DOI:
10.1039/C8LC00863A
(Paper)
Lab Chip, 2019,
19, 120-135
Automated high-content phenotyping from the first larval stage till the onset of adulthood of the nematode Caenorhabditis elegans†
Received
19th August 2018
, Accepted 21st November 2018
First published on 28th November 2018
Abstract
The nematode Caenorhabditis elegans is increasingly used as a model for human biology. However, in vivo culturing platforms for C. elegans allowing high-content phenotyping during their life cycle in an automated fashion are lacking so far. Here, a multiplexed microfluidic platform for the rapid high-content phenotyping of populations of C. elegans down to single animal resolution is presented. Nematodes are (i) reversibly and regularly confined during their life inside tapered channels for imaging fluorescence signal expression and to measure their growth parameters, and (ii) allowed to freely move in microfluidic chambers, during which the swimming behavior was video-recorded. The obtained data sets are analyzed in an automated way and 19 phenotypic parameters are extracted. Our platform is employed for studying the effect of bacteria dilution, a form of dietary restriction (DR) in nematodes, on a worm model of Huntington's disease and demonstrates the influence of DR on disease regression.
Introduction
Recent advances in microfluidics, electronics, and materials science have enabled the miniaturization of bioanalytical applications into Lab-on-a-Chip (LoC) systems.1,2 LoC devices are conveniently applicable to cell-based and single organism studies, as they can easily be fabricated in an adaptable size, depending on the biological sample of interest.3,4 This feature together with the opportunity for parallel analysis has allowed the design of various drug delivery platforms for living multicellular organisms. For example, microfluidic gradient generators were developed for drug application on zebrafish,5,6 while other work on Drosophila larvae included application of gradients of airborne chemical cues.7 Drug delivery platforms were also developed for droplet-based mammalian cells.8
Caenorhabditis elegans is a frequently studied multicellular organism that is an excellent model to address fundamental biological questions,9 motivated by its relatively small size, transparency, short life cycle, hermaphroditism and well-annotated genome. C. elegans culture is traditionally based on growth on agar plates that are pre-coated with Escherichia coli bacteria for feeding.10 Commonly used methods to handle C. elegans include tedious manual manipulation, while automated tools for nematode manipulation also exist, but these are expensive, bulky, and lack the connection with worm culture and the possibility of complex phenotyping. Furthermore, the high motility of the nematodes necessitates a temporary immobilization method for accurate imaging. Some common methods include the utilization of anesthetics for this purpose.11 Different approaches with LoC platforms have been reported for arraying, culturing and immobilizing highly mobile C. elegans in a microfluidic chip for automated manipulation and long-term imaging. One such approach used “behavior” and “olfactory” chips, to effectively trap young adult nematodes, but the monitoring of spontaneous locomotion was somehow hindered by this design.12 Another study developed a microfluidic chip that performed high-speed sorting and could also isolate and immobilize the nematodes for screening phenotypic features.13 Alternatively, deflecting polydimethylsiloxane (PDMS) membranes were utilized to temporarily immobilize and capture time-lapse images of green fluorescent protein (GFP) expression of different nematode strains.14 This technology was also combined with on-chip culturing and high-resolution imaging albeit rather tedious initial worm distribution and low throughput.15 Long-term immobilization over multiple worm development stages was demonstrated at high imaging resolution.16 Droplet encapsulation was also used as an alternative method to isolate and immobilize C. elegans at the L1 stage,17 while gel-based techniques were employed to temporarily arrest nematodes at various larval stages.18,19 Gel-based techniques were also proven to be ideal platforms for high-resolution longitudinal imaging and long-term culture, but without a microfluidic approach, they lacked an adequate bacterial replenishing system and automated late progeny wash off.20–22
It has become clear that rapid and high-content phenotyping of C. elegans is essential to obtain significant amounts of data that allow conclusions to be drawn on a solid statistical basis. Image and video processing approaches are commonly used to obtain phenotypic data by quantifying the development and the motility of C. elegans.23,24 Yet, most of the studies focused on short-term behavior without culturing the nematodes for the long term.25–27 Some previous aging and drug screening platforms had nematode growth chambers but lacked immobilization,28 had good lateral confinement but were missing early larval stage development and phenotyping,29,30 and exhibited confinement for a larval stage but were missing on-chip culturing31 or had less precise initial worm distribution.32 Other microfluidic approaches also provided long-term culture and phenotypic analysis, either in populations or down to single worm resolution, but these papers did not report multiplexing of experimental parameters or automated high-content phenotyping results.33–36 To the best of our knowledge, there has been no C. elegans platform that (i) enables life cycle on-chip culturing, (ii) includes features for reversibly immobilizing worms at all different life stages, and (iii) has an integrated image and video processing approach to extract multiple swimming gait and development properties.
Here, we present an automated, multiplexed, rapid and high-content phenotyping platform for the quantification of growth, fluorescence and motility of a statistically relevant C. elegans population down to single worm resolution. Our microfluidic chip has ∼30 culture and immobilization chambers that enable culturing several worms in parallel, ranging from one to three worms per chamber, and include only passive hydrodynamic filter structures that are straightforward in operation by applying pressure-pulsed liquid flows. We performed life cycle culturing and phenotyping, which allowed us to observe the motility and development parameters of the nematodes starting from their first larval stage. Besides video recordings, we provided tapered channels to reversibly immobilize worms with a high accuracy at all stages for high-resolution imaging. We think that our platform is one of the most advanced systems out there, providing in-depth disease progression studies on nematodes with its unique features of high-content phenotyping, multiplicity, automation and ease of use, all at once. In order to validate our approach, we focused on the effects of DR in a C. elegans model of Huntington's disease (HD). DR is defined as the restriction of nutrients without malnutrition and has been shown to extend the lifespan in a wide variety of species.37,38 DR not only prolongs the lifespan but also delays a variety of age-related diseases.39,40 In Caenorhabditis elegans, a broad range of DR regimens have been proposed and they all extend the lifespan to various degrees by separate or partially overlapping molecular pathways.41,42 However, little is known about the effect of DR on proteotoxic stress and neurodegenerative diseases. Additionally, DR studies on worms cultured on agar plates have been extremely tedious to perform due to the lack of a suitable food control system. We first identified the optimal food dosing for normal development and for studying DR on wild-type C. elegans, and subsequently analyzed the effects of DR on protein aggregation, in a worm model for HD that expresses Yellow Fluorescent Protein (YFP)-tagged poly-glutamine repeats in body-wall muscle.43 We present a comprehensive study that correlates worm motility, development and aggregate formation behavior, depending on the food concentration.
Materials and methods
Materials and chemicals
4 inch 550 μm thick Si wafers were purchased from Center of Micro- and Nanotechnology of EPFL (Lausanne, Switzerland). MicroChem SU8-3050 1 L negative photoresist was bought from Micro Resist Technology GmbH (Berlin, Germany). PDMS Sylgard 184 was acquired from Dow Corning (Wiesbaden, Germany). 1 mL borosilicate H-TLL-PE syringes were obtained from Innovative Labor Systeme (Stutzerbach, Germany). Corning microscope slides (75 mm × 38 mm) were bought from Sigma-Aldrich (Buchs, Switzerland). A Microline ethyl vinyl acetate tube with 0.51 mm inner and 1.52 mm outer diameters was purchased from Fisher Scientific (Wohlen, Switzerland). L-broth bacterial culture medium was obtained by adding 10 g of Bacto-tryptone, 5 g of Bacto-yeast, and 5 g of NaCl in 1 L of H2O. S-basal was obtained by adding 5.85 g of NaCl, 1 g of K2HPO4, 6 g of KH2PO4, and 1 ml of cholesterol (5 mg ml−1 in ethanol) in 1 L of H2O. S-medium was obtained by adding 0.5 ml of 1 M potassium citrate (pH 6), 0.5 ml of trace metal solution, 0.15 ml of 1 M CaCl2, and 0.15 ml of 1 M MgSO4 in 50 mL of S-basal. S-basal, L-broth and S-medium were sterilized by autoclaving. mPEG5K-silane was bought from Sigma-Aldrich (Buchs, Switzerland). mPEG5K-silane solution was prepared by diluting 200 mg of mPEG5K-silane in 10 mL of 95% ethanol.
Worm and bacteria culture and preparation
A single colony of Escherichia coli strain OP50 was used from the streak plate and injected into L-broth. The injected cultures were shaken at 37 °C overnight. The L-broth medium was removed after the overnight culturing by centrifugation. Freshly prepared and filtered S-medium was added and the suspension was vortexed to obtain a uniform bacterial distribution. The same procedure was also applied to obtain E. coli strain HT115 for the bacterial feeding of worms inside the microfluidic chip. An optical cell density meter was used to realize a stock concentration of 4 × 109 cells per mL of HT115 strain in S-medium. After the initial optimization of 4 × 109 cells per mL, further dilutions of this concentration in S-medium were performed to reach 2 × 109, 1 × 109 and 5 × 108 cells per mL bacteria concentrations. Nematode growth medium (NGM) plates were then seeded with this E. coli OP50 food source for C. elegans culturing at 20 °C. For L1 synchronization, an NMG plate with a mixed larvae population was suspended in a 1.5 mL Eppendorf tube. After 2–3 minutes, the top 100 μL of this suspension was extracted. As the older larval stages sink faster in a suspension, this extracted suspension contained mostly L1 nematodes. It was diluted accordingly in order to have 10 to 15 worms per 200 μL of S-medium for the worm distribution in the microfluidic lanes. 10 μL of mixed larvae suspension was injected onto a new NGM plate to continue culturing the strains for further experiments. Strains used were SJ4100 (hsp-6::gfp) and AM140 (punc-54::Q40::YFP) and were provided by the Caenorhabditis Genetics Center (University of Minnesota).
