1.2. Detection of single nucleotide polymorphisms in ctDNA.
Single base variations or single nucleotide polymorphisms (SNPs) are the most common genetic mutations, and are associated with several health disorders including cancer.204 Because many studies have proved the correlation between these mutations and the clinico-pathological features of multiple types of tumors, single nucleotide polymorphisms are being explored as cancer biomarkers.205 In order to achieve the sensitivity and specificity required to distinguish one mutation within long ctDNA sequences, most of the assays combined the particle sensing principle with enzymatic amplification techniques. For instance, a common strategy has been amplifying the target sequence with PCR, and using the oligonucleotides to protect AuNPs against salt-induced aggregation.206–208 The interactions between DNA and the Au surface could be enhanced by using thiolated PCR products.209 More sophisticated methods combined PCR-amplified targets with additional enzymes, such as ligases, where only perfectly matched targets and probes were ligated inducing permanent particle aggregation (Fig. 4).210 The sensitivity of the ligase reaction assay could be improved down to 1 pM by attaching one probe to the Au surface and using SPR spectroscopy.192 Although PCR amplification is the most common protocol before the ligase detection reaction, target amplification could be eliminated for SNP detection by combining ligases and two primers, one containing a Raman tag and the other a AuNP acting as a Raman enhancer.211,212 Beyond PCR and ligase detection reaction, other enzymatic amplification reactions, including the ligase chain reaction,213,214 helicase-dependent isothermal amplification,215 and strand displacement amplification,216 have been coupled with mNP-based assays with limits of detection in the pM range. It is worth mentioning that a recent publication developed a colorimetric assay based on an enzyme-free click chemical ligation reaction capable of reaching a limit of detection as low as 50 zM.217Table 1 summarizes the current progress on SNP detection by mNP-based assays with enzymatic reactions.
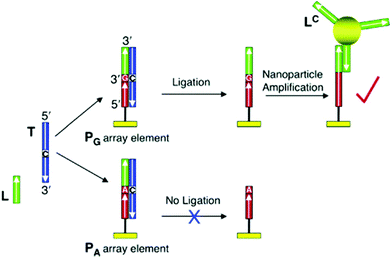 |
| Fig. 4 Scheme of SNP identification based on the combination of a ligase enzyme and nanoparticle-enhanced SPR. Reproduced with permission from ref. 192 (Copyright 2006 American Chemical Society). | |
Table 1 mNP-based assays with enzymatic reactions for SNP detection in ctDNA
Type of MNP |
Sensing principle |
Enzymatic reaction |
Gene of SNP |
Limit of detection (LOD) |
Ref. |
Unmodified AuNP |
Colorimetric |
PCR |
XXYLT1 |
5 fM |
206
|
Unmodified AuNP |
Colorimetric |
PCR |
EGFR |
— |
207
|
Unmodified AuNP |
Colorimetric |
PCR |
KCNE1 |
— |
208
|
AuNPs with thiolated PCR products |
Colorimetric |
PCR |
BRCA1 |
— |
209
|
ssDNA-AuNPs |
Colorimetric |
PCR + ligation reaction |
KRAS |
74 pM |
210
|
ssDNA-AuNPs |
SPR spectroscopy |
PCR + ligation reaction |
BRCA1 |
1 pM |
192
|
ssDNA-AuNPs |
SERS |
Ligase detection reaction |
KRAS |
20 pM |
211
|
ssDNA-AuNPs and ssDNA-AgNPs |
SERS |
Ligase detection reaction |
KRAS |
10 pM |
212
|
ssDNA-AuNPs |
Colorimetric |
Ligase chain reaction |
KRAS |
20 pg |
213
|
ssDNA-AuNPs |
Electrochemical |
Ligase chain reaction |
p53 |
0.9 pM |
214
|
ssDNA-AuNPs |
Colorimetric |
Helicase-dependent isothermal amplification |
KRAS |
20 pM |
215
|
ssDNA-AuNPs |
SERS |
Strand displacement amplification |
KRAS |
1.4 pM |
216
|
ssDNA-AuNPs |
Colorimetric |
Enzyme-free click chemical ligation reaction |
HAV |
50 zM |
217
|
3.1.3. Analysis of copy-number variations, chromosomal translocations, and DNA methylation in ctDNA.
Copy-number variations are types of structural variations, where sections of DNA (ranging from two base pairs up to entire genes) are amplified or deleted, and can contribute to tumorigenesis.218 Comparative genomic hybridization is a technique developed to survey DNA copy-number variations, where the DNA target and a reference sequence are labelled with dyes, and the fluorescence intensities along the DNA sequences are compared.219 Mirkin et al. were the first to adopt this approach for DNA detection in scanometric arrays, where the fluorescent dye labels were replaced with AuNPs.220 By introducing Ag deposition following the hybridization of AuNPs with the arrays containing the DNA analyte, the signal intensity could be increased by 105 fold. Based on this sensor design, Northbrook (IL, USA) has developed a mNP-based technology capable of genotyping DNA in 300 to 500 base fragments.221,222
Chromosomal translocations are another type of gene mutation associated with tumors, where parts of the chromosomes are rearranged.223 The standard techniques to screen these genetic abnormalities are PCR and agarose gel electrophoresis with ethidium bromide staining.224,225 Kalogianni et al. developed a AuNP-based colorimetric lateral flow assay for chromosomal translocations that showed 10-fold higher detectability than agarose gel electrophoresis without the need for special instrumentation.226 Using this lateral flow assay, they were able to screen seven chromosomal translocations associated with different types of leukemia.
Another promising biomarker for the early detection of malignancy is hypermethylation of ctDNA, since it is an early biochemical event of tumorigenesis.227 Colorimetric and SERS assays have been developed to simultaneously detect SNP and epigenetic methylations on ctDNA based on AuNPs and enzymatic reactions.228,229 Alternatively, Zhang et al. combined a hairpin DNA probe containing a quantum dot and a AuNP with the bisulfite reaction (i.e. a conventional technique used to identify DNA methylation) for the electrochemiluminescence detection of methylation levels and position in ctDNA.230
3.1.4. Current challenges in ctDNA sensing and diagnostics.
Although there are extensive studies highlighting the potential of ctDNA in cancer diagnostics, currently available mNP-based assays are mostly focused only on the detection of SNPs. Many tumors, however, lack recurring DNA mutations and require technologies capable of identifying other cancer signatures through genome-wide analyses.14 Only a small amount of mNP assays has been developed with genome-wide characterization capabilities and future efforts should be pursued towards that end. There is also a lack of assays that can detect deletion mutation in ctDNA, except one most recent study.231 Furthermore, a vast majority of the assays still rely on enzyme amplification reactions, which introduce biases due to polymerase and ligase error rates.14 Designs that use techniques with high sensitivity that do not require enzyme amplification, such as SERS, offer unique opportunities in this regard.
3.2. microRNA
microRNAs (miRNAs) are endogenous short RNA sequences (between 21 to 25 nucleotides) that regulate gene expression by translational repression.38,232 Over 1000 miRNAs have been identified in humans,233 which participate in a wide range of regulatory processes, such as cell proliferation and tissue growth,234 apoptosis,235 developmental timing236 and haematopoiesis.237 Hence, aberrant expression of miRNAs has been linked to the onset and progression of multiple pathologies, including cancer,238,239 central nervous system diseases,240 metabolic disorders241 and kidney242 and liver failures.243
Because abnormal miRNA expression profiles can be correlated to the type of tumor and stage, miRNAs are being studied for cancer diagnostics.244,245 For instance, underexpression of miR-26, miR-143 and miR-145, and overexpression of miR-21 have been observed in multiple cancers, such as colorectal and pituitary adenomas.246 Furthermore, miRNAs are very stable in blood because of their adsorption on proteins and encapsulation in membrane-bound vesicles, which provide high protection against blood RNases.247–249 Prior to analysis, however, miRNAs need to be isolated and enriched.250 Similar to normal total RNA isolation, commercially available products based on chaotropic salts and solid-phase extraction on silica columns are commonly used.
Northern blotting, microarrays and qPCR are the common methods to detect and quantify miRNAs through oligonucleotide hybridization.233 However, these methods present several challenges: (1) northern blotting requires large amounts of RNA as a starting material and usually is not sensitive enough to detect less abundant miRNAs,246 (2) microarrays show lower dynamic range and sensitivities than the other two methods,233 and (3) miRNA detection by qPCR employs short primers, which result in very low melting temperatures that hamper oligonucleotide replication and quantification.251
3.2.1. Detection of miRNAs.
Similar to DNA detection, miRNAs can be analyzed through mNP-based techniques. The fluorescence recovery of a dye-labelled probe upon hybridization with a target miRNA, such as PRET, is one of the most common systems. In these designs the hairpin-structured probe is assembled on AuNPs. Because the dye is in close proximity to the particle surface, the fluorescence is quenched. Upon hybridization with the miRNA target, the conformation of the probe changes and promotes the fluorescence recovery. This PRET design achieved a LOD of 0.01 pM for miR-122 extracted from liver cancer cells.252 In addition to fluorescent dyes, other emitters, such as metal nanoclusters, have also been used.253 The sensitivities of these conformation-switching based PRET assays have been further improved by coupling them to enzymatic reactions, where LODs as low as 200 and 2 aM have been reported for the dye (Fig. 5)254 and the metal nanocluster-based assays,255 respectively.
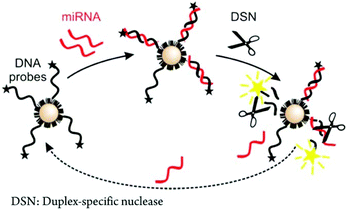 |
| Fig. 5 Scheme of a conformation-switching assay coupled with an enzymatic reaction for miRNA analysis. Reproduced with permission from ref. 254 (Copyright 2006 American Chemical Society). | |
Metal nanoclusters have also been used in other sensing designs beyond fluorescence-based ones. For instance, oligonucleotide encapsulated Ag nanoclusters were immobilized on Au electrodes and used as electrochemical probes for miRNA detection with a LOD of 67 fM.256 This assay was based on both sequence recognition for miRNA hybridization and AgNC catalyzing H2O2 reduction. More common electrochemical approaches involved sandwich-type assays between Au or carbon electrodes, miRNA target and functionalized AuNPs.257–260 The presence of AuNPs amplified the sensor voltammetric signals through a catalytic reaction, which achieved LODs between 10 and 60 fM depending on the AuNP functionalization and type of electrode. The particles can also be used to enhance the signal transduction between the electrode and different enzymes, such as phosphatases261 and peroxidases.262
Although not as common as the PRET techniques, SERS and colorimetric assays have also been used for miRNA sensing. Driskell et al. demonstrated that several miRNAs could be detected and distinguished through their unique SERS fingerprints on silver nanorod (AgNR) modified substrates.263 Based on this concept, a study reported distinct features in the serum miRNA spectra of healthy individuals and colorectal cancer patients. Using AgNP aggregates as substrates the authors could distinguish both populations with a sensitivity and a specificity of 89 and 96%, respectively.264 Regarding colorimetric assays, only a few studies have been published over the years. Because the detection levels required are very low, traditional AuNP aggregation-based designs were not sensitive enough. They required signal amplification techniques, such as isothermal exponential amplification reaction265 (i.e. an enzymatic reaction with DNA polymerase), strand displacement amplification,266 or Ag0 deposition enhancement.267 Alternatively, the peroxidase-like property of Cu nanoclusters has been used for the colorimetric detection of miRNAs, reaching a LOD of 0.6 pM.268
All the previous assays were able to detect a specific target sequence; however, they did not allow the profiling of samples with multiple miRNAs. To address this challenge, a few platforms based on high-density oligonucleotide microarrays have been developed. Fang et al. demonstrated a multiplexing design for the detection of hepatic miRNAs built on a SPR imaging array.269 This approach took advantage of the AuNP-enhanced SPR imaging measurements (through a sandwich assay) and polymerase amplification reaction to achieve a LOD of 10 fM. Furthermore, this method used locked nucleic acids (LNAs) as capturing probes, which show stronger binding affinity with miRNAs than conventional oligonucleotides and have been reported to increase the sensitivity of assays ten-fold.270 Mirkin et al. designed a scanometric microarray based on particle light scattering capable of characterizing body fluid samples.271 The assay took advantage of the deposition of Au0 onto the immobilized AuNPs to amplify the sensor response and decrease the LOD down to 1 fM.
