DOI:
10.1039/C9NR01105F
(Paper)
Nanoscale, 2019,
11, 18224-18231
Quantitative profiling of integrin αvβ3 on single cells with quantum dot labeling to reveal the phenotypic heterogeneity of glioblastoma†
Received
3rd February 2019
, Accepted 11th September 2019
First published on 26th September 2019
Abstract
The distribution, localization and density of individual molecules (e.g. drug-specific receptors) on single cells can offer profound information about cell phenotypes. Profiling this information is a new research direction within the field of single cell biology, but it remains technically challenging. Through the combined use of quantum dot labeling, structured illumination microscopy (SIM) and computer-aided local surface reconstruction, we acquired a 3D imaging map of a drug target molecule, integrin αvβ3, on glioblastoma cells at the single cell level. The results revealed that integrin αvβ3 exhibits discrete distribution on the surface of glioblastoma cells, with its density differing significantly among cell lines. The density is illustrated as the approximate number of target molecules per μm2 on the irregular cell surface, ranging from 0 to 1.6. Functional studies revealed that the sensitivity of glioblastoma cells to inhibitor molecules depends on the density of the target molecules. After inhibitor treatment, the viability and invasion ability of different glioblastoma cells were highly correlated with the density of integrin αvβ3 on their surfaces. This study not only provides a novel protocol for the quantitative analysis of surface proteins from irregular single cells, but also offers a clue for understanding the heterogeneity of tumor cells on the basis of molecular phenotypes. Thus, this work has potential significance in guiding targeted therapies for cancers.
Introduction
The development of precise analytical techniques has greatly expedited the study of genomics,1,2 transcriptomics3–5 and proteomics,6–8 as well as epigenomics9 at the single-cell level. A large number of studies have revealed diversity and heterogeneity within the same type of cells, thus providing invaluable insights into the complexity of biological processes and disease mechanisms. However, it is still a big challenge to study the phenotypic heterogeneity of cells, as important in situ information, including distribution and quantity of proteins on a single cell, is difficult to acquire.
Cell surface receptors, such as G Protein-Coupled Receptor (GPCR) and programmed cell death protein-1 (PD-1)/programmed death-ligand 1 (PD-L1) receptors, play important roles in cellular processes and cell–cell communications.10,11 Precise in situ information about the distribution, localization and density of these receptors on the cell surface can provide immediate evidence for the phenotypic heterogeneity of cells. To acquire this information at the single cell level, technologies with super high resolution, localization accuracy and quantitative information are needed. Flow cytometry is a widely used technique for cell sorting, which can estimate 500–107 molecules by measuring the total fluorescence intensity of a fluorescent probe on a single cell.12 However, the resolution of flow cytometry is not high enough to distinguish single molecules. Mass cytometry (CyTOF) is a powerful high-throughput technology, which can simultaneously determine more than 30 kinds of protein in a single cell, with a detection sensitivity of about 1500 molecules per cell.12–14 However, CyTOF is unable to provide information about the distribution and localization of target molecules on single cells. Recently, we introduced quantum dot labeling and super-resolution microscopy for the proposed purpose. Quantum dot labels exhibit extremely high brightness and resistance to photobleaching, making them ideal for single-molecule imaging.15–17 The lateral and axial resolutions of Structured Illumination Microscopy (SIM) are 80–120 and 300 nm, respectively, which are high enough for 3D single-cell imaging. By combining these two techniques, we made the first attempt to profile a target molecule on single acute myeloid leukemia cells.18 This method, however, is not readily applicable to solid tumor cells. The reason is that it is difficult to determine their surface area and the density of a given target molecule on the cell surface, because they normally exhibit adherent growth with irregular morphology. To solve this problem, and as a systematic study, here, we used quantum dot labeling and super-resolution microscopy with computer-aided local surface reconstruction to obtain information on the density and spatial distribution of target molecules on single adherent tumor cells.
To demonstrate the concept, integrin αvβ3, one of the most intensely studied integrin receptors of glioblastoma cells,19 was used as a model molecule in this study. Glioma is a typical heterogeneous disease.20,21 Immunohistochemistry tests have shown that the expression of integrin αvβ3 is associated with the transformation from low-grade astroglial-derived tumors to advanced glioblastoma.22,23 We hypothesized that the expression level of integrin αvβ3 in glioblastoma is associated with tumor cell properties, and that profiling integrin αvβ3 on glioblastoma cells may provide insights into its function in disease mechanisms and tumor heterogeneity, as well as the efficiency of chemotherapy. As a result, we obtained 3D images of intact cells which exhibit the distribution (discrete), localization (cell membrane bound) and density (molecule numbers per μm2) of the target receptor molecules on irregular and adherent cell surfaces. The density was found to be significantly different among the three glioblastoma cell lines. Functional studies revealed that the density of integrin αvβ3 on the cell surface is highly associated with both the invasion ability and viability of glioblastoma cells treated with inhibitors of integrin αvβ3.