Fabrication of the microfluidic chip
We fabricated our microfluidic chips by soft lithography using single layer SU8 molds. Conventional photolithography was utilized to deposit a 65 μm thick layer of SU8 on 4 inch wafers. The SU8 mold was treated with trimethylchlorosilane (TMCS) in a vacuum chamber for 15 minutes to prohibit adhesion of PDMS during molding. A liquid PDMS mixture (base-to-curing agent ratio 10
:
1) was poured on the SU8 mold, degassed and cured at 80 °C for 2 hours. Once the PDMS mixture was cured, we removed the device from the SU8 mold and punched 1.5 mm inlets and outlets using a biopsy punch. Both the PDMS device and 75 mm × 38 mm glass slide were plasma activated and sealed together. After keeping the bonded microfluidic chip on a hotplate at 80 °C for 10 minutes to enhance the bonding, the chip was removed from the hotplate to continue the silanization process to prevent bacteria adhesion during life cycle culturing. All inlets and outlets of the chip were connected by tubing such that the whole 8-inlet and 8-outlet device was converted into a single inlet and single outlet version. A 1 mL borosilicate syringe was filled with 500 μL of mPEG5K-silane solution and connected to the microfluidic chip. An initial flow of 3 μL s−1 was set to remove bubbles and after having two thirds of the solution washed off at this speed, we dropped the flow rate to 4 nL s−1 and kept this configuration for 1.5 hours. These microfluidic operations were performed with Nemesys syringe pumps. We then flushed the microfluidic device with 1 mL of 95% ethanol and dried it with an air gun. This cycle was repeated three times to be sure that there were no silane crystals left. Subsequently, all the tube connections were removed and the microfluidic chip was placed inside a vacuum chamber for 1.5 hours. After removing the chip from the vacuum chamber, it was gently cleaned with an ethanol washed cleanroom wipe and it was ready for the experiment.
Experimental preparation
A 12-port rotary valve was connected to a Kloehn syringe pump and it was coupled to the microfluidic device (Fig. 1). The microfluidic device was mounted on a microscopy control system (Visitron, Puchheim, Germany). As the worms passed through different larval stages, the flow rates in the chips were adjusted, the camera was automatically triggered to take images or videos for each chamber, and the motorized stage was moved to the related positions by the script. During experimentation, the microfluidic chip was mounted on the motorized stage, having the 8 inlets connected to the syringe pump and 8 outlets interchangeably to the bacteria and worm reservoirs. The two additional ports of the 12-port valve were linked to waste and S-medium reservoirs, respectively. A 10 × (0.45 NA) objective was mounted on the setup, as the field of view through it completely covered the largest confined adult worm. The illumination source was alternated between white light for bright-field microscopy and a fluorescence excitation source for fluorescence microscopy. All the 32 unit cell positions were set by utilizing only two unit cell positions, which were implemented by a Python script. The camera exposure time, brightness and focal plane were adjusted each time regarding the type of study.
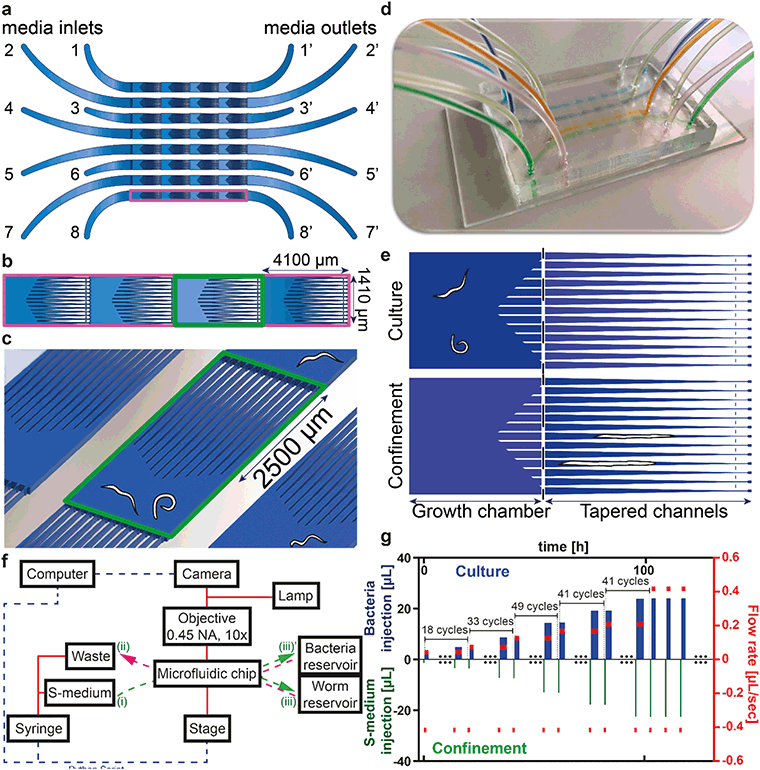 |
| Fig. 1 Details of the microfluidic platform for automated high-content phenotyping of C. elegans. (a) Schematic representation of the microfluidic chip with eight lanes, having each four unit cells, consisting of a growth chamber and tapered channels. Each lane has a media inlet (1 to 8) and outlet (1′ to 8′). (b) Zoom on one microfluidic lane consisting of four growth chambers and tapered channels, each unit cell being of rectangular shape (4100 μm × 1410 μm). (c) Three-dimensional schematic view on one unit cell, including young adult worms for size comparison, displaying the shortest tapered edge channel of 2500 μm. (d) Picture of the microfluidic chip (35 mm × 53 mm) bonded to a standard glass microscope slide (38 mm × 75 mm). (e) The two operation modes of the unit cell. Nematodes are kept in “culture” mode after the suction of fresh E. coli from the media outlets. In “confinement” mode, they are confined in the tapered channels for imaging and phenotyping. (f) Block diagram of the overall platform with indication of different connections made to the inlets ((i), (ii)) and outlets ((iii), (iii)′) (see text). (g) S-medium injection volumes during confinement (lower part of the plot and left axis) and bacteria injection volumes during culture (upper part of the plot and left axis) and the corresponding flow rates (red symbols and right axis). For example, in the first confinement step, 1.4 μL of S-medium is injected at a flow rate of 417 nL s−1. This step is followed by a 2.9 μL bacteria injection at a flow rate of 42 nL s−1 in the first culture step. The S-medium injection volume is gradually increased during worm development. Bacteria injection is always kept 1.5 μL more than the previous S-medium injection volume to retain E. coli uniformity. 18 cycles were applied with the same flow rate during culture, after which the flow rate increases. After 100 hours, the flow rate during culture no longer increases and remains constant. | |
Automated fluidic protocol
After the initialization of the experiment, the first confinement step was executed with S-medium injection from the media inlet (Fig. 1). After the worms were confined, they were released back and the first video recording was performed. A fresh bacteria solution was injected right after the video recording to keep the E. coli concentration in the chip uniform. The bacteria injection volume was always kept 1.5 μL more than the medium injection volume in order to retain bacteria uniformity in the chip. The medium injection volume was gradually increased over time, as the more advanced larval stages required more fluidic force to make the worms enter the tapered channels. It was also necessary to wash off late L1 progeny. Consequently, the bacteria injection volume was also incremented with the same amount in order to maintain a 1.5 μL media volume difference at all times. After 100 hours, there was no further requirement to increase the injection volume any more, as the worms then reached their adult stage; hence, the bacteria and medium injection flow rates and amount were preserved constant. Approximately every 35 minutes, images of the tapered channels with the confined worms were captured during the medium injection and, roughly every 7 hours, videos of the growth chambers were recorded right before the bacteria injection.
Automated operation of the platform
All types of studies had similar semi-automatic operation. Initially, filtered S-medium was used to fill the microfluidic chip at around 1.67 μL s−1. The same flow rate was utilized to degas the microfluidic chip having the outlet tubes clamped for approximately 15 minutes. After a successful degassing step, the clamps were released and tubes were placed inside the worm suspension. Worms were loaded inside the microfluidic chip with a flow rate of 0.6 μL s−1 and the desired number of worms was collected in front of the first filter set. Short injection pulses of 3.33 μL s−1 were required to distribute the worms among growth chambers by passive hydrodynamics. Filtered S-medium pushback was also required from time to time to send back excessive worms inside the chambers. When the desired number of worms in the chambers was reached (around one to three worms), the excessive amount of worms was pushed back with S-medium from the inlet side at a flow rate of 42 nL s−1. This flow rate was carefully chosen as at this point, trapped worms inside the chambers might move to the neighboring chambers, causing a deviation in the distribution. After having all the excessive worms pushed backed to the worm reservoirs, the bacteria reservoir was plugged into the media outlet. The same flow rate was used to cover the entire microfluidic lane with a uniform E. coli HT115 solution of 25 μL, having a direction from the bacteria reservoir to waste reservoir. We developed an automation script with Python to control the bacterial feeding amount and flow rates, confinement pushback amount and flow rates, stage positions, and image and video acquisition time instants throughout the experiment. After the first trigger of this Python script, the user did not need to communicate through the experiment anymore with the only exception of filling the bacteria reservoirs every night. The first confinement and image acquisition step took place, and after it was terminated, video recording started. This was followed by the first bacterial feeding and then continued with further confinement and feeding steps.