3.2.2. Future prospects and challenges of miRNA sensors.
To address the challenges of quantification of multiple miRNAs for diagnosis and prognosis of tumours,246 future mNP-based assays must provide multiplex capabilities. Specifically, even after isolation, miRNAs are still mixed with different RNAs, such as siRNAs or other non-cancer related miRNAs. Therefore, high specificity is required to identify the targeted sequences. The concentrations of these cancer-related miRNAs are between atto- and nanomolar ranges, and the LOD of the assays should reach these low concentration levels. Taking into consideration the current status of the field, mNP-based assays should emphasize the following aspects: (1) High-density oligonucleotide microarrays have been the only mNP-based designs capable of profiling efficiently samples containing multiple miRNAs. Further studies should consider combining microarrays with the different sensing techniques available (e.g. SERS, SPR, light scattering or fluorescence, among others). (2) The analytical designs that used locked nucleic acid recognition or signal amplification enzymes showed enhanced specificity, which future studies could benefit from. (3) Due to the small amount of miRNAs in body fluids, techniques that offer low concentration or even single molecule detection, such as SERS, should be further developed.
3.3. Circulating tumor cells
Circulating tumor cells (CTCs) are shed from primary tumors during their early formation and growth, and released to the bloodstream.16 Scientific reports have highlighted that CTC levels can be used to predict the prognosis of metastatic patients.272,273 As an example, the Food and Drug Administration (FDA) has approved the CTC assays for metastatic castration-resistant prostate cancer (CRPC) evaluation, since these assays present the highest accuracy in the prediction of overall survival in CRPC patients.274 Furthermore, metastasis-initiating cells (MICs) have been identified within CTC populations of breast275 and renal cancers.276 These MICs have been later used in xenograft studies to confirm that they give rise to metastasis in other organs. Therefore, the presence and levels of CTCs can provide key information regarding the patient condition, such as cancer aggressiveness, metastatic burden and relapse.277
The analysis and quantification of CTCs are challenging due to their low concentration in body fluids, i.e. a few CTCs are found in a background of millions of blood cells in blood.16 Therefore, most CTC samples are enriched before or during their quantification through biological or physical-based techniques. Biological techniques initially capture the CTCs through biological interactions, such as antigen–antibody interaction, and then isolate them. Magnetic separation,278–280 where the antibody is coupled to a magnetic bead, or microfluidic devices are the main techniques used for cell separation.281–283 Sub-populations of CTCs, such as epithelial cell adhesion molecule (EpCAM)-positive cancer cells, have also been enriched by electrical responsive conducting polymers and anti-EpCAM antibodies.284,285 Physical-based techniques are label-free and exploit physical properties through size-based filtration or centrifugation.286,287
After the cells have been captured and separated, they need to be identified. CTCs are the result of heterogeneous processes, which can manifest in different ways and vary from cell to cell.288–290 Therefore, CTC distinction from normal cells is challenging. Conventional sensing techniques can be classified based on whether they target the cell phenotype or genotype.
In phenotype detection, CTCs are detected based on their physical properties, such as buoyant density291–293 and increased size,294–296 or their distinctive cell surface antigens. EpCAM is the most common target, since this protein is expressed in all cells of epithelial origin, but not in blood cells.43 The FDA-approved CellSearch system (Veridex, LLC, Raritan, NJ, USA) is a standardized technology that captures CTCs with anti-EpCAM antibody-loaded ferrofluids and visualizes them through immunostaining.297 Nevertheless, this technique presents low sensitivity, since only a small percentage of metastatic cancer patients score positive.298
In genotype detection, CTCs are collected and enriched and their DNA is subsequently extracted and analysed.299 Nevertheless, it has been reported that ctDNA presents greater mutation detectability over DNA extracted from CTCs.39 Thus, the genotype analysis of CTCs is not a common protocol, and ctDNA analysis is preferred.40
Although CTCs have been the subject of extensive research, both their low concentration in body fluids and their high heterogeneity are still limiting their clinical applications. Interestingly, mNPs present unique features that can overcome these limitations, such as large surface-to-volume ratios that allow the attachment of multiple recognition elements for enhanced specificity in CTC recognition.
3.3.1. Detection of the CTC phenotype.
Most mNP-based sensing techniques target the CTC phenotype, where mNPs are functionalized with antibodies or aptamers targeting specific surface analytes. Recent advances in the systematic evolution of ligands by exponential enrichment (SELEX), i.e. a synthetic technique that generates aptamers by an in vitro selection process, have extended the range of targets to complex mixtures of proteins in living cell surfaces.300 Thus, new aptamers have been discovered against previously unidentifiable CTC-surface analytes.
AuNPs with different morphologies have been used for SERS-based sensing of CTCs. These nanoparticles are dispersed in solution301 or adsorbed on substrates, such as single-walled carbon nanotubes (SWCNTs).302 When placed on SWCNTs, the AuNPs are able to produce hot spots, decreasing the LOD down to 10 cells per ml due to field-enhancement effects.302 Furthermore, the SWCNT–AuNP hybrids have been used as dual systems for sensing and photothermal therapy applications.302–304 Nevertheless, these assays lack the separation steps and they have been solely applied to samples in buffer.
So far, several SERS-based bioassays compatible with a complex medium have been published. Sha et al. were able to detect as low as 50 SKBR3 cancer cells per ml in whole blood by combining functionalized AuNPs with magnetic beads.305 Lung cancer cells have also been detected in whole blood (LOD of 34 cells per ml) by integrating AuNPs and a low cost CTC-capture substrate made of a nitrocellulose membrane.306 Alternatively, AuNPs can also be functionalized with proteins or biomolecules, yielding probes that bind to the cancer cell surface through surface receptor recognition. As an example, carcinoma cells overexpress the cell-surface epidermal growth factor receptor (EGFR). Thus, AuNPs functionalized with the epidermal growth factor have been employed in a SERS-based assay to detect squamous cell carcinoma in the peripheral blood of 19 patients.307 Folic acid (FA) has also been used in the detection of CTCs through SERS assays, since several cancer types (e.g. ovarian, brain, kidney, breast, lung, cervical and nasopharyngeal) overexpress folate receptor α. FA-functionalized AuNPs have been reported to detect as low as 5 CTCs per ml in rabbit blood.308 Both AuNPs and magnetic nanoparticles functionalized with FA have also been combined for magnetic enrichment and SERS-based detection of HeLa cells in rat blood.309 Recently, this protocol has been applied for the detection of CTCs from cervical cancer in the blood of two first-stage clinical patients.310
mNP-based colorimetric assays have also been developed to detect and quantify CTCs. These assays are based on the analyte-triggered aggregation or dispersion of mNPs and the corresponding changes in mNP optical properties. AuNPs were conjugated with different aptamers that targeted proteins overexpressed on cancer cell membranes, such as nucleolin.311,312 If AuNPs contained aptamers and antibodies (double-targeting), the sensitivity could be increased to 100 CTCs per ml in buffer.313 In order to expand the colorimetric assay to whole blood samples, a lateral flow device was developed that allowed the detection of 4000 CTCs by the naked eye and 800 CTCs using a spectrometer in a 15 μL sample. Alternatively, a chemiluminescent assay was designed, where aptamer-functionalized AuNPs catalyzed the reaction between luminol and H2O2. This assay could detect 30 cells in 3 μL of blood sample.314 Real-time detection of CTCs in vivo has been achieved by photoacoustic flow cytometry.315 Magnetic nanoparticles were used for separating cells from blood and FA-targeting AuNPs on CNTs as photoacoustic contrast agents.
3.3.2. Detection of the CTC genotype.
Mirkin et al. have developed Au nanoFlares for the detection of messenger RNA (mRNA) for a target gene (Fig. 6).316 The nanoFlares are spherical AuNPs chemically functionalized with ssDNA, which is complementary to the target mRNA. The ssDNA is pre-hybridized with a shorter oligonucleotide labelled with a fluorescent dye, which is quenched by the AuNP. When the mRNA binds to the recognition sequence, the labelled oligonucleotide is released and the fluorescence increases. Au nanoFlares interact with scavenger receptors in the cell membrane, triggering their cellular uptake.317 NanoFlare technology has been used for the isolation and detection of live CTCs from whole blood.318 Furthermore, these AuNPs have also been applied to the spatiotemporal location of a target mRNA in living cells,319 and attempted to be used for the analysis of different transcripts in several cancer cell lines.352
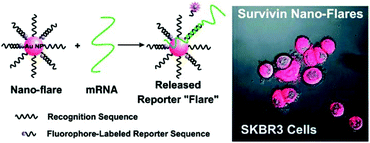 |
| Fig. 6 Au nanoFlare detection of mRNA expressed in CTCs. Reproduced with permission from ref. 316 (Copyright 2007 American Chemical Society). | |
3.3.3. Cancer stem cell detection and quantification.
Although CTCs are a heterogeneous group of cells released by the tumors into the bloodstream, there is a specific type that has received increasing attention in recent years. Cancer stem cells (CSCs) are a sub-category of CTCs that share similarities to normal stem cell capabilities, including proliferation and differentiation.320 Hence, CSCs have been identified as a precursor of metastases and relapses in cancer patients. Antibody functionalized mNPs have been used in the detection of the CSC phenotype through both SERS and colorimetric principles in PBS buffer.321,322
3.4. Exosomes
Exosomes are vesicles released by cells during the fusion of multivesicular bodies with plasma membranes.323 The vesicles have diameters between 50 and 90 nm and participate in the regulation of cell waste disposal, intercellular communication and coagulation.324 Exosomes offer unique opportunities as circulating cancer biomarkers because they contain proteins, DNA and RNA,248,325 and can be found in multiple biological fluids, such as saliva, blood, serum, urine and breast milk.326–328
In order to obtain accurate biological information, exosomes have to be concentrated and isolated from components with similar sizes also found in biological fluids, such as protein complexes, calcium-phosphate precipitates and other lipid vesicles.329–332 The common protocols for exosome concentration include multi-step ultracentrifugation with speeds up to 2 × 105g.333 The recovery yields are low (5 to 25%) and the samples are rich in contaminants, such as protein aggregates, which can lead to wrong biological diagnosis.334 Furthermore, the whole concentration process is time consuming (4 to 6 h). In order to improve the detection sensitivity and decrease the number of false-negative results, isolation protocols consisting of microfluidics335,336 and/or immunoaffinity separations are performed.337,338
The detection of isolated and concentrated exosomes is still very challenging since these vesicles are very small and highly heterogeneous.339 Similar to CTCs, traditional detection methods can be classified based on whether they target the phenotype or the genotype.
For detection based on the phenotype, flow cytometry is the gold standard technique to characterize exosomes and other vesicles in clinical samples.340 A large percentage of the vesicles, however, are smaller than the detection limit of conventional flow cytometers, and modifications, such as labelling with membrane intercalating dyes, are necessary to measure most of the sample contents.341
Alternative techniques based on light scattering, such as dynamic light scattering and nanoparticle tracking analysis, can measure the absolute and relative size distributions of exosomes but cannot distinguish them from other particles with similar sizes.339 Fluorescence-based techniques, including stimulated emission depletion microscopy and fluorescence correlation spectroscopy, can provide both size distributions and biochemical information if antibody labelling is used.339
For detection based on the genotype, the DNA and RNA contained in exosomes are isolated and analyzed similarly to ctDNA and miRNA46 (please refer to sections 3.1 and 3.2 of this review).
