DOI:
10.1039/C8LC01275J
(Paper)
Lab Chip, 2019,
19, 626-633
Ultra-high capacity microfluidic trapping of giant vesicles for high-throughput membrane studies†
Received
21st November 2018
, Accepted 21st December 2018
First published on 4th January 2019
Abstract
Biomimetic systems such as model lipid membranes are vital to many research fields including synthetic biology, drug discovery and membrane biophysics. One of the most commonly used are giant unilamellar vesicles (GUVs) due to their size similarity with biological cells and their ease of production. Typical methods for handling such delicate objects are low-throughput and do not allow solution exchange or long-term observations, all of which limits the experimental options. Herein, we present a new device designed to confine large assemblies of GUVs in microfluidic traps but is still able to perform precise and fast solution exchanges. An optimised design allows efficient filling with as many as 114 GUVs per trap and over 23
000 GUVs per device. This allows high-throughput dataset acquisitions which we demonstrate with two proof-of-concept experiments: (i) end-point measurements of vesicle interior pH and (ii) membrane transport kinetics. Moreover, we show that the design is able to selectively trap sub-populations of specific vesicle sizes and assemble them in different layers. The device can easily be applied to other high-throughput membrane studies and will pave the way for future applications using vesicle assemblies to model cellular tissues or even prototissues.
Introduction
Understanding the biological cell at its minimal complexity can unravel the role of specific components in existing complex cellular systems. For this reason, biomimetic systems such as model lipid membranes have proven to be extremely useful platforms for understanding biomembranes and their role in cellular function.1 Of all the available types of model membranes, giant unilamellar vesicles (GUVs) are one of the most commonly used owing to their ease of production, compatibility with optical microscopy, as well as the fact that their size and membrane curvature resembles that of a typical biological cell.2 These advantages have led to a wide range of uses including biophysical studies of membrane–protein interactions,3 lipid raft investigations,4 as micro-reactors,5 and applications in drug discovery.6 They are also proving to be instrumental in the creation of minimal cells in the emerging topic of bottom-up synthetic biology.7–10
In all of the above applications, analysing large numbers of individual GUVs is essential for obtaining statistically robust data. It is well known that common methods to produce GUVs such as electroformation or gentle hydration11 suffer from membrane compositional heterogeneities12–14 and defects.15 The resulting vesicle-to-vesicle differences can lead to a large scatter in the data. To reduce this effect, one can perform repeat experiments for a given condition or examine a large population of vesicles simultaneously. While this can be sufficient for end-point measurements, in the case of time sensitive membrane experiments, such data sets are difficult to obtain. Moreover, end-point population analysis can lead to bias in the data if certain sub-sets do not survive. Therefore, to overcome these issues, a method to perform live acquisitions of large populations of GUVs, while still being able to add external analytes is desirable.
In typical bulk observation chambers, however, the addition of external molecules often results in loss of vesicles from the field of view due to flow or dilutions, making live image acquisitions difficult. Surface functionalisation strategies can be implemented to immobilise GUVs16–18 but the membrane incorporated moieties may adversely affect the biophysical properties of the lipid bilayer.19 Gel-assisted vesicle immobilisation20 is an alternative but limits the type of molecules that can be added. Moreover, the diffusive mixing of any added solution to a micro- or millilitre volume chamber introduces an unknown dead-time which can reduce the accuracy of any kinetic data.
Advances in microfluidic technology over the past 20 years have led to an explosion of applications involving biological samples contained and analysed within microchannel environments. Recently, micro-structured features fabricated from PDMS have been used to successfully immobilise GUVs in microfluidic channels. These approaches include posts,21–24 side chambers25 and wells.26 Being able to spatially confine giant vesicles in microfluidic channels is particularly attractive as it allows external solution exchange whilst still keeping them within the field of view. This has opened up possibilities for a number of biophysical experiments. For example, full solution exchange can be used to observe morphological changes under different osmotic conditions,25 or to study the effects of transmembrane solution asymmetries on membrane phase behaviour.27 Fluidic control also allows biomolecules to be delivered to the immobilised vesicles to investigate membrane interactions with peptides28 or proteins21 for example. Moreover, the precise fluidic control can be used to apply specific flows to observe the effects of shear forces on lipid domains29 or to measure the mechanical properties of lipid bilayers.26 These microfluidic approaches have so far focussed on strategies to trap single isolated GUVs, which is particularly advantageous as it avoids population heterogeneities. For example, data obtained from vesicles exhibiting structural defects can be avoided.30 Being able to sample individual vesicles can also be particularly powerful for studying phase separation,31 measuring the mechanical properties of membranes,26 or permeation rates18,32 all of which can be influenced by compositional or tension differences. Individual isolation within microfluidic valves can also be used for long-term observations of the same single vesicle.31 In order to immobilise larger numbers for statistics, these single vesicle approaches can be scaled up in an array format to trap 10 s or 100 s of vesicles per device.24,26 Nuss et al. presented a microfluidic chip that could confine up to 30 GUVs in specific locations which could potentially be scaled up to immobilised 100 s per device.23 However, these approaches are still limited in overall capacity and because only low numbers are visible in a particular field of view, the throughput per experiment is restricted.
