DOI:
10.1039/C8RA05430D
(Paper)
RSC Adv., 2018,
8, 32440-32453
Implementation of a dynamic intestinal gut-on-a-chip barrier model for transport studies of lipophilic dioxin congeners†
Received
25th June 2018
, Accepted 10th September 2018
First published on 19th September 2018
Abstract
Novel microfluidic technologies allow the manufacture of in vitro organ-on-a-chip systems that hold great promise to adequately recapitulate the biophysical and functional complexity of organs found in vivo. In this study, a gut-on-a-chip model was developed aiming to study the potential cellular association and transport of food contaminants. Intestinal epithelial cells (Caco-2) were cultured on a porous polyester membrane that was tightly clamped between two glass slides to form two separate flow chambers. Glass syringes, polytetrafluoroethylene tubing and glass microfluidic chips were selected to minimize surface adsorption of the studied compounds (i.e. highly lipophilic dioxins), during the transport studies. Confocal microscopy studies revealed that, upon culturing under constant flow for 7 days, Caco-2 cells formed complete and polarized monolayers as observed after culturing for 21 days under static conditions in Transwells. We exposed Caco-2 monolayers in the chip and Transwell to a mixture of 17 dioxin congeners (7 polychlorinated dibenzo-p-dioxins and 10 polychlorinated dibenzofurans) for 24 h. Gas chromatography-high resolution mass spectrometry was used to assess the cellular association and transport of individual dioxin congeners across the Caco-2 cell monolayers. After 24 h, the amount of transported dioxin mixture was similar in both the dynamic gut-on-a-chip model and the static Transwell model. The transport of individual congeners corresponded with their number of chlorine atoms and substitution patterns as revealed by quantitative structure–property relationship modelling. These results show that the gut-on-a-chip model can be used, as well as the traditional static Transwell system, to study the cellular association and transport of lipophilic compounds like dioxins.
1. Introduction
Determination of the oral bioavailability of compounds after ingestion is a crucial element in the toxicological risk assessment of food chemicals and environmental contaminants. Caco-2 cells, a cell line established from human epithelial adenocarcinoma cells, grown in Transwells® have been widely used as a general in vitro model for the prediction of in vivo intestinal food chemicals and drug absorption and to investigate local effects on the intestinal epithelium.1–3 However, grown under static conditions, such a model may not fully capture the physical and extracellular complexity found in vivo. Two main shortcomings of this model are the relatively long culture period that is needed for these cells to differentiate,4 and the static culturing conditions which fail to mimic the luminal and blood plasma flows as observed in vivo.
Recent advances in micro engineering and microfluidics have led to unprecedented organ-on-a-chip models that recapitulate not only in vivo fluid flows, but also the structure and function of human tissues.5–9 For this reason, microfluidic technology has been introduced into the field of drug discovery. This already now has resulted in a variety of models recapitulating specific organ functions, including the small intestine, in so-called gut-on-a-chip models.5,6,10,11 In a limited number of studies, drug transport has been reported on Caco-2 cell-based microfluidic devices to evaluate the permeability potential of these models.12,13 However, for the purpose of gut-on-a-chip model development and optimization, its efficiency for compound permeability should not only be investigated for drugs, but also for chemical substances in general. The gut epithelium acts as the important barrier in defence to protect the body from exogenous substances as present in food. Therefore we assessed the barrier properties of the gut-on-a-chip model for dioxins which are an important class food contaminants. Furthermore, the predictive capacity of a gut-on-a-chip model should be evaluated in terms of the comparison to the Transwell system that has been used in the past decades for this type of studies.