Results and discussion
Scheme for the quantitative profiling of integrin αvβ3 on a single glioblastoma cell
The experiment scheme has two parts, as shown in Fig. 1. Part I is the labeling of integrin αvβ3 on the cell surface. The integrin was first incubated with the primary antibody, and then labeled with QD605 secondary antibody (Fig. 1a). In part II, the labeled glioblastoma cells were observed under SIM to acquire 3D images of single cells. Fig. 1b shows the maximum intensity projection (MIP) image of a glioblastoma cell with the quantum dot-labeled integrin αvβ3 on its surface. Since the glioblastoma cells present adherent growth with irregular shapes, it is difficult to directly acquire 3D images of whole cells. To solve this problem, a local surface reconstruction method was applied through the QD-labeled receptor continuous recognition and delineation on 2D slices (Fig. 1b). After the reconstruction, the number of integrin αvβ3 molecules per μm2 on the surface of the glioblastoma cells was quantified. Through the two-step experiment, we were able to profile the target molecules, with the quantitative information from both the intact cell and the local details. Consequently, we could investigate the relationship between the density of integrin αvβ3 on glioblastoma cells and cancer cell invasion ability and viability upon drug treatment.
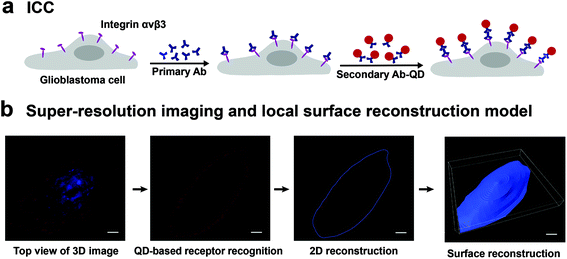 |
| Fig. 1 A schematic representation of the super-resolution imaging and quantitative analysis of integrin αvβ3 on a single glioblastoma cell. (a) Cell labeling: cells are labeled with QD-antibody-conjugated probes by immunocytochemistry (ICC). (b) 3D SIM images (left): the maximum intensity projection (MIP) images of integrin αvβ3 on a glioblastoma cell. Surface reconstruction model: the surface reconstruction of 3D SIM images of the glioblastoma cell for quantitative analyses. Scale bars: 5 μm. | |
3D super-resolution imaging of integrin αvβ3 on a single glioblastoma cell
The glioblastoma cells labeled with QD605 were examined under SIM. The software SIMcheck was used to profile the channel intensity of raw SIM data and to produce Fourier plots of reconstructed SIM data to assess the quality of the SIM imaging.24 The data showed the discrete distribution of integrin αvβ3 on the cell surface in the form of red fluorescent dots, as shown in Fig. 2a and Movie S1.† No obvious variations of signal intensity from the Channel intensity profile was observed (Fig. 2b), which indicates that little photobleaching appeared between the illumination pattern angles. The reconstructed SIM data were further subject to Fourier transform to test the resolution. Fig. 2c shows representative Fourier plots from the images along the xy cross-section (right panels) and xz cross-section (left panels), respectively. As can be seen, the lateral Fourier spectrum of the integrin αvβ3 image presents hexagonal symmetry, which indicates that there is no loss of signal at high frequency. Importantly, the concentric rings in the Fourier spectrum indicate the corresponding lateral resolution of ∼0.1 μm and axial resolution of ∼0.3 μm. Taken together, our results clearly demonstrate the high quality of the SIM images to be used for further analyses.