Automated image and video analysis
We developed an automated image and video analysis script with Matlab (MathWorks, Natick, MA, U.S.A.), including a custom-made GUI, to allow the user to extract the phenotyping results rapidly. The above-mentioned Matlab script and GUI served three purposes, namely bright-field confinement studies, fluorescence measurement and motility analysis. Depending on the computational power of the computer, the processing times can vary significantly. On average, confinement and fluorescence analyses can be finished in less than 8 hours for an average computer, whereas it will take 1–2 days for the motility analysis.
Motility phenotypes
We adjusted a motility tracking algorithm for C. elegans in M9 buffer for our study.25 Further details in the calculation of these phenotypes can be found in the related research of the same authors.44,45
Volume calculation, fluorescence intensity correction and data normalization
For the volume calculation, assuming that worms were approximately radially symmetric, we utilized the following formula:46 |
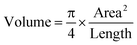 | (1) |
We determined the corrected value of the average fluorescence intensity with the following formula:
|
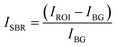 | (2) |
where
ISBR,
IROI and
IBG are the corrected, the average worm, representing the region of interest, and the average background fluorescence intensity values, respectively. We followed an initial background noise removal and then a standard signal-to-noise ratio (SNR) correction method.
For the normalization of the data in clustergrams, the following equation was used for all the individual parameters:
|
 | (3) |
where
POI,
PIN and
PCNT are the normalized parameter of interest, the parameter of interest and the corresponding control parameter, respectively.
Statistical analysis
Data from raw images were extracted to fill an array for statistical tests with Matlab (MathWorks, Natick, MA, U.S.A.). Fertility and dose response data were analyzed for statistical significance using one-way ANOVA. Motility and growth data of hsp-6::gfp worms were analyzed for statistical significance using Repeated Measures one-way ANOVA by taking the mean of the values at every 7 hours. Motility and growth data of punc-54::Q40::YFP worms were analyzed for statistical significance using a two-tailed student t-test by taking the mean of the values at every 7 hours. These statistical analyses were done with Graphpad Prism (Graphpad Software, San Diego, CA, U.S.A.). Mean values were computed to represent in graphs when measurements were repeated in multiple batches. For the DR study with the hsp-6::gfp worm strain, we had 18, 16, 21 and 18 worms for 4 × 109, 2 × 109, 1 × 109 and 5 × 108 cells per mL conditions, respectively. For the DR study with the punc-54::Q40::YFP worm strain, we had 20 and 18 worms for 4 × 109 and 5 × 108 cells per mL conditions, respectively. The worm distribution among each chamber for three experiments is illustrated in Fig. S1.†
Experimental
Microfluidic chip design
Our platform enables reversible immobilization for high-resolution imaging of C. elegans nematodes with, as additional features, the possibility of multiplexed studies and full automation. Therefore the user interaction can be reduced to a minimum level during the whole execution of the experiment. The system is based on passive hydrodynamics, without requiring additional active components on-chip. The latter contains eight lanes for performing multiplexed experiments, each lane having four unit cells, each of which consists of a growth chamber and thirteen tapered channels (Fig. 1a). Each of these microfluidic lanes has one inlet, which is connected to a syringe pump through a distributing valve, and one outlet, which is connected to either a worm or a bacterial reservoir. Each of the unit cells has a rectangular shape of 4100 μm × 1410 μm (Fig. 1b). The growth chamber size is carefully chosen such that up to three worms have enough space to be successfully cultured throughout their life cycle. These spacious growth chambers facilitate accommodation of enough bacterial food for the growth of the worms. The tapered channels were optimally designed so that the minimum channel length (2500 μm) was two times longer than the largest observed adult worm (∼1200 μm) to ensure full coverage of the nematode during imaging (Fig. 1c). We designed our channel entrances with a certain angle (∼30°) such that worms easily find their way inside the channels for immobilization with the least microfluidic flow increase. Thirteen channels are conjugated side-by-side to increase the possibility of confinement during high-resolution imaging (see details in Fig. S2†). The PDMS part has a size of 35 mm × 53 mm, so that a 38 mm × 75 mm-sized glass microscope slide can be used as the sealing part (Fig. 1d).
Platform working principle
A semi-automated experiment initialization protocol was established (see Fig. S3†). Synchronized L1 worms in S-medium with a concentration of 10–15 L1 worms per 200 μL of suspension were first injected into the chip through the media outlet of each microfluidic lane. In particular, L1 worms were collected in front of the first filter set and short injection pulses – by suction from the syringe side – were then utilized to distribute them among the chambers using passive hydrodynamics (i.e. not using active valves on the chip). When needed, we pushed the nematodes back to their prior chambers – by S-medium push from the syringe side – in order to get an even worm distribution among the chambers. As soon as the desired number of worms per chamber was reached, any worms remaining in front of the first filter set were pushed back at a flow rate of 42 nL s−1. The flow rate at this step was chosen so that the worms in the chambers did not exit. Successively, a tube containing 600 μL of E. coli suspension was connected to each microfluidic lane and all the lanes were covered with a uniform bacteria solution from the media outlet towards the media inlet. Right after, the sequence was initiated, with the first confinement cycle having fresh S-medium pushed from the media inlet towards the media outlet.
There are two operation modes after the initiation of an experiment (Fig. 1e). In the first part, E. coli injection is carried out from the media outlets, during which the worms are pushed towards the passive filters. Once this step is finalized, the worms are left inside the growth chambers for some dedicated amount of time to continue their development with the fresh bacterial solution. This operation mode is termed “culture” mode. During this operation, every 7 hours, videos were recorded for our motility study. In the second operation mode, namely “confinement”, roughly every 35 minutes, the worms are pushed towards the tapered channels for imaging and phenotyping. A pulsed microfluidic flow with one-second interruptions is utilized to confine the worms temporarily. If the confinement flow rate was too high (>417 nL s−1), the flow-induced viscous drag force exerted on the later stage larvae and adult nematodes moved them towards the end of the 8 μm-wide tapered channels. Hence, the nematodes were either tightly squeezed, which hindered their development, or they could inadvertently move to the neighboring growth chamber. Meanwhile, pulsed flow with one-second interruptions during the confinement enabled the worms to grow adequately and provided smooth repositioning of the worms inside the tapered channels. Our approach enabled confinement during all life stages of C. elegans (see Fig. S4†).
A block diagram of the experimental setup is displayed in Fig. 1f. A custom Python script was written to automate and synchronize the operations of the camera, the syringe pump and the motor stage with the microfluidic chip. This permits the full automation of the experiment with no need for user intervention, potentially throughout the whole duration of the worms' life cycle. Chip connections (i)–(iii)′, (i)–(iii), (ii)–(iii)′, and (ii)–(iii) are used for confinement with fresh S-medium injection, gentle L1 push back after the initial L1 distribution and fresh S-medium loading, E. coli injection and worm injection – distribution, respectively. We represent the used flow profiles over time in Fig. 1g. Despite having more than 200 confinement–culture cycles in an experimental run, for illustrative purposes, we only represent the experimental flow profile during a few of the cycles. Initially, we selected the ideal confinement S-medium flow rate (417 nL s−1) and injection volume (1.4 μL) by manual operator tests for L1 worms, so that the latter were laterally confined but at the same time not be pushed to the neighboring chamber (lower bars of Fig. 1g). If the confinement flow rate was higher than the chosen 417 nL s−1, we observed that late stage larvae and adult nematodes would be squeezed through the 8 μm filters. When applying the same S-medium flow rate and injection volume to the other larval stages, we could no longer force larger worms to enter into the confinement channels. We therefore linearly increased the injection volume over time but did not increase the confinement flow rate. Consequently, the duration of the confinement progressively increased in a linear fashion, starting from around 1 minute from the first confinement cycle (=width of the first green bar). After 100 hours, when the worms had reached their largest size, we no longer increased the S-medium injection volume per cycle. After each confinement cycle, a culture cycle started during which bacteria were injected from the other side of the chip (upper bars of Fig. 1g). We used initially a flow rate of 42 nL s−1 and kept in each cycle the bacteria injection volume 1.5 μL higher than the previous S-medium injection volume to ensure a uniform E. coli concentration everywhere. Therefore the duration of the bacteria injection (=width of a blue bar) was increasing too over time, starting from an initial duration of 10 minutes. After bacterial injection, the nematodes were left in culture for 24 minutes until the next confinement cycle. The flow rates of bacteria injection during culture were increased in steps from 42 nL s−1 to 73, 125, 167, 208 and 417 nL s−1 after 18, 33, 49, 41 and 41 cycles, respectively.
