DOI:
10.1039/C8RA07686C
(Paper)
RSC Adv., 2018,
8, 37855-37865
Using accelerated molecular dynamics simulation to shed light on the mechanism of activation/deactivation upon mutations for CCR5
Received
15th September 2018
, Accepted 6th November 2018
First published on 13th November 2018
Abstract
In this work, accelerated molecular dynamics (aMD) simulations were used to study different effects of G286F and R126 mutations on the activity of CCR5. Potential of Mean Force (PMF) results indicate that there are stable inactive-like states and active-like ones existing in the conformation space of the wild type (WT), confirming that CCR5 could possess to some extent constitutive activity. But the R126N mutation could constrain CCR5 in the inactive state through influencing the TXP motif and limiting the movements of TM5 and TM6. In contrast, the G286F mutation promotes the activity of the receptor by increasing the distance of TM2–TM6 and the flexibility of the intracellular part of TM5 and changing the H-bonding in the TXP motif. The observations from the cross correlation analysis further show that the R126N mutation dramatically reduces the motion correlations between TMs, which should partly contribute to the deactivation of CCR5. Compared with the WT system, TM6 and TM7 in the G286F mutant are loosely correlated with other regions, which should be conducive to drive the movement of TM6 and TM7 toward the active conformation. In addition, the result from the protein structure network (PSN) analysis reveals that the shortest pathways connecting the extracellular and the intracellular domains are highly conserved in the three systems despite the different mutations, in which the hydrogen bond plays a pivotal role. However, the G286F mutation shortens the lifetime of the pathway with respect to the R126N mutation, which may be associated with the different activities of the two mutants. The pathway connecting the ligand-binding site and the G-protein region reveals that the allosteric communication between TM6 and TM7 is enhanced by the R126N mutation while the G286F mutation induces the activation of the G-protein pocket by arousing more residues in the NPxxY region to participate in the pathway.
1 Introduction
Chemokines are small cytokines, which mediate cell migration during immune surveillance, inflammation and development.1 Chemokines and their receptors are divided into four families (C, CC, CXC and CX3C) in terms of the pattern of cysteine residues in the chemokines. Chemokine receptors are also members of G protein-coupled receptor (GPCR) superfamily, and are closely associated with the pathogenesis of many human diseases.2–5 C–C chemokine receptor type 5 (CCR5) is the receptor of RANTES, macrophage inflammatory protein 1 alpha (MIP-1α) and macrophage inflammatory protein 1 beta (MIP-1β), meanwhile acting as a coreceptor for macrophage tropic strains (M-tropic) of HIV-1. Naturally occurring polymorphisms of the CCR5 gene can provide resistance to the HIV-1 infection, clearly showing the importance of CCR5.6 The implication of CCR5 in cancer progression and metastasis has already been proved by many researches.4,7–9 Therefore, CCR5 is an excellent target for drug design and the treatment of related diseases.
Similar to the other A-class GPCRs, CCR5 first undergoes conformational changes from the inactive state to the active one, and then transmits signals. It was implicated that the activation of CCR5 shares some common features with the other A-class GPCRs, but still has its specific characteristics. For instance, similar to some other A-class GPCRs, CCR5 also has high conserved DRY domain in the transmembrane (TM) helix 3 (TM3).10 However, the “ionic lock”11,12 between TM3 and TM6, which could stabilize the inactive state, is not presented in CCR5. But, what similarity and difference between CCR5 and the other A-class of GPCRs haven't been well elucidated. In addition, mutation experiments of CCR5 found that replacement of the residue Gly2867.42 in TM7 with Phe could increase its constitutive activity,13 whereas changing Arg1263.50 in TM3 by Asn would result in a drop in the constitutive activity.14 But, the reason why the two mutations could drive CCR5 to different states has not been clear, which should be to large extent attributed to the absence of their structures, in particular for the active state. In 2013, the crystal structure of CCR5 was first resolved in an inactive state with an antagonist Maraviroc bound.15 There have been only two crystal structures available for CCR5 so far, which are both in the inactive state. Some experimental works already studied interactions between CCR5 and ligands, for example, the binding of TAK-779 to CCR5 and the resistance of TAK-779 to HIV-1 infection,16 the binding site of AVC to CCR5 (ref. 17) and the inhibition mechanism of two CCR5 antagonists SCH-351125 and SCH-350581.18 However, the information about the structure and function mechanism of CCR5 has been still very limited on experiments. Alternatively, computational studies, in particular for molecular dynamics simulation, have devoted great contributions to help experiments understand the issues. For example, the interactions of CCR5 with agonist and antagonist were studied using molecular dynamic (MD) simulations.19 Some potential antagonists were revealed by the molecular dynamic simulation combining virtual screening.20 The inhibition mechanism of Maraviroc to CCR5 was studied through the MD simulation.21 In addition, the interaction pattern of CCR5 with CCL5 was predicted by the MD simulation and free energy calculations.22 However, the previous MD works most focused on the interactions between CCR5 and ligands, and less concerned the activation of CCR5. As known, activation of GPCR generally occurs on millisecond timescales23 on experiments. It is difficult for conventional molecular dynamics (cMD) simulations to achieve the time scale. Accelerated molecular dynamics (aMD)24–26 simulation is capable of sampling millisecond time scale events within nanosecond time scale. By raising energy minima that are below a certain threshold level and keeping the areas above this threshold unaltered, aMD could modify the potential energy landscape without any prior knowledge about the location of either potential energy wells or barriers. The method has been successfully applied to study a number of systems including GPCRs like M2 muscarinic receptor27 and M3 receptor.28
Based on all the considerations above, we, in the work, utilized aMD coupled with potential of mean force (PMF) method to study the wild type (WT) of CCR5 and its two mutation systems (G286F and R126N) in order to capture important events in the state transition. With the aid of principal component analysis (PCA) and correlation analysis, we explored the origin of the change in the activity of CCR5 upon the two mutations. In addition, protein structure network (PSN) analysis was used to identify crucial pathways for the allosteric regulation. The observations from the work could advance our understanding of the mutation induced-changes in the activity of GPCRs.
2 Materials and methods
2.1 System preparation
X-ray crystal structure of CCR5 (PDB ID: 4MBS) was taken for this study but removing rubredoxin and ligands. The CCR5 structure is comprised of the segment Pro19 to Gln313. Following some previous calculation works of GPCRs,29–31 N-termini and C-termini were capped by the acetyl and N-methylamide groups. Missing residues in loop ICL3 were modeled using Modeller 9.16.32 Disulfide bonds in the crystal structure were retained. The wild type (WT), R126N and G286F mutants were constructed in terms of the X-ray crystal structure. Each receptor system was inserted into a POPC lipid bilayer along the Z-axis, and then solvated with TIP3P water molecules and 0.15 M NaCl. Each of the resulting systems contains about 50
000 atoms. These operations above were carried out via CHARMM-GUI.33
2.2 Molecular dynamics simulations
All-atom molecular dynamics simulations were carried out using Amber 14,34 including the conventional MD (cMD) and accelerated MD (aMD) methods. The ff14SB force-field35 was used for the receptor. The SHAKE algorithm36 was applied to constrain all hydrogen-containing bonds. The cutoff for non-bonded interactions was set to be 10 Å and the electrostatic interactions were calculated via the particle mesh Ewald (PME) method.37 To remove bad contacts, 20
000-step energy minimization was performed. Then, the system was heated from 0 K to 310 K within 250 ps and further pre-equilibrated using NVT run at 1 bar and 310 K for 5 ns. After that, a 150 ns cMD simulation was performed at 1 atm pressure and 310 K for each system in order to collect the potential statistics, through which the acceleration parameters were calculated for the subsequent aMD simulation. In addition, the final structure from the cMD simulation was served as starting structure for the aMD simulation.
