DOI:
10.1039/C8MO00193F
(Review Article)
Mol. Omics, 2019,
15, 7-20
Current and future biomarkers for risk-stratification and treatment personalisation in multiple myeloma
Received
16th August 2018
, Accepted 20th December 2018
First published on 7th January 2019
Abstract
Multiple myeloma, an incurable malignancy of the plasma cells in the bone marrow, has a complex pathogenesis due to clonal heterogeneity. Over the years, many clinical trials and researches have led to the development of effective myeloma treatments, resulting in survival prolongation. Molecular prognostic markers for risk-stratification to predict survival, and predictive markers for treatment response are being extensively explored. This review discusses the current risk-adaptive strategies based on genetic and molecular risk signatures that are in practice to predict survival and describes the future prognostic and predictive biomarkers across the fields of genomics, proteomics, and glycomics in myeloma. Gene expression profiling and next generation sequencing are coming to the forefront of risk-stratification and therapeutic-response prediction. Similarly, proteomic and glycomic-based platforms are gaining momentum in biomarker discovery to predict drug resistance and disease progression.
Introduction
Multiple myeloma (MM) represents a model of translational cancer research, affirming the effectiveness of collaborative effort between basic research and clinical medicine.3 The hallmark of MM is characterised by the accumulation of neoplastic plasma cells in the bone marrow resulting in end-organ damage (renal impairment, hypercalcemia, anaemia, and bone destruction). Worldwide data from 2016 showed the age-standardised MM incidence rate was 2.1 per 100
000, with the highest number of cases in Australasia, North America, and Western Europe.6 Progression to MM from the pre-malignant monoclonal gammopathy of undetermined significance (MGUS) occurs at approximately 1% per year, while the risk of transformation from smouldering myeloma to MM is 10% per year for the first 5 years, then 3% per year for the subsequent 5 years, and 1% per year thereafter.9 MGUS and SMM lack end-organ damage and myeloma-defining events (60% or greater bone marrow clonal plasma cells, serum involved/uninvolved free light chain ratio of 100 or greater, more than one focal lesion on Magnetic Resonance Imaging), and do not require treatment. Relapsed MM is defined as progression of previously treated MM requiring salvage therapy, while relapsed and refractory MM refers to disease that is nonresponse to salvage therapy or disease progression within 60 days of last therapy in patients who have achieved minimal response.10,11
MM remains incurable, however remarkable progress has been made leading to advances in treatment that prolong survival (Fig. 1). In the early 1960s, the activity of melphalan was first reported in the treatment of MM,16 resulting in more than 2 years survival increase of MM patients who were melphalan responsive.18 In the late 1980s, treatment for MM took a step forward with the trial of high dose melphalan followed by autologous bone marrow transplantation,23,24 pushing the overall survival to greater than 3 years.25,26 The emergence of immunomodulatory drugs (IMiDs: thalidomide, lenalidomide) and the proteasome inhibitor (PI) bortezomib represented a paradigm shift in the treatment for MM. In general, for newly diagnosed, transplant-eligible patients, the standard anti-myeloma regimen is a 3-drug combination consisting of IMiD, PI, and glucocorticoid, followed by autologous stem cell transplantation maintenance therapy with low-dose IMiD or PI.27 For patients who are ineligible for transplantation, treatment regimen depends on factors such as comorbidities and performance status. The addition of bortezomib to lenalidomide and dexamethasone was shown to improve progression-free and overall survival.28 Thus, either a 3-drug combination can be given for 12–18 months, or alternatively, a 2-drug regimen can be used until disease progression.29
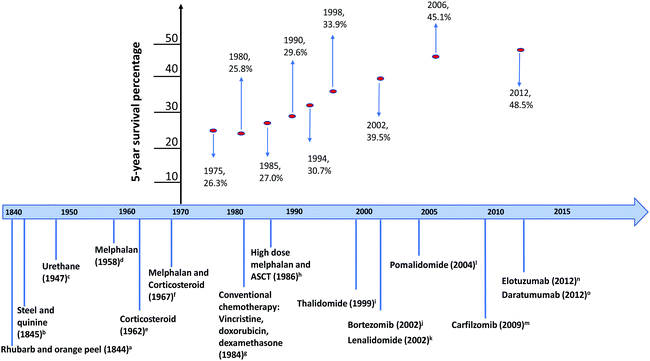 |
| Fig. 1 Treatment timeline and survival improvement in multiple myeloma. 5 year overall survival data obtained from National Cancer Institute Surveillance, Epidemiology, and End Results Program (SEER). a S. Solley,1b T. Watson,2c N. Alwall,4d N. Blokhin et al.,5e R. E. Maas,7f R. Alexanian et al.,8g B. Barlogie et al.,12h T. McElwain and R. Powles,13i S. Singhal et al.,14j R. Orlowski et al.,15k P. G. Richardson et al.,17l S. A. Schey et al.,19m O. Connor et al.,20n J. A. Zonder et al.,21o T. Plesner et al.22 | |
In the relapse setting, pomalidomide (a third generation IMiD)30–32 and carfilzomib (a second generation PI)33 have been shown to improve survival outcome. Recently, immunotherapy is taking centre stage in myeloma therapy, showing the synergistic benefit between elotuzumab (a humanised IgG1 kappa immunostimulatory monoclonal antibody targeting the signalling lymphocytic activation molecule family on natural killer cells) and lenalidomide/dexamethasone extending progression-free survival.34 Significant survival benefit in relapsed refractory MM has been shown with daratumumab, also a humanised IgG1 kappa monoclonal antibody against the transmembrane molecule CD38 overexpressed on myeloma cells, triggering complement-dependent cytotoxicity.35,36
However, as the disease progresses, treatment failure eventually develops due to the emergence of acquired drug resistance and clonal evolution. Even with recent advances in oncogenomics, the molecular mechanisms of MM have not been fully deciphered due to the heterogeneity of the disease. Distinct genetic changes at the time of diagnosis and relapse have been identified, suggesting the possible expansion of resistant clones that are present at low frequency at diagnosis or emerging mutations as MM progresses.37 MM therapy can no longer be considered as a “one size fits all” approach. To individualise treatment for maximum survival benefit, prognostic and/or predictive biomarkers need to be utilised. Prognostic markers provide information about the overall outcome and are often used for risk-stratification, while predictive markers relate to treatment response.38 Biomarkers can be prognostic or predictive or both. In the clinical setting, biomarker assessment should be carried out at diagnosis and at relapse to prognosticate the likely outcome and guide treatment. For patients with newly-diagnosed MM, identification of high and low risk groups is beneficial in selecting the appropriate treatment regimen to maximize the chance of survival. In current practice, genetic and molecular markers have their usefulness in both prognostication and therapeutic prediction, while proteomic and glycomic markers are still in development. Therefore, this review discusses the current risk-adaptive strategies and the potential genomic, proteomic, and glycomic biomarkers for risk-profiling and treatment personalisation.
The current status of risk-profiling: the genetic and molecular biomarkers
The staging systems
The concept of risk-adaptive strategies in MM started in 1975 when Durie and Salmon introduced their clinical staging system using clinical parameters to predict tumour burden.39 The Durie Salmon (DS) system (consisting of haemoglobin, calcium, monoclonal protein level, and lytic bone lesions) became the standard for prognostication. In 2005, the International Staging System (ISS) was validated using only β2-microglobulin and serum albumin as prognostic indicators. The simpler ISS was comparable to the DS system, and thus became widely accepted. Patients with ISS stage I have a median survival of 62 months; while those with stage III have 29 months.40 However, neither the DS nor ISS incorporated the disease aggressiveness. The Revised ISS (RISS) has been recommended by the International Myeloma Working Group (IMWG) to improve prognostication by including lactate dehydrogenase (LDH) level and high-risk cytogenetics.41 High LDH level indicates proliferative disease, thus conferring poor prognosis.42
MM cytogenetics are assessed by metaphase karyotyping and fluorescent in situ hybridization (FISH). Due to low level of proliferating cells, karyotyping is usually not sensitive enough. Therefore, specific FISH probes are required to detect trisomies, translocations, and chromosomal abnormalities.43 Risk stratification should be assessed at diagnosis and at relapse since patients can acquire secondary mutations throughout the course of their disease, placing them in the high-risk category at relapse, despite being low-risk at initial diagnosis. Patients with RISS I, II, and III had a 5 year survival rate of 82%, 62%, and 40%, respectively.44 However, despite improvement, the RISS only incorporates 3 cytogenetic abnormalities [t(4;14), t(14;16) and del(17p)]. Other high-risk cytogenetic aberrations also have prognostic impact on overall survival and choice of therapy. The IMWG considers t(4;14), t(14;16), t(14;20), del(17/17p), gain(1q), nonhyperdiploid, and del(13) as high-risk, while a combination of ≥3 cytogenetic abnormalities indicates ultra-high-risk disease.45 For transplant-eligible patients, high-risk disease denotes an overall survival <3 years, and ultra-high-risk having a survival <2 years. Transplant-ineligible patients with <2 years survival are considered as having high-risk disease. Treatment with a 3-drug regime that combines a PI, an IMiD, and dexamethasone followed by high-dose therapy and autologous stem cell transplantation in transplant-eligible patients only partly overcomes the negative effect of high risk cytogenetics.33,45,46 An integrated analysis by Cavo et al. showed that high-dose therapy plus double autologous stem cell transplantation improves progression-free and overall survival in patients with high-risk cytogenetics who failed complete response after bortezomib-based therapy.47
The strategy by The Mayo Stratification of Myeloma and Risk-Adaptive Therapy (mSMART) classifies MM into high, intermediate, and standard risk according to cytogenetic abnormalities, gene expression profiling (GEP), and plasma cell labelling index (PCLI).48 Currently, GEP is not yet recommended outside of research, however, it is likely to be useful in the clinical setting in the near future. Unlike the RISS, t(4;14) and del(13) are considered as intermediate risk due to improved outcome with bortezomib-based therapy49,50 (Table 1). mSMART consensus also suggests a combination of PI, IMiD, and glucocorticoid, followed by consolidation with autologous stem cell transplantation for high-risk MM.
