DOI:
10.1039/C8AY02123F
(Paper)
Anal. Methods, 2019,
11, 17-20
Heavy metal ion discrimination based on distinct interaction between single-stranded DNA and methylene blue†
Received
28th September 2018
, Accepted 6th November 2018
First published on 6th November 2018
Abstract
In this work, we developed a facile and extensible colorimetric sensor array based on different interactions between methylene blue (MB) and single-stranded DNA (ssDNA) for the discrimination of heavy metal ions. Different target metal ions can make MB exhibit diversified color and absorbance changes in the presence of different types of DNA as receptors. Linear discriminant analysis (LDA) results give clear identification of each heavy metal ion on a two-dimensional (2D) spatial dispersion plot. Therefore, a new sensor array composed of three types of ssDNA as receptors and MB as a colorimetric probe is designed for fast discrimination of metal ions, and it achieves accurate discrimination of 9 species of heavy metal ions at 30 nM in buffer and tap water samples.
Introduction
The harmful impact of heavy metal ions has attracted wide attention all over the world.1–4 So far, a number of approaches have been reported for the accurate detection of heavy metal ions, such as spectrophotometry,5 atomic absorption spectrometry (AAS),6 fluorescence,7 and inductively coupled plasma spectrometry (ICP-MS).8 All these methods, however, are expensive and time-consuming as well as intensive technical training is required for performing them because of their complex instrumentation.
The implementation of colorimetric sensor array techniques not only adopted all the merits of colorimetric methods but also greatly enhanced the feasibility of discrimination of multiple targets. Colorimetric sensor arrays use cross-responsive sensing elements to imitate mammalian olfactory and gustatory systems.9–11 The changes in color and absorbance induced by the interactions between targets and receptors of a sensor array can be quantified by digital imaging and multivariate statistical techniques.12 Wei et al. developed a combinatorial array of gold nanoparticles with dyes for colorimetric sensing of metal ions.13 Zhou et al. presented a functionalized ionic microgel sensor array for colorimetric discrimination of metal ions.14 Our group has developed a dual channel (colorimetric and fluorescence) sensor array for metal ion discrimination.15 However, these sensor arrays were very complex in design, and not suitable for rapid on-site discrimination of heavy metal ions in sophisticated environments.
In this study, based on the specific interaction between methylene blue (MB) and single-stranded DNA (ssDNA), and the sequence and structure dual-dependent property of the interaction, a new sensor array composed of three types of ssDNA (24A, 24C, and 24T) and MB was designed for discrimination of heavy metal ions. Nine heavy metal ions, including Fe3+, Cd2+, Pb2+, Hg2+, Ag+, Sn4+, Ni2+, Mn2+, and Cu2+, were chosen as analytes to evaluate the sensor array. The color characteristics of MB and the different interactions between three types of DNA and target metal ions are responsible for the sensor array's fast response to heavy metal ions, resulting in color and absorbance change. Linear discriminant analysis (LDA) was applied to process the resulting response pattern data.
Experimental
Reagents and apparatus
Methylene blue (MB) was purchased from Sigma-Aldrich. All oligonucleotides were synthesized by Sangon Biotechnology Co. Ltd. (Shanghai, China): 5′-AAA AAA AAA AAA AAA AAA AAA AAA-3′ (24A), 5′-CCC CCC CCC CCC CCC CCC CCC CCC-3′ (24C), and 5′-TTT TTT TTT TTT TTT TTT TTT TTT-3′ (24T). All other reagents were of analytical reagent grade. Ultrapure water was provided by a Direct-Q3 system and used as a solvent in all experiments.
Instrumentation
Absorption spectra were recorded on SpectraMax®M2e Multi-Mode Microplate Reader (Molecular Devices, California, USA) at room temperature. The 96-well plates were obtained from Costar (3590, USA).
