DOI:
10.1039/C8RA06832A
(Paper)
RSC Adv., 2018,
8, 38872-38882
Alteration of glycosylation in serum proteins: a new potential indicator to distinguish non-diabetic renal diseases from diabetic nephropathy†
Received
15th August 2018
, Accepted 13th November 2018
First published on 19th November 2018
Abstract
Diabetic nephropathy (DN) and nondiabetic renal disease (NDRD) are two major categories of renal diseases in diabetes mellitus patients. The clinical differentiation among them is usually not so clear and effective. In this study, sera from DN and NDRD patients were collected, and glycan profiles of serum proteins from DN and NDRD patients were investigated and compared by using lectin microarray and lectin blot. Then, altered glycoproteins were enriched by lectin coupled magnetic particle conjugate and characterized by LC-MS/MS. We found significant change in glycan patterns between DN and NDRD patients. In particular, the relative abundance of the glycopattern of Galβ1-3GalNAc which was identified by BPL (Bauhinia purpurea lectin) was significantly decreased in DN patients compared to four types of NDRD patients (p < 0.05). Moreover, BPL blotting indicated that the proteins with a molecular weight of about 53 kDa exhibited low staining signal in DN compared to all NDRD groups, which was consistent with results of lectin microarrays. After enriching by BPL and identification by LC-MS/MS, a total of 235 and 258 proteins were characterized from NDRD and DN respectively. Among these, the relative abundance of 12 isolated serum proteins exhibited significantly alteration between DN and NDRD (p < 0.05). Our findings indicated not only the relative abundance of Galβ1-3GalNAc on serum proteins but also certain glycoproteins modified with this glycopattern showed a difference between DN and NDRD patients. This suggested that the analysis of this alteration by using urine specimens may constitute an additional valuable diagnostic tool for differentiating DN and NDRD with a non-invasive method.
Introduction
DN is a well-known complication of diabetes and is a major cause of ESRD (end-stage renal disease) worldwide.1,2 The term “NDRD” is not a diagnosis, which includes a variety of diseases that are often grouped together in epidemiologic studies and clinical trials but that differ widely in terms of the patient's history, the clinical presentation, the risk of progression, and the response to treatment.3 IgA nephropathy (IgAN), membranous nephropathy (MN), mesangial proliferative glomerulonephritis (MPGN) and focal segmental glomerulosclerosis (FSGS) are the main types of NDRD. The treatment of DN and NDRD is quite different. Certain clinical parameters such as glycated haemoglobin (HbA1c), duration of diabetes mellitus, estimated glomerular filtration rate, urine osmotic pressure values as well as a glomerular hematuria were reported to differentiate DN and NDRD.4–6 However, the reliability of these clinical indexes has not been verified. Renal biopsy is a reliable method to differentiate NDRD from DN. Nevertheless, it reported that the positive predictive value of renal biopsy was 50.1% for diabetic nephropathy and 36.9% for NDRD. However, due to the variability of clinical courses and the frequency of confounding medical comorbidities in this population, differentiating between DN and NDRD in individual patients without the assistance of renal biopsy remains problematic and invasive.7
Glycosylation is a prevalent form of posttranslational modification of proteins. It is estimated that over half of mammalian proteins are glycosylated, especially, most of membrane proteins are glycoproteins. There are two major types of glycosylation exist on protein: N-link and O-link glycosylation. It play an important role in the folding of newly proteins and many biological processes such as cellular recognition, migration and endocytosis.8–10 In last decade, many studies focused on the alteration glycosylation in diseases such as cancer. The structures as well as biological functions of some abnormal glycans have been characterized and showed to contribute to the tumor progression.11–13 Certain specific glycans which called tumor-associated carbohydrate antigen (TACA) have been demonstrated to become enriched on cell surface following neoplastic transformation.14,15 It well known that the level of prostate-specific antigen (PSA) in serum is one of the most widely used tumor biomarkers for prostate cancer screening, however, it does not increase in some tumor cases. Recently, it revealed that the detection of abnormal glycan patterns on PSA is a more specific method for screening than PSA itself.16,17 In addition, the serological assay of sialyl Lewis a antigen (SLea) has been demonstrated to be a potential biomarker for early diagnosis of cancer such as pancreatic, colorectal and gastric cancer, moreover, it also correlated with poor prognosis and survival in patients with colon or gastric carcinoma.18,19 These findings indicated the aberrant glycosylation not only is a characteristic of tumors, but also could reflect the disease's progression.
Testa et al. reported that certain N-glycan compositions in sera, such as α(1,6)-linked arm monogalactosylated and core-fucosylated diantennary N-glycans showed significantly altered between healthy controls and T2DM patients, moreover, the level of two serum N-glycans were strongly correlated with metabolic syndrome.20 There have been several studies revealed that the aberrant glycosylation on IgA is correlated with IgAN. The aberrant glycan terminal with GalNAc or sialylated GalNAc on IgA are predominantly detected in the glomerular immune deposits and in circulating immune complexes in IgAN patients.21,22 The glycan patterns of 2,6-sialic acid and Gal exhibited significantly altered between different types of IgAN which suggested it associate with pathologic phenotypes of IgAN.23 In addition, the aberrant glycosylation of IgA1 contribute to form large Gal-deficient IgA1–IgG immune complexes and promote the progression of IgAN.24 The altered protein glycosylation could also reflect the progression of diabetic nephropathy in rat model.25 Our previous study showed that the glycan patterns of Sia-α2,6 of urinary protein exhibited significantly increased tendency with the development of diabetic nephropathy, and the glycan patterns of (GlcNAc)2-4 of urinary protein could serve as a potential indicator to differentiate the patients with DN from NDRD.26,27
In this study, we compared the glycan profile of serum glycoproteins between DN and NDRD patients by using lectin microarray. Our results revealed that the relative abundance of the glycan patterns which identified by 9 lectins (such as DBA, AAL and BPL etc.) exhibited significant differences between DN and NDRD groups. In particularly, the results of lectin microarray and lectin blotting, the glycan patterns of Galβ1-3GalNAc and terminal GalNAc showed significantly increased in all four NDRD groups compared to DN samples. Subsequently, the serum glycoproteins containing glycan pattern of Galβ1-3GalNAc from DN and NDRD patients were affinity captured by BPL and then identified by LC-MS/MS. We have identified 235 proteins in NDRD patients and 258 proteins in DN patients. Among these, the relative abundance of 8 isolated serum proteins showed significantly increased and 4 proteins exhibited significantly decreased in NDRD patients compared to DN patients. All together, our findings indicated that glycosylation of serum proteins were different between DN and NDRD patients. These alterations may provide a non-invasive alternative marker for differentiating DN and NDRD.
