The effect of math SAT on women's chemistry competency beliefs†
Received
21st July 2017
, Accepted 24th November 2017
First published on 27th November 2017
Abstract
In chemistry, lack of academic preparation and math ability have been offered as explanations as to why women seem to enroll, perform, and graduate at lower levels than men. In this paper, we explore the alternative possibility that the gender gap in chemistry instead originates from differential gender effects of academic factors on students’ motivation. Using a sample of approximately 670 students enrolled in a mid-sized university in the United States we conducted: (1) t-tests to understand incoming academic differences between freshman students by gender, (2) regression analysis to determine which academic and attitudinal factors predict success in General Chemistry 1, and (3) a mediation analysis to understand the underlying mechanisms of how academic performance affects students’ beliefs about their competency in chemistry, which in turn has an effect on chemistry achievement. We demonstrate the importance of math ability as a contributor to chemistry achievement, but further that ability differences in math are important because they affect students’ chemistry competency beliefs. Critically, this link between ability and competency beliefs is stronger for women than men. These results suggest that interventions geared towards improving women's chemistry competency beliefs could have an important influence in improving their achievement in the classroom, and in consequence reduce the gender gap in chemistry.
Introduction
Despite decades of effort, there is still a sizable gender gap in science (National Science Foundation, 2017). Proposed explanations for the gap include: (1) differences in intrinsic skills like spatial ability (Wai et al., 2009); (2) lack of preparation as documented by lower scores on standardized math testing (Cole, 1997); (3) attitudinal differences towards science (Quinn and Spencer, 2001; Carlone and Johnson, 2007; Hazari et al., 2010; Vincent-Ruz and Schunn, 2017); (4) biased curricula and pedagogy (Hughes, 2000; 2001); (5) an exclusionary culture of science (Moss-Racusin et al., 2012; Legewie and DiPrete, 2014); and (6) identities that do not include science (i.e., women are less likely to identify themselves as “science people” (Archer et al., 2010; Hazari et al., 2010)). However, the degree of under-representation of women in science is quite heterogeneous by science discipline, ranging from large gaps with no progress across thirty years to slight over-representation of women (National Student Clearinghouse Research Center, 2015). So, it is important to understand underlying mechanisms within each discipline.
For example in chemistry, lack of academic preparation (M. H. Andrews and Andrews, 1979; Boli et al., 1985; Darlington and Bowyer, 2016), spatial ability (Carter and Larussa, 1987), and motivation (Ferrell and Barbera, 2015; Ferrell et al., 2016) have regularly been implicated by research, especially at the university level. From a mechanistic perspective, each of these has their own plausible bases, so it is possible that all three sources are independent causes (e.g., spatial ability differences cause challenges for reasoning about three-dimensional molecular structure and motivational differences cause debilitating anxiety during exams or less persistence in the face of early failures). However, the mechanisms by which these differences emerge are unclear. For example, it is possible that these differences are a result of mediated relationships (e.g., ability differences cause motivational differences). Therefore, it is theoretically important (to understand mechanisms) and practically important (to target root causes) to explore mediational relationships and gender differences in mediational relationships. As the current work will show, prior studies have over-estimated the direct effects of academic ability differences on chemistry performance in university settings by ignoring motivation and attitude effects. Further, we argue that links between ability differences and motivation can shed light on the origin of gender gaps in chemistry. Specifically, we suggest that apart from simple differences in ability, men and women make different inferences about their competency in chemistry based on prior evaluations of their math ability levels. We begin with a review of arguments and evidence for mathematics and related cognitive abilities in chemistry learning, and gender differences in these abilities.
Mathematical skills and abilities and chemistry learning
Some fundamental mathematical skills and abilities are necessary to perform chemical calculations and understand concepts, such as for stoichiometry, Ideal Gas Law, and balancing equations (Weisman, 1981). These foundational skills include use of parentheses, manipulation of fractions, use of decimals, understanding of exponents, understanding of percentages, manipulation of one-variable equations, and understanding of ratio and proportion (Denny, 1971; Weisman, 1981; Gabel and Sherwood, 1984). Spatial reasoning is also an important ability related to mathematical skills. Spatial reasoning is needed to understand three-dimensional representations (Donaghy and Saxton, 2012) and organic chemistry concepts (Stieff et al., 2012), and to predict the structures of ionic solids (Carter and Larussa, 1987). As these foundational skills and abilities are important for early success in chemistry, it is important to consider whether there are gender differences in these skills.
Women have historically had a lower mean score on the mathematics component of university admissions exams in the US such as the SAT and ACT (Friedman, 1989; Camara, 2000), and differences remain despite the gap gradually closing over time (Allspach and Briening, 2005). Internationally, the gender gap in mathematics is more variable, but it is still found at the top performance levels (Bramley et al., 2015). Therefore, one could imagine that women would struggle more on mathematics-infused chemistry topics at university. However, that explanation seems implausible given the typical characteristics of students enrolling in science classes in college. Both men and women with poor math scores are unlikely to enroll in mainstream physical science courses like General Chemistry or Organic Chemistry (Crisp et al., 2009). Also, the foundational math skills identified in the literature tend to be the more basic concepts on the admission exams. More advanced concepts like higher-order equations, trigonometry, and complex numbers are not needed for introductory university chemistry courses.
Gender differences in chemistry achievement have also been discussed in terms of gender differences in spatial ability (Casey et al., 1995; Rohde et al., 2007). Gender differences in spatial abilities are consistently found, although the gap reduces with age (Linn and Petersen, 1985). However, this gender difference is not necessarily biological, since it can be partially explained by differential experiences (Baenninger and Newcombe, 1989) and testing conditions (Goldstein et al., 1990); it can even be removed through training manipulations such as playing video games (Feng et al., 2016). In addition, spatial ability has a stronger correlation with mathematics performance when it comes to women (rwomen = 0.35, rmen = 0.13) (Casey et al., 1995), which hints at motivational rather than purely cognitive effects. Further, observed correlations of spatial ability with chemistry performance occur even for chemistry topics that are not spatial (e.g., stoichiometry), raising a question about actual underlying mechanisms. That is, are women underperforming on college chemistry classes due to the connection of math to a semi-malleable skill like mental rotation ability or is there another underlying issue?
