The effect of motivation on the choice of chemistry in secondary schools: adaptation and validation of the Science Motivation Questionnaire II to Spanish students
Received
5th April 2018
, Accepted 28th May 2018
First published on 28th May 2018
Abstract
The present study aims to analyse the effect of motivational variables and previous academic achievement on students’ future choice of chemistry once this subject becomes optional in the educational system. Toward this goal, the translation and adaptation of the Science Motivation Questionnaire II (SMQII) has been undertaken in our investigation to measure students’ motivation towards physics and chemistry. The sample comprised 1060 secondary school Spanish students divided into two groups: 695 students who chose the subject when it became optional for the first time and 365 who decided to leave it. Factor analysis confirmed the original structure of latent variables in our sample, providing validity for this adaptation to a new language and context. A segmentation analysis confirmed that career motivation was the best predictor of students’ retention in physics and chemistry before previous academic achievement and the rest of the motivational variables. Although significant gender differences were found in self-efficacy and self-determination, these seem not to be relevant in students’ choice.
Introduction
The great scientific and technological progress, together with its incredibly fast development within the last few decades, necessitates scientifically literate citizens able to take part in the development of the 21st century society (Hurd, 1998; Roth and Lee, 2004). Scientific literacy is necessary for people to understand these advances and make well-founded decisions about science-related issues, i.e. to become able to think critically and participate actively in the knowledge society in a responsible and efficient way (Hurd, 1998; Gil-Pérez and Vilches, 2005; Rocard et al., 2007; Holbrook and Rannikmae, 2009; Blanco-López et al., 2015; OECD, 2016). Thus, since scientific literacy must be a target every citizen ought to reach, science should be considered as a key subject in childrens’ and teenagers’ education (DeBoer, 2000; European Commission, 2004; Dillon, 2009). In fact, the importance and applications of science in everyone's daily life should be highlighted (Longbottom and Butler, 1999; Roth and Lee, 2004; Feinstein, 2011; Hofstein et al., 2011) and critical thinking promoted (Osborne, 2014; Blanco-López et al., 2015).
Despite the significant need for science education, chemistry and chemistry-related subjects are not compulsory during the whole secondary education in many countries. In fact, students must decide whether to take them or not around the age of 15–16, with the exact age being dependent on the country and its particular educational system (European Commission, 2004; Tripney et al., 2010; Bennett et al., 2013; BøE and Henriksen, 2013; Mujtaba and Reiss, 2014). This early choice of science subjects when they are no longer compulsory has a great influence on pupils’ higher studies, since it defines their future trajectories relative to pursuing science careers. This is due to the fact that once they decide to opt out of science subjects, it is difficult for them to enter a STEM (Science, Technology, Engineering and Mathematics) degree, where those previous courses are required (Van Langen and Dekkers, 2005).
A significant decrease in the number of students taking STEM studies has been observed in recent years, in spite of the aforementioned increasing importance of scientific issues (Lyons, 2006a; Bøe et al., 2011). This problem appears both at the tertiary level (Oon and Subramaniam, 2010) and in secondary education (Smyth and Hannan, 2006; Lyons, 2006b; Solbes et al., 2007; Solbes, 2011; Bennett et al., 2013), and does not affect all disciplines to the same extent. In particular, physics and chemistry are among the most affected areas (Osborne et al., 2003; Solbes et al., 2007; Ulriksen et al., 2010; Solbes, 2011). An international concern about this situation exists nowadays. The Rocard Report (Rocard et al., 2007) shows how this problem affects the European Union and suggests a number of efficient initiatives to increase students’ interest and motivation towards science. However, this situation does not appear exclusively in Europe, but exists in many other countries around the world (Lyons, 2006a; Ulriksen et al., 2010; Bøe, 2012). Lastly, regarding the problem of retention in STEM studies, it is important to distinguish between two concepts: drop out and opt out. As pointed out by Ulriksen et al. (2010), “The term ‘drop out’ is commonly used to describe those students leaving their study before they pass the final examination” (p. 209). However, the idea of opting out a subject refers to not choosing it among a variety of optional courses.
In the case of Spain, the curriculum in Compulsory Secondary Education requires the development of a key competence called Mathematical competence and basic competences in science and technology (Ministerio de Educación, Cultura y Deporte, 2015). However, students can opt out of physics and chemistry at the age of 15, one year before finishing the Compulsory Secondary Education (Jefatura del Estado, 2013). At this point, there is a significant loss of students, since it is the moment when chemistry becomes an optional subject in the Spanish educational system. According to official reports available, a third of students opt out of chemistry every year in the Community of Asturias, which may be representative of the situation in the whole country (Consejería de Educación y Cultura del Principado de Asturias, 2017).
Theoretical framework
Factors influencing the choice of physics and chemistry in secondary schools
Intensive research aiming to determine the factors responsible for the choice of physics and chemistry when they are no longer compulsory has been carried out in the last few years both at the college level (see for example: Cerinsek et al. (2013)) and at the secondary level (see for example: Potvin and Hasni (2014), or Shirazi (2017)). Previous studies reported a complex picture of the variables affecting students’ choice. Moreover, it has been suggested that the choice seems to be affected by the interaction of several of these variables (Cleaves, 2005; Bennett et al., 2013). In this section, we present a brief description of the factors influencing the decision of opting in or out of physics and chemistry subjects in secondary schools.
Social and cultural factors.
Social and cultural factors like the ethnicity (Gorard and See, 2009; Gill and Bell, 2013; Mujtaba and Reiss, 2014; DeWitt and Archer, 2015; Yazilitas et al., 2017), the socio-economic background (Gorard and See, 2009; Smith and Gorard, 2011; Anderhag et al., 2013; Mujtaba et al., 2018) and the parents’ influence (Stokking, 2000; Lyons, 2006b; Harackiewicz et al., 2012; Anderhag et al., 2013; Mujtaba and Reiss, 2014; Mujtaba et al., 2018) have been the subject of previous research regarding participation in science. Additionally, differences between countries have been established by several authors both at the secondary (Potvin and Hasni, 2014) and tertiary level (Van Langen and Dekkers, 2005; Elster, 2014; Blanco-López et al., 2015).
School factors.
Besides factors like the ethos and the management of the school (Smyth and Hannan, 2006; Bennett et al., 2013), the convenience of the subject timetable, the availability of the subjects at the school or the level of information at students’ disposal (Palmer et al., 2017), there are important variables, such as the science curriculum on offer, whose relevance in chemistry uptake has been pointed out (Lyons, 2006a; Bennett et al., 2013; Homer and Ryder, 2015). It is claimed that both students’ participation in classroom activities and emphasis on laboratory work positively influence students’ intentions to participate in science subjects (Cerinsek et al., 2013; Broman and Simon, 2015). However, Abrahams (2009) and Shirazi (2017), independently, concluded that practical activities are not efficient in influencing students’ choice; instead they generate a short-term engagement because they are preferred to traditional classes. Additionally, connecting chemistry to everyday life can help students opt for chemistry (Stokking, 2000; Broman and Simon, 2015; Palmer et al., 2017; Sheldrake et al., 2017). The role of teachers and their didactic models also have a great effect on students’ choice (Stokking, 2000; Smyth and Hannan, 2006; Gorard, 2010; Cerinsek et al., 2013; Broman and Simon, 2015; Juuti and Lavonen, 2016; McDonald, 2016; Palmer et al., 2017; Shirazi, 2017), together with the encouragement and advice they give to their pupils to continue studying the subject (Mujtaba and Reiss, 2014; Mujtaba et al., 2018). In fact, enjoyment and interest, as well as previous school experiences, are claimed to be among the key factors influencing the choice of scientific subjects (Osborne et al., 2003; Palmer et al., 2017).
Difficulty of physics and chemistry subjects.
Both physics and chemistry are among the subjects that are perceived by the students as the most difficult and demanding (Osborne et al., 2003; Cleaves, 2005; Lyons, 2006a; Bøe et al., 2011). Importantly, this is a factor that has been proven to influence students’ decision on enrolling in physics and chemistry subjects (Stokking, 2000; Smyth and Hannan, 2006; Palmer et al., 2017; Shirazi, 2017). In particular, Smyth and Hannan (2006) related a higher probability of opting out of science subjects with the perception of science as difficult. Moreover, they also stated that students who find these subjects interesting and useful will more probably take them. Interestingly, Solbes (2011) found in his study with Spanish students, that physics and chemistry was perceived as the most difficult subject, excessively theoretical, boring, and only slightly useful and interesting.
Future studies.
Many studies have found a clear relationship between students’ choice of physics and chemistry subjects and their intentions to pursue university studies related to these disciplines (Stokking, 2000; Lyons, 2006a; Bøe et al., 2011; Bøe, 2012; Bennett et al., 2013; Broman and Simon, 2015; Palmer et al., 2017). For example, Stokking (2000) found that future relevance was the main predictor of students’ choice. Besides, Palmer et al. (2017) concluded that although the need of a subject in future studies was an important factor for students to enroll in it, a lack of necessity did not imply that they were opting out of it. Along these lines, Bøe et al. (2011) commented that many students decide to get further in their science studies just to keep their options open. What's more, to promote physics and chemistry choice, students should be informed about scientific occupations, which are quite unfamiliar to them (Cleaves, 2005; Bennett et al., 2013; BøE and Henriksen, 2013). Thus, it is important that students perceive science as a useful discipline, since this perception, together with their intrinsic interest in science, has been recently related to their aspirations in the field of chemistry (Mujtaba et al., 2018).