The term dioxins, also called polychlorinated dibenzodioxins, refers to two groups of compounds: polychlorinated dibenzo-p-dioxins (PCDDs) and dibenzofurans (PCDFs) (see Fig. 1). These are a group of chemically-related compounds (i.e. congeners) that are classified as persistent organic pollutants (POPs) and cause concern over environmental and human health impact. Dioxins are highly toxic and longer term exposure can cause reproductive and developmental problems, skin lesions, damage to the immune system, interference with the hormonal balance, and lastly dioxins may also cause cancer.14,15 Because of their lipophilic properties, or high log
P values (the partition coefficient of a molecule between an aqueous and lipophilic phase, usually octanol and water; Table 1), dioxins accumulate in the food chain, mainly in the fatty tissue of farm animals. Consumption of contaminated food, like contaminated beef and dairy products, is the primary source of dioxin entry into the human body.16–18 In fact, more than 90% of human dioxin exposure occurs through food ingestion.14,19 About 20 congeners of dioxins can be absorbed through daily meals,20,21 17 of these congeners are present in a reference mixture in the legal food monitoring programmes of the EU.22 The molecular composition (i.e. degree of chlorination), solubility, and molecular size of each congener influences its absorption rate, toxicity, tissue distribution, and elimination.23
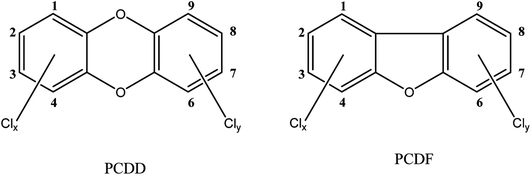 |
| Fig. 1 Structures of investigated compounds. | |
Table 1 The predicted log
P values of dioxin congeners,25 and limit of quantification (LOQ) by GC-HRMS
Congeners |
log P |
LOQ (pg mL−1) |
2,3,7,8-TCDF |
6.5 |
0.086 |
1,2,3,7,8-PeCDF |
6.9 |
0.201 |
2,3,4,7,8-PeCDF |
6.9 |
0.185 |
1,2,3,4,7,8-HxCDF |
6.9 |
0.137 |
1,2,3,6,7,8-HxCDF |
6.9 |
0.161 |
2,3,4,6,7,8-HxCDF |
7.1 |
0.186 |
1,2,3,7,8,9-HxCDF |
7.4 |
0.212 |
1,2,3,4,6,7,8-HpCDF |
7.9 |
0.165 |
1,2,3,4,7,8,9-HpCDF |
7.5 |
0.133 |
OCDF |
8.5 |
0.210 |
2,3,7,8-TCDD |
6.4 |
0.095 |
1,2,3,7,8-PeCDD |
6.6 |
0.184 |
1,2,3,4,7,8-HxCDD |
8.4 |
0.175 |
1,2,3,6,7,8-HxCDD |
7.4 |
0.185 |
1,2,3,7,8,9-HxCDD |
7.4 |
0.145 |
1,2,3,4,6,7,8-HpCDD |
7.5 |
0.147 |
OCDD |
8.1 |
0.300 |
In this study, we aimed to compare the transport of 17 different dioxin congeners (7 PCDDs and 10 PCDFs) in a dynamic microfluidic Caco-2 model with their transport in a traditional static Transwell Caco-2 model. For this, we developed a dynamic in vitro microfluidic gut-on-a-chip model with Caco-2 cells for the purpose of mimicking the in vivo conditions. Quantification of the dioxin mixture in the cellular fraction as well as the amount transported across the intestinal monolayers was performed using gas chromatography-high resolution mass spectrometry (GC-HRMS). The GC-HRMS method is considered a highly sensitive method suitable for dioxin measurements at low concentration (0.08–0.30 pg mL−1) ranges (Table 1).24 The resulting cellular association and transport of dioxins were not only compared between the two models, but we also performed quantitative structure–property relationship modelling to explain different biological behaviour of the dioxin congeners.