Automated post-experiment image and video analysis
Automated image and video analyses were performed after the finalization of the experiment. All the analyses were implemented with Matlab (MathWorks, Natick, MA, U.S.A.). The length and area of a worm were obtained from the raw bright-field images that were captured during the confinement of nematodes in the tapered channels (Fig. 2a). The first step was to create a dynamic background that provided the best result for the isolation of the confined worms (see details in Fig. S5†) and subtract it from the raw image. After the binarization of the intensity values, the confined worms and the remaining background clusters were marked (Fig. 2b). The brightness-adjusted image was thresholded in order to remove background clusters and the largest connected components matching the number of worms accommodated in the related chamber were automatically detected (Fig. 2c). The total pixel number of each component was scored as the area. Morphological operations were applied to obtain the splines that were spatial fits to the worms. The total pixel number of the spline that corresponded to a worm was scored as the length of the latter.
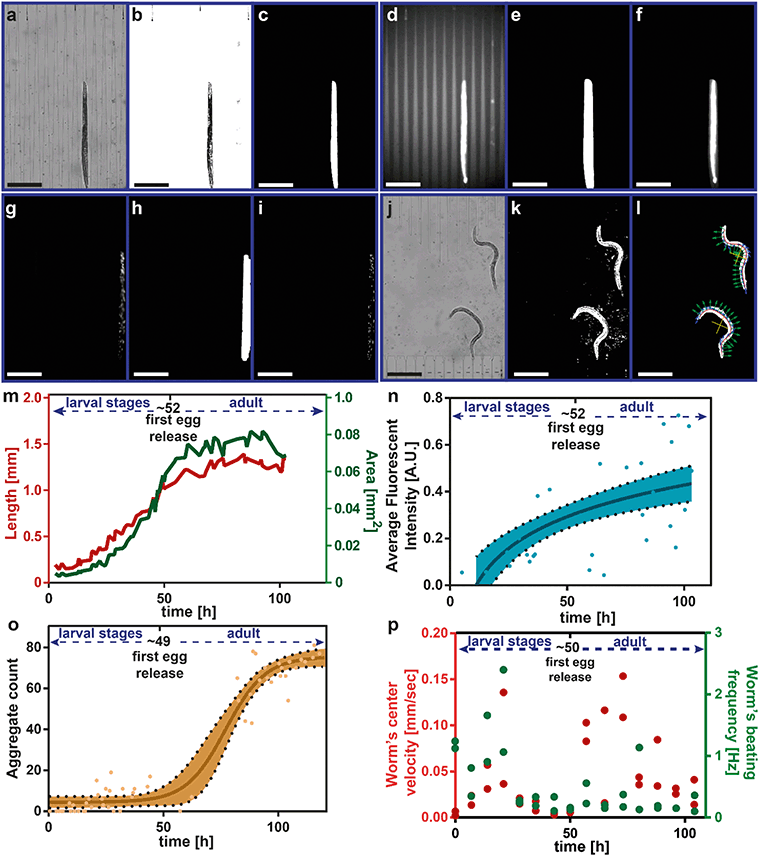 |
| Fig. 2 Automated image and video analysis for extraction of worm phenotypes. (a–c) For worm's length and area measurement, a raw bright-field image is captured, background-subtracted, and thresholded. The algorithm is forced to find the largest connected components matching to the number of worms accommodated in that chamber. (d–f) In parallel, a raw fluorescence image is taken and multiplied with the dilated version of the thresholded image (c) obtained from the bright-field confinement, thereby acting as a binary mask to the fluorescence raw image (d) for quantifying the fluorescence intensity inside the hsp-6::gfp worms. (g–i) A similar procedure is also applied for analyzing fluorescent protein aggregates in punc-54::Q40::YFP worms, but this time after application of intermediate steps of Laplacian of Gaussian filters, followed by a thresholding step. (j) Screenshot of a video that was captured for 10 seconds at a rate of 5 frames per second for motility analysis. (k and l) After background subtraction, an automatic algorithm detects the locations of worms in a growth chamber, after which the worms' positions are fitted with splines enabling quantification up to 12 swimming parameters per worm. (m–p) Typical results obtained for a single worm's length and area, average fluorescence intensity, aggregate count, worm's center velocity, and beating frequency, after analysis of the cases introduced in (a–c), (d–f), (g–i), and (j–l), respectively. Semilog and sigmoidal interpolation lines are used for the average fluorescence intensity (R2 = 0.43) and aggregate count (R2 = 0.95) measurements, respectively, in order to remove outliers. Scale bars: 400 μm. | |
We analyzed two different strains, having slightly different fluorescence signal detection approaches, i.e. the mitochondrial unfolded protein response reporter hsp-6::gfp strain47 –which essentially behaves like a wildtype (WT) strain of worms – and the HD model punc-54::Q40::YFP.43 Along with bright-field images, at each confinement instance, we also acquired fluorescence images (Fig. 2d). In the first part, we wanted to observe the maximum and average fluorescence signal expression inside the nematodes. For this purpose, we dilated the resultant image from the bright-field image analysis (Fig. 2e) and used it as a mask for the fluorescence image. Our goal was to not unintentionally discard the fluorescence intensity values for hsp-6::gfp or aggregate clusters for punc-54::Q40::YFP nematodes on the nematode edges when the chosen mask was too tight. We directly multiplied those two images so that we would only locate the worm of interest and avoid false fluorescence signal detection from the background (Fig. 2f). An inverted version of the worm mask was also multiplied with the raw fluorescence image separately to quantify the average background intensity and to calculate the actual intensity value (see details in Fig. S6†). A similar approach was adapted for the HD model in which a quantification of the protein aggregates was required (see details in Fig. S7†). A fluorescence image was captured (Fig. 2g) and multiplied with a dilated version of the confinement result converted to a mask (Fig. 2h). Herein, we applied a Laplacian of Gaussian filter (σ = 10), which was followed by an image binarization step29 and the resultant image is depicted in Fig. 2i.
Approximately every 7 hours, videos at 5 Hz for 10 seconds were recorded. A frame of the video stack is shown in Fig. 2j. For video analysis, we adapted a software algorithm, which was previously proposed to quantify the motility of C. elegans in M9 buffer medium.25 Similar to the bright-field confinement image processing, a dynamic background subtraction was required to isolate the nematodes in the chambers. For this, we created a dynamic background image combining the median of the video frames of interest and the median of all the video frames (see details in Fig. S8 and S9†). After the background removal, each frame was thresholded (Fig. 2k), smoothed and forced to detect the number of the largest connected components matching to the worms accommodated in that chamber. A worm in the chamber was detected and skeletonized; the center point of the worm (shown as yellow), the tangential (shown as blue) and perpendicular components (shown as green) of each skeleton point were denoted (Fig. 2l). All these data were combined to extract the central velocity, beating frequency (also known as “locomotor frequency”), head, center and tail beating amplitudes, minimum, mean and maximum curvature, bending wavelength and bending wave amplitude change in time (wave speed), bending force and mechanical power.
For each of the recorded results, we also annotated the first egg release times, which were obtained from the confinement images by operator-observation of the first egg that was trapped in the tapered channels. We present a single worm phenotypic analysis in Fig. 2m–p. The raw data from each algorithm required some post-adjustment to obtain reliable data that prevented incorrect detection. Our method was to check the closeness of neighboring data points. For this purpose, we computed the difference of a measurement point of interest to the three previous time point measurements. If three different difference values were in a certain interval, the data point was counted as a reliable measurement; otherwise, it was classified as an outlier and discarded. Our method discarded, on average, 72% of the overall data. The main reason for such a high discarding rate is due to mainly (i) the low confinement accuracy for the adults after 100 hours of experiments, and (ii) assurance that no false data were included, which eventually results in correct measurements being discarded as well. During the development of our platform, we therefore systematically performed operator-based visual controls to ensure the correctness of our method. The resultant data were recorded (Fig. 2m) and used later to combine with other worms grown under the same conditions. Similarly, we discarded incorrect average fluorescence intensity values by deselecting eventual outliers at any time scale (Fig. 2n). The remaining average fluorescent intensity data was plotted and a semi-log line was fit. This raw data was again combined with the other raw data of the worms that were grown under the same conditions. By identifying the incorrect length and fluorescence measurements and, hence, by excluding the related measurement times with our method, we obtained the corrected aggregate count plot in time (Fig. 2o). A sigmoidal fit was performed on the corrected data. The worm's center velocity and beating frequency required a manual approach to correct the raw data, as worms could alternate periods of activity and rest (Fig. 2p). We plotted all data in time and whenever the spline fit was rather poor in a single video recording, we manually discarded these data.