Thus, despite the fact that SAT math is a strong statistical correlate of chemistry performance, it does not fully explain why women would under-perform in Chemistry. Rather than propose an ability basis for this statistical relationship, here we propose a motivational mediation.
Gender differences in chemistry competency beliefs
In this report, we build on prior research suggesting that stereotypes about women and science, especially physical sciences that are perceived to rely heavily on mathematics (Vockell and Lobonc, 1981; Jacobs, 2005; Beilock et al., 2010), may be one important factor impacting women's outcomes in chemistry (Pajares, 2002). In particular, stereotypes that women are less capable in math and math-related disciplines may make women susceptible to adopting lower estimates of their competency in chemistry. Chemistry competency beliefs describe the level of confidence that students have in their ability to be successful at solving a chemistry-related activity or having a chemistry-related skill (Dalgety and Coll, 2006b; Boz et al., 2016). Such attitudinal factors have been consistently implicated in chemistry learning (Dhindsa and Chung, 1999; Herron and Nurrenbern, 1999), even when controlling for prior knowledge and mathematical ability (Xu et al., 2013). Competency beliefs have been shown to be important for success in university-level chemistry (Ferrell et al., 2016), and gender differences are consistently found in chemistry competency beliefs (Bong, 1999; Dalgety and Coll, 2006a; Tenaw, 2013). As such, evidence points to competency beliefs as an important source of the gender gap in chemistry education outcomes.
However, very little research has sought to understand the sources of gender differences in competency beliefs, nor has research sought to understand the role of competency beliefs in the mapping between students’ math ability (e.g., as reflected by SAT math scores) and their college chemistry outcomes (e.g., course grades). In this report, we test a model explaining how men and women may derive different competency beliefs from the same performance information (e.g., SAT math scores), and we seek to show how such beliefs may in turn shed light on the gender gap in college chemistry outcomes.
Although not focused specifically on chemistry, research in psychology has explored the factors that shape competency beliefs following different forms of academic feedback (e.g., receiving high versus low scores on an academic tasks). Such research has generated several insights pertinent to the present research. For example, one line of work found that people who have generalized doubts about their adequacy and ability (i.e., lower self-esteem) tend to overgeneralize the implications of negative academic feedback. For example, in one study, participants received feedback that they had either succeeded or failed at a cognitive task (a remote-associates test). Compared to when they succeeded, participants with low self-esteem reported significantly lower feelings of self-worth, lower academic competency, and lower intelligence following failure feedback (Brown and Dutton, 1995). By contrast, participants with high self-esteem—that is, participants who felt confident in their personal adequacy and abilities at the outset—showed no such declines following failure feedback. It was as though participants who doubted themselves in the first place tended to regard failure as a confirmation of their initial doubts. They tended to see themselves as less capable, not just at the task at hand, but more globally as well. Participants who did not doubt themselves, by contrast, tended not to tie poor outcomes in one domain to their abilities in other domains.
We argue that a similar effect may occur for men and women's chemistry competency beliefs. Namely, we suggest that prevalent negative stereotypes about women in mathematics may plant doubts that influence the inferences women draw from their SAT math scores. That is, women, on average, may hold greater doubts about their mathematics abilities, analogously to the participants with low self-esteem in the aforementioned study. If they then receive relatively low scores in SAT math, women, more so than men, may tend to see such an outcome as a more general confirmation of the stereotypes and, therefore, they may be more likely to generalize their math scores to their competency beliefs about related areas, like physical sciences. In other words, we predict that men and women receiving relatively low scores on the SAT math will come away with different chemistry competency beliefs. Whereas women will regard their low math score as a confirmation of a general negative stereotype, men will see their math score as more isolated and not as relevant to their chemistry competency beliefs. The result will be that women on average will hold lower chemistry competency beliefs than men.
Lower competency beliefs, in turn, may contribute to lower performance in college chemistry. For example, lower competency beliefs can lead to excessive worrying during exams, which may reduce performance (Quinn and Spencer, 2001). In terms of gender, lower competency beliefs may make women more susceptible to stereotype threat, which describes the fear of confirming negative stereotypes about one's group and has been shown to sap cognitive resources. In other words, cultural stereotypes may shape the inferences that women make about their SAT math performance, which may then foster generalized doubts about competency in chemistry, which further depresses performance, and so on in a recursive cycle. We seek to uncover evidence for this cycle by collecting SAT math scores of men and women from high school, measuring their self-reported competency beliefs in college, and then examining their downstream academic outcomes in college chemistry.
Research questions
In this paper, we propose that the gender achievement gap in science that emerges during the early university years is a consequence of women's competency beliefs having a growing impact on their academic achievement, rather than from inherent differences in relevant academic preparation. Specifically, we asked the following research questions in a study of first-year university students in a General Chemistry I class.
(1) Are there important academic ability and attitudinal differences between men and women even among the selective set of students enrolled in General Chemistry?
(2) Which academic ability and attitudinal factors are independent predictors of performance in General Chemistry?
(3) How do these factors interrelate in explaining gender differences in General Chemistry performance? Does the strength of these relationships vary by gender?
Methods
Participants
Our sample consisted of approximately 670 students enrolled across 7 General Chemistry I sections (classrooms) in their first semester in a mid-sized research university in the US. Table 1 contains descriptions of the different sections of course; most of the sample came from the version of General Chemistry I open to all students in the School of Arts & Sciences. Demographics for the sample are also shown in Table 1, revealing that a slight majority of students identified as women and 70% of the students were Caucasian. The university provided researchers students’ records and grades in a de-identified manner.
Table 1 Gender, ethnicity information, along with proportion of students in each class type
Variable |
Indicator |
% of students |
“In the survey”, students were asked about their gender identity. 0.8% preferred not to answer the question and 0.4% of them identified as transgender or genderqueer. Though we recognize the importance of non-binary gender identity as a factor of attrition in STEM, we elected to remove the non-binary cases due to their small sample size in this dataset.