2. Experimental
2.1. The gut-on-a-chip device
The microfluidic gut-on-a-chip device consists of three resealable glass slides, with the same width (15 mm) and length (45 mm), upon assembly resulting in two flow chambers separated by a middle layer that contains a cell culture membrane (Fig. 2). In more detail, a polyester (PET) membrane fixed on a glass slide, with a 0.4 μm pore size, 12 μm thickness, 1.6 × 106 pore density and 1 cm2 surface area, separates the upper apical (AP) and the lower basolateral (BL) chambers (Micronit, Enschede, The Netherlands). Both the upper and lower glass slides were spaced from the middle layer membrane by 0.25 mm thick silicone bands resulting in a volume of 110 mm3 and 75 mm3 for the AP and BL side respectively, which makes the total volume of the device 185 mm3 (μL). The distance between the middle layer and the top layer was 0.25 mm and 0.65 mm for the glass and membrane section, respectively. The distance between the middle layer and bottom layer was 0.25 mm. The chip was mounted with a quick locking mechanism in the chip holder constructed for connecting external tubing to the chip via specific ferrules to ensure tight connections and a leak-free system. Constant flow was carried out using a microsyringe pump (NE-4000, New Era Pump Systems, Inc.) equipped with two glass syringes (5 mL, Luer lock tip, Fortuna®, Sigma), with each syringe connected to either the AP or the BL compartment of the chip with PTFE (Teflon) tubing (0.25 mm inner diameter, 14.5 cm length). On the outlets of the chip the same tubing was used and connected with glass collecting reservoirs. Before starting experiments, all tubing and chip parts were sterilized by autoclave and 70% ethanol and the tubing was filled with medium in order to eliminate air bubbles in the microfluidic system. The entire system was put in an incubator at 37 °C to maintain cell culture conditions. All experiments were performed at a constant flow rate of 25 μL h−1 resulting in a shear rate in the AP compartment of ∼0.0001–0.0005 dyne per cm2 (at the membrane and glass surface, respectively) and in the BL compartment of ∼0.0005 dyne per cm2.
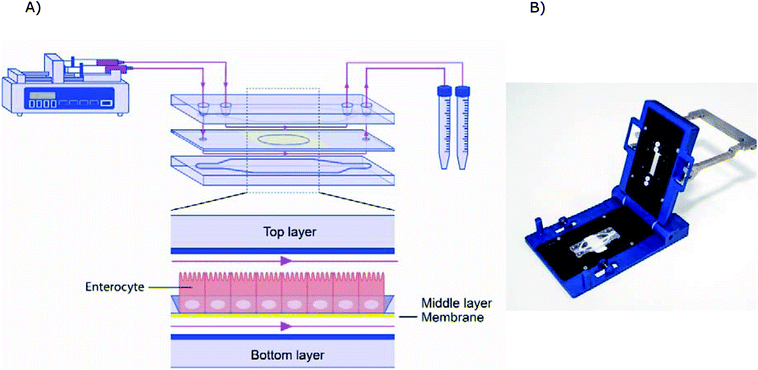 |
| Fig. 2 (A) Schematic design of the microfluidic system. (B) Image of the chip holder used to clamp the chip together and allowing connection of the tubing. | |
2.2. Optimization of the gut-on-a-chip system for dioxin mixture absorption properties
Before starting the transport experiment, the fraction of dioxins absorbed by the different types of tubing and syringes to be used in the microfluidic system was determined. The following conditions were evaluated: glass or polypropylene syringes equipped with ethylene tetrafluoroethylene (ETFE), stainless steel or Teflon tubing. Different combinations (n = 2 for each combination) were tested by rinsing with cell culture medium containing 0.2 ng mL−1 dioxin mixture (in 1% DMSO) at a flow rate of 25 μL h−1 for 24 h at 37 °C (conditions as used in the final transport experiment). The amount of total dioxins in culture medium before and after flowing through the microfluidic systems was determined by using a most cost effective and sensitive CALUX bioassay that is routinely used in our laboratories, as described previously.26 Briefly, rat H4IIE hepatoma cells, stably transfected with an AhR-controlled luciferase reporter gene plasmid (pGudLuc1.1), were grown in 48 well microtiter plates. Upon cell confluence, they were exposed in triplicate to standards and the dioxin mixture dissolved in culture medium. After 20–24 h of exposure, the cells were washed, lysed and an aliquot was used to determine the luciferase content using a plate reader (Luminoskan, Labsystems). Dioxin concentrations in the culture medium samples are expressed as total bioanalytical equivalent (BEQ) of 2,3,7,8-tetrachlorodibenzo-p-dioxin (TCDD), and were estimated from a calibration curve of the reference dioxin mixture samples whose response was fitted with an exponential curve fit. The entire experiment was repeated twice and the average of both experiments is reported.