Results and discussion
High-content phenotyping of worms at various concentrations of bacterial food
In many animal species such as rats, monkeys, yeast cells, Drosophila melanogaster, C. elegans, and mice that were tested so far, DR has been shown to slow down the aging process, resulting in longer maintenance of health and an extended mean and maximum lifespan.37,39,48–51 Interestingly, DR not only promotes lifespan extension but also decreases the occurrence of age-dependent diseases, including neurodegeneration.52 Among all the model organisms employed in this field, C. elegans continues to serve as a powerful model of longevity induction caused by DR.53 As a proof-of-concept for our technology, we intended to use C. elegans and our microfluidic platform in order to better define the effect of DR on neurodegenerative diseases.
We first wanted to determine the ideal bacterial dilution that could be provided in the microfluidic platform for establishing appropriate DR conditions on C. elegans growth and motility (Fig. 3). We have selected four different E. coli concentrations, namely, 4 × 109, which corresponds to the ad libitum (AL) feeding condition, 2 × 109, 1 × 109 and 5 × 108 cells per mL.41,54 DR is known to delay the growth rate and to reduce the fertility in numerous organisms including C. elegans,38 but very little is known about the timing resolution of DR effects during the development. In order to explore the impact of DR during development, we first investigated the timing of first egg laying by visually checking the tapered channels for the presence of a first confined egg. The first temporal instance when an egg was observed was recorded and compared for different E. coli feeding concentrations (Fig. 3a). The first egg release time demonstrated a similar profile for the highest two feeding conditions and a 5% time increase was obtained for the lowest feeding condition. In addition, we quantified the embryo release rate by subtracting the number of embryos trapped in the initial frame in which the laid embryo was first noticed from the fifth following frame, then dividing it by the number of worms accommodated and the time interval between those two frames (Fig. 3b). This extrapolated data delivered an estimation of the progeny release rate under various feeding conditions. In accordance with previous works done on NGM plates, we observed that DR increases the egg release rate.55 By merging the corrected data from the confinement in tapered channels (both bright-field and fluorescence), we observed the influence of bacterial dilution on the length, area and volume (see “Materials and methods”).
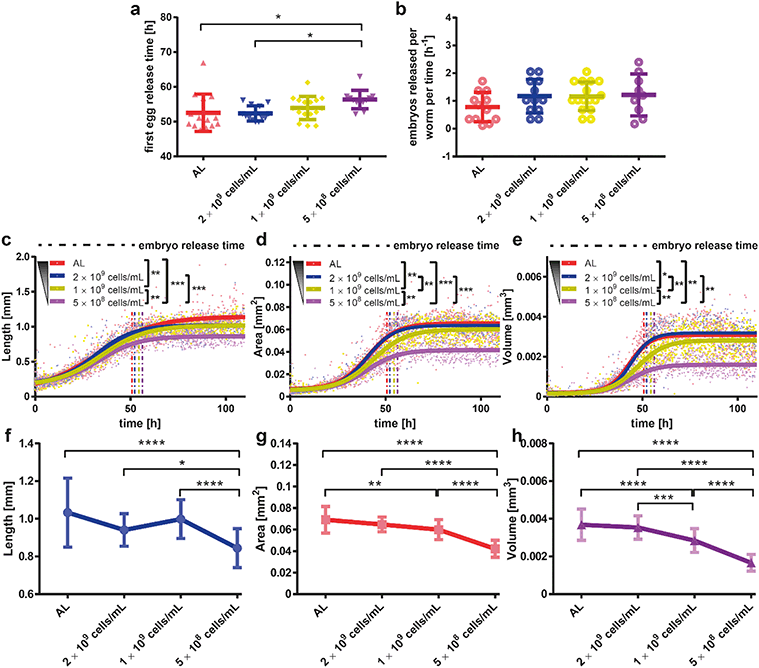 |
| Fig. 3 Study of the effect of E. coli concentration on the worm development of hsp-6::gfp worms. (a and b) The first egg release time and embryos released per worm per time results obtained from the embryo collection inside tapered channels. (c–e) Length, area and volume obtained by feeding with different bacteria concentrations. A sigmoidal fit is utilized for length, area and volume estimation. R2 values representing the quality of sigmoidal fits are calculated to be [0.87, 0.92, 0.93, 0.89], [0.89, 0.94, 0.92, 0.87], and [0.81, 0.89, 0.87, 0.81], for the feeding conditions [AL, 2 × 109 cells per mL, 1 × 109 cells per mL, 5 × 108 cells per mL] from panel (c) to (e), respectively. The average first embryo release time was also plotted as a vertical dashed line to indicate the transition from the larval stages to the adult stage. (f–h) The dose response curve of length, area and volume obtained at 80 hours by feeding with different bacteria concentrations. Data are expressed as mean ± SEM, * p ≤ 0.05, ** p ≤ 0.01, *** p ≤ 0.001, **** p ≤ 0.0001. All measurements are based on N = 18 to 24 worms. | |
The maximum worm length decreases with bacterial food dilution in a dose-dependent manner (Fig. 3c). Interestingly, the area of the worms demonstrated a different growth dynamics (Fig. 3d). For AL E. coli feeding, with 2 × 109 cells per mL and 1 × 109 cells per mL concentrations, the maximum area reached by the worms at the adult stage was similar. However, a 10 hour time delay in the worm area growth was typically observed for the 1 × 109 cells per mL condition. This piece of information would have been missed if we could not follow the full dynamics of the area increase as enabled by our platform. Moreover, for the 5 × 108 cells per mL feeding condition, the worms were much smaller at all stages compared to any other feeding condition. The maximum area a worm could cover with this feeding was in fact almost half of that of a properly fed worm. A similar trend was also detected for the worm volume (Fig. 3e). In comparison to previous studies,54 we could confirm a similar length and volume profile, and hence, possibly the same area results.
We extracted the dose-dependent curves of the length (Fig. 3f), area (Fig. 3g) and volume (Fig. 3h) at 80 hours of development. Besides the length curve, we observed a dose-dependent sigmoidal decrease in the parameters, which demonstrates the effect of the E. coli concentration on the worms' growth. Real time images of growth chambers at 65 hours were captured and detailed to demonstrate the developmental difference of worms under different feeding conditions (Fig. 4a–d). At the time, when all the worms reached the adult stage and laid embryos, there was a significant size difference among the worms that were raised under different feeding conditions.
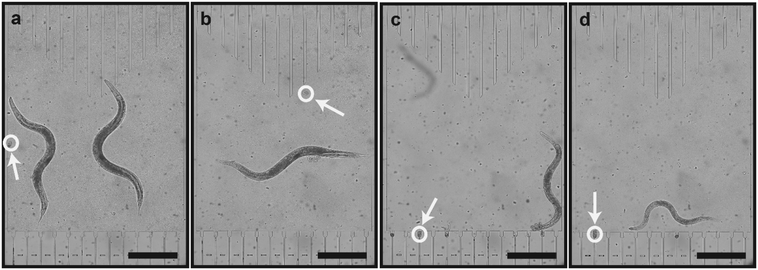 |
| Fig. 4 Representative real-time growth chamber images of wild-type hsp-6::gfp nematodes under (a) 4 × 109 cells per mL (AL), (b) 2 × 109 cells per mL, (c) 1 × 109 cells per mL, and (d) 5 × 108 cells per mL E. coli concentrations at 65 hours with eggs laid indicated. Scale bars: 400 μm. | |
In order to extract more information about the impact of DR on the worm physiology, we monitored the modulation of the mitochondrial unfolded protein response (UPRmt) upon DR treatment. The mitochondrial function as well as its associated stress response is considered today as a pivotal player for aging regulation, but the role of the so-called UPRmt in DR is not well understood so far. We employed our automated fluorescence image analysis algorithm to quantify the expression of the hsp-6::gfp reporter for evaluating the induction of the UPRmt (see details in Fig. S10†). Interestingly, DR has no significant effect on UPRmt, confirming previous observation using a genetic model of DR in C. elegans.56 Of note, we observed 50% reduction in the intensity of the fluorescence signal in the adult population subjected to the lowest concentration of food, indicative of less stress in mitochondria.
DR is also reported to have an effect on motility behavior.57 The central velocity (Fig. 5a) and the beating frequency (Fig. 5b) of the worms were rather invariant at the early larval stages. The influence of different feedings became more significant after 20 hours of development. At 1 × 109 cells per mL feeding, the worms were more inclined to move around inside their chamber, likely pushed by the need for more food. In line with this speculation, at AL and 2 × 109 cells per mL feeding, the worms were not so mobile. A variation of the motion at 5 × 108 cells per mL feeding was observed. Because of poor feeding, the worms remained smaller at this E. coli concentration and, similarly, their movement speed was reduced compared to the 1 × 109 cells per mL feeding condition, where the movement was most intense. The head (Fig. 5c) and center (Fig. 5d) beating amplitudes were also detected and showed a similar effect that confirmed this phenomenon. The variation in the beating amplitudes was more pronounced after 35 hours. We also performed a feeding dose-dependent analysis for these four motility parameters (see details in Fig. S11†) to observe the effect of DR on the motility.