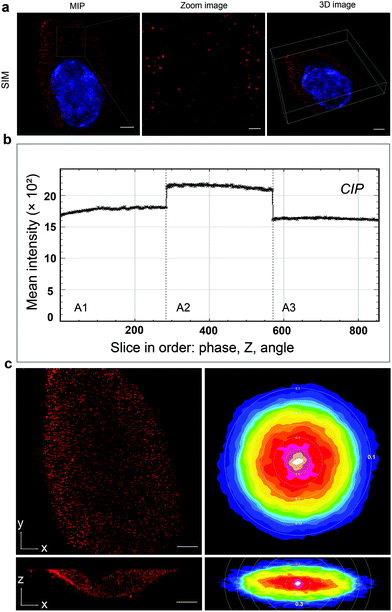 |
| Fig. 2 3D super-resolution imaging of integrin αvβ3 on a single glioblastoma cell. (a) MIP (maximal intensity projection) image, zoom image and 3D image of integrin αvβ3 on a single glioblastoma cell under SIM mode. (b) Channel intensity profiles (CIP) of raw SIM data show the differences in fluorescence intensity between illumination pattern angles. (c) The MIP of the images along the xy cross-section and xz cross-section with SIM (left panels) and Fourier plots of lateral and orthogonal cross-sections of reconstructed SIM data. Scale bars: zoom image, 1 μm; others, 5 μm. | |
Local cell-surface reconstruction and quantification of integrin αvβ3 expression
Cell-surface reconstruction and estimation of the expression level of integrin αvβ3 were performed to gain more quantitative information.25,26 However, due to the adherent growth and irregular size of the cells, and the presence of microvesicles (Fig. S3c†), we could not obtain the total amount and density of integrin αvβ3 on single cells. To avoid these interferences, we developed a protocol to reconstruct the local cell surface for calculating the surface area and to measure the expression quantity of the membrane-bound integrin. Through smoothing the biomarker distribution outline, local cell-surface reconstruction was implemented to obtain accurate spatial distribution information on integrin αvβ3. The procedures involved in our strategy are introduced in Fig. 3, and related details are provided in Fig. S3.†
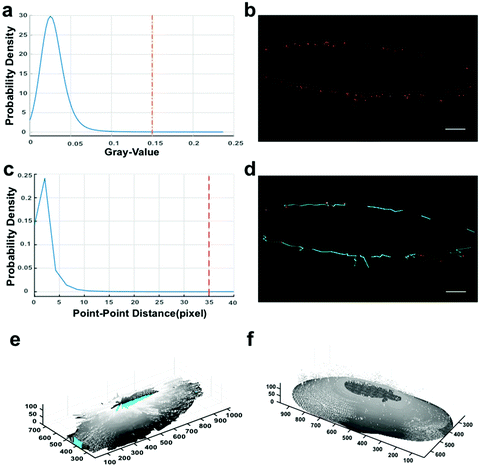 |
| Fig. 3 Local cell-surface reconstruction based on bio-imaging markers from SIM data. The default size unit is the pixel, and the corresponding physical size unit (39.5 nm per X/Y-pixel, 125 nm per Z-pixel) depends on the SIM metadata. (a and c) Probability densities of gray values and point-point distances. To adapt all the stacks of SIM data, we set the cut-off values as 0.15 for thresholding and 35 pixels (i.e. 1383 nm) for determining the potential adjacent connections. (b) Fluorescent dot identification by labeling connected components in 2D binary images.27 (d) Connecting adjacent fluorescent dots. Between the two points of one determined connection, linear interpolation (cyan lines) was performed in their respective dimensions to fill up the contour gap for the next contour smoothing. (e) Outline sketch. (f) The integrated outcome of circumferential and z-axial smoothing. Related details in the Methods section. Scale bars: 5 μm. | |
By verifying whether the quantum dots are distributed around the reconstructed surfaces (Fig. S3a†) to prove the reliability of our reconstruction approach, we performed a chi-square goodness-of-fit test and accepted the null hypothesis. The results suggest that the distribution takes the form of “single peak and symmetry” around the surface. Meanwhile, the analyses displayed in Fig. S3d† prove that the distribution of fluorescent dots around the surface approximates a normal distribution. Therefore, we conclude that fluorescence signals centralize at the boundary of the reconstructed local cell surface, and the processing is reliable for further analyses.
Quantitative analysis of the density of integrin αvβ3 on different subtypes of glioblastoma cells
To determine the heterogeneity of glioblastoma cells, we imaged and quantified integrin αvβ3 on three different glioblastoma cell lines (U87MG, U251, A172). Fig. 4a clearly demonstrates the expression level and distribution of integrin αvβ3 on these three subtypes of glioblastoma cells at horizontal viewing angles of 0°, 120° and 240°. Our data illustrate that U87MG cells show significantly higher expression of integrin αvβ3, and the U251 cell line has a relatively low expression of the integrin, which is in line with other studies28 but at much higher resolution. Meanwhile, the A172 cell line has no obvious expression of the integrin. The super-resolution imaging and local surface reconstruction allowed us to quantify integrin αvβ3 by counting the number of fluorescent dots on the reconstructed 3D surfaces of glioblastoma cells. We found that there are 2880 ± 150 fluorescent dots on the U87MG cells and 1270 ± 80 fluorescent dots on U251 cells by imaging 50 individual cells for each subtype of glioblastoma cells (data not shown); mean ± SEM (Standard Error of Measurement). As the cells of each cell line possess different size, the whole cell surface cannot be explored due to a limited field of view. Thus, the expression of integrin αvβ3 was calculated by the fluorescent dots per μm2 of the cell surface, which is easier and more valuable for calculating the density of the receptor molecules. Fig. 4b shows that the density (molecule numbers per μm2) of integrin αvβ3 molecules on the surface of U87MG and U251 cells is approximately 1.6 μm−2 and 1.2 μm−2, respectively, and the integrin was not detected on the surface of A172 cells.