Gender effects.
The effect of gender on students’ decision of future studies has been studied in the last few decades. Although inconclusive results have been reported, overall, previous research found that females' attitudes towards science are less favourable than men's (Brotman and Moore, 2008). This fact leads to a different behaviour between both genders when options for prospective careers are considered. With regard to future choices, secondary school girls are less likely than boys to choose mathematics or physical science studies. In turn, girls prefer to pursue biology and health-related degrees (Jacobs, 2005). Isaacs (2001) found that the gap in the percentage of students by gender in engineering studies at college is more related to their little enrolment in this kind of study than to the rate of abandonment once they become college students (Kimmel et al., 2012). Self-constructs and motivational reasons have been proposed to explain the distinct gender behaviour regarding the choice of future studies (Jacobs, 2005). For example, Cheryan et al. (2017) suggested the gender difference in self-efficacy as one of the reasons to explain the imbalance of certain university STEM fields. On the other hand, other studies found no significant differences regarding the choice of physics and chemistry between genders (Stokking, 2000; Sheldrake et al., 2017). For example, in a study conducted in Ireland, Smyth and Hannan (2006) reported that the gender effect on the take-up of science subjects depends on the specific disciple: physics and biology are mostly taken by boys and girls, respectively, whereas chemistry tends to be gender neutral.
Previous achievement.
For some students, the grades obtained in prior courses are among the most important factors influencing their choice of future participation in chemistry-related subjects (Palmer et al., 2017). As claimed by Anderhag et al. (2013), previous grades in scientific subjects had a greater impact than final grades. For Stokking (2000) and Gill and Bell (2013), the marks obtained in physics were an important predictor of students’ future choice. Moreover, students with great success in previous science subjects are encouraged to continue with them once they are no longer compulsory (Smith and Gorard, 2011). Besides, previous achievement has been related to the involvement in out-of-school activities (Simpkins et al., 2006). Thus, this type of activities could be, in some cases, a mediating variable between academic performance and a future choice in the field of science. For example, Cerinsek et al. (2013) found that watching science television programs was the most influencing out-of-school factor in students’ choice of pursuing STEM studies in Slovenia.
Motivation, interest and attitudes towards science.
The relationship between students’ motivation towards science and their future choice of science subjects in secondary education has been widely investigated (Mujtaba and Reiss, 2014; Sheldrake et al., 2015; Sheldrake, 2016; Palmer et al., 2017). Moreover, motivation towards science seems to be related to the choice of a STEM career (Salta et al., 2012; Cerinsek et al., 2013; Vázquez-Alonso and Manassero-Mas, 2015; Aeschlimann et al., 2016) or to the persistence in them (Shedlosky-Shoemaker and Fautch, 2015). Importantly, science motivation has been found to be higher in college students than in high school students (Tüysüz et al., 2010). Both extrinsic motivation and intrinsic interest have been found to be strongly associated with chemistry aspirations, even in students from disadvantaged backgrounds (Mujtaba et al., 2018). In particular, self-efficacy, which is the confidence students have that they will achieve well in science (Lawson et al., 2007; Ferrell and Barbera, 2015), has also been claimed to influence students’ future participation in science subjects (Palmer et al., 2017). For example, Quinn and Lyons (2011) found that higher expectations of success in science would lead to a higher probability of students entering a STEM degree at university. However, it should also be taken into account that this expectation of success is also affected by the perceived difficulty of the subject (Bøe et al., 2011).
In this context, self-efficacy is not as important as interest in science in predicting students’ science choice preferences (Sha et al., 2015). Interest in science has also been recurrently investigated as a factor influencing students’ future participation in science (Bøe, 2012; Cerinsek et al., 2013). Arandia et al. (2016) concluded that the interest towards physics and its learning seemed to be the same for both high school and university students. In relation to this, Anderhag et al. (2016) found that interest in science does not decrease from primary to secondary education, but students may never acquire it. Besides, making science more relevant to students’ everyday lives may foster their interest in it (Sheldrake et al., 2017).
Interest and enjoyment of science have been claimed to be closely related (Ainley and Ainley, 2011; Bøe et al., 2011), and the relationship between both of them and science choice seems to be complex (Broman and Simon, 2015). An association between students’ motivation towards science, enjoyment, future orientation and learning activities was also found by Hampden-Thompson and Bennett (2013). Thus, fostering students’ enjoyment of a subject will lead to future participation in it (Mujtaba and Reiss, 2014; Palmer et al., 2017). Finally, attitudes towards science also influence students’ future choices for science (Osborne et al., 2003). However, as suggested by DeWitt et al. (2014), students’ positive attitudes towards science and their interest in becoming a scientist are not related at the beginning of secondary education. This fact suggests that the problem may be that students experience difficulties when trying to picture themselves pursuing a career in science.
Measuring motivation to learn science
In order to improve students’ academic achievement in science, measuring their motivation is important for both teachers and researchers (Glynn et al., 2011). However, carrying out this measurement is challenging because motivational constructs are not directly observable variables but correspond to latent variables (Kline, 2011). This is why they need to be measured by certain items that serve as empirical indicators of how the specific construct is conceptualized.
With the aim of measuring motivation to learn science, several scales have been developed. Tuan et al. (2005) developed the Students’ Motivation Towards Science Learning (SMTSL) questionnaire and validated it with junior high school students from central Taiwan. It consists of six scales: self-efficacy, active learning experiences, science learning value, performance goal, achievement goal, and learning environment stimulation. The first two constructs were found to highly correlate with achievement scores, whereas the last one showed a higher correlation with science attitude. Along these lines, the instrument developed by Velayutham et al. (2011) targeted the evaluation of important factors related to the motivation and self-regulation in science learning. In this case, the validation of the Students’ Adaptive Learning Engagement in Science (SALES) survey was conducted with lower secondary education students and included four scales: learning goal orientation, task value, self-efficacy and self-regulation.
Additionally, other authors have collected different scales measuring complementary constructs related to motivation in order to study the relationship between them, and even with other variables such as course performance (Ferrell et al., 2016). In other cases, adaptations of general instruments to chemistry-related disciplines have been carried out. For instance, Liu et al. (2017) adapted the Academic Motivation Scale (AMS) to measure students’ motivation toward chemistry at the college level. The AMS was developed in 1992 by Vallerand et al. to measure seven subscales of motivation, including amotivation, three different types of extrinsic motivation, and three different types of intrinsic motivation. The AMS-Chemistry measures the same seven types of student motivation, but specifically towards chemistry.
One of the most recent and reliable instruments is the Science Motivation Questionnaire (SMQ), which was designed by Glynn and Koballa (2006). To develop it, the authors centered their attention on the motivational components that influence self-regulatory learning (Glynn and Koballa, 2006; Glynn et al., 2007, 2009; Bryan et al., 2011). To improve its construct validity, a subsequent version of this instrument, the Science Motivation Questionnaire II (SMQII) was developed some years later (Glynn et al., 2011). This instrument, which will be further explained in the following section, was validated with science majors and nonscience majors, showing the lower motivation of the latter towards science. The five motivation scales of the SMQII were found to be highly related not only between them, but also to students’ achievement.
Science Motivation Questionnaire II.
The SMQII examines the motivation of students to learn science at university and is based on the social cognitive theory, originally developed by Bandura (1986), and extended by himself and others (Bandura, 2001; Pajares and Schunk, 2001; Pintrich, 2003). This theory considers the process of human learning as a series of reciprocal interactions among personal characteristics, environmental factors and behaviors, being most effective when it is self-regulated. The social cognitive theory defines the motivation to learn science as the “internal state that arouses, directs, and sustains science-learning behavior” (Glynn et al., 2011, p. 1160), and conceptualizes it as a multidimensional construct. This implies that each component is a type of motivation (Glynn et al., 2011). Among the motivational components suggested by the social cognitive theory are intrinsic motivation, which involves the inherent satisfaction in learning science because it is found to be interesting, challenging or enjoyable (Ryan and Deci, 2000); extrinsic motivation, which refers to learning science because it drives to a separable outcome or external reward, such as a career or a mark (Ryan and Deci, 2000); self-determination, which involves the control that students believe they have over their learning of science (Black and Deci, 2000); and self-efficacy, which as stated before, refers to students’ confidence to successfully complete science-related tasks (Lawson et al., 2007; Ferrell and Barbera, 2015). In relation to the aforementioned motivation components, the SMQII assesses intrinsic motivation, self-determination, and self-efficacy and adds career motivation and grade motivation, both connected to extrinsic motivation. Each scale consists of 5 items that students have to rate on a Likert-type scale from 0 (never), to 1 (rarely), 2 (sometimes), 3 (often) and 4 (always). Thus, the obtained scores range from 0 to 20. This instrument has been initially validated for a sample of USA college science and nonscience majors (Glynn et al., 2011).