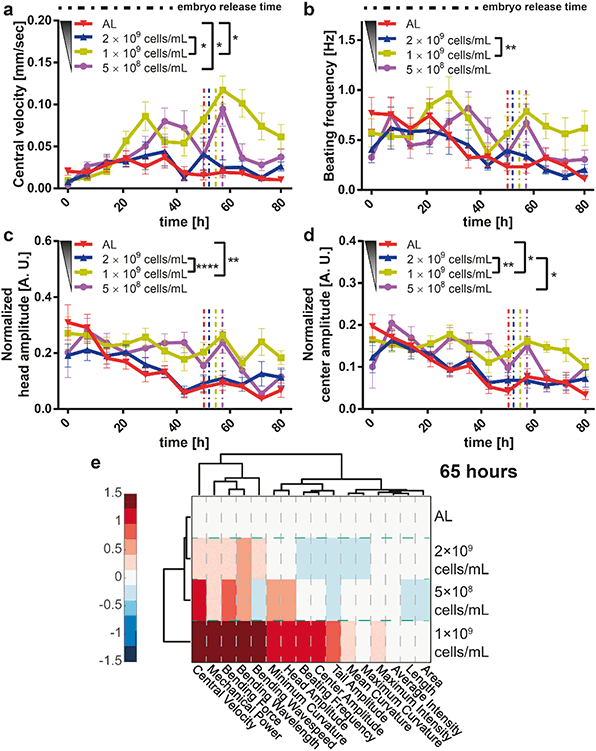 |
| Fig. 5 Study of the effect of E. coli concentration on the motility of hsp-6::gfp nematodes. (a–d) Comparison of four motility parameters, namely the worms' center velocity, beating frequency, and normalized head and center beating amplitudes after feeding with different E. coli concentrations. The average first embryo release time was also plotted as a vertical dashed line to indicate the transition from the larval stages to the adult stage. Data are expressed as mean ± SEM, * p ≤ 0.05, ** p ≤ 0.01, *** p ≤ 0.001, **** p ≤ 0.0001. (e) Clustergram comparison of motility and development parameters obtained at 65 hours after the first bacteria injection on the chip. Different E. coli concentrations fed are indicated. All measurements are based on N = 18 to 24 worms. | |
In order to compile all together the generated data and facilitate the visualization of eventual variations in the motility and growth parameters in time, we built clustergram profiles with the normalized data – see “Materials and methods” – (Fig. 5e). At 65 hours of development, the isolation of different feeding conditions was not significantly different except for the 1 × 109 cells per mL concentration. The 5 × 108 cells per mL feeding condition's movement parameters approached the remaining two feeding conditions around this time point. We hence speculate that the relatively small size of worms under the most severe DR conditions caused an alteration in the swimming motion (see also Fig. S12† for additional time points). From these data, it was clear that DR has a significant impact on the motility parameters and an adverse influence on the growth and fertility parameters.
Influence of DR on a Huntington's disease worm model
DR is a relevant intervention to increase the resistance of the nervous system to age-related neurodegenerative disorders.58 Based on previous observations, we wanted to analyze the effect of DR on a worm model of neurodegenerative disease. HD is one of the most common genetically determined neurodegenerative diseases, with approximately 90% of HD cases being hereditary and transmitted in an autosomal dominant fashion.59 This disease belongs to a group of neurological disorders caused by expanded cytosine–adenine–guanine (CAG) triplet repeats encoding self-aggregating polyglutamine (polyQ) tracts in their respective proteins. In the case of HD the polyQ expansion is in the huntingtin gene.60 In order to model HD in worms, we used a C. elegans strain that carries the transgene punc-54::Q40::YFP resulting in the expression of the fusion protein Q40::YFP specifically in worm muscle cells, causing an age-dependent aggregation phenotype whose readout is progressive motility deficient.43 Based on the results obtained in WT worms, we selected two E. coli feeding conditions, i.e. 4 × 109 cells per mL (ad libitum AL) and 5 × 108 cells per mL (DR), which demonstrated the most significant difference between proper feeding and settling the DR condition. The feeding at 5 × 108 cells per mL yielded more phenotypic changes in the worms as opposed to the feeding at 1 × 109 cells per mL, in which worms were smaller but still active. Therefore, a 5 × 108 cells per mL feeding concentration was chosen as the ideal condition for DR studies.
We extracted the growth and motility parameters and studied the polyQ aggregation in a time-dependent manner (Fig. 6). The length (Fig. 6a), area (Fig. 6b) and volume (Fig. 6c) of the HD worms displayed a similar profile to that in the WT strain. The HD worms fed with the AL concentration were longer and larger in terms of area and volume throughout the whole study compared to the worms that were on DR. The total aggregate number (Fig. 6d) and the total aggregate size (Fig. 6e) were then quantified. Interestingly, HD worms subjected to a DR regimen have a significant reduction of the total aggregate number and total aggregate size compared to HD worms fed with AL. Furthermore, the average aggregate size in time (Fig. 6f), which was calculated as the total aggregate area size divided by the total number of aggregates, showed a similar behavior. Indeed, the average aggregate size of the worms under DR were all the time, except during the initial few hours, smaller than the ones under AL conditions. We also calculated the aggregate number per length, which corresponds to the total number of aggregates divided by the length of the worm (Fig. 6g). This plot established that, as the worms grow in time, the feeding has less of an influence and the aggregate number per length saturates. We thereby demonstrated that DR has a progressive influence on the delay of protein aggregation and size of HD worms, suggesting that the disease is significantly attenuated by food restriction. The progressive loss of motility in the HD worm model is a well-established phenotype.61 We observed that the central velocity (Fig. 7a) and the beating frequency (Fig. 7b) of the HD worms were significantly improved under the DR regimen. Indeed, the restricted worms were moving faster and with a greater beating frequency than the ones that were fed with a higher E. coli concentration. Interestingly, head (Fig. 7c) and center (Fig. 7d) beating amplitudes did not show much of a difference. In the early larval stages, the beating amplitudes were similar and only between 20 and 60 hours were the worms fed with the low E. coli concentration more mobile. After 60 hours, the worms with a low E. coli concentration were disturbed and the beating amplitude patterns became invariant once more. This indicated that the curvature of the body remains similar whereas the movement speed and beating frequency increase under the DR regimen. Similar to the aggregation, the motility was also improved with less feeding, reflecting the attenuated development of the disease.
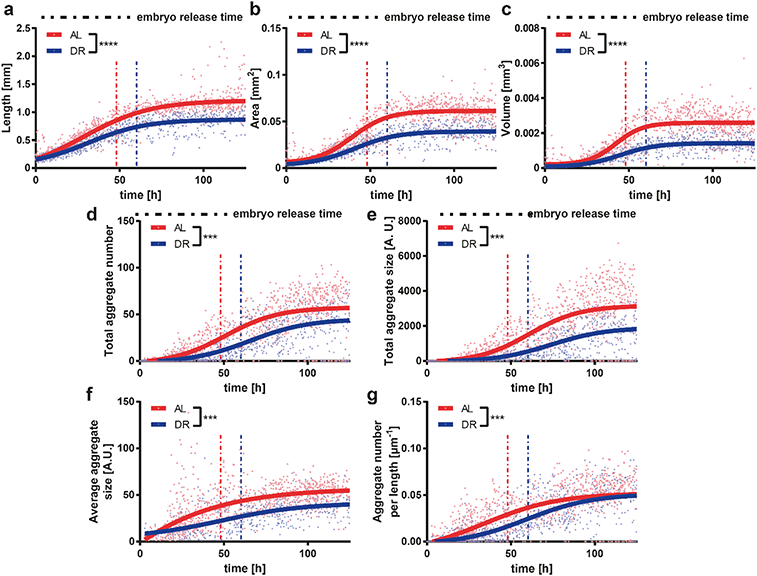 |
| Fig. 6 Study of the effect of E. coli concentration on the longitudinal and aggregate growth of the punc-54::Q40::YFP mutant worms, a model for HD. (a–c) Length, area and volume obtained by feeding worms with two different bacteria concentrations. (d–g) Total aggregate number, total aggregate size, average aggregate size and aggregate number per worm's length, obtained by feeding with two different bacteria concentrations. The average first embryo release time was also plotted as a vertical dashed line to indicate the transition from the larval stages to the adult stage. A sigmoidal fit is utilized for all the plots. R2 values representing the quality of sigmoidal fits are calculated to be [0.86, 0.84], [0.85, 0.84], [0.76, 0.78], [0.66, 0.66], [0.64, 0.58], [N/A, 0.34], and [0.57, 0.63], for the feeding conditions [AL, DR] from panel (a) to (g), respectively. Data are expressed as mean ± SEM, *** p ≤ 0.001, **** p ≤ 0.0001. All measurements are based on N = 18 to 20 worms. | |
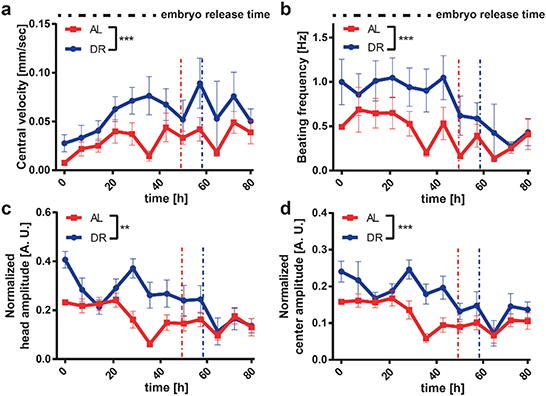 |
| Fig. 7 Study of the effect of E. coli concentration on the motility parameters of punc-54::Q40::YFP mutant worms. (a–d) Comparison of four motility parameters including, central velocity, beating frequency, and normalized head and center amplitudes of the nematodes for two different E. coli concentrations. The average first embryo release time was also plotted as a vertical dashed line to indicate the transition from the larval stages to the adult stage. Data are expressed as mean ± SEM, *** p ≤ 0.001, **** p ≤ 0.0001. All measurements are based on N = 18 to 20 worms. | |
We again decided to look at clustergram plots in time to spot the variance of motility, development and aggregate parameters for the two feeding conditions. The AL feeding concentration was used as the control source and DR results were normalized accordingly, hence only normalized DR results were displayed. At 21 hours (Fig. 8a), there were not yet enough features to distinguish the worms under two different E. coli concentrations. The first distinguishable features were seen at 35 hours (Fig. 8b), when HD worms under DR feeding have greater mechanical power, central velocity, beating frequency, bending force and fewer aggregates compared to the AL condition. At 43 hours (Fig. 8c), the DR worms demonstrated an increase in all motility parameters, which could be considered as an improvement in the disease attenuation. Central velocity, beating frequency and aggregate clusters were most distinctive among the worms under DR at 65 hours (Fig. 8d). Additional development features, such as the length and area, were also different at 65 hours between the 2 conditions. These clustergrams hence demonstrated that, in addition to a reduction in protein aggregation, there were also significant improvements in swimming performance.