3.4.1. Detection of exosomes.
The strong near-field enhancements of mNPs have been used to study the biological composition of exosomes through SERS assays. For instance, AgNPs adsorbed on silicon micropillars342 and AuNP aggregates343 have been used as hot spots in SERS substrates to distinguish between exosomes released by healthy and tumor cells based on the vesicle membrane composition. The magnetic properties of magnetic nanobeads covered by a Au shell have been exploited for both exosome separation and particle aggregation (to generate hot spots) during SERS detection.344 If the exosomes were dried prior to analysis, additional SERS peaks developed as a consequence of the rupture of the vesicle membranes, exposing the internal content of the exosome.345 Alternatively, different types of assays have targeted surface antigens to quantify and characterize exosomes, since surface proteins are fundamental for the vesicle biological functions and can provide tumor fingerprints. For instance, the electro-oxidation of AgNPs and copper (Cu) NPs by Au electrodes was used to detect exosomes through an electrochemical assay.346 The NPs and electrodes were functionalized with anti-EpCAM and anti-PSMA aptamers for multiplex detection and a LOD of 50 exosomes per sensing chip was achieved.
mNPs are not only being used as sensing materials, but are also used for signal amplification of other sensing technologies. For example, AuNPs were coupled with a SPR Au film to detect multiple myeloma-originated exosomes348 through a structural analogue of heparin sulfate that mediates in exosome endocytosis. In a sandwich arrangement, AuNPs bind to the exosomes, which then are captured on the SPR chip. The addition of AuNPs as signal amplifiers improved the LOD down to tens of pM. A recent study by Im et al. demonstrated that if the SPR film was replaced with a periodic Au nanohole array film functionalized with antibodies, and then combined with AuNPs as signal amplification elements, 3000 exosomes derived from ovarian cancer cells (LOD of 670 aM) could be detected (Fig. 7).347 In this design, the use of 10 nm AuNPs or 50 nm AuNSs improved the signal by 20 and 300%, respectively. The nanohole-based sensor benefited from a significant advantage over traditional SPR platforms: the small array size necessary (below 10 mm2) to achieve large optical transmission allowed a high array density (more than 106 detection sites per cm2). In addition to the detection and quantification of vesicles, antibody-functionalized AuNPs have been employed to label exosomes for morphology characterization through electron microscopy.349–351
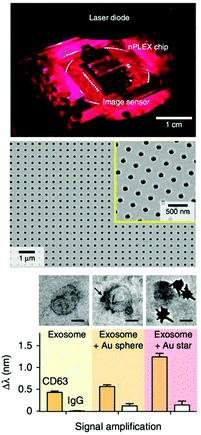 |
| Fig. 7 SPR design based on Au nanohole arrays for CTC analysis (top image). SEM image of a Au nanohole array (central image). Effect of nanoparticle labelling on the SPR wavelength (bottom image). Adapted with permission from ref. 347 (Copyright 2014 Springer Nature). | |
3.4.2. Current challenges on exosome-based diagnostics.
Although promising results have been achieved in the field of cancer diagnostics using tumor-derived exosomes,45 there is still a lack of understanding of their biology. Over the last decade, multiple studies revealed that these vesicles participate in most tumor-promoting pathways, such as angiogenesis, cancer stemness and hypoxia-induced epithelial–mesenchymal transition.17 Nevertheless, these new discoveries seem to have only scratched the surface as new work is constantly being published. Further efforts need to be pursued towards establishing and validating the specificity and sensitivity requirements of exosome-based analysis for multiple populations and types of cancers. Only after these requirements have been clearly identified, the clinical utility of exosomes in comparison with other circulating biomarkers will be established.
4. Summary and outlook
Circulating cancer biomarker analysis shows promise in the early diagnosis and prognosis of cancer. The clinical application of these biomarkers, nevertheless, is still limited by their low concentrations in body fluids and heterogeneity. Analytical tools capable of achieving high sensitivities and low LOD (below nM in many cases) are necessary for taking advantage of all the information that circulating cancer biomarkers provide regarding the patient condition.
In this comprehensive review, we summarize the current progress on mNP-based analytical methods for the sensing of circulating cancer biomarkers, including cfDNA, ctDNA, miRNAs, CTCs and exosomes. The sensing principles include those where mNPs are used as sensing/transducing materials, such as aggregation induced colorimetric assays, PRET, SERS, and refractive index sensing, as well as those where mNPs are used as signal amplification materials, for example, SPR and electrochemical sensors. We evaluate the advantages and limitations of each analytical design based on the type of analyte detected, sensing principle, sensitivity and sample preparation. In order to understand the possible impact of mNPs on cancer diagnosis, we analyze the deficiencies of the standard techniques used to detect cancer biomarkers, such as the need for low LOD, ability to work with a complex sample matrix and multiplex detection, and how mNP-based assays can overcome these. Finally, we also revise the current challenges and promising directions of sensing with mNPs. By systematically evaluating the nano- and bioassays, we believe our review will help to inform the current status of the field and to identify future research opportunities.
Conflicts of interest
There are no conflicts to declare.
Acknowledgements
N. T. K. T. thanks the EPSRC (EP/M018016/1 and EP/M015157/1) for funding and R. M. P. thanks UCL and the ARAP program of A*STAR for the scholarship and facilities.
Notes and references
- M. Gerlinger, A. J. Rowan, S. Horswell, J. Larkin, D. Endesfelder, E. Gronroos, P. Martinez, N. Matthews, A. Stewart, P. Tarpey, I. Varela, B. Phillimore, S. Begum, N. Q. McDonald, A. Butler, D. Jones, K. Raine, C. Latimer, C. R. Santos, M. Nohadani, A. C. Eklund, B. Spencer-Dene, G. Clark, L. Pickering, G. Stamp, M. Gore, Z. Szallasi, J. Downward, P. A. Futreal and C. Swanton, N. Engl. J. Med., 2012, 366, 883–892 CrossRef CAS.
- L. A. Diaz and A. Bardelli, J. Clin. Oncol., 2014, 32, 579–586 CrossRef PubMed.
- E. G. Robertson and G. Baxter, Clin. Radiol., 2011, 66, 1007–1014 CrossRef CAS PubMed.
- M. J. Overman, J. Modak, S. Kopetz, R. Murthy, J. C. Yao, M. E. Hicks, J. L. Abbruzzese and A. L. Tam, J. Clin. Oncol., 2013, 31, 17–22 CrossRef PubMed.
- M. C. Chamberlain, Curr. Treat. Options Neurol., 2004, 6, 297–305 CrossRef PubMed.
- L. A. Liotta, M. Ferrari and E. Petricoin, Nature, 2003, 425, 905 CrossRef CAS PubMed.
- E. F. Petricoin, C. Belluco, R. P. Araujo and L. A. Liotta, Nat. Rev. Cancer, 2006, 6, 961–967 CrossRef CAS PubMed.
- J. Nilsson, J. Skog, A. Nordstrand, V. Baranov, L. Mincheva-Nilsson, X. O. Breakefield and A. Widmark, Br. J. Cancer, 2009, 100, 1603–1607 CrossRef CAS PubMed.
- N. Raskin, N. A. Babu and K. M. K. Masthan, Biomed. Pharmacol. J., 2015, 8SE, 701–704 CrossRef.
- M. J. Glantz, B. F. Cole, L. K. Glantz, J. Cobb, P. Mills, A. Lekos, B. C. Walters and L. D. Recht, Cancer, 1998, 82, 733–739 CrossRef CAS.
- I. M. Thompson, D. K. Pauler, P. J. Goodman, C. M. Tangen, M. S. Lucia, H. L. Parnes, L. M. Minasian, L. G. Ford, S. M. Lippman, E. D. Crawford, J. J. Crowley and C. A. Coltman, N. Engl. J. Med., 2004, 350, 2239–2246 CrossRef CAS PubMed.
- J. R. Prensner, M. A. Rubin, J. T. Wei and A. M. Chinnaiyan, Sci. Transl. Med., 2012, 4, 127rv3 Search PubMed.
- E. Björnsson, A. Kilander and R. Olsson, Liver, 1999, 19, 501–508 CrossRef PubMed.
- E. Heitzer, P. Ulz and J. B. Geigl, Clin. Chem., 2015, 61, 112–123 CrossRef CAS PubMed.
- P. S. Mitchell, R. K. Parkin, E. M. Kroh, B. R. Fritz, S. K. Wyman, E. L. Pogosova-Agadjanyan, A. Peterson, J. Noteboom, K. C. O'Briant, A. Allen, D. W. Lin, N. Urban, C. W. Drescher, B. S. Knudsen, D. L. Stirewalt, R. Gentleman, R. L. Vessella, P. S. Nelson, D. B. Martin and M. Tewari, Proc. Natl. Acad. Sci. U. S. A., 2008, 105, 10513–10518 CrossRef CAS PubMed.
- C. Alix-Panabières and K. Pantel, Clin. Chem., 2013, 59, 110–118 CrossRef PubMed.
- A. S. Azmi, B. Bao and F. H. Sarkar, Cancer Metastasis Rev., 2013, 32, 623–642 CrossRef CAS PubMed.
- Y. M. Lo, J. Zhang, T. N. Leung, T. K. Lau, A. M. Chang and N. M. Hjelm, Am. J. Hum. Genet., 1999, 64, 218–224 CrossRef CAS PubMed.
- S. P. Pricker, Gold Bull., 1996, 29, 53–60 CrossRef.
- J. W. Alexander, Surg. Infect. (Larchmt), 2009, 10, 289–292 CrossRef.
- A. J. Lewis and D. T. Walz, Prog. Med. Chem., 1982, 19, 1–58 CrossRef CAS.
- S. Chernousova and M. Epple, Angew. Chem., Int. Ed., 2013, 52, 1636–1653 CrossRef CAS PubMed.
- R. Mukerji, G. Mukerji and M. McGurk, Br. J. Oral Maxillofac. Surg., 2006, 44, 222–228 CrossRef CAS PubMed.
- M. C. Daniel and D. Astruc, Chem. Rev., 2004, 104, 293–346 CrossRef CAS.
- M. Brust and C. J. Kiely, Colloids Surf., A, 2002, 202, 175–186 CrossRef CAS.
- S. Mourdikoudis, R. M. Pallares and N. T. K. Thanh, Nanoscale, 2018, 10, 12871–12934 RSC.
- K. Saha, S. S. Agasti, C. Kim, X. Li and V. M. Rotello, Chem. Rev., 2012, 112, 2739–2779 CrossRef CAS PubMed.
- N. Li, X. Su and Y. Lu, Analyst, 2015, 140, 2916–2943 RSC.
- L. Sutarlie, S. Y. Ow and X. Su, Biotechnol. J., 2017, 12, 1500459 Search PubMed.
- R. M. Pallares, P. Choo, L. E. Cole, C. A. Mirkin, A. Lee and T. W. Odom, Bioconjugate Chem., 2019, 30, 2032–2037 CrossRef CAS PubMed.
- J. Yue, R. M. Pallares, L. E. Cole, E. E. Coughlin, C. A. Mirkin, A. Lee and T. W. Odom, ACS Appl. Mater. Interfaces, 2018, 10, 21920–21926 CrossRef CAS.
- H. Jans and Q. Huo, Chem. Soc. Rev., 2012, 41, 2849 RSC.
- Q. Shou, C. Guo, L. Yang, L. Jia, C. Liu and H. Liu, J. Colloid Interface Sci., 2011, 363, 481–489 CrossRef CAS PubMed.
- Y.-J. Kang, J.-W. Oh, Y.-R. Kim, J. S. Kim and H. Kim, Chem. Commun., 2010, 46, 5665–5667 RSC.
- Y. Li, K. Burnham, J. Dykes and N. Chopra, MRS Commun., 2018, 8, 79–87 CrossRef CAS.
- Y. Liu, Y. Tang, N. N. Barashkov, I. S. Irgibaeva, J. W. Y. Lam, R. Hu, D. Birimzhanova, Y. Yu and B. Z. Tang, J. Am. Chem. Soc., 2010, 132, 13951–13953 CrossRef CAS PubMed.
- Y. Uludag and I. E. Tothill, Anal. Chem., 2012, 84, 5898–5904 CrossRef CAS PubMed.
- D. P. Bartel, Cell, 2004, 116, 281–297 CrossRef CAS.
- M. B. Freidin, D. V. Freydina, M. Leung, A. M. Fernandez, A. G. Nicholson and E. Lim, Clin. Chem., 2015, 61, 1299–1304 CrossRef CAS PubMed.
- D. L. Peters and P. J. Pretorius, Clin. Chim. Acta, 2011, 412, 806–811 CrossRef CAS.
- V. Swarup and M. R. Rajeswari, FEBS Lett., 2007, 581, 795–799 CrossRef CAS PubMed.
- E. Crowley, F. Di Nicolantonio, F. Loupakis and A. Bardelli, Nat. Rev. Clin. Oncol., 2013, 10, 472–484 CrossRef CAS.