Although the SMQII was first developed to assess motivation to learn science in college students, the authors claimed that it was possible to adapt it to other scientific disciplines, establishing the validation of the specific questionnaire. In fact, it has been adapted to definite college subjects, such as organic chemistry (Austin et al., 2018) or biology (Cleveland et al., 2017). Moreover, with the aim of evaluating the level of motivation toward chemistry or physics in secondary school students, it has also been translated into other languages, such as Greek (Salta and Koulougliotis, 2015) or German (Schumm and Bogner, 2016). To the best of our knowledge, no translation into Spanish targeting high school students has been validated to date.
Description and aims of the present investigation
As stated above, early leaving of chemistry in secondary school leads students to mostly discard a future science-related trajectory at a very young age. At this point, students’ knowledge of the possibilities of a career in science is necessarily very limited. We estimate that about 30% of Spanish secondary students opt out of chemistry once they face the first opportunity to do it. The main goal of the present investigation was to analyse the possible role of previous academic achievement and motivational traits in secondary school students’ choice of chemistry when it becomes an optional subject in the Spanish educational system. We also aim to investigate possible gender effects in the decision making. Toward this aim, we measured five motivational variables using the SMQII to study the possible effect of motivation on students’ choice. As a consequence, we first undertook the translation and adaptation of this instrument and the study of the validity and reliability of the questionnaire in our secondary school Spanish sample of students.
Method
Sample and application context
The data gathering was performed in 15 secondary schools located in the Spanish Communities of Asturias (n = 9) and Aragón (n = 6), which were selected through convenience sampling based on the accessibility of the schools. Ten schools were public and five were private. The participants were 1060 students, aged 14–17 (average age: 15.03), in the 4th year of Compulsory Secondary Education. Of this total, 522 were males (49.2%) and 538 females (50.8%). The percentage of students from Asturias and Aragón was 60.1% and 39.9%, respectively. Given the purposes of the investigation, a two-group research design was chosen. All the students who were enrolled in the physics and chemistry subject, 695 (65.6%), were assigned to the first group (PC group) and those who decided to opt out of physics and chemistry, 365 (34.4%), were assigned to the second group (nonPC). The number of males studying physics and chemistry (n = 359, 51.7%) is slightly higher than that of females (n = 336, 48.3%). In contrast, the non physics and chemistry group was made up of 163 males (44.7%) and 202 females (55.3%). The students in the sample participated voluntarily in the data gathering.
Questionnaire translation and adaptation
The SMQII was developed by Glynn and his coworkers (Glynn et al., 2011). As stated in the original validation work, this questionnaire is adaptable to different branches of science. In our case, this questionnaire was adapted to our purposes by simply replacing the word “science” with “physics and chemistry”. The instrument was translated into Spanish by the two authors of this study independently. Both researchers have a certified degree in English and one of them has lived in North America for two years during his postdoc. This same person has been a science teacher at a Spanish secondary school for over twenty years. Previously, the translators agreed to reach a balance between a culturally meaningful translation and a literal transcription of the original, as suggested by Muñiz et al. (2013). Besides, an English teacher of one of the schools participating in the data gathering, with 15 years of experience in the field of education, was asked to back-translate the questionnaire into English. The comparison between the original instrument and the back-translation confirmed the same content and meaning of the items in both versions. Once the instrument was ready, two pilot analyses were conducted with students from the two Communities participating in our investigation. The discussion with the students about the meaning of the items revealed no need for any change in the translated instrument.
Variables
After the validation of the instrument, the SMQII allowed the measurement of five science motivational variables: career motivation (CM), self-efficacy (SE), self-determination (SD), intrinsic motivation (IM) and grade motivation (GD). The score in these variables ranges from 0 to 20. Besides, three academic variables were measured in the sample of students. First, the students were asked about their grade point average (GPA) in the previous year. Second, their final grade point average in physics and chemistry (PCPA) was also enquired. Both variables range from 0 to 10. Third, the students stated if they had repeated any previous year. Finally, the students were also asked whether they regretted or not their choice on opting in/out of physics and chemistry.
Procedure and analyses
The schools were contacted in September 2017 at the beginning of the academic year 2017–2018. The aim of the study and the procedure that was going to be used were explained to the principals and teachers. Two possibilities were offered: to complete the survey on-line through a link that was provided to them or paper-based. Data were collected in the classrooms during the first weeks of the new academic year, just after students had opted in or out of the subject of physics and chemistry, since the 4th year of Compulsory Secondary Education is the very first moment right after they made the decision. At the beginning of the data gathering, participants were informed about the aim of the study and were thanked for their useful collaboration. They were also asked to answer the survey sincerely, reminding them that it was anonymous.
To reach the aims of this investigation, several statistical analyses were used. First, two different complementary approaches were employed to undertake the validation of the SMQII. An exploratory factor analysis allowed the identification of the latent variables of the questionnaire. Besides, the use of structural equation models facilitated a confirmatory factor analysis of the instrument. Once the underlying factors of the instrument were well defined, a reliability analysis was conducted in each variable by means of Cronbach's alpha. Second, descriptive, correlational and inferential analyses were performed. According to the Kolmogorov–Smirnov tests, all the motivational variables were non-normally distributed: CM, D(1060) = 0.089, p < 0.01 with z-scores of kurtosis and skewness of −7.42 and +0.82; SE, D(1060) = 0.099, p < 0.01 with z-scores of kurtosis and skewness of −2.99 and −6.39; SD, D(1060) = 0.093, p < 0.01 with z-scores of kurtosis and skewness of −1.24 and −6.84; IM, D(1060) = 0.075, p < 0.01 with z-scores of kurtosis and skewness of −5.47 and −0.90; and GD, D(1060) = 0.125, p < 0.01 with z-scores of kurtosis and skewness of −1.47 and −9.49. For this reason, inferential analyses were based on non-parametric tests. In particular, the Mann–Whitney U test was used for mean comparisons and Spearman coefficients are reported as correlation indices. The effect size estimates were computed using Rosenthal's formula (Rosenthal, 1991). Effect size provides information about the importance of a given effect. The commonly accepted indicative values are: r = 0.10 (small effect), r = 0.30 (medium effect) and r = 0.50 (large effect). Finally, a CHAID segmentation analysis was employed to propose a tree to understand the role of the variables involved in this investigation on students’ decision of opting in/out of physics and chemistry. All the computations were made using SPSS and AMOS (Arbuckle, 2010).
Results
First, we will present the results of the validation of the Science Motivation Questionnaire II (SMQII) for a Spanish sample of secondary school students, adapted to the subject of physics and chemistry. The reliability of the scales defined by the questionnaire will also be discussed. Second, the descriptive statistics of the academic and motivational variables and the correlation analyses will be presented. Besides, PC students and nonPC students will be statistically compared. Finally, the results of the segmentation analysis will be described in order to gain insights into the effect of motivation and previous academic achievement on the decision of opting in/out of physics and chemistry.
Validity and reliability of the SMQII
Exploratory factor analysis is one of the approaches to study the validity of a measuring instrument (Kachigan, 1991). This type of analysis allows the identification of underlying factors in a large set of variables by clustering them into a smaller number of homogeneous sets, each of which constitutes a new variable. Besides, the use of structural equation models (SEMs) allows the confirmation of the factorial validity of a theoretical construct (Byrne, 2016). In this section, we present the results for both of the approaches applied to validate the SMQII in our sample. To use cross-validation, the 1060 students in the sample were divided randomly into two samples of 530 students each. These two samples were used in the exploratory and confirmatory factor analysis, respectively. Since the students in our investigation were supposed to be so distinct regarding their motivation towards chemistry, we also undertook the validation analysis separately for the ones who opted for physics and chemistry and for those who opted out.
Exploratory factor analysis of the SMQII.
Factors were extracted through principal component analysis (PCA) of the 25 items of the original questionnaire. To rotate these factors an orthogonal rotation (varimax) was used. The Kaiser–Meyer–Olkin measure tested the sampling adequacy for the analysis (KMO = 0.955). As indicated by Barlett's test of sphericity, χ2(300) = 9027.65, p < 0.01, the correlation between items was large enough for PCA. Using the Kaiser–Guttman rule, five factors, which accounted for 69.73% of the variance, were found in our analysis (see Table 1). Factor loadings for each item measure the percentage of variance accounted by the factors. In our case, all factor loadings were higher than 0.45. In view of the groups of items for each one, the five factors extracted by the PCA and their corresponding explained variance were: career motivation (45.83%), self-determination (9.25%), self-efficacy (6.81%), intrinsic motivation (4.57%) and grade motivation (3.35%). The factor analyses when the sample was divided into PC students and nonPC students yielded very similar results (see Table 1).