Dual-boost aMD27,38 was chosen to enhance sampling conformational space. In the dual-boost aMD, a dihedral potential and a total boost potential were added to all the atoms in the systems. The dihedral and total boost acceleration parameters are defined in terms of eqn (1) and (2):
|
Edihed = Vdihed_avg + λ × Vdihed_avg, αdihed = λ × Vdihed_avg/5
| (1) |
|
Etotal = Vtotal_avg + 0.2 × Natoms, αtotal = 0.2 × Natoms
| (2) |
where
Natoms is the total number of atoms, and
Vdihed_avg and
Vtotal_avg are the average dihedral and total potential energies calculated from 150 ns cMD simulations.
λ is an adjustable acceleration parameter and
λ = 0.3 was found to work best for GPCRs.
27,39 The 500 ns aMD simulation was performed for the WT, R126N and G286F systems, respectively.
2.3 Free energy calculation
The potential of mean force (PMF) derived from the free energy landscape portrays the change of the free energy as a function of some specific reaction coordinates in a system. The TM2–TM6 distance on the cytoplasmic side of CCR5 and root-mean-square deviation (RMSD) values of the TXP domain with respect to the crystal structure in the inactive state were used as two reaction coordinates in the PMF calculation. The free energy landscape was calculated for the aMD simulation trajectory, using the following equation (eqn (3)): |
A(ζJ,ζI) = −kBT ln(ρ(ζJ,ζI))
| (3) |
where ζJ and ζI are reaction coordinates, kB is the Boltzmann constant, T is the temperature, and ρ is the probability of the protein located at bin i, j. Theoretically, the free energy landscape of the aMD simulations can be reweighted but overflow errors may arise in calculating the weights due to the large size of the system.40 In addition, large energetic noise was encountered in aMD simulations on M2 muscarinic receptor when attempting to reweight, which caused large fluctuations in the calculation of the free energy. Consequently, it was found that the unweighted PMF profiles from the aMD simulations match well to PMF profiles of cMD simulations.40 Therefore, we used the unweighted free energy landscapes in the work.
2.4 Cross correlation analysis
The cross correlation analysis could reveal how atomic displacements are coupled and provide information for the impact of mutations on the protein dynamics. We employed a linear mutual information (LMI) algorithm41,42 to calculate the cross correlations between the residues. LMI values were computed according to the following equation (eqn (4)): |
 | (4) |
where i and j are atoms or residues, Cij is the covariance matrix of i and j, LMI values vary from 0 to 1, which represent a completely uncorrelated displacement and a completely correlated one, respectively.
2.5 Principal component analysis
Principal component analysis (PCA) is able to separate large amplitude motions along aMD trajectory. The obtained trajectory data could be utilized to generate a covariance matrix between Ca atoms of i and j residues, which is defined by the following equation (eqn (5)): |
Cij = 〈(xi − 〈xi〉)(xj − 〈xj〉)〉 (i, j = 1, 2, 3,⋯,3N)
| (5) |
where xi and xj are Cartesian coordinates of the ith and jth Ca atoms, respectively, N is the number of the Ca atoms considered, and 〈xi〉 and 〈xj〉 represent the time average over all the configurations obtained from the molecular dynamics simulation. In this approach, a set of eigenvectors and corresponding eigenvalues was obtained by diagonalizing the covariance matrix of atomic fluctuations with respect to the average structure, which show the axes of maximal variance in the protein motion.
2.6 Protein structure network
The protein structure network (PSN) analysis43 has been successfully applied to gain insight into the holistic properties of protein structures since it could provide information for intra-molecular and inter-molecular communications, which are pivotal for proteins to execute their biological functions. In PSN, the residues are taken as the nodes of the network. The edge of the two nodes is defined if the percentage of the interaction (see eqn (6)) between them is greater than or equal to a given interaction strength cutoff. |
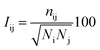 | (6) |
where Iij is the interaction percentage between nodes i and j, nij presents the pair number of side-chain atoms within a given distance cutoff. Ni and Nj denote the normalization factors for residues i and j, respectively.
The percentage of interaction of a hub node is calculated in terms of eqn (7):
|
 | (7) |
where
Ii is the hub interaction percentage of node i,
nij is the number of side-chain atom pairs within a given distance cutoff and
Ni is the normalization factor of residue i. The shortest non-covalently connected pathways between residues in trajectories were identified by combining PSN node inter-connectivities and residue correlated motions.
Cross correlation analysis and PSN as well as PCA were performed using Wordom44 software. All images visualizing the receptor were produced by Pymol.45 The other MD analyses were carried out using the Ptraj module of Amber 14.
3 Results and discussions
3.1 Stable states in conformation space
For the WT, R126N, and G286F systems, the potential of mean force (PMF) was calculated to capture stable states in the process of conformation transitions. As accepted, the cytoplasmic displacement of TM6 is an important requisite for the activation of GPCRs.46 Unfortunately, there has not been the crystal structure of CCR5 in the active state resolved so far, leading to the unknown value about the TM6 deviation from the inactive state to the active one. However, several crystal structures of class A GPCRs in the active state were available, for example US28 (PDB ID: 4XT1, 4XT3)47 and μ-OR (PDB ID: 5C1M).48 The values of TM2–TM6 distance in the active states were about 20.0 Å for US28 (PDB ID: 4XT1, 4XT3) and 24.1 Å for μ-OR (PDB ID: 5C1M), which share 28% and 58% homology with CCR5, respectively. For μ-OR, the distance is about 15.6 Å in its inactive crystal-structure (PDB ID: 4DKL).49 The comparisons clearly show that the TM2–TM6 distance could reflect the outward displacement of TM6 in the activation process, which is probably in the range of 20.0–24.1 Å in the active state. In fact, Alexander already used the TM2–TM6 distance to characterize the outward movement of TM6 in β2AR.31 Highly conserved TXP motif is consisted of threonine (Thr at the position 822.56), a variable hydrophobic residue (X at the position 832.57) and proline (Pro at the position 842.58), which is found only in the chemokine receptors and a few related peptide receptors. It was revealed that the TXP motif governs the conformation of the extracellular part of TM2, which is closely associated with the chemokine receptor activation.50–52 Thus, the distance of TM2–TM6 in the cytoplasmic side and the RMSD value of the conserved TXP domain with respect to the inactive crystal-structure were chosen as two-dimension reaction coordinates. Table 1 lists the distance of TM2–TM6 and RMSDs of the TXP motif with respect to the crystal structure of CCR5 in the inactive state.