2.3. Culture of human intestine epithelial cells
The Caco-2 cell line (HTB-37), derived from a human colorectal adenocarcinoma, was obtained from The American Type Culture Collection (ATCC, Manassas, VA, USA). They were grown (at passage number 29-45) in Dulbecco's Modified Eagle Medium (Lonza) supplemented with 10% heat-inactivated fetal bovine serum (Gibco, Waltham, MA USA), 1% penicillin–streptomycin (Sigma), 1% non-essential amino acids (Gibco), further referred to as DMEM+.
The cells were seeded at density of 40
000 cells per cm2 in 12 well Transwell polyester inserts (0.4 μm pore size, 1.12 cm2 surface area, Corning Amsterdam, The Netherlands) cultured in DMEM+. During culture period, medium was changed for every other day.
In the microfluidic chip cells were seeded at a density of 75
000 cell per cm2, the cells were allowed to attach to the membrane without flow for 72 h and then were perfused with low sodium bicarbonate (10 mM) DMEM+ (Sigma) for optimizing pH buffering capacity, through the AP and BL side for 7 days.
2.4. Immunohistochemistry
For morphological assessment of the monolayers grown in the microfluidic chips or Transwell cells were seeded (n = 3) in a separate experiment. After 10 days of culture (i.e. 7 days under flow conditions) the microfluidic chips were opened, and cells were fixed on the membrane. The monolayer of cells was sequentially fixed with 4% formaldehyde at room temperature for 10 min and rinsed with PBS. Cells were then permeabilized with 0.25% Triton X100 in PBS for 10 min, rinsed with PBS and blocked with 1% acetylated bovine serum albumin in PBS for 30 min. Tight junctions were stained with 10 μg mL−1 of the conjugated antibody ZO-1/TJP1-Alexa Fluor 594 (Invitrogen, Waltham, MA). The nuclei were stained with 5 μg mL−1 of DAPI (Invitrogen, Waltham, MA) and 4 U mL−1 of Phalloidin Alexa Fluor 488 (Life technologies) was used to stain actin filaments (i.e. cytoskeleton). The incubation time for all stainings was 10 min. Each membrane was then cut out and placed between two cover slips separated by a spacer (0.12 mm depth × 20 mm diameter). Anti-fading mounting medium was dropped on the membrane. The cells cultured on Transwell membranes for 21 days were stained with the same methods and conditions. The stained monolayers of cells were analysed using a confocal microscope (LSM 510 UVMETA; Carl Zeiss, Germany). Samples were excited with 405, 488 and 543 nm lasers. Multi-tracked images were captured to avoid bleed through. The used pinholes were in the range of 148–152 μm at magnification 40×.The gain and offset for the different channels were kept constant during the entire experiment.
2.5. Compounds
The dioxin mixtures were obtained from Cambridge Isotope Laboratories, Inc. (Tewksbury, USA) and contained 10 PCDF congeners: 2,3,7,8-TCDF, 1,2,3,7,8-PeCDF, 2,3,4,7,8-PeCDF, 1,2,3,4,7,8-HxCDF, 1,2,3,6,7,8-HxCDF, 2,3,4,6,7,8-HxCDF, 1,2,3,7,8,9-HxCDF, 1,2,3,4,6,7,8-HpCDF, 1,2,3,4,7,8,9-HpCDF and OCDF, and 7 PCDD congeners: 2,3,7,8-TCDD, 1,2,3,7,8-PeCDD, 1,2,3,4,7,8-HxCDD, 1,2,3,6,7,8-HxCDD, 1,2,3,7,8,9-HxCDD, 1,2,3,4,6,7,8-HpCDD and OCDD.
2.6. Cell viability
To exclude cytotoxicity of the dioxin mixture at the concentration used for the transport studies, a WST-1 assay was performed on Caco-2 cells. The WST-1 assay quantifies cellular mitochondrial activity, which directly correlates to cell viability and thus cytotoxicity. For this, cells were seeded in 96 well plates at a density of 50
000 cells per cm2 and were incubated overnight and were subsequently exposed to the dioxin mixture in DMEM+ at various concentration ranging from 0.025 to 2.0 ng mL−1, for 24 h. After exposure, the cells were washed with Hank's Balanced Salt Solution (HBSS) and incubated with 10 μL WST-1 reagent (Roche Diagnostics GmbH, Mannheim, Germany) for 3 h. The plate was read at 440 nm and 640 nm using a microplate reader (BioTek, Winooski, VT). The background signal at 640 nm, which is directly correlated with the cell quantity, was subtracted from the reaction signal at 440 nm.