- M. Yu, S. Stott, M. Toner, S. Maheswaran and D. A. Haber, J. Cell Biol., 2011, 192, 373–382 CrossRef CAS.
- J. Jen, L. Wu and D. Sidransky, Ann. N. Y. Acad. Sci., 2010, 906, 8–12 CrossRef.
- J. Ko, E. Carpenter and D. Issadore, Analyst, 2016, 141, 450–460 RSC.
- E. Zeringer, World J. Methodol., 2013, 3, 11 CrossRef PubMed.
- X. Huang, R. O'Connor and E. A. Kwizera, Nanotheranostics, 2017, 1, 80–102 CrossRef PubMed.
- H. J. Yoon, M. Kozminsky and S. Nagrath, ACS Nano, 2014, 8, 1995–2017 CrossRef CAS PubMed.
- V. Myroshnychenko, J. Rodríguez-Fernández, I. Pastoriza-Santos, A. M. Funston, C. Novo, P. Mulvaney, L. M. Liz-Marzán and F. J. García de Abajo, Chem. Soc. Rev., 2008, 37, 1792–1805 RSC.
- P. K. Jain, X. Huang, I. H. El-Sayed and M. A. El-Sayed, Acc. Chem. Res., 2008, 41, 1578–1586 CrossRef CAS PubMed.
- G. Mie, Ann. Phys., 1908, 330, 377–445 CrossRef.
- L. M. Liz-Marzán, Langmuir, 2006, 22, 32–41 CrossRef PubMed.
- J. Pérez-Juste, I. Pastoriza-Santos, L. M. Liz-Marzán and P. Mulvaney, Coord. Chem. Rev., 2005, 249, 1870–1901 CrossRef.
- P. K. Jain, K. S. Lee, I. H. El-Sayed and M. A. El-Sayed, J. Phys. Chem. B, 2006, 110, 7238–7248 CrossRef CAS PubMed.
- H. Chen, X. Kou, Z. Yang, W. Ni and J. Wang, Langmuir, 2008, 24, 5233–5237 CrossRef CAS.
- X. Liu, M. Atwater, J. Wang and Q. Huo, Colloids Surf., B, 2007, 58, 3–7 CrossRef CAS PubMed.
- S. Link and M. El-Sayed, J. Phys. Chem. B, 1999, 103, 4212–4217 CrossRef CAS.
- N. J. Halas, S. Lal, W.-S. Chang, S. Link and P. Nordlander, Chem. Rev., 2011, 111, 3913–3961 CrossRef CAS PubMed.
- K. H. Su, Q. H. Wei, X. Zhang, J. J. Mock, D. R. Smith and S. Schultz, Nano Lett., 2003, 3, 1087–1090 CrossRef CAS.
- L. A. Austin, B. Kang and M. A. El-Sayed, Nano Today, 2015, 10, 542–558 CrossRef CAS.
- M. L. Juan, M. Righini and R. Quidant, Nat. Photonics, 2011, 5, 349–356 CrossRef CAS.
- T. Chung, S. Y. Lee, E. Y. Song, H. Chun and B. Lee, Sensors, 2011, 11, 10907–10929 CrossRef CAS PubMed.
- N. N. M. Adnan, Y. Y. Cheng, N. M. N. Ong, T. T. Kamaruddin, E. Rozlan, T. W. Schmidt, H. T. T. Duong and C. Boyer, Polym. Chem., 2016, 7, 2888–2903 RSC.
- R. A. Alvarez-Puebla and L. M. Liz-Marzán, Small, 2010, 6, 604–610 CrossRef CAS.
- C. C. Huang, Z. Yang, K. H. Lee and H. T. Chang, Angew. Chem., Int. Ed., 2007, 46, 6824–6828 CrossRef CAS PubMed.
-
S. A. Maier, Plasmonics: Fundamentals and
applications, Springer Science & Business Media, 2007 Search PubMed.
- P. K. Jain and M. A. El-Sayed, Chem. Phys. Lett., 2010, 487, 153–164 CrossRef CAS.
-
G. C. Schatz, M. A. Young and R. P. Duyne, in Electromagnetic Mechanism of SERS BT – Surface-Enhanced Raman Scattering: Physics and Applications, ed. K. Kneipp, M. Moskovits and H. Kneipp, Springer Berlin Heidelberg, Berlin, Heidelberg, 2006, pp. 19–45 Search PubMed.
- J. A. Creighton and D. G. Eadon, J. Chem. Soc., Faraday Trans., 1991, 87, 3881–3891 RSC.
- P. Kanninen, C. Johans, J. Merta and K. Kontturi, J. Colloid Interface Sci., 2008, 318, 88–95 CrossRef CAS PubMed.
- J. Turkevich, P. C. Stevenson and J. Hillier, Discuss. Faraday Soc., 1951, 11, 55–75 RSC.
- G. Frens, Nat. Phys. Sci., 1973, 241, 20–22 CrossRef CAS.
- J. Kimling, M. Maier, B. Okenve, V. Kotaidis, H. Ballot and A. Plech, J. Phys. Chem. B, 2006, 110, 15700–15707 CrossRef CAS PubMed.
- J. Polte, T. T. Ahner, F. Delissen, S. Sokolov, F. Emmerling, A. F. Thünemann and R. Kraehnert, J. Am. Chem. Soc., 2010, 132, 1296–1301 CrossRef CAS PubMed.
- M. Wuithschick, A. Birnbaum, S. Witte, M. Sztucki, U. Vainio, N. Pinna, K. Rademann, F. Emmerling, R. Kraehnert and J. Polte, ACS Nano, 2015, 9, 7052–7071 CrossRef CAS PubMed.
- M. O. Besenhard, R. Baber, A. P. LaGrow, L. Mazzei, N. T. K. Thanh and A. Gavriilidis, CrystEngComm, 2018, 20, 7082–7093 RSC.
- M. Brust, M. Walker, D. Bethell, D. J. Schiffrin and R. Whyman, J. Chem. Soc., Chem. Commun., 1994, 801 RSC.
- H. Huang, H. du Toit, S. Ben Jaber, G. Wu, L. Panariello, N. T. K. Thanh, I. P. Parkin and A. Gavriilidis, React. Chem. Eng., 2019, 4, 884–890 RSC.
- R. Baber, L. Mazzei, N. T. K. Thanh and A. Gavriilidis, Nanoscale, 2017, 9, 14149–14161 RSC.
- N. R. Jana, L. Gearheart and C. J. Murphy, Adv. Mater., 2001, 13, 1389–1393 CrossRef CAS.
- B. Nikoobakht and M. A. El-Sayed, Chem. Mater., 2003, 15, 1957–1962 CrossRef CAS.
- X. Ye, L. Jin, H. Caglayan, J. Chen, G. Xing, C. Zheng, V. Doan-Nguyen, Y. Kang, N. Engheta, C. R. Kagan and C. B. Murray, ACS Nano, 2012, 6, 2804–2817 CrossRef CAS PubMed.
- R. M. Pallares, X. Su, S. H. Lim and N. T. K. Thanh, J. Mater. Chem. C, 2016, 4, 53–61 RSC.
- R. M. Pallares, Y. Wang, S. H. Lim, N. T. K. Thanh and X. Su, Nanomedicine, 2016, 11, 2845–2860 CAS.
- C. L. Nehl, H. Liao and J. H. Hafner, Nano Lett., 2006, 6, 683–688 CrossRef CAS PubMed.
- P. Senthil Kumar, I. Pastoriza-Santos, B. Rodríguez-González, F. Javier, G. de Abajo and L. M. Liz-Marzán, Nanotechnology, 2008, 19, 15606 CrossRef PubMed.
- R. M. Pallares, T. Stilson, P. Choo, J. Hu and T. W. Odom, ACS Appl. Nano Mater., 2019, 2, 5266–5271 CrossRef.
- F. Hao, C. L. Nehl, J. H. Hafner and P. Nordlander, Nano Lett., 2007, 7, 729–732 CrossRef CAS PubMed.
- L. Rodríguez-Lorenzo, R. A. Álvarez-Puebla, I. Pastoriza-Santos, S. Mazzucco, O. Stéphan, M. Kociak, L. M. Liz-Marzán and F. J. G. De Abajo, J. Am. Chem. Soc., 2009, 131, 4616–4618 CrossRef PubMed.
- P. C. Lee and D. Meisel, J. Phys. Chem., 1982, 86, 3391–3395 CrossRef CAS.
- P. V. Kamat, M. Flumiani and G. V. Hartland, J. Phys. Chem. B, 1998, 102, 3123–3128 CrossRef CAS.
- R. S. Sheng, L. Zhu and M. D. Morris, Anal. Chem., 1986, 58, 1116–1119 CrossRef CAS.
- A. Henglein and M. Giersig, J. Phys. Chem. B, 1999, 103, 9533–9539 CrossRef CAS.
- R. Baber, L. Mazzei, N. T. K. Thanh and A. Gavriilidis, J. Flow Chem., 2016, 6, 268–278 CrossRef CAS.
- K. S. Lee and M. A. El-Sayed, J. Phys. Chem. B, 2006, 110, 7238–7248 CrossRef PubMed.
- R. Jin, Nanoscale, 2010, 2, 343–362 RSC.
- Y. Lu and W. Chen, Chem. Soc. Rev., 2012, 41, 3594–3623 RSC.
- O. Varnavski, G. Ramakrishna, J. Kim, D. Lee and T. Goodson, J. Am. Chem. Soc., 2010, 132, 16–17 CrossRef CAS PubMed.
- H. Häkkinen, Nat. Chem., 2012, 4, 443–455 CrossRef PubMed.
- Y. Li, G. Galli and F. Gygi, ACS Nano, 2008, 2, 1896–1902 CrossRef CAS PubMed.
- L. Shang, S. Dong and G. U. Nienhaus, Nano Today, 2011, 6, 401–418 CrossRef CAS.
- Y. Yu, Q. Yao, Z. Luo, X. Yuan, J. Y. Lee and J. Xie, Nanoscale, 2013, 5, 4606–4620 RSC.
- S. Chen, R. S. Ingram, M. J. Hostetler, J. J. Pietron, R. W. Murray, T. G. Schaaff, J. T. Khoury, M. M. Alvarez and R. L. Whetten, Science, 1998, 280, 2098–2101 CrossRef CAS PubMed.
- W. Chen and S. Chen, Angew. Chem., Int. Ed., 2009, 48, 4386–4389 CrossRef CAS PubMed.
- Y. Liu, H. Tsunoyama, T. Akita and T. Tsukuda, Chem. Commun., 2010, 46, 550–552 RSC.
- Y. Liu, H. Tsunoyama, T. Akita, S. Xie and T. Tsukuda, ACS Catal., 2011, 1, 2–6 CrossRef CAS.
- G. Ramakrishna, O. Varnavski, J. Kim, D. Lee and T. Goodson, J. Am. Chem. Soc., 2008, 130, 5032–5033 CrossRef CAS PubMed.
- M. A. Habeeb Muhammed, A. K. Shaw, S. K. Pal and T. Pradeep, J. Phys. Chem. C, 2008, 112, 14324–14330 CrossRef.
- Z. Wu and R. Jin, Nano Lett., 2010, 10, 2568–2573 CrossRef CAS PubMed.
- S. Link, A. Beeby, S. FitzGerald, M. A. El-Sayed, T. G. Schaaff and R. L. Whetten, J. Phys. Chem. B, 2002, 106, 3410–3415 CrossRef CAS.
- Y. Negishi, Y. Takasugi, S. Sato, H. Yao, K. Kimura and T. Tsukuda, J. Am. Chem. Soc., 2004, 126, 6518–6519 CrossRef CAS PubMed.
- Y. Negishi, K. Nobusada and T. Tsukuda, J. Am. Chem. Soc., 2005, 127, 5261–5270 CrossRef CAS.
- J. T. Petty, J. Zheng, N. V. Hud and R. M. Dickson, J. Am. Chem. Soc., 2004, 126, 5207–5212 CrossRef CAS PubMed.
- H. C. Yeh, J. Sharma, J. J. Han, J. S. Martinez and J. H. Werner, Nano Lett., 2010, 10, 3106–3110 CrossRef CAS PubMed.
- L. Sutarlie, K. M. M. Aung, M. G. L. Lim, S. Lukman, E. Cheung and X. Su, Plasmonics, 2014, 9, 753–763 CrossRef CAS.