“Regular” General Chemistry I class covers the fundamental properties of matter, including stoichiometry, the properties of solids, liquids and gases, thermochemistry and the electronic structure of atoms and molecules. This is the course offered to the majority of students enrolled in the School of Arts & Sciences. The “Honors” sections additionally cover periodic behavior, theories of bonding, and spectroscopy. To enroll in honors classes, students must have high standardized assessment scores, have an “A” average in a challenging high school curriculum, and be in the top 5 percent of their high school class. The “Engineering” sections cover the same material as the “Regular” class but emphasize applications to industrial, environmental chemistry, and biochemistry. This class is offered only to students in the Engineering School but is taught by the Chemistry Department.
|
Gendera |
Female = 1 |
57 |
Male = 0 |
43 |
|
Ethnicity |
White = 1 |
69 |
Asian = 1 |
15 |
Black = 0 |
6 |
Latinx = 0 |
3 |
Other = 0 |
7 |
|
General Chemistry I classb |
Regular |
67 |
Engineering |
29 |
Honors |
4 |
IRB procedure
Since the current study used data which involve privacy concerns, it required review and approval by the university's Institutional Review Board. In fact, it was approved via an expedited IRB that involved connecting survey and institutional data without revealing student identities to the research team using an honest broker system, along with two exempt IRB protocols respectively covering the use of surveys (with consent) and existing institutional data (no consent required).
Measures
The institutional data provided demographics (sex, race/ethnicity, parental income, and first-generation status),‡ Advanced Placement (AP) scores, SAT scores,§ and grades for all university courses. AP courses are elective high school courses that are meant to be equivalent to introductory university courses. Students are given a standard end-of-year assessment that is independently scored by the College Board. In practice, some universities count these courses as substitutes if students obtain a score of 4 or 5 on the exam, but selective universities rarely do so; nonetheless, any prior exposure to advanced concepts can help student performance at university. Therefore, we include two measures: whether students took AP Chemistry and what score they obtained on the national test.
Additionally, students completed an online attitudinal survey (in exchange for extra credit in class) during the first few weeks of the semester to produce measures of Chemistry Fascination (interest in and mastery goals for chemistry content and skills), Chemistry Values (perceived value of chemistry knowledge for everyday life), Chemistry competency beliefs (beliefs about being having chemistry-related skills and being able to successfully complete chemistry-related tasks), Mathematics Competency Beliefs (beliefs about being able to successfully complete mathematics-related tasks) and Science Identity (self and other perception of student as science type of person). All items were 4-point Likert style items (Strongly Agree–Agree–Disagree–Strongly Disagree), and scale scores were computed by calculating the mean across items.
The attitudes measures were developed by adapting previously validated scales: Colorado Learning Science Survey for Use in Chemistry (CLASS-Chem) (Adams et al., 2008), the Chemistry Self-Concept Inventory (CSCI) (Bauer, 2005), a science identity survey (Hazari et al., 2010), and a math competency beliefs survey (Good et al., 2003). Additional psychometric analyses (Exploratory Factor Analyses and Item Response Theory analyses) were conducted based on pilot data in multiple levels of university coursework to remove poorly behaving survey items and insure overall acceptable scale properties. See ESI† for sample items and additional psychometric information for each scale.
Reliability and means, are provided in Table 2, while inter-correlations of the resulting scales are provided in Table 3. Reliability for all measures are all above 0.75, meaning they are all have acceptable internal consistency (Tavakol and Dennick, 2011). All the attitudinal measures, except math competency beliefs were slightly skewed right, which is not surprising given that students that choose to enroll in chemistry tend to be science oriented. All attitudinal measures were moderately correlated with one another, consistent with previous research, and further highlighting the need for research to measure each of them to unpack unique contributions (Bandura, 1995).
Table 2 Measure means, standard deviations, observed min and max, and skew. The number of items and scale reliabilities are presented for attitudinal scales
|
Variable |
Mean |
SD |
Min |
Max |
Skew |
Academic |
SAT math |
678 |
70 |
480 |
800 |
−0.05 |
SAT writing |
628 |
75 |
400 |
800 |
−0.11 |
AP chemistry |
3.2 |
1.0 |
1 |
5 |
0.1 |
|
Variable |
Mean |
SD |
Min |
Max |
Skew |
# items |
α
|
Attitudinal |
Chemistry fascination |
2.85 |
0.63 |
1 |
4 |
−0.3 |
3 |
0.77 |
Chemistry values |
2.93 |
0.50 |
1.5 |
4 |
−0.41 |
6 |
0.78 |
Chemistry competency beliefs |
2.72 |
0.57 |
1 |
4 |
−0.38 |
4 |
0.85 |
Math competency beliefs |
2.73 |
0.40 |
1.67 |
3.75 |
0.14 |
4 |
0.84 |
Science identity |
3.36 |
0.54 |
1.5 |
4 |
−0.56 |
4 |
0.89 |
Table 3 Correlations between academic and attitudinal scales
|
SAT math |
SAT writing |
AP chemistry |
Fascination |
Values |
Chem CB |
Math CB |
Identity |
*, **, and *** denotes significance at the 0.10, 0.05, and 0.01 levels, respectively. |
Academic |
SAT math |
1 |
|
|
|
|
|
|
|
SAT writing |
0.54*** |
1 |
|
|
|
|
|
|
AP chemistry |
0.54*** |
0.46*** |
1 |
|
|
|
|
|
|
Attitudinal |
Chemistry fascination |
−0.09
|
−0.05
|
0.11
|
1 |
|
|
|
|
Chemistry values |
−0.08
|
−0.07
|
0.07
|
0.69*** |
1 |
|
|
|
Chemistry competency beliefs |
0.23** |
0.12
|
0.39*** |
0.47*** |
0.39*** |
1 |
|
|
Math competency beliefs |
0.20* |
0.11
|
0.08
|
0.23** |
0.24** |
0.35*** |
1 |
|
Science identity |
0.13
|
0.22* |
0.19* |
0.24** |
0.30*** |
0.31*** |
0.30*** |
1 |
Survey administration
Attitudinal surveys were all administered in an online format as a single survey. Approximately two weeks after the school term started (i.e., once enrollment was finalized), students received an email from our honest broker with instructions and the survey link. The email noted the collaboration with a research team, extra-credit given for completion of the survey, and assurances that the instructor would not be shared the instructor. Students had 2 weeks to complete the surveys to ensure responses were obtained before the administration of the first exam.
