DOI:
10.1039/C8RA07604A
(Paper)
RSC Adv., 2018,
8, 38229-38237
Chemical space, diversity and activity landscape analysis of estrogen receptor binders†
Received
12th September 2018
, Accepted 5th November 2018
First published on 14th November 2018
Abstract
Understanding the structure–activity relationships (SAR) of endocrine-disrupting chemicals has a major importance in toxicology. Despite the fact that classifiers and predictive models have been developed for estrogens for the past 20 years, to the best of our knowledge, there are no studies of their activity landscape or the identification of activity cliffs. Herein, we report the first SAR of a public dataset of 121 chemicals with reported estrogen receptor binding affinities using activity landscape modeling. To this end, we conducted a systematic quantitative and visual analysis of the chemical space of the 121 chemicals. The global diversity of the dataset was characterized by means of Consensus Diversity Plot, a recently developed method. Adding pairwise activity difference information to the chemical space gave rise to the activity landscape of the data set uncovering a heterogeneous SAR, in particular for some structural classes. At least eight compounds were identified with high propensity to form activity cliffs. The findings of this work further expand the current knowledge of the underlying SAR of estrogenic compounds and can be the starting point to develop novel and potentially improved predictive models.
1. Introduction
Endocrine disrupting chemicals (EDCs) affect normal hormonal action related to the endocrine system of humans and other organisms.1,2 These chemicals can produce a vast range of adverse effects including developmental, reproductive, neurological, and immune system related effects. EDCs act through endocrine system pathways, including those related to estrogens, androgens, and thyroid hormones. Many investigations to derive robust and predictive quantitative structure–activity relationship (QSAR) models for EDCs interacting with endocrine hormone receptors, and in particular the estrogen receptor (ER), have been performed over the past 15 years.3–13 Xenoestrogens are known to have large chemical diversity including, for instance, estrogen diethylstilbestrol, polychlorinated biphenyls, alkylphenols, phthalates, and parabens, among others.14 Several structure–activity relationship (SAR) analysis and predictive models of estrogens have been developed over the past years and commented on extensively.14 However, there are no reports on the activity landscape of the EDCs.
One of the consistent manners to characterize the SAR of compound data sets is through the systematic pairwise comparison of the structure with the activity. This approach termed “activity landscape modeling”15–17 is based upon the similarity principle of chemical data sets, i.e., structurally similar compounds have similar activity values. Activity landscape modelling identifies activity cliffs i.e., pairs of compounds with high structure similarity but large potency difference.18 Depending on the scope, activity cliffs can have beneficial or detrimental consequences in many cases of study because they are major exceptions to the similarity principle. On one hand, activity cliffs challenge the development of many predictive models founded on the similarity principle. On the other hand, activity cliffs lead directly to key structural information that influence the property.19 Over the past few years, several quantitative and/or visual approaches have been published to get the profile of the activity landscape of compounds with one20 or several endpoints.21 Of note, to the best of our knowledge, these approaches have not been used to explore the property landscapes of estrogenic binding compounds despite their major importance.
Because all pairwise comparisons can lead to large amounts of structure–activity information difficult to mine and visualize, an approach called ‘activity landscape sweeping’ was developed. This is a dissection of the global activity landscape i.e., global SAR, into smaller but more structural interpretable local landscapes i.e., local SARs.22
Herein we report an activity landscape study of 121 chemicals with measured ER binding affinities. One of the main goals was to identify activity cliffs and “activity cliff generators”,23 i.e., compounds that are frequently associated with cliffs. The activity landscape sweeping approach was implemented to further understanding the activity landscape of particular groups of compounds. To this end, an analysis of the chemical space, diversity and clustering of the compounds was conducted before doing the activity landscape modeling.
2. Materials and Methods
2.1. Data sets
We focused the study on a set of 121 molecules with published values of measured binding affinities.14 This is a set of experimentally active estrogens of different structural families including steroids, DES-like, phytoestrogens, diphenylmethanes, biphenyls and phenols. The chemical structures were prepared and standardized with MOE 2016, including manual curation to avoid duplicate entries and structural errors, as well as salt removal, charges neutralization and keeping only the largest fragment if more than one molecule was present.