- J. Sun, Y. Xianyu and X. Jiang, Chem. Soc. Rev., 2014, 43, 6239–6253 RSC.
- H. Nishi, K. Asami and T. Tatsuma, Opt. Mater. Express, 2016, 6, 1043 CrossRef CAS.
- S. K. Ghosh and T. Pal, Chem. Rev., 2007, 107, 4797–4862 CrossRef CAS PubMed.
- R. Liu, R. Liew, J. Zhou and B. Xing, Angew. Chem., 2007, 119, 8955–8959 CrossRef.
- N. Nath and A. Chilkoti, Anal. Chem., 2002, 74, 504–509 CrossRef CAS PubMed.
- J. Liu and Y. Lu, J. Am. Chem. Soc., 2003, 125, 6642–6643 CrossRef CAS PubMed.
- C. A. Mirkin, R. L. Letsinger, R. C. Mucic and J. J. Storhoff, Nature, 1996, 382, 607–609 CrossRef CAS PubMed.
- T. M. H. Lee, Sensors, 2008, 8, 5535–5559 CrossRef CAS PubMed.
- L. R. Hirsch, J. B. Jackson, A. Lee, N. J. Halas and J. L. West, Anal. Chem., 2003, 75, 2377–2381 CrossRef CAS PubMed.
- N. T. Kim Thanh and Z. Rosenzweig, Anal. Chem., 2002, 74, 1624–1628 CrossRef PubMed.
- R. M. Pallares, M. Bosman, N. T. K. Thanh and X. Su, Nanoscale, 2016, 8, 19973–19977 RSC.
- R. M. Pallares, S. L. Kong, T. H. Ru, N. T. K. Thanh, Y. Lu and X. Su, Chem. Commun., 2015, 51, 14524–14527 RSC.
- R. Kanjanawarut and X. Su, Anal. Chem., 2009, 81, 6122–6129 CrossRef CAS PubMed.
- X. Su and R. Kanjanawarut, ACS Nano, 2009, 3, 2751–2759 CrossRef CAS PubMed.
- A. P. Demchenko, Methods Appl. Fluoresc., 2013, 1 Search PubMed.
- R. M. Pallares, L. Sutarlie, N. T. K. Thanh and X. Su, Sens. Actuators, B, 2018, 271, 97–103 CrossRef CAS.
- L. Dykman and N. Khlebtsov, Chem. Soc. Rev., 2012, 41, 2256–2282 RSC.
- K. A. Willets and R. P. Van Duyne, Annu. Rev. Phys. Chem., 2007, 58, 267–297 CrossRef CAS PubMed.
- J. G. Gordon and S. Ernst, Surf. Sci., 1980, 101, 499–506 CrossRef CAS.
- C. Nylander, B. Liedberg and T. Lind, Sens. Actuators, 1982, 3, 79–88 CrossRef CAS.
- J. Homola, Chem. Rev., 2008, 108, 462–493 CrossRef CAS PubMed.
- J. Ye, K. Bonroy, D. Nelis, F. Frederix, J. D'Haen, G. Maes and G. Borghs, Colloids Surf., A, 2008, 321, 313–317 CrossRef CAS.
- N. Verellen, P. Van Dorpe, C. Huang, K. Lodewijks, G. A. E. Vandenbosch, L. Lagae and V. V. Moshchalkov, Nano Lett., 2011, 11, 391–397 CrossRef CAS PubMed.
- A. G. Brolo, R. Gordon, B. Leathem and K. L. Kavanagh, Langmuir, 2004, 20, 4813–4815 CrossRef CAS PubMed.
- A. V. Whitney, J. W. Elam, S. Zou, A. V. Zinovev, P. C. Stair, G. C. Schatz and R. P. Van Duyne, J. Phys. Chem. B, 2005, 109, 20522–20528 CrossRef CAS PubMed.
-
E. Smith and G. Dent, Modern Raman Spectroscopy – A Practical Approach, John Wiler & Sons, New York, 2005 Search PubMed.
- K. Kneipp, H. Kneipp, I. Itzkan, R. R. Dasari and M. S. Feld, Chem. Rev., 1999, 99, 2957–2976 CrossRef CAS.
- M. J. Banholzer, J. E. Millstone, L. Qin and C. A. Mirkin, Chem. Soc. Rev., 2008, 37, 885–897 RSC.
- K. Kneipp, H. Kneipp and J. Kneipp, Acc. Chem. Res., 2006, 39, 443–450 CrossRef CAS PubMed.
- W. E. Doering and S. Nie, J. Phys. Chem. B, 2002, 106, 311–317 CrossRef CAS.
- P. G. Etchegoin and E. C. Le Ru, Phys. Chem. Chem. Phys., 2008, 10, 6079–6089 RSC.
- H. Xu, E. Bjerneld, M. Käll and L. Börjesson, Phys. Rev. Lett., 1999, 83, 4357–4360 CrossRef CAS.
- B. N. J. Persson, Chem. Phys. Lett., 1981, 82, 561–565 CrossRef CAS.
- A. Otto, J. Raman Spectrosc., 2005, 36, 497–509 CrossRef CAS.
- X. X. Han, B. Zhao and Y. Ozaki, Anal. Bioanal. Chem., 2009, 394, 1719–1727 CrossRef CAS PubMed.
-
R. Thompson, in Fluorescence sensors and biosensors, CRC press, Boca Raton, FL, 2005, p. 377 Search PubMed.
- K. Aslan, I. Gryczynski, J. Malicka, E. Matveeva, J. R. Lakowicz and C. D. Geddes, Curr. Opin. Biotechnol., 2005, 16, 55–62 CrossRef CAS PubMed.
- X. Kong, E. Nir, K. Hamadani and S. Weiss, J. Am. Chem. Soc., 2007, 129, 4643–4654 CrossRef CAS PubMed.
- V. L. Mosiman, B. K. Patterson, L. Canterero and C. L. Goolsby, Commun. Clin. Cytom., 1997, 30, 151–156 CrossRef CAS.
- E. Dulkeith, A. C. Morteani, T. Niedereichholz, T. A. Klar, J. Feldmann, S. A. Levi, F. C. J. M. van Veggel, D. N. Reinhoudt, M. Möller and D. I. Gittins, Phys. Rev. Lett., 2002, 89, 203002 CrossRef CAS PubMed.
- J. Gersten and A. Nitzan, J. Chem. Phys., 1981, 75, 1139–1152 CrossRef CAS.
- E. A. Jares-Erijman and T. M. Jovin, Nat. Biotechnol., 2003, 21, 1387–1395 CrossRef CAS.
- T. L. Jennings, M. P. Singh and G. F. Strouse, J. Am. Chem. Soc., 2006, 128, 5462–5467 CrossRef CAS PubMed.
- C. C. Huang and H. T. Chang, Anal. Chem., 2006, 78, 8332–8338 CrossRef CAS PubMed.
- B.-C. Ye and B.-C. Yin, Angew. Chem., Int. Ed., 2008, 47, 8386–8389 CrossRef CAS PubMed.
- E. Oh, M.-Y. Hong, D. Lee, S.-H. Nam, H. C. Yoon and H.-S. Kim, J. Am. Chem. Soc., 2005, 127, 3270–3271 CrossRef CAS PubMed.
- Y. P. Kim, Y. H. Oh, E. Oh, S. Ko, M. K. Han and H. S. Kim, Anal. Chem., 2008, 80, 4634–4641 CrossRef CAS.
- M. Li, Q. Wang, X. Shi, L. A. Hornak and N. Wu, Anal. Chem., 2011, 83, 7061–7065 CrossRef CAS PubMed.
- J. M. Liu, J. T. Chen and X. P. Yan, Anal. Chem., 2013, 85, 3238–3245 CrossRef CAS.
- J.-L. Ma, B.-C. Yin, H.-N. Le and B.-C. Ye, ACS Appl. Mater. Interfaces, 2015, 7, 12856–12863 CrossRef CAS PubMed.
- S. Lukman, K. M. M. Aung, J. Liu, B. Liu and X. Su, ACS Appl. Mater. Interfaces, 2013, 5, 12725–12734 CrossRef CAS.
- S. Lukman, K. M. Moh Aung, M. G. Liang Lim, S. Hong, S. K. Tan, E. Cheung and X. Su, RSC Adv., 2014, 4, 8883 RSC.
- X. He, H. Liu, Y. Li, S. Wang, Y. Li, N. Wang, J. Xiao, X. Xu and D. Zhu, Adv. Mater., 2005, 17, 2811–2815 CrossRef CAS.
- N. Zhang, Y. Liu, L. Tong, K. Xu, L. Zhuo and B. Tang, Analyst, 2008, 133, 1176–1181 RSC.
- J.-H. Lin, C.-W. Chang and W.-L. Tseng, Analyst, 2010, 135, 104–110 RSC.
- M. De, S. Rana, H. Akpinar, O. R. Miranda, R. R. Arvizo, U. H. F. Bunz and V. M. Rotello, Nat. Chem., 2009, 1, 461–465 CrossRef CAS.
- R. L. Phillips, O. R. Miranda, C.-C. You, V. M. Rotello and U. H. F. Bunz, Angew. Chem., Int. Ed., 2008, 47, 2590–2594 CrossRef CAS.
- B. Dubertret, M. Calame and A. J. Libchaber, Nat. Biotechnol., 2001, 19, 365–370 CrossRef CAS.
- P. C. Ray, A. Fortner and G. K. Darbha, J. Phys. Chem. B, 2006, 110, 20745–20748 CrossRef CAS.
- R. Bardhan, N. K. Grady, J. R. Cole, A. Joshi and N. J. Halas, ACS Nano, 2009, 3, 744–752 CrossRef CAS.
- Y. Fu, J. Zhang and J. R. Lakowicz, J. Am. Chem. Soc., 2010, 132, 5540–5541 CrossRef CAS.
- D. Nepal, L. F. Drummy, S. Biswas, K. Park and R. A. Vaia, ACS Nano, 2013, 7, 9064–9074 CrossRef CAS PubMed.
- N. S. Abadeer, M. R. Brennan, W. L. Wilson and C. J. Murphy, ACS Nano, 2014, 8, 8392–8406 CrossRef CAS.
- N. Gandra, C. Portz, L. Tian, R. Tang, B. Xu, S. Achilefu and S. Singamaneni, Angew. Chem., Int. Ed., 2014, 53, 866–870 CrossRef CAS PubMed.
- C. D. Geddes and J. R. Lakowicz, J. Fluoresc., 2002, 12, 121–129 CrossRef.
- K. Aslan, S. N. Malyn and C. D. Geddes, J. Fluoresc., 2007, 17, 7–13 CrossRef CAS PubMed.
- F. Xie, M. S. Baker and E. M. Goldys, Chem. Mater., 2008, 20, 1788–1797 CrossRef CAS.
- Y. Cheng, T. Stakenborg, P. Van Dorpe, L. Lagae, M. Wang, H. Chen and G. Borghs, Anal. Chem., 2011, 83, 1307–1314 CrossRef CAS PubMed.
- S. H. Lee, S. M. Wabaidur, Z. A. Alothman and S. M. Alam, Luminescence, 2011, 26, 768–773 CrossRef CAS PubMed.
- S. Guo and E. Wang, Anal. Chim. Acta, 2007, 598, 181–192 CrossRef CAS PubMed.
- J. M. Pingarrón, P. Yáñez-Sedeño and A. González-Cortés, Electrochim. Acta, 2008, 53, 5848–5866 CrossRef.
- J. Wang, Analyst, 2005, 130, 421–426 RSC.
- X. Luo, A. Morrin, A. J. Killard and M. R. Smyth, Electroanalysis, 2006, 18, 319–326 CrossRef CAS.
- P. M. P. Mandel, C. R. Acad. Sci., 1948, 142, 241–243 CAS.
- N. Suzuki, A. Kamataki, J. Yamaki and Y. Homma, Clin. Chim. Acta, 2008, 387, 55–58 CrossRef CAS PubMed.
- K. Jung, M. Fleischhacker and A. Rabien, Clin. Chim. Acta, 2010, 411, 1611–1624 CrossRef CAS PubMed.