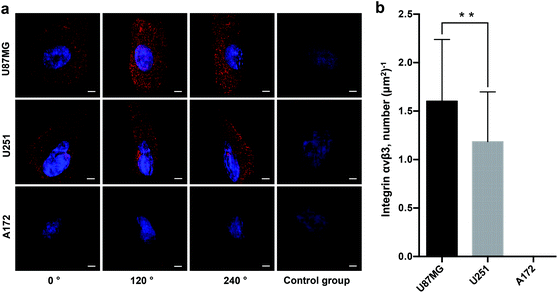 |
| Fig. 4 Quantitative profiling of integrin αvβ3 on different glioblastoma cell lines with SIM and quantitative analyses. (a) QD-based super-resolution imaging shows the expression and distribution of αvβ3 on different glioblastoma cell lines (U87MG, U251 and A172). Images were taken at horizontal viewing angles of 0°, 120° and 240°. The right-side images are of a control group without primary antibody. (b) The density of αvβ3 receptors was averaged from 50 individual cells for each cell line. Scale bars: 5 μm. Data are presented as mean ± SD. **p < 0.01. | |
Here, we put forward two arguments about the accuracy of this quantification. First, the integrin receptors are not stationary within the live cell membrane. In a cancer cell, the initial integrin-ligand binding event would recruit additional integrin receptors and other cytosolic proteins to the adhesion site in order to form adhesion complexes. These complexes are dynamic multiprotein structures that can range in size from nascent adhesions (<250 nm) to focal adhesions (up to 500 nm).29 In this case, two problems arise: (1) due to their large size, the antibody/QD complexes may only bind to one receptor molecule while hindering the attachment of more due to size constraints; and (2) even if two receptor molecules in an adhesion complex (with size <150 nm) can be successfully labeled, the signals from two QDs are hard to distinguish with SIM, as SIM's xy resolution limit is 80–120 nm. Therefore, the number of integrin αvβ3 molecules is underestimated. However, such underestimation is unlikely to occur in this experiment, largely because labeling was applied to immobilized cells, on which no ligand molecules were applied in advance; thus, there should be no integrin-ligand binding-triggered recruitment of receptor molecules. The signal dots on the cell surface presented a highly discrete distribution, with distances larger than 200 nm, which is also supported by TEM images (Fig. S2†). Nevertheless, if there are focal adhesions formed by the target receptor molecules with unknown reasons before labeling, the quantification would be underestimated.
Second, the QD signals of some vesicular protrusions were suspected to originate from microvesicles secreted from the cells and thus were excluded in building the QD signal dataset for cell surface reconstruction. It is worth noting that this treatment may affect the quantification of the integrin αvβ3 molecules. However, there were only a few numbers of these structures per U87MG cell, and the U251 cells were mostly free of them. Compared to the large area of the whole cell surface, the area of a small number of these structures is too small. Neglecting the signal dots from these structures would have no effect on the final quantification result.
Taken together, the quantity measured by the proposed method might be underestimated for a number of reasons, but not significantly.
Correlation analysis of αvβ3 density and drug susceptibility of glioblastoma cells
Various studies have shown that integrin αvβ3 exhibits multifaceted functions in gliomas, including tumor migration/invasion, angiogenesis30,31 and cell survival.32 Further, transwell invasion and cell viability experiments were performed, as we were interested to know the effect of the density of integrin αvβ3 on the survival and invasion of glioblastoma cells. The data showed that the numbers of invaded cells were significantly reduced in U87MG and U251 cells, while no apparent change was observed in A172 cells after blocking the integrin αvβ3 using the antibody LM609, as shown in Fig. S1.† The reduction in the invasion of U87MG and U251 cells compared to A172 cells after blocking the activity of integrin αvβ3 using the LM609 antibody (as shown in Fig. 5a), demonstrates that cells containing a higher density of integrin αvβ3 are more sensitive to LM609 antibody than cells containing a lower density of integrin αvβ3. Therefore, it was concluded that the density of integrin αvβ3 may exhibit a positive correlation with the invasion ability.