To this end, we developed a microfluidic platform with multiple traps designed to capture large assembles of GUVs. A unique design allows efficient filling with as many as 114 GUVs per trap and over 23
000 GUVs per device. We characterise the volume of vesicle suspension that is required to completely occupy the traps and fill the device. By changing the height of the channels, we demonstrate size selection of a particular GUV sub-population as well as control over the 3-D layering. We show that solution exchange can be used to deliver analytes to the vesicles in a controlled manner allowing high-throughput biophysical measurements. As a proof-of-concept of the high-throughput capabilities of the device, we performed end-point measurements of pH equilibration across multiple membranes as well as recording parallel kinetics of molecular transport through a membrane pore. The latter of which we show can be used to determine surface protein densities.
Experimental
Chemicals
1-Palmitoyl-2-oleoyl-sn-glycero-3-phosphocholine (POPC) was obtained from Avanti polar lipids. 1,1′-Dioctadecyl-3,3,3′,3′-tetramethylindotricarbocyanine perchlorate (DiIC18) and calcein were purchased from ThermoFisher Scientific. Polydimethylsiloxane (PDMS) and curing agent were obtained as SYLGARD® 184 silicone elastomer kit from Dow Corning. 1H,1H,2H,2H-Perfluorodecyltrichlorosilane was purchased from abcr GmbH. Indium tin oxide (ITO) glass slides are from Präzisions Glas & Optik GmbH. Glucose, sucrose and chloroform were obtained from Merck. Fluorescein, α-hemolysin (α-HL), and β-casein were purchased from Sigma. Millipore® MilliQ water has been used to make all the aqueous solutions.
GUV production
GUVs were produced using the electroformation method. Briefly, 2 mM total lipid concentration of 99.9 mol% POPC and 0.1 mol% DiIC18 in chloroform was smeared on two ITO coated glass slides (2 × 10 μL). Traces of chloroform left in the dried lipid film were removed by desiccation for one hour. Both ITO slides with their lipid coated sides facing each other were sealed to form a chamber using a Teflon spacer and paper clips. The resulting space was filled with 1.7 mL of 300 mM sucrose solution with or without 10 μM fluorescein at pH 11 adjusted with sodium hydroxide. The entire set-up was connected to an AC generator providing 2 V at a frequency of 10 Hz for three hours to produce the GUVs. The resulting vesicles were carefully collected by pipetting and used within three days.
Microfluidic device fabrication
Fabrication of the microfluidic devices was performed using a procedure described previously.21 Briefly, 4′′ silicon wafers (Si-Mat) with master forms at heights 20, 40 and 60 μm were produced via soft photolithography by UV exposure of SU8 3025 (Microchem) through a film mask (Micro Lithography Services). Once the silicon wafer master molds were produced, they were silanised to prevent unwanted PDMS adhesion. This was performed by placing them overnight inside a desiccator with 50 μl of 1H,1H,2H,2H-perfluorodecyltrichlorosilane. To produce the microfluidic chips, PDMS was mixed with the curing agent in 10
:
1 ratio and poured on top of the silanised wafer to a height of 5 mm. The PDMS was degassed in a desiccator for 30 min and cured at 90 °C for three hours. The designs were cut out from the PDMS and holes for the fluidic inlet and outlet were made using a 1.5 mm biopsy puncher (Kai Europe GmbH). A small sample reservoir, cut from a 200 μl pipette tip, with a capacity of 150 μl was placed above the inlet and sealed with PDMS at 90 °C for 30 minutes. To seal the channels, the PDMS part was bonded to a glass coverslip by air plasma treatment (Plasma Cleaner PDC-002-CE, Harrick Plasma) at 0.6 mbar for 1 min. Immediately afterwards, the device was heated for 2 h at 60 °C to aid with the bonding. A photograph of a final assembled device is shown in S1 in the ESI.†
Microfluidic device operation
The microchannels of the device were first flushed with β-casein solution (2 mg ml−1 in 300 mM sucrose) via centrifugation and incubated at room temperature for 30 minutes. This avoids unwanted GUV surface adhesion and rupture. After successful passivation of the channels, β-casein solution was replaced with 100 μl glucose solution of same osmolality using a syringe pump (neMESYS, cetoni) in withdraw mode at 10 μl min−1 connected to the outlet of the chip. Later, specific GUV suspension volumes were loaded into the reservoir and drawn through the channels to occupy the traps (Fig. S1 in the ESI†). To exchange the solutions surrounding the trapped GUVs, the flow was stopped and the reservoir was washed (at least 5 times) with the new solution before starting the flow again. All used solutions were osmotically balanced to avoid vesicle rupture or deflation.
Microscopy and data analysis
DiIC18 labelled GUVs captured in the traps were imaged using a confocal microscope (SP8, Leica) with laser excitation of 552 nm and emission was detected between 565 nm to 635 nm. For GUVs flushed with external calcein solution or containing fluorescein, the excitation was 488 nm and emission was detected in the range of 498 to 540 nm. Sequential acquisitions were performed whenever multiple fluorophores were employed. For all acquisitions, bright-field transmission images were also obtained. Image analysis was performed using LASX (Leica) and Fiji (ImageJ). Curve fitting was performed using Origin (OriginLab).