Table 1 Factor loadings
Item |
Factor loadings |
Statement |
Total |
PC |
NonPC |
Factor: career motivation |
13 |
0.824 |
0.799 |
0.687 |
Understanding physics and chemistry will benefit me in my career. |
23 |
0.803 |
0.794 |
0.819 |
My career will involve physics and chemistry. |
25 |
0.791 |
0.774 |
0.826 |
I will use physics and chemistry problem-solving skills in my career. |
7 |
0.742 |
0.750 |
0.638 |
Learning physics and chemistry will help me get a good job. |
10 |
0.717 |
0.723 |
0.498 |
Knowing physics and chemistry will give me a career advantage. |
|
Factor: self-determination |
9 |
0.884 |
0.840 |
0.815 |
I study hard to learn physics and chemistry. |
15 |
0.820 |
0.827 |
0.825 |
I spend a lot of time learning physics and chemistry. |
21 |
0.778 |
0.810 |
0.771 |
I prepare well for physics and chemistry tests. |
18 |
0.729 |
0.636 |
0.789 |
I put enough effort into learning physics and chemistry. |
14 |
0.667 |
0.581 |
0.708 |
I use strategies to learn physics and chemistry well. |
|
Factor: self-efficacy |
11 |
0.785 |
0.749 |
0.735 |
I believe I can master physics and chemistry knowledge and skills. |
22 |
0.760 |
0.726 |
0.760 |
I am confident I will do well on physics and chemistry tests. |
16 |
0.710 |
0.726 |
0.744 |
I believe I can earn a grade of ‘‘A’’ in physics and chemistry. |
6 |
0.680 |
0.721 |
0.561 |
I am confident I will do well on physics and chemistry labs and projects. |
5 |
0.670 |
0.601 |
0.620 |
I am sure I can understand physics and chemistry. |
|
Factor: intrinsic motivation |
19 |
0.733 |
0.767 |
0.813 |
I enjoy learning physics and chemistry. |
17 |
0.675 |
0.723 |
0.713 |
Learning physics and chemistry makes my life more meaningful. |
3 |
0.671 |
0.698 |
0.598 |
I am curious about discoveries in physics and chemistry. |
12 |
0.634 |
0.663 |
0.533 |
Learning physics and chemistry is interesting. |
1 |
0.585 |
0.597 |
0.438 |
The physics and chemistry I learn is relevant to my life. |
|
Factor: grade motivation |
2 |
0.713 |
0.701 |
0.718 |
Scoring high on physics and chemistry tests and labs matters to me. |
20 |
0.632 |
0.642 |
0.619 |
Getting a good physics and chemistry grade is important to me. |
4 |
0.594 |
0.591 |
0.664 |
It is important that I get an ‘‘A’’ in physics and chemistry. |
8 |
0.530 |
0.551 |
0.702 |
I think about the grade I will get in physics and chemistry. |
24 |
0.505 |
0.532 |
0.490 |
I like to do better than other students on physics and chemistry tests. |
Confirmatory factor analysis.
In order to test the model obtained by the exploratory factor analysis, a confirmatory factor analysis using a structural equation model (SEM) was performed using the second part of the sample (n = 530). This procedure confirmed the structure of items grouped into five underlying factors described in the previous section. A set of indices has been developed to assess the model, i.e. to check if the relationships between the model variables accurately reproduce the relationships found in the data set. The structural equation analysis rendered a χ2 value of 768.64 and a probability of less than 0.01. This would support the fact that the hypothesized model does not adequately fit the data. However, the sensitivity of this test for large samples is well known (Blunch, 2013). To overcome this problem the χ2/df test was suggested. If this ratio is in the range between 1.0 and 3.0, we can conclude that the model fits reasonably well to the data. In our analysis we found a value of 2.90. Other indices commonly used in SEM investigations are (Blunch, 2013): the Goodness of Fit Index (GFI), the Comparative Fit Index (CFI), the Root Mean Square Error Approximation (RMSEA), and the Standardized Root Mean Square Residual (SRMR). The five factor model suggested by the exploratory factor analysis presents indices which acknowledge a good fit between the model and the experimental data: GFI = 0.89, CFI = 0.92, RMSEA = 0.07, SRMR = 0.05. When the same analysis was carried out using a multiple group approach in the complete sample, the indices behave similarly for PC students (χ2/df = 2.87, GFI = 0.91, CFI = 0.91, RMSEA = 0.05, SRMR = 0.05) and for nonPC students (χ2/df = 2.96, GFI = 0.88, CFI = 0.87, RMSEA = 0.07, SRMR = 0.07) were slightly worse but still within the range of values suggested in the literature for a reasonable fit. A multiple group analysis rendered no significant differences between the fit of the model for both groups of students (p = 0.256).
Reliability of the SMQII.
The Cronbach's alphas of the five motivational variables were: 0.92 for career motivation, 0.86 for self-efficacy, 0.87 for self-determination, 0.88 for intrinsic motivation, and 0.82 for grade motivation. These values were slightly lower when the two groups of students were considered independently. For PC students these values were, respectively: 0.90, 0.83, 0.83, 0.84, and 0.72. In the case of nonPC students, the Cronbach's alpha values were: 0.80, 0.83, 0.89, 0.82, and 0.70, respectively. Consequently, according to Cohen et al. (2011) all the scales were highly reliable, except the last one, which can be considered reliable.
Descriptive statistics and group comparisons
The effect of academic performance.
Academic performance is key with regard to differentiating the students in both groups. In the case of GPA, PC students averaged 0.86 points more than their nonPC counterparts (see Table 2). When PCPA was compared, the difference was even larger (1.37) and the effect size also increased from 0.34 to 0.40.
Table 2 Statistics of students’ grade point average, physics and chemistry point average and SMQII motivational variables globally and by option
|
Total (n = 1060) |
PC group (n = 695) |
NonPC group (n = 365) |
U
|
p
|
r
|
Grade point average (min = 0, max = 10) |
M |
7.24 |
7.54 |
6.68 |
75997.0 |
<0.01 |
0.34 |
SD |
1.21 |
1.19 |
1.02 |
|
Physics and chemistry point average (min = 0, max = 10) |
M |
6.82 |
7.30 |
5.93 |
65904.0 |
<0.01 |
0.40 |
SD |
1.66 |
1.57 |
1.45 |
|
Career motivation (min = 0, max = 20) |
M |
9.71 |
12.33 |
4.72 |
29147.5 |
<0.01 |
0.63 |
SD |
5.75 |
4.85 |
3.63 |
|
Self-efficacy (min = 0, max = 20) |
M |
12.27 |
13.97 |
9.05 |
53336.0 |
<0.01 |
0.48 |
SD |
4.75 |
3.84 |
4.64 |
|
Self-determination (min = 0, max = 20) |
M |
12.12 |
13.05 |
10.35 |
86258.0 |
<0.01 |
0.26 |
SD |
4.41 |
3.76 |
4.98 |
|
Intrinsic motivation (min = 0, max = 20) |
M |
9.51 |
11.44 |
5.85 |
43582.0 |
<0.01 |
0.54 |
SD |
4.91 |
4.13 |
4.13 |
|
Grade motivation (min = 0, max = 20) |
M |
13.66 |
15.42 |
10.33 |
50828.0 |
<0.01 |
0.49 |
SD |
4.72 |
3.59 |
4.80 |
A repetition rate of one or more years of 32.9% was found in the nonPC students. In contrast, only 6% of the students who chose physics and chemistry repeated at least one year during their past studies. It is interesting to note that when the students were asked if they regretted their choice, only 3.8% of the students declared that opting out of physics and chemistry was a mistake. However, 8.3% of the physics and chemistry students believed they made the wrong choice. When students of both groups are compared by gender, the same differences hold: boys and girls in the PC group outperformed their corresponding gender counterparts in the nonPC group (see Table 3). It is worth noting that intragroup comparisons rendered distinct results for GPA and PCGA. In the former, significant differences were found by gender in the PC group and in the nonPC group. However, in the latter, significant differences were only found in the PC group but non-significant differences were encountered in the case of nonPC students (see Table 3).
Table 3 Statistics of students’ grade point average, physics and chemistry point average and SMQII motivational variables by gender within each group
|
PC group |
|
NonPC group |
Boys (n = 359) |
Girls (n = 336) |
U
|
p
|
r
|
Boys (n = 163) |
Girls (n = 202) |
U
|
p
|
r
|
Grade point average (min = 0, max = 10) |
M |
7.22 |
7.88 |
41647.5 |
<0.01 |
0.28 |
M |
6.48 |
6.85 |
13267.0 |
<0.01 |
0.17 |
SD |
1.15 |
1.15 |
SD |
0.92 |
1.08 |
|
Physics and chemistry point average (min = 0, max = 10) |
M |
6.99 |
7.62 |
46684.0 |
<0.01 |
0.24 |
M |
5.83 |
6.00 |
15525.0 |
0.330 |
0.05 |
SD |
1.53 |
1.56 |
SD |
1.38 |
1.49 |
|
Career motivation (min = 0, max = 20) |
M |
12.20 |
12.47 |
57823.5 |
0.346 |
0.04 |
M |
5.21 |
4.32 |
14320.0 |
0.032 |
0.11 |
SD |
4.81 |
4.89 |
SD |
3.86 |
3.39 |
|
Self-efficacy (min = 0, max = 20) |
M |
14.22 |
13.70 |
56069.5 |
0.107 |
0.06 |
M |
9.23 |
8.90 |
15697.5 |
0.444 |
0.04 |
SD |
3.77 |
3.90 |
SD |
4.70 |
4.59 |
|
Self-determination (min = 0, max = 20) |
M |
12.13 |
14.03 |
41893.5 |
<0.01 |
0.27 |
M |
9.48 |
11.05 |
13327.0 |
<0.01 |
0.16 |
SD |
3.71 |
3.57 |
|
|
SD |
4.78 |
5.03 |
|
Intrinsic motivation (min = 0, max = 20) |
M |
11.66 |
11.21 |
56754.5 |
0.117 |
0.05 |
M |
5.71 |
5.96 |
15952.0 |
0.609 |
0.03 |
SD |
4.11 |
4.13 |
SD |
4.08 |
4.17 |
|
Grade motivation (min = 0, max = 20) |
M |
15.02 |
15.84 |
51882.5 |
<0.01 |
0.12 |
M |
10.17 |
10.45 |
16045.0 |
0.676 |
0.02 |
SD |
3.67 |
3.46 |
SD |
4.88 |
4.74 |
The effect of motivational variables.