Table 1 A comparison between the genetic and molecular markers in the RISS and mSMART stratification
Stage |
RISS |
mSMART |
Risk |
I |
Serum albumin ≥3.5 g dL−1 |
5 year OS 82% |
t(11;14) |
Median OS |
Standard |
Serum β2M <3.5 mg L−1 |
t(6;14) |
8–10 years |
No high-risk cytogenetics |
|
|
Normal LDH level |
|
|
|
II |
Not fitting stage I or III |
5 year OS 62% |
t(4;14) |
Median OS |
Intermediate |
del(13) |
4–5 years |
Hypodiploidy |
PCLI ≥ 3% |
|
|
III |
Serum β2M >5.5 mg L−1 |
5 year OS 40% |
del(17p) |
Median OS |
High |
High-risk cytogenetics: t(4;14), t(4;16), or del(17p) or elevated LDH |
t(14;16) |
3 years |
|
t(14;20) |
|
|
GEP high-risk signatures |
|
IgH translocations
Translocations t(11;14) and t(6;14), associating with a favourable prognosis, result in upregulation of cyclin D1 and cyclin D3, respectively. t(11;14) is also present in 35–50% of patients with light chain amyloidosis and 90% of IgM MM. These patients can have lymphoplasmacytic morphology with CD20 expression.51
t(4;14), associated with aggressive disease, affects the telomeres of chromosome 4, disrupting both the FGFR3 and MMSET genes, resulting in IgH/MMSET hybrid transcripts and causes over-expression of FGFR3 in 70% of cases and MMSET in all cases.52–54 MMSET encodes for a histone methyltransferase causing methylation of histone 3 at lysine 36 and subsequent alteration of the chromatin structure. FGFR3 belongs to the transmembrane tyrosine kinase receptors family involved in intracellular signalling pathways.52 Studies in mice bone marrow have reported that over-expression of FGFR3 led to tumour development, and antibody against FGFR3 in vitro has been shown to be effective against MM cells with t(4;14).55,56 Clinically, t(4;14) MM patients have shorter remission duration after high-dose chemotherapy, and more aggressive relapses displaying resistance to alkylating agents.51,57 Therefore, it is recommended that t(4;14) MM should have induction and maintenance treatment with bortezomib-based therapy.44,58 The MAF oncogenes, resulted from t(14;16) and t(14;20), are rare in MM with a prevalence of ∼5%44 (Fig. 2). The consequences of MAF upregulation include dysregulation of cyclin D2 and apoptosis resistance. t(14;20) has a higher incidence in MGUS than in MM and smouldering myeloma. It also carries a strong association with del(13), non-hyperdiploidy and gain of 1q in myeloma. In contrast, MGUS patients with t(14;20) do not carry the same high-risk profile as those with active disease.59
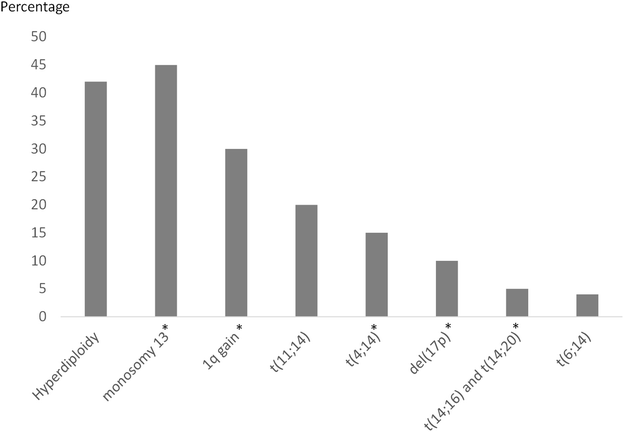 |
| Fig. 2 Prevalence of the common cytogenetic abnormalities used in risk stratification in plasma cell disorders. * denotes high-risk disease. Multiple mutations can occur simultaneously in a plasma cell clone. | |
Chromosomal instability
Approximately 42% of MM cases involve trisomies (hyperdiploid) of odd-numbered chromosomes, belonging to the standard-risk with good prognosis, with the exception of 1q gain.44 MM with trisomies generally displays a good response to lenalidomide-based therapy.60,61 Gain of chromosome 1 at band q21, usually associated with t(4;14) and del(13), is linked with elevated expression level of CKS1B gene, leading to cell cycle progression and disease proliferation.62 Hamamura et al. reported increased frequency of 1q21 gain in relapsed MM, and showed that 1q21 gain is an independent poor prognostic factor of disease progression.63 Monosomy 13/13q deletion's association with poor survival and clonal tumour expansion is related to its link to high-risk genetic translocations such as t(4;14), t(14;16) and t(14;20) in the majority of cases.51
Deletion of 17p13 (p53 locus), occurring in approximately 10% of cases, confers very aggressive disease, a higher rate of extramedullary plasmacytomas, hypercalcemia, and short overall survival.51 After autologous stem cell transplantation, progression-free and overall survival were significantly shorter for patients with p53 deletion than those without (7.9 months vs. 25.7 months, 14 months vs. 48 months, respectively).64 Several trials had shown that bortezomib can improve outcome with t(4;14) but not del(17p).65,66 However, a phase II study showed front-line autologous stem cell transplantation with bortezomib, lenalidomide, dexamethasone in combination for induction and consolidation, followed by lenalidomide maintenance had similar 3 year progression-free survival rate in newly-diagnosed patients with either del(17p), or t(4;14), or del(13q)67 and those with standard risk cytogenetics. Carfilzomib and dexamethasone, with thalidomide68 or lenalidomide69 also showed similar response rates between high-risk and standard-risk newly-diagnosed patients.
For relapsed refractory myeloma patients with del(17p) (whether from the original clone or newly emerging clone), pomalidomide with low-dose dexamethasone have shown encouraging results with similar median overall survival compared to those with standard-risk cytogenetics (12.6 months vs. 14 months, respectively).70 Previous clinical trials had reported the activity of pomalidomide in patients who were refractory to both lenalidomide and bortezomib.30,32 Similar overall response rate was also observed in both high-risk and standard-risk relapse refractory patients when treated with carfilzomib, pomalidomide, and dexamethasone in combination.71
Genetic and molecular biomarkers in the staging system can be both prognostic and predictive. At diagnosis or relapse, the presence of high-risk translocations and/or del(17p) confer poor survival outcome. t(4;14) predicts poor response to alkylating agents, therefore treatment with a PI-based therapy is recommended. Similarly, a triplet combination of PI, IMiD, and glucocorticoid should be used for patients with del(17) at diagnosis, while at relapse, a third-generation IMiD and second or third-generation PI should be considered to overcome clonal resistance.
Serum M-protein and light chains as biomarkers
Monoclonal antibody (M-protein) is the main pathognomonic feature of plasma cell disorders. Although M-protein alone is not part of the diagnostic criteria of MM, in most cases it is elevated except in the cases of oligosecretory and non-secretory myeloma. Traditionally, serial measurement of M-protein is carried out during and after treatment to monitor response and relapse. However, its role as prognostic or predictive marker is not clearly defined.
Meanwhile, serum free light chain (sFLC) ratio not only has a role in the diagnosis and monitoring of disease response, it is also a prognostic marker. Involved to uninvolved sFLC ratio ≥100 is one of the myeloma defining events, and has proven an accurate predictor of progression from SMM to myeloma.44 Abnormal sFLC ratio prior to autologous stem cell transplantation was linked to early disease progression.72 The prognostic impact of abnormal sFLC on progression-free and overall survival was found to be independent of high-risk translocations t(4;14) and t(14;16).73 Patients who relapse with sFLC alone or together with M-protein have shorter survival than those who relapse with M-protein alone, suggesting the clonal heterogeneity model, whereby one clone secretes the intact monoclonal antibody while another more resistant clone produces the light chains.74 A recent study showed that patients in the high-risk group, defined as kappa:lambda ratio ≤0.03 or ≥32, had a significantly lower overall survival compared to the low-risk group.75
Risk stratification in smouldering myeloma
The change in the IMWG diagnostic criteria to include 3 specific biomarkers: clonal plasma cells ≥60%, serum free light chain ratio ≥100, and >1 focal lesion on MRI has shifted the spot-light to ultra-high-risk smouldering myeloma. Patients positive with these markers have an 80% risk of progressing to symptomatic end organ damage.44 Other high-risk criteria include: serum M-protein ≥30 g L−1, IgA subtype, immunoparesis, serum free light chain ratio ≥8 (but <100), progressive increase in M-protein by ≥25% within a 6 month period, bone marrow clonal plasmacytosis 50–60%, elevated circulating plasma cells, MRI with 1 focal lesion or diffuse abnormalities, and PET-CT showing a focal lesion with increased uptake (without osteolysis).76 Investigating the impact of cytogenetics on progression trajectory, Rajkumar et al. showed that patients with t(4;14), del(17p), and 1q gain have a high-risk median time-to-progression (TTP) of 24 months. Those with intermediate risk (trisomies) and standard risk [t(11;14), t(14;16), t(14;20), isolated monosomy 13] have a median TTP of 34 and 55 months, respectively; while patients with no cytogenetic abnormality did not reach their median TTP.77
Despite this best effort of risk-adaptive stratification, the heterogeneity/clonal evolution of the disease and the monitoring of therapeutic response have not been taken into account.78 In most cases, the placement of patients in their respective risk categories occurs at initial diagnosis and the subsequent initial therapeutic regimen is chosen. However, genomic instability of the myeloma clone can lead to therapeutic ineffectiveness during the treatment course. Therefore, potential genomic, proteomic, and glycomic biomarkers for predicting disease progression and therapeutic response are needed to improve risk stratification and disease outcome.