2.7. Dioxin mixture transport experiments
In the dynamic gut-on-a-chip model, cells were cultured for 10 days, of which 7 days under continuous flow of 25 μL h−1. At day 10, DMEM+ containing 0.2 ng mL−1 of each congener of dioxin (in 1% DMSO) was pumped into the apical channel, and DMEM+ without the dioxin mixture was perfused into the basolateral channel. After 24 h, a total of 600 μL of eluent from each compartment was collected for further analysis.
In the Transwell model, cells were cultured for 21 days, and subsequently exposed to 600 μL DMEM+ containing 0.2 ng mL−1 of the dioxin mixture (in 1% DMSO) in the apical compartment. The basolateral compartment was filled with 600 μL DMEM+. After 24 h, all DMEM+ was collected from both compartments.
In both the chip and Transwell models, cells were collected at the end of the exposure to determine compound accumulation in the cells. For this, cells were trypsinized with 0.025% Trypsin/EDTA, rinsed with HBSS and lysed by three freeze–thaw cycles. To establish a complete mass balance, the fraction of absorbed dioxins on membranes, tubing and culture chambers after exposure was collected by rinsing all compartments with 200 μL hexane. The experiment was performed in triplicate, all collected samples were analysed together using GC-HRMS.
2.8. GC-HRMS analysis
Samples were analysed by GC-HRMS using previously described methods.24 In short, prior to the extraction, the samples were spiked with 13C-isotope labelled internal standards (50 pg of each of the 7 PCDDs and of 9 out of 10 PCDFs (except 2,3,4,6,7,8-HxCDF)). Consequently, 1 mL of methanol was added and extracted with once 4 mL hexane and twice with 5 mL hexane. The hexane phases were merged and the samples were purified as described in earlier studies.24 Using an automated clean-up (PowerPrep system, Fluid Management Systems, Waltham, USA), extracts were purified on an acid silica column, a neutral silica column, a basic alumina column and an activated carbon/Celite column. For the elution of the columns, custom made solvents and mixtures were used, respectively being hexane, hexane/dichloromethane (1
:
1, v/v), ethyl acetate/toluene (1
:
1, v/v) and toluene. The volume of the final extract was reduced to 0.5 mL using an automated evaporation system with a fixed endpoint of 0.5 mL. The recovery standards 13C-labelled 1,2,3,4-TCDD and 2,3,4,6,7,8-HxCDF, 100 pg, were added for the analysis of PCDD/F, after which the volume of the extracts were again reduced to 0.5 mL. PCDD/F analysis was performed by GC/HRMS using an Agilent (Wilmington, USA) 6890 Series gas chromatograph and an AutoSpec Ultima high resolution mass spectrometer (Waters, Milford, USA) operated at a resolution of 10
000 (10% peak valley). The GC column was a DB5 MS (60 m, 0.25 mm i.d., 0.25 μm; J&W, Folson, USA). The mass spectrometer was operated in electron impact ionization mode, using selected-ion monitoring. A large volume injector (LVI) was used to inject 100 μL of the extract containing PCDD/Fs on the GC. The results were corrected for recovery of the 13C-labelled internal standards and the performance was checked through an in-house reference sample sunflower oil spiked at approximately 1 pg TEQ g−1 fat PCDD/F, 0.8 pg TEQ g−1 fat dl-PCBs and 15 ng g−1 fat non-dioxin like-PCBs. The performance of the methods is regularly checked by participation in PT-tests (EURL dioxins and PCBs twice a year, Folkehelse Institute, once a year), showing good performance.