Results
Alterations of glycan patterns between DN and NDRD
In this study, lectin microarray was used to investigate and comparison the glycan patterns of serum from patients with DN and NDRD. The layout of lectin microarray was showed in Fig. 1A. It showed that the glycan patterns of serum glycoproteins from patients with DN was different to that from NDRD groups (Fig. 1B–F). The normalized fluorescent intensities (NFIs) for each lectin were summarized as the mean values ± SD in Table S1.† The NFIs of each lectin from DN and NDRD were compared, and the results showed that the NFIs of PHA-E and DBA were significantly higher in DN groups than all NDRD groups (fold change > 1.5, p < 0.05), which indicated the relative abundance of bisecting GlcNAc and biantennary complex-type N-glycan and α-GalNAc was higher in DN groups than all NDRD groups. The Fucα1-3/6GlcNAc binders AAL showed stronger binding signals in DN than NDRD groups but except FSGS (fold change > 2, p < 0.05). In addition, the NFIs of T antigen binders MPL (fold change > 1.5, p < 0.05), MAL-I (fold change > 2, p < 0.05) as well as LacNAc and poly LacNAc binder LEL (fold change > 2, p < 0.05) showed significantly higher in DN groups than that in MPGN and FSGS. By contrast, the NFIs of BPL exhibited significantly decreased in DN groups compared with all NDRD groups (fold change < 0.5, p < 0.05), which suggested the relative abundance the glycan patterns of Galβ1-3GalNAc and terminal GalNAc were higher in all NDRD groups than DN groups. Moreover, the relative abundance of glycan patterns of Fucα1-2Galβ1-4Glc(NAc) and α-Gal/GalNAc which identified by UEA-I and BS-I were higher in MN, IgAN and FSGS than DN groups (Fig. 1G). Further, the NFIs of 9 lectins which showed difference between DN and NDRD groups were imported EXPANDER 6.4 for hierarchical clustering analysis (HCA). According to the result of HCA, the DN and NDRD groups were separated, which indicated these glycan patterns have a potential to differentiate DN and NDRD groups (Fig. 1H).
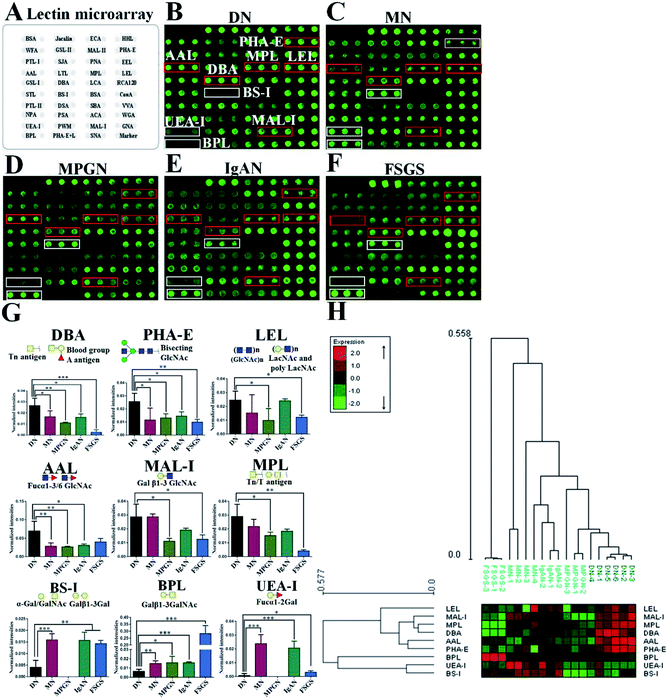 |
| Fig. 1 Comparision of glycan patterns of serum glycoproteins between DN and NDRD groups. (A) Layout of lectin microarray. The profile of Cy3-labelled serum proteins from DN (B), MN (C), MPGN (D), IgAN (E) and FSGS (F) bound to the lectin microarrays, respectively. The lectins showing significantly increased or decreased in DN compared to four NDRD groups were marked with red or white frames. (G) The NFIs of 9 lectins were significantly different in DN compared to NDRD groups (including MN, MPGN, IgAN and FSGS) reference based on fold change and One-way ANOVA (*p < 0.05, **p < 0.01, and ***p < 0.001). The data are presented as the averaged NFI ± SD. (H) Heat map and hierarchical clustering analysis of the 9 lectins exhibiting significantly difference NFIs between DN and NDRD. Glycan profiles of DN and NDRD groups (including MN, MPGN, IgAN and FSGS) were clustered (average linkage, correlation similarity). Samples are listed in columns and the lectins are listed in rows. The color and intensity of each square indicated expression levels relative to other data in the row. Red, high; green, low; black, medium. | |
Lectin and immunoblotting verification
According to the result of lectin microarrays, several glycan patterns showed a significant difference between DN and NDRD groups, such as Tn antigen and Fucα1-3/6GlcNAc. To confirm these alterations, lectin blotting was performed with AAL, DBA and BPL. The result of AAL blotting showed that there were 4 distinct protein bands (170 kDa, 100 kDa, 73 kDa and 70 kDa) presented in all groups, of which, the staining intensities of protein band about 70 kDa was increased in DN compared to all four DNRD groups (Fig. 2A). DBA blotting result revealed that the protein band about 53 kDa showed distinct binding in both DN and NDRD groups, and staining intensities of this protein band in DN sample was stronger than all NDRD groups (Fig. 2B). There were two apparent binding bands (53 kDa and 30 kDa) in both DN and NDRD groups, according to the result of BPL blotting. Among these, the proteins with molecular weight of 53 kDa exhibited stronger staining intensities than other bands.
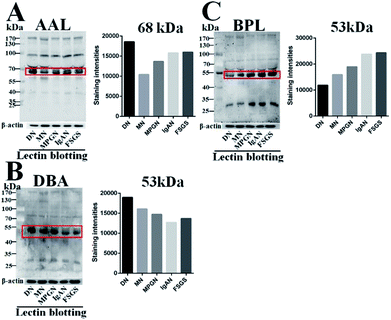 |
| Fig. 2 Binding patterns of glycoproteins in pooled sera from DN and NDRD groups for AAL, DBA and BPL. The serum proteins from DN and NDRD groups were separated by 10% SDS-PAGE and transferred in PVDF membrane. The 30 μg of Cy5 labelled AAL (A), DBA (B) and BPL (C) were incubated with membranes, and images were acquired by STROM fluorImager respectively. The gray values of the protein bands marked with red frames were measured by imageJ software. | |
Furthermore, this band marked by a red frame exhibited low staining signal in DN compared to NDRD groups, and the signal intensities also showed difference between DN and NDRD groups. In addition, the staining signal of glycoprotein band with molecular weight of 30 kDa was obviously higher in IgAN, MPGN and FSGS than that in DN and MN samples (Fig. 2C).
Characterization of protein by LC-MS/MS
According to the results of lectin microarrays and lectin blotting, the glycopattern of Galβ1-3GalNAc and terminal GalNAc and glycoproteins with molecular weight of 53 kDa which identified by BPL showed increased in DN patients compared to four NDRD groups. Therefore, the four NDRD groups were pooled equally, and lectin affinity separation and LC-MS/MS was used to isolated and characterized the proteins from DN and NDRD respectively. A total of 3508 (corresponding 258 proteins) and 3545 (corresponding 235 proteins) peptides were identified in DN and NDRD (Fig. 3A). Among these, 3140 peptides, corresponding 180 proteins were common to both, whereas 78 and 55 proteins were specially identified in DN and NDRD respectively (Fig. 3B). Among the proteins identified in both DN and NDRD, the relative abundance of 12 proteins were altered between DN and NDRD (fold change > 1.5 or < 0.67, p < 0.05) (Fig. 3C), especially, 8 proteins (such as Adenylate kinase 9, Ig gamma-4 chain C region and N-acetylglucosamine-1-phosphotransferase subunit gamma etc.) showed significantly increased in NDRD, and 4 proteins (Ig delta chain C region, Mannose-binding protein C, Transgelin-2 and IgGFc-binding protein) exhibited significantly decreased in NDRD compared to DN. The detail information of these proteins was summarized in Table 1.