- Y. Li, A. W. Wark, H. J. Lee and R. M. Corn, Anal. Chem., 2006, 78, 3158–3164 CrossRef CAS PubMed.
- S. Jahr, H. Hentze, S. Englisch, D. Hardt, F. O. Fackelmayer, R. D. Hesch and R. Knippers, Cancer Res., 2001, 61, 1659–1665 CAS.
- H. Schwarzenbach, D. S. B. Hoon and K. Pantel, Nat. Rev. Cancer, 2011, 11, 426–437 CrossRef CAS PubMed.
- C. Bettegowda, M. Sausen, R. J. Leary, I. Kinde, Y. Wang, N. Agrawal, B. R. Bartlett, H. Wang, B. Luber and R. M. Alani,
et al.
, Sci. Transl. Med., 2014, 6, 224ra24–224ra24 CrossRef PubMed.
- S. J. Dawson, D. W. Tsui, M. Murtaza, H. Biggs, O. M. Rueda, S. F. Chin, M. J. Dunning, D. Gale, T. Forshew, B. Mahler-Araujo and S. Rajan, N. Engl. J. Med., 2013, 368, 1199–1209 CrossRef CAS PubMed.
- R. van Eijk, J. Licht, M. Schrumpf, M. T. Yazdi, D. Ruano, G. I. Forte, P. M. Nederlof, M. Veselic, K. F. Rabe, J. T. Annema, V. Smit, H. Morreau and T. van Wezel, PLoS One, 2011, 6, e17791 CrossRef CAS PubMed.
- A. Strati, A. Markou, C. Parisi, E. Politaki, D. Mavroudis, V. Georgoulias and E. Lianidou, BMC Cancer, 2011, 11, 422 CrossRef CAS PubMed.
- R. J. Leary, I. Kinde, F. Diehl, K. Schmidt, C. Clouser, C. Duncan, A. Antipova, C. Lee, K. McKernan, F. M. De La Vega, K. W. Kinzler, B. Vogelstein, L. A. Diaz and V. E. Velculescu, Sci. Transl. Med., 2010, 2, 20ra14–20ra14 CrossRef PubMed.
- R. J. Leary, J. Cummins, T. L. Wang and V. E. Velculescu, Nat. Protoc., 2007, 2, 1973 CrossRef CAS PubMed.
- H. Ito, H. Inoue, K. Hasegawa, Y. Hasegawa, T. Shimizu, S. Kimura, M. Onimaru, H. Ikeda and S. ei Kudo, Nanomedicine, 2014, 10, 599–608 CrossRef CAS PubMed.
- H. Ito, K. Hasegawa, Y. Hasegawa, T. Nishimaki, K. Hosomichi, S. Kimura, M. Ohba, H. Yao, M. Onimaru, I. Inoue and H. Inoue, Sci. Rep., 2015, 5, 10455 CrossRef PubMed.
- R. M. Pallares, N. T. K. Thanh and X. Su, Chem. Commun., 2018, 54, 11260–11263 RSC.
- R. Sachidanandam, D. Weissman, S. C. Schmidt, J. M. Kakol, L. D. Stein, G. Marth, S. Sherry, J. C. Mullikin, B. J. Mortimore, D. L. Willey, S. E. Hunt, C. G. Cole, P. C. Coggill, C. M. Rice, Z. Ning, J. Rogers, D. R. Bentley, P. Y. Kwok, E. R. Mardis, R. T. Yeh, B. Schultz, L. Cook, R. Davenport, M. Dante, L. Fulton, L. Hillier, R. H. Waterston, J. D. McPherson, B. Gilman, S. Schaffner, W. J. Van Etten, D. Reich, J. Higgins, M. J. Daly, B. Blumenstiel, J. Baldwin, N. Stange-Thomann, M. C. Zody, L. Linton, E. S. Lander and D. Altshuler, Nature, 2001, 409, 928 CrossRef CAS PubMed.
- E. R. Bleecker, W. B. Isaacs, A. R. Turner, H. Grönberg, D. Duggan, Y. Zhu, S. Smith, P. Stattin, S. L. Zheng, J. Sun, G. Li, F.-C. Hsu, D. A. Meyers, J. D. Carpten, B.-L. Chang, A. K. Kader, F. Wiklund, W. Liu, H.-O. Adami, K. Bälter and J. Xu, N. Engl. J. Med., 2008, 358, 910–919 CrossRef PubMed.
- H. Lee, S. W. Joo, S. Y. Lee, C. H. Lee, K. A. Yoon and K. Lee, Biosens. Bioelectron., 2010, 26, 730–735 CrossRef CAS PubMed.
- H. Lee, T. Kang, K. A. Yoon, S. Y. Lee, S. W. Joo and K. Lee, Biosens. Bioelectron., 2010, 25, 1669–1674 CrossRef CAS PubMed.
- H. Li and L. J. Rothberg, J. Am. Chem. Soc., 2004, 126, 10958–10961 CrossRef CAS PubMed.
- Y. L. Jung, C. Jung, H. Parab, D. Y. Cho and H. G. Park, ChemBioChem, 2011, 12, 1387–1390 CrossRef CAS PubMed.
- J. Li, X. Chu, Y. Liu, J. H. Jiang, Z. He, Z. Zhang, G. Shen and R. Q. Yu, Nucleic Acids Res., 2005, 33, 1–9 CrossRef PubMed.
- Y. S. Huh, A. J. Lowe, A. D. Strickland, C. A. Batt and D. Erickson, J. Am. Chem. Soc., 2009, 131, 2208–2213 CrossRef CAS PubMed.
- A. J. Lowe, Y. S. Huh, A. D. Strickland, D. Erickson and C. A. Batt, Anal. Chem., 2010, 82, 5810–5814 CrossRef CAS PubMed.
- W. Shen, H. Deng, A. K. L. Teo and Z. Gao, Chem. Commun., 2012, 48, 10225–10227 RSC.
- Q. Wang, L. Yang, X. Yang, K. Wang, L. He and J. Zhu, Anal. Chim. Acta, 2011, 688, 163–167 CrossRef CAS PubMed.
- P. Valentini, R. Fiammengo, S. Sabella, M. Gariboldi, G. Maiorano, R. Cingolani and P. P. Pompa, ACS Nano, 2013, 7, 5530–5538 CrossRef CAS PubMed.
- S. Q. Huang, J. Hu, G. Zhu and C. Y. Zhang, Biosens. Bioelectron., 2015, 65, 191–197 CrossRef CAS PubMed.
- D. Kato and M. Oishi, ACS Nano, 2014, 8, 9988–9997 CrossRef CAS PubMed.
- S. S. Jeffrey, A.-L. Borresen-Dale, C. A. Rees, R. Tibshirani, P. E. Lonning, J. R. Pollack, P. O. Brown, T. Sorlie, D. Botstein and C. M. Perou, Proc. Natl. Acad. Sci. U. S. A., 2002, 99, 12963–12968 CrossRef PubMed.
- J. R. Pollack, C. M. Perou, A. A. Alizadeh, M. B. Eisen, A. Pergamenschikov, C. F. Williams, S. S. Jeffrey, D. Botstein and P. O. Brown, Nat. Genet., 1999, 23, 41–46 CrossRef CAS PubMed.
- T. A. Taton, C. A. Mirkin and R. L. Letsinger, Science, 2000, 289, 1757–1760 CrossRef CAS PubMed.
- J. J. Storhoff, S. S. Marla, P. Bao, S. Hagenow, H. Mehta, A. Lucas, V. Garimella, T. Patno, W. Buckingham, W. Cork and U. R. Müller, Biosens. Bioelectron., 2004, 19, 875–883 CrossRef CAS PubMed.
- Y. P. Bao, M. Huber, T. F. Wei, S. S. Marla, J. J. Storhoff and U. R. Müller, Nucleic Acids Res., 2005, 33, e15 CrossRef PubMed.
- T. H. Rabbitts and T. H. Rabbitts, Nature, 1994, 372, 143–149 CrossRef CAS PubMed.
- N. Pallisgaard, P. Hokland, D. C. Riishøj, B. Pedersen and P. Jørgensen, Blood, 1998, 92, 574–588 CAS.
- K. Edwards, C. Johnstone and C. Thompson, Nucleic Acids Res., 1991, 19, 1349 CrossRef CAS PubMed.
- D. P. Kalogianni, V. Bravou, T. K. Christopoulos, P. C. Ioannou and N. C. Zoumbos, Nucleic Acids Res., 2007, 35, e23 CrossRef.
- M. Li, W. D. Chen, N. Papadopoulos, S. N. Goodman, N. C. Bjerregaard, S. Laurberg, B. Levin, H. Juhl, N. Arber, H. Moinova, K. Durkee, K. Schmidt, Y. He, F. Diehl, V. E. Velculescu, S. Zhou, L. A. Diaz, K. W. Kinzler, S. D. Markowitz and B. Vogelstein, Nat. Biotechnol., 2009, 27, 858–863 CrossRef CAS.
- A. H. Nguyen and S. J. Sim, Biosens. Bioelectron., 2014, 67, 443–449 CrossRef PubMed.
- J. Hu and C.-y Zhang, Biosens. Bioelectron., 2012, 31, 451–457 CrossRef CAS PubMed.
- H. Zhang, M. Li, M. Fan, J. Gu, P. Wu and C. Cai, Chem. Commun., 2014, 50, 2932–2934 RSC.
- Filled.
- L. He and G. J. Hannon, Nat. Rev. Genet., 2004, 5, 522 CrossRef CAS PubMed.
- H. Dong, J. Lei, L. Ding, Y. Wen, H. Ju and X. Zhang, Chem. Rev., 2013, 113, 6207–6233 CrossRef CAS.
- J. Brennecke, D. R. Hipfner, A. Stark, R. B. Russell and S. M. Cohen, Cell, 2003, 113, 25–36 CrossRef CAS.
- P. Xu, S. Y. Vernooy, M. Guo and B. A. Hay, Curr. Biol., 2003, 13, 790–795 CrossRef CAS.
- V. Ambros, Cell, 2003, 113, 673–676 CrossRef CAS.
- C. Z. Chen, L. Li, H. F. Lodish and D. P. Bartel, Science, 2004, 5654, 83–86 CrossRef PubMed.
- W. C. S. Cho, Biochim. Biophys. Acta, Rev. Cancer, 2010, 1805, 209–217 CrossRef CAS PubMed.
- T. Dalmay and D. R. Edwards, Oncogene, 2006, 25, 6170 CrossRef CAS PubMed.
- J. Kocerha, S. Kauppinen and C. Wahlestedt, Neuromol. Med., 2009, 11, 162–172 CrossRef CAS PubMed.
- A. K. Pandey, P. Agarwal, K. Kaur and M. Datta, Cell. Physiol. Biochem., 2009, 23, 221–232 CrossRef CAS PubMed.
- S. Saal and S. J. Harvey, Curr. Opin. Nephrol. Hypertens., 2009, 18, 317–323 CrossRef CAS PubMed.
- X. M. Chen, World J. Gastroenterol., 2009, 15, 1665 CrossRef CAS PubMed.
-
C. López-Camarillo and L. A. Marchat, MicroRNAs in cancer, CRC Press, Boca Raton, FL, 2013 Search PubMed.
- G. Di Leva and C. M. Croce, Trends Mol. Med., 2010, 16, 257–267 CrossRef CAS PubMed.
-
C. Love and S. Dave, in Hematological Malignancies, Humana Press, Totowa, NJ, 2013, pp. 285–296 Search PubMed.
- P. S. Mitchell, R. K. Parkin, E. M. Kroh, B. R. Fritz, S. K. Wyman, E. L. Pogosova-Agadjanyan, A. Peterson, J. Noteboom, K. C. O'Briant, A. Allen, D. W. Lin, N. Urban, C. W. Drescher, B. S. Knudsen, D. L. Stirewalt, R. Gentleman, R. L. Vessella, P. S. Nelson, D. B. Martin and M. Tewari, Proc. Natl. Acad. Sci. U. S. A., 2008, 105, 10513–10518 CrossRef CAS PubMed.
- H. Valadi, K. Ekström, A. Bossios, M. Sjöstrand, J. J. Lee and J. O. Lötvall, Nat. Cell Biol., 2007, 9, 654 CrossRef CAS PubMed.