As expected, the physics and chemistry motivational variables showed significantly higher levels in the case of the students who chose the subject (see Table 2). Besides, the motivational profile of both groups of students was different. In the case of PC students, the five motivational variables presented relatively high scores. Grade motivation had the highest mean score of all of them (15.42). This fact highlights the importance of extrinsic motivation in secondary students. On the other hand, the lowest motivational score was found in intrinsic motivation (11.44), reinforcing the idea that students seem to be more driven by external rewards than by their interest or enjoyment in learning science. However, among their nonPC counterparts, self-determination (10.35) and grade motivation (10.33) were the highest motivational traits found. Consequently, the students who opted out of physics and chemistry present a sense of control towards learning science and retain the extrinsic motivation of getting a good grade. In turn, as expected, the students in this group showed less interest in science (IM = 5.85). The difference with the highest effect size was found in the career motivation variable with a value of 0.63. In second place, intrinsic motivation, grade motivation and self-efficacy displayed a medium effect size. Finally, the mean comparison of self-determination had a small size effect.
Intragroup comparisons showed distinct gender effects in motivational variables for both groups of students. On the one hand, in the PC group, only self-determination and grade motivation were significantly affected by gender. Besides, in self-efficacy, career motivation and intrinsic motivation, the effect of gender was not significant (see Table 3). On the other hand, in the nonPC group, gender affected significantly only self-determination and career motivation whereas self-efficacy, grade motivation and intrinsic motivation were not dependent on gender (see Table 3).
Correlational analysis
All the motivational variables presented significant and positive intercorrelations both in the PC and in the nonPC groups (see Table 4). In the first group, all motivational variables correlated significantly with GPA and PCPA. However, in the group of students who opted out of physics and chemistry, career motivation correlated neither with GPA nor with PCPA and intrinsic motivation only correlated significantly with PCPA. Pairwise statistic comparisons rendered several differences between the correlations in the two groups of students. The strength of the relationship between GPA and PCPA is higher in the case of the students who chose physics and chemistry than in their counterparts (z = −4.9, p < 0.01). Self-determination correlates more strongly with PCPA in the case of nonPC students than in PC students (z = 1.98, p = 0.02). Besides, the correlation between GM and PCPA is stronger in the nonPC group (z = −2.83, p < 0.01). Regarding the comparison of the intercorrelations of the motivational variables, the Spearman coefficients of the pairs which involve CM and SE (z = −2.43, p < 0.01), SD (z = −1.97, p = 0.024) and GM (z = −4.21, p < 0.01) in the case of the PC group of students were larger than those in the other group. The rest of the statistically different intercorrelations were stronger in the nonPC students (see Table 4).
Table 4 Study variable correlations between PC students (upper part) and nonPC students (lower part)a
|
GPA |
PCPA |
CM |
SE |
SD |
IM |
GM |
*p < 0.05; **p < 0.01.
|
Grade point average |
1 |
0.804** |
0.229** |
0.348** |
0.262** |
0.184** |
0.344** |
Physics and chemistry point average |
0.665** |
1 |
0.236** |
0.414** |
0.233** |
0.237** |
0.341** |
Career motivation |
−0.054 |
0.075 |
1 |
0.451** |
0.363** |
0.589** |
0.530** |
Self-efficacy |
0.272** |
0.485** |
0.320** |
1 |
0.272** |
0.537** |
0.471** |
Self-determination |
0.345** |
0.347** |
0.251** |
0.411* |
1 |
0.326** |
0.436** |
Intrinsic motivation |
0.062 |
0.287** |
0.580** |
0.545** |
0.427** |
1 |
0.402** |
Grade motivation |
0.362** |
0.488* |
0.314** |
0.610** |
0.570** |
0.476** |
1 |
The decision of choosing (or not) physics and chemistry, regarding students’ academic performance and motivational traits
Investigations on decision making can be tackled by means of segmentation procedures. Among them, the Chi-squared Automatic Interaction Detector (CHAID) is one of the most commonly used techniques (Gulati et al., 2016). One of the aims of segmentation research is to discover the groups of a population (segments) which differ in the probability of an event. The segmentation analysis eases the choice of the independent variables (predictors) and the characterization of the dependent variable as a function of the predictors. Thus, one of the applications of this technique is the interpretation of the individuals’ behaviour with respect to a decision-making situation. The interpretation of a CHAID analysis is facilitated by the decision trees which build a classification model based on flux diagrams.
In the present investigation, the decision of opting in/out of physics and chemistry was the dependent variable. The GPA, the PCPA, gender, and the five motivational variables measured using the SMQII were chosen as the independent variables. As a measurement of the goodness of fit, the analysis renders an overall correct classification of 81.8% of the individuals. Besides, the correct percentage of classification of PC students and their nonPC counterparts was 82.6% and 80.3%, respectively. In Fig. 1, we present the tree plot obtained in our analysis. In view of the diagram, several findings can be extracted from the analysis. The most relevant variables with regard to the decision making of opting in/out of physics and chemistry are career motivation, GPA, self-efficacy and PCPA. Among them, career motivation is the best predictor for the decision of opting for physics and chemistry (χ2 = 44.43, p < 0.01). On the one hand, the students with the highest probability of choosing physics and chemistry (0.95) are those with very high career motivation, a GPA greater than 6.50 and those with a high level of self-efficacy. On the other hand, students with a low career motivation and with an academic achievement in physics and chemistry equal or lower than 5 points are the ones with the highest probability (0.89) of opting out of physics and chemistry (see Fig. 1).
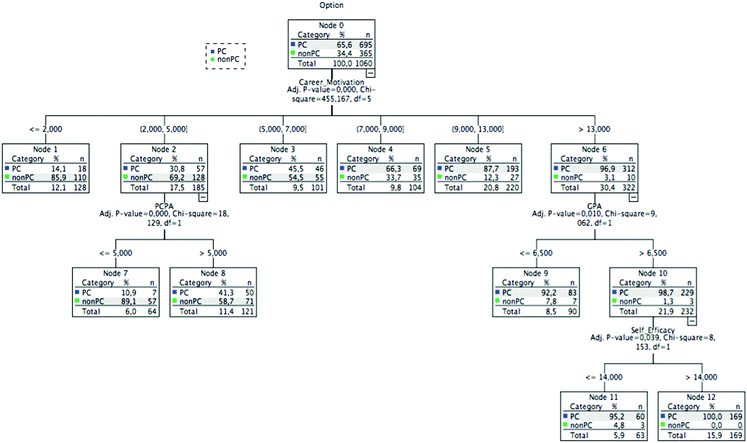 |
| Fig. 1 The CHAID decision tree. | |
Discussion
The translation and adaptation of questionnaires is basic when an instrument created specifically in a particular language and cultural context is to be applied in a different situation (Beaton et al., 2000). Rather than the creation of a new one, adaptations of existing instruments are faster and produce equivalent measures, which facilitate cross-cultural studies. The main purpose of the present investigation was to study the effect of students’ motivation towards science on the decision of choosing physics and chemistry when it becomes optional in an educational system. Toward this aim, we ought to undertake the validation of the SMQII, which was originally designed for USA college students (Glynn et al., 2011). Therefore, the study we present in this paper widens the applicability of this instrument to secondary school students whose mother tongue is Spanish.
Besides the original version (Glynn et al., 2011), Salta and Koulougliotis (2015) developed a Greek version of the questionnaire for secondary school chemistry students and Schumm and Bogner (2016) developed the corresponding German version. In our validation, career motivation was the factor which explained the largest percentage of variance whereas in the case of the Greek and German versions it was grade motivation. In turn, in the original validation in the USA context, intrinsic motivation captured the highest percentage of variance. Nevertheless, the total proportion of explained variance in our validation was similar to that found by Glynn and his coworkers in the original version of the instrument (Glynn et al., 2011). Our reliability analysis rendered Cronbach's alphas slightly higher than those measured by Salta and Koulougliotis (2015) and Schumm and Bogner (2016) in the Greek and German versions for secondary school students.
The levels of the different motivational scales measured using the SMQII in our sample yielded similar average results to those found in the Greek (Salta and Koulougliotis, 2015) and German (Schumm and Bogner, 2016) samples. In line with these previous studies at the secondary level, students scored higher in grade motivation and self-efficacy. However, in our case, intrinsic motivation was the lowest motivational trait whereas in the Greek and German samples intrinsic motivation was higher than career motivation and self-determination. This difference could be due to the contribution of the nonPC students whose level of interest in the subject was much lower.