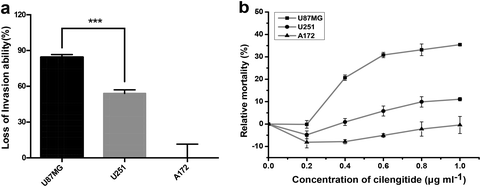 |
| Fig. 5 Cell invasion ability and cell viability inhibited by integrin αvβ3 antibody or small-molecule inhibitors. (a) U87MG, U251 and A172 cells expressing different amounts of αvβ3 were cultured in a serum-free medium for 12 h, and the cell invasion ability was assessed over 24 h using the transwell invasion assay (the chamber coated with matrigel) with or without 10 μg ml−1 LM609 Ab. Histograms show the loss of invasion ability of three glioblastoma cell lines after adding LM609 Ab. (b) The three types of glioma cells (U87MG, U251 and A172) were treated with Cilengitide for 12 h, and then cell viabilities were measured with the CellTiter Blue Cell Viability Assay. The relative mortality was obtained with different concentrations of Cilengitide. Data are presented as the mean ± SD. ***p < 0.001. | |
To further investigate the relationship between the density of integrin αvβ3 and cell viability, glioblastoma cells were treated with Cilengitide, a small-molecule inhibitor of integrin αvβ3. The data showed that the relative cell mortality rates among the three cell lines were different with increasing concentration of Cilengitide, as shown in Fig. 5b. U87MG cells with a higher density of integrin αvβ3 were more sensitive to Cilengitide compared to A172 cells, which showed the lowest expression of integrin αvβ3. Therefore, the density of integrin αvβ3 may exhibit the same positive correlation with cell viability as the invasion ability. Taken together, the data clearly showed that the density of the integrin was related to tumor invasion and survival of the glioblastoma cells under the targeted drug treatment, i.e. the higher the density of the integrin, the more obvious the loss of invasion ability and the lower the survival rate. It is suggested that the density of the target molecules has a potential reference value in clinical targeted therapy. However, cancer is a complex disease, and the behavior of tumor cells is often determined by multiple factors. Establishing a multi-target profiling map for a type of tumor cells will undoubtedly enhance the efficiency of the targeted therapy.
Experimental section
Cell culture and reagents
U87MG, U251 and A172 cells (KeyGEN, China) were cultured in Dulbecco's modified Eagle Medium (DMEM) supplemented with 10% fetal bovine serum (FBS, Gibco) at 37 °C with 5% CO2 in a humidified atmosphere. Mouse anti-αvβ3 human antibody (LM 609) was purchased from Merck Millipore (Germany). Anti-mouse IgG (H + L)-conjugated quantum dots (QD-605) were purchased from Thermo Fisher Scientific (USA). Goat serum was purchased from Beyotime (China). Cilengitide was purchased from SelleckChem (USA).
Immunocytochemistry assay
The cells adhered to the bottom of the glass dish were fixed with 4% paraformaldehyde for 15 min at room temperature, and blocked with 10% goat serum in PBS for 1 h at 37 °C. Cells were then incubated with anti-αvβ3 antibody (1 μg ml−1) at 37 °C for 1 h. Cells were washed three times with PBS and incubated with 20 nM QD-605-conjugated goat anti-mouse IgG at 37 °C for 1 h. Cells were washed again with PBS and incubated with Hoechst 33258 (Sigma, USA) at 37 °C for 10 min. Following staining, cells were washed again and images were taken using a Delta Vision OMX V3 imaging system (GE Healthcare, USA).
Transwell invasion assay
An invasion assay was performed on a transwell chamber with an 8 μm diameter pore size filter membrane (Corning, USA). After 12 h of serum starvation, cells were trypsinized and resuspended in serum-free DMEM. 2 × 105 cells diluted in 200 μL serum-free medium containing the LM 609 (10 μg ml−1) were placed in the upper transwell chamber. Medium containing 10% FBS was added to the lower chamber of the wells as a chemoattractant. The invasion assay was performed at 37 °C in an incubator with 5% CO2 for 24 h. Cells at the top chamber surface were lightly scraped off with cotton swabs. Cells that had invaded through the filters to the bottom chamber surface were fixed with 4% paraformaldehyde for 20 min and stained with crystal violet for 30 min. The images were taken using a Cytation 3 (BioTek, Gene Company Limited, Hong Kong); the numbers of invaded cells were counted in six fields (394 × 291 μm per field) per membrane using image J software.
Cell viability assay
Cell viability was determined using the Cell Titer-Blue Cell Viability Assay Kit (Promega, USA). According to manufacturer's instructions, glioma cells were seeded in triplicate with 2 × 104 cells per well in a black 96-well clear flat-bottomed microplate. After cells had been attached to the bottom, Cilengitide, a small-molecule inhibitor of integrin αvβ3, was added at different concentrations. After 12 h, 20 μL CellTiter-Blue Reagent was added and cells were incubated at 37 °C for 2 h in a CO2 incubator. Then, cells were detected using a microplate reader, and the fluorescence was recorded at 560/590 nm.