Results and discussion
Design of chip & loading of GUVs
The overall design of the microfluidic chip is shown schematically in Fig. 1. After the inlet, there is a filter to avoid any unwanted particles from clogging the main channels. The channels then branch out into a series of 12 parallel channels containing micro-structured posts which hydrodynamically capture multiple GUVs at specific locations, herein referred to as ‘traps’. Having the traps separated into parallel channels minimises the fluidic delivery time compared to a single longer channel of the same volume. The individual posts of the traps have dimensions of 20 μm by 20 μm with a gap size of 10 μm. Each of the 12 channels contains 17 traps, making a total of 204 traps per device. Each trap has an open corral style design with traps 2 to 17 having additional alternating side-ward extensions to the posts (Fig. 1). The purpose of such a design is to maximise the overall trapping efficiency of the device.
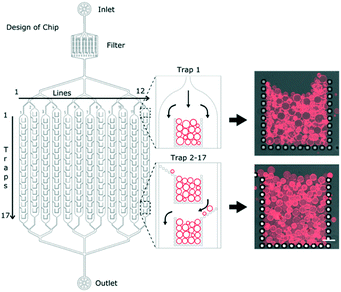 |
| Fig. 1 Microfluidic design for high capacity trapping of GUVs. Left: Schematic of the overall channel network design. The channels split into 12 separate lines, each with 17 traps totalling 204 per device. Inserts show the trap number 1 and traps 2 to 17 designed to maximise GUV capture. Right: Confocal images showing GUVs in trap numbers 1 (top) and 8 (bottom) with DiIC18 fluorescence from the membranes overlaid with the bright-field transmission images of the PDMS posts. Scale bar: 50 μm. | |
GUVs produced using the electroformation method are first loaded into the reservoir (Fig. S1 in the ESI†). Upon applying a reverse flow with the syringe pump, they are drawn into the 12 channels and begin to occupy the traps. A time series was acquired of one of the first traps (Movie S1 in the ESI†) and shows the typical mode by which the traps are filled. Using a flow rate of 10 μl min−1, it takes less than 20 minutes to completely fill a single trap. Note that this filling time depends on the number of the vesicles in the sample: if the yield is low then longer filling times are required. The loading time can be reduced by increasing the flow rate but care should be taken as some GUVs can be pushed between the posts and lost at higher flow rates.
Having a series of posts (rather than a solid wall for example) allows complete filling of the traps; once the first row of vesicles is trapped, subsequent ones are still able to enter into the traps due to continued flow through the side posts as illustrated in Fig. S2 in the ESI.† This continues layer-by-layer until finally the entire trap is occupied and the flow is diverted around the trap (Fig. 1). Crucially, the diverted flow prevents high hydrodynamic forces on the GUVs and therefore avoids bursting or loss.
Complete filling of device
In order to better understand the loading and filling of the device, GUV suspension volumes of 50, 100, 500 and 2000 μl were flushed into the device and we acquired images of all 17 traps and across three different channels. Data were then obtained on the average number of vesicles per trap and the % occupancies. Fig. 2 shows that for 50 μl, approximately 75% occupancy was reached in the first trap but almost half of the remaining traps were empty. Here we define 100% occupancy when the vesicles completely fill the area within the traps. Importantly, the data shows that even for low volumes and short loading times, hundreds of vesicles are captured in total which is advantageous for time-sensitive experiments. For complete filling, where the occupancy of each trap is greater than 90%, the device requires at least 2000 μl of vesicle suspension. It should be noted that different preparation techniques will result in different GUV yields which may require different volumes to completely fill the device. However, electroformation is the most commonly used approach so the data presented here is representative of a typical loading experiment.
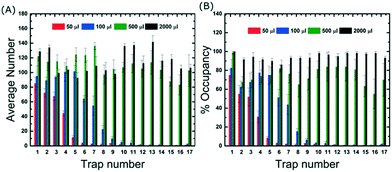 |
| Fig. 2 Complete filling of device. The average number (A) and % occupancy (B) of GUVs captured in each trap (1 to 17) for increasing volumes flushed in at a flow rate of 10 μL min−1. Vesicle counting is performed manually. Mean values are from three different channels and error bars are taken from the standard deviation of the mean. | |
Traps 2 to 17 boast additional side-posts extending at a 45° angle (Fig. 1) designed to improve the trapping efficiency of the device. Without them, the first trap can still be filled, but the loading efficiency decreases considerably in subsequent traps with most GUVs flowing past (see Movie S2 in the ESI†). The side-posts deflect the vesicles into the adjacent trap which then becomes fully occupied. This continues in a cascade manner until all traps in the channels are filled. A quantitative comparison of the trapping performance with and without the side-posts shows that they increase the number of captured vesicles by 250% and require 20 times less volume to trap the same number of GUVs (see Section S3 of ESI†). Importantly, without the side-posts the device is not able to fill up completely.