Table 1 The distances of TM2–TM6 and the RMSD values of the TXP motif in eight stable states
States |
The distance of TM2–TM6 (Å) |
The RMSD values of the TXP motif (Å) |
State I |
18.3 |
0.35 |
State II |
22.4 |
0.35 |
State III |
21.3 |
0.85 |
State IV |
17.1 |
0.85 |
State V |
15.2 |
0.31 |
State VI |
15.3 |
0.81 |
State VII |
22.3 |
0.37 |
State VIII |
22.4 |
0.90 |
The PMF of WT exhibits four stable states, as reflected by Fig. 1. In state I, the TM2–TM6 distance is 18.3 Å and the RMSD value of TXP is 0.35 Å while they are 22.4 Å and 0.35 Å in state II, respectively. The TM2–TM6 distance and the RMSD value are 21.3 Å and 0.85 Å in state III, respectively. The state IV shows 17.1 Å TM2–TM6 distance versus the 0.85 Å RMSD value. Judged from the criterion of TM2–TM6 distance, the states II and III are already in the range of the active state. But the TXP domain of state II is closer to the inactive state than the state III, judged from its smaller RMSD value. The TM2–TM6 distances of the state I and the state IV are close to the inactive state. The TXP domain of the state I is closer to the inactive state than the state IV, as reflected by the smaller RMSD value in the state I. The distance of TM2–TM6 of the four states varies from 17.1 Å to 22.4 Å, suggesting that the WT type of CCR5 could adopt diverse conformations and achieve to some extent constitutive activity.10 The constitutive activity was also observed for some other A-class GPCRs.53 Fig. 2 shows the overlap of the representative conformations from the inactive-like state (state I), the active-like state (state III) and the crystal structure in the inactive state. It is clear that TM6 of the state III moves outward with respect to the inactive crystal-structure while TM5 moves inward, presenting typical activation-features found from some class A GPCRs.54,55 In contrast, the state I shows a slightly outward displacement for TM5 and TM6 with respect to the inactive crystal-structure, thus close to the inactive conformation rather than the active one.
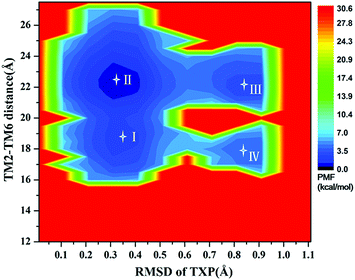 |
| Fig. 1 Free energy landscape of WT. Four stable energy wells are shown: state I to IV. | |
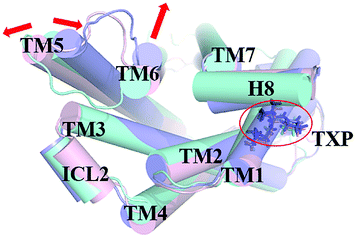 |
| Fig. 2 Structural comparisons of CCR5 crystal structure (blue) and representative conformations of state I (pink) and state III (purple) for the WT system, which are viewed from the intracellular side. The proteins are shown in cartoon, in which the TXP motif is highlighted in stick. The arrow represents the direction of the TM movement. | |
The PMF of R126N exhibits two stable states (Fig. 3): state V and state VI. The TM2–TM6 distance of the two states are about 15.3 Å (vide Table 1), close to the inactive crystal-structure. The TXP domain of the state V is closer to the inactive state than the state VI, judged from the RMSD values. The two stable states of R126N do not present the active features like the large outward movement of TM6 and small inward movement of TM5, as evidenced by Fig. 4. For the G286F system, the PMF result (vide Fig. 5) exhibits two stable states (state VII with 22.3 Å distance versus 0.37 Å RMSD value, and state VIII with 22.4 Å distance versus 0.90 Å RMSD value). It can be seen from Fig. 6 that the two states of the G286F mutant all present active features involved in TM5 and TM6 so that the TM2–TM6 distance is increased to be larger than 22 Å, suggesting that the mutation promotes the receptor to the active state.
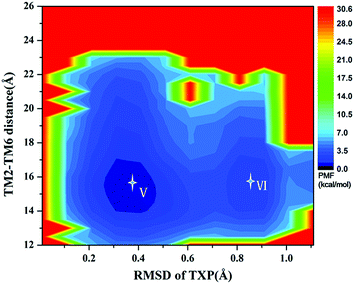 |
| Fig. 3 Free energy landscape of R126N. Two stable energy wells are shown: state V and state VI. | |
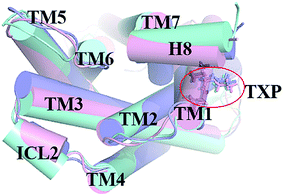 |
| Fig. 4 Structural comparisons of CCR5 crystal structure (blue) and representative conformations of state V (pink) and state VI (purple) for the R126N system, which are viewed from the intracellular side. The proteins are shown in cartoon, in which the TXP motif is highlighted in stick. The arrow represents the direction of the TM movement. | |
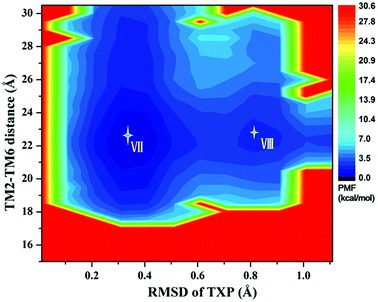 |
| Fig. 5 Free energy landscape of G286F. Two stable energy wells are shown: state VII and state VIII. | |
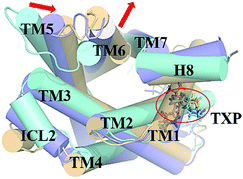 |
| Fig. 6 Structural comparisons of CCR5 crystal structure (blue) and representative conformations of state VII (purple) and state VIII (yellow) for the G286F system, which are viewed from the intracellular side. The proteins are shown in cartoon, in which the TXP motif is highlighted in stick. The arrow represents the direction of the TM movement. | |
The TXP motif is consisted of threonine-x-proline, which is found only in the chemokine receptors and a few related peptide receptors. The region was revealed to be important in the chemokine-induced activation.56 In order to observe the structural change of the TXP motif, we monitored its RMSD value (vide Fig. 7). In addition, Fig. 2, 4 and 6 also show their conformations. It can be seen from Fig. 7 that G286F has more conformations located in the region with high RMSD values than the other systems, presenting relatively significant difference from the inactive state. Fig. 6 also shows the change trend. In order to gain insight into the conformation change of TXP, we further analyzed interactions between the TXP residues, as depicted in Table 2. It can be observed that Thr822.56 and Trp862.60 form a stable hydrogen bond in all the three systems. R126N has the highest lifetime for the hydrogen bond. As revealed, Trp862.60 is one residue located in the ligand binding site of CCR5. The stable H-bonding of Thr822.56–Trp862.60 indicates that one correlation exists between the TXP motif and the ligand binding pocket. Similar to the H-bond of Thr822.56–Trp862.60, the H-bonding between Val832.57 and Ala872.61 also exists in all the three systems and presents higher stability. The two steady hydrogen bonds (Val832.57–Ala872.61 and Thr822.56–Trp862.60) should stabilize the conformation of the TXP motif and restrain its structure change, for example, small RMSDs observed above. It can be seen from Table 2, the mutation of R126N enhances the two H-bonds with respect to the WT system, which would restrain the movement of the TXP motif to the active conformation. H-bond between Pro842.58 and His882.62 is presented in both the R126N and WT systems, but disappears in the G286F system. Alternatively, the H-bond of Thr822.56–Phe792.53 is formed for the G286F mutant. The significant change in the H-bonding in the TXP motif upon the G286F mutation may be associated with the activation of the receptor.