2.9. Quantitative structure–property relationship modelling
Quantitative structure–property relationship (QSPR) modelling is based on the assumption that the structure of compound (i.e. geometry, mass, surface) is responsible for its chemical, physical and biological properties.27 For the QSPR modelling data were logarithmized to make them more linear and sorted by increasing values. To be able to validate the QSPR models the data were split into two sets: a training set (to calibrate of model equations), and a validation set (used for verifying the predictive ability of the developed models). For this we used the “Z:1” algorithm, which places every Zth compound in the validation set (objects from this set are marked as #2) and all the remaining objects in the training set (those objects are marked as #1). We took Z = 5 for cellular uptake and association data and Z = 4 for transport data.
Molecular structures of the PCDD and PCDF congeners were build using the ConGENER software.28 The molecular geometries energy was optimized in Gaussian package (version 09, Gaussian Inc, Wallingford, Connecticut USA) with the B3LYP/6-311+G* density functional method as described before.29,30 After optimization the molecular structures were used to calculate the molecular descriptors, in order to obtain a numerical representation of the compound structure. To this end, we used DRAGON software (version 6.0),31 which allowed us to obtain a set of 2622 molecular descriptors.
Based on the experimental data and molecular descriptors we calibrated the models equations using a Multiple Linear Regression (MLR) method.32 In order to select from such a large descriptors set the models that best correlate with the biological endpoint we used genetic algorithm (GA)33 implemented in the QSARINS software (version 2.2.2).34 The genetic algorithm is able to search for the best solutions from a large number of possibilities by a maximizing/minimizing fitness function. The procedure of GA was inspired by evolution mechanism, where the data respond to chromosomes and genes.35 In our study GA was applied with the following parameters: the population size: 200, the mutation rate: 20%. Due to the small number of compounds in the models training sets we chose 2 descriptors for each model, to avoid their overfitting.36,37 The chosen descriptors are presented in the Table 4. We selected four autocorrelation descriptors: ATSC3v and GATS5v for uptake and/or cellular association models, ATS5m and MATS5m for transport models. In general autocorrelation descriptors are based on the autocorrelation function ACk presented in the eqn (1).
|
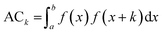 | (1) |
where
f(
x) is a function of the variable
x,
k is the lag representing an interval of
x and
a,
b define the total studied interval of the function.
37
To meet the requirements of QSPR models defined by the Organization for Economic Co-operation and Development,37 we carried out a two-stage model validation of each model: for the internal validation we chose the leave-one-out method (cross-validation),38 and for the external validation we used the validation set of data.
After the validation process we examined the applicability domain (AD) of our models. AD is a theoretical space determined by values of molecular descriptors, and so resulting from structural similarity of the compounds, in which the predictions are reliable. For this purpose we calculated leverage values (h) and the standardized residual values. The h values that defines the similarity of ith compound to the training set were calculated according to the eqn (2), where xi is a vector containing descriptors for ith compound and X is a matrix containing descriptors for every compound from the training set.
When the h value for a compound is higher than the critical value h* (calculated based on the eqn (3)), then the model predicted the endpoint value for this compound by extrapolation.
where
p is the number of variables in model plus one,
n is the number of the compounds in the training set.
39
We verified the fitting of the models by determination coefficient (R2) and root mean square error of calibration (RMSEC). Their robustness and stability was evaluated by the cross-validation coefficient (QCV2) and the root mean square error of cross-validation (RMSECV). The predicting capabilities of the models were examined by external validation coefficient (QEXT2) and root mean square error of prediction (RMSEEXT).40
2.10. Statistical analysis
SPSS (IBM) was used for all statistical evaluations. The cellular accumulation and transport data was evaluated using an independent paired t-test for the dioxin mixture transport experiment and a one-way ANOVA with Dunnett's post hoc test was used for the cell viability test. A p-value of ≤0.05 was considered significant.