One of the important aspects of this device is the amount of quantitative data that can be generated. Once all the traps are occupied, the average number of vesicles per trap is 114 ± 7 which means that there are 23
256 GUVs per device. For a typical solution prepared by electroformation, the volume containing the same number of GUVs is about 0.71 mL. Comparing this to the 2 mL volume required to fill the chip, the trapping efficiency of the device was calculated to be ∼36% (see eqn S1 in ESI†).
It is evident that although the packing of the vesicles is passive, the efficiency appears to be high with spaces between vesicles being minimised as they rearrange. Importantly, GUVs with diameters larger than the gap between the posts and smaller than the height of the channels are preferentially trapped. This suggests that the device can segregate the GUVs based on their size by varying the height of the channels.
Size based trapping of GUVs
To further investigate the effect of the channel height on the size of the trapped vesicles, we loaded vesicles into three different devices with heights of 20, 40 and 60 μm. The diameters of the GUVs were then analysed and Fig. 3 shows the resulting histograms. The data shows that the device is able to trap different sized sub-populations of GUVs with mean average diameters of 13.7 ± 1.4, 23 ± 3.5, and 40 ± 6 μm for heights of 20, 40 and 60 μm, respectively. Vesicles and other debris smaller than ∼10 μm are not trapped due to the fixed 10 μm gap between the posts. The ability to confine only vesicles of a defined size is beneficial for a number of experiments; for example, studies of membrane morphologies depend heavily on the radius of the vesicle under observation.33 Mono-disperse vesicles are also advantageous when encapsulating a reaction which requires a specific number of reactants. It should be noted that microfluidic trapping devices have been used to tune the size of the trapped vesicles either dynamically by varying the flow rates23 or by using arrays of posts.24 However, these methods do not allow for high-capacities as demonstrated here. Vesicles of equal size can of course be prepared directly using microfluidic techniques34 but complex setups and issues with residual oil remaining in the bilayer can be a limitation. Our method herein can be used with giant vesicles prepared from standard techniques making it more applicable. Bulk filtering methods also provide ways to produce mono-disperse vesicles from poly-disperse populations,35–37 but multiple extrusion or dialysis steps can cause unwanted rupture lowering the final yield and reducing encapsulation efficiencies.
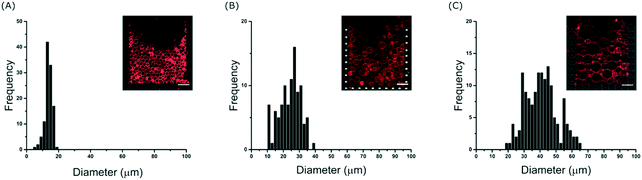 |
| Fig. 3 Size selection of GUVs. Histograms of trapped GUV diameters for channel heights of (A) 20, (B) 40 and (C) 60 μm. Average diameters were found to be 14 ± 1, 23 ± 4, and 40 ± 6 μm for heights of 20, 40 and 60 μm respectively taken from three separate trap measurements. Inserts: representative fluorescence and bright-field overlays. Scale bars: 50 μm. | |
Interestingly, the device is able to tune the assembly of GUVs not only in 2-D but also in 3-D. Fig. S4 in the ESI† shows side views (rendered from confocal microscopy stacks) of different GUV layers depending on the height of the channels. With 20 μm the GUVs are assembled with one layer, with 40 μm two layers, and with 60 μm three layers. This could be further tuned by introducing monodisperse vesicles if desired. Such a 3-D structured membrane-based system has the potential to be used to model the assembly of cellular tissues. In particular, we envision that the device could be used to model and study cell-to-cell transport or diffusion in the extra cellular medium in a controlled model environment. By increasing the height, the total number of vesicles also increases.
Fluid exchange around the vesicles
The ability to fully exchange the solution surrounding the trapped GUVs without losing them is crucial for delivery of molecules to the membranes. In addition, it can also be used to remove molecules from the surrounding solution, which is a valuable tool to investigate dissociation rates or the permeation of encapsulated molecules out of vesicles. Even though the majority of the flow is diverted around the traps once they are fully occupied, we wanted to explore whether or not small molecules are still able to enter the traps. To test this, we exchanged the GUV sample in the reservoir with 10 μM calcein in 300 mM sucrose and flushed it through the channels at 10 μl min−1. Fig. 4 shows that within a minute, the entire solution inside the chip (and surrounding the vesicles) is exchanged. This differs from a typical bulk dilution where the vesicles are also diluted. Importantly, even though some vesicles are closely packed together (due to the flow), there is enough space between them for the fluorophore to reach all regions of the trap. This is clearly visible after 50 s of fluid exchange (Fig. 4A). If required, faster fluidic exchange times could be achieved with higher flow rates, but above 10 μl min−1 some vesicles are displaced from the entrance of the trap when it is close to complete occupancy. However, this loss is small, at 15 ± 1%. It is important to note that the volume required to replace the solution around the vesicles is approximately 2 μl which is advantageous for precious samples such as nanoparticles, drugs, or proteins. Once incubated with a particular fluidic condition, the treated vesicles can be unloaded from the traps and harvested from the device simply by reversing the flow (see Movie S3 in ESI†). This will allow future off-line analysis of the lipid membranes with methods such as NMR or X-ray scattering which are difficult to apply inside microchannels.