With regard to the differentiation of the students’ profile in the two groups chosen in our investigation, it is worth noting that students in the PC group outperformed their nonPC counterparts both in the previous year GPA and in the PCPA. Interestingly, the effect size was larger in the latter. These results are in agreement with those found by Anderhag et al. (2013) in Swedish secondary school students. In the same vein, several investigations reported that previous academic performance in science is a good predictor of a future academic choice of science (Gill and Bell, 2013). Moreover, Simpkins et al. (2006) found that grades in science and maths are related to students’ participation in after-school activities related to science which, in turn, would reinforce their interest and involvement in this discipline.
Interestingly, our results show how motivation seems to have a higher impact than previous academic achievement when describing the differences between students who opt in and those who opt out of physics and chemistry. In fact, according to our inferential analysis, four motivational variables, CM, IM, GM, and SE, present a higher effect size in the comparison of both groups of students than previous GPA and PCPA. In line with this finding, several previous studies reported the high relevance of motivation in students’ future preferences for science education (Mujtaba and Reiss, 2014; Shedlosky-Shoemaker and Fautch, 2015; Sheldrake, 2016). Besides, our CHAID analysis found that the best predictor for the decision of choosing physics and chemistry is career motivation. It is interesting to note that in agreement with our results, career motivation has been previously found to be relevant in students’ future decisions. For example, several authors arrived at the conclusion that the perceived relevance of a particular discipline may be key in future choices of science (Stokking, 2000; Cleaves, 2005; Mujtaba and Reiss, 2014; Palmer et al., 2017; Yazilitas et al., 2017).
To compare gender effects in our research context with previous applications of the SMQII in secondary school students, we undertook an inferential analysis. In agreement with Salta and Koulougliotis (2015), who worked in the context of Greek secondary education, GM was statistically different for boys and girls in our PC sample. However, it is interesting to note that, in the context of Greek secondary education, gender differences were also found in IM. In the present study, distinct gender effects on the motivational variables were found in the two groups selected. While no significant gender differences were found in SE and IM between both groups, different gender effects were found for the rest of the motivational variables. In the nonPC group, boys were more motivated towards a future career in science than girls. However, the latter effect was not present in their PC counterparts. In turn, girls were more motivated towards grades than boys in the PC groups, but this difference was not present in the other group. Finally, in both groups of students, girls presented a higher self-determination than boys. However, it is worth noting that the effect size in the case of the PC group was higher than in the nonPC groups. In the study by Glynn et al. (2011), a similar comparison was made between science and nonscience majors. In contrast with our results, these authors reported a similar behavior in both types of students, as SE and SD were the only variables significantly affected by gender in both groups. In spite of the importance of these findings, in accordance with our CHAID analysis, the interaction between motivation and gender does not seem to be crucial when explaining students’ future preferences for physics and chemistry at a high school level.
In agreement with our CHAID segmentation analysis, the lack of science self-efficacy could be an inhibitor for the choice of physics and chemistry in students with high career motivation and good GPA. These results back those reported by Salta et al. (2012). Consequently, our results show that the role of self-efficacy is less relevant when other motivational features are simultaneously taken into account. This finding seems to support those found by Dalgety and Coll (2006), who stated that chemistry self-efficacy predisposes students who achieved their goals in their first year chemistry to enroll in the second year.
Limitations and future studies
Besides the usual constraints of educational research, several limitations should be taken into account and addressed in future research. First, given the complex and multifaceted nature of the problem we undertook in this study, it would be interesting to incorporate more variables which could be relevant to explain students’ choices such as socio-economic aspects of their lives, school factors or the influence of other people. Second, since the students can opt out of physics and chemistry also in the following years and, in fact, a descent in the number of students has also been detected, it would be interesting to follow their whole trajectories along secondary education to study how motivation towards science changes over time and how it affects their future choices. Third, more applications of the SMQII would be desirable for cross-cultural studies and context comparisons. Finally, the complexity of the research on the droppint/opting out of science subjects raises the need for the use of mixed methods in which qualitative techniques may be useful tools to address important issues about this problem. For example, the study of students’ causal attributions about choosing or not chemistry-related courses should be tackled in future projects.
Conclusions and educational implications
The Spanish version of the SMQII has been proven to be valid for Spanish samples. Therefore, the validation we present in this paper broadens the applicability of this instrument to the context of Spanish-speaking countries in South America. This new adaptation would increase the possibilities of measuring motivational traits towards chemistry to chemistry education researchers which, in turn, would hopefully result in the improvement of teaching and learning strategies. Besides, the questionnaire can also be used by teachers and school counselors as a diagnostic tool.
The main conclusion of our study is that career motivation is a key factor when students have to decide whether to choose physics and chemistry once it is no longer a compulsory subject in their curriculum. In agreement with our findings, this motivational trait is more important than previous academic achievement and their intrinsic interest for science. Therefore, the decision seems to have a pragmatic component: the students make their choices on the basis of their prospective professional careers rather than backing their choice with sheer interest in chemistry-related courses. Several methods have been proven to be useful for providing career information and counseling, for example, the use of career-related examples in core curriculum instruction (Orthner et al., 2013) or shifting the learning experience to an everyday context (Potvin and Hasni, 2014). However, in agreement with a recent study in this field (Salonen et al., 2018), future attempts on integrating career-based activities into chemistry lessons should be carefully designed. These authors investigated the implementation of career-related instruction in secondary schools and very useful advice to teachers interested in this approach is given in their paper. It is important to note that, in spite of increasing students’ motivation and interest in science, the connection of the science topics with their future careers may not be straightforward due to their lack of knowledge about the possibilities and diversity of science careers. Given the successful application of programs like the Undergraduate Research Experience (Linn et al., 2015) in the promotion of scientific careers, an effective way to overcome instruction limitations in classrooms may be enriching student background with experiences in real professional environments (Jacobs, 2005).
Conflicts of interest
There are no conflicts to declare.
Acknowledgements
The authors would like to acknowledge the students, teachers and schools involved in the data gathering for their help. We are also thankful to the Colegio Oficial de Químicos de Asturias y León and la Asociación de Químicos del Principado de Asturias for their help with our first contact with some of the schools. A. P.-B. thanks the Spanish Ministerio de Educación, Cultura y Deporte for a grant (FPU15/03940).
References
- Abrahams I., (2009), Does Practical Work Really Motivate? A study of the affective value of practical work in secondary school science, Int. J. Sci. Educ., 31(17), 2335–2353.
- Aeschlimann B., Herzog W. and Makarova E., (2016), How to foster students’ motivation in mathematics and science classes and promote students’ STEM career choice. A study in Swiss high schools, Int. J. Educ. Res., 79, 31–41.
- Ainley M. and Ainley J., (2011), Student engagement with science in early adolescence: The contribution of enjoyment to students’ continuing interest in learning about science, Contemp. Educ. Psychol., 36(1), 4–12.
- Anderhag P., Emanuelsson P., Wickman P.-O. and Hamza K. M., (2013), Students’ Choice of Post-Compulsory Science: in search of schools that compensate for the socio-economic background of their students, Int. J. Sci. Educ., 35(18), 3141–3160.
- Anderhag P., Wickman P.-O., Bergqvist K., Jakobson B., Hamza K. M. and Säljö R., (2016), Why Do Secondary School Students Lose Their Interest in Science? Or Does it Never Emerge? A Possible and Overlooked Explanation, Sci. Educ., 100(5), 791–813.
- Arandia E., Zuza K. and Guisasola J., (2016), Attitudes and motivations towards physics and its learning at both high school and university, Int. J. Educ. Inf. Technol., 10, 58–65.
- Arbuckle J. L., (2010), SPSS (version 19.0) [Computer program], Chicago: SPSS.
- Austin A. C., Hammond N. B., Barrows N., Gould D. L. and Gould I. R., (2018), Relating motivation and student outcomes in general organic chemistry, Chem. Educ. Res. Pract., 19(1), 331–341.
- Bandura A., (1986), Social foundations of thought and action: a social cognitive theory, Englewood Cliffs, NJ: Prentice-Hall.
- Bandura A., (2001), Social Cognitive Theory: An Agentic Perspective, Annu. Rev. Psychol., 52(1), 1–26.
- Beaton D. E., Bombardier C., Guillemin F. and Ferraz M. B., (2000), Guidelines for the process of cross-cultural adaptation of self-report measures, Spine, 25(24), 3186–3191.
- Bennett J., Lubben F. and Hampden-Thompson G., (2013), Schools That Make a Difference to Post-Compulsory Uptake of Physical Science Subjects: some comparative case studies in England, Int. J. Sci. Educ., 35(4), 663–689.
- Black A. E. and Deci E. L., (2000), The effects of instructors’ autonomy support and students’ autonomous motivation on learning organic chemistry: a self-determination theory perspective, Sci. Educ., 84(6), 740–756.
- Blanco-López Á., España-Ramos E., González-García F. J. and Franco-Mariscal A. J., (2015), Key aspects of scientific competence for citizenship: a Delphi study of the expert community in Spain, J. Res. Sci. Teach., 52(2), 164–198.
- Blunch N. J., (2013), Introduction to Structural Equation Modeling Using IBM SPSS Statistics and AMOS, 2nd edn, Thousand Oaks, CA: Sage.