3D-SIM microscopy
All 3D-SIM images of cells were acquired on the Delta Vision OMX V3 imaging system (GE Healthcare, USA) with a 100× 1.4 NA oil-immersion objective (Olympus, UPlanSApo), solid-state multimode lasers (405 nm), and electron-multiplying CCD (charge-coupled device) cameras (Evolve 512 × 512, Photometrics). Serial z-stack sectioning was performed at 125 nm intervals for the SIM mode. To obtain optimal images, immersion oils with refractive index of 1.518 were used for glioblastoma cells on glass bottom dish. The microscope was routinely calibrated with 100 nm fluorescent spheres to calculate both the lateral and axial limits of image resolution. SIM image stacks were reconstructed using SoftWoRx 6.1.1 (GE Healthcare, USA) with the following settings: pixel size, 39.5 nm; channel-specific optical transfer functions; Wiener filter constant, 0.0010; discard negative intensities background; drift correction with respect to first angle; and custom K0 guess angles for camera positions. The reconstructed images were further processed for maximum-intensity projections with SoftWoRx 6.1.1. Pixel registration was corrected to be less than 1 pixel for all channels using 100 nm Tetraspeck beads. Images in Fig. 1b, 2a and 4a are 3D SIM images acquired on the DeltaVision OMX V3 imaging system.
Transmission electron microscopy
Cells were fixed with 2.5% (vol/vol) glutaraldehyde in Phosphate Buffer (PB) (0.1 M, pH 7.4), and washed four times in PB. Then, cells were post fixed with 1% (wt/vol) osmium tetraoxide in PB for 2 h at 4 °C, dehydrated through a graded ethanol series (30, 50, 70, 80, 90, 100%, 7 min each) into pure acetone (2 × 10 min). Samples were infiltrated in graded mixtures (3
:
1, 1
:
1, 1
:
3) of acetone and SPI-PON812 resin (16.2 g SPI-PON812, 10 g DDSA and 8.9 g NMA), and also pure resin. Finally, cells were embedded in pure resin with 1.5% BDMA and polymerized for 12 h at 45 °C, and 48 h at 60 °C. The ultrathin sections (70 nm thick) were sectioned with microtome (Leica EM UC6), double-stained by uranyl acetate and lead citrate, and examined using a transmission electron microscope (FEI Tecnai Spirit 120 kV).
Integrin αvβ3 expression quantity measurement
Considering the XY-resolution and Z-resolution of SIM are 100 nm and 300 nm, respectively, and the Z-spacing of cell scanning is 125 nm, we codified the measurement regarding 100 nm × 100 nm × 125 nm as a “patch” voxel unit to obtain quantitative information on the targets and the coordinate information for the next cell-surface reconstruction. The “patches” were determined using the gray cut-off value. In the present study, we chose the gray value 0.15, of which the tangential direction is close to the X-axis in the estimated probability density distribution curve of gray values (Fig. 3a), which confirmed that the distribution of integrin αvβ3 observed under both SIM (Fig. 3b) and TEM (Fig. S2†) showed similar discrete-topology, indicating that the current fluorescent dots represent most of the signals on the cell surface. The same cut-off value was applied to all stacks to obtain 2D (two-dimensional) binary images.27 Then, the connected components were identified to obtain the coordinates and the number of target molecules (Fig. 3b). Because the Z-axis resolution of SIM is 300 nm and the Z-spacing is 125 nm, in the z direction, the fluorescent dots of one conjugate might repeatedly appear in three adjacent slices and be counted three times. Therefore, the numbers of “patches” were divided by three to evaluate the number of integrin αvβ3 molecules.
Local cell-surface reconstruction
With the 300 nm Z-axis resolution for the current SIM images, to our knowledge there is currently no perfect solution of surface reconstruction for a complex multiform uneven curved surface. Given that the intention in our study was to roughly reconstruct the cell surface and calculate the cell surface area for the density evaluation, we eventually took advantage of the robustness of the LOESS algorithm to generate a contour plot of the slice data to illustrate the cell surface. Based on the probability density estimation of point–point distances (Fig. 3c) and the argument–polar radius curve of the polar coordinate system whose pole is the corresponding projective point from the centroid of the whole convex hull, we chose the point–point distance value of 1383 nm for connecting the adjacent points so that most of the key contour points are included. Linear interpolation was implemented in each respective dimension to fill up the contour gap, and the curves were smoothed using LOESS.33,34 During processing, for each set of raw data in the usual vector form, we calculated the smooth ratio, which is less than or equal to 1, and is a percentage of the total number of data points. A 2nd degree polynomial model was used on the correlation-weighted indices to smooth the raw data and avoid noise. The circumferential (LOESS smooth ratio: 0.01) and z-axial (LOESS smooth ratio: 0.01) smooth processing were based on argument–polar radius curves and slice index–polar radius curves, respectively. An integrated computation of the above contours was implemented to obtain the local surface area for estimating the density. Considering the mentioned interference factors, we used only 85% of slices close to the cell bottom to reconstruct the local cell surface.