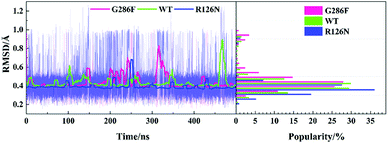 |
| Fig. 7 Changes in the RMSD values of the TXP motif along with the 500 ns aMD simulation time (left) and distributions of the RMSD values (right) at the interval of 0.05 Å from the smallest RMSD value to the maximum one. The RMSD values were calculated with respect to the initial structure. | |
Table 2 H-bonds in the TXP motif and their lifetimes characterized by percentages
H-bonds |
R126N |
WT |
G286F |
Thr822.56–Trp862.60 |
42% |
41% |
31% |
Thr822.56–Phe792.53 |
— |
— |
44% |
Val832.57–Ala872.61 |
68% |
52% |
59% |
Pro842.58–His882.62 |
38% |
34% |
— |
The DRY motif, which is consisted of aspartic acid, arginine and tyrosine, is located at the boundary between TM3 and ICL2 of class A GPCRs. The highly conserved stretch plays a crucial role in regulating the GPCR conformation state.57,58 It was found from many reported class A GPCR structures (including all chemokine receptors) that Asp3.49 in the DRY motif engages in a polar interaction with an arginine residue in the ICL2,47 which contributes to stabilize the inactive state.59 In contrast, the active crystal-structures of US28 (ref. 47) do not present the ionic interaction. For CCR5, its ICL2 has only one arginine residue at the position of 140 (e.g., Arg140ICL2). Thus, we used the RIN method to analyze the interaction between Asp1253.49 in TM3 and Arg140ICL2 in ICL2 for all the stable states obtained from the PMF analysis. Since the ionic interaction is similar between the state I and the state IV, between the state II and the state III, between the state V and the state VI, and between the state VII and the state VIII, Fig. 8 only representatively shows the ionic interaction for the states I, III, V and VIII. As shown in Fig. 8, for WT, Asp1253.49 and Arg140ICL2 form a stable ionic lock in the states I and IV while it is broken in the states II and III, further supporting that the states I and IV are close to the inactive conformations and the states II and III approach to the active ones. The two stable states (V and VI) in the R126N system both present the ionic interaction between Asp1253.49 and Arg140ICL2, which should be associated with the stabilization of the R126N mutation on the inactive state. However, the interaction disappears in the two stable states of G286F, in which Arg140ICL2 exhibits a distinct outward, as shown in Fig. 8. The observations also provide further support for the role of DRY in regulating the CCR5 conformation states.
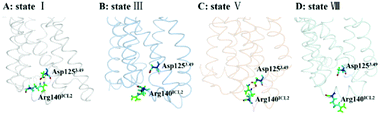 |
| Fig. 8 Conformations of Arg140ICL2 and Asp1253.49 in representative conformations from the stable states of the WT, R126N and G286F systems ((A) state I in WT, (B) state III in WT, (C) state V in R126N, (D) state VIII in G286F). The proteins are shown in ribbon, in which Arg140ICL2 and Asp1253.49 are highlighted in stick. | |
3.2 Principal component analysis of the dynamics behavior
To gain insight into effects of the two mutations on the main dynamical behavior, we performed principle component analysis (PCA) for the Ca atoms of residues. PCA could characterize the important dynamics features by reducing the dimensionality of the trajectory data. The PCA results show that the eigenvalue contribution of the first four components could capture about 60–70% of all the motions for the three systems, representing main contributions. Thus, Fig. 9 shows the projection of the first four eigenvectors on the subspace defined by the coordinates of the atoms. Red to blue colors correspond to movements from large to small. As can be seen from Fig. 9, compared to the other two systems, there is no region presenting significant movement for R126N. Whereas for the WT system, the intracellular part of TM5 and ICL3 connecting TM5 and TM6 present a noticeable contribution to the receptor movement. For G286F, TM5 exhibits significant movement, as shown in Fig. 9. These observations also show the role of the cytoplasmic part of TM5 in influencing CCR5 activation. Besides TM5, ICL2 also considerably contributes to the overall dynamics for G286F. It was reported that ICL2 takes part in the interactions of GPCR with the G protein, arrestins and other downstream effectors.60,61 Thus, we calculated the RMSD value of ICL2, as depicted by Fig. 10. It can be seen that the fluctuation of ICL2 in G286F is significant, which should favor the receptor to adopt active conformations. However, ICL2 in R126N does not present so obvious changes as G286F and WT. In addition, we also calculated RMSD values of ICL3 (vide Fig. 11) to monitor its structural change because ICL3 was considered to control the selectivity of GPCRs to different downstream signaling proteins and functional regulations.62,63 Although the RMSD value of ICL3 only presents slightly different between the three systems, R126N still shows more distributions at the region of low RMSD values, as shown by Fig. 11, while there are more conformations presenting high RMSD values in the G286F system than the other two systems. Taken together, it may be assumed that the G286F mutation induces the receptor to adopt active conformations through increasing the cytoplasmic flexibility of TM5 and the fluctuations of ICL2 and ICL3.