Analytic approach
The reported analyses involve multiple linear regression and mediation analyses. Analyses were conducted corresponding to the three research questions as follows:
(1) For every academic and attitudinal student characteristic, we conducted an independent sample t-test between women and men separately to assess initial differences in underlying distributions by gender (RQ1)
(2) We constructed a multiple regression model to test which factors are independent predictors of performance in General Chemistry 1 (RQ2)
(3) We conducted mediation analyses using attitudinal variables, with possible moderation by gender (Fig. 1), to identify underlying mechanisms for interactions obtained in the previous step (RQ3)
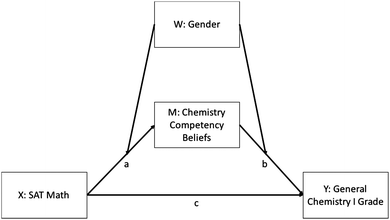 |
| Fig. 1 Relationship between SAT math and General Chemistry I grade mediated by chemistry competency beliefs and moderated by gender. Where X (inependent variable), Y (dependent variable), M (mediator), and W (moderator) represent the variables of inteterest to our research question. | |
Multiple regression model building procedure
Models were systematically built in ways supported by prior theories/empirical findings and best practice in statistical methods (Box, 1979) using the following steps:
(1) Including key control predictors: variables that are most likely have a strong relationship to General Chemistry I grade (e.g., AP Chemistry Scores) (Xu et al., 2013).
(2) Adding key variables of interest for a given research question (e.g., SAT math).
(3) Adding variables that according to prior research could also have also have an effect on final grades (e.g., gender, race/ethnicity, first generation status, SAT Verbal, SAT writing) (Hazari et al., 2010; Oyserman et al., 2016). These variables were only kept in the model when statistically significant.
(4) Checking rival hypothesis: other variables that could offer alternative explanations to observed patterns (e.g., attitudinal variables like math competency beliefs, science identity and chemistry competency beliefs.) (Bauer, 2005; Xu et al., 2013; Ferrell and Barbera, 2015).
Mediation analysis procedure
To test the full model that was derived from our literature review, we used the PROCESS v.2 SPSS macro (Hayes, 2012). PROCESS uses ordinary least squares regression-based path analytic framework for estimating direct and indirect effects in models testing moderation, mediation, and various types of moderated-mediation. The analysis employs a non-parametric bootstrapping procedure (Preacher and Hayes, 2004), in which a subset of the participants is randomly sampled with replacement 1000 times. The proposed model (direct and indirect effects) is estimated with each of the 1000 samples. Mean and confidence intervals for the effect parameters are calculated. If the confidence interval around a relevant statistic does not include zero, we infer that the effect of interest is statistically significant. This approach yields increased power over the more traditional Sobel test, which does not appropriately handle the typically skewed distributions of indirect effects.
Our proposed moderated-mediation model (based upon PROCESS Model Template 7 in Hayes, 2012) is shown in Fig. 1. It tests whether part of the relation between SAT math (X) and Chemistry grades (Y) is mediated (M) through chemistry competency beliefs. In addition, based on the prior literature, it statistically tests whether the mediation is moderated by Gender (W). That is, we hypothesized that gender will vary the strength for this relationship between men and women; this is formally called a moderated-mediation.
Results and analysis
Gender differences in academic ability and attitudinal differences in general chemistry students
Before assessing which academic and attitudinal factors are important for success in General Chemistry 1, it is important to first assess which ability and attitudinal variables show gender differences within this select university population. We applied t-tests by gender on each of the ability and attitudinal variables. Table 4 shows means by gender along with t-tests results and effect size using Cohen's d (Cohen, 1992).¶ In terms of academic measures, we only found statistically significant differences for SAT math, where women on average had lower scores. This difference had a medium effect size, consistent to what has been previously reported in the US (Friedman, 1989). We also found statistically significant differences in all attitudinal measures. However, most of them had small effect sizes, except for chemistry competency beliefs, which had a medium size effect with women having a lower mean score. Differences in competency beliefs across different science subjects favoring males have also been reported previously (Ferrell and Barbera, 2015; Boz et al., 2016; Vincent-Ruz and Schunn, 2017).
Table 4 Means for important academic and motivational factors, separately by gender, along with Cohen's d and t-test p-values for the gender difference
Variable |
Men |
Women |
Cohen's d |
p-Value |
Academic |
SAT math |
697 |
662 |
−0.51 |
<0.001 |
SAT writing |
626 |
631 |
0.10 |
0.19 |
High AP chemistry score |
26% |
22% |
−0.06 |
0.27 |
Low AP chemistry score |
16% |
15% |
−0.05 |
0.52 |
Took AP chemistry |
26% |
22% |
−0.08 |
0.27 |
|
Attitudinal |
Chemistry competency beliefs |
2.88 |
2.59 |
−0.53 |
<0.001 |
Chemistry fascination |
2.94 |
2.77 |
−0.26 |
<0.001 |
Science identity |
3.26 |
3.41 |
0.28 |
<0.001 |
Math competency beliefs |
2.80 |
2.67 |
−0.30 |
<0.001 |
Predictors of performance in general chemistry
Since men had higher SAT math and attitudinal scores than women in this sample, the next critical question involved whether these factors were predictive of General Chemistry 1 performance. We first developed a regression model with academic preparation variables as predictors and General Chemistry 1 performance as the dependent variable. Model 1 presented in Table 5 includes only statistically significant predictors. Students who took AP chemistry in high school tended to outperform students who did not take AP Chemistry. This was true even for students who did not perform well in AP Chemistry (scores of 1 to 3), as indicated by the positive relationship comparing low performing AP Chemistry students to students who did not take AP Chemistry. This effect may reflect motivation differences (explored below) or more recent exposure to the material. Most saliently, SAT math was the strongest predictor of students’ General Chemistry I grade, with a beta weight that was even larger than the beta associated with taking AP Chemistry and doing well on that exam.