“On the horizon” prognostic and predictive biomarkers in MM
The genomic-based approach
Even though metaphase karyotyping and interphase FISH are currently used to identify high-risk patients, their use has limitation. Since MM is a widely heterogeneous disease, one could argue that karyotyping and FISH cannot fully characterize MM at a molecular level. Gene expression analysis on the other hand, using DNA microarray technology has been shown to be a powerful tool in prognostic prediction. The UAMS group (University of Arkansas for Medical Sciences) characterized 7 molecular subgroups (PR, LB, MS, HY, CD-1, CD-2, MF) for MM based on GEP.79 The LB, HY, CD-1 and CD-2 groups were associated with a good outcome following high-dose chemotherapy and stem cell transplantation. It was also noted that the high-risk subgroups (MS, PR) over-expressed genes mapping to chromosome 1q. Furthermore, Shaughnessy et al. from UAMS identified a set of 70 genes whose expression correlated with high-risk disease and early death. The frequency of high-risk designation increased from 13% at diagnosis to 76% at relapse confirming the molecular proof of disease burden.80
The Intergroupe Francophone de Myelome (IMF) developed a 15-gene model to predict survival in newly diagnosed MM patients treated with high dose therapy. These patients have over-expression of genes involved in essential cell cycle control, DNA replication, DNA repair, mitosis and spindle-assembly checkpoint, leading to chromosomal instability. The IMF 15-gene model is superior to the ISS and more discriminating than FISH for risk stratification.81 Following the IMF, a 6-gene signature was identified for risk prognostication in newly-diagnosed myeloma patients enrolled in the MRC Myeloma IX Study. Even though only present in 12% of cases in the Myeloma IX data set, this specific marker for poor prognosis depends on the ratio of ≥1 for any of these gene pairs: BUB1B (spindle checkpoint and chromosome segregation involvement) versus HDAC3 (transcription regulator), CDC2 (cell cycle control) versus FIS1 (mitochondrial fission involvement), and RAD21 (repairment of DNA double-strand breaks) versus ITM2B (inhibition of beta-amyloid).82 Furthermore, proliferation index (GPI) assessed by GEP was found to be a poor prognostic factor. Newly-diagnosed MM patients with high GPI have a shorter event-free survival compared to those with low GPI.83 A novel survival prediction model (RS for risk score) was proposed by Rème et al. based on GEP of 206 untreated MM patients from Germany and France, which was validated with data from 345 patients in the Total Therapy 2 protocol (TT2 cohort) from UAMS. The RS risk stratifies patients into low, medium, and high risk (RS1, RS2, and RS3, respectively), with the median survival of RS3 between 24–30 months.84
Using newly diagnosed MM patients from the HOVON65/GMMG-HD4 trial and validating with patients from TT2, TT3, MRC IX, and APEX trials, Kuiper et al. comprehensively generated a 92-gene signature to predict overall survival. The EMC-92 was able to determine high risk group across the validation sets, representing different drug regimens across many patient groups including both transplant-eligible and non-transplant eligible, newly diagnosed, and relapse patients.85 Most recently, the biology-based combination of ISS and EMC-92 is even a stronger prognosticator for overall survival, stratifying patients into 4 risk groups. The median overall survival was not reached after 96 months in the low risk cohort (ISS1 and EMC-92 standard risk), while patients in the EMC-92 high risk group only have 24 months (Table 2).86
Table 2 GEP models and their associated overall survival (OS), event-free survival (EFS), or progression-free survival (PFS) for risk-stratification
Study |
Risk model |
Platform |
Patient cohort |
Patient number (N) |
OS |
EFS/PFS |
HR: high risk, I-HR: intermediate high risk, IR: intermediate risk, I-LR: intermediate low risk, LR: low risk, ND MM: newly-diagnosed myeloma, N/A: data not available. Patients were 48 hour post treatment with bortezomib. 8 GEP data sets were used: EMC-92, UAMS-17, UAMS-70, UAMS-80, IFM-15, MRCIX-6, HM-19, and GPI-50. |
Shaughnessy et al. [2007]80 |
UAMS-70 |
Affymetrix U133 Plus 2.0 |
ND MM |
532 |
Median 24mo (HR) |
Median 16.7mo (HR) |
UAMS-17 |
Not reached (LR) |
Not reached (LR) |
Decaux et al. [2008]81 |
IFM-15 |
Single-channel microarray |
ND MM |
250 |
3 year OS: 47.4% (HR) |
N/A |
90.5% (LR) |
Shaughnessy et al. [2010]91 |
UAMS-80 |
Affymetrix U133 Plus 2.0 |
TT3 triala |
142 |
2 year OS: 64% (HR) |
2 year: 54% (HR) |
93% (LR) |
89% (LR) |
Dickens et al. [2010]82 |
MRC myeloma IX-6 |
Affymetrix U133 Plus 2.0 |
ND MM |
258 |
Median 13mo (HR) |
Median 11mo (HR) |
Median 45mo (LR) |
Median 22mo (LR) |
Hose et al. [2011]83 |
GPI |
Affymetrix U133 A + B GeneChip |
ND MM |
757 |
N/A |
Median 12.7mo (HR) |
Affymetrix U133 Plus 2.0 |
Median 26.2mo (IR) |
|
Median 40.6mo (LR) |
Kuiper et al. [2012]85 |
EMC-92 |
Affymetrix U133 Plus 2.0 |
ND MM |
290 |
OS <2years (HR) |
N/A |
Kuiper et al. [2015]86 |
EMC-92+ ISS |
Affymetrix U133 Plus 2.0 |
GEP data sets from 8 studiesb |
Median 24mo (HR) |
N/A |
Affymetrix U133 A + B |
Median 47mo (I-HR) |
|
Median 61mo (I-LR) |
|
Median >96mo (LR) |
With many prognostic indicators, the challenge that arises is how to integrate them into one risk stratification tool that is applicable to clinical practice. Meiβner et al. developed an open-source software platform that provides a GEP report and an HM-metascore, an algorithmic integration of gene-expression based assessment (from the UAMS and IFM scores), proliferation index, target gene expression of aurora kinase A, fibroblast growth factor receptor 3 (FGFR3), insulin-like growth factor 1 receptor (IGF1R), ISS, and cytogenetics. The HM-score delineates 3 groups of patients with different event-free and overall survival. Patients with HM-score of 0 have a 6 year survival rate of 89.3%, those with score of 0.5–3 have a 60.6% rate, and finally those with score of ≥3 have a 18.6% rate. The HM-score was validated using published information on the UAMS TT2 cohort's parameters.87 The GEP report succinctly includes gene expression-based risk assessment/classification and targets for immunotherapy, allowing for individualized treatment.
GEP is also useful to generate predictive markers. Recently, a panel of 14 gene signatures (IMiD-14) predicting response to immunomodulatory agents was developed from newly-diagnosed or relapse refractory myeloma patients, and validated using cohorts of patients who were treated with IMiD-containing regimens from clinical trials.88 Patients with high IMiD-14 scores had a significantly shorter progression-free survival compared to those with low scores, warranting further evaluation of the IMiD-14 as both prognostic and predictive markers for survival and drug resistance.
Going one step further, Terragna et al. identified an 8-gene GEP signature to predict response to thalidomide/dexamethasone. These genes were down-regulated in patients achieving complete response to thalidomide/dexamethasone therapy. Correct prediction was found in 71% of cases analysed.89 GEP can also be useful in pharmacogenomic profiling for tailored treatment. Signatures of bortezomib sensitivity and resistance are being studied using GEP in mouse model of MM. Bortezomib resistant cell lines showed cross-resistance to the next generation proteasome inhibitors, but sensitivity to histone deacetylase inhibitors.90 Although currently, the use of GEP as prognostic and predictive markers has not been validated for clinical practice, mainly due to its limited availability outside research setting, GEP will be critical in the future, not only in MM, but also in other haematological malignancies.
Single-nucleotide polymorphisms (SNPs), located in promotors, exons, introns, or 5′ and 3′ untranslated regions (UTRs) of genes that control DNA mismatch repair, cell cycle, cell metabolism, and inflammatory response are known to associate with cancer susceptibility.92 SNPs are being investigated as potential prognostic and predictive markers for MM. Toll-like receptor 4 (TLR4) and toll/interleukin-1 receptor associated protein (TIRAP) polymorphisms were reported to correlate with progression and survival of MM patients. Carriers of TLR4 SNPs (rs4986790 and rs4986791) who were treated with IMiDs have lower overall response rates compared to non-carriers (62.5% and 57% versus 88%, respectively), while TIRAP SNP (rs8177374) carriers have significantly shorter progression-free and overall survival independent of other prognostic factors such as age, transplant status, ISS, cytogenetics, and LDH.93 Patients who harbour the cytotoxic T lymphocyte antigen-4 (CTLA-4) SNP rs733618 GG have lower survival when they received bortezomib-based therapy.94
Furthermore, MM patients with pre-existing type 2 diabetes mellitus have higher complication and mortality rates compared to non-diabetic patients.95 Constant hyperglycaemia, insulin resistance, and subsequent hyperinsulinaemia stimulate myeloma cell proliferation.96,97 Investigating the correlation between diabetogenic SNPs and MM by variants selection based on genome-wide association studies (GWAS) and genotyping patients from the International Multiple Myeloma rESEarch (IMMENSE) consortium, Rios-Tamayo et al. reported that carriers of the C allele of HNF1B SNP (rs7501939), encoding a transcription factor associated with early onset type 2 diabetes, have poor overall survival compared to the non-diabetogenic T/T genotype.98 Interactions between SNPs affecting immune response genes were also found to influence prognosis. A reduction in 5 year overall survival was observed with gene–gene interactions between the interleukin-6 SNP (IL-6-174GG) and interleukin-10 SNP (IL-10-3575AA) genotypes, as well as between the tumour necrosis factor alpha SNP (TNF-A-308AA + GA) and interleukin-4 SNP (IL-4-1098GG + TG) genotypes.99
Next generation sequencing (NGS), including whole genome sequencing, whole exome sequencing, and targeted NGS panels, offer a powerful platform to explore mutated genes and their prognostic and predictive roles in MM. 15 genes (IRF4, KRAS, NRAS, MAX, HIST1H1E, RB1, EGFR1, TP53, TRAF3, FAM46C, DIS3, BRAF, LTB, CYLD, and FGFR3) were found to be commonly mutated in myeloma, with the mitogen-activated protein kinase (MAPK) and the nuclear factor-κB pathways mostly affected.100,101 Mutations in these genes can be detected in circulating tumour cells as well bone marrow myeloma cells, reflecting the sensitivity of the NGS platform.102 Mutated genes associated with the MAPK pathway (KRAS, NRAS, and BRAF) and the NF-κB pathway (TRAF, CYLD, LTB) are considered to have neutral prognosis, while mutated DNA repair genes (TP53, ATM, and ATR) confer poor prognosis.103 4% of patients with mutated BRAF have higher risk of extramedullary disease and shorter overall survival. Treatment with the BRAF inhibitor vemurafenib had resulted in a reduction in M-protein level and an improvement in performance status.104,105 Whole-genome sequencing of patients from the CoMMpass trial identified targetable genes (IRF4, PRDM1, BCL6, PAX5) in the plasma cell differentiation pathways that can be potential candidates for prognostic and predictive biomarkers.106 Targeted panel sequencing of untreated, newly diagnosed MM patients from the Mayo Clinic reported shortened progression-free and overall survival with STAT3 mutation, while mutations in the cereblon pathway (CRBN, CUL4B, IRF4, IKZF1) may have potential impact on IMiD treatment response.107
Despite the challenges such as high expense, time-consuming analysis, large data sets and limited interpretation, the advantage of NGS to rapidly identify mutations across the myeloma genome makes it a powerful tool in clinical practice to guide treatment.
Potential proteomic biomarkers
Proteomic profiling is one of the most universally applied strategies for cancer biomarker discovery. As blood and plasma circulate in the body, and other bio fluids are near specific organs, they effectively sample most tissues and can contain proteins/peptides. Methods employed in proteomics have been extensively discussed.108,109 The main challenges of proteomic methods in clinical practice are the instrumental technicality, the biological variability of protein levels in patients with MM compared to those of MGUS or normal population, and the numerous factors affecting the proteome during the time of sample collection. Recent advances in proteomics technologies and sample preparation have enabled in-depth analysis of many low abundant proteins/peptides, unlocking a large pool of potentially clinically valuable prognostic and predictive biomarkers for MM.