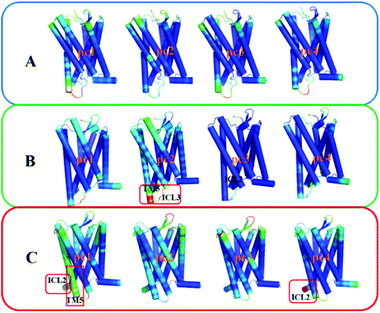 |
| Fig. 9 The projection of the first four eigenvectors colored by the length of the atomic component of the eigenvectors, representing the mobility (red: large; green: intermediate; blue: small). (A) R126N, (B) WT, (C) G286F. The receptor is oriented in the membrane along the Z-axis. | |
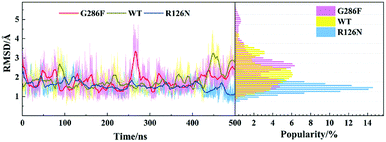 |
| Fig. 10 Changes in RMSD values of ICL2 along with the 500 ns aMD simulation time (left) and distributions of the RMSD values (right) at the interval of 0.1 Å from the smallest RMSD value to the maximum one. The RMSD values were calculated with respect to the initial structure. | |
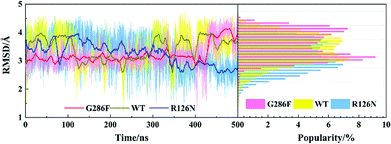 |
| Fig. 11 Changes in RMSD values of ICL3 along with the 500 ns aMD simulation time (left) and distributions of the RMSD values (right) at the interval of 0.1 Å from the smallest RMSD value to the maximum one. The RMSD values were calculated with respect to the initial structure. | |
3.3 Analysis of correlated motions
The movement of disparate regions is not independent for the conformation change of the receptor. To gain insight into the correlation between the regions, we calculated the residue cross-correlations for the R126N, WT and G286F systems, as depicted in Fig. 12 and Table 3. It can be seen that there are many highly related regions with cross-correlation values > 0.6 in the WT system, for example, TM6–TM3, TM7–TM3 and TM7–TM5. In addition, ECL2 also exhibits strong correlation with TM3 due to the disulfide bond formed by Cys178ECL2 and Cys1013.25. Whereas for the R126N system, there is nearly no high correlation regions, as evidenced by Fig. 12. Miao used molecular simulation to study activation of the M2 muscarinic receptor and indicated that the inactive receptor bound by the antagonist QNB presents poor correlation with nearly all cross-correlation values < 0.6. In contrast, the apo receptor exhibit significantly higher correlations and transitions between the inactive, intermediate and active conformation states.27 These observations are in line with our R126N and WT systems. In addition, we also calculated the H-bonding for the three systems under study, based on their 500 ns aMD trajectories. It was found that the number of the H-bonds with frequencies higher than 50% are in the order of R126N (70) < G286F (81) < WT (93), which is consistent with the change trend of the correlation. It indicates that the H-bonding play a role in the correlation. Taken together, it may be assumed that the reduced correlations upon the R126N mutation should be conducive to stabilize the receptor in the inactive state while the high correlation in WT would facilitate the receptor to conduct synergic movements of these functional regions to achieve the multiple states (the inactive-like state and active-like one), as observed above. Compared to WT, the number of the highly related regions are significantly declined upon the G286F mutation but still presents a few highly correlated regions. For example, the highly correlations between TM6, TM7 and some helixes (e.g., TM6–TM7, TM3–TM6, TM3–TM7 and TM5–TM7) existing in WT disappears upon the G286F mutation, which would lessen the restriction on the movement of TM6 and TM7, in turn facilitating the increase in the distance of TM2–TM6.
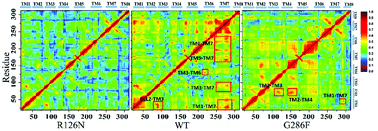 |
| Fig. 12 Correlated motions between residues shown by a dynamic map of color-coded residue cross-correlations for the three systems. | |
Table 3 Regions involved in highly correlated residue motions with the value of correlations > 0.6
Systems |
Regions |
WT |
TM2–TM6 |
TM5–TM7 |
TM3–TM7 |
TM3–TM6 |
TM6–TM7 |
ECL2–TM3 |
G286F |
TM2–TM3 |
TM2–TM4 |
TM1–TM7 |
3.4 Structural communication pathways
To gain insight into the impact of the two mutations on the allosteric communication pathways,55 we utilized the PSN analysis tool to search for the shortest pathway from extracellular (EC) domains to intracellular (IC) ones for the three systems. We selected not only all the extracellular residues, but also some residues located in the extracellular side of TMs and closest to ECLs as the starting point. Similarly, all the intracellular residues and some residues in the cytoplasmic side of TMs closest to ICLs were served as the ending point. These residues of TMs closest to ECLs and ICLs were reported to also belong to the extracellular and the intercellular domains in some other class A GPCRs.64–67 Unexpectedly, the pathways connecting the extracellular (EC) domains and the intracellular (IC) ones with the highest frequency are same for the R126N, WT, and G286F systems, as shown in Fig. 13. This pathway is consisted of Tyr892.63, Trp862.60, Thr822.56, Leu1073.31, Phe782.52, Trp1534.50, Phe1183.42, Tyr682.42 and Met642.38, involving in three transmembrane helices (e.g., TM2, TM3, and TM4). Both Tyr892.63 and Trp862.60 are key residues for CCR5 to bind Maraviroc.15 Thr822.56 is one residue in the TXP conserved motif, which was reported to play an important role in controlling the conformation change of the extracellular part of TM2 and to be associated with the CCR5 activation.52 The participation of Thr822.56 in the allosteric pathway from EC to IC indicates the role of TXP in the allosteric pathway associated with the activation of CCR5. The structural communication is transferred to TM3 via Leu1073.31. The analysis of hydrogen bonds shows that Leu1073.31 forms two pairs of stable H-bonds with Gly1113.35 and Leu1033.27, respectively (vide Table 4) and the two H-bonds exist in all the three systems with relatively long lifetime. The two pairs of H-bonds involved in Leu1073.31 connect the extracellular part of TM3 and the interior of TM3, and simultaneously link TM2 and TM3. Consequently, it can be inferred that Leu1073.31 is crucial to executive the important function of TM3. Phe782.52 forms H-bond with Ile742.48 in all the three systems with lifetime over 60%, indicating that the shortest pathway could influence the conformation of the receptor through the H-bonds between Phe782.52 and the other residues. Then, the structural signal transmits to TM4 through the highly conserved residue Trp1534.50. Studies on some A-class GPCRs revealed that Trp1534.50 interacts with TM3 by means of non-covalent interactions either for inactive or active structures.68 The appearance of Trp1534.50 in the pathway further confirms its importance in stabilizing the receptor. The pathway goes down from Trp1534.50 to Phe1183.42 in TM3 while Phe1183.42 interacts with Ser1143.38 and Leu1223.46 through hydrogen bonds. Studies on some other A-class GPCRs also showed that residues at 3.38 position make conserved connections with 4.50 position through no-covalent interactions to communicate TM3 and TM4.54 In addition, the no-covalent interaction between residues at 3.46 and 2.42 positions connecting TM2 and TM3 is highly conserved in various A-class GPCRs.68 The structural signal passes from Phe1183.42 to Tyr682.42 with the aid of these H-bond interactions, and finally come to Met642.38. Furthermore, there is also H-bonding between Tyr682.42 and Met642.38 (vide Table 4). The observations indicate the important role of the hydrogen bonds in the signaling pathway from the extracellular domains to the intracellular ones for CCR5. In spite of the same composition of this pathway for the three systems, their frequencies are different, which are 47% for R126N, 46% for WT and 39% for G286F. It should be reasonable to assume that the mutations could alter the stability of this pathway, in turn changing the conformation state of the receptor.