2.2. Molecular representations
Standard 2D chemical features were studied to characterize the chemical space. The analysis focused on molecular fingerprints (ECFP4, i.e., Extended Connectivity Fingerprints diameter 4),24 molecular scaffold (as computed using the Bemis and Murcko approach25), and six physicochemical properties (PCP) of pharmaceutical relevance, namely: octanol/water partition coefficient (Slog
P), molecular weight (MW), topological polar surface area (TPSA), number of rotatable bonds (RB), number of hydrogen bond donors and number of hydrogen bond acceptors (HBD/HBA). The molecular fingerprints, scaffolds and properties were computed with KNIME26 RDkit and CDK nodes.27
2.3. Chemical space and clustering
In order to aid the activity landscape modeling of the 121 chemicals and explore local SARs, we conducted an analysis of the chemical space. It has been previously shown that principal component analysis (PCA) and k-means clustering applied to structural similarity data using ECFP4 is a useful approach for finding and visualizing different subsets of compounds that are structurally related, for which it is feasible to find local SAR differences.22 Herein this approach was followed, and by direct inspection of the first 3 principal components (55.7% of variance) we concluded that at least four clusters could be defined. Clustering was performed with k-means on the first 7 principal components (72.7% of the variance). To further characterize these subsets, we analyzed their structural diversity through the molecular scaffolds (computed as described in Section 2.1).
2.4. Global diversity
The “global” or total diversity of the entire compound data set and each individual cluster was evaluated using Consensus Diversity Plots.28 Briefly, these are low dimensional graphs that are aimed to integrate different but complementary measures of diversity of databases. Typically, Consensus Diversity Plots represent fingerprint, scaffold, property diversity and size i.e., number of compounds in different datasets. The position of the data points in the plot, the color and size provide a quick assessment of the relative diversity of data sets. Further details of these plots and their use are elaborated elsewhere28,29 As discussed in the Results and discussion section, it would be expected that the clusters tend towards lower fingerprint-based diversity than the original data, given that they are being put together by this very criterion.
2.5. Activity landscape modeling
Activity landscape analysis was done for the data set with all the 121 compounds and for each of the clusters (4 in total) identified during the analysis of the chemical space (Section 2.3). The activity landscape analysis was performed using Structure–Activity Similarity (SAS) map which is one of the first approaches in order to perform activity landscape modeling and identify activity cliffs.30 A schematic representation of a SAS map is presented in Fig. 1. Briefly, a SAS map is a two-dimensional graph where pairwise structure and activity similarity of usually all pairwise comparisons of a data set are plotted. The structure similarity is represented on the X-axis and the activity difference (or activity similarity) is plotted on the Y-axis. In this work, the structure similarity was computed using ECFP4 fingerprints and the Tanimoto coefficient. The activity difference was computed as the absolute value of the activity difference initially expressed in relative binding affinity units (RBA), obtained by means of dividing the determined potency (IC50) by the IC50 of 17β-estradiol.14 Information from the activity landscape was contrasted with the diversity analysis, to find whether some areas of the chemical space are more susceptible to form activity cliffs. As presented in Fig. 1A, activity cliffs are identified in the top-right quadrant of the SAS map that identifies pairs of molecules with high structure similarity but large activity difference.
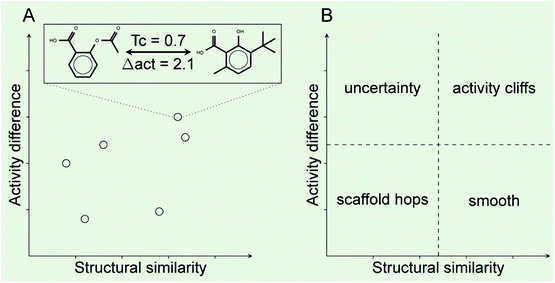 |
| Fig. 1 General form of a Structure–Activity Similarity (SAS) map. (A) Each data point represents a pair-wise comparison. Hypothetical distribution five pairs of compounds. The two example chemical structures illustrate an activity cliff: compounds with similar chemical structures but large activity difference e.g., larger than two potency units. (B) Four major regions that can be roughly identified in a SAS map. Each quadrant is labeled with the overall type of landscape. | |
2.6. Activity cliffs and generators
As mentioned in the Introduction, activity cliff generators are molecules frequently identified in the activity cliff region of the activity landscape.23 In other words, activity cliff generators are molecules that are commonly found in activity cliff pairs. In this work, compounds involved in at least five activity cliffs were selected as activity cliff generators and subject to further analysis. Direct analysis and interpretation of these activity cliffs generators is expected to yield insights into the relevant features providing estrogenic activity. All analyses were done using KNIME version 3.5.3 and its corresponding RDkit and CDK nodes.
3. Results and discussion
Results are presented and discussed in two major parts. In the first part an analysis of the chemical space diversity and content of the data set of the 121 compounds is described (Subsections 3.1 and 3.2). The second part (Subsection 3.3) addresses the activity landscape analysis that was developed based on the analysis of the chemical space.