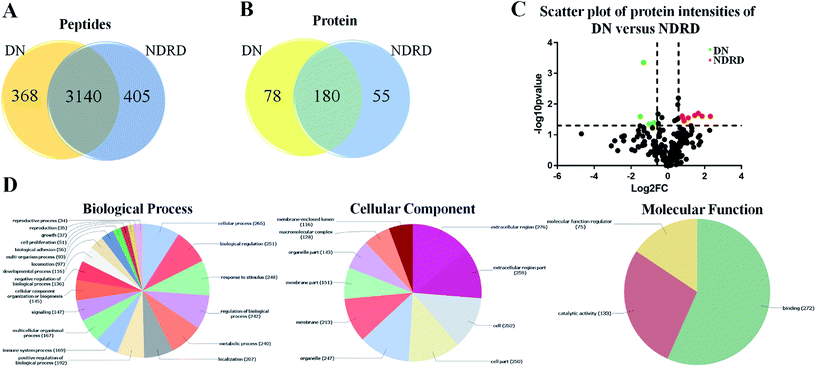 |
| Fig. 3 Characterization and bioinformatic analysis of glycoproteins isolated from DN and NDRD. (A) and (B) Cross-correlation of the isolated glycoproteins from DN and NDRD by BPL coupled magnetic particle conjugates. The Venn diagram presents the number of peptides and proteins identified. (C) Volcano plot of protein abundance differences as a function of statistical significance between DN and NDRD. Y-axis is p values (−log10) versus protein log2 fold change (x-axis) in NDRD/DN. The color code indicates upregulation (red) (fold change > 1.5, p < 0.05) and downregulation (green) (fold change < 0.67, p < 0.05). Proteins with no statistically significant difference in expression between NDRD and DN are in black. (D) Classification of the identified proteins in biological process, cellular component and molecular function by Blast2GO. | |
Table 1 Proteins with significantly altered relative abundance between DN and NDRD
No. |
Uniprot accession |
Protein name |
Gene |
Glycosylationa |
Mol. weight [kDa] |
Fold changeb (NDRD/DN) |
p-valuec |
“YN” and “YO” represent the identified protein annotated as “N-linked glycosylated” and “O-linked glycosylated” in Swiss-Prot; “PN” and “PO” represents potential N-linked glycoproteins and potential O-linked glycoproteins predicted by the software NetNGlyc 1.0 and NetOGlyc 4.0 Servers; “N” represents proteins with no typical glycosylation site. The fold change was calculated to compare protein expression level between NDRD and DN. The differences identified as significant (with fold change > 1.5 or <0.66 and p < 0.05). P value was calculated by two tailed Student's t test. |
1 |
Q5TCS8-5 |
Adenylate kinase 9 |
AK9 |
PN,O |
39.0 |
4.95 |
0.02 |
2 |
A0A0G2JPD4 |
Ig gamma-4 chain C region |
IGHG4 |
PN,O |
35.9 |
3.63 |
0.02 |
3 |
Q9UJJ9 |
N-acetylglucosamine-1-phosphotransferase subunit gamma |
GNPTG |
YN, PN,O |
34.0 |
3.13 |
0.02 |
4 |
G3XAM2 |
Complement factor I |
CFI |
PN,O |
65.1 |
2.76 |
0.02 |
5 |
P43652 |
Afamin |
AFM |
YN, PN,O |
69.1 |
2.17 |
0.03 |
6 |
A0A087X1J7 |
Glutathione peroxidase 3 |
GPX3 |
PO |
25.4 |
1.84 |
0.04 |
7 |
P63261 |
Actin, cytoplasmic 2 |
ACTB |
PN,O |
41.8 |
1.75 |
0.03 |
8 |
P01714 |
Ig lambda chain V-III region SH |
IGLV3-19 |
N |
11.4 |
1.72 |
0.02 |
9 |
A0A087WXI2 |
IgGFc-binding protein |
FCGBP |
PN,O |
445.2 |
0.60 |
0.04 |
10 |
X6RJP6 |
Transgelin-2 |
TAGLN2 |
PN,O |
21.1 |
0.49 |
0.04 |
11 |
P11226 |
Mannose-binding protein C |
MBL2 |
PO |
26.1 |
0.40 |
0.00 |
12 |
P01880 |
Ig delta chain C region |
IGHD |
YN, PN,O |
42.3 |
0.35 |
0.03 |
Bioinformatics analysis of the proteins isolated from DN and NDRD
To better understand the biological functions of serum glycoproteins containing Galβ1-3GalNAc which isolated from DN and NDRD patients, Blast2GO (http://www.blast2go.org/) software was used to obtain Gene Ontology (GO) annotations of the isolated proteins and classified into cellular component, biological process, and molecular function. Totally, 313 proteins were identified in DN and NDRD patients, among these, 294 proteins were annotated successfully. As the results of classifying, it revealed that, in terms of biological processes, 265 proteins (90.14%) were involved in cellular process and 251 proteins (85.37%) were involved in biological regulation. In the cellular component group, 276 and 259 proteins (93.88% and 88.10%) were extracellular region and extracellular region part proteins, and 252 and 250 proteins (85.71% and 85.03%) were cell and cell part proteins. In terms of molecular function, proteins with binding ability formed the largest group (272, 92.52%), and other smaller groups identified included catalytic activity (133, 45.24%) and molecular function regulator activity (75, 25.51%) (Fig. 3D).
There were 78 proteins were specially identified in DN and four proteins showed significantly increased in DN compared to NDRD patients, otherwise, 55 proteins were identified only in NDRD patients and eight proteins exhibited significantly decreased in DN compared to NDRD patients. To analysis potential differences in the GO annotations and biological function, these differential proteins were subjected to GO annotation, pathway mapping and network analysis. It observed that the differential proteins of DN and NDRD involved in the similar biological processes such as cellular process and biological regulation (Fig. 4A). However, in term of cellular component, the percentage of macromolecular complex of differential proteins showed difference between DN (65.43%) and NDRD (32.84%) (Fig. 4B). In terms of molecular function, proteins involved in molecular function regulator showed difference between DN (24.69%) and NDRD (8.82%) (Fig. 4C). According to the result of pathway enrichment analysis, it revealed that three signal pathways showed enriched in proteins that increased in DN (such as focal adhesion, ECM–receptor interaction and leukocyte transendothelial migration pathway). In contrast, three signal pathways (complement and coagulation cascades, hypertrophic cardiomyopathy (HCM) and dilated cardiomyopathy pathway) exhibited enriched in isolated proteins that increased in NDRD (Table S2†). Then, protein–protein interaction networks were identified for proteins that were unique or significantly higher in DN or NDRD. There was one distinct protein–protein interaction set (including CLEC3B, ALDOA and CFD, etc.) observed in differential protein of DN (Fig. 4D). There were two protein–protein interaction sets were observed in differential protein of NDRD. The protein–protein interaction set (TPM3, TPM4 and ACTG1) involved in the pathway of cardiac muscle contraction (Fig. 4E).