Cell-surface validation
To verify the reconstruction processing, we supplied a simple but efficient validation, i.e. the probability density estimation of signal points around the reconstructed cell surface in the radial direction. We obtained a series of numbers of quantum dots at different Euclidean distances to the reconstructed surface-based zoom ratio (Fig. S3d†) in the radial direction, and then a chi-square goodness-of-fit test was performed to test the null hypothesis, whereby data come from a normal distribution with a mean and variance estimated from itself at a 1% significance level, indicating that signals centralize at the boundary of the reconstructed cell surface and the method fits well with the morphological distribution of signals.
Statistical analysis
Data are shown as mean ± SD (Standard Deviation), and the two-tailed t-test was used to analyze statistically significant differences, unless otherwise stated. When analyzing the distribution, the probability density estimation was based on a normal kernel function and evaluated at 100 equally spaced points. We conducted all analyses using in-house scripts.
Conclusions
In summary, by integrating quantum dot labeling with 3D super-resolution microscopy and local surface reconstruction, we successfully profiled the distribution and density of integrin αvβ3 on single glioblastoma cells, which exhibit adherent growth with irregular morphology, with the approximate number of target receptors per μm2 on the cell surface. The research quantitatively demonstrated the phenotypical heterogeneity of glioblastoma at the single cell level, and revealed how drug susceptibility relates to the receptor density on the test cells. The protocol, in principle, could be exploited for quantitatively profiling many other surface proteins on cells of interest.
Conflicts of interest
There are no conflicts to declare.
Acknowledgements
This work was supported by a grant from the Strategic Priority Research Program of the Chinese Academy of Sciences (No. XDB29050100) and the National Key Research and Development Program of China (No. 2017YFA0205500). We would like to thank the Center for Biological Imaging, IBP-CAS, in particular, Shuoguo Li, Yun Feng and Can Peng for technique support with SIM and TEM work. We would also like to thank Tahir Mehmood for his valuable suggestions.
Notes and references
- K. J. Livak, Q. F. Wills, A. J. Tipping, K. Datta, R. Mittal, A. J. Goldson, D. W. Sexton and C. C. Holmes, Methods, 2013, 59, 71–79 CrossRef CAS PubMed.
- S. K. Min, W. Y. Kim, Y. Cho and K. S. Kim, Nat. Nanotechnol., 2011, 6, 162–165 CrossRef CAS PubMed.
- A. M. Streets, X. Zhang, C. Cao, Y. Pang, X. Wu, L. Xiong, L. Yang, Y. Fu, L. Zhao, F. Tang and Y. Huang, Proc. Natl. Acad. Sci. U. S. A., 2014, 111, 7048–7053 CrossRef CAS PubMed.
- A. K. White, M. VanInsberghe, O. I. Petriv, M. Hamidi, D. Sikorski, M. A. Marra, J. Piret, S. Aparicio and C. L. Hansen, Proc. Natl. Acad. Sci. U. S. A., 2011, 108, 13999–14004 CrossRef CAS PubMed.
- O. I. Petriv, F. Kuchenbauer, A. D. Delaney, V. Lecault, A. White, D. Kent, L. Marmolejo, M. Heuser, T. Berg, M. Copley, J. Ruschmann, S. Sekulovic, C. Benz, E. Kuroda, V. Ho, F. Antignano, T. Halim, V. Giambra, G. Krystal, C. J. Takei, A. P. Weng, J. Piret, C. Eaves, M. A. Marra, R. K. Humphries and C. L. Hansen, Proc. Natl. Acad. Sci. U. S. A., 2010, 107, 15443–15448 CrossRef CAS PubMed.
- S. Bockenhauer, A. Furstenberg, X. J. Yao, B. K. Kobilka and W. E. Moerner, J. Phys. Chem. B, 2011, 115, 13328–13338 CrossRef CAS.
- S. L. Faley, M. Copland, J. Reboud and J. M. Cooper, Biomicrofluidics, 2011, 5, 24106 CrossRef PubMed.
- Y. Zhan, V. A. Martin, R. L. Geahlen and C. Lu, Lab. Chip, 2010, 10, 2046–2048 RSC.
- B. R. Hyun, J. L. McElwee and P. D. Soloway, Methods, 2015, 72, 41–50 CrossRef CAS PubMed.
- Y. Li, F. Li, F. Jiang, X. Lv, R. Zhang, A. Lu and G. Zhang, Int. J. Mol. Sci., 2016, 17, 1151 CrossRef PubMed.