3.1. Chemical space and clustering
Fig. 2 shows a visual representation of the chemical space of the 121 compounds using PCA based on six drug-like properties of pharmaceutical relevance. The first three principal components captured 55.7% of the variance. As described on the Methods section, the 121 compounds where further clustered into four groups based on the pairwise structure similarity computed with ECFP4 fingerprints and the Tanimoto coefficient. In Fig. 2 compounds (data points) are color-coded by the cluster number of each compound. Table 1 summarizes the number of compounds in each cluster. Overall, Fig. 2 shows a reasonable good qualitative relationship between the PCP and fingerprint-based similarity. In other words, compounds with similar PCP also have similar chemical structures as captured by the ECFP4/Tanimoto combination.
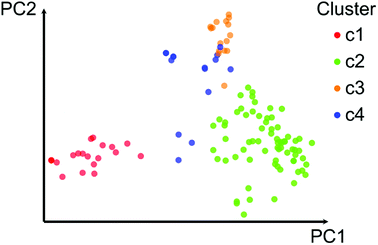 |
| Fig. 2 Visual representation of the chemical space of the data set with 121 compounds. The visual representation was generated with principal component analysis of six drug-like physicochemical properties. The first two principal components account for 43.7% of the variance. Data points are color-coded by the cluster each compound belongs based on pairwise structure similarity computed with ECFP4/Tanimoto. Clustering was performed with k-means on the first 7 principal components (72.7% of the variance). | |
Table 1 Total diversity profile of compounds in each of the four clusters (sub sets of compounds; local SAR) and for ALL compounds (global SAR)a
Cluster |
No. cpds |
Median MACCS keys/Tanimoto |
AUC |
Median PCP |
AUC: area under the curve. PCP: physicochemical properties. |
1 |
20 |
0.37 |
0.64 |
2.99 |
2 |
70 |
0.42 |
0.72 |
3.12 |
3 |
16 |
0.48 |
0.72 |
2.99 |
4 |
15 |
0.83 |
0.71 |
3.18 |
ALL |
121 |
0.40 |
0.77 |
2.75 |
In order to further interpret the type of compounds present in each cluster, the main chemical scaffolds (computed as described in the Methods section) present in each cluster were identified. Fig. 3 shows representative Bemis and Murcko scaffolds. Cluster 1 with 20 (17%) compounds is characterized by the presence of steroidal scaffolds. Cluster 2 with 70 (58%) compounds is the largest group: it contains 20 molecules that share the ubiquitous benzene scaffold, compounds related to the DES, hexestrol and tetraphenylethylene derivatives. Cluster 3 with 16 (13%) compounds contain flavones. Finally, cluster 4 has 15 (12%) compounds containing flavanones, mycoestrogens and other scaffolds. We want to emphasize that the clustering was performed based on molecular fingerprints considering the entire chemical structures.
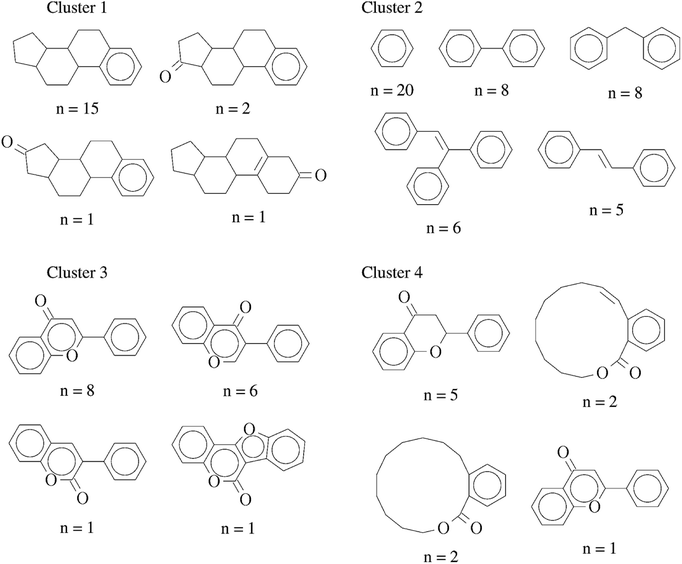 |
| Fig. 3 Representative chemical scaffolds found in each of the four clusters. The number of compounds (n) containing each cluster is indicated. | |
3.2. Global diversity
Fig. 4 shows the Consensus Diversity Plot comparing the relative global diversity of each cluster (or subset described in Section 3.1) as compared to the diversity of the entire data set. In this plot, each data point represents one compound cluster. As described in the Methods section, the fingerprint-based diversity of each cluster is represented on the X-axis, in this case measured as the median MACCS keys (166 bits) and Tanimoto similarity of the cluster. Hence, data points to the left have, in general, lower molecular similarity e.g., larger diversity. The scaffold diversity is represented on the Y-axis as measured by the area under the curve (AUC) of the scaffold recovery curve. Thus, clusters at the bottom of the plot with lower AUC values have higher scaffold diversity. Of note, as described in detail elsewhere, in a scaffold recovery curve the minimum value of AUC is 0.5 that means that a compound data set has the largest scaffold diversity: each molecule would have their own scaffold.31 The diversity based on PCP is represented with a continuous color scale from less diverse (red) to most diverse (green). Finally, the size of the data point is a relative measure of the number of compounds in each cluster e.g., smaller clusters have fewer number of molecules.