The procedure of metal ion discrimination
For the discrimination of heavy metal ions, 10 μL of 100 μM DNA and different concentrations of heavy metal ions were added to 70 μL of TE buffer (10 mM Tris, 1 mM EDTA, pH = 8.0). The different affinities between DNA and target metal ions resulted in diverse DNA–metal ion binding. The resulting solution was incubated at 37 °C for 30 min. After incubation, 50 μL of 200 μM MB was added to the above mixture for 10 min of incubation. MB can interact with ssDNA in an enthalpy-driven process.16 After that, the absorbance (OD660 nm and OD538 nm) of the detection solution in disposable 96-well plates was recorded using a microplate reader. The raw data matrix was processed using classical LDA in SPSS (version 11.03).
Results and discussion
Detection mechanism
The principle of this sensing strategy is illustrated in Scheme 1. The sensor array is comprised of three types of ssDNA (24A, 24C, and 24T) as receptors, and MB as the indicator. In the absence of target metal ions, DNA can interact with MB in an enthalpy-driven process. In this case, the diverse driving forces existing between MB and 24A, between MB and 24T, between MB and 24C result in that the solution color is blue, blue, and purple, respectively. However, in the presence of target metal ions, the DNA gives a different interaction with target metal ions. Owing to the sequence and structure dual-dependent property of the interaction between ssDNA and MB,16 the combination of the target metal ion-induced diversified interactions results in distinct differences in absorbance and solution color. For each metal ion, the array generates a unique response pattern that can be further differentiated using LDA.
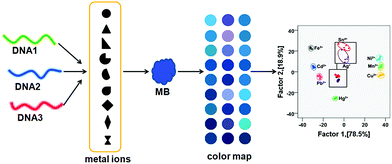 |
| Scheme 1 Schematic illustration of the transduction principle of the MB–DNA colorimetric sensor array for heavy metal ions. | |
Array's responses to metal ions
To establish a clear metal ion discrimination model based on statistical multivariate processing, we performed LDA17,18 for the colorimetric responses of this sensor array against heavy metal ions (Fe3+, Cd2+, Pb2+, Hg2+, Ag+, Sn4+, Ni2+, Mn2+, and Cu2+). The differential parameter from each single metal ion against the sensing system, i.e., K/K0, where K and K0 are UV absorption (K = OD660 nm/OD538 nm) of the solution in the presence and absence of the target metal ions, respectively (Table S1†), forms a specific pattern for the discrimination of the metal ion from others by means of LDA. Two discrimination factors (Factor 1 and Factor 2) were employed to generate a two-dimensional (2D) plot, as shown in Fig. 1A, in which each point represents the response pattern for an individual metal ion sample against the sensor array. 9 metal ions were well-clustered and discriminated clearly from each other on the 2D graph. The first two canonical factors accounted for about 78.5% and 18.9% of the total variation, respectively (Fig. 1A). The 100% classification accuracy indicated the validity of this single-channel sensor array in the discrimination of metal ions (Fig. 1B). The above results confirmed our postulation that this sensor array could be applied for differentiation of heavy metal ions. To provide further evidence for the array's ability to identify heavy metal ions, hierarchical cluster analysis (HCA) was also performed. As shown in Fig. 2, similar to LDA, HCA further shows that metal ions at the lower investigated concentration (i.e., 30 nM) could be accurately identified against one another with no error or misclassifications out of 45 cases (9 metal ions × 5 replicates). In addition, the jackknifed classification matrix shows that the classification accuracy of metal ions was improved from 68.6% for 24T–MB, to 97.5% for the combination of 24A and 24C–MB, to 100% for the combination of 24A, 24C and 24T–MB (Fig. S1†).