Sun et al. showed significant increased expression of protein disulphide isomerase A3 (PDIA3) and decreased expression of cytokine-induced apoptosis inhibitor 1 (CPIN1) upon treatment with bendamustine in myeloma cell line RPMI8226.110 Using SILAC (stable isotope labelling with amino acids in cell culture) in combination with phosphoprotein enrichment and LC-MS/MS analysis, Ge et al. profiled phosphorylation changes upon bortezomib treatment, and more specifically an increase of Ser38 phosphorylation on stathmin, furthering the understanding of bortezomib-induced apoptosis in myeloma cells.111 In a recent study, Yang et al. employed iTRAQ (isobaric tag for relative and absolute quantitation) mass spectrometry (MS) to compare profiles of protein expression in drug-resistant and sensitive isogenic myeloma cell lines. They observed overexpression of myristolylated alanine-rich C kinase substrate (MARCKS) in drug-resistant cell lines. Bortezomib treatment in MARCKS silence cells overcame bortezomib-resistance, suggesting a new therapeutic target in refractory/relapsed myeloma.112 Using serum samples from MM patients treated with bortezomib, Hsieh et al. identified high expression levels of apolipoprotein C-1 (apoC-1) and its truncated isoform apolipoprotein C-1′ (apoC-1′) in those who did not respond to treatment.113 Comparison between normal and myeloma plasma cells also showed clusters of differentially expressed proteins.114 Exploring potential biomarkers for myeloma bone disease in serum samples, Bhattacharyya et al. identified mass spectral differences discriminating patients with skeletal lytic lesions and those without.115 Furthermore, label-free relative quantitative proteomics enabled our group to identify the association of complement C4 and serum paraoxonase/arylesterase 1 (PON1) with severe bone disease in myeloma.116 Zingone et al. predicted transformation from smouldering myeloma (SMM) to active disease (MM) by observing significant increase in the levels of key cytokines, in particular CXCL8 (IL-8).117
There is now a general agreement that panels of biomarkers or bio signatures are more sensitive and specific than single markers. The use of large panels of biomarkers is less likely to yield unreliable results owing to the substantial heterogeneity among cancers. We reported a set of novel protein biomarkers comprised of zinc-α-2-glycoprotein (ZAG), vitamin D-binding protein (VDB), serum amyloid-A protein (SAA) to predict therapeutic response to thalidomide with associated 81.8% sensitivity and 86.2% specificity.118 By analysing the serum proteomes of responders and non-responders to bortezomib, our group developed a panel of biomarkers including clusterin, angiogenin, complement C1q, albumin, beta-2-microglobulin, M-protein, and kappa/lambda ratio to predict treatment response119 (Table 3).
Table 3 Proteomic-based studies to identify potential prognostic and predictive biomarkers
Study |
Sample type |
Patient number (N) |
Method |
Markers |
N/A: not applicable, 2D-DIGE: 2-dimensional difference gel electrophoresis, MALDI: matrix-assisted laser desorption/ionisation, SELDI: surface-enhanced laser desorption/ionisation, TOF: time-of-flight, LC-MS: liquid chromatography-mass spectrometry, ELISA: enzyme-linked immunosorbent assay, BM: bone marrow. |
Xiao et al. [2009]114 |
Cell lines |
N/A |
2D-DIGE |
43 differentially expressed proteins between normal and myeloma plasma cells |
MALDI-TOF/TOF MS |
Ge et al. [2010]111 |
Cell line |
N/A |
SILAC |
Increased in phosphorylation on stathmin in response to bortezomib treatment |
LC-MS/MS |
Sun et al. [2013]110 |
Cell line |
N/A |
2D-DIGE |
Increased level of PDIA3 and decreased level of CPIN1 in response to bendamustine treatment |
MALDI-TOF/TOF MS |
Yang et al. [2015]112 |
Cell line |
N/A |
iTRAQ |
MARCKS overexpression in drug-resistant myeloma cells |
Bhattacharyya et al. [2006]115 |
Serum |
48 |
SELDI-TOF MS |
Identification of differentially expressed proteins in myeloma bone disease |
Hsieh et al. [2009]113 |
Serum |
10 |
LC-MS |
ApoC-1 and apoC-1′ as early predictors of bortezomib response |
Rajpal et al. [2011]118 |
Serum |
39 |
2D-DIGE |
Biomarker panel (ZAG, VDB, and SAA) to predict response to thalidomide |
LC-MS |
Dowling et al. [2014]116 |
Serum |
111 |
LC-MS |
Complement C4 and PON1 are associated with severe myeloma bone disease |
Zingone et al. [2014]117 |
Serum |
52 |
ELISA |
CXCL8 is associated with disease progression from smouldering to active myeloma |
BM aspirate |
Ting et al. [2017]119 |
Serum |
37 |
LC-MS/MS |
Development of a biomarker panel to predict response to bortezomib |
ELISA |
The role of glycomic biomarkers in myeloma
Glycosylation is an important post translational modification affecting many biological functions. Previous discoveries had shown the utility of glycoproteins in cancer biomarkers, for example Her2/Neu in breast cancer, CA125 in ovarian cancer, carcinoembryonic antigen (CEA) in colorectal cancer, and prostate specific antigen (PSA) in prostate cancer.120 Altered glycosylation pattern on glycoproteins produced by malignant cells had been associated with cancer progression and metastasis.121 This expansion of biomarker discovery highlights the role of glycoproteomics in translational research, with the unique advantage of profiling both the protein and the glycan structures.
Glycosylation research in myeloma, both at the immunoglobulin and cell surface level, has gained momentum in recent years. The pathognomonic monoclonal immunoglobulin is heavily glycosylated, both at the fragment crystallisable region (Fc) and the antigen-binding fragment (Fab). The biantennary N-glycan at the asparagine position 297 in the Fc region lends structural stability and functional capacity to the immunoglobulin. Modification of this crucial N-glycan affects the binding of the immunoglobulin to Fc gamma receptors on effector cells.122 On the other hand, glycosylation at the Fab portion can alter antigen binding, immune complex formation, and half-life extension.123 Glycome profiling of the myeloma immunoglobulin had shown characteristic differences between disease stages. Since the late 1990's, investigations of the N-glycan profile of myeloma IgG revealed similar traits to the polyclonal IgG background, and more specifically, IgG sialylation was elevated in active myeloma compared to MGUS.124,125 Using lectin blotting assay in myeloma patients and healthy controls, Aurer et al. found aberrant galactosylation and sialylation in myeloma IgG heavy chain.126 Employing DNA sequencer-assisted fluorophore-assisted capillary electrophoresis (DSA-FACE) to evaluate for potential biomarkers, Chen et al. was able to profile total serum N-glycans and determine their quantitative abundance in myeloma patients. Elevated level of sialylation and decreased level of core fucosylation were observed in light chain myeloma patients when compared to healthy controls.127 More recently, with a sensitive glycomic platform consisting of hydrophilic interaction UPLC, weak anion exchange chromatography, and mass spectrometry, our group identified differential IgG glycosylation patterns in patients across the plasma cell disorder spectrum, showing transformation from benign to malignant state. The abundances of three afucosyl-biantennary-digalactosylated-sialylated glycan species (A2G2S1, A2BG2S1, and A2BG2S2) decrease across the spectrum from benign MGUS to active myeloma (Fig. 3). Patients in CR had the lowest level of bisecting N-acetylglucosamine in comparison to the highest level in those with relapse disease.128 These findings allude to the possibility that the alteration in glycotraits affect binding and functional capacity of IgG across the spectrum of plasma cell disorder. With the increasing use of immunotherapy in MM treatment, precise regulation of N-glycosylation on the Fc of therapeutic monoclonal IgG (mAb) becomes crucial due to its influence on structure and effector function. Fc N-glycans stabilise the mAb, thus prevent unfolding and aggregation. The antibody-dependent cellular cytotoxicity activity of mAb increases up to 100-fold due to enhanced binding to Fc gamma receptor IIIa when core fucosylation is absence.129 Sialylation at the terminating end of the mAb Fc N-glycans induces an anti-inflammatory effect by increased binding to type II Fc receptor known as dendritic cell-specific intercellular adhesion molecule-3-grabbing non-integrin (DC-SIGN).130
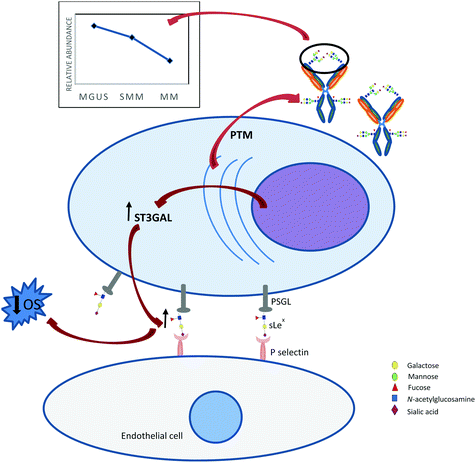 |
| Fig. 3 Glycosylation in myeloma. Sialyl-Lewisx (sLex) on the P selectin glycoprotein ligand 1 (PSGL1) expressed on myeloma cell is the binding site for P selectin. Elevated expression of ST3GAL6 leads to increased level of sLex resulting in a decrease in overall survival. Myeloma IgG is heavily glycosylated at Fc and F(ab′)2 regions after post translational modification (PTM). A reduction in the abundances of afucosyl-sialylated glycans was observed across the MGUS, SMM, and MM groups. | |
The role of glycosylation on myeloma cell surface is also being extensively explored as a potential therapeutic target. It is known that cell surface glycans affect cell adhesion and migration. Changes in cell surface glycosylation are likely involved in tumour cell metastasis, i.e. reduced adhesion at the primary site causing tumour cells migration into the vascular and lymphatic system, or formation of new adhesion processes causing seeding of secondary tumour sites. The metastatic capability of cancer cells is related to the level of cell surface sialylation. Myeloma cells have high expression of P-selectin glycoprotein ligand 1 allowing binding to both E- and P-selectins.131 The main ligand for these selectins is sialyl-Lewisx, characterised by a terminal fucose and a sialic acid residues. Glavey et al. observed high expression of ST3GAL6, the sialyltransferase involved in the synthesis of sialyl-Lewisx, was associated with inferior overall survival in myeloma132 (Fig. 3). Furthermore, they also found that low level of FUCA1 expression, a tissue alpha-L-fucosidase involved in the degradation of fucose-containing glycoproteins, correlated with high level of alpha1,3 and alpha-1,6 linked fucose in myeloma cells, also led to inferior overall survival. The combination of low FUCA1 expression and high ST3GAL6 or ST3GAL1 expression identified a subset of myeloma patients at high risk of early disease progression and poor survival outcome.
Conclusion
For the past four decades, risk-adaptive strategies have evolved alongside treatment for MM. As a heterogeneous disease, MM defies the one-treatment-for-all concept. To improve patient care, we need to risk-stratify patients for outcome prediction and to match the right treatment to the right patient. This personalisation of treatment was initially conceived with the DS staging and ISS, then developed in the era of cytogenetics and FISH, and now growing with GEP and NGS. Furthering the stride in myeloma research, the fields of proteomics and glycomics provide the platforms for discovering potential biomarkers to monitor disease response and predict drug-resistance. The maturation process of personalised MM therapy requires further research and validation. Most importantly, the obvious barriers to the success of personalised therapy are resource allocation and the implementation of new techniques such as GEP, NGS, and proteomic/glycomic biomarkers in clinical practice.
Conflicts of interest
There are no conflicts to declare.