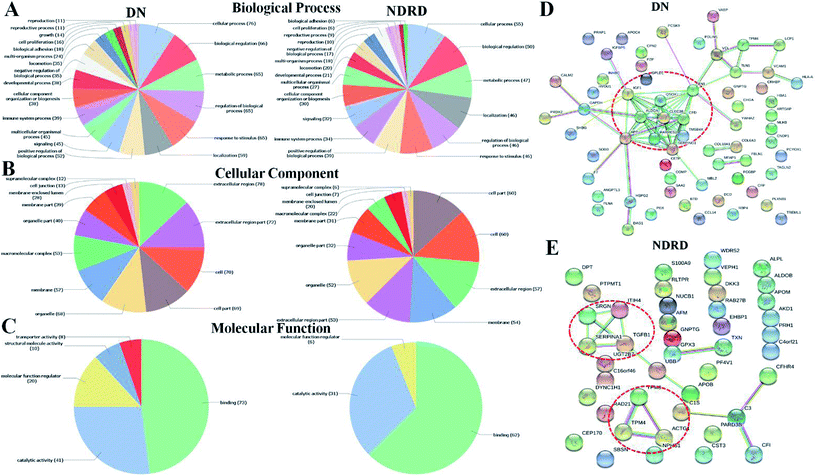 |
| Fig. 4 Characterization and bioinformatic analysis of differential glycoproteins isolated from DN and NDRD. Gene Ontology (GO) domain overview of differential proteins. The differential proteins of DN and NDRD were input into the three GO domains: biological process (A), cellular component (B), and molecular function (C) and the resultant terms associated with these terms are visualized as pie charts. Term names are located next to their position on the chart. (D and E) Protein interaction network generated and visualized with STRING 9.0 for differential proteins of DN or NDRD. The strength of the associations is represented by line thickness. Networks with three or more protein interactions are shown. Required confidence (score) of protein association was high confidence. Selected, functionally important protein core complexes and the proteins involved in same biochemical reaction are marked with red imaginary line. | |
Discussion
It is well-established that altered glycosylation in serum is a potential biomarker for rapid screening and early diagnosis of certain diseases.28–30 When a disease occurs, or during the disease process, the glycan pattern can change dramatically.31
Based on these, the detection of glycosylation of certain tumor-related proteins such as prostate specific antigen (PSA) and alpha fetoprotein (AFP) could improve of prostate and liver cancer diagnosis.32–34 Lectin microarray is a powerful tool for investigating the glycopatterns of protein for decades. Recently, Mise et al. utilized lectin microarray to investigate urinary glycan profile of patients with type 2 diabetes, their findings indicated that some glycan patterns could reflect the progression of diabetes and these glycan indexes improved prediction of the outcome of diabetes.35
In this study, the serum from patients with DN and four main types of NDRD (MN, IgAN, MPGN and FSGS) were collected and the glycan profiles were compared by using lectin microarray. It revealed that the relative abundance of glycan patterns identified by 6 and 3 lectins showed significantly increased or decreased in DN compared to NDRD groups. Notably, the glycan patterns of Tn antigen, blood group A antigen binder DBA showed significantly increased in DN compared to all NDRD groups (p < 0.05). The bisecting GlcNAc and biantennary complex-type N-glycan binder PHA-E (p < 0.05) also showed significantly increased in DN compared to all NDRD groups. However, the fucosylation exhibited heterogeneity between DN and NDRD. The expression level of Fucα1-3/6GlcNAc showed significantly increased in DN than that in all NDRD groups except FSGS, but the glycan patterns of Fucα1-2Gal which identified by UEA-I showed significantly decreased in DN compared to MN, IgAN and FSGS. Conversely, the expression of glycan patterns of Galβ1-3GalNAc and terminal GalNAc which identified by BPL showed significantly increased in all NDRD groups compared to DN (fold change < 0.43, p < 0.05). Our findings indicated that the glycan patterns showed difference between DN and NDRD.
Changes in the glycosylation profiles of serum proteins could also be caused by some reasons such as alterations in biosynthesis of glycans and changes in the clearance rate of the glycoproteins.36 There is growing evidence that alterations of glycosylation is characteristic of diabetes. It reported that elevation in serum fucose levels was observed in diabetic rat and mouse model.37,38 The increased mRNA levels of α-1,6-fucosyltransferase and glycoproteins (such as α1-acid glycoprotein and UT-A1 urea transporter) containing fucose residue have been indicated to contributed to this altered.38–40 There are some reports stating that the glycan patterns of bisecting GlcNAc which identified by PHA-E showed remarkable disease-associated differences in kidney glycoprotein expression between rats with diabetic nephropathy and controls.25 IgAN is a main type of NDRD, it known that the aberrant O-glycosylation on IgA1 is feature of IgA nephropathy and the antibodies recognizing aberrant IgA1 could promote pathogenetic process.41,42 Several studies revealed that downregulation of C1GalT1 and/or Cosmc is the key event, and excessive sialylation of GalNAc by α-2,6-sialyltransferase also contribute to the altered in IgA1 O-glycosylation.43
Subsequently, the serum glycoproteins containing Galβ1-3GalNAc and terminal GalNAc from DN and NDRD patients were isolated by BPL coupled magnetic particle conjugate, and identified by LC-MS/MS respectively. It revealed that 78 proteins were identified in DN patients uniquely and 55 proteins were only identified in NDRD patients. Besides that, the expression of 8 proteins were significantly increased and 4 proteins were significantly decreased in NDRD compared to DN. Among these differential expression proteins, the expression of adenylate kinase 9 was remarkably higher in NDRD than that in DN. Adenylate kinase leads to the production of adenosine monophosphate, which promotes glycolysis through the activation of phosphorylase and phosphofructokinase. The up-regulated of anaerobic glycolysis was observed in serum of patents with IgAN.44 IGH4 is one of four known IGHG subclasses, which located in constant region of immunoglobulin heavy chains. It reported that the expression of IGH4 showed significantly decreased in umbilical vein plasma of patients with gestational diabetes mellitus compared to controls.45 IgAN is characterized by glomerular co-deposition of IgA and complement components, and the IgA could activate the alternative pathway of complement.46 Complement factor I(CFI) involved in cleavage of C3b to inactive iC3b and dissociate the C3 and C5-convertases.47 Complement factor H could act as a cofactor for CFI to regulates the alternative complement pathway. It known that alternative pathway of complement is activated in IgAN.48 Based on these, it suggested increasing of CFI correlated with the activation of the alternative complement pathway. Afamin is a vitamin E binding protein which involved in the regulation and transport of vitamin E at the blood–brain barrier. It reported that afamin was found to be abundant in the urine of IgAN patients, which could be a predictive biomarker for severity of IgA nephropathy.49,50 In addition, the upregulated of afamin was correlated with FSGS and MPGN.51,52 Elevated levels of mannose-binding lectin was correlated with type 1 diabetes and which could serve as a predictor for the progression of diabetic nephropathy in type 1 diabetes.53–55 Furthermore, mannose-binding protein C could be used to predict diabetic nephropathy.44
Experimental
Study population
Patients who underwent renal biopsies from March 2015 to March 2016 at the Chinese People's Liberation Army General Hospital were enrolled. The renal biopsy standard was consistent with the guideline for the 2008 Kidney Disease Outcomes Quality Initiative (KDOQI) guidelines; the guidelines include the following clinical manifestations for suspected NDRD patients: naked eye/microscopic hematuria, elevated serum creatinine not accompanied by significant proteinuria, persistent large amounts of proteinuria with normal renal function, and no diabetic retinopathy. Patients who had a renal biopsy analysis following the criteria above were screened according to the following inclusion/exclusion criteria. Inclusion criteria were as follows: age between 20 and 70 years of age; diagnosis of type 2 diabetes and persistent urinary protein positivity (urinary micro albumin excretion rate ≥ 300 mg/24 h or urinary protein excretion rate ≥ 500 mg/24 h at least twice, and excluding urinary tract infection); serum creatinine < 442 μmol L−1; and voluntary acceptance of renal biopsy. Exclusion criteria were as follows: diagnosis of kidney disease prior to diagnosis as type 2 diabetes; can be diagnosed as NDRD clinically, including lupus nephritis, Henoch–Schonlein purpura nephritis, family hereditary nephropathy, such as autosomal dominant polycystic kidney disease; and unclear pathological diagnosis. At least two pathology experts and two nephrology doctors were asked to make a diagnosis using renal histopathology. Thus, 24 cases were diagnosed as DN and 53 cases were diagnosed as NDRD, of which, four main types of NDRD, including MN, MPGN, IgAN and FSGS were recruited in this study. 2 mL whole blood from each patient was collected in Pro-coagulation tube (BD Biosciences, San Diego, CA, USA). After 0.5 h incubation at room temperature, the tubes were centrifuged at 3000 rpm for 15 min, then serum was separated and store at −80 °C. The clinical information of the patients was summarized in Table 2.