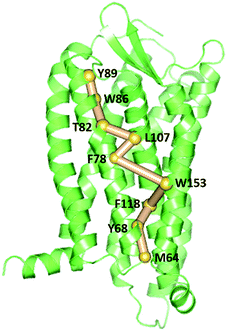 |
| Fig. 13 The pathways with the highest frequency communicating the extracellular (EC) domains and the intracellular (IC) ones for the three systems. The receptor is oriented in the membrane along the Z-axis. | |
Table 4 H-bonds involved in residues of the EC-IC pathway and their lifetimes characterized by percentages
H-bonds |
R126N |
WT |
G286F |
Leu1073.31–Gly1113.35 |
53% |
62% |
73% |
Leu1073.31–Leu1033.27 |
52% |
69% |
73% |
Phe782.52–Ile742.48 |
61% |
78% |
61% |
Phe1183.42–Ser1143.38 |
79% |
69% |
58% |
Phe1183.42–Leu1223.46 |
71% |
62% |
43% |
Tyr682.42–Met642.38 |
68% |
69% |
63% |
Since the GPCR activation is generally initiated by various agonists, we further analyzed the propagation of the structural signal from the ligand binding site to the G-protein-coupling pocket. Fig. 14 shows the pathway with the highest frequency in the three systems. The pathway in R126N is consisted of Glu2837.39, Tyr2516.51, Trp2486.48, His2897.45, Asn2937.49, Ile2406.40, Tyr2977.53 and Leu2366.36. Among the eight residues, Glu2837.39, Tyr2516.51 and Trp2486.48 were demonstrated to be key residues in binding ligands including Maraviroc for CCR5.15,69,70 Topologically equivalent residues at position 6.48 take part in interactions with various ligands and act as ‘transmission switch’ for the A-class GPCRs.68,71 The appearance of Trp2486.48 in the pathways of the three systems further shows its importance for the function of CCR5. It is found that the interaction between residues at positions 6.51 and 6.48 is maintained in most A-class GPCR structures.63 The interaction between Tyr2516.51 and Trp2486.48 appears in the R126N and G286F systems, indicating its high conservation. Compared the pathway between the three systems, it was found that the connection of Trp2486.48–His2897.45–Asn2937.49 is also highly conserved. The interaction between the residue at position 7.45 and one at 6.47 position regulates the relative location of TM6 and TM7.68 In addition, Asn2937.49 is one residue of the NPxxY domain and was considered to associate with the activation of CCR5.72,73 Hence, it can be assumed that the ‘transmission switch’ residue and the NPxxY domain are important for the signaling from the ligand binding site to the G protein pocket. In the R126N and WT systems, the pathway transmits from Asn2937.49 to Ile2406.40 at TM6. However, G286F presents the transmission from Asn2937.49 to Tyr2977.53 in the NPxxY domain, rather than Ile2406.40. In R126N, the pathway goes back to TM7 via Tyr2977.53 and eventually ends at Leu2366.36 in TM6. In WT, the pathway comes to Leu2366.36 directly from Ile2406.40. In G286F, it goes to the residue Leu2366.36 in the G protein pocket through the NPxxY domain. These observations indicate that TM6 tightly connects with TM7 in the R126N system, thus constraining the outward movement of TM6 and disfavoring the G protein pocket opening, finally restricting the activation of CCR5. Whereas the G286F mutation arouses more residues in the NPxxY domain to participate in the allosteric regulation, thus favoring its movement to the active state.
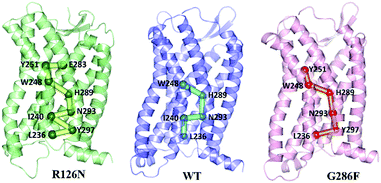 |
| Fig. 14 The communication pathways with the highest frequency from the ligand binding site to the G-protein coupling pocket. The receptor is oriented in the membrane along the Z-axis. | |
4 Conclusions
In this work, we used accelerated molecular dynamics (aMD) simulations to study the effect of the R126N and G286F mutations on the structure, the dynamics behavior and the allosteric pathway for CCR5. Our results indicate that the wild type of CCR5 could adopt multiple conformations like the inactive-like and the active-like states, providing a further support for the existence of the constitutive activity even if there is no any external stimulus to GPCRs. The R126N mutation restricts the receptor in the inactive state by stabilizing the intracellular part of TM5, restricting the outward displacement of TM2 and TM6, and enhancing the stability of TXP motif. In contrast, the G286F mutation could stabilize the receptor in the active state through enhancing the flexibility of the intracellular part of TM5 and increasing the distance between TM2 and TM6. In addition, the G286F mutation changes the H-bond interactions in the TXP motif and moves the motif to the active conformation. The results from the correlation analysis indicates that the correlations between some functional regions are important for the change in the conformation transitions. The absence of the high correlations in the R126N system would disfavor its synergic movement to the multiple states including the active state. Although the G286F mutation also decreases the correlations compared to the WT system, there are still a few high correlations existing in some regions. In addition, G286F reduces connections between TM6/TM7 and the other parts, in turn weakening the restriction on the movements of TM6 and TM7, accordingly facilitating their shifts to the active conformations. With the aid of the protein structure network method, it is revealed that the shortest pathways connecting the extracellular domains and the intracellular ones are highly conserved in the three systems despite the different mutations, in which the hydrogen bonds play a pivotal role. However, the mutations could alter the frequency of this pathway, which should contribute to the difference in the activity change between the three systems. The analysis of the pathway communicating the ligand binding site and the G protein pocket suggests that the R126N mutation reinforces the structural connections between TM6 and TM7, which could constrain the receptor in the inactive conformations while the G286F mutation induces more residues of NPxxY to attend in the pathway, in turn promoting the activation of the G protein binding site. In addition, the results from the PSN-pathway indicate that no matter what state the receptor takes, the ‘transmission switch’ residue (Trp2486.48) is a requisite for the regulation of conformations. In summary, the observations from the work could provide molecular information for elucidating the mechanism of the mutation-caused activation and deactivation for CCR5, thus helping us better understanding of the structure and function of GPCRs at the molecular level.
Conflicts of interest
There are no conflicts to declare.
Acknowledgements
This project is supported by the National Science Foundation of China (Grant No. 21573151, 21575094, 2170511) and NSAF (Grand No. U1730127).
References
- G. Lazennec and A. Richmond, Trends Mol. Med., 2010, 16, 133–144 CrossRef CAS PubMed.
- P. Allavena, G. Germano, F. Marchesi and A. Mantovaniab, Exp. Cell Res., 2011, 317, 664–673 CrossRef CAS PubMed.
- J. A. Burger, Semin. Cancer Biol., 2010, 20, 424–430 CrossRef CAS PubMed.
- A. Mantovani, B. Savino, M. Locati, L. Zammataro, P. Allavena and R. Bonecchi, Cytokine Growth Factor Rev., 2010, 21, 27–39 CrossRef CAS PubMed.
- P. Schröttner, M. Leick and M. Burger, Ann. Hematol., 2010, 89, 437–446 CrossRef PubMed.
- R. Liu, W. A. Paxton, S. Choe, D. Ceradini, S. R. Martin, R. Horuk, M. E. Macdonald, H. Stuhlmann, R. A. Koup and N. R. Landau, Cell, 1996, 86, 367–377 CrossRef CAS PubMed.
- S. Ali and G. Lazennec, Cancer Metastasis Rev., 2007, 26, 401–420 CrossRef CAS PubMed.
- H. Kulbe, T. Hagemann, P. W. Szlosarek, F. R. Balkwill and J. L. Wilson, Cancer Res., 2005, 65, 10355–10362 CrossRef CAS PubMed.