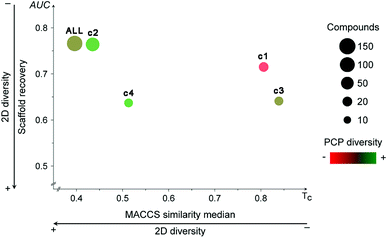 |
| Fig. 4 Consensus Diversity Plot comparing the global diversity of the four different clusters and the entire data set (ALL). Each cluster is represented with a data point. The structural diversity (X-axis) is defined as the median Tanimoto coefficient of MACCS keys fingerprints. The scaffold diversity (Y-axis) is defined as the area under the corresponding scaffold recovery curve. The diversity based on physicochemical properties (PCP) was defined as the Euclidean distance of six auto-scaled properties (Slog P, TPSA, AMW, RB, HBD, and HBA) and is shown as the filling of the data points using a continuous color scale. The relative number of compounds is represented with a different size of the data points (larger clusters are represented with larger data points). | |
Fig. 4 indicates that the entire data set (labeled as “ALL”) has a relative large fingerprint diversity but a low scaffold diversity. Cluster 2 (58% of compounds) is almost as diverse as the entire data set in terms of fingerprints and scaffolds. In contrast, cluster 4 (12% of compounds) has the relative largest combined scaffold and fingerprint diversity while cluster 1 is the least diverse with the overall lowest scaffold and fingerprint diversity. This observation is consistent with the type of molecules present in cluster 1, most of them have a steroid scaffold (a relative large scaffold that should be related to the entire diversity-vide supra). Also in contrast, compounds in cluster 2 have a small core scaffold and it would be expected that the fingerprint-diversity is influenced by the side chains. Regarding the diversity in terms of PCP, the Consensus Diversity Plot in Fig. 4 also highlights the opposite diversity of compounds in clusters 2 and 3.
3.3. Activity landscape analysis
Following the concept of activity landscape sweeping described in the Introduction and Methods, herein we analyzed the landscape for all compounds in the data set and activity landscapes for each of the four clusters. Fig. 5 shows the SAS maps for all compounds and for each of the four clusters. Thus, Fig. 5 represents the “global” and “local” activity landscapes. The SAS maps are colored coded by the density of the data points i.e., density SAS maps. Overall, most of the data points, in particular for ALL compounds and for compounds in cluster 2 are located in the lower left region of the SAS map e.g., compounds with low molecular similarity (e.g., high diversity), and low activity difference. In general, this result is consistent with the known observation that there are a large number of chemicals with diverse chemical structures but with small variations in ER binding affinity properties. Visual inspection of Fig. 5 also suggests that the activity landscape of compounds in cluster 2 resemble the landscape of the entire data set (ALL). However, a quantitative analysis would provide more insights.