3. Results and discussion
3.1. Microfluidic model development for lipophilic compounds
Dioxins are highly lipophilic, and thus tend to adsorb to surfaces of materials used in experiments depending on their chemical composition and their subsequent compound–material interactions.41,42 Polydimethylsiloxane (PDMS) is often used to create microfluidic devices because of its low cost, ease of use, high compliance, microscope compatibility and fast fabrication properties.43–45 However, PDMS has a number of important limitations regarding its use in biological studies.46 One of those limitations is adsorption of various molecules by PDMS because of its permeable and hydrophobic properties.47 It has been reported that PDMS based material adsorbed more small-molecules and hydrophobic compounds compared to polystyrene based material,48,49 and glass,50 and thus dramatically affecting free compound concentrations.49 Obviously, the degree of adsorption relies on lipophilic properties of compounds.50,51 While it has been shown that continuous rinsing with buffer might result in desorption of compounds from PDMS,50 it is clear that PDMS might not be the ideal material for highly lipophilic compounds like dioxins. Therefore, in this study, glass-based chips were used, to reduce the effect of surface adsorption as much as possible. Selection of the optimal type of tubing for the compounds of interest in a microfluidic system is also an essential element of the microfluidic model development, especially since the (relative) surface areas of the capillary tubing is large. Therefore, the concentration of the dioxin mixture in cell culture medium was determined after use in different materials of syringes and tubing (i.e. glass vs. polypropylene syringes and ethylene tetrafluoroethylene (ETFE), stainless steel and Teflon tubing), under the conditions as used in the final experiments. The fraction of dioxin mixture adsorbed onto the material surface after incubation was determined by assessing the amount of dioxins in the cell culture medium expressed in BEQ using the dioxin CALUX bioassay, as a cost effective measurement technique. Highest residual amounts of the dioxin mixture in cell culture media were detected when using glass syringes and Teflon tubes, about 60% higher compared to the set-up with the lowest yield using polypropylene syringes and ETFE tubing (Table 2). Therefore Teflon tubing is used in the final experiments.
Table 2 Effect of different tubing and syringe material of the microfluidic systems on recovery of the dioxin mixture measured by CALUX (n = 2)
Syringes and tubing |
Dioxin concentration (ng BEQ per ml) |
Recoverya (%) |
The initial concentration of Dioxin in DMEM+ is 0.328 ng BEQ per ml. |
Polypropylene + ETFE |
0.085 ± 0.005 |
26 ± 1 |
Glass + ETFE |
0.199 ± 0.006 |
61 ± 2 |
Glass + steel |
0.212 ± 0.012 |
65 ± 4 |
Glass + Teflon |
0.219 ± 0.003 |
67 ± 1 |
Dioxin adsorption to the microfluidic system was also assessed in the exposure/translocation experiment by GC-HRMS. For this, the system was rinsed with hexane after the exposure and the amount of dioxins in this fraction was determined with GC-HRMS and compared to the exposure mixture. On average, hexane rinses of the chip compartments after incubation with the dioxin mixture contained 3% of the total amount of dioxins as present in the exposure mixture in both systems, indicating low adsorption (see ESI Table 1†). This information, together with an average measured mass balance of 93%, (the sum of apical, basolateral and cellular fractions, as discussed in detail later) shows a good recovery in the chip system.
3.2. Morphology of intestinal barriers