- J. D. Arroyo, J. R. Chevillet, E. M. Kroh, I. K. Ruf, C. C. Pritchard, D. F. Gibson, P. S. Mitchell, C. F. Bennett, E. L. Pogosova-Agadjanyan, D. L. Stirewalt, J. F. Tait and M. Tewari, Proc. Natl. Acad. Sci. U. S. A., 2011, 108, 5003–5008 CrossRef CAS PubMed.
-
M. Accerbi, S. A. Schmidt, E. De Paoli, S. Park, D.-H. Jeong and P. J. Green, in Methods for Isolation of Total RNA to Recover miRNAs and Other Small RNAs from Diverse Species BT – Plant MicroRNAs: Methods and Protocols, ed. B. C. Meyers and P. J. Green, Humana Press, Totowa, NJ, 2010, pp. 31–50 Search PubMed.
- K. A. Cissell, S. Shrestha and S. K. Deo, Anal. Chem., 2007, 4754–4761 CrossRef CAS.
- Y. Tu, P. Wu, H. Zhang and C. Cai, Chem. Commun., 2012, 48, 10718–10720 RSC.
- S. W. Yang and T. Vosch, Anal. Chem., 2011, 83, 6935–6939 CrossRef CAS.
- F. Degliangeli, P. Kshirsagar, V. Brunetti, P. P. Pompa and R. Fiammengo, J. Am. Chem. Soc., 2014, 136, 2264–2267 CrossRef CAS PubMed.
- Y. Q. Liu, M. Zhang, B. C. Yin and B. C. Ye, Anal. Chem., 2012, 84, 5165–5169 CrossRef CAS PubMed.
- H. Dong, S. Jin, H. Ju, K. Hao, L. P. Xu, H. Lu and X. Zhang, Anal. Chem., 2012, 84, 8670–8674 CrossRef CAS PubMed.
- H. Yin, Y. Zhou, C. Chen, L. Zhu and S. Ai, Analyst, 2012, 137, 1389–1395 RSC.
- H. Yin, Y. Zhou, H. Zhang, X. Meng and S. Ai, Biosens. Bioelectron., 2012, 33, 247–253 CrossRef CAS PubMed.
- N. Xia, L. Zhang, G. Wang, Q. Feng and L. Liu, Biosens. Bioelectron., 2013, 47, 461–466 CrossRef CAS PubMed.
- J. Wang, X. Yi, H. Tang, H. Han, M. Wu and F. Zhou, Anal. Chem., 2012, 84, 6400–6406 CrossRef CAS PubMed.
- J. Mandli, H. Mohammadi and A. Amine, Bioelectrochemistry, 2017, 116, 17–23 CrossRef CAS PubMed.
- H. Zhang, M. Fan, J. Jiang, Q. Shen, C. Cai and J. Shen, Anal. Chim. Acta, 2019, 1064, 33–39 CrossRef CAS PubMed.
- J. D. Driskell, A. G. Seto, L. P. Jones, S. Jokela, R. A. Dluhy, Y. P. Zhao and R. A. Tripp, Biosens. Bioelectron., 2008, 24, 917–922 CrossRef CAS PubMed.
- Y. Chen, G. Chen, S. Feng, J. Pan, X. Zheng, Y. Su, Y. Chen, Z. Huang, X. Lin, F. Lan, R. Chen and H. Zeng, J. Biomed. Opt., 2012, 17, 67003 CrossRef PubMed.
- R. D. Li, B. C. Yin and B. C. Ye, Biosens. Bioelectron., 2016, 86, 1011–1016 CrossRef CAS PubMed.
- P. Miao, Y. Tang, Z. Mao and Y. Liu, Part. Part. Syst. Charact., 2017, 34, 1600405 CrossRef.
- W. J. Yang, X. B. Li, Y. Y. Li, L. F. Zhao, W. L. He, Y. Q. Gao, Y. J. Wan, W. Xia, T. Chen, H. Zheng, M. Li and S.-q. Xu, Anal. Biochem., 2008, 376, 183–188 CrossRef CAS PubMed.
- Y.-S. Borghei, M. Hosseini and M. R. Ganjali, Clin. Chim. Acta, 2018, 483, 119–125 CrossRef CAS PubMed.
- S. Fang, H. J. Lee, A. W. Wark and R. M. Corn, J. Am. Chem. Soc., 2006, 128, 14044–14046 CrossRef CAS PubMed.
- M. Castoldi, S. Schmidt, V. Benes, M. Noerholm, A. E. Kulozik, M. W. Hentze and M. U. Muckenthaler, RNA, 2006, 12, 913–920 CrossRef CAS PubMed.
- A. H. Alhasan, D. Y. Kim, W. L. Daniel, E. Watson, J. J. Meeks, C. S. Thaxton and C. A. Mirkin, Anal. Chem., 2012, 84, 4153–4160 CrossRef CAS PubMed.
- M. Cristofanilli, G. T. Budd, M. J. Ellis, A. Stopeck, J. Matera, M. C. Miller, J. M. Reuben, G. V. Doyle, W. J. Allard, L. W. M. M. Terstappen and D. F. Hayes, N. Engl. J. Med., 2004, 351, 781–791 CrossRef CAS PubMed.
- S. J. Cohen, C. J. A. Punt, N. Iannotti, B. H. Saidman, K. D. Sabbath, N. Y. Gabrail, J. Picus, M. Morse, E. Mitchell, M. C. Miller, G. V. Doyle, H. Tissing, L. W. M. M. Terstappen and N. J. Meropol, J. Clin. Oncol., 2008, 26, 3213–3221 CrossRef PubMed.
- J. S. de Bono, H. I. Scher, R. B. Montgomery, C. Parker, M. C. Miller, H. Tissing, G. V. Doyle, L. W. W. M. Terstappen, K. J. Pienta and D. Raghavan, Clin. Cancer Res., 2008, 14, 6302–6309 CrossRef CAS PubMed.
- I. Baccelli, A. Schneeweiss, S. Riethdorf, A. Stenzinger, A. Schillert, V. Vogel, C. Klein, M. Saini, T. Bauerle, M. Wallwiener, T. Holland-Letz, T. Hofner, M. Sprick, M. Scharpff, F. Marme, H. P. Sinn, K. Pantel, W. Weichert and A. Trumpp, Nat. Biotechnol., 2013, 31, 539–544 CrossRef CAS PubMed.
- M. I. Khan, A. M. Czarnecka, R. Duchnowska, W. Kukwa and C. Szczylik, Curr. Signal Transduction Ther., 2014, 8, 240–246 CrossRef PubMed.
- C. L. Chaffer and R. A. Weinberg, Science, 2011, 331, 1559–1564 CrossRef CAS PubMed.
- A. H. Talasaz, A. A. Powell, D. E. Huber, J. G. Berbee, K.-H. Roh, W. Yu, W. Xiao, M. M. Davis, R. F. Pease, M. N. Mindrinos, S. S. Jeffrey and R. W. Davis, Proc. Natl. Acad. Sci. U. S. A., 2009, 106, 3970–3975 CrossRef CAS PubMed.
- S. Sieben, C. Bergemann, A. Lübbe, B. Brockmann and D. Rescheleit, J. Magn. Magn. Mater., 2001, 225, 175–179 CrossRef CAS.
- R. E. Zigeuner, R. Riesenberg, H. Pohla, A. Hofstetter and R. Oberneder, J. Urol., 2003, 169, 701–705 CrossRef CAS.
- S. Nagrath, L. V. Sequist, S. Maheswaran, D. W. Bell, D. Irimia, L. Ulkus, M. R. Smith, E. L. Kwak, S. Digumarthy, A. Muzikansky and P. Ryan, Nature, 2007, 450, 1235–1239 CrossRef CAS PubMed.
- S. L. Stott, C.-H. Hsu, D. I. Tsukrov, M. Yu, D. T. Miyamoto, B. A. Waltman, S. M. Rothenberg, A. M. Shah, M. E. Smas, G. K. Korir, F. P. Floyd, A. J. Gilman, J. B. Lord, D. Winokur, S. Springer, D. Irimia, S. Nagrath, L. V. Sequist, R. J. Lee, K. J. Isselbacher, S. Maheswaran, D. A. Haber and M. Toner, Proc. Natl. Acad. Sci. U. S. A., 2010, 107, 18392–18397 CrossRef CAS.
- A. A. Adams, P. I. Okagbare, J. Feng, M. L. Hupert, D. Patterson, J. Götten, R. L. McCarley, D. Nikitopoulos, M. C. Murphy and S. A. Soper, J. Am. Chem. Soc., 2008, 130, 8633–8641 CrossRef CAS PubMed.
- S. Jeon, J. M. Moon, E. S. Lee, Y. H. Kim and Y. Cho, Angew. Chem., Int. Ed., 2014, 53, 4597–4602 CrossRef CAS.
- W. Y. Hong, S. H. Jeon, E. S. Lee and Y. Cho, Biomaterials, 2014, 35, 9573–9580 CrossRef CAS PubMed.
- H. W. Hou, M. E. Warkiani, B. L. Khoo, Z. R. Li, R. A. Soo, D. S.-W. Tan, W.-T. Lim, J. Han, A. A. S. Bhagat and C. T. Lim, Sci. Rep., 2013, 3, 1259 CrossRef PubMed.
- S. Zheng, H. Lin, J. Q. Liu, M. Balic, R. Datar, R. J. Cote and Y. C. Tai, J. Chromatogr. A, 2007, 1162, 154–161 CrossRef CAS.
- R. A. Burrell, N. McGranahan, J. Bartek and C. Swanton, Nature, 2013, 501, 338–345 CrossRef CAS.
- V. Almendro, A. Marusyk and K. Polyak, Annu. Rev. Pathol., 2013, 8, 277–302 CrossRef CAS.
- C. E. Meacham and S. J. Morrison, Nature, 2013, 501, 328–337 CrossRef CAS.
- R. Gertler, R. Rosenberg, K. Fuehrer, M. Dahm, H. Nekarda and J. R. Siewert, Recent Results Cancer Res., 2003, 162, 149–155 Search PubMed.
- V. Müller, N. Stahmann, S. Riethdorf, T. Rau, T. Zabel, A. Goetz, F. Jänicke and K. Pantel, Clin. Cancer Res., 2005, 11, 3678–3685 CrossRef.
- T. M. Morgan, P. H. Lange and R. L. Vessella, Front. Biosci., 2007, 12, 3000–3009 CrossRef CAS PubMed.
- G. Vona, A. Sabile, M. Louha, V. Sitruk, S. Romana, D. Franco, M. Pazzagli, M. Vekemans, B. Lacour and P. Paterlini-bre, Am. J. Pathol., 2000, 156, 57–63 CrossRef CAS.
- H. Mohamed, M. Murray, J. N. Turner and M. Caggana, J. Chromatogr. A, 2009, 1216, 8289–8295 CrossRef CAS.
- S. J. Tan, L. Yobas, G. Y. H. Lee, C. N. Ong and C. T. Lim, Biomed. Microdevices, 2009, 11, 883–892 CrossRef.
- S. Riethdorf, H. Fritsche, V. Müller, T. Rau, C. Schindlbeck, B. Rack, W. Janni, C. Coith, K. Beck, F. Jänicke, S. Jackson, T. Gornet, M. Cristofanilli and K. Pantel, Clin. Cancer Res., 2007, 13, 920–928 CrossRef CAS.
- W. J. Allard, J. Matera, M. C. Miller, M. Repollet, M. C. Connelly, C. Rao, A. G. J. Tibbe, J. W. Uhr and L. W. M. M. Terstappen, Clin. Cancer Res., 2004, 10, 6897–6904 CrossRef.
- S. Maheswaran, L. V. Sequist, S. Nagrath, L. Ulkus, B. Brannigan, C. V. Collura, E. Inserra, S. Diederichs, A. J. Iafrate, D. W. Bell, S. Digumarthy, A. Muzikansky, D. Irimia, J. Settleman, R. G. Tompkins, T. J. Lynch, M. Toner and D. A. Haber, N. Engl. J. Med., 2008, 359, 366–377 CrossRef CAS.
- S. M. Shamah, J. M. Healy and S. T. Cload, Acc. Chem. Res., 2008, 41, 130–138 CrossRef CAS PubMed.