Acknowledgements
G. N. L. acknowledges the funding support from the Irish. Research Council Postgraduate Scholarship (GOIPG/2015/3379) and from the Health Research Board (HPF-2016-1710).
References
- S. Solly, Med. Chir. Trans., 1844, 435–461 CrossRef CAS.
- W. Macintyre, Med. Chir. Trans., 1850, 211–232 CrossRef CAS.
- Y. Hiroshi, T. Ishida and R. Maruyama, Cancer Sci., 2012, 1907–1912 Search PubMed.
- N. Alwall, Lancet, 1947, 388–389 CrossRef CAS.
- N. Blokhin, L. Larionov, N. Pervodchikova, L. Chebotareva and N. Merkulova, Ann. N. Y. Acad. Sci., 1958, 1128–1132 CrossRef CAS.
- A. J. Cowan, C. Allen and A. Barac,
et al.
, JAMA Oncol., 2018, 4, 1221–1227 CrossRef PubMed.
- R. Maas, Cancer Chemother. Rep., 1962, 257–259 Search PubMed.
- R. Alexanian, A. Haut and A. U. Khan,
et al.
, JAMA, 1969, 208, 1680–1685 CrossRef CAS PubMed.
- R. A. Kyle, E. D. Remstein, T. M. Therneau, A. Dispenzieri, P. J. Kurtin, J. M. Hodnefield, D. R. Larson, M. F. Plevak, D. F. Jelinek, R. Fonseca, L. J. Melton and S. V. Rajkumar, N. Engl. J. Med., 2007, 356, 2582–2590 CrossRef CAS PubMed.
- B. G. M. Durie, J. L. Harousseau, J. S. Miguel, J. Bladé, B. Barlogie, K. Anderson, M. Gertz, M. Dimopoulos, J. Westin, P. Sonneveld, H. Ludwig, G. Gahrton, M. Beksac, J. Crowley, A. Belch, M. Boccadaro, I. Turesson, D. Joshua, D. Vesole, R. Kyle, R. Alexanian, G. Tricot, M. Attal, G. Merlini, R. Powles, P. Richardson, K. Shimizu, P. Tosi, G. Morgan and S. V. Rajkumar, Leukemia, 2006, 20, 1467 CrossRef CAS PubMed.
- S. V. Rajkumar, J.-L. Harousseau, B. Durie, K. C. Anderson, M. Dimopoulos, R. Kyle, J. Blade, P. Richardson, R. Orlowski, D. Siegel, S. Jagannath, T. Facon, H. Avet-Loiseau, S. Lonial, A. Palumbo, J. Zonder, H. Ludwig, D. Vesole, O. Sezer, N. C. Munshi and J. San Miguel, Blood, 2011, 117, 4691–4695 CrossRef CAS PubMed.
- B. Barlogie, L. Smith and R. Alexanian, N. Engl. J. Med., 1984, 310, 1353–1356 CrossRef CAS PubMed.
- T. McElwain and R. Powles, Lancet, 1983, 822–824 CrossRef CAS.
- S. Singhal, J. Mehta, R. Desikan, D. Ayers, P. Roberson, P. Eddlemon, N. Munshi, E. Anaissie, C. Wilson, M. Dhodapkar, J. Zeldis, D. Siegel, J. Crowley and B. Barlogie, N. Engl. J. Med., 1999, 341, 1565–1571 CrossRef CAS PubMed.
- R. Z. Orlowski, T. E. Stinchcombe, B. S. Mitchell, T. C. Shea, A. S. Baldwin, S. Stahl, J. Adams, D.-L. Esseltine, P. J. Elliott, C. S. Pien, R. Guerciolini, J. K. Anderson, N. D. Depcik-Smith, R. Bhagat, M. J. Lehman, S. C. Novick, O. A. O’Connor and S. L. Soignet, J. Clin. Oncol., 2002, 20, 4420–4427 CrossRef CAS PubMed.
- D. E. Bergsagel, C. C. Sprague, C. Austin and K. M. Griffith, Cancer Chemother. Rep., 1962, 21, 87 CAS.
- P. G. Richardson, R. L. Schlossman, E. Weller, T. Hideshima, C. Mitsiades, F. Davies, R. LeBlanc, L. P. Catley, D. Doss, K. Kelly, M. McKenney, J. Mechlowicz, A. Freeman, R. Deocampo, R. Rich, J. J. Ryoo, D. Chauhan, K. Balinski, J. Zeldis and K. C. Anderson, Blood, 2002, 100, 3063–3067 CrossRef CAS PubMed.
- R. Alexanian, D. E. Bergsagel, P. J. Migliore, W. K. Vaughn and C. D. Howe, Blood, 1968, 31, 1–10 CAS.
- S. A. Schey, P. Fields, J. B. Bartlett, I. A. Clarke, G. Ashan, R. D. Knight, M. Streetly and A. G. Dalgleish, J. Clin. Oncol., 2004, 22, 3269–3276 CrossRef CAS PubMed.
- O. A. Connor, A. K. Stewart, M. Vallone, C. J. Molineaux, L. A. Kunkel, J. F. Gerecitano and R. Z. Orlowski, Clin. Cancer Res., 2009, 15, 7085 CrossRef PubMed.
- J. A. Zonder, A. F. Mohrbacher, S. Singhal, F. van Rhee, W. I. Bensinger, H. Ding, J. Fry, D. E. H. Afar and A. K. Singhal, Blood, 2012, 120, 552 CrossRef CAS PubMed.
-
T. Plesner, H. Lokhorst, P. Gimsing, H. Nahi, S. Lisby and P. G. Richardson American Society Haematology Annual Meeting, 9 December, 2012.
- B. Barlogie, R. Hall, A. Zander, K. Dicke and R. Alexanian, Blood, 1986, 67, 1298 CAS.
- B. Barlogie, R. Alexanian, K. A. Dicke, G. Zagars, G. Spitzer, S. Jagannath and L. Horwitz, Blood, 1987, 70, 869 CAS.
- B. Barlogie and G. Gahrton, Bone Marrow Transplant., 1991, 7, 71–79 CAS.
- M. Attal, J. L. Harousseau, A. M. Stoppa, J. J. Otto, J. G. Fuzibet, J. F. Rossi, P. Casassus, H. Maisonneuve, T. Facon, N. Ifrah, C. Payen, R. Bataille and i. F. d. Myelome, N. Engl. J. Med., 1996, 335, 91–97 CrossRef CAS.
- M. Attal, V. Lauwers-Cances, C. Hulin, X. Leleu, D. Caillot, M. Escoffre, B. Arnulf, M. Macro, K. Belhadj, L. Garderet, M. Roussel, C. Payen, C. Mathiot, J. P. Fermand, N. Meuleman, S. Rollet, M. E. Maglio, A. A. Zeytoonjian, E. A. Weller, N. Munshi, K. C. Anderson, P. G. Richardson, T. Facon, H. Avet-Loiseau, J.-L. Harousseau and P. Moreau, N. Engl. J. Med., 2017, 376, 1311–1320 CrossRef CAS PubMed.
- B. G. M. Durie, A. Hoering, M. H. Abidi, S. V. Rajkumar, J. Epstein, S. P. Kahanic, M. Thakuri, F. Reu, C. M. Reynolds, R. Sexton, R. Z. Orlowski, B. Barlogie and A. Dispenzieri, Lancet, 2017, 389, 519–527 CrossRef CAS.
- S. V. Rajkumar, Am. J. Hematol., 2016, 91, 719–734 CrossRef CAS PubMed.
- P. G. Richardson, D. S. Siegel, R. Vij, C. C. Hofmeister, R. Baz, S. Jagannath, C. Chen, S. Lonial, A. Jakubowiak, N. Bahlis, K. Song, A. Belch, N. Raje, C. Shustik, S. Lentzsch, M. Lacy, J. Mikhael, J. Matous, D. Vesole, M. Chen, M. H. Zaki, C. Jacques, Z. Yu and K. C. Anderson, Blood, 2014, 123, 1826 CrossRef CAS PubMed.
- M. Q. Lacy, S. R. Hayman, M. A. Gertz, A. Dispenzieri, F. Buadi, S. Kumar, P. R. Greipp, J. A. Lust, S. J. Russell, D. Dingli, R. A. Kyle, R. Fonseca, P. L. Bergsagel, V. Roy, J. R. Mikhael, A. K. Stewart, K. Laumann, J. B. Allred, S. J. Mandrekar and S. V. Rajkumar, J. Clin. Oncol., 2009, 27, 5008–5014 CrossRef CAS PubMed.
- M. Q. Lacy, J. B. Allred, M. A. Gertz, S. R. Hayman, K. D. Short, F. Buadi, A. Dispenzieri, S. Kumar, P. R. Greipp, J. A. Lust, S. J. Russell, D. Dingli, S. Zeldenrust, R. Fonseca, P. L. Bergsagel, V. Roy, A. K. Stewart, K. Laumann, S. J. Mandrekar, C. Reeder, S. V. Rajkumar and J. R. Mikhael, Blood, 2011, 118, 2970 CrossRef CAS PubMed.
- A. K. Stewart, S. V. Rajkumar, M. A. Dimopoulos, T. Masszi, I. Špička, A. Oriol, R. Hájek, L. Rosiñol, D. S. Siegel, G. G. Mihaylov, V. Goranova-Marinova, P. Rajnics, A. Suvorov, R. Niesvizky, A. J. Jakubowiak, J. F. San-Miguel, H. Ludwig, M. Wang, V. Maisnar, J. Minarik, W. I. Bensinger, M.-V. Mateos, D. Ben-Yehuda, V. Kukreti, N. Zojwalla, M. E. Tonda, X. Yang, B. Xing, P. Moreau and A. Palumbo, N. Engl. J. Med., 2015, 372, 142–152 CrossRef PubMed.
- S. Lonial, M. Dimopoulos, A. Palumbo, D. White, S. Grosicki, I. Spicka, A. Walter-Croneck, P. Moreau, M.-V. Mateos, H. Magen, A. Belch, D. Reece, M. Beksac, A. Spencer, H. Oakervee, R. Z. Orlowski, M. Taniwaki, C. Röllig, H. Einsele, K. L. Wu, A. Singhal, J. San-Miguel, M. Matsumoto, J. Katz, E. Bleickardt, V. Poulart, K. C. Anderson and P. Richardson, N. Engl. J. Med., 2015, 373, 621–631 CrossRef CAS PubMed.
- S. Lonial, B. Weiss, S. Usmani, S. Singhal, A. Chari, N. Bahlis, A. Belch, A. Krishnan, R. Vescio, M.-V. Mateos, A. Mazumder, R. Orlowski, H. J. Sutherland, J. Blade, E. C. Scott, H. Feng, I. Khan, C. M. Uhlar, T. Ahmadi and P. M. Voorhees, J. Clin. Oncol., 2015, LBA8512 Search PubMed.
- L. Sanchez, Y. Wang, D. S. Siegel and M. L. Wang, J. Hematol. Oncol., 2016, 9, 51 CrossRef PubMed.