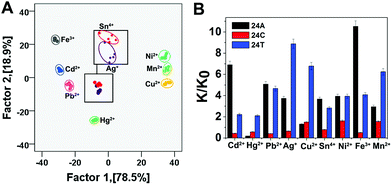 |
| Fig. 1 (A) Canonical score plot for the discrimination of the 9 metal ions at 30 nM using the sensor array. (B) Fingerprints of the 9 metal ions based on the colorimetric response patterns of the sensor array against the metal ions at 30 nM. | |
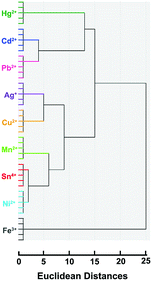 |
| Fig. 2 Hierarchical cluster analysis for metal ions at 30 nM. All experiments were performed in quintuplicate. | |
In order to prove that the discrimination capability of this sensor array is equally effective for the identification of metal ion mixtures, we performed experiments with the mixtures of Ag+ and Sn4+ at 30 nM with Ag+/Sn4+ molar ratios of 1/9, 3/7, 1/1, 7/3 and 9/1 as well as with pure Ag+ and Sn4+ as a model (Table S2†). As shown in Fig. 3, these metal ion mixtures were distinguished from each other in the LDA plot with 100% accuracy. The mixtures of the 9 metal ions also were well identified (Fig. S2†).
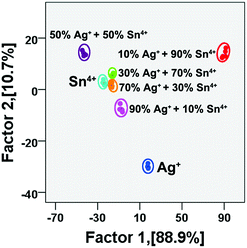 |
| Fig. 3 Canonical score plot for discrimination of mixtures of Ag+ and Sn4+ at different molar ratios (total metal ion concentration: 30 nM). | |
The as-developed sensor array shows good reproducibility and can also be used for the fast quantitative detection of metal ions. Taking the detection of Cd2+ as an example, as shown in Fig. 4 and Table S3,† this sensor array was sufficiently sensitive to detect Cd2+ at nanomolar levels within a linear range of 30–200 nM (R2 = 0.99), and the limit of detection was derived to be 24 nM. The linearity of the dose–response curve revealed that the interactions between metal ions and DNA were homogeneous and stable, which indicated that the detection of metal ions with this sensor array was highly reproducible.19
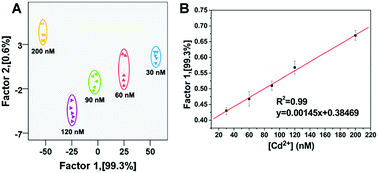 |
| Fig. 4 (A) Canonical score plot for colorimetric response patterns against different concentrations of Cd2+ (30, 60, 90, 120 and 200 nM). (B) Plot of the first discriminant factor vs. Cd2+ concentration. The error bars represent standard deviation obtained from five independent measurements. | |
To investigate the robustness of the sensor array, unknown samples randomly taken from the training set were tested. Out of 45 metal ion samples, only 27 were identified correctly using 24T, which indicated 60% identification accuracy. The identification accuracy of the same unknown samples improved from 60% for 24T to 97.78% for 24C + 24T. Whereas the 45 unknown samples were correctly discriminated using the three sensing elements (24A + 24C + 24T) with 100% accuracy (Tables S4–S6†).
Discrimination of metal ions in real samples
Finally, the potential of the sensor array to detect heavy metal ions in real samples (e.g., tap water) was evaluated. As we know, tap water contains various ions (Na+, K+, Cl−, PO43−, etc.) and small molecules (disinfectants, sugars, etc.). No target metal ions (Fe3+, Cd2+, Pb2+, Hg2+, Ag+, Sn4+, Ni2+, Mn2+, and Cu2+) were found in the tap water collected from our lab as detected by ICP-MS analysis. The nine metal ions were spiked into the tap water at 30 nM, respectively. However, in the present case, as shown in Fig. 5 and Table S7,† each of the nine samples generated a distinct response without overlap in the presence of the tap water sample, and a 100% identification accuracy was achieved for all the nine heavy metal ions. From the above result, it could be concluded that the sensor array has great potential for metal ion discrimination in real samples.
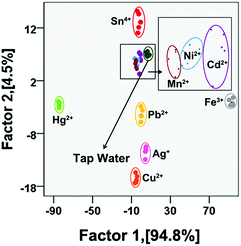 |
| Fig. 5 Canonical score plots for the discrimination of tap water and tap water spiked with different metal ions (five parallel measurements, the concentration of metal ions is 30 nM). | |
Conclusions
In summary, we found that the interaction between ssDNA and target metal ions was diverse, affecting the colorimetric responses of metal ions. On the basis of this phenomenon, we took advantage of three types of single-stranded DNA as receptors obtaining a distinct colorimetric response pattern to each target metal ion. This approach was facile, label-free, and sensitive. Nine heavy metal ions at the 30 nM level could be differentiated using the sensor array. In addition, the metal ions at various concentrations and the binary mixtures were also effectively discriminated. Moreover, nine metal ions spiked in tap water at 30 nM were well distinguished by the sensor array with an accuracy of 100%. The robust nature of DNA receptors, which can bind not only with metal ions but also with biological molecules, and other targets, gives this strategy the potential to detect a broad range of targets in environmental monitoring and diagnosis.