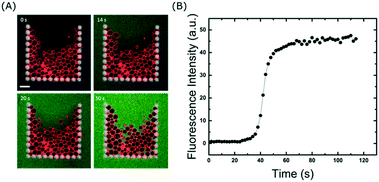 |
| Fig. 4 Fast and complete fluid exchange. A) Confocal time series of flushing calcein solution into the device at a flow rate of 10 μl min−1. Scale bar: 50 μm. B) Plot of calcein fluorescence taken inside the trap area. Complete solution exchange is achieved after approximately 50 to 60 s. | |
High-throughput measurements
End-point pH detection measurements.
As a proof-of-concept demonstration of high-throughput end-point measurements, we employed a pH detection assay inside the vesicles. GUVs were electroformed in the presence of 10 μM fluorescein in 300 mM sucrose solution at pH 11. The fluorescence intensity of fluorescein is sensitive to pH changes and can therefore serve as a means to monitor the transport of protons across membranes.38 After loading them into a device, the solution surrounding the vesicles was exchanged with 300 mM glucose solutions buffered at pH values from 10 to 5 using a flow rate of 5 μl min−1 for 15 min. Note that the osmolarities of the solutions were all matched to avoid vesicle deflation or bursting. For each pH condition the flow was stopped and fluorescence intensities of 395 GUVs interiors were measured across five traps. Fig. 5 shows that for decreasing pH values the final fluorescence intensity decreases as the available hydrogen ions are able to permeate across the lipid membranes and protonate the fluorophore.
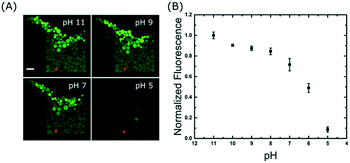 |
| Fig. 5 High-throughput end-point measurements using a pH detection assay. (A) Fluorescein encapsulating vesicles incubated for 15 min at various pH conditions. Confocal fluorescence images of DiIC18 (red) and fluorescein (green) are overlaid with the bright-field images of the posts. Scale bar: 50 μm. (B) Plot showing the change in fluorescence intensity inside the GUVs after incubation in various pH buffers. Mean values are from 395 GUVs across 5 traps and errors bars are taken from the standard deviation of the mean. | |
A time series was acquired during a pH drop from 11 to 5 and reveals that the change in signal occurs in less than 25 seconds: the outer GUVs lose their intensities first, followed by the inner ones (see Movie S4 in the ESI†). The precise mechanism of proton transport across lipid bilayers is not fully understood39 but the rapid change observed here could be due to a combination of permeation and transient pore formation after a sudden pH drop. However, we do not believe the membranes were porous to other molecules because we did not observe any loss of contrast in the bright-field transmitted light images (Fig. S5 in the ESI†), which is an indication that the sugar membrane asymmetry was retained. Fig. 5A shows the end-point images of the traps; it is interesting to note that not all GUVs have equal intensities after incubation at each pH buffer. This is partly due to inhomogeneous encapsulation of fluorescein, but also because some vesicles contain more than one bilayer (see pH 5). These artefacts are limitations of the electroformation method and are the main source of error in Fig. 5B. However, this further highlights the need to analyse large numbers of vesicles to obtain statistically robust data.
Kinetics of membrane transport.
As another proof-of-concept, we recorded high-throughput membrane transport kinetics using the membrane protein α-hemolysin (α-HL). This water-soluble protein self-assembles into lipid bilayers to form a heptameric transmembrane pore which allows passive transport of small molecules.40 First, GUVs produced in 300 mM sucrose were loaded, then the surrounding solution was exchanged for 2.5 mg ml−1 α-HL (in 300 mM glucose) and incubated for 15 min. Subsequently, 10 μM calcein (also in 300 mM glucose) was flushed into the device at 5 μl min−1 and a confocal time series was acquired. The fluorescence intensities of 66 GUVs were then analysed and plotted in Fig. 6A. The calcein fluorescence increases within each vesicle indicating the presence of the pre-formed membrane pores but the rate of transport is not equal across all GUVs. Using a method developed by Bleicken et al.41 we fitted each curve with eqn (1),where F(t) is the time dependent fluorescence inside the vesicle and τflux is the rate of molecule influx. An example of a fitted curve is shown in Fig. S6 in the ESI.† Here, the analysis is greatly simplified compared to previous bulk approaches41 as the concentration of calcein outside the GUVs remains constant throughout the experiment. Subsequently, τflux was used to obtain the total porated area, AT, of each vesicle according to eqn (2), |  | (2) |
where V is the vesicle volume, D is the diffusion coefficient of calcein (248 μm2 s−1 in 300 mM sucrose42) and l is the length of the pore (10 nm (ref. 40)). Fig. 6B shows each value of AT plotted against each GUV diameter with a straight line fit as a guide to the eye. As can be seen, larger vesicles have greater porated areas as more pores are able to assemble at the surface, which is in agreement with other findings.41 The scatter in the data may originate from inhomogeneous distribution of the pores, membrane defects, and regions of membrane adhesion. Using eqn (3) we were able to calculate the pore number density ρ for each vesicle. |  | (3) |
Here, Ap is the cross-sectional area of a single α-HL pore and As is the surface area of each vesicle. Finally, the average ρ across all of the vesicles was calculated to be 0.11 ± 0.04 μm−2.