Table 5 Two linear regression model results for General Chemistry 1 grade using only academic factors or both academic and attitudinal factors. Models control for gender, race/ethnicity, and first-generation status (not shown)
|
Model 1: academic factors |
Model 2: academic + attitudinal factor |
Std beta |
p-Value |
Std beta |
p-Value |
Academic factors |
SAT math |
0.25 |
<0.001 |
0.20 |
<0.001 |
SAT writing |
0.20 |
<0.001 |
0.20 |
<0.001 |
|
AP chemistry |
High vs. didn’t take |
0.23 |
<0.001 |
0.16 |
0.002 |
Low vs. didn’t take |
0.15 |
<0.001 |
0.12 |
0.004 |
|
Attitudinal factors |
Chemistry competency beliefs |
|
|
0.16 |
0.003 |
Chemistry fascination |
|
|
0.15 |
0.002 |
Science identity |
|
|
0.12 |
0.004 |
|
Observations |
407 |
403 |
Adjusted R2 |
0.28 |
0.39 |
As noted in the introduction, this SAT math effect size seems too large for this relatively selective population (i.e., Math SATs all above the national mean) and the kind of mathematics that General Chemistry requires. We obtained and content analyzed the exams administered that semester. Similar to prior such analyses (Weisman, 1981): (1) General Chemistry 1, as tested, is mostly a conceptual course, rather than an applied mathematics course, and (2) the problems that require mathematics skills can be solved using basic algebra concepts.
Next, we added attitudinal predictors into the regression. Model 2 in Table 5 presents the estimates of direct effects of the three attitudinal variables that were significant predictors of General Chemistry grades (when controlling for academic preparation variables). These attitudinal variables explain an additional 10% of the variance, and Chemistry Self-efficacy and Fascination were the stronger predictors. These variables had effects similar in size to the effect of having taken AP chemistry and scoring well on the exam.
Particularly relevant to our research questions, there is a reduction from Models 1 to 2 (see Table 5) in the standardized beta coefficient for both SAT and AP Chemistry academic factors, suggesting a possible mediation mechanism. That is, receiving a high SAT math score may have shaped students’ attitudes and beliefs about their competency. By instilling confidence and a sense of efficacy, high SAT math performance may have contributed to stronger competency beliefs, which, in turn, shaped students’ Chemistry performance. More formally, we hypothesized that students’ competency beliefs mediated the relationship between SAT math scores and General Chemistry 1 outcomes (explored in the next section).
Mediation of effects and moderation by gender
We tested mediation regression models with the different attitudinal variables as possible mediators between SAT mathematics and General Chemistry grades. The only significant mediator was chemistry competency beliefs, a somewhat surprising result given it was competing against math competency beliefs, which, on face value, is more directly related to math skills.
In addition, we tested whether gender moderated the mediation (i.e., whether the mediation was present and equally large for both genders). To formally test and localize moderation effects, we calculated an index of moderated mediation (Hayes, 2015) and tested a possible moderation of gender in both a & b paths in Fig. 1. We found an index of −0.18 (lower CI: −0.36, upper CI: −0.06) for path a & an index of −0.0025 (lower CI: −0.0056, upper CI: 0.0003), indicating there was a significant pattern of moderated mediation on path a but not on path b (i.e., gender changes the strength of the relation between SAT math and chemistry competency beliefs, but not the strength of the relation between chemistry competency beliefs and General Chemistry performance). Model 3 (Table 6) shows the beta coefficients for this moderated relationship (path a). To better understand this relationship, we plotted the estimated marginal means of the moderation (see Fig. 2): means by gender for different levels of SAT math scores that are also adjusted for differences in other academic covariates (AP Chemistry and SAT writing). Both genders show a connection between SAT math and chemistry self-efficacy (i.e., both slopes are bigger than zero), but the relationship is much stronger in women (i.e., the slope for women is larger). There is almost no self-efficacy gender difference at high SAT math levels, but there is a large gender difference at lower SAT math levels.
Table 6 Linear regression parameters for Model 3 (predicting chemistry competency beliefs using academic variables) and Model 4 (predicting General Chemistry grades using both academic and attitudinal variables). Both models control for AP chemistry scores and SAT writing (not shown)
|
Model 3: chemistry competency beliefs |
Model 4: general chemistry I grade |
Beta |
p
|
Beta |
p
|
z-SAT math |
0.66 |
<0.001 |
0.89 |
<0.001 |
Female |
0.35 |
<0.001 |
|
|
z-SAT math × female |
−0.23 |
0.005 |
|
|
z-Chemistry CB |
|
|
0.96 |
<0.001 |
|
Observations |
526 |
526 |
Adj. R2 |
0.17 |
0.15 |
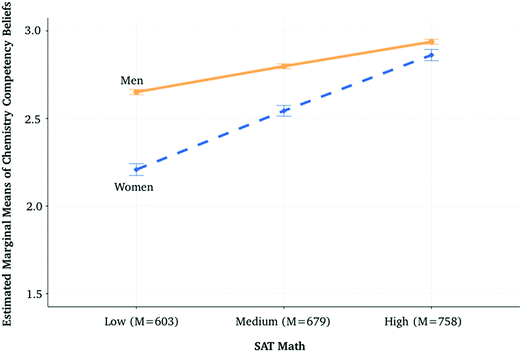 |
| Fig. 2 Estimated marginal means of chemistry competency beliefs by math SAT and gender. | |
In sum, the relationship between SAT math and General Chemistry I involves moderated mediation with gender and competency beliefs. A moderated mediation is present when the mediation is only significant at certain levels of a moderator variable (Muller et al., 2005), in other words when the mediating process is dependent on the context (D. Muller et al., 2005). In this case, the mediation involves competency beliefs and the strength of the mediation is dependent of the context of being a woman. This result is interesting because it not only shows that an academic variable is partially explained by attitudinal factors, but it also suggests that specifically women's achievement in General Chemistry I could be improved by helping them improve their chemistry competency beliefs.
General discussion
We revisit each of our primary research questions to discuss theoretical and practical implications of our findings.
Initial gender differences in general chemistry students
Among the relatively selective students enrolled in General Chemistry I university classes, we found two gender differences that are at least moderate in size: SAT math and chemistry competency beliefs. Both of these differences have been documented on the literature (Casey et al., 1995; Allspach and Briening, 2005; Dalgety and Coll, 2006b; Vincent-Ruz and Schunn, 2017), and, as mentioned earlier, these differences are rooted in gendered social expectations leading to different experiences rather than biological effects (Carter and Larussa, 1987; Baenninger and Newcombe, 1989; Burkam et al., 1997; Quinn and Spencer, 2001). Although SAT scores varied across the participants, overall participants in this sample generally had relatively high SAT math scores (almost two standard deviations above the national pool), meaning that poor mathematics preparation should, theoretically, not be a major factor in chemistry performance.