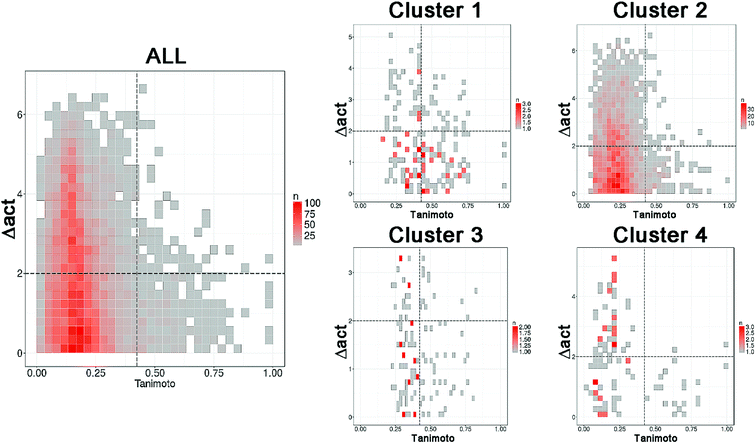 |
| Fig. 5 Density Structure–Activity Similarity (SAS) maps for the entire set (ALL, 121 compounds) i.e., global activity landscape and for each of the four individual clusters i.e., local landscapes. More red areas contain more data points. A quantitative description of the SAS maps is summarized in Table 2. | |
Table 2 summarizes a quantitative characterization of the activity landscape based on the contents of the SAS maps. A key point in the quantitative analysis of the SAS maps is setting the thresholds that define the four major quadrants of the plots i.e., the thresholds used in this study to define high/low/structural similarity (along the X-axis) and high/low activity difference. Several valid approaches have been used to define such thresholds in the SAS maps.32 Herein, we used a potency difference of two log units in potency difference along the Y-axis. This criterion has been adopted in several studies as a reasonable large potency difference. To define high/low structure similarity we used the median of the distribution of the pairwise similarity values of the 121 compounds plus two standard deviations i.e., the threshold was set to 0.424. Again, another criterion could have been used. Table 2 indicates the total number of pairwise comparisons for ALL and each of the four sets, i.e., the number of data points in the plots. Table 2 also summarize the percentage of compounds in each quadrant (major region of the SAS map as defined in Fig. 1) after setting up the thresholds.
Table 2 Quantitative analysis of the SAS maps and further analysis of the compounds in each cluster
Cluster |
Uncertaina |
Hopsa |
Cliffsa |
Smootha |
Cliffs/smoothb |
nc |
Pairsd |
Xsime |
n scaffsf |
Percentage of pairs of compounds in each of the four regions of the SAS map. Ratio of the number of pairs of compounds in the activity cliff/smooth region of the SAS map. Number of compounds in the set (n). Number of pairwise comparisons. Median similarity of the compounds in each cluster (Xsim). Number of different Bemis–Murcko scaffolds in each cluster. |
ALL |
41% |
54.5% |
1% |
3.5% |
0.286 |
121 |
7260 |
0.192 |
39 |
1 |
21% |
28% |
13% |
38% |
0.342 |
20 |
190 |
0.451 |
5 |
2 |
34% |
60% |
1.2% |
4.8% |
0.250 |
70 |
2415 |
0.239 |
21 |
3 |
17.5% |
44% |
11.5% |
27% |
0.426 |
16 |
120 |
0.417 |
4 |
4 |
44% |
37% |
1.9% |
17.1% |
0.111 |
15 |
105 |
0.267 |
9 |
The quantitative analysis indicates that compounds in clusters 1 and 3 have the largest proportion of activity cliffs (13% and 11%, respectively). This can also be seen in the SAS maps (Fig. 5) with a relative larger number of data points in the top right region of the plots. In contrast, cluster 2 has the lowest proportion of activity cliffs (1.2%), followed by cluster 4, comparable to the proportion of activity cliffs in the entire data set (1.0%). Interestingly compounds in cluster 1 (with steroid-type scaffolds) and cluster 3 (with several flavones) also have the largest proportion of data points in the smooth region of the landscape (38% and 27%, respectively). Since cluster 1 and 3 have the largest proportion of compounds in both, smooth and activity cliff regions, clusters 1 and 3 have the relative most rough or heterogeneous landscape. Table 2 also indicates that the more diverse compounds (i.e., in cluster 4) have an activity profile similar to the entire dataset (ALL).
3.3.1. Activity cliff generators and interpretation of the SAR. In this work we consider an activity generator a molecule found in at least five activity cliff pairs. Based on this criterion, eight compounds were identified as activity cliff generators. Fig. 6 shows the chemical structures of three representative cliff generators: 16beta-ol-16alfa-methyl-3-methyl-estradiol, diethylstilbestrol, and genistein. Examples of activity cliffs pairs for each activity cliff generator are illustrated.