- Bøe M. V., (2012), Science Choices in Norwegian Upper Secondary School: What Matters? Sci. Educ., 96(1), 1–20.
- BøE M. V. and Henriksen E. K., (2013), Love It or Leave It: Norwegian Students’ Motivations and Expectations for Postcompulsory Physics, Sci. Educ., 97(4), 550–573.
- Bøe M. V., Henriksen E. K., Lyons T. and Schreiner C., (2011), Participation in science and technology: young people's achievement-related choices in late-modern societies, Stud. Sci. Educ., 47(1), 37–72.
- Broman K. and Simon S., (2015), Upper Secondary School Students’ Choice and Their Ideas on How to Improve Chemistry Education, Int. J. Sci. Math. Educ., 13(6), 1255–1278.
- Brotman J. S. and Moore F. M., (2008), Girls and science: A review of four themes in the science education literature. J. Res. Sci. Teach., 45(9), 971–1002.
- Bryan R. R., Glynn S. M. and Kittleson J. M., (2011), Motivation, Achievement, and Advanced Placement Intent of High School Students Learning Science, Sci. Educ., 95(6), 1049–1065.
- Byrne B. M., (2016), Structural Equation Modeling with AMOS: Basic Concepts, Applications, and Programming, 3rd edn, New York, NY: Routledge.
- Cerinsek G., Hribar T., Glodez N. and Dolinsek S., (2013), Which are my Future Career Priorities and What Influenced my Choice of Studying Science, Technology, Engineering or Mathematics? Some Insights on Educational Choice—Case of Slovenia, Int. J. Sci. Educ., 35(17), 2999–3025.
- Cheryan S., Ziegler S. A., Montoya A. K. and Jiang L., (2017), Why are some STEM fields more gender balanced than others? Psychol. Bull., 143(1), 1–35.
- Cleaves A., (2005), The formation of science choices in secondary school, Int. J. Sci. Educ., 27(4), 471–486.
- Cleveland L. M., Olimpo J. T. and DeChenne-Peters S. E., (2017), Investigating the Relationship between Instructors’ Use of Active-Learning Strategies and Students’ Conceptual Understanding and Affective Changes in Introductory Biology: A Comparison of Two Active-Learning Environments, Cell Biol. Educ., 16(2), ar19.
- Cohen L., Manion L. and Morrison K., (2011), Research Methods in Education, 7th edn, New York, NY: Routledge.
- Consejería de Educación y Cultura del Principado de Asturias, (2017), Resultados académicos de la educación asturiana 2015/2016, Consejería de Educación y Cultura del Principado de Asturias.
- Dalgety J. and Coll R. K., (2006), The influence of first-year chemistry students’ learning experiences on their educational choices, Assess. Eval. High. Educ., 31(3), 303–328.
- DeBoer G. E., (2000), Scientific Literacy: Another Look at Its Historical and Contemporary Meanings and Its Relationship to Science Education Reform, J. Res. Sci. Teach., 37(6), 582–601.
- DeWitt J. and Archer L., (2015), Who Aspires to a Science Career? A comparison of survey responses from primary and secondary school students, Int. J. Sci. Educ., 37(13), 2170–2192.
- DeWitt J., Archer L. and Osborne J., (2014), Science-related Aspirations Across the Primary–Secondary Divide: Evidence from two surveys in England. Int. J. Sci. Educ., 36(10), 1609–1629.
- Dillon J., (2009), On Scientific Literacy and Curriculum Reform, Int. J. Environ. Sci. Educ., 4(3), 201–213.
- Elster D., (2014), First-Year Students’ Priorities and Choices in STEM Studies–IRIS Findings from Germany and Austria, Sci. Educ. Int., 25(1), 52–59.
- European Commission, (2004), in Increasing human resources for science and technology in Europe: report of the High Level Group on Human Resources for Science and Technology in Europe, Gago J. M. (ed.), Luxembourg: Office for Official Publications of the European Communities.
- Feinstein N., (2011), Salvaging Science Literacy, Sci. Educ., 95(1), 168–185.
- Ferrell B. and Barbera J., (2015), Analysis of students’ self-efficacy, interest, and effort beliefs in general chemistry, Chem. Educ. Res. Pract., 16(2), 318–337.
- Ferrell B., Phillips
M. M. and Barbera J., (2016), Connecting achievement motivation to performance in general chemistry, Chem. Educ. Res. Pract., 17(4), 1054–1066.
- Gill T. and Bell J. F., (2013), What Factors Determine the Uptake of A-level Physics? Int. J. Sci. Educ., 35(5), 753–772.
- Gil-Pérez D. and Vilches A., (2005), The contribution of science and technological education to citizens’ culture, Can. J. Sci. Math. Technol. Educ., 5(2), 253–263.
- Glynn S. M. and Koballa Jr. T. R., (2006), Motivation to Learn in College Science, in Mintzes J. J. and Leonard W. H. (ed.), Handbook of College Science Teaching, Arlington, VA: National Science Teachers Association Press, pp. 25–32.
- Glynn S. M., Taasoobshirazi G. and Brickman P., (2007), Nonscience majors learning science: a theoretical model of motivation, J. Res. Sci. Teach., 44(8), 1088–1107.
- Glynn S. M., Taasoobshirazi G. and Brickman P., (2009), Science Motivation Questionnaire: construct validation with nonscience majors, J. Res. Sci. Teach., 46(2), 127–146.
- Glynn S. M., Brickman P., Armstrong N. and Taasoobshirazi G., (2011), Science motivation questionnaire II: validation with science majors and nonscience majors, J. Res. Sci. Teach., 48(10), 1159–1176.
- Gorard S., (2010), School experience as a potential determinant of post-compulsory participation, Eval. Res. Educ., 23(1), 3–17.
- Gorard S. and See B. H., (2009), The impact of socio-economic status on participation and attainment in science, Stud. Sci. Educ., 45(1), 93–129.
- Gulati P., Sharma A. and Gupta M., (2016), Theoretical study of decision tree algorithms to identify pivotal factors for performance improvement: a review, Int. J. Comput. Appl., 141, 19–25.
- Hampden-Thompson G. and Bennett J., (2013), Science Teaching and Learning Activities and Students’ Engagement in Science, Int. J. Sci. Educ., 35(8), 1325–1343.
- Harackiewicz J. M., Rozek C. S., Hulleman C. S. and Hyde J. S., (2012), Helping Parents to Motivate Adolescents in Mathematics and Science: An Experimental Test of a Utility-Value Intervention, Psychol. Sci., 23(8), 899–906.
- Hofstein A., Eilks I. and Bybee R., (2011), Societal Issues and Their Importance for Contemporary Science Education—A Pedagogical Justification and the State-of-the-Art in Israel, Germany, and the USA, Int. J. Sci. Math. Educ., 9(6), 1459–1483.
- Holbrook J. and Rannikmae M., (2009), The Meaning of Scientific Literacy, Int. J. Environ. Sci. Educ., 4(3), 275–288.
- Homer M. and Ryder J., (2015), The Impact of a Science Qualification Emphasising Scientific Literacy on Post-compulsory Science Participation: an analysis using national data, Int. J. Sci. Educ., 37(9), 1364–1380.
- Hurd P. D., (1998), Scientific literacy: new minds for a changing world, Sci. Educ., 82(3), 407–416.
- Isaacs B., (2001), Mystery of the Missing Women Engineers: A Solution, J. Prof. Issues Eng. Educ. Pract., 127(2), 85–91.
- Jacobs J. E., (2005), Twenty-five years of research on gender and ethnic differences in math and science career choices: what have we learned? New Dir. Child Adolesc. Dev., 2005(110), 85–94.
- Jefatura del Estado, (2013), Ley Orgánica 8/2013, de 9 de diciembre, para la mejora de la calidad educativa.
- Juuti K. and Lavonen J., (2016), How teaching practices are connected to student intention to enrol in upper secondary school physics courses, Res. Sci. Technol. Educ., 34(2), 204–218.
- Kachigan S. K., (1991), Multivariate statistical analysis, 2nd edn, New York: Radius Press.
- Kimmel L. G., Miller J. D. and Eccles J. S., (2012), Do the Paths to STEMM Professions Differ by Gender? Peabody J. Educ., 87(1), 92–113.
- Kline R. B., (2011), Principles and practice of structural equation modeling, 3rd edn, New York: Guildford Press.
- Lawson A. E., Banks D. L. and Logvin M., (2007), Self-efficacy, reasoning ability, and achievement in college biology, J. Res. Sci. Teach., 44(5), 706–724.
- Linn M. C., Palmer E., Baranger A., Gerard E. and Stone E., (2015), Undergraduate research experiences: impacts and opportunities, Science, 347(6222), 1261757.
- Liu Y., Ferrell B., Barbera J. and Lewis J. E., (2017), Development and evaluation of a chemistry-specific version of the academic motivation scale (AMS-Chemistry), Chem. Educ. Res. Pract., 18(1), 191–213.
- Longbottom J. E. and Butler P. H., (1999), Why Teach Science? Setting Rational Goals for Science Education, Sci. Educ., 83(4), 473–492.