Table 2 Biochemical characteristics of clinical specimensa
|
DN |
MN |
IgAN |
MPGN |
FSGS |
All values are presented as mean ± SD. DN: diabetic nephropathy; MN: membranous nephropathy; IgAN: IgA nephropathy; MPGN: mesangial proliferative glomerular nephritis; FSGS: focal segmental glomerular sclerosis. |
Number of subjects |
24 |
16 |
12 |
14 |
11 |
Age (years) |
49.1 ± 9.7 |
58.6 ± 6.0 |
48.5 ± 4.0 |
54.7 ± 10.9 |
47.2 ± 7.8 |
Duration of diabetes (months) |
144 (36–216) |
66 (1.2–156) |
66 (48–180) |
90 (12–300) |
48 (12–192) |
Systolic blood pressure (mm Hg) |
148.3 ± 23.3 |
135.3 ± 22.3 |
140.0 ± 18.0 |
150.0 ± 24.6 |
153.0 ± 15.6 |
Diastolic blood pressure (mm Hg) |
83.3 ± 15.4 |
82.0 ± 14.1 |
86.0 ± 10.9 |
89.3 ± 6.6 |
90.8 ± 12.6 |
Creatinine (μmol L−1) |
116.0 ± 84.6 |
77.5 ± 23.6 |
93.1 ± 27.4 |
104.9 ± 46.0 |
97.8 ± 5 2.3 |
Hemoglobin A1c (%) |
7.0 ± 1.2 |
6.4 ± 1.2 |
6.4 ± 1.0 |
7.2 ± 1.0 |
6.5 ± 0.4 |
Hemoglobin (g L−1) |
118.6 ± 15.4 |
118.2 ± 24.9 |
130.7 ± 20.0 |
143.2 ± 13.3 |
127.5 ± 24.7 |
Serum albumin (g L−1) |
35.7 ± 6.6 |
22.4 ± 7.8 |
37.1 ± 9.5 |
42.5 ± 4.9 |
37.6 ± 8.9 |
Total cholesterol (mmol L−1) |
5.3 ± 1.5 |
7.3 ± 2.1 |
4.3 ± 0.8 |
4.8 ± 0.9 |
4.8 ± 1.2 |
Triglyceride (mmol L−1) |
2.4 ± 1.1 |
2.5 ± 1.5 |
2.3 ± 1.2 |
1.7 ± 0.6 |
2.3 ± 0.8 |
Urinary protein (g/24 h) |
2.6 (0.6–15.5) |
4.3 (1.3–5.7) |
0.8 (0.2–2.2) |
1.7 (0.2–8.4) |
2.7 (0.4–4.5) |
Diabetic retinopathy |
16.6% |
16.0% |
20.0% |
14.3% |
9.0% |
Hematuria |
16.6% |
50.0% |
70.0% |
7.1% |
27.3% |
Study approval
The collection of human serum specimen was carried out in accordance with the approved guidelines, approved by the Human Ethics Committee of all participating units (the Department of Nephrology, Chinese PLA General Hospital, Chinese PLA Institute of Nephrology, State Key Laboratory of Kidney Diseases, National Clinical Research Center of Kidney Diseases, Beijing Key Laboratory of Kidney Disease (Beijing, China)). Written informed consent was received from participants for the collection of their serum samples. This study was conducted in accordance with the ethical guidelines of the Declaration of Helsinki.
Lectin microarray fabrication and data analysis
The lectin microarray was fabricated as described previously.56–58 In brief, 37 lectins (purchased from Vector Laboratories and Sigma-Aldrich) were dissolved in recommended buffer to the final concentration of 1 mg mL−1, and then spotted on the epoxysilane-coated slides (homemade) in triplicate per microarray. After blocking by blocking solution (2% BSA in 1× PBST (0.2% Tween 20 (v/v) in 1× PBS, pH 7.4)) for 1 h, the lectin microarray was ready to be used. The concentration of serum protein of each patients was determined by BCA protein assay kit (Beyotime Biotechnology, China). To reduce the differences between subjects and to tolerate individual variation, 24 cases of DN was divided into six groups; 12 cases of IgAN, 14 cases of MPGN and 11 cases of FSGS was divided into three groups respectively, 16 cases of MN was divided into four groups. In each group, serum proteins were pooled equally. 100 μg of the pooled serum protein was mixed with equivalent volume of 0.1 M NaHCO3 (pH 9.3) and incubated with Cy3 dye (GE healthcare, Piscataway, NJ) for 3 h. After purified, 4 μg of Cy3 labelled protein was incubated with lectin microarray at 37 °C for 3 h. After that, microarray was washed and centrifuged to dry. The fluorescence intensity of each lectin spot was extracted by GenePix software (version 6.0; Axon Instruments Inc.; Sunnyvale, CA). To eliminate the influence of non-specific adsorption, the fluorescence intensities of the lectin spots which were less than average background + standard deviations (SD) were excluded, and the method of global normalization was used to eliminate fluoresce bias between groups. The normalized data of DN and four NDRD groups (IgAN, MN, MPGN and FSGS) were compared based upon fold-changes according to the following criteria: fold changes ≥ 1.5 or ≤ 0.67 and p < 0.05 in the pairs indicated increased or decreased of certain kind of glycan patterns respectively. Differences between DN and NDRD groups were tested by one-way ANOVA followed by Dunnett's post hoc test. The lectins which the normalized fluorescence intensities exhibited a significant difference between DN and NDRD groups were further analyzed by Expander 6.4 (version 6.4; http://acgt.cs.tau.ac.il/expander/). In order to perform hierarchical clustering analysis, the unweighted pair group method was constructed with arithmetic-mean tree using Pearson's correlation as the metric of similarity. All statistical analyses were performed using GraphPad Prism (version 7.0; Graphpad Prism Software Inc., San Diego, USA).