Performance predictors in general chemistry
As both academic and attitudinal factors have been put forward as possible explanations for achievement differences between men and women in chemistry (M. H. Andrews and Andrews, 1979; Xu et al., 2013; Ferrell and Barbera, 2015; Darlington and Bowyer, 2016), we built multiple regression models to directly contrast these competing hypotheses. SAT math was a strong predictor when only considering academic preparation variables along with performance on AP Chemistry. Critically, some of the effects of both of these academic preparation factors appear to be attitudinal in nature. That is, weaker outcomes from high school appear to lead to poor university performance in part because they reduces a student's self-confidence rather than only because they limits what students can do.
Based on prior research, one could have expected that the influence of SAT math on General Chemistry I to be partially explained through math competency beliefs, since students with low math competency beliefs can suffer from anxiety while performing math problems (Quinn and Spencer, 2001; Beilock et al., 2010). However, we found that the pathway through which SAT math appears to influence General Chemistry I performance was through chemistry competency beliefs. This relationship between SAT math and chemistry competency beliefs may be due to a stereotype that all physical sciences heavily rely on mathematics (Vockell and Lobonc, 1981; Jacobs, 2005; Beilock et al., 2010).
Why competency beliefs play such an important role for gender effects in chemistry performance
Given beliefs about chemistry and mathematics, and stereotypes about women's mathematics performance (Quinn and Spencer, 2001), it may not be surprising that women exhibited a much stronger relationship between SAT math and chemistry competency beliefs than did men. That is, women were more likely to translate their performance feedback on the SATs into self-efficacy beliefs. Furthermore, it was interesting that the relationship between SAT math and chemistry grade was mediated by chemistry competency beliefs and not by math competency beliefs. That is, the mechanism doesn’t seem to be through anxiety or tension while performing math tasks (Spencer et al., 1999) related to chemistry but to a possible overgeneralization of the importance of mathematical skills for overall success in chemistry. Prior research suggests that the higher the competency beliefs, the more a student will think their success was due to their own competencies rather than luck or simplicity of the task (Pajares, 2002). We hypothesize that when negative stereotypes of women being “bad at math” have been internalized and affect competency beliefs, students are more likely not only to score lower (Xu et al., 2013) but also to attribute success to chance and therefore make it harder to improve their competency beliefs through new feedback.
Conclusions
The observed moderated mediation has important theoretical and pragmatical aspects. Theoretically, the current findings suggests that the gender differences in competency beliefs are likely linked to social expectations of women's math performance and abilities (Vockell and Lobonc, 1981; Spencer et al., 1999; Quinn and Spencer, 2001), otherwise the SAT-competency belief relationship would not be gendered. A second theoretical contribution comes from the observed relationship between math performance and chemistry domain-specific competency beliefs. Domain-specific competency beliefs are generally conceptualized as determined by performance within that domain (Bandura, 1995). However, our results suggests that students likely hold a personal epistemology of science in which math plays a central role, thus enabling a broadly generalized effects of math performance on competency belief beyond just mathematics. Practically speaking, the current study points to underlying mechanisms of gender gaps in chemistry performance, which is important for developing effective interventions. In particular, these results suggest that interventions geared towards improving women's chemistry competency beliefs could have an important influence in improving their achievement in the classroom, and thereby reducing the gender gap.
Conflicts of interest
There are no conflicts to declare.
References
- Adams W. K., Wieman C. E., Perkins K. K., and Barbera J., (2008), Modifying and validating the Colorado Learning Attitudes about Science Survey for use in chemistry, J. Chem. Educ., 85(10), 1435.
- Allspach J. R. and Briening K., (2005), Gender Differences and Trends over time for the SAT Reasonong Test. Educational Testing Service, Retrieved from http://https://www.ets.org/Media/Research/pdf/conf_achgapwomen_allspach.pdf.
- Andrews M. H. and Andrews L., (1979), First-year chemistry grades and SAT math scores, J. Chem. Educ., 56(4), 231 DOI:10.1021/ed056p231.
- Archer L., DeWitt J., Osborne J., Dillon J., Willis B. and Wong B., (2010), “Doing” science versus ‘being’ a scientist: examining 10/11-year-old schoolchildren's constructions of science through the lens of identity, Sci. Educ., 94(4), 617–639 DOI:10.1002/sce.20399.
- Baenninger M. and Newcombe N., (1989), The role of experience in spatial test performance: a meta-analysis, Sex Roles, 20(5–6), 327–344 DOI:10.1007/BF00287729.
- Bandura A., (1995), Self-efficacy in changing societies, Cambridge university press.
- Bauer C. F., (2005), Beyond “Student Attitudes”: Chemistry Self-Concept Inventory for Assessment of the Affective Component of Student Learning, J. Chem. Educ., 82(12), 1864 DOI:10.1021/ed082p1864.
- Beilock S. L., Gunderson E. A., Ramirez G. and Levine S. C., (2010), Female teachers’ math anxiety affects girls’ math achievement, Proc. Natl. Acad. Sci. U. S. A., 107(5), 1860–1863, Retrieved from http://www.pnas.org/content/107/5/1860.abstract.
- Boli J., Allen M. L. and Payne A., (1985), High-Ability Women and Men in Undergraduate Mathematics and Chemistry Courses, Am. Educ. Res. J., 22(4), 605–626 DOI:10.3102/00028312022004605.
- Bong M., (1999), Personal Factors Affecting the Generality of Academic Self-Efficacy Judgments: Gender, Ethnicity, and Relative Expertise, J. Exp. Educ., 67(4), 315–331 DOI:10.1080/00220979909598486.
- Box G. E., (1979), Robustness in the strategy of scientific model building, Robustness in Statistics, 1, 201–236.