-
K. C. Anderson, Controversies and updates in multiple myeloma, 2011, pp. 184–190 Search PubMed.
- W. J. Chng, A. Dispenzieri, C. S. Chim, R. Fonseca, H. Goldschmidt, S. Lentzsch, N. Munshi, A. Palumbo, J. S. Miguel, P. Sonneveld, M. Cavo, S. Usmani, B. G. M. Durie, H. Avet-Loiseau, G. Int Myeloma Working and G. International Myeloma Working, Leukemia, 2014, 28, 269–277 CrossRef CAS PubMed.
- B. Durie and S. E. Salmon, Cancer, 1975, 36, 842–854 CrossRef CAS PubMed.
- P. R. Greipp, J. San Miguel, B. G. Durie, J. J. Crowley, B. Barlogie, J. Blade, M. Boccadoro, J. A. Child, H. Avet-Loiseau, R. A. Kyle, J. J. Lahuerta, H. Ludwig, G. Morgan, R. Powles, K. Shimizu, C. Shustik, P. Sonneveld, P. Tosi, I. Turesson and J. Westin, J. Clin. Oncol., 2005, 23, 3412–3420 CrossRef PubMed.
- A. Palumbo, H. Avet-Loiseau, S. Oliva, H. M. Lokhorst, H. Goldschmidt, L. Rosinol, P. Richardson, S. Caltagirone, J. J. Lahuerta, T. Facon, S. Bringhen, F. Gay, M. Attal, R. Passera, A. Spencer, M. Offidani, S. Kumar, P. Musto, S. Lonial, M. T. Petrucci, R. Z. Orlowski, E. Zamagni, G. Morgan, M. A. Dimopoulos, B. G. M. Durie, K. C. Anderson, P. Sonneveld, J. San Miguel, M. Cavo, S. V. Rajkumar and P. Moreau, J. Clin. Oncol., 2015, 33, 2863–2869 CrossRef CAS PubMed.
- N. C. Munshi, K. C. Anderson, P. L. Bergsagel, J. Shaughnessy, A. Palumbo, B. Durie, R. Fonseca, A. K. Stewart, J. L. Harousseau, M. Dimopoulos, S. Jagannath, R. Hajek, O. Sezer, R. Kyle, P. Sonneveld, M. Cavo, S. V. Rajkumar, J. San Miguel, J. Crowley, H. Avet-Loiseau and P. International Myeloma Workshop Consensus, Blood, 2011, 117, 4696–4700 CrossRef CAS PubMed.
- A. M. Rajan and S. V. Rajkumar, Blood Cancer J., 2015, 5, e365 CrossRef CAS PubMed.
- S. V. Rajkumar, Am. J. Hematol., 2016, 91, 90–100 CrossRef CAS PubMed.
- P. Sonneveld, H. Avet-Loiseau, S. Lonial, S. Usmani, D. Siegel, K. C. Anderson, W.-J. Chng, P. Moreau, M. Attal, R. A. Kyle, J. Caers, J. Hillengass, J. San Miguel, N. W. C. J. van de Donk, H. Einsele, J. Bladé, B. G. M. Durie, H. Goldschmidt, M.-V. Mateos, A. Palumbo and R. Orlowski, Blood, 2016, 127, 2955–2962 CrossRef CAS PubMed.
- P. G. Richardson, E. Weller, S. Lonial, A. J. Jakubowiak, S. Jagannath, N. S. Raje, D. E. Avigan, W. Xie, I. M. Ghobrial, R. L. Schlossman, A. Mazumder, N. C. Munshi, D. H. Vesole, R. Joyce, J. L. Kaufman, D. Doss, D. L. Warren, L. E. Lunde, S. Kaster, C. DeLaney, T. Hideshima, C. S. Mitsiades, R. Knight, D.-L. Esseltine and K. C. Anderson, Blood, 2010, 116, 679 CrossRef CAS PubMed.
- M. Cavo, H. Salwender, L. Rosinol, P. Moreau, M. T. Petrucci, I. W. Blau, J. Blade, M. Attal, F. Patriarca, K. Weisel, J. F. San Miguel, H. Avet-Loiseau, N. Testoni, M. Pfreundschuh, J. Lahuerta, T. Facon, L. Pantani, C. Scheid, N. Gutierrez, G. Marit, A. Palumbo, M. L. Martin, D. Caillot and H. Goldschmidt, Blood, 2013, 122(21) Search PubMed , abstract 767.
- J. R. Mikhael, D. Dingli, V. Roy, C. B. Reeder, F. K. Buadi, S. R. Hayman, A. Dispenzieri, R. Fonseca, T. Sher, R. A. Kyle, Y. Lin, S. J. Russell, S. Kumar, P. L. Bergsagel, S. R. Zeldenrust, N. Leung, M. T. Drake, P. Kapoor, S. M. Ansell, T. E. Witzig, J. A. Lust, R. J. Dalton, M. A. Gertz, A. K. Stewart, S. V. Rajkumar, A. Chanan-Khan, M. Q. Lacy and C. Mayo, Mayo Clin. Proc., 2013, 88, 360–376 CrossRef PubMed.
- M. Cavo, L. Pantani, M. T. Petrucci, F. Patriarca, E. Zamagni, D. Donnarumma, C. Crippa, M. Boccadoro, G. Perrone, A. Falcone, C. Nozzoli, R. Zambello, L. Masini, A. Furlan, A. Brioli, D. Derudas, S. Ballanti, M. L. Dessanti, V. De Stefano, A. M. Carella, M. Marcatti, A. Nozza, F. Ferrara, V. Callea, C. Califano, A. Pezzi, A. Baraldi, M. Grasso, P. Musto and A. Palumbo, Blood, 2012, 120, 9 CrossRef CAS PubMed.
- S. Jagannath, P. G. Richardson, P. Sonneveld, M. W. Schuster, D. Irwin, E. A. Stadtmauer, T. Facon, J. L. Harousseau, J. M. Cowan and K. C. Anderson, Leukemia, 2006, 21, 151–157 CrossRef PubMed.
- R. Fonseca, P. L. Bergsagel, J. Drach, J. Shaughnessy, N. Gutierrez, A. K. Stewart, G. Morgan, B. Van Ness, M. Chesi, S. Minvielle, A. Neri, B. Barlogie, W. M. Kuehl, P. Liebisch, F. Davies, S. Chen-Kiang, B. G. Durie, R. Carrasco, O. Sezer, T. Reiman, L. Pilarski, H. Avet-Loiseau and G. International Myeloma Working, Leukemia, 2009, 23, 2210–2221 CrossRef CAS PubMed.
- A. Kalff and A. Spencer, Blood Cancer J., 2012, 2, e89 CrossRef CAS PubMed.
- M. Santra, F. Zhan, E. Tian, B. Barlogie and J. Shaughnessy, Blood, 2003, 101, 2374 CrossRef CAS.
- J. J. Keats, C. A. Maxwell, B. J. Taylor, M. J. Hendzel, M. Chesi, P. L. Bergsagel, L. M. Larratt, M. J. Mant, T. Reiman, A. R. Belch and L. M. Pilarski, Blood, 2005, 105, 4060 CrossRef CAS PubMed.
- Z. Li, Y. X. Zhu, E. E. Plowright, P. L. Bergsagel, M. Chesi, B. Patterson, T. S. Hawley, R. G. Hawley and A. K. Stewart, Blood, 2001, 97, 2413 CrossRef CAS.
- J. Qing, X. Du, Y. Chen, P. Chan, H. Li, P. Wu, S. Marsters, S. Stawicki, J. Tien, K. Totpal, S. Ross, S. Stinson, D. Dornan, D. French, Q.-R. Wang, J.-P. Stephan, Y. Wu, C. Wiesmann and A. Ashkenazi, J. Clin. Invest., 2009, 119, 1216–1229 CrossRef CAS.
- W. Jaksic, S. Trudel, H. Chang, Y. Trieu, X. Qi, J. Mikhael, D. Reece, C. Chen and A. K. Stewart, J. Clin. Oncol., 2005, 23, 7069–7073 CrossRef CAS PubMed.
- P. Sonneveld, I. G. H. Schmidt-Wolf, B. van der Holt, L. el Jarari, U. Bertsch, H. Salwender, S. Zweegman, E. Vellenga, A. Broyl, I. W. Blau, K. C. Weisel, S. Wittebol, G. M. J. Bos, M. Stevens-Kroef, C. Scheid, M. Pfreundschuh, D. Hose, A. Jauch, H. van der Velde, R. Raymakers, M. R. Schaafsma, M.-J. Kersten, M. van Marwijk-Kooy, U. Duehrsen, W. Lindemann, P. W. Wijermans, H. M. Lokhorst and H. M. Goldschmidt, J. Clin. Oncol., 2012, 30, 2946–2955 CrossRef CAS.
- F. M. Ross, L. Chiecchio, G. Dagrada, R. K. Protheroe, D. M. Stockley, C. J. Harrison, N. C. Cross, A. J. Szubert, M. T. Drayson, G. J. Morgan and U. K. M. Forum, Haematologica, 2010, 95, 1221–1225 CrossRef PubMed.
- S. Pandey, S. V. Rajkumar, P. Kapoor, R. P. Ketterling, M. Q. Lacy, M. A. Gertz, F. Buadi, D. Dingli, S. R. Hayman, A. Dispenzieri and S. K. Kumar, Blood, 2013, 122, 3210 CrossRef.
- T. Vu, W. Gonsalves, S. Kumar, A. Dispenzieri, M. Q. Lacy, F. Buadi, M. A. Gertz and S. V. Rajkumar, Blood Cancer J., 2015, 5, e363 CrossRef CAS PubMed.
- R. Fonseca, S. A. Van Wier, W. J. Chng, R. Ketterling, M. Q. Lacy, A. Dispenzieri, P. L. Bergsagel, S. V. Rajkumar, P. R. Greipp, M. R. Litzow, T. Price-Troska, K. J. Henderson, G. J. Ahmann and M. A. Gertz, Leukemia, 2006, 20, 2034–2040 CrossRef CAS PubMed.
- I. Hanamura, J. P. Stewart, Y. Huang, F. Zhan, M. Santra, J. R. Sawyer, K. Hollmig, M. Zangarri, M. Pineda-Roman, F. van Rhee, F. Cavallo, B. Burington, J. Crowley, G. Tricot, B. Barlogie and J. D. Shaughnessy, Blood, 2006, 108, 1724 CrossRef CAS PubMed.
- H. Chang, C. Qi, Q.-L. Yi, D. Reece and A. K. Stewart, Blood, 2004, 105, 358 CrossRef PubMed.
- H. Avet-Loiseau, X. Leleu, M. Roussel, P. Moreau, C. Guerin-Charbonnel, D. Caillot, G. Marit, L. Benboubker, L. Voillat, C. Mathiot, B. Kolb, M. Macro, L. Campion, M. Wetterwald, A.-M. Stoppa, C. Hulin, T. Facon, M. Attal, S. Minvielle and J.-L. Harousseau, J. Clin. Oncol., 2010, 28, 4630–4634 CrossRef CAS PubMed.