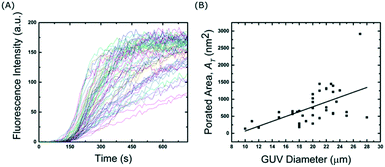 |
| Fig. 6 High-throughput membrane transport measurements using a single device. (A) Fluorescence traces of calcein influx from 66 GUVs pre-incubated with α-HL membrane pores. (B) Total porated area plotted against GUV diameter. The straight line fit is a guide to the eye. | |
Conclusions & discussion
We have presented a microfluidic device comprising a series of micro-structured posts or ‘traps’ designed to hydrodynamically capture multiple GUVs at specific spatial locations. The layout of the posts was optimised to maximise the efficiency of the vesicle capture. This is vital for minimising loss of precious or expensive samples or when vesicle yields are low when produced using electroformation in high salt buffers for example.27,43 After complete filling, each trap contains 114 GUVs resulting in a total of over 23
000 GUVs per device. Other devices reported in the literature present tens or hundreds of immobilised vesicles per device.21,23,24,26,44 The high-capacity trapping ability of the design presented here has huge potential for a range of biophysical membrane applications where high volumes of data are needed for statically robust analyses. This is essential when vesicle-to-vesicle differences in the population such as lipid compositions, encapsulation efficiencies, tensions, or membrane protein reconstitution are high. In the case of vesicles produced by swelling on poly(vinyl alcohol) (PVA)45 or agarose,46 and formation via oil-based emulsion methods,37 the membrane properties can be altered due to unwanted incorporation of polymers47,48 or oil49,50 into the lipid bilayers. Therefore, the ultra-high capacity trapping of this device will be especially beneficial as high volumes of quantitative data can be obtained from a single on-chip experiment thus reducing the impact of unwanted heterogeneities.
We showed that the device can also be used to filter GUVs by size. Typical GUV preparation techniques result in a wide range of sizes (1 to ∼100 μm), but by varying the channel height the average trapped diameter can be tuned to 14, 23, or 40 μm. This is an advantage when the encapsulated volume inside the vesicles needs to be controlled. We also demonstrated that the 3-D packing of trapped vesicles can be controlled by changing the height of the channels. By increasing the channel height, the device is able to tune the layering of the vesicles to either one, two or three.
Once trapped, we demonstrated that molecules can be homogeneously added or removed to all of the vesicles in less than 60 s. This is particularity useful as many liposome production methods result in encapsulated molecules being both outside as well as inside the vesicles. Therefore, by removing the outer molecules one can investigate the efflux as well as influx of permeating molecules for example. It is interesting to note that capturing high numbers of vesicles in such a small volume increases the lipid concentration. With GUVs observed in a 1 ml bulk chamber, the lipid concentration is typically tens of pM, but here the lipid concentration per line is ∼80–200 nM. This has the advantage of reducing any unwanted effects due to impurities in the buffer51 and could also be used to shift the chemical equilibrium of ligand–membrane interactions.
Finally, high-throughput measurements on a single device were demonstrated with two experiments. The first was to apply a chemical gradient to the vesicles and acquire end-point measurements. The vesicles were subjected to different proton gradients and the equilibrated pH was measured using an encapsulated dye. In a single device, we were able to record ∼400 vesicles for 7 different pH conditions thereby reducing any impact from sample differences or experimental errors. While end-point measurements can of course be performed in a bulk setting, being able to record multiple data points, where the vesicles have been subjected to the exact same condition, is unique to this microfluidic platform. Here they were incubated with a pH buffer for a specific time period, but other conditions such as osmotic stress or the effects of anti-microbial agents could be investigated. The second high-throughput experiment involved measurements of membrane transport kinetics. Calcein influx through a membrane pore was recorded for 66 vesicles simultaneously. The data was then used to calculate the average number density of membrane pores using the data from a single experiment. This was made possible as the device is able to concentrate multiple vesicles at specific spatial locations, and for a given field-of-view one can record the kinetics of tens of vesicles simultaneously. Both of these experiments show the potential of the device for high-throughput experiments in order to obtain statistically robust data sets.
Outlook
Overall, we present this device as a high-throughput platform for GUV-based membrane studies but it also opens up the possibility for minimal tissue studies. Captured GUVs arranged in specific assemblies such as the ones shown herein could be used for designing complex tissue mimicking structures with higher order architectures.52 Densely packed vesicles could also be an ideal model system for investigating how early protocells might have assembled into the colonies or prototissues.53,54 Finally, the platform could be used to produce controlled assemblies of real cells for cell-to-cell communication studies55,56 or even to investigate the behaviour of bacterial biofilms.57
Conflicts of interest
There are no conflicts to declare.
Acknowledgements
The authors would like to thank René Genz for creation of the 3-D cartoon in the TOC graphic and Albert Bae at the Max Planck Institute for Dynamics and Self-Organization in Göttingen for wafer fabrication. We acknowledge Reinhard Lipowsky for institutional and financial support. We thank Jan Steinkühler and Rumiana Dimova helpful discussions and careful reading of the manuscript. Finally, we thank Eleanor Ewins for proofreading. This work is part of the MaxSynBio consortium which is jointly funded by the Federal Ministry of Education and Research of Germany and the Max Planck Society. Open Access funding provided by the Max Planck Society.