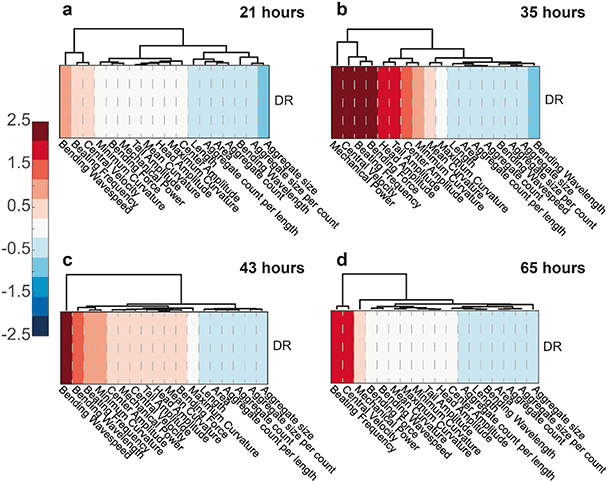 |
| Fig. 8 Clustergram comparison of motility and development parameters obtained at (a) 21, (b) 35, (c) 43 and (d) 65 hours after the first bacteria injection on the chip, respectively. All measurements are based on N = 18 to 20 worms. | |
Conclusions
Here we described a new microfluidic platform for multiplexed high-content phenotyping studies in a fully automated way. To the best of our knowledge, no other platform provided such automated phenotyping over the worm life cycle. Different microfluidic chambers were independently configured for different conditions, which allowed multiple cases to be studied side-by-side. The platform's high-resolution imaging capacity furthermore enabled 19 phenotyping results to be accurately extracted almost in real time. We optimized the experimental conditions such that the worms could be kept for more than 120 hours on a chip without any bacterial clogging. The initial silanization process and the parallel integration of multiple filter channels were key to the well-controlled bacterial concentrations in the microfluidic chip. This feature enabled the culture and phenotyping of the worm population during the entire larval development and several days after the adult stage – more particularly up to around three days of the adult stage – without any problem and any human intervention. As an average over all experiments, we observed a 30% confinement rate. Thanks to the adequate bacterial supply, we did not observe any stress-related phenotypes such as larval death, bagging or arrest among animals. Additionally, we did not notice any L1 injury during the initial L1 distribution and all the nematodes completed their life cycle. We retained all animals from the beginning of the experiment till the end. We had an average of 88% occupancy rate of chambers in all experiments, with 71%, 24%, and 5% of the chambers occupied by a single worm, two and three worms, respectively. Moreover, the high microfluidic flow rates and injection volumes during the adult stage confinement enabled us to easily wash off late L1 progeny at 100% efficiency and thereby allowed to keep track of the identity of the same worm from the very beginning.
A significant advantage of our platform is that it does not include any active on-chip components. We also avoided complex micro-fabrication steps to provide a reliable and easy-to-operate microfluidic chip. Instead of using alternative immobilization approaches that required, on-chip valves or chemical treatment, we integrated tapered confinement channels for the temporary and reversible immobilization of the worms. Unlike previously proposed microfluidic platforms with integrated tapered channels, we optimized the channel size such that we could confine all larval stages in a single channel geometry.
Furthermore, our platform allowed us to reduce the need for user interaction with very limited requirements for the experimental setup. Once the user initiated the experiment with semi-automated commands, there was no longer a need for any communication with the only exception of bacteria refilling if needed. The semi-automated experimental pipeline also diminished external operator-based variations in the experiments and increased the reproducibility. Also, we built an integrated, automated and fast image and video analysis tool. We extracted up to twelve swimming gait properties, three development properties, two fertility parameters and up to four fluorescent phenotypes of the worms. We could combine all the phenotypic data obtained from bright-field and fluorescence image acquisition and video recording to create clustergrams to provide in-depth analysis at each time point of worm development. Thanks to the clustergrams, we were able to isolate the most or least dominant parameters of different feeding conditions.
In order to validate our platform and its applicability to biomedical research, we studied the effect of an environmental challenge (i.e., food concentration) on the development of a complex neurodegenerative disease. For this purpose, we employed a C. elegans model of HD, which is shown to mimic the disease progression in humans.62 By combining the possibility to accurately control the food concentration in our system and to perform high-content analysis, we monitored the change in growth, reproduction, protein aggregation, motility and disease progression by feeding the worms with four different concentrations of E. coli. As previously reported, DR increased the worm motility, and retarded the growth and fertility. In addition, DR delayed the onset and progression of protein aggregation disease in a HD worm model that we used, highlighting the potential utility of dietary interventions in the setting of proteotoxic neurodegenerative diseases.
In summary, we provided an integrated, automated, multiplexed, long-term culturing platform with automated image and video analysis tools for the collection, extraction, and analysis of phenotypic data. Our platform hence allowed high throughput and high-content phenotypic analysis of C. elegans strains. We expect that such a platform will stimulate the use of C. elegans as a model organism for longitudinal and dynamic studies, starting from the early larval stages onwards.
Author contributions
H. B. A. and M. C. designed the platform, H. B. A., M. C. and L. M. designed the experiments, H. B. A. designed and fabricated the microfluidic chip, H. B. A. performed the experiments, L. M. provided the worms, H. B. A. prepared the automated image and video processing algorithms and analyzed the data, H. B. A., M. C., L. M., J. A. and M. G. wrote the paper.
Conflicts of interest
The authors declare no conflict of interest.
Acknowledgements
Work in the MG laboratory was supported by the Ecole Polytechnique Fédérale de Lausanne and the EU Ideas Program (ERC-2012-AdG-320404). We thank the staff of the Center of Micro- and Nanotechnology of EPFL for assistance in microfabrication processes, P. Gönczy and M. Pierron (EPFL) for sharing the reagents, and the Caenorhabditis Genetics Center, which is funded by the NIH Office of Research Infrastructure Programs (P40 OD010440), for providing worm strains. The research in the JA laboratory is supported by the EPFL, NIH (R01AG043930), Systems X (SySX.ch 2013/153), Velux Stiftung (1019), and the Swiss National Science Foundation (31003A-140780).
References
- J. El-Ali, P. K. Sorger and K. F. Jensen, Nature, 2006, 442, 403–411 CrossRef CAS PubMed
.
- H. Andersson and A. Van den Berg, Sens. Actuators, B, 2003, 92, 315–325 CrossRef CAS
.
- S. Le Gac and A. van den Berg, Trends Biotechnol., 2010, 28, 55–62 CrossRef CAS PubMed
.
- D. Di Carlo and L. P. Lee, Anal. Chem., 2006, 78, 7918–7925 CrossRef CAS PubMed
.
- F. Yang, Z. Chen, J. Pan, X. Li, J. Feng and H. Yang, Biomicrofluidics, 2011, 5, 024115 CrossRef PubMed
.
- D. Choudhury, D. van Noort, C. Iliescu, B. Zheng, K.-L. Poon, S. Korzh, V. Korzhgf and H. Yu, Lab Chip, 2012, 12, 892–900 RSC
.
- M. Gershow, M. Berck, D. Mathew, L. Luo, E. A. Kane, R. Carlson and A. D. T. Samuel, Nat. Methods, 2012, 9, 290–296 CrossRef CAS PubMed
.
- E. Brouzes, M. Medkova, N. Savenelli, D. Marran, M. Twardowski, J. B. Hutchison, J. M. Rothberg, D. R. Link, N. Perrimon and M. L. Samuels, Proc. Natl. Acad. Sci. U. S. A., 2009, 106, 14195–14200 CrossRef CAS PubMed
.