Conflicts of interest
There are no conflicts to declare.
Notes and references
- L. Tan, Z. Chen, Z. Yan, X. Wei, Y. Li, Z. Chi, X. Wei and X. Hu, Biosens. Bioelectron., 2016, 85, 414 CrossRef CAS.
- L. Liu and H. Lin, Anal. Chem., 2014, 86, 8829 CrossRef CAS.
- S. Pihlasalo, I. Montoya Perez, N. Hollo, E. Hokkanen, T. Pahikkala and H. Harma, Anal. Chem., 2016, 88, 5271 CrossRef CAS.
- G. Aragay, J. Pons and A. Merkoci, Chem. Rev., 2011, 111, 3433 CrossRef CAS PubMed.
- X. Wang, Y. Yang, J. Dong, F. Bei and S. Ai, Sens. Actuators, B, 2014, 204, 119 CrossRef CAS.
- P. Novak, J. Dedina and J. Kratzer, Anal. Chem., 2016, 88, 6064 CrossRef CAS.
- J. Hou, J. Dong, H. Zhu, X. Teng, S. Ai and M. Mang, Biosens. Bioelectron., 2015, 68, 20 CrossRef CAS PubMed.
- D. Udhayakumari, S. Suganya, S. Velmathi and D. Mubarakali, J. Mol. Recognit., 2014, 27, 151 CrossRef CAS.
- J. R. Askim, M. Mahmoudi and K. S. Suslick, Chem. Soc. Rev., 2013, 42, 8649 RSC.
- Y. Tao, X. Ran, J. Ren and X. Qu, Small, 2014, 10, 3667 CrossRef CAS PubMed.
- O. R. Miranda, B. Creran and V. M. Rotello, Curr. Opin. Chem. Biol., 2010, 14, 728 CrossRef CAS PubMed.
- H. E. Tahir, Z. Xiaobo, H. Xiaowei, S. Jiyong and A. A. Mariod, Food Chem., 2016, 206, 37 CrossRef CAS PubMed.
- X. L. Wei and Z. H. Mo, Anal. Methods, 2014, 6, 979 RSC.
- X. J. Zhou, J. J. Nie and B. Y. Du, ACS Appl. Mater. Interfaces, 2017, 9, 20913 CrossRef CAS.
- L. L. Tan, Z. B. Chen, Y. Zhao, X. C. Wei, Y. H. Li, C. Zhang, X. L. Wei and X. C. Hu, Biosens. Bioelectron., 2016, 85, 414 CrossRef CAS.
- Z. Y. Wang, J. Zhao, Z. J. Li, J. C. Bao and Z. H. Dai, Anal. Chem., 2017, 89, 6815 CrossRef CAS.
- J. R. Askim, M. Mahmoudi and K. S. Suslick, Chem. Soc. Rev., 2013, 42, 8649 RSC.
- Z. Long, D. C. Fang, H. Ren, J. Ouyang, L. He and N. Na, Anal. Chem., 2016, 88, 7660 CrossRef CAS.
- H. Pei, J. Li, M. Lv, J. Wang, J. Gao, J. Lu, Y. Li, Q. Huang, J. Hu and C. Fan, J. Am. Chem. Soc., 2012, 134, 13843 CrossRef CAS PubMed.
Footnote |
† Electronic supplementary information (ESI) available. See DOI: 10.1039/c8ay02123f |
|
This journal is © The Royal Society of Chemistry 2019 |
Click here to see how this site uses Cookies. View our privacy policy here.