- Boz Y., Boz Y., Yerdelen-Damar S., Yerdelen-Damar S., Aydemir N., Aydemir N. and Aydemir M., (2016), Investigating the relationships among students’ self-efficacy beliefs, their perceptions of classroom learning environment, gender, and chemistry achievement through structural equation modeling, Res. Sci. Technol. Educ., 34(3), 307–324 DOI:10.1080/02635143.2016.1174931.
- Bramley T., Rodeiro C. and Vitello S., (2015), Gender differences in GCSE, Cambridge Assessment Research Report, http://www.cambridgeassessment.org.uk/Images/gender-differences-at-gcsereport.pdf.
- Brown J. D. and Dutton K. A., (1995), The thrill of victory, the complexity of defeat: self-esteem and people's emotional reactions to success and failure, J. Pers. Soc. Psychol., 68(4), 712–722, Retrieved from http://eutils.ncbi.nlm.nih.gov/entrez/eutils/elink.fcgi?dbfrom=pubmed&id=7738773&retmode=ref&cmd=prlinks.
- Burkam D. T., Lee V. E. and Smerdon B. A., (1997), Gender and Science Learning Early in High School: Subject Matter and Laboratory Experiences, Am. Educ. Res. J., 34(2), 297–331 DOI:10.3102/00028312034002297.
- Camara W. J. E. G., (2000), The SAT[R] I and High School Grades: Utility in Predicting Success in College. Research Notes, College Board, Retrieved from http://https://eric.ed.gov/?id=ED446592.
- Carlone H. B. and Johnson A., (2007), Understanding the science experiences of successful women of color: science identity as an analytic lens, J. Res. Sci. Teach., 44(8), 1187–1218 DOI:10.1002/tea.20237.
- Carter C. S. and Larussa M. A., (1987), A study of two measures of spatial ability as predictors of success in different levels of general chemistry, J. Res. Sci. Teach., 24, 645–657, Retrieved from http://onlinelibrary.wiley.com/doi/10.1002/tea.3660240705/full.
- Casey M. B., Nuttall R., Casey M. B., Pezaris E., Nuttall R., Pezaris E. and Benbow C. P., (1995), The influence of spatial ability on gender differences in mathematics college entrance test scores across diverse samples, Dev. Psychol., 31(4), 697–705 DOI:10.1037/0012-1649.31.4.697.
- Cohen J., (1992), A power primer, Psychological bulletin, 112(1), 155.
- Cole N. S., (1997), The ETS Gender Study: How Females and Males Perform in Educational Settings.
- Crisp G., Nora A. and Taggart A., (2009), Student Characteristics, Pre-College, College, and Environmental Factors as Predictors of Majoring in and Earning a STEM Degree: An Analysis of Students Attending a Hispanic Serving Institution, Am. Educ. Res. J., 46(4), 924–942 DOI:10.3102/0002831209349460.
- Dalgety J. and Coll R. K., (2006a), Exploring First-Year Science Students' Chemistry Self-Efficacy, Int. J. Sci. Math. Educ., 4(1), 97–116 DOI:10.1007/s10763-005-1080-3.
- Dalgety J. and Coll R. K., (2006b), The Influence of First-Year Chemistry Students' Learning Experiences on Their Educational Choices 1, Assessment & Evaluation in Higher Education, 31(3), 303–328 DOI:10.1080/02602930500352931.
- Darlington E. and Bowyer J., (2016), How well does A-level Mathematics prepare students for the mathematical demands of chemistry degrees? Chem. Educ. Res. Pract., 17(4), 1190–1202 10.1039/C6RP00170J.
- Denny R. T., (1971), The mathematics skill test (MAST) for chemistry, J. Chem. Educ., 48(12), 845.
- Dhindsa H. S. and Chung G., (1999), Motivation, anxiety, enjoyment and values associated with chemistry learning among form 5 Bruneian students, Presented at the MERA-ERA joint conference, Malacca, Malaysia.
- Donaghy K. J. and Saxton K. J., (2012), Connecting Geometry and Chemistry: A Three-Step Approach to Three-Dimensional Thinking, J. Chem. Educ., 89(7), 917–920 DOI:10.1021/ed200345w.
- Feng J., Spence I. and Pratt J., (2016), Playing an Action Video Game Reduces Gender Differences in Spatial Cognition, Psychol. Sci., 18(10), 850–855 DOI:10.1111/j.1467-9280.2007.01990.x.
- Ferrell B. and Barbera J., (2015), Analysis of students' self-efficacy, interest, and effort beliefs in general chemistry, Chem. Educ. Res. Pract., 16(2), 318–337 10.1039/C4RP00152D.
- Ferrell B., Phillips M. M. and Barbera J., (2016), Connecting achievement motivation to performance in general chemistry, Chem. Educ. Res. Pract., 17(4), 1054–1066 10.1039/C6RP00148C.
- Friedman L., (1989), Mathematics and the Gender Gap: A Met-Analysis of Recent Studies on Sex Differences in Mathematical Tasks, Rev. Educ. Res., 59(2), 185–213 DOI:10.3102/00346543059002185.
- Gabel D. and Sherwood R. D., (1984), Analyzing difficulties with mole-concept tasks by using familiar analog tasks, J. Res. Sci. Teach., 21(8), 843–851.
- Goldstein D., Haldane D. and Mitchell C., (1990), Sex differences in visual-spatial ability: the role of performance factors, Mem. Cognit., 18(5), 546–550 DOI:10.3758/BF03198487.pdf.
- Good C., Aronson J. and Inzlicht M., (2003), Improving adolescents' standardized test performance: an intervention to reduce the effects of stereotype threat, J. Appl. Dev. Psychol., 24(6), 645–662.
- Hayes A. F., (2012), PROCESS: A versatile computational tool for observed variable mediation, moderation, and conditional process modeling, http://www.afhayes.com.
- Hayes A. F., (2015), An index and test of linear moderated mediation, Multivariate Behav. Res., 50(1), 1–22.
- Hazari Z., Sonnert G., Sadler P. M. and Shanahan M. C., (2010), Connecting High School Physics Experiences, Outcome Expectations, Physics Identity, and Physics Career Choice: a Gender Study, J. Res. Sci. Teach., 47(8), Wiley Subscription Services, Inc., A Wiley Company, 978–1003, DOI:10.1002/tea.20363.