- D. Vindrieux, P. Escobar and G. Lazennec, Endocr.-Relat. Cancer, 2009, 16, 663–673 CAS.
- C. A. Flanagan, Chapter Eight – Receptor Conformation and Constitutive Activity in CCR5 Chemokine Receptor Function and HIV Infection, Elsevier Inc., 2014, vol. 70, pp. 215–263 Search PubMed.
- E. Y. Chien, W. Liu, Q. Zhao, V. Katritch, G. W. Han, M. A. Hanson, L. Shi, A. H. Newman, J. A. Javitch and V. Cherezov, Science, 2010, 330, 1091–1095 CrossRef CAS PubMed.
- K. Palczewski, T. Kumasaka, T. Hori, C. A. Behnke, H. Motoshima, B. A. Fox, I. L. Trong, D. C. Teller, T. Okada and R. E. Stenkamp, Science, 2000, 289, 739–745 CrossRef CAS PubMed.
- A. Steen, S. Thiele, D. Guo, L. S. Hansen, T. M. Frimurer and M. M. Rosenkilde, J. Biol. Chem., 2013, 288, 12511–12521 CrossRef CAS PubMed.
- B. Lagane, S. Ballet, T. Planchenault, K. Balabanian, P. E. Le, C. Blanpain, Y. Percherancier, I. Staropoli, G. Vassart and M. Oppermann, Mol. Pharmacol., 2005, 67, 1966–1976 CrossRef CAS PubMed.
- Q. Tan, Y. Zhu, J. Li, Z. Chen, G. W. Han, I. Kufareva, T. Li, L. Ma, G. Fenalti and J. Li, Science, 2013, 341, 1387–1390 CrossRef CAS PubMed.
- T. Dragic, A. Trkola, D. A. D. Thompson, E. G. Cormier, F. A. Kajumo, E. Maxwell, S. W. Lin, W. Ying, S. O. Smith and T. P. Sakmar, Proc. Natl. Acad. Sci. U. S. A., 2000, 97, 5639–5644 CrossRef CAS PubMed.
- K. Maeda, D. Das, H. Ogata-Aoki, H. Nakata, T. Miyakawa, Y. Tojo, R. Norman, Y. Takaoka, J. Ding and G. F. Arnold, J. Biol. Chem., 2006, 281, 12688–12698 CrossRef CAS PubMed.
- F. Tsamis, S. Gavrilov, F. Kajumo, C. Seibert, S. Kuhmann, T. Ketas, A. Trkola, A. Palani, J. W. Clader and J. R. Tagat, J. Virol., 2003, 77, 5201–5208 CrossRef CAS PubMed.
- A. Fano, D. W. Ritchie and A. Carrieri, J. Chem. Inf. Model., 2006, 46, 1223–1235 CrossRef CAS PubMed.
- J. Wang, M. Shu, Y. Wang, Y. Hu, Y. Wang, Y. Luo and Z. Lin, Mol. BioSyst., 2016, 12, 3396–3406 RSC.
- R. E. Salmas, M. Yurtsever and S. Durdagi, Sci. Rep., 2015, 5, 13180 CrossRef CAS PubMed.
- P. Tamamis and C. A. Floudas, Sci. Rep., 2014, 4, 5447 CrossRef CAS PubMed.
- J. P. Vilardaga, M. Bünemann, C. Krasel, M. Castro and M. J. Lohse, Nat. Biotechnol., 2003, 21, 807–812 CrossRef CAS PubMed.
- D. Hamelberg, C. A. de Oliveira and J. A. Mccammon, J. Chem. Phys., 2007, 127, 155102 CrossRef PubMed.
- D. Hamelberg, J. Chem. Phys., 2004, 120, 11919–11929 CrossRef CAS PubMed.
- P. R. Markwick and J. A. Mccammon, Phys. Chem. Chem. Phys., 2011, 13, 20053–20065 RSC.
- Y. Miao, S. E. Nichols, P. M. Gasper, V. T. Metzger and J. A. Mccammon, Proc. Natl. Acad. Sci. U. S. A., 2013, 110, 10982–10987 CrossRef CAS PubMed.
- K. Kalli, M. Yinglong and M. J Andrew, Q. Rev. Biophys., 2015, 48, 479–487 CrossRef PubMed.
- J. M. Perez-Aguilar, J. Shan, M. V. LeVine, G. Khelashvili and H. Weinstein, J. Am. Chem. Soc., 2014, 136, 16044–16054 CrossRef CAS PubMed.
- Y. Miao and J. A. McCammon, Proc. Natl. Acad. Sci. U. S. A., 2016, 113, 12162–12167 CrossRef CAS PubMed.
- A. S. Rose, M. Elgeti, U. Zachariae, H. Grubmüller, K. P. Hofmann, P. Scheerer and P. W. Hildebrand, J. Am. Chem. Soc., 2014, 136, 11244–11247 CrossRef CAS PubMed.
- N. Eswar, B. Webb, M. A. Marti-Renom, M. Madhusudhan, D. Eramian, M. y. Shen, U. Pieper and A. Sali, Curr. Protoc. Bioinf., 2006, 15, 5.6.1–5.6.30 CrossRef PubMed.
- J. Lee, X. Cheng, J. M. Swails, S. Y. Min, P. K. Eastman, J. A. Lemkul, S. Wei, J. Buckner, J. C. Jeong and Y. Qi, Biophys. J., 2016, 12, 405–413 CAS.
- D. A. Pearlman, D. A. Case, J. W. Caldwell, W. S. Ross, T. E. C. Iii, S. Debolt, D. Ferguson, G. Seibel and P. Kollman, Comput. Phys. Commun., 1995, 91, 1–41 CrossRef CAS.
- J. A. Maier, C. Martinez, K. Kasavajhala, L. Wickstrom, K. E. Hauser and C. Simmerling, J. Chem. Theory Comput., 2015, 11, 3696–3713 CrossRef CAS PubMed.
- H. J. C. Berendsen, J. P. M. Postma, W. F. V. Gunsteren, A. Dinola and J. R. Haak, J. Chem. Phys., 1984, 81, 3684–3690 CrossRef CAS.
- U. Essmann, J. Chem. Phys., 1995, 103, 8577–8593 CrossRef CAS.
- K. W. Kastner, D. A. Shoue, G. L. Estiu, J. Wolford, M. F. Fuerst, L. D. Markley, J. A. Izaguirre and M. A. Mcdowell, Malar. J., 2014, 13, 1–14 CrossRef PubMed.
- K. W. Kastner and J. A. Izaguirre, Proteins: Struct., Funct., Bioinf., 2016, 84, 1480–1489 CrossRef CAS PubMed.
- Y. Miao, S. E. Nichols and J. A. Mccammon, Phys. Chem. Chem. Phys., 2014, 16, 6398–6406 RSC.
- O. F. Lange and H. Grubmüller, Proteins: Struct., Funct., Bioinf., 2006, 62, 1053–1061 CrossRef CAS PubMed.