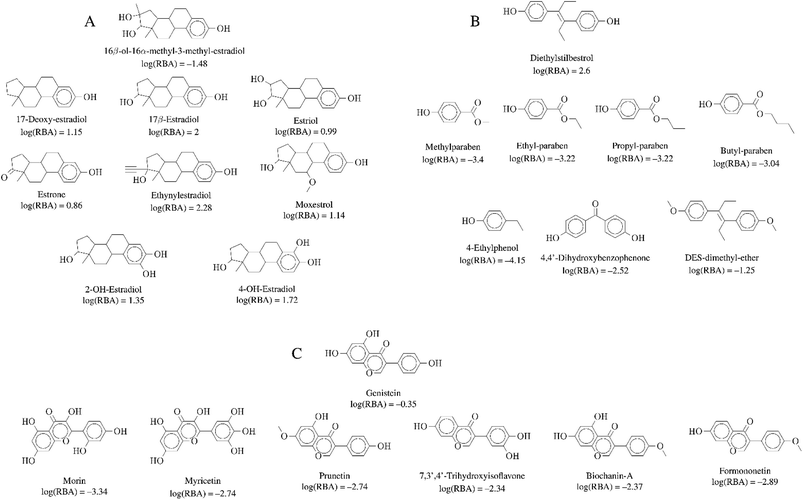 |
| Fig. 6 Representative activity cliff generators and selected pairs of compounds formed with the generators (A) 16beta-ol-16alfa-methyl-3-methyl-estradiol, (B) diethylstilbestrol and (C) genistein. The figure includes the value of the relative binding affinity (RBA) as reported by.14 | |
Activity cliffs associated with 16beta-ol-16alfa-methyl-3-methyl-estradiol (Fig. 6A) highlights the relevance and sensitivity of the hydroxyl groups around the estradiol molecule for binding. Of note, all activity cliff pairs in Fig. 6A are steroids with a phenolic ring. The cliffs in the figure points to the high relevance of both hydroxyl groups the 3- and 17beta positions of the molecule as discussed by,14 a crystallographic structure of the estrogen receptor with 17beta-estradiol indicate that the two hydroxyl groups serve as H-bond donors and acceptors at the binding site. The hydroxyl group at the 3-position is more crucial. Similarly, the activity cliffs formed with the generator diethylstilbestrol e.g., which is one of the highest-affinity synthetic estrogens (Fig. 6B), also indicates the critical role of the two symmetrical position of the hydroxyl groups of diethylstilbestrol. The distance of these two groups and rigidity of the molecule (due to the double bond) facilitates the formation of hydrophobic and hydrogen bond interactions of diethylstilbestrol. Finally, activity cliffs formed with the isoflavone genistein (Fig. 6C) further highlights the key position and distance of the two hydroxyl groups at positions 7 and 4′ around the isoflavone scaffold that mimic the 4 and 4′ hydroxyl groups of diethylstilbestrol.
The large changes in activity can be rationalized from a molecular perspective. This is illustrated in Fig. 7 for the activity cliff generator, genistein and morin (chemical structures also in Fig. 6C). Both compounds have interactions with the side chain of Glu353 through its hydroxyl group at the position 4′ of the isoflavone scaffold. In addition, both compounds have conserved pi–pi interactions with the side chain of Phe404. However, genistein makes additional key interactions between a hydroxyl group of the position 7 of the isoflavone scaffold with His524. This key interaction is not formed by morin. Similar conclusions can be reached by two- and three-dimensional representations of the protein–ligand contacts of the pairs of activity cliffs 16beta-ol-16alfa-methyl-3-methyl-estradiol and estrone (Fig. S1 in the ESI†) and diethylstilbestrol and 4-ethylphenol (Fig. S2 in the ESI†).
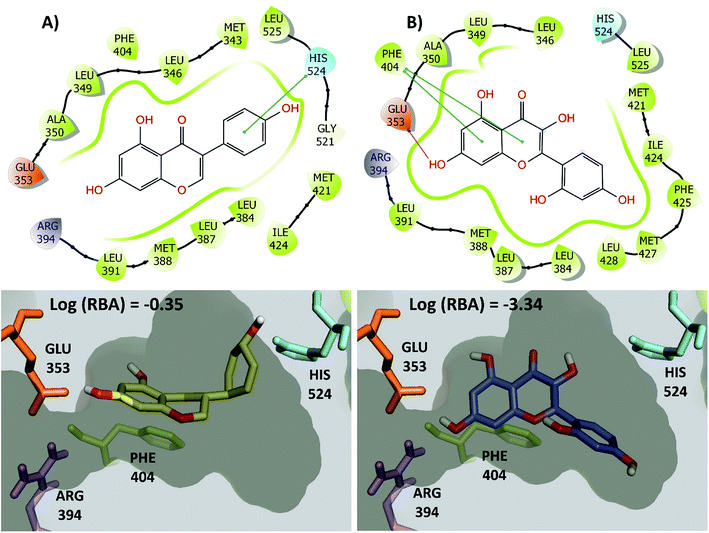 |
| Fig. 7 2D and 3D representation of representative activity cliff generators and selected pairs of compounds with greater difference in activity. (A) Genistein and (B) morin. The figure includes the value of the relative binding affinity (RBA) as reported by.14 | |
As discussed in detail elsewhere,16 the detection of activity cliffs in compound data sets can be crucial to guide the development of predictive models. Specifically, it is hypothesized that removing activity cliffs from compounds data sets would increase the performance of predictive models that are specially based on the similarity principle, for instance, classical QSAR approaches. For compound data set studied in this work, it would remain to develop and test different predictive models with and without the activity cliffs and assess quantitatively the predictive power.