- K. L. Pierce, R. T. Premont and R. J. Lefkowitz, Nat. Rev. Mol. Cell Biol., 2002, 3, 639–650 CrossRef CAS PubMed.
- S. C. Bendall and G. P. Nolan, Nat. Biotechnol., 2012, 30, 639–647 CrossRef CAS PubMed.
- S. C. Bendall, G. P. Nolan, M. Roederer and P. K. Chattopadhyay, Trends Immunol., 2012, 33, 323–332 CrossRef CAS PubMed.
- D. R. Bandura, V. I. Baranov, O. I. Ornatsky, A. Antonov, R. Kinach, X. Lou, S. Pavlov, S. Vorobiev, J. E. Dick and S. D. Tanner, Anal. Chem., 2009, 81, 6813–6822 CrossRef CAS PubMed.
- F. Pinaud, S. Clarke, A. Sittner and M. Dahan, Nat. Methods, 2010, 7, 275–285 CrossRef CAS PubMed.
- P. Alivisatos, Nat. Biotechnol., 2004, 22, 47–52 CrossRef CAS PubMed.
- X. Gao, L. Yang, J. A. Petros, F. F. Marshall, J. W. Simons and S. Nie, Curr. Opin. Biotechnol., 2005, 16, 63–72 CrossRef CAS PubMed.
- Y. Xi, D. B. Wang, T. T. Wang, L. Huang and X. E. Zhang, Nanoscale, 2018, 4, 1737–1744 Search PubMed.
- C. M. Wu, T. M. Li, T. W. Tan, Y. C. Fong and C. H. Tang, J. Evidence-Based Complementary Altern. Med., 2013, 2013, 423164 Search PubMed.
- D. N. Louis, A. Perry, G. Reifenberger, A. von Deimling, D. Figarella-Branger, W. K. Cavenee, H. Ohgaki, O. D. Wiestler, P. Kleihues and D. W. Ellison, Acta Neuropathol., 2016, 131, 803–820 CrossRef PubMed.
- K. Masui, P. S. Mischel and G. Reifenberger, Handb. Clin. Neurol., 2016, 134, 97–120 Search PubMed.
- O. Schnell, B. Krebs, E. Wagner, A. Romagna, A. J. Beer, S. J. Grau, N. Thon, C. Goetz, H. A. Kretzschmar, J. C. Tonn and R. H. Goldbrunner, Brain Pathol., 2008, 18, 378–386 CrossRef CAS PubMed.
- C. L. Gladson and D. A. Cheresh, J. Clin. Invest., 1991, 88, 1924–1932 CrossRef CAS PubMed.
- G. Ball, J. Demmerle, R. Kaufmann, I. Davis, I. M. Dobbie and L. Schermelleh, Sci. Rep., 2016, 6, 20754 CrossRef CAS PubMed.
- J. R. Swedlow, I. G. Goldberg, K. W. Eliceiri and O. M. E. Consortium, Annu. Rev. Biophys., 2009, 38, 327–346 CrossRef CAS PubMed.
- J. R. Swedlow, I. Goldberg, E. Brauner and P. K. Sorger, Science, 2003, 300, 100–102 CrossRef CAS PubMed.
-
R. M. Haralick and L. G. Shapiro, Computer and robot vision, 1992 Search PubMed.
- S. Benedetto, R. Pulito, S. G. Crich, G. Tarone, S. Aime, L. Silengo and J. Hamm, Magn. Reson. Med., 2006, 56, 711–716 CrossRef CAS PubMed.
- F. Karimi, A. J. O'Connor, G. G. Qiao and D. E. Heath, Adv. Healthcare Mater., 2018, 7, e1701324 CrossRef PubMed.
- L. Bello, M. Francolini, P. Marthyn, J. Zhang, R. S. Carroll, D. C. Nikas, J. F. Strasser, R. Villani, D. A. Cheresh and P. M. Black, Neurosurgery, 2001, 49, 380–389 CAS ; discussion 390.
- J. H. Uhm, C. L. Gladson and J. S. Rao, Front. Biosci., 1999, 4, D188–D199 CrossRef CAS PubMed.
- J. H. Kim, L. T. Zheng, W. H. Lee and K. Suk, J. Neurochem., 2011, 117, 494–503 CrossRef CAS PubMed.
- W. S. Cleveland, J. Am. Stat. Assoc., 1979, 74, 829–836 CrossRef.
- W. S. Cleveland, Am. Stat., 1981, 35, 54–54 CrossRef.
Footnotes |
† Electronic supplementary information (ESI) available. See DOI: 10.1039/c9nr01105f |
‡ These authors contributed equally to this work. |
|
This journal is © The Royal Society of Chemistry 2019 |
Click here to see how this site uses Cookies. View our privacy policy here.