- Herron J. D. and Nurrenbern S. C., (1999), Chemical Education Research: Improving Chemistry Learning, J. Chem. Educ., 76(10), 1353 DOI:10.1021/ed076p1353.
- Hughes G., (2000), Marginalization of Socioscientific Material in Science-Technology-Society Science Curricula: Some Implications for Gender Inclusivity and Curriculum Reform, J. Res. Sci. Teach., 37(5), 426–440 DOI:10.1002/(SICI)1098-2736(200005)37:5<426::AID-TEA3>3.0.CO;2-U.
- Hughes G., (2001), Exploring the Availability of Student Scientist Identities within Curriculum Discourse: An anti-essentialist approach to gender-inclusive science, Gend. Educ., 13(3), 275–290 DOI:10.1080/09540250120063562.
- Jacobs J. E., (2005), Twenty-five years of research on gender and ethnic differences in math and science career choices: What have we learned? New Dir. Child. Adolesc. Dev., 2005(110), 85–94.
- Legewie J. and DiPrete T. A., (2014), The High School Environment and the Gender Gap in Science and Engineering, Sociol. Educ., 87(4), 259–280 DOI:10.1177/0038040714547770.
- Linn M. C. and Petersen A. C., (1985), Emergence and Characterization of Sex Differences in Spatial Ability: A Meta-Analysis, Child Dev., 56(6), 1479 DOI:10.2307/1130467.
- Moss-Racusin C. A., Moss-Racusin C. A., Dovidio J. F., Dovidio J. F., Brescoll V. L., Brescoll V. L., et al., (2012), Science faculty's subtle gender biases favor male students, Proc. Natl. Acad. Sci. U. S. A., 109(41), 16474–16479 DOI:10.1073/pnas.1211286109.
- Muller D., Judd C. M. and Yzerbyt V. Y., (2005), When moderation is mediated and mediation is moderated, J. Pers. Soc. Psychol., 89(6), 852.
- National Science Foundation, (2017), Women, Minorities, and Persons with Disabilities in Science and Engineering: 2017, Arlintgton, VA: NSF, Retrieved from http://www.nsf.gov/statistics/wmpd/.
- National Student Clearinghouse Research Center, (2015), Science & Engineering Degree Attainment: 2004–2014. nscresearchcenter.org, Retrieved from http://https://nscresearchcenter.org/wp-content/uploads/SnapshotReport15-DegreeAttainment.pdf.
- Oyserman D., Harrison K. and Bybee D., (2016), Can racial identity be promotive of academic efficacy? Int. J. Behav. Dev., 25(4), 379–385 DOI:10.1080/01650250042000401.
- Pajares F., (2002), Gender and Perceived Self-Efficacy in Self-Regulated Learning, Theory Pract., 41(2), 116–125 DOI:10.1207/s15430421tip4102_8.
- Preacher K. J. and Hayes A. F., (2004), SPSS and SAS procedures for estimating indirect effects in simple mediation models, Behavior research methods, 36(4), 717–731.
- Quinn D. M. and Spencer S. J., (2001), The Interference of Stereotype Threat With Women's Generation of Mathematical Problem-Solving Strategies, J. Soc. Issues, 57(1), 55–71 DOI:10.1111/0022-4537.00201.
- Rohde T. E., Thompson L. A., Rohde T. E. and Thompson L. A., (2007), Predicting academic achievement with cognitive ability, Intelligence, 35(1), 83–92 DOI:10.1016/j.intell.2006.05.004.
- Spencer S. J., Steele C. M. and Quinn D. M., (1999), Stereotype Threat and Women's Math Performance, J. Exp. Soc. Psychol., 35(1), 4–28 DOI:10.1006/jesp.1998.1373.
- Stieff M., Ryu M., Dixon B. and Hegarty M., (2012), The Role of Spatial Ability and Strategy Preference for Spatial Problem Solving in Organic Chemistry, J. Chem. Educ., 89(7), 854–859 DOI:10.1021/ed200071d.
- Tavakol M. and Dennick R., (2011), Making sense of Cronbach's alpha, Int. J. Med. Educ., 2, 53.
- Tenaw Y. A., (2013), Relationship between self-efficacy, academic achievement and gender in analytical chemistry at Debre Markos College of Teacher Education, Afr. J. Chem. Educ., 3(1), 3–28, Retrieved from http://https://www.ajol.info/index.php/ajce/article/view/84850.
- Vincent-Ruz P. and Schunn C. D., (2017), The increasingly important role of science competency beliefs for science learning in girls, J. Res. Sci. Teach., 54(6), 790–822 DOI:10.1002/tea.21387.
- Vockell E. L. and Lobonc S., (1981), Sex-role stereotyping by high school females in science, J. Res. Sci. Teach., 18(3), 209–219.
- Wai J., Lubinski D. and Benbow C. P., (2009), Spatial ability for STEM domains: aligning over 50 years of cumulative psychological knowledge solidifies its importance, J. Educ. Psychol., 101(4), 817.
- Weisman R. L., (1981), A mathematics readiness test for prospective chemistry students, J. Chem. Educ., 58(7), 564.
- Xu X., Villafane S. M. and Lewis J. E., (2013), College students’ attitudes toward chemistry, conceptual knowledge and achievement: structural equation model analysis, Chem. Educ. Res. Pract., 14(2), 188–200 10.1039/C3RP20170H.
Footnotes |
† Electronic supplementary information (ESI) available. See DOI: 10.1039/c7rp00137a |
‡ Some demographic information like parental college attending and parent income is only available from the roughly 67% of students who completed the Free Application for Federal Student Aid. |
§ SATs scores are obtained in Math, Verbal, and Writing. Each score has a maximum of 800 and scores are normed each year to produce a national mean of approximately 500. Some students only have scores from the competing ACT exam. The university converted these scores into SAT units. |
¶ Effect size emphasizes the size of the difference between two groups rather than just their statistical significance and it is independent of sample size. Under Cohen's terminology a small effect size is one in which the difference is statistically significant but the overlap between the distribution of both groups (d < 0.5) is. A large effect size is an effect which is statistically significant and where there is almost no overlap between both group's distributions (d ≥ 0.8). |
|
This journal is © The Royal Society of Chemistry 2018 |