- W. Lu, A. K. Singh, S. A. Khan, D. Senapati, H. Yu and P. C. Ray, J. Am. Chem. Soc., 2010, 132, 18103–18114 CrossRef CAS.
- L. Beqa, Z. Fan, A. K. Singh, D. Senapati and P. C. Ray, ACS Appl. Mater. Interfaces, 2011, 3, 3316–3324 CrossRef CAS PubMed.
- X. Wang, C. Wang, L. Cheng, S. T. Lee and Z. Liu, J. Am. Chem. Soc., 2012, 134, 7414–7422 CrossRef CAS PubMed.
- S. A. Khan, R. Kanchanapally, Z. Fan, L. Beqa, A. K. Singh, D. Senapati and P. C. Ray, Chem. Commun., 2012, 48, 6711–6713 RSC.
- M. Y. Sha, H. Xu, M. J. Natan and R. Cromer, J. Am. Chem. Soc., 2008, 130, 17214–17215 CrossRef CAS PubMed.
- P. Zhang, R. Zhang, M. Gao and X. Zhang, ACS Appl. Mater. Interfaces, 2014, 6, 370–376 CrossRef CAS.
- X. Wang, X. Qian, J. J. Beitler, Z. G. Chen, F. R. Khuri, M. M. Lewis, H. J. C. Shin, S. Nie and D. M. Shin, Cancer Res., 2011, 71, 1526–1532 CrossRef CAS.
- X. Wu, L. Luo, S. Yang, X. Ma, Y. Li, C. Dong, Y. Tian, L. Zhang, Z. Shen and A. Wu, ACS Appl. Mater. Interfaces, 2015, 7, 9965–9971 CrossRef CAS.
- W. Shi, R. J. Paproski, R. Moore and R. Zemp, J. Biomed. Opt., 2014, 19, 56014 CrossRef PubMed.
- T. Xue, S. Wang, G. Ou, Y. Li, H. Ruan, Z. Li, Y. Ma, R. Zou, J. Qiu, Z. Shen and A. Wu, Anal. Methods, 2019, 11, 2918–2928 RSC.
- Y. S. Borghei, M. Hosseini, M. Dadmehr, S. Hosseinkhani, M. R. Ganjali and R. Sheikhnejad, Anal. Chim. Acta, 2016, 904, 92–97 CrossRef CAS.
- C. D. Medley, J. E. Smith, Z. Tang, Y. Wu, S. Bamrungsap and W. Tan, Anal. Chem., 2008, 80, 1067–1072 CrossRef CAS.
- W. Lu, S. R. Arumugam, D. Senapati, A. K. Singh, T. Arbneshi, S. A. Khan, H. Yu and P. C. Ray, ACS Nano, 2010, 4, 1739–1749 CrossRef CAS.
- W. Liu, H. Wei, Z. Lin, S. Mao and J. M. Lin, Biosens. Bioelectron., 2011, 28, 438–442 CrossRef CAS.
- E. I. Galanzha, E. V. Shashkov, T. Kelly, J. W. Kim, L. Yang and V. P. Zharov, Nat. Nanotechnol., 2009, 4, 855 CrossRef CAS.
- D. S. Seferos, D. A. Giljohann, H. D. Hill, A. E. Prigodich and C. A. Mirkin, J. Am. Chem. Soc., 2007, 129, 15477–15479 CrossRef CAS PubMed.
- C. H. J. Choi, L. Hao, S. P. Narayan, E. Auyeung and C. A. Mirkin, Proc. Natl. Acad. Sci. U. S. A., 2013, 110, 7625–7630 CrossRef CAS.
- T. L. Halo, K. M. McMahon, N. L. Angeloni, Y. Xu, W. Wang, A. B. Chinen, D. Malin, E. Strekalova, V. L. Cryns, C. Cheng, C. A. Mirkin and C. S. Thaxton, Proc. Natl. Acad. Sci. U. S. A., 2014, 111, 17104–17109 CrossRef CAS.
- W. E. Briley, M. H. Bondy, P. S. Randeria, T. J. Dupper and C. A. Mirkin, Proc. Natl. Acad. Sci. U. S. A., 2015, 112, 9591–9595 CrossRef CAS.
- M. Toloudi, P. Apostolou, M. Chatziioannou and I. Papasotiriou, Case Rep. Oncol., 2011, 4, 44–54 CrossRef PubMed.
- K. Lee, V. P. Drachev and J. Irudayaraj, ACS Nano, 2011, 5, 2109–2117 CrossRef CAS PubMed.
- M. A. Woo, S. M. Lee, G. Kim, J. H. Baek, M. S. Noh, J. E. Kim, S. J. Park, A. Minai-Tehrani, S. C. Park, Y. T. Seo, Y. K. Kim, Y. S. Lee, D. H. Jeong and M. H. Cho, Anal. Chem., 2009, 81, 1008–1015 CrossRef CAS PubMed.
- G. Van Niel, I. Porto-Carreiro, S. Simoes and G. Raposo, J. Biochem., 2006, 140, 13–21 CrossRef CAS PubMed.
- E. van der Pol, A. N. Boing, P. Harrison, A. Sturk and R. Nieuwland, Pharmacol. Rev., 2012, 64, 676–705 CrossRef CAS.
- J. M. Street, P. E. Barran, C. L. Mackay, S. Weidt, C. Balmforth, T. S. Walsh, R. T. A. Chalmers, D. J. Webb and J. W. Dear, J. Transl. Med., 2012, 10, 5 CrossRef CAS PubMed.
- C. Lässer, V. Seyed Alikhani, K. Ekström, M. Eldh, P. Torregrosa Paredes, A. Bossios, M. Sjöstrand, S. Gabrielsson, J. Lötvall and H. Valadi, J. Transl. Med., 2011, 9, 9 CrossRef PubMed.
- P. A. Gonzales, T. Pisitkun, J. D. Hoffert, D. Tchapyjnikov, R. A. Star, R. Kleta, N. S. Wang and M. A. Knepper, J. Am. Soc. Nephrol., 2009, 20, 363–379 CrossRef CAS.
- M. P. Caby, D. Lankar, C. Vincendeau-Scherrer, G. Raposo and C. Bonnerot, Int. Immunol., 2005, 17, 879–887 CrossRef CAS PubMed.
- Z. Wang, H. J. Wu, D. Fine, J. Schmulen, Y. Hu, B. Godin, J. X. J. Zhang and X. Liu, Lab Chip, 2013, 13, 2879–2882 RSC.
- B. Gyorgy, K. Modos, E. Pallinger, K. Paloczi, M. Pasztoi, P. Misjak, M. A. Deli, A. Sipos, A. Szalai, I. Voszka, A. Polgar, K. Toth, M. Csete, G. Nagy, S. Gay, A. Falus, A. Kittle and E. I. Buzas, Blood, 2011, 117, e39–e48 CrossRef CAS PubMed.
- M. C. Larson, M. R. Luthi, N. Hogg and C. A. Hillery, Cytometry, Part A, 2013, 83, 242–250 CrossRef.
- R. A. Dragovic, C. Gardiner, A. S. Brooks, D. S. Tannetta, D. J. P. Ferguson, P. Hole, B. Carr, C. W. G. Redman, A. L. Harris, P. J. Dobson, P. Harrison and I. L. Sargent, Nanomedicine, 2011, 7, 780–788 CrossRef CAS PubMed.
- F. Momen-Heravi, L. Balaj, S. Alian, P. Y. Mantel, A. E. Halleck, A. J. Trachtenberg, C. E. Soria, S. Oquin, C. M. Bonebreak, E. Saracoglu, J. Skog and W. P. Kuo, Biol. Chem., 2013, 394, 1253–1262 CAS.
- H. G. Lamparski, A. Metha-Damani, J. Y. Yao, S. Patel, D. H. Hsu, C. Ruegg and J. B. Le Pecq, J. Immunol. Methods, 2002, 270, 211–226 CrossRef CAS.
- A. Liga, A. D. B. Vliegenthart, W. Oosthuyzen, J. W. Dear and M. Kersaudy-Kerhoas, Lab Chip, 2015, 15, 2388–2394 RSC.
- C. Chen, J. Skog, C. H. Hsu, R. T. Lessard, L. Balaj, T. Wurdinger, B. S. Carter, X. O. Breakefield, M. Toner and D. Irimia, Lab Chip, 2010, 10, 505–511 RSC.
- A. Clayton, J. Court, H. Navabi, M. Adams, M. D. Mason, J. A. Hobot, G. R. Newman and B. Jasani, J. Immunol. Methods, 2001, 247, 163–174 CrossRef CAS PubMed.
- B. J. Tauro, D. W. Greening, R. A. Mathias, H. Ji, S. Mathivanan, A. M. Scott and R. J. Simpson, Methods, 2012, 56, 293–304 CrossRef CAS PubMed.
- E. Van Der Pol, A. G. Hoekstra, A. Sturk, C. Otto, T. G. Van Leeuwen and R. Nieuwland, J. Thromb. Haemost., 2010, 8, 2596–2607 CrossRef CAS.
- R. Lacroix, S. Robert, P. Poncelet, R. S. Kasthuri, N. S. Key and F. Dignat-George, J. Thromb. Haemostasis, 2010, 8, 2571–2574 CrossRef CAS PubMed.
- E. N. M. Nolte-'t Hoen, E. J. van der Vlist, M. Aalberts, H. C. H. Mertens, B. J. Bosch, W. Bartelink, E. Mastrobattista, E. V. B. van Gaal, W. Stoorvogel, G. J. A. Arkesteijn and M. H. M. Wauben, Nanomedicine, 2012, 8, 712–720 CrossRef PubMed.
- L. Tirinato, F. Gentile, D. Di Mascolo, M. L. Coluccio, G. Das, C. Liberale, S. A. Pullano, G. Perozziello, M. Francardi, A. Accardo, F. De Angelis, P. Candeloro and E. Di Fabrizio, Microelectron. Eng., 2012, 97, 337–340 CrossRef CAS.
-
L. T. Kerr, L. Gubbins, K. W. Gorzel, S. Sharma, M. Kell, A. McCann and B. M. Hennelly, Biophotonics: Photonic Solutions for Better Health Care IV, International Society for Optics and Photonics, 2014, vol. 9129, pp. 91292Q–1–91292Q–9 Search PubMed.
- Z. Wang, S. Zong, Y. Wang, N. Li, L. Li, J. Lu, Z. Wang, B. Chen and Y. Cui, Nanoscale, 2018, 10, 9053–9062 RSC.
- C. Lee, R. P. Carney, S. Hazari, Z. J. Smith, A. Knudson, C. S. Robertson, K. S. Lam and S. Wachsmann-Hogiu, Nanoscale, 2015, 7, 9290–9297 RSC.
- Y. G. Zhou, R. M. Mohamadi, M. Poudineh, L. Kermanshah, S. Ahmed, T. S. Safaei, J. Stojcic, R. K. Nam, E. H. Sargent and S. O. Kelley, Small, 2016, 12, 727–732 CrossRef CAS PubMed.
- H. Im, H. Shao, Y. I. Park, V. M. Peterson, C. M. Castro, R. Weissleder and H. Lee, Nat. Biotechnol., 2014, 32, 490 CrossRef CAS PubMed.
- G. Di Noto, A. Bugatti, A. Zendrini, E. L. Mazzoldi, A. Montanelli, L. Caimi, M. Rusnati, D. Ricotta and P. Bergese, Biosens. Bioelectron., 2016, 77, 518–524 CrossRef CAS.
- S. A. Melo, H. Sugimoto, J. T. O'Connell, N. Kato, A. Villanueva, A. Vidal, L. Qiu, E. Vitkin, L. T. Perelman, C. A. Melo, A. Lucci, C. Ivan, G. A. Calin and R. Kalluri, Cancer Cell, 2014, 26, 707–721 CrossRef CAS PubMed.
- S. S. Kanwar, C. J. Dunlay, D. M. Simeone and S. Nagrath, Lab Chip, 2014, 14, 1891–1900 RSC.
- C. Théry, S. Amigorena, G. Raposo and A. Clayton, Curr. Protoc. Cell Biol., 2006, 30, 3–22 Search PubMed.
- M. Czarnek and J. Bereta, Sci. Rep., 2017, 7, 11682 CrossRef PubMed.
|
This journal is © The Royal Society of Chemistry 2019 |