Lectin blotting and western blotting
The lectin blotting was performed as described.59 Similar with immunoblotting, 40 μg serum protein from DN and four NDRD groups (IgAN, MN, MPGN and FSGS) was separated by 10% polyacrylamide resolving gel and a 3% stacking gel. And then, the proteins in gel were transferred into PVDF membrane (0.22 μm Millipore, Bedford, MA, USA) by using TE70 Semi-Dry Transfer Unit (GE healthcare). The membrane was blocked by 1× carbo-free blocking solution (vector labs, Burlingame, CA) for 1 h. Then, 30 μg of Cy5 labelled AAL, DBA and BPL were added into blocking solution respectively, membranes were incubated overnight at 4 °C with protected from light. Membranes were washed three time with TBST buffer and the image was acquired by STROM fluorImager (Molecular Dynamics, Sunnyvale, CA, USA). The gray values of the protein bands were measured by imageJ software (NIH). The β-actin served as the internal control. Briefly, after scanned by STORM, the membrane was washed and incubated with anti-β actin mouse monoclonal antibody (dilution 1
:
1000, CoWin Biosciences; Beijing, China) at 4 °C overnight. After washing three times with TBST, the membrane was incubated with HRP-labelled secondary antibody (dilution 1
:
5000; wanlei bio, China) for 1 h. Finally, the membrane was developed with Immobilon Western Chemiluminescent HRP Substrate (Millipore Billerica, MA) and visualized by Chemiluminescent Imaging System4200 (Tanon Science & Technology Co. Shanghai, China).
Isolation of glycoproteins by BPL coupled magnetic particle conjugate
The isolation was performed as described.60 Briefly, 400 μg of BPL was dissolved into 400 μL binding solution (0.1 M Tris-HCl, 150 mM NaCl, 1 mM CaCl2, 1 mM MgCl2, and 1 mM MnCl2, pH 7.4) and coupled with epoxysilane-coated magnetic particles (homemade) in binding buffer for 3 h. After washing with washing buffer (binding solution contained 0.02% Tween-20 (v/v)) for three times, the BPL coupled magnetic particle conjugate was blocked by 1× carbo-free blocking solution (vectorlabs) for 1 h at room temperature. Then the conjugate was mixed with 1 mg pooled serum protein and the mixture was incubated at room temperature for 3 h with shaking to enrich glycoproteins. After that, three times washing were performed to wash away non-specific binding proteins, and specific binding glycoproteins were eluted by competitive elution buffer (100 mM lactose). The concentration of eluted protein was determined by BCA protein assay kit.
Nano LC-MS/MS analysis
Firstly, the eluted protein was exchanged buffer by using 3K centrifugal ultrafiltration (Amicon Ultra, Millipore, USA). Proteins were reduced with DTT and alkylated using iodoacetamide, and digested by sequencing-grade modified trypsin (Promega, USA) overnight at 37 °C with shaking. And then, peptides were purified by OASIS HLB cartridge (Waters, Milford, MA, USA). The purified peptides were eluted by elution solution I (60% ACN containing 0.5% TFA) and elution solution II (40% ACN containing 0.5% TFA) and evaporated to dryness and dissolved into 0.1% TFA solution and analyzed in triplicate. All nano LC-MS/MS experiments were performed on a Q Exactive (Thermo Scientific) equipped with an Easy n-LC 1000 HPLC system (Thermo Scientific). The peptides were loaded onto a 100 μm id × 2 cm fused silica trap column packed in-house with reversed phase silica (Reprosil-Pur C18 AQ, 5 μm, Dr Maisch GmbH) and then separated on an a 75 μm id × 20 cm C18 column packed with reversed phase silica (Reprosil-Pur C18 AQ, 3 μm, Dr Maisch GmbH). The peptides bounded on the column were eluted with a 75 min linear gradient. The solvent A consisted of 0.1% formic acid (FA) in water solution and the solvent B consisted of 0.1% FA in ACN solution. The segmented gradient was 4–12% B, 5 min; 12–22% B, 50 min; 22–32% B, 12 min; 32–90% B, 1 min; 90% B, 7 min at a flow rate of 280 nL min−1.
Database searching and analysis
The MS analysis was performed with Q Exactive mass spectrometer (Thermo Scientific) with the data-dependent acquisition mode, the instrument settings were as follows: the MS data were acquired at a high resolution 70
000 (m/z 200) across the mass range of 300–1600 m/z. The target value was 3.00 × 106 with a maximum injection time of 60 ms. The top 20 precursor ions were selected from each MS full scan with isolation width of 2 m/z for fragmentation in the HCD collision cell with normalized collision energy of 32%. Subsequently, MS/MS spectra were acquired at resolution 17
500 at m/z 200. The target value was 5.00 × 104 with a maximum injection time of 80 ms. The dynamic exclusion time was 40 s. For nano-electrospray ion source setting, the spray voltage was 2.0 kV; no sheath gas flow; the heated capillary temperature was 320 °C. For each analysis, 2 μg peptides were injected and each sample was measured in triplicate. Following LC-MS/MS acquisition, the data were searched using MaxQuant software (version v1.5.3.30), against the Uniprot_Human_20160226 as described:61,62 a tolerance level of 20 ppm for MS and 0.02 Da for MS/MS. Trypsin was used as the digesting enzyme, and two missed cleavages were allowed. The carbamidomethylation of cysteines was set as a fixed modification, the oxidation of methionines and acetylation of N-terminal of proteins were allowed as a variable modification. The maximum false peptide and protein discovery rate ≤ 0.01. Match between runs was used with 0.7 min match time window and 20 min alignment time window.
The raw data generated by MaxQuant was assessed using Perseus software (Version 1.5.5.3, http://www.perseus-framework.org) as previously described.63 The analysis of the plasma samples was based on the label-free quantification (LFQ) intensities. The fold changes in the level of the proteins were assessed by comparing the mean LFQ intensities between DN and NDRD. The proteins were considered to be increased or decreased if the fold changes > 1.5 or <0.66 and the difference was statistically significant (p < 0.05).
Bioinformatics analysis
Gene-Ontology (GO) analysis was used to get more information about biological function and significance of proteins. In this study, the biological processes, molecular function and cellular component of all isolated proteins and differential proteins were assessed using Blast2GO software (version 5.0). In addition, the pathway enrichment analysis of differential proteins was performed by DAVID Bioinformatics Resources (version 6.8). The mapped KEGG pathways with thresholds of count ≥ 4 and a p value < 0.05 versus the background signal of the human genome were considered as enriched pathways. Functional interaction network analysis of differential proteins was performed using STRING database. The interaction score = 0.7 (high confidence) and interactions derived from text-mining were excluded.
Conclusions
Although certain clinical indexes such as systolic blood pressure, glycated HbA1c, hematuria, diabetic retinopathy as well as hemoglobin are independently related to DN but renal biopsy remain the standard approach to differentiate DN and NDRD. In present study, our findings showed that the glycan patterns of serum proteins were different between DN and NDRD patients, especially the glycan pattern of Galβ1-3GalNAc showed significant difference between DN and NDRD groups. This finding provided a new method to distinguish between DN and NDRD. Furtherly, the proteins modified with Galβ1-3GalNAc were isolated by BPL coupled magnetic particle conjugate. The relative abundance of 12 proteins showed significantly difference between DN and NDRD patients. The altered of these proteins may attribute to the pathological differences between DN and NDRD. Our findings indicated that the glycosylation of serum proteins showed difference between DN and NDRD, including the altered of glycan patterns and glycoproteins. A large sample size is being recruited to verify our findings and evaluate the diagnostic value of altered of glycan patterns and glycoproteins in next step.