References
- K. Simons and W. L. C. Vaz, Annu. Rev. Biophys. Biomol. Struct., 2004, 33, 269–295 CrossRef CAS PubMed.
- P. Walde, K. Cosentino, H. Engel and P. Stano, ChemBioChem, 2010, 11, 848–865 CrossRef CAS PubMed.
- E. Sezgin and P. Schwille, Mol. Membr. Biol., 2012, 29, 144–154 CrossRef CAS PubMed.
- K. Simons and M. J. Gerl, Nat. Rev. Mol. Cell Biol., 2010, 11, 688–699 CrossRef CAS PubMed.
- P. Yang, R. Lipowsky and R. Dimova, Small, 2009, 5, 2033–2037 CrossRef CAS PubMed.
- C. Peetla, A. Stine and V. Labhasetwar, Mol. Pharmaceutics, 2009, 6, 1264–1276 CrossRef CAS PubMed.
- P. L. Luisi and P. Stano, Nat. Chem., 2011, 3, 755–756 CrossRef CAS PubMed.
- P. Schwille, Science, 2011, 333, 1252–1254 CrossRef CAS PubMed.
- P. Schwille, J. Spatz, K. Landfester, E. Bodenschatz, S. Herminghaus and V. Sourjik,
et al.
, Angew. Chem., Int. Ed., 2018, 57(41), 13382–13392 CrossRef CAS PubMed.
- P. Schwille and S. Diez, Crit. Rev. Biochem. Mol. Biol., 2009, 44, 223–242 CrossRef CAS PubMed.
- R. Dimova, S. Aranda, N. Bezlyepkina, V. Nikolov, K. A. Riske and R. Lipowsky, J. Phys.: Condens. Matter, 2006, 18, S1151–S1176 CrossRef CAS PubMed.
- E. Baykal-Caglar, E. Hassan-Zadeh, B. Saremi and J. Huang, Biochim. Biophys. Acta, Biomembr., 2012, 1818, 2598–2604 CrossRef CAS PubMed.
- T. Bhatia, P. Husen, J. Brewer, L. A. Bagatolli, P. L. Hansen, J. H. Ipsen and O. G. Mouritsen, Biochim. Biophys. Acta, Biomembr., 2015, 1848, 3175–3180 CrossRef CAS PubMed.
- N. Bezlyepkina, R. S. Gracià, P. Shchelokovskyy, R. Lipowsky and R. Dimova, Biophys. J., 2013, 104, 1456–1464 CrossRef CAS PubMed.
- N. Rodriguez, J. Heuvingh, F. Pincet and S. Cribier, Biochim. Biophys. Acta, Gen. Subj., 2005, 1724, 281–287 CrossRef CAS PubMed.
- P. Kuhn, K. Eyer, T. Robinson, F. I. Schmidt, J. Mercer and P. S. Dittrich, Integr. Biol., 2012, 4, 1550–1555 CrossRef CAS PubMed.
- D. Stamou, C. Duschl, E. Delamarche and H. Vogel, Angew. Chem., Int. Ed., 2003, 42, 5580–5583 CrossRef CAS PubMed.
- S. Li, P. C. Hu and N. Malmstadt, Biophys. J., 2011, 101, 700–708 CrossRef CAS PubMed.
- M. J. Sarmento, M. Prieto and F. Fernandes, Biochim. Biophys. Acta, Biomembr., 2012, 1818, 2605–2615 CrossRef CAS PubMed.
- R. B. Lira, J. Steinkühler, R. L. Knorr, R. Dimova and K. A. Riske, Sci. Rep., 2016, 6, 25254 CrossRef CAS PubMed.
- T. Robinson, P. Kuhn, K. Eyer and P. S. Dittrich, Biomicrofluidics, 2013, 7, 44105 CrossRef CAS PubMed.
- T. Robinson, P. E. Verboket, K. Eyer and P. S. Dittrich, Lab Chip, 2014, 2852–2859 RSC.
- H. Nuss, C. Chevallard, P. Guenoun and F. Malloggi, Lab Chip, 2012, 12, 5257 RSC.
- Y. Kazayama, T. Teshima, T. Osaki, S. Takeuchi and T. Toyota, Anal. Chem., 2016, 88, 1111–1116 CrossRef CAS PubMed.
- S. Vrhovec, M. Mally, B. Kavčič and J. Derganc, Lab Chip, 2011, 11, 4200–4206 RSC.
- A. Yamada, S. Lee, P. Bassereau and C. N. Baroud, Soft Matter, 2014, 26–28 Search PubMed.
- B. Kubsch, T. Robinson, R. Lipowsky and R. Dimova, Biophys. J., 2016, 110, 2581–2584 CrossRef CAS PubMed.
- N. Purkayastha, K. Eyer, T. Robinson, P. S. Dittrich, A. K. Beck, D. Seebach, B. Kolesinska and R. Cadalbert, Chem. Biodiversity, 2013, 10, 1165–1184 CrossRef CAS PubMed.