4. Conclusions
Activity landscape analysis of a diverse set of 121 compounds with ER binding affinities revealed an overall heterogenous SAR with the presence of compounds with high propensity to form activity cliffs. Distinct activity cliff generators are 16beta-ol-16alfa-methyl-3-methyl-estradiol, diethylstilbestrol, and genistein, that represent major structural classes with known ER affinity, namely; a steroid, a DES-like chemical and a phytoestrogen. SAR analysis around these compounds enabled to identify specific structural features associated with a large difference in the ER binding affinities further highlighting the critical role of two hydroxyl groups for binding recognition to the binding site of the ER. Reported crystallographic structures provide a structure-based context of these cliffs. Chemical space and diversity analysis of the entire data set helped to identify four major groups of compounds, each with a distinct activity landscape e.g., local SAR. Thus, compounds with the more rigid steroid-like scaffold and molecules with a flavone-type scaffold have the most heterogeneous SAR. Global and local activity landscape regions identified in this work with a smooth SAR could be more amenable for developing predictive models. To the best of our knowledge, this is the first activity landscape analysis of compounds with ER binding affinities.
Conflicts of interest
There are no conflicts to declare.
Abbreviations
AUC | Area under the curve |
DES | Diethylstilbestrol |
ECPF4 | Extended connectivity fingerprints diameter 4 |
EDCs | Endocrine-disrupting chemicals |
HBA | Hydrogen bond acceptors |
HBD | Hydrogen bond donors |
MW | Molecular weight |
PCA | Principal component analysis |
PCP | Physicochemical properties |
RB | Number of rotatable bonds |
RBA | Relative binding affinity |
SAR | Structure–activity relationships |
SAS | Structure–activity similarity |
Slog P | Octanol/water partition coefficient |
TPSA | Topological polar surface area |
Acknowledgements
The research at Swetox (UN, DM) was supported by Knut and Alice Wallenberg Foundation [2013.0253] and Swedish Research Council FORMAS [2016-02031]. Jesus is grateful to Consejo Nacional de Ciencia y Tecnología (CONACyT, Mexico) scholarship number 622969, and DAAD programme number 57378443 for funding. Authors also acknowledge the support of the School of Chemistry of the Universidad Nacional Autónoma de México (UNAM), grant PAIP 5000-9163 and the Programa de Apoyo a Proyectos para la Innovación y Mejoramiento de la Enseñanza (PAPIME) grant PE200118, UNAM.
References
- E. Diamanti-Kandarakis, J.-P. Bourguignon, L. C. Giudice, R. Hauser, G. S. Prins, A. M. Soto, R. T. Zoeller and A. C. Gore, Endocr. Rev., 2009, 30, 293–342 CrossRef CAS PubMed.
- WHO/UNEP report, State of the Science of Endocrine Disrupting Chemicals – 2012, http://www.who.int/ceh/publications/endocrine/en/ Search PubMed.
- D. Ding, L. Xu, H. Fang, H. Hong, R. Perkins, S. Harris, E. D. Bearden, L. Shi and W. Tong, BMC Bioinf., 2010, 11, S5 CrossRef PubMed.
- G. Klopman and S. K. Chakravarti, Chemosphere, 2003, 51, 445–459 CrossRef CAS PubMed.
- H. Hong, W. Tong, Q. Xie, H. Fang and R. Perkins, SAR QSAR Environ. Res., 2005, 16, 339–347 CrossRef CAS PubMed.
- W. Tong, Q. Xie, H. Hong, L. Shi, H. Fang and R. Perkins, Environ. Health Perspect., 2004, 112, 1249–1254 CAS.
- S.-P. Korhonen, K. Tuppurainen, R. Laatikainen and M. Peräkylä, J. Chem. Inf. Model., 2005, 45, 1874–1883 CrossRef CAS PubMed.
- T. Ghafourian and M. T. D. Cronin, QSAR Comb. Sci., 2006, 25, 824–835 CrossRef CAS.
- H. Liu, E. Papa and P. Gramatica, Chem. Res. Toxicol., 2006, 19, 1540–1548 Search PubMed.
- L. Ji, X. Wang, S. Luo, L. Qin, X. Yang, S. Liu and L. Wang, Sci. China, Ser. B: Chem., 2008, 51, 677 CrossRef CAS.