Conflicts of interest
The authors declare no conflict of interest.
Acknowledgements
This study was supported by (1) National Key R&D Program of China (2016YFC1305500); (2) National Natural Science Foundation of China (No. 61471399; No. 61671479); (3) Innovation Nursery Fund of PLA General Hospital (No. 15KMZ04).
References
- Y. S. Kanwar, L. Sun, P. Xie, F. Y. Liu and S. Chen, Annu. Rev. Pathol.: Mech. Dis., 2011, 6, 395 CrossRef CAS PubMed.
- D. Fineberg, K. A. Jandeleit-Dahm and M. E. Cooper, Nat. Rev. Endocrinol., 2013, 9, 713 CrossRef CAS PubMed.
- A. S. Levey, N. Engl. J. Med., 2002, 347, 1505 CrossRef PubMed.
- S. Liang, X. Zhang, G. Cai, H. Zhu, J. Zhou, J. Wu, P. Chen, S. Lin, Q. Qiu and X. Chen, PLoS One, 2013, 8, e64184 CrossRef CAS PubMed.
- Z. Dong, Y. Wang, Q. Qiu, X. Zhang, L. Zhang, J. Wu, R. Wei, H. Zhu, G. Cai, X. Sun and X. Chen, Diabetes Res. Clin. Pract., 2016, 121, 112 CrossRef PubMed.
- M. Y. Liu, X. M. Chen, X. F. Sun, J. H. Zhou, X. G. Zhang, H. Y. Zhu, Y. Z. Chen, S. W. Liu, R. B. Wei, L. Tang, G. Y. Cai, L. Zhang and X. Y. Bai, J. Diabetes, 2014, 6, 519 CrossRef CAS PubMed.
- K. Kritmetapak, S. Anutrakulchai, C. Pongchaiyakul and A. Puapairoj, Nephrol., Dial., Transplant., 2016, 31, i220 CrossRef.
- K. Ohtsubo and J. D. Marth, Cell, 2006, 126, 855 CrossRef CAS PubMed.
- H. B. Guo, I. Lee, M. Kamar, S. K. Akiyama and M. Pierce, Cancer Res., 2002, 62, 6837 CAS.
- E. A. Partridge, C. Le Roy, G. M. Di Guglielmo, J. Pawling, P. Cheung, M. Granovsky, I. R. Nabi, J. L. Wrana and J. W. Dennis, Science, 2004, 306, 120 CrossRef CAS PubMed.
- C. A. Reis, H. Osorio, L. Silva, C. Gomes and L. David, J. Clin. Pathol., 2010, 63, 322 CrossRef CAS PubMed.
- S. S. Pinho, S. Carvalho, R. Marcos-Pinto, A. Magalhães, C. Oliveira, J. Gu, M. Dinis-Ribeiro, F. Carneiro, R. Seruca and C. A. Reis, Trends Mol. Med., 2013, 19, 664 CrossRef CAS PubMed.
- S. S. Pinho and C. A. Reis, Nat. Rev. Cancer, 2015, 15, 540 CrossRef CAS PubMed.
- S. Julien, A. Ivetic, A. Grigoriadis, D. QiZe, B. Burford, D. Sproviero, G. Picco, C. Gillett, S. L. Papp, L. Schaffer, A. Tutt, J. Taylor-Papadimitriou, S. E. Pinder and J. M. Burchell, Cancer Res., 2011, 71, 7683 CrossRef CAS PubMed.
- S. R. Stowell, T. Ju and R. D. Cummings, Annu. Rev. Pathol.: Mech. Dis., 2015, 10, 473 CrossRef CAS PubMed.
- S. Gilgunn, P. J. Conroy, R. Saldova, P. M. Rudd and R. J. O'Kennedy, Nat. Rev. Urol., 2013, 10, 99 CrossRef CAS PubMed.
- E. Llop, M. Ferrer-Batallé, S. Barrabés, P. E. Guerrero, M. Ramírez, R. Saldova, P. M. Rudd, R. N. Aleixandre, J. Comet, R. de Llorens and R. Peracaula, Theranostics, 2016, 6, 1190 CrossRef CAS PubMed.
- U. Jeschke, I. Mylonas, N. Shabani, C. Kunert-Keil, C. Schindlbeck, B. Gerber and K. Friese, Anticancer Res., 2005, 25, 1615 CAS.
- D. H. Dube and C. R. Bertozzi, Nat. Rev. Drug Discovery, 2005, 4, 477 CrossRef CAS PubMed.
- R. Testa, V. Vanhooren, A. R. Bonfigli, M. Boemi, F. Olivieri, A. Ceriello, S. Genovese, L. Spazzafumo, V. Borelli, M. G. Bacalini, S. Salvioli, P. Garagnani, S. Dewaele, C. Libert and C. Franceschi, PLoS One, 2015, 10, e0119983 CrossRef PubMed.
- B. A. Novak J Julian, M. Tomana and J. Mestecky, Semin. Nephrol., 2008, 28, 78 CrossRef PubMed.
- A. C. Allen, E. M. Bailey, P. E. Brenchley, K. S. Buck, J. Barratt and J. Feehally, Kidney Int., 2001, 60, 969 CrossRef CAS PubMed.
- L. X. Xu and M. H. Zhao, Kidney Int., 2005, 68, 167 CrossRef CAS PubMed.
- J. Mestecky, M. Tomana, Z. Moldoveanu, B. A. Julian, H. Suzuki, K. Matousovic, M. B. Renfrow, L. Novak, R. J. Wyatt and J. Novak, Kidney Blood Pressure Res., 2008, 31, 29 CrossRef CAS PubMed.
- A. Ravidà, L. Musante, M. Kreivi, I. Miinalainen, B. Byrne, M. Saraswat, M. Henry, P. Meleady, M. Clynes and H. Holthofer, Kidney Int., 2015, 87, 963 CrossRef PubMed.
- H. Zhu, M. Liu, H. Yu, X. Liu, Y. Zhong, J. Shu, X. Fu, G. Cai, X. Chen, W. Geng, X. Yang, M. Wu, Z. Li and D. Zhang, J. Diabetes Res., 2017, 2017, 5728087 Search PubMed.
- X. Yang, H. Yu, H. Zhu, Y. Zheng, Q. Han, G. Cai and X. Chen, Chin. Med. J., 2018, 131, 180 CrossRef PubMed.
- G. Tabarés, C. M. Radcliffe, S. Barrabés, M. Ramírez, R. N. Aleixandre, W. Hoesel, R. A. Dwek, P. M. Rudd, R. Peracaula and R. de Llorens, Glycobiology, 2006, 16, 132 CrossRef PubMed.
- R. Saldova, Y. Fan, J. M. Fitzpatrick, R. W. Watson and P. M. Rudd, Glycobiology, 2011, 21, 195 CrossRef CAS PubMed.
- S. Gilgunn, P. J. Conroy, R. Saldova, P. M. Rudd and R. J. O'Kennedy, Nat. Rev. Urol., 2013, 10, 99 CrossRef CAS PubMed.
- H. K. Einarsdottir, M. H. Selman, R. Kapur, S. Scherjon, C. A. Koeleman, A. M. Deelder, C. E. van der Schoot, G. Vidarsson and M. Wuhrer, Glycoconjugate J., 2013, 30, 147 CrossRef CAS PubMed.