- F. Sturzenegger, T. Robinson, D. Hess and P. S. Dittrich, Soft Matter, 2016, 12, 5072–5076 RSC.
- B. Kubsch, T. Robinson, J. Steinkühler and R. Dimova, J. Visualized Exp., 2017,(128) DOI:10.3791/56034.
- B. Kubsch, T. Robinson, R. Lipowsky and R. Dimova, Biophys. J., 2016, 110(12), 2581–2584 CrossRef CAS PubMed.
- B. Kolesinska, K. Eyer, T. Robinson, P. S. Dittrich, A. K. Beck, D. Seebach and P. Walde, Chem. Biodiversity, 2015, 12, 697–732 CrossRef CAS PubMed.
- R. Lipowsky, Curr. Opin. Struct. Biol., 1995, 5, 531–540 CrossRef CAS PubMed.
- D. van Swaay and A. DeMello, Lab Chip, 2013, 13, 752–767 RSC.
- T. F. Zhu and J. W. Szostak, PLoS One, 2009, 4, e5009 CrossRef PubMed.
- Y. Tamba, H. Terashima and M. Yamazaki, Chem. Phys. Lipids, 2011, 164, 351–358 CrossRef CAS PubMed.
- M. Hadorn, E. Boenzli, P. Eggenberger Hotz and M. M. Hanczyc, PLoS One, 2012, 7(11), e50156 CrossRef CAS PubMed.
- M. M. Martin and L. Lindqvist, J. Lumin., 1975, 10, 381–390 CrossRef CAS.
- H. L. Tepper and G. A. Voth, Biophys. J., 2005, 88, 3095–3108 CrossRef CAS PubMed.
- L. Song, M. R. Hobaugh, C. Shustak, S. Cheley, H. Bayley and J. E. Gouaux, Science, 1996, 274, 1859–1865 CrossRef CAS PubMed.
- S. Bleicken, O. Landeta, A. Landajuela, G. Basañez and A. J. García-Sáez, J. Biol. Chem., 2013, 288, 33241–33252 CrossRef CAS PubMed.
-
R. C. Weast, Handbook of Chemistry and Physics, 69th edn, 1988 Search PubMed.
- T. Pott, H. Bouvrais and P. Méléard, Chem. Phys. Lipids, 2008, 154, 115–119 CrossRef CAS PubMed.
- D. J. Paterson, J. Reboud, R. Wilson, M. Tassieri and J. M. Cooper, Lab Chip, 2014, 14, 1806 RSC.
- A. Weinberger, F. C. Tsai, G. H. Koenderink, T. F. Schmidt, R. Itri, W. Meier, T. Schmatko, A. Schröder and C. Marques, Biophys. J., 2013, 105, 154–164 CrossRef CAS PubMed.
- K. S. Horger, D. J. Estes, R. Capone and M. Mayer, J. Am. Chem. Soc., 2009, 131, 1810–1819 CrossRef CAS PubMed.
- T. P. T. Dao, M. Fauquignon, F. Fernandes, E. Ibarboure, A. Vax, M. Prieto and J. F. Le Meins, Colloids Surf., A, 2017, 533, 347–353 CrossRef CAS.
- R. B. Lira, R. Dimova and K. A. Riske, Biophys. J., 2014, 107, 1609–1619 CrossRef CAS PubMed.
- E. A. Kubatta and H. Rehage, Colloid Polym. Sci., 2009, 287, 1117–1122 CrossRef CAS.
- C. Campillo, P. Sens, D. Köster, L.-L. Pontani, D. Lévy, P. Bassereau, P. Nassoy and C. Sykes, Biophys. J., 2013, 104, 1248–1256 CrossRef CAS PubMed.
- R. L. Knorr, J. Steinkühler and R. Dimova, Biochim. Biophys. Acta, Biomembr., 2018, 1860, 1957–1964 CrossRef CAS PubMed.
- G. Villar, A. D. Graham and H. Bayley, Science, 2013, 48, 48–53 CrossRef PubMed.
- P. Carrara, P. Stano and P. L. Luisi, ChemBioChem, 2012, 13, 1497–1502 CrossRef CAS PubMed.
- T. P. de Souza, G. V. Bossa, P. Stano, F. Steiniger, S. May, P. L. Luisi and A. Fahr, Phys. Chem. Chem. Phys., 2017, 19, 20082–20092 RSC.
- J. C. Hervé and M. Derangeon, Cell Tissue Res., 2013, 352, 21–31 CrossRef PubMed.
- C. M. Waters and B. L. Bassler, Annu. Rev. Cell Dev. Biol., 2005, 21, 319–346 CrossRef CAS PubMed.
- H. Koo, R. N. Allan, R. P. Howlin, P. Stoodley and L. Hall-Stoodley, Nat. Rev. Microbiol., 2017, 15, 740–755 CrossRef CAS PubMed.
Footnote |
† Electronic supplementary information (ESI) available. See DOI: 10.1039/c8lc01275j |
|
This journal is © The Royal Society of Chemistry 2019 |
Click here to see how this site uses Cookies. View our privacy policy here.