- L. Ji, X. Wang, X. Yang, S. Liu and L. Wang, Chin. Sci. Bull., 2008, 53, 33–39 CrossRef CAS.
- N. Stojić, S. Erić and I. Kuzmanovski, J. Mol. Graphics Modell., 2010, 29, 450–460 CrossRef PubMed.
- L. Zhang, A. Sedykh, A. Tripathi, H. Zhu, A. Afantitis, V. D. Mouchlis, G. Melagraki, I. Rusyn and A. Tropsha, Toxicol. Appl. Pharmacol., 2013, 272, 67–76 CrossRef CAS PubMed.
- H. Fang, W. Tong, L. M. Shi, R. Blair, R. Perkins, W. Branham, B. S. Hass, Q. Xie, S. L. Dial, C. L. Moland and D. M. Sheehan, Chem. Res. Toxicol., 2001, 14, 280–294 Search PubMed.
- J. Bajorath, L. Peltason, M. Wawer, R. Guha, M. S. Lajiness and J. H. Van Drie, Drug Discovery Today, 2009, 14, 698–705 CrossRef CAS PubMed.
- L. Peltason and J. Bajorath, Chem. Biol., 2007, 14, 489–497 CrossRef CAS PubMed.
- M. Reutlinger, W. Guba, R. E. Martin, A. I. Alanine, T. Hoffmann, A. Klenner, J. A. Hiss, P. Schneider and G. Schneider, Angew. Chem., Int. Ed., 2011, 50, 11633–11636 CrossRef CAS PubMed.
- G. M. Maggiora, J. Chem. Inf. Model., 2006, 46, 1535 CrossRef CAS PubMed.
- M. Cruz-Monteagudo, J. L. Medina-Franco, Y. Pérez-Castillo, O. Nicolotti, M. N. D. S. Cordeiro and F. Borges, Drug Discovery Today, 2014, 19, 1069–1080 CrossRef CAS PubMed.
- D. Stumpfe, A. de la Vega de León, D. Dimova and J. Bajorath, F1000Research, 2014, 3, 75 Search PubMed.
- F. I. Saldívar-González, J. J. Naveja, O. Palomino-Hernández and J. L. Medina-Franco, RSC Adv., 2017, 7, 632–641 RSC.
- J. J. Naveja and J. L. Medina-Franco, RSC Adv., 2015, 5, 63882–63895 RSC.
- O. Mendez-Lucio, J. Perez-Villanueva, R. Castillo and J. L. Medina-Franco, Mol. Inf., 2012, 31, 837–846 CrossRef CAS PubMed.
- D. Rogers and M. Hahn, J. Chem. Inf. Model., 2010, 50, 742–754 CrossRef CAS PubMed.
- G. W. Bemis and M. A. Murcko, J. Med. Chem., 1996, 39, 2887–2893 CrossRef CAS PubMed.
- M. R. Berthold, N. Cebron, F. Dill, T. R. Gabriel, T. Kötter, T. Meinl, P. Ohl, C. Sieb, K. Thiel and B. Wiswedel, in Data Analysis, Machine Learning and Applications: Proceedings of the 31st Annual Conference of the Gesellschaft für Klassifikation e.V., Albert-Ludwigs-Universität Freiburg, March 7–9, 2007, ed. C. Preisach, H. Burkhardt, L. Schmidt-Thieme and R. Decker, Springer, Berlin, Heidelberg, 2008, pp. 319–326, DOI:10.1007/978-3-540-78246-9_38.
- S. Beisken, T. Meinl, B. Wiswedel, L. F. de Figueiredo, M. Berthold and C. Steinbeck, BMC Bioinf., 2013, 14, 257 CrossRef PubMed.
- M. González-Medina, F. D. Prieto-Martínez and J. L. Medina-Franco, J. Cheminf., 2016, 8, 63 Search PubMed.
- J. Naveja, M. Rico-Hidalgo and J. Medina-Franco, F1000Research, 2018, 7, 993 Search PubMed.
- V. Shanmugasundaram and G. M. Maggiora, Presented in part at the 222nd ACS National Meeting, Chicago, IL, United States, August 26–30, 2001 Search PubMed.
- J. L. Medina-Franco, K. Martínez-Mayorga, A. Bender and T. Scior, QSAR Comb. Sci., 2009, 28, 1551–1560 CrossRef CAS.
- J. L. Medina-Franco, J. Chem. Inf. Model., 2012, 52, 2485–2493 CrossRef CAS PubMed.
Footnote |
† Electronic supplementary information (ESI) available. See DOI: 10.1039/c8ra07604a |
|
This journal is © The Royal Society of Chemistry 2018 |