- Lyons T., (2006a), Different Countries, Same Science Classes: Students’ Experiences of School Science in Their Own Words, Int. J. Sci. Educ., 28(6), 591–613.
- Lyons T., (2006b), The Puzzle of Falling Enrolments in Physics and Chemistry Courses: Putting Some Pieces Together, Res. Sci. Educ., 36(3), 285–311.
- McDonald C. V., (2016), STEM Education: a review of the contribution of the disciplines of science, technology, engineering and mathematics, Sci. Educ. Int., 27(4), 530–569.
- Ministerio de Educación, Cultura y Deporte, (2015), Orden ECD/65/2015, de 21 de enero, por la que se describen las relaciones entre las competencias, los contenidos y los criterios de evaluación de la educación primaria, la educación secundaria obligatoria y el bachillerato.
- Mujtaba T. and Reiss M. J., (2014), A Survey of Psyschological, Motivational, Family and Perceptions of Physics Education Factors that Explain 15-Year-Old Student's Aspirations to Study Physics in Post-Compulsory English Schools, Int. J. Sci. Math. Educ., 12(2), 371–393.
- Mujtaba T., Sheldrake R., Reiss M. J. and Simon S., (2018), Students’ science attitudes, beliefs, and context: associations with science and chemistry aspirations, Int. J. Sci. Educ., 40(6), 644–667.
- Muñiz J., Elosúa P. and Hambleton R. K., (2013), Directrices para la traducción y adaptación de los tests: segunda edición, Psicothema, 52(2), 151–157.
- OECD, (2016), PISA 2015 Assessment and Analytical Framework, OECD Publishing.
- Oon P. and Subramaniam R., (2010), Views of physics teachers on how to address the declining enrolment in physics at the university level, Res. Sci. Technol. Educ., 28(3), 277–289.
- Orthner D. K., Jones-Sanpei H., Akos P. and Rose R. A., (2013), Improving Middle School Student Engagement Through Career-Relevant Instruction in the Core Curriculum, J. Educ. Res., 106(1), 27–38.
- Osborne J., (2014), Teaching critical thinking? New directions in science education, Sch. Sci. Rev., 352, 53–62.
- Osborne J., Simon S. and Collins S., (2003), Attitudes towards science: a review of the literature and its implications, Int. J. Sci. Educ., 25(9), 1049–1079.
- Pajares F. and Schunk D. H., (2001), Self-beliefs and school success:
self-efficacy, self-concept, and school achievement, in Riding R. and Rayner S. (ed.), Perception, London: Ablex, pp. 239–266.
- Palmer T.-A., Burke P. F. and Aubusson P., (2017), Why school students choose and reject science: a study of the factors that students consider when selecting subjects, Int. J. Sci. Educ., 39(6), 645–662.
- Pintrich P. R., (2003), A Motivational Science Perspective on the Role of Student Motivation in Learning and Teaching Contexts, J. Educ. Psychol., 95(4), 667–686.
- Potvin P. and Hasni A., (2014), Interest, motivation and attitude towards science and technology at K-12 levels: a systematic review of 12 years of educational research, Stud. Sci. Educ., 50(1), 85–129.
- Quinn F. and Lyons T., (2011), High School Students’ Perceptions of School Science and Science Careers: A Critical Look at a Critical Issue, Sci. Educ. Int., 22(4), 225–238.
- Rocard M., Csermely P., Jorde D., Lenzen D., Walberg-Henriksson H. and Hemmo V., (2007), Science Education Now: A Renewed Pedagogy for the Future of Europe, Brussels, Belgium: Directorate-General for Research, European Commission.
- Rosenthal R., (1991), Meta-analytic procedures for social research, Newbury Park, CA: Sage.
- Roth W.-M. and Lee S., (2004), Science Education as/for Participation in the Community, Sci. Educ., 88(2), 263–291.
- Ryan R. M. and Deci E. L., (2000), Intrinsic and Extrinsic Motivations: Classic Definitions and New Directions, Contemp. Educ. Psychol., 25(1), 54–67.
- Salonen A., Kärkkäinen S. and Keinonen T., (2018), Career-related instruction promoting students’ career awareness and interest towards science learning, Chem. Educ. Res. Pract., 19, 474–483.
- Salta K. and Koulougliotis D., (2015), Assessing motivation to learn chemistry: adaptation and validation of Science Motivation Questionnaire II with Greek secondary school students, Chem. Educ. Res. Pract., 16(2), 237–250.
- Salta K., Gekos M., Petsimeri I. and Koulougliotis D., (2012), Discovering factors that influence the decision to pursue a chemistry-related career: a comparative analysis of the experiences of non scientist adults and chemistry teachers in Greece, Chem. Educ. Res. Pract., 13(4), 437–446.
- Schumm M. F. and Bogner F. X., (2016), Measuring adolescent science motivation, Int. J. Sci. Educ., 38(3), 434–449.
- Sha L., Schunn C. and Bathgate M., (2015), Measuring Choice to Participate in Optional Science Learning Experiences During Early Adolescence, J. Res. Sci. Teach., 52(5), 686–709.
- Shedlosky-Shoemaker R. and Fautch J. M., (2015), Who Leaves, Who Stays? Psychological Predictors of Undergraduate Chemistry Students’ Persistence, J. Chem. Educ., 92(3), 408–414.
- Sheldrake R., (2016), Students’ intentions towards studying science at upper-secondary school: the differential effects of under-confidence and over-confidence, Int. J. Sci. Educ., 38(8), 1256–1277.
- Sheldrake R., Mujtaba T. and Reiss M. J., (2015), Students’ intentions to study non-compulsory mathematics: the importance of how good you think you are, Br. Educ. Res. J., 41(3), 462–488.
- Sheldrake R., Mujtaba T. and Reiss M. J., (2017), Science teaching and students’ attitudes and aspirations: the importance of conveying the applications and relevance of science, Int. J. Educ. Res., 85, 167–183.
- Shirazi S., (2017), Student experience of school science, Int. J. Sci. Educ., 39(14), 1891–1912.
- Simpkins S. D., Davis-Kean P. E. and Eccles J. S., (2006), Math and Science Motivation: A Longitudinal Examination of the Links between Choices and Beliefs, Dev. Psychol., 42(1), 70–83.
- Smith E. and Gorard S., (2011), Is there a shortage of scientists? A re-analysis of supply for the UK, Br. J. Educ. Stud., 59(2), 159–177.
- Smyth E. and Hannan C., (2006), School Effects and Subject Choice: the uptake of scientific subjects in Ireland, Sch. Eff. Sch. Improv., 17(3), 303–327.
- Solbes J., (2011), ¿Por qué disminuye el alumnado de ciencias? Alamb. Did. Cienc. Exp., 67, 53–61.
- Solbes J., Montserrat R. and Más C. F., (2007), El desinterés del alumnado hacia el aprendizaje de la ciencia: implicaciones en su enseñanza, Did. Cienc. Exp. Soc., 21, 91–117.
- Stokking K. M., (2000), Predicting the choice of physics in secondary education, Int. J. Sci. Educ., 22(12), 1261–1283.
- Tripney J., Newman M., Bangpan M., Niza C., MacKintosh M. and Sinclair J., (2010), Subject Choice in STEM: Factors Influencing Young People (Aged 14–19) in Education: a systematic review of the UK literature, London: Wellcome Trust.
- Tuan H., Chin C. and Shieh S., (2005), The development of a questionnaire to measure students’ motivation towards science learning, Int. J. Sci. Educ., 27(6), 639–654.
- Tüysüz M., Yıldıran D. and Demirci N., (2010), What is the motivation difference between university students and high school students? Procedia – Soc. Behav. Sci., 2(2), 1543–1548.
- Ulriksen L., Madsen L. M. and Holmegaard H. T., (2010), What do we know about explanations for drop out/opt out among young people from STM higher education programmes? Stud. Sci. Educ., 46(2), 209–244.
- Vallerand R. J., Pelletier L. G., Blais M. R., Briere N. M., Senecal C. and Vallieres E. F., (1992), The Academic Motivation Scale: A Measure of Intrinsic, Extrinsic, and Amotivation in Education, Educ. Psychol. Meas., 52(4), 1003–1017.
- Van Langen A. and Dekkers H., (2005), Cross-national differences in participating in tertiary science, technology, engineering and mathematics education, Comp. Educ., 41(3), 329–350.
- Vázquez-Alonso Á. and Manassero-Mas M.-A., (2015), The Choice of Scientific and Engineering Higher Studies: Analysis of Some Influential Factors across Six Countries, Rev. Eureka Ens. Div. Cienc., 12(2), 264–277.
- Velayutham S., Aldridge J. and Fraser B., (2011), Development and Validation of an Instrument to Measure Students’ Motivation and Self-Regulation in Science Learning, Int. J. Sci. Educ., 33(15), 2159–2179.
- Yazilitas D., Saharso S., de Vries G. C. and Svensson J. S., (2017), The postmodern perfectionist, the pragmatic hedonist and the materialist maximalist: understanding high school students’ profile choices towards or away from mathematics, science and technology (MST) fields in the Netherlands, Gend. Educ., 29(7), 831–849.
|
This journal is © The Royal Society of Chemistry 2018 |
Click here to see how this site uses Cookies. View our privacy policy here.