- H. StöGbauer, A. Kraskov, S. A. Astakhov and P. Grassberger, Phys. Rev. E: Stat., Nonlinear, Soft Matter Phys., 2004, 70, 066123 CrossRef PubMed.
- N. Kannan and S. Vishveshwara, J. Mol. Biol., 1999, 292, 441–464 CrossRef CAS PubMed.
- S. Michele, F. Angelo, R. Francesco, M. Stefanie, F. Ran, F. Rao, C. Amedeo and F. Francesca, J. Comput. Chem., 2011, 32, 1183–1194 CrossRef PubMed.
- E. Lilkova, P. Petkov, N. Ilieva and L. Litov, Gaussian, Inc, 2015, pp. 10919–10925 Search PubMed.
- A. S. Rose, M. Elgeti, U. Zachariae, H. Grubmüller, K. P. Hofmann, P. Scheerer and P. W. Hildebrand, J. Am. Chem. Soc., 2014, 136, 11244–11247 CrossRef CAS PubMed.
- J. S. Burg, J. R. Ingram, A. Venkatakrishnan, K. M. Jude, A. Dukkipati, E. N. Feinberg, A. Angelini, D. Waghray, R. O. Dror and H. L. Ploegh, Science, 2015, 347, 1113–1117 CrossRef CAS PubMed.
- W. Huang, A. Manglik, A. Venkatakrishnan, T. Laeremans, E. N. Feinberg, A. L. Sanborn, H. E. Kato, K. E. Livingston, T. S. Thorsen and R. C. Kling, Nature, 2015, 524, 315–321 CrossRef CAS PubMed.
- A. Manglik, A. C. Kruse, T. S. Kobilka, F. S. Thian, J. M. Mathiesen, R. K. Sunahara, L. Pardo, W. I. Weis, B. K. Kobilka and S. Granier, Nature, 2012, 485, 321–326 CrossRef CAS PubMed.
- C. Govaerts, C. Blanpain, X. Deupi, S. Ballet, J. A. Ballesteros, S. J. Wodak, G. Vassart, L. Pardo and M. Parmentier, J. Biol. Chem., 2001, 276, 13217–13225 CrossRef CAS PubMed.
- A. Brelot and L. A. Chakrabarti, J. Mol. Biol., 2018, 430, 2557–2589 CrossRef CAS PubMed.
- C. Govaerts, A. Bondue, J.-Y. Springael, M. Olivella, X. Deupi, E. Le Poul, S. J. Wodak, M. Parmentier, L. Pardo and C. Blanpain, J. Biol. Chem., 2002, 278, 1892–1903 CrossRef PubMed.
- R. A. Bond and A. P. IJzerman, Trends Pharmacol. Sci., 2006, 27, 92–96 CrossRef CAS PubMed.
- N. R. Latorraca, A. Venkatakrishnan and R. O. Dror, Chem. Rev., 2016, 117, 139–155 CrossRef PubMed.
- D. M. Thal, A. Glukhova, P. M. Sexton and A. Christopoulos, Nature, 2018, 559, 45–53 CrossRef CAS PubMed.
- C. Govaerts, C. Blanpain, X. Deupi, S. Ballet, J. A. Ballesteros, S. J. Wodak, G. Vassart, L. Pardo and M. Parmentier, J. Biol. Chem., 2001, 276, 13217–13225 CrossRef CAS PubMed.
- S. G. Rasmussen, H.-J. Choi, J. J. Fung, E. Pardon, P. Casarosa, P. S. Chae, B. T. DeVree, D. M. Rosenbaum, F. S. Thian and T. S. Kobilka, Nature, 2011, 469, 175–180 CrossRef CAS PubMed.
- G. E. Rovati, V. Capra and R. R. Neubig, Mol. Pharmacol., 2006, 71, 959–964 CrossRef PubMed.
- E. S. Burstein, T. A. Spalding and M. R. Brann, J. Biol. Chem., 1998, 273, 24322–24327 CrossRef CAS PubMed.
- V. Katritch, V. Cherezov and R. C. Stevens, Trends Pharmacol. Sci., 2012, 33, 17–27 CrossRef CAS PubMed.
- S. G. Rasmussen, B. T. Devree, Y. Zou, A. C. Kruse, K. Y. Chung, T. S. Kobilka, F. S. Thian, P. S. Chae, E. Pardon and D. Calinski, Nature, 2011, 477, 549–555 CrossRef CAS PubMed.
- M. M. Babu, R. W. Kriwacki and R. V. Pappu, Science, 2012, 337, 1460–1461 CrossRef CAS PubMed.
- J. Gsponer and M. M. Babu, Prog. Biophys. Mol. Biol., 2009, 99, 94–103 CrossRef CAS PubMed.
- D. T. Lodowski and K. Palczewski, Curr. Opin. HIV AIDS, 2009, 4, 88–95 CrossRef PubMed.
- K. Palczewski, T. Kumasaka, T. Hori, C. A. Behnke, H. Motoshima, B. A. Fox, I. Le Trong, D. C. Teller, T. Okada and R. E. Stenkamp, Science, 2000, 289, 739–745 CrossRef CAS PubMed.
- T. Warne, M. J. Serrano-Vega, J. G. Baker, R. Moukhametzianov, P. C. Edwards, R. Henderson, A. G. Leslie, C. G. Tate and G. F. Schertler, Nature, 2008, 454, 486–491 CrossRef CAS PubMed.
- V. Cherezov, D. M. Rosenbaum, M. A. Hanson, S. G. Rasmussen, F. S. Thian, T. S. Kobilka, H.-J. Choi, P. Kuhn, W. I. Weis and B. K. Kobilka, Science, 2007, 318, 1258–1265 CrossRef CAS PubMed.
- A. J. Venkatakrishnan, X. Deupi, G. Lebon, C. G. Tate, G. F. Schertler and M. M. Babu, Nature, 2013, 494, 185–194 CrossRef CAS PubMed.
- J. Garciaperez, P. Rueda, J. Alcami, D. Rognan, F. Arenzanaseisdedos, B. Lagane and E. Kellenberger, J. Biol. Chem., 2011, 286, 33409–33421 CrossRef CAS PubMed.
- D. J. Scholten, M. Canals, D. Maussang, L. Roumen, M. J. Smit, M. Wijtmans, C. D. Graaf, H. F. Vischer and R. Leurs, Br. J. Pharmacol., 2012, 165, 1617–1643 CrossRef CAS PubMed.
- X. Deupi and J. Standfuss, Curr. Opin. Struct. Biol., 2011, 21, 541–551 CrossRef CAS PubMed.
- O. Fritze, S. Filipek, V. Kuksa, K. Palczewski, K. P. Hofmann and O. P. Ernst, Proc. Natl. Acad. Sci. U. S. A., 2003, 100, 2290–2295 CrossRef CAS PubMed.
- P. Scheerer, J. H. Park, P. W. Hildebrand, Y. J. Kim, N. Krauss, H. W. Choe, K. P. Hofmann and O. P. Ernst, Nature, 2008, 455, 497–502 CrossRef CAS PubMed.
|
This journal is © The Royal Society of Chemistry 2018 |
Click here to see how this site uses Cookies. View our privacy policy here.