- M. Markaki and N. Tavernarakis, Biotechnol. J., 2010, 5, 1261–1276 CrossRef CAS PubMed
.
- S. Brenner, Genetics, 1974, 77, 71–94 CAS
.
- E. Kim, L. Sun, C. V. Gabel and C. Fang-Yen, PLoS One, 2013, 8, 1–6 CrossRef
.
- N. Chronis, M. Zimmer and C. I. Bargmann, Nat. Methods, 2007, 4, 727–731 CrossRef CAS PubMed
.
- C. B. Rohde, F. Zeng, R. Gonzalez-rubio, M. Angel and M. F. Yanik, Proc. Natl. Acad. Sci. U. S. A., 2007, 104, 13891–13895 CrossRef CAS PubMed
.
- S. Mondal, S. Ahlawat, K. Rau, V. Venkataraman and S. P. Koushika, Traffic, 2011, 12, 372–385 CrossRef CAS PubMed
.
- W. Keil, L. M. Kutscher, S. Shaham and E. D. Siggia, Dev. Cell, 2017, 40, 202–214 CrossRef CAS PubMed
.
- S. Berger, E. Lattmann, T. Aegerter-Wilmsen, M. Hengartner, A. Hajnal, A. Demello, X. Casadevall and I. Solvas, Lab Chip, 2018, 18, 1359–1368 RSC
.
- W. Shi, J. Qin, N. Ye and B. Lin, Lab Chip, 2008, 8, 1432–1435 RSC
.
- M. Cornaglia, G. Krishnamani, L. Mouchiroud, V. Sorrentino, T. Lehnert, J. Auwerx and M. A. M. Gijs, Mol. Neurodegener., 2016, 11, 17 CrossRef PubMed
.
- J. Krajniak and H. Lu, Lab Chip, 2010, 10, 1862–1868 RSC
.
- H. Bringmann, J. Neurosci. Methods, 2011, 201, 78–88 CrossRef PubMed
.
- W. E. Pittman, D. B. Sinha, W. B. Zhang, H. E. Kinser and Z. Pincus, Lab Chip, 2017, 17, 3909–3920 RSC
.
- M. A. Churgin, S. K. Jung, C. C. Yu, X. Chen, D. M. Raizen and C. Fang-Yen, eLife, 2017, 6, 1–25 CrossRef PubMed
.
- J. Sznitman, P. K. Purohit, P. Krajacic, T. Lamitina and P. E. Arratia, Biophys. J., 2010, 98, 617–627 CrossRef CAS PubMed
.
- C. Wählby, L. Kamentsky, Z. H. Liu, T. Riklin-Raviv, A. L. Conery, E. J. O'Rourke, K. L. Sokolnicki, O. Visvikis, V. Ljosa, J. E. Irazoqui, P. Golland, G. Ruvkun, F. M. Ausubel and A. E. Carpenter, Nat. Methods, 2012, 9, 714–716 CrossRef PubMed
.
- P. Krajacic, X. Shen, P. K. Purohit, P. Arratia and T. Lamitina, Genetics, 2012, 191, 1015–1021 CrossRef PubMed
.
- L. Lin and H. Chuang, PLoS One, 2017, 12, 1–14 Search PubMed
.
- J. Sznitman, X. Shen, P. K. Purohit and P. E. Arratia, Exp. Mech., 2010, 50, 1303–1311 CrossRef
.
- K. Chung, M. Zhan, J. Srinivasan, P. W. Sternberg, E. Gong, F. C. Schroeder and H. Lu, Lab Chip, 2011, 11, 3689–3697 RSC
.
- S. Mondal, E. Hegarty, C. Martin, S. K. Gökçe, N. Ghorashian and A. Ben-Yakar, Nat. Commun., 2016, 7, 13023 CrossRef CAS PubMed
.
- S. E. Hulme, S. S. Shevkoplyas, A. P. McGuigan, J. Apfeld, W. Fontana and G. M. Whitesides, Lab Chip, 2010, 10, 589–597 RSC
.
- S. K. Gokce, E. M. Hegarty, S. Mondal, P. Zhao, N. Ghorashian, M. A. Hilliard and A. Ben-Yakar, Sci. Rep., 2017, 7, 9837 CrossRef PubMed
.
- H. Wen, W. Shi and J. Qin, Biomed. Microdevices, 2012, 14, 721–728 CrossRef PubMed
.
- S. Uppaluri and C. P. Brangwynne, Proc. R. Soc. B, 2015, 282, 1813 Search PubMed
.
- M. C. Letizia, M. Cornaglia, R. Trouillon, V. Sorrentino, L. Mouchiroud, M. S. Bou Sleiman, J. Auwerx and M. A. M. Gijs, Microsyst. Nanoeng., 2018, 4, 6 CrossRef
.
- S. Li, H. A. Stone and C. T. Murphy, Lab Chip, 2015, 15, 524–531 RSC
.
- S. Saberi-Bosari, J. Huayta and A. San-Miguel, Lab Chip, 2018, 18, 3090–3100 RSC
.
- C. M. McCay, M. F. Crowell and L. A. Maynard, J. Nutr., 1935, 10, 63–79 CrossRef CAS
.
- W. Mair and A. Dillin, Annu. Rev. Biochem., 2008, 77, 727–754 CrossRef CAS PubMed
.
- R. J. Colman, T. M. Beasley, J. W. Kemnitz, S. C. Johnson, R. Weindruch and R. M. Anderson, Nat. Commun., 2014, 5, 1–5 Search PubMed
.
- D. Omodei and L. Fontana, FEBS Lett., 2011, 585, 1537–1542 CrossRef CAS PubMed
.
- E. L. Greer and A. Brunet, Aging Cell, 2009, 8, 113–127 CrossRef CAS PubMed
.
- W. Mair, S. H. Panowski, R. J. Shaw and A. Dillin, PLoS One, 2009, 4, e4535 CrossRef PubMed
.
- J. F. Morley, H. R. Brignull, J. J. Weyers and R. I. Morimoto, Proc. Natl. Acad. Sci. U. S. A., 2002, 99, 10417–10422 CrossRef PubMed
.
- Y. Koren, R. Sznitman, P. E. Arratia, C. Carls, P. Krajacic, A. E. X. Brown and J. Sznitman, PLoS One, 2015, 10, 1–16 CrossRef PubMed
.
- J. Sznitman, X. Shen, R. Sznitman and P. E. Arratia, Phys. Fluids, 2010, 22, 121901 CrossRef
.
- W. Gilpin, S. Uppaluri and C. P. Brangwynne, Biophys. J., 2015, 108, 1887–1898 CrossRef CAS PubMed
.
- T. Yoneda, J. Cell Sci., 2004, 117, 4055–4066 CrossRef CAS PubMed
.
- S.-J. Lin, Science, 2000, 289, 2126–2128 CrossRef CAS PubMed
.
- A. K. Chippindale, A. M. Leroi, H. Saing, D. J. Borash and M. R. Rose, J. Evol. Biol., 1997, 10, 269–293 CrossRef
.
- M. R. Klass, Mech. Ageing Dev., 1977, 6, 413–429 CrossRef CAS PubMed
.
- R. Weindruch and R. Walford, Science, 1982, 215, 1415–1418 CrossRef CAS PubMed
.
- R. H. Houtkooper, E. Pirinen and J. Auwerx, Nat. Rev. Mol. Cell Biol., 2012, 13, 225–238 CrossRef CAS PubMed
.
- E. L. Greer, D. Dowlatshahi, M. R. Banko, J. Villen, K. Hoang, D. Blanchard, S. P. Gygi and A. Brunet, Curr. Biol., 2007, 17, 1646–1656 CrossRef CAS PubMed
.
- D. Palgunow, M. Klapper and F. Döring, PLoS One, 2012, 7, 11 CrossRef PubMed
.
- M. Klapper, D. Findeis, H. Koefeler and F. Döring, Genes Nutr., 2016, 11, 4 CrossRef PubMed
.
- J. Durieux, S. Wolff and A. Dillin, Cell, 2011, 144, 79–91 CrossRef CAS PubMed
.
- K. Luersen, U. Faust, D.-C. Gottschling and F. Doring, J. Exp. Biol., 2014, 217, 2480–2488 CrossRef PubMed
.
- T. A. Prolla and M. P. Mattson, Trends Neurosci., 2001, 24, 21–31 CrossRef
.
- L. Bertram, L. Bertram, R. E. Tanzi and R. E. Tanzi, J. Clin. Invest., 2005, 115, 1449–1457 CrossRef CAS PubMed
.
- G. P. Bates, Nature, 2005, 6, 766–773 CAS
.
- S. Finkbeiner, A. M. Cuervo, R. I. Morimoto and P. J. Muchowski, J. Neurosci., 2006, 26, 10349–10357 CrossRef CAS PubMed
.
- J. Li and W. Le, Exp. Neurol., 2013, 250, 94–103 CrossRef CAS PubMed
.
Footnote |
† Electronic supplementary information (ESI) available. See DOI: 10.1039/c8lc00863a |
|
This journal is © The Royal Society of Chemistry 2019 |
Click here to see how this site uses Cookies. View our privacy policy here.