- L. Rosiñol, A. Oriol, A. I. Teruel, D. Hernández, J. López-Jiménez, J. de la Rubia, M. Granell, J. Besalduch, L. Palomera, Y. González, M. A. Etxebeste, J. Díaz-Mediavilla, M. T. Hernández, F. de Arriba, N. C. Gutiérrez, M. L. Martín-Ramos, M. T. Cibeira, M. V. Mateos, J. Martínez, A. Alegre, J. J. Lahuerta, J. San Miguel and J. Bladé, Blood, 2012, 120, 1589 CrossRef PubMed.
- M. Roussel, V. Lauwers-Cances, N. Robillard, C. Hulin, X. Leleu, L. Benboubker, G. Marit, P. Moreau, B. Pegourie, D. Caillot, C. Fruchart, A.-M. Stoppa, C. Gentil, S. Wuilleme, A. Huynh, B. Hebraud, J. Corre, M.-L. Chretien, T. Facon, H. Avet-Loiseau and M. Attal, J. Clin. Oncol., 2014, 32, 2712–2717 CrossRef CAS PubMed.
- P. Sonneveld, E. Asselbergs, S. Zweegman, B. van der Holt, M. J. Kersten, E. Vellenga, M. van Marwijk-Kooy, A. Broyl, O. de Weerdt, S. Lonergan, A. Palumbo and H. Lokhorst, Blood, 2015, 125, 449 CrossRef CAS PubMed.
- A. J. Jakubowiak, D. Dytfeld, K. A. Griffith, D. Lebovic, D. H. Vesole, S. Jagannath, A. Al-Zoubi, T. Anderson, B. Nordgren, K. Detweiler-Short, K. Stockerl-Goldstein, A. Ahmed, T. Jobkar, D. E. Durecki, K. McDonnell, M. Mietzel, D. Couriel, M. Kaminski and R. Vij, Blood, 2012, 120, 1801 CrossRef CAS PubMed.
- X. Leleu, L. Karlin, M. Macro, C. Hulin, L. Garderet, M. Roussel, B. Arnulf, B. Pegourie, B. Kolb, A. M. Stoppa, S. Brechiniac, G. Marit, B. Thielemans, B. Onraed, C. Mathiot, A. Banos, L. Lacotte, M. Tiab, M. Dib, J.-G. Fuzibet, M. O. Petillon, P. Rodon, M. Wetterwald, B. Royer, L. Legros, L. Benboubker, O. Decaux, M. Escoffre-Barbe, D. Caillot, J. P. Fermand, P. Moreau, M. Attal, H. Avet-Loiseau and T. Facon, Blood, 2015, 125, 1411 CrossRef CAS PubMed.
- J. J. Shah, E. A. Stadtmauer, R. Abonour, A. D. Cohen, W. I. Bensinger, C. Gasparetto, J. L. Kaufman, S. Lentzsch, D. T. Vogl, C. L. Gomes, N. Pascucci, D. D. Smith, R. Z. Orlowski and B. G. M. Durie, Blood, 2015, 126, 2284 CrossRef CAS PubMed.
- Z. N. Özkurt, G. T. Sucak, Ş. Z. Akı, M. Yağcı and R. Haznedar, Cancer Invest., 2017, 35, 195–201 CrossRef PubMed.
- S. Kumar, L. Zhang, A. Dispenzieri, S. Van Wier, J. A. Katzmann, M. Snyder, E. Blood, R. DeGoey, K. Henderson, R. A. Kyle, A. R. Bradwell, P. R. Greipp, S. V. Rajkumar and R. Fonseca, Leukemia, 2010, 24, 1498–1505 CrossRef CAS PubMed.
- A. Brioli, H. Giles, C. Pawlyn, J. P. Campbell, M. F. Kaiser, L. Melchor, G. H. Jackson, W. M. Gregory, R. G. Owen, J. A. Child, F. E. Davies, M. Cavo, M. T. Drayson and G. J. Morgan, Blood, 2014, 123, 3414 CrossRef CAS PubMed.
- Y. Meddour, M. Rahali, S. Belakehal, F. Ardjoun, S. Chaib and R. Djidjik, Clin. Lab., 2018, 64, 551–558 Search PubMed.
- S. V. Rajkumar, O. Landgren and M.-V. Mateos, Blood, 2015, 125, 3069 CrossRef CAS PubMed.
- S. V. Rajkumar, V. Gupta, R. Fonseca, A. Dispenzieri, W. I. Gonsalves, D. Larson, R. P. Ketterling, J. A. Lust, R. A. Kyle and S. K. Kumar, Leukemia, 2013, 27, 1738–1744 CrossRef CAS PubMed.
- P. Moreau and S. Minvielle, Haematologica, 2013, 98, 487–489 CrossRef PubMed.
- F. Zhan, Y. Huang, S. Colla, J. P. Stewart, I. Hanamura, S. Gupta, J. Epstein, S. Yaccoby, J. Sawyer, B. Burington, E. Anaissie, K. Hollmig, M. Pineda-Roman, G. Tricot, F. van Rhee, R. Walker, M. Zangari, J. Crowley, B. Barlogie and J. D. Shaughnessy Jr., Blood, 2006, 108, 2020–2028 CrossRef CAS PubMed.
- J. D. Shaughnessy Jr., F. Zhan, B. E. Burington, Y. Huang, S. Colla, I. Hanamura, J. P. Stewart, B. Kordsmeier, C. Randolph, D. R. Williams, Y. Xiao, H. Xu, J. Epstein, E. Anaissie, S. G. Krishna, M. Cottler-Fox, K. Hollmig, A. Mohiuddin, M. Pineda-Roman, G. Tricot, F. van Rhee, J. Sawyer, Y. Alsayed, R. Walker, M. Zangari, J. Crowley and B. Barlogie, Blood, 2007, 109, 2276–2284 CrossRef PubMed.
- O. Decaux, L. Lode, F. Magrangeas, C. Charbonnel, W. Gouraud, P. Jezequel, M. Attal, J. L. Harousseau, P. Moreau, R. Bataille, L. Campion, H. Avet-Loiseau, S. Minvielle and M. Intergroupe Francophone du, J. Clin. Oncol., 2008, 26, 4798–4805 CrossRef CAS PubMed.
- N. J. Dickens, B. A. Walker, P. E. Leone, D. C. Johnson, J. L. Brito, A. Zeisig, M. W. Jenner, K. D. Boyd, D. Gonzalez, W. M. Gregory, F. M. Ross, F. E. Davies and G. J. Morgan, Clin. Cancer Res., 2010, 16, 1856–1864 CrossRef CAS PubMed.
- D. Hose, T. Rème, T. Hielscher, J. Moreaux, T. Messner, A. Seckinger, A. Benner, J. D. Shaughnessy, B. Barlogie, Y. Zhou, J. Hillengass, U. Bertsch, K. Neben, T. Möhler, J. F. Rossi, A. Jauch, B. Klein and H. Goldschmidt, Haematologica, 2011, 96, 87–95 CrossRef PubMed.
- T. Reme, D. Hose, C. Theillet and B. Klein, Bioinformatics, 2013, 29, 1149–1157 CrossRef CAS PubMed.
- R. Kuiper, A. Broyl, Y. De Knegt, M. H. Van Vliet, E. H. Van Beers, B. Van Der Holt, L. El Jarari, G. Mulligan, W. Gregory, G. Morgan, H. Goldschmidt, H. M. Lokhorst, M. Van Duin and P. Sonneveld, Leukemia, 2012, 26, 2406–2413 CrossRef CAS PubMed.
- R. Kuiper, M. van Duin, M. H. van Vliet, A. Broijl, B. van der Holt, L. el Jarari, E. H. van Beers, G. Mulligan, H. Avet-Loiseau, W. M. Gregory, G. Morgan, H. Goldschmidt, H. M. Lokhorst and P. Sonneveld, Blood, 2015, 126, 1996 CrossRef CAS PubMed.
- T. MeiBner, A. Seckinger, T. Reme, T. Hielscher, T. Mohler, K. Neben, H. Goldschmidt, B. Klein and D. Hose, Clin. Cancer Res., 2011, 17, 7240–7247 CrossRef PubMed.
- M. Bhutani, Q. Zhang, R. Friend, P. M. Voorhees, L. J. Druhan, B. Barlogie, P. Sonneveld, G. J. Morgan, J. T. Symanowski, B. R. Avalos, E. A. Copelan and S. Z. Usmani, Lancet Haematol., 2017, 4, e443–e451 CrossRef.
- C. Terragna, M. Renzulli, D. Remondini, E. Tagliafico, F. Di Raimondo, F. Patriarca, G. Martinelli, E. Roncaglia, L. Masini, P. Tosi, E. Zamagni, P. Tacchetti, A. Ledda, A. Brioli, E. Angelucci, N. Testoni, G. Marzocchi, P. Galieni, A. Gozzetti, M. Martello, F. Dico, K. Mancuso and M. Cavo, Ann. Hematol., 2013, 92, 1271–1280 CrossRef CAS PubMed.
- H. A. F. Stessman, L. B. Baughn, A. Sarver, T. Xia, R. Deshpande, A. Mansoor, S. A. Walsh, J. J. Sunderland, N. G. Dolloff, M. A. Linden, F. Zhan, S. Janz, C. L. Myers and B. G. Van Ness, Mol. Cancer Ther., 2013, 12, 1140 CrossRef CAS PubMed.
- J. D. Shaughnessy, P. Qu, S. Usmani, C. J. Heuck, Q. Zhang, Y. Zhou, E. Tian, I. Hanamura, F. van Rhee, E. Anaissie, J. Epstein, B. Nair, O. Stephens, R. Williams, S. Waheed, Y. Alsayed, J. Crowley and B. Barlogie, Blood, 2011, 118, 3512 CrossRef CAS PubMed.
- N. Deng, H. Zhou, H. Fan and Y. Yuan, Oncotarget, 2017, 8, 110635 Search PubMed.
- T. Bagratuni, T. Evangelos, E.-P. Evangelos, K. Despoina, G. Maria, M. Magdalini, L. Christine-Ivy, T. Anna, M. Charis, M. Despoina, M. A. Dimopoulos and K. Efstathios, Br. J. Haematol., 2016, 172, 44–47 CrossRef CAS PubMed.
- X.-Y. Qin, J. Lu, G.-X. Li, L. Wen, Y. Liu, L.-P. Xu, Y.-J. Chang, K.-Y. Liu, Z.-F. Jiang and X.-J. Huang, Ann. Hematol., 2018, 97, 485–495 CrossRef CAS PubMed.
- Y. Chou, C. Yang, H. Chen, S. Yang, Y. Yu, Y. Hong, C. Liu, J. Gau, J. Liu, P. Chen, T. Chiou, C. Tzeng and L. Hsiao, Eur. J. Haematol., 2012, 89, 320–327 CrossRef CAS PubMed.