- E. Llop, M. Ferrer-Batallé, S. Barrabés, P. E. Guerrero, M. Ramírez, R. Saldova, P. M. Rudd, R. N. Aleixandre, J. Comet, R. de Llorens and R. Peracaula, Theranostics, 2016, 6, 1190 CrossRef CAS PubMed.
- S. Gilgunn, P. J. Conroy, R. Saldova, P. M. Rudd and R. J. O'Kennedy, Nat. Rev. Urol., 2013, 10, 99 CrossRef CAS PubMed.
- A. Kirwan, M. Utratna, M. E. O'Dwyer, L. Joshi and M. Kilcoyne, BioMed Res. Int., 2015, 2015, 490531 Search PubMed.
- K. Mise, M. Imamura, S. Yamaguchi, S. Teshigawara, A. Tone, H. A. Uchida, J. Eguchi, A. Nakatsuka, D. Ogawa, M. Yoshida, M. Yamada, K. Shikata and J. Wada, Diabetes Care, 2018, 41, 1765 Search PubMed.
- A. Kobata, Biochimie, 2003, 85, 13 CrossRef CAS PubMed.
- G. Saravanan, P. Ponmurugan, G. P. Senthil Kumar and T. Rajarajan, Phytomedicine, 2010, 17, 1086 CrossRef CAS PubMed.
- N. Itoh, S. Sakaue, H. Nakagawa, M. Kurogochi, H. Ohira, K. Deguchi, S. Nishimura and M. Nishimura, Am. J. Physiol.: Endocrinol. Metab., 2007, 293, E1069 CrossRef CAS PubMed.
- D. C. Poland, C. G. Schalkwijk, C. D. Stehouwer, C. A. Koeleman, B. van het Hof and W. van Dijk, Glycoconjugate J., 2001, 18, 261 CrossRef CAS PubMed.
- X. Qian, X. Li, T. O. Ilori, J. D. Klein, R. P. Hughey, C. J. Li, A. A. Alli, Z. Guo, P. Yu, X. Song and G. Chen, Front. Physiol., 2015, 6, 274 Search PubMed.
- M. Stuchlová Horynová, M. Raška, H. Clausen and J. Novak, Cell. Mol. Life Sci., 2013, 70, 829 CrossRef PubMed.
- R. J. Wyatt and B. A. Julian, N. Engl. J. Med., 2013, 368, 2402 CrossRef CAS PubMed.
- J. K. Boyd, C. K. Cheung, K. Molyneux, J. Feehally and J. Barratt, Kidney Int., 2012, 81, 833 CrossRef CAS PubMed.
- W. Sui, L. Li, W. Che, Z. Guimai, J. Chen, W. Li and Y. Dai, Clinics, 2012, 67, 363 CrossRef PubMed.
- Z. Miao, J. Wang, F. Wang, L. Liu, H. Ding and Z. Shi, Proteomics: Clin. Appl., 2016, 10, 1122 CAS.
- A. Roos, M. P. Rastaldi, N. Calvaresi, B. D. Oortwijn, N. Schlagwein, D. J. van Gijlswijk-Janssen, G. L. Stahl, M. Matsushita, T. Fujita, C. van Kooten and M. R. Daha, J. Am. Soc. Nephrol., 2006, 17, 1724 CrossRef CAS PubMed.
- P. F. Zipfel and C. Skerka, Nat. Rev. Immunol., 2009, 9, 729 CrossRef CAS PubMed.
- H. Hu, A. Nagra, M. R. Haq and R. D. Gilbert, Pediatr. Nephrol., 2014, 29, 1103 CrossRef PubMed.
- S. Kalantari, D. Rutishauser, S. Samavat, M. Nafar, L. Mahmudieh, M. Rezaei-Tavirani and R. A. Zubarev, PLoS One, 2013, 8, e80830 CrossRef PubMed.
- P. Prikryl, L. Vojtova, D. Maixnerova, M. Vokurka, M. Neprasova, T. Zima and V. Tesar, Physiol. Res., 2017, 66, 621 CAS.
- M. Zhao, M. Li, X. Li, C. Shao, J. Yin and Y. Gao, Proteome Sci., 2014, 12, 42 CrossRef PubMed.
- Y. Lu, X. Chen, Z. Yin, S. Zhu, D. Wu and X. Chen, Proteomics, 2016, 16, 1015 CrossRef CAS PubMed.
- T. K. Hansen, S. Thiel, S. T. Knudsen, C. H. Gravholt, J. S. Christiansen, C. E. Mogensen and P. L. Poulsen, J. Clin. Endocrinol. Metab., 2003, 88, 4857 CrossRef CAS PubMed.
- P. Hovind, T. K. Hansen, L. Tarnow, S. Thiel, R. Steffensen, A. Flyvbjerg and H. H. Parving, Diabetes, 2005, 54, 1523 CrossRef CAS PubMed.
- T. K. Hansen, C. Forsblom, M. Saraheimo, L. Thorn, J. Wadén, P. Høyem, J. Østergaard, A. Flyvbjerg, P. H. Groop and FinnDiane Study Group, Diabetologia, 2010, 53, 1517 CrossRef CAS PubMed.
- H. Yu, M. Zhu, Y. Qin, Y. Zhong, H. Yan, Q. Wang, H. Bian and Z. Li, J. Proteome Res., 2012, 11, 5277 CrossRef CAS PubMed.
- J. Shu, H. Yu, X. Li, D. Zhang, X. Liu, H. Du, J. Zhang, Z. Yang, H. Xie and Z. Li, Oncotarget, 2017, 8, 35718 Search PubMed.
- Y. Zhong, Y. Qin, H. Yu, J. Yu, H. Wu, L. Chen, P. Zhang, X. Wang, Z. Jia, Y. Guo, H. Zhang, J. Shan, Y. Wang, H. Xie, X. Li and Z. Li, Sci. Rep., 2015, 5, 8971 CrossRef CAS PubMed.
- Y. Qin, Y. Zhong, M. Zhu, L. Dang, H. Yu, Z. Chen, W. Chen, X. Wang, H. Zhang and Z. Li, J. Proteome Res., 2013, 12, 2742 CrossRef CAS PubMed.
- G. Yang, T. Cui, Y. Wang, S. Sun, T. Ma, T. Wang, Q. Chen and Z. Li, Proteomics, 2013, 13, 1481 CrossRef CAS PubMed.
- J. Cox and M. Mann, Nat. Biotechnol., 2008, 26, 1367 CrossRef CAS PubMed.
- S. Kalayou, C. Granum, H. F. Berntsen, P. K. Groseth, S. Verhaegen, L. Connolly, I. Brandt, G. A. de Souza and E. Ropstad, J. Proteomics, 2016, 137, 68 CrossRef CAS PubMed.
- R. Sivadasan, D. Hornburg, C. Drepper, N. Frank, S. Jablonka, A. Hansel, X. Lojewski, J. Sterneckert, A. Hermann, P. J. Shaw, P. G. Ince, M. Mann, F. Meissner and M. Sendtner, Nat. Neurosci., 2016, 19, 1610 CrossRef CAS PubMed.
Footnote |
† Electronic supplementary information (ESI) available. See DOI: 10.1039/c8ra06832a |
|
This journal is © The Royal Society of Chemistry 2018 |
Click here to see how this site uses Cookies. View our privacy policy here.