- A. Grimberg, Cancer Biol. Ther., 2003, 2, 630–635 CAS.
- A. C. Sprynski, D. Hose, A. Kassambara, L. Vincent, M. Jourdan, J. F. Rossi, H. Goldschmidt and B. Klein, Leukemia, 2010, 24, 1940 CrossRef CAS PubMed.
- R. Ríos-Tamayo, C. B. Lupiañez, D. Campa, T. Hielscher, N. Weinhold, J. Martínez-López, A. Jerez, S. Landi, K. Jamroziak, C. Dumontet, M. Wątek, F. Lesueur, R. M. Reis, H. Marques, A. Jurczyszyn, U. Vogel, G. Buda, R. García-Sanz, E. Orciuolo, M. Petrini, A. J. Vangsted, F. Gemignani, A. Försti, H. Goldschmidt, K. Hemminki, F. Canzian, M. Jurado and J. Sainz, Oncotarget, 2016, 7, 59029–59048 CrossRef PubMed.
- K. R. Nielsen, M. Rodrigo-Domingo, R. Steffensen, J. Baech, K. S. Bergkvist, L. Oosterhof, A. Schmitz, J. S. Bødker, P. Johansen, U. Vogel, A. Vangsted, K. Dybkær, M. Bøgsted and H. E. Johnsen, Leuk. Lymphoma, 2017, 58, 2695–2704 CrossRef CAS PubMed.
- B. A. Walker, E. M. Boyle, C. P. Wardell, A. Murison, D. B. Begum, N. M. Dahir, P. Z. Proszek, D. C. Johnson, M. F. Kaiser, L. Melchor, L. I. Aronson, M. Scales, C. Pawlyn, F. Mirabella, J. R. Jones, A. Brioli, A. Mikulasova, D. A. Cairns, W. M. Gregory, A. Quartilho, M. T. Drayson, N. Russell, G. Cook, G. H. Jackson, X. Leleu, F. E. Davies and G. J. Morgan, J. Clin. Oncol., 2015, 33, 3911–3920 CrossRef CAS PubMed.
- J. G. Lohr, P. Stojanov, S. L. Carter, P. Cruz-Gordillo, M. S. Lawrence, D. Auclair, C. Sougnez, B. Knoechel, J. Gould, G. Saksena, K. Cibulskis, A. McKenna, M. A. Chapman, R. Straussman, J. Levy, L. M. Perkins, J. J. Keats, S. E. Schumacher, M. Rosenberg, G. Getz and T. R. Golub, Cancer Cell, 2014, 25, 91–101 CrossRef CAS PubMed.
- Y. Mishima, B. Paiva, J. Shi, J. Park, S. Manier, S. Takagi, M. Massoud, A. Perilla-Glen, Y. Aljawai, D. Huynh, A. M. Roccaro, A. Sacco, M. Capelletti, A. Detappe, D. Alignani, K. C. Anderson, N. C. Munshi, F. Prosper, J. G. Lohr, G. Ha, S. S. Freeman, E. M. Van Allen, V. A. Adalsteinsson, F. Michor, J. F. San Miguel and I. M. Ghobrial, Cell Rep., 2017, 19, 218–224 CrossRef CAS PubMed.
-
M. Bustoros, T. H. Mouhieddine, A. Detappe and I. M. Ghobrial, ASCO Education Book, 2017 Search PubMed.
- J. P. Sharman, J. Chmielecki, D. Morosini, G. A. Palmer, J. S. Ross, P. J. Stephens, J. Stafl, V. A. Miller and S. M. Ali, Clin. Lymphoma, Myeloma Leuk., 2014, 14, e161–e163 CrossRef CAS PubMed.
- M. Andrulis, N. Lehners, D. Capper, R. Penzel, C. Heining, J. Huellein, T. Zenz, A. von Deimling, P. Schirmacher, A. D. Ho, H. Goldschmidt, K. Neben and M. S. Raab, Cancer Discovery, 2013, 3, 862 CrossRef CAS PubMed.
- P. H. Hoang, S. E. Dobbins, A. J. Cornish, D. Chubb, P. J. Law, M. Kaiser and R. S. Houlston, Leukemia, 2018, 32, 2459–2470 CrossRef CAS PubMed.
- K. M. Kortuem, E. Braggio, L. Bruins, S. Barrio, C. S. Shi, Y. X. Zhu, R. Tibes, D. Viswanatha, P. Votruba, G. Ahmann, R. Fonseca, P. Jedlowski, I. Schlam, S. Kumar, P. L. Bergsagel and A. K. Stewart, Blood Cancer J., 2016, 6, e397 CrossRef CAS PubMed.
- J. Cumova, A. Potacova, Z. Zdrahal and R. Hajek, Mol. Biotechnol., 2011, 47, 83–93 CrossRef CAS PubMed.
- G. Feng, B. Li-Jun, T. Sheng-Ce, X. Xu-dong, Z. Zhi-Ping, K. Kaio and Z. Xian-En, Proteomics: Clin. Appl., 2011, 5, 30–37 Search PubMed.
- C. Sun, J. Li, J. Gu, J. Liu and B. Huang, J. BUON, 2013, 18, 996–1005 Search PubMed.
- F. Ge, C.-L. Xiao, L.-J. Bi, S.-C. Tao, S. Xiong, X.-F. Yin, L.-P. Li, C.-H. Lu, H.-T. Jia and Q.-Y. He, PLoS One, 2010, 5, e13095 CrossRef PubMed.
- Y. Yang, Y. Chen, M. N. Saha, J. Chen, K. Evans, L. Qiu, D. Reece, G. A. Chen and H. Chang, Leukemia, 2015, 29, 715–726 CrossRef CAS PubMed.
- F. Y. Hsieh, E. Tengstrand, T. M. Pekol, R. Guerciolini and G. Miwa, J. Pharm. Biomed. Anal., 2009, 49, 115–122 CrossRef CAS PubMed.
- C.-L. Xiao, Z.-P. Zhang, S. Xiong, C.-H. Lu, H.-P. Wei, H.-L. Zeng, Z. Liu, X.-E. Zhang and F. Ge, Proteomics: Clin. Appl., 2009, 3, 1348–1360 CAS.
- S. Bhattacharyya, J. Epstein and L. J. Suva, Dis. Markers, 2006, 22, 245–255 CrossRef CAS PubMed.
- P. Dowling, C. Hayes, K. R. Ting, A. Hameed, J. Meiller, C. Mitsiades, K. C. Anderson, M. Clynes, C. Clarke, P. Richardson and P. O’Gorman, BMC Genomics, 2014, 15, 1–12 CrossRef PubMed.
- A. Zingone, W. Wang, M. Corrigan-Cummins, S. P. Wu, R. Plyler, N. Korde, M. Kwok, E. E. Manasanch, N. Tageja, M. Bhutani, M. Mulquin, D. Zuchlinski, M. A. Yancey, M. Roschewski, Y. Zhang, A. M. Roccaro, I. M. Ghobrial, K. R. Calvo and O. Landgren, Cytokine, 2014, 69, 294–297 CrossRef CAS PubMed.
- R. Rajpal, P. Dowling, J. Meiller, C. Clarke, W. G. Murphy, R. O'Connor, M. Kell, C. Mitsiades, P. Richardson, K. C. Anderson, M. Clynes and P. O'Gorman, Proteomics, 2011, 11, 1391–1402 CrossRef CAS PubMed.
- K. R. Ting, M. Henry, J. Meiller, A. Larkin, M. Clynes, P. Meleady, D. Bazou, P. Dowling and P. O'Gorman, BBA Clin., 2017, 8, 28–34 CrossRef PubMed.
- Y. Zhang, J. Jiao, P. Yang and H. Lu, Clin. Proteomics, 2014, 11, 1–14 CrossRef PubMed.
- A. Kobata and J. Amano, Immunol. Cell Biol., 2005, 83, 429–439 CrossRef CAS PubMed.
- G. Vidarsson, G. Dekkers and T. Rispens, Front. Immunol., 2014, 5, 520 Search PubMed.
- F. S. van de Bovenkamp, L. Hafkenscheid, T. Rispens and Y. Rombouts, J. Immunol., 2016, 196, 1435–1441 CrossRef CAS PubMed.
- M. Farooq, N. Takahashi, H. Arrol, M. Drayson and R. Jefferis, Glycoconjugate J., 1997, 14, 489–492 CrossRef CAS PubMed.
- S. C. Fleming, S. Smith, D. Knowles, A. Skillen and C. H. Self, J. Clin. Pathol., 1998, 51, 825–830 CrossRef CAS PubMed.
- I. Aurer, G. Lauc, J. Dumic, D. Rendic, D. Matisic, M. Milos, M. Heffer-Lauc, M. Flogel and B. Labar, Coll. Antropol., 2007, 31, 247–251 CAS.
- J. Chen, M. Fang, Y.-P. Zhao, C.-H. Yi, J. Ji, C. Cheng, M.-M. Wang, X. Gu, Q.-S. Sun, X.-L. Chen and C.-F. Gao, PLoS One, 2015, 10, e0127022 CrossRef PubMed.
- S. Mittermayr, G. N. Lê, C. Clarke, S. Millán Martín, A.-M. Larkin, P. O’Gorman and J. Bones, J. Proteome Res., 2017, 16, 748–762 CrossRef CAS PubMed.
- R. L. Shields, J. Lai, R. Keck, L. Y. O'Connell, K. Hong, Y. G. Meng, S. H. A. Weikert and L. G. Presta, J. Biol. Chem., 2002, 277, 26733–26740 CrossRef CAS PubMed.
- A. Pincetic, S. Bournazos, D. J. DiLillo, J. Maamary, T. T. Wang, R. Dahan, B. M. Fiebiger and J. V. Ravetch, Nat. Immunol., 2014, 15, 707–716 CrossRef CAS PubMed.
- A. K. Azab, P. Quang, F. Azab, C. Pitsillides, B. Thompson, T. Chonghaile, J. T. Patton, P. Maiso, V. Monrose, A. Sacco, H. T. Ngo, L. M. Flores, C. P. Lin, J. L. Magnani, A. L. Kung, A. Letai, R. Carrasco, A. M. Roccaro and I. M. Ghobrial, Blood, 2012, 119, 1468–1478 CrossRef CAS PubMed.
- S. V. Glavey, S. Manier, A. Natoni, A. Sacco, M. Moschetta, M. R. Reagan, L. S. Murillo, I. Sahin, P. Wu, Y. Mishima, Y. Zhang, W. Zhang, Y. Zhang, G. Morgan, L. Joshi, A. M. Roccaro, I. M. Ghobrial and M. E. O’Dwyer, Blood, 2014, 124, 1765 CrossRef CAS PubMed.
|
This journal is © The Royal Society of Chemistry 2019 |
Click here to see how this site uses Cookies. View our privacy policy here.