Monolayer integrity of Caco-2 cells grown under dynamic flow and static conditions was evaluated using fluorescence confocal microscopy. Representative images are shown in Fig. 3. In the chip the Caco-2 cells were grown using a continuous flow of 25 μL h−1 for 7 days. Cells cultured under continuous flow have been described to form intact and polarized monolayers faster compared to Caco-2 cells cultured using traditional static conditions.6 This is confirmed in the present study by showing complete and polarized monolayers of cells at day 10 (i.e. 7 days of flow) in the chip model. For comparative analysis Caco-2 cells were grown for 21 days under static conditions in Transwells. Cells grown under continuous flow showed a comparable monolayer formation to cells grown without continuous flow, as can be seen from the tight junction immunofluorescence staining (Fig. 3A and B). Cell polarisation was visualized using immunofluorescence staining of actin filaments, nuclei, and tight junctions (Fig. 3C–H). Core bundles of actin filaments in the microvilli were seen in cells grown under both conditions. After cell polarisation, the cells grown without continuous flow exhibited densely packed actin filaments on the apical side in the microvilli of the cells, while cells grown under dynamic conditions also exhibited pronounced actin filaments on the basolateral side (Fig. 3E and F). Likely this is caused by the shear stress (0.0001–0.0005 dyne per cm2), induced by the continuous flow, as shown before for endothelial cells exposed to a shear stress of 15 dyne per cm2,52 and placenta (i.e. BeWo) cells exposed to shear stresses ranging from 0.001 to 0.12 dyne per cm2.53 In addition, we observed that cells grown under continuous flow seem to be larger compared to the cells grown without continuous flow. Likely this can also be explained by the shear stress exposure. Since actin filaments are associated with cell adhesion and mechanics, remodelling of actin filaments might alter cell spread, migration, elongation, or enlargement of the cells.52,53 While the thickness of the monolayers of cells grown under both conditions was comparable (10 μm height; Fig. 3G and H), the continuous flow seemed to induce a more pronounced 3D structure, which has more prominently been shown in Caco-2 containing chip models that also employ stretchable membranes (cyclic strain of 0.15 Hz frequency together with a shear stress of 0.02 dyne per cm2).6
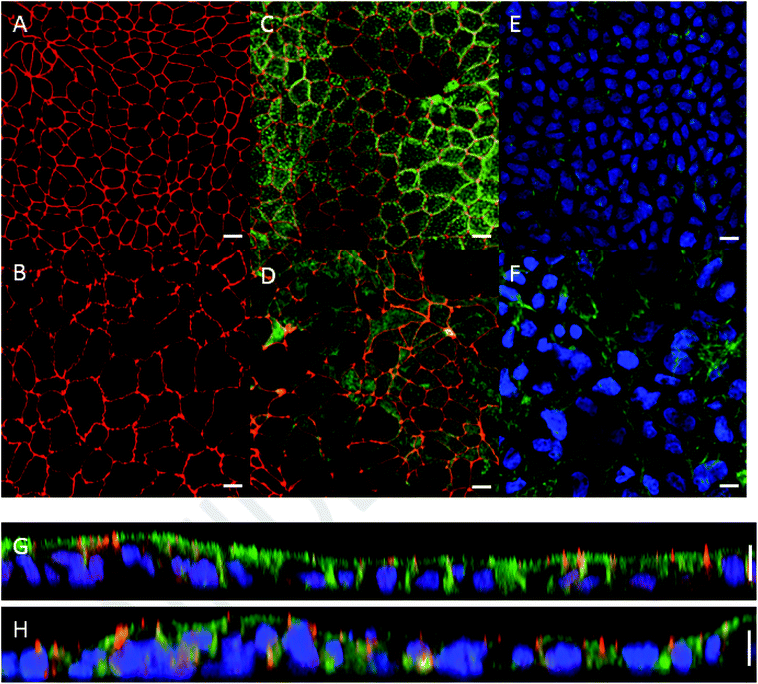 |
| Fig. 3 Morphology of Caco-2 cells cultured in a Transwell for 21 days versus in chip for 10 days (of which 7 days under a continuous flow of 25 μL h−1) visualized by confocal microscopy. Tight junctions of cells cultured in (A) a Transwell and in (B) a chip were stained for ZO-1/TJP1 (red). Actin filaments on the apical side of the cells, stained by Phalloidin (green), and tight junctions of cells were visualized on merged images in (C) a Transwell and (D) a chip. Merged images showing nuclei (DAPI; blue) and actin filaments on the basolateral side of cells cultured in (E) a Transwell, and (F) a chip. Note the more pronounced expression of actin in the chip. Vertical cross-section of the cell monolayer in (G) a Transwell and (H) a chip. The scale bar represents 10 μm. | |
3.3. Comparative cellular uptake and absorption, and transport of dioxins
The human gut epithelium acts as an important barrier in defence to protect the body from exogenous substances that can be present in our food.54,55 To further optimize and implement dynamic gut-on-a-chip models, we determined the transport of 17 individual dioxin congeners across a monolayer of Caco-2 cells under dynamic and static culturing and exposure conditions.
Transport studies can only be reliably performed using dioxin concentrations that do not affect the viability of cells used in the experiment. A WST-1 assay was used to select a non-toxic dioxin mixture concentration. As can be seen in Fig. 4, decreased cell viability was only observed following an exposure to ≥0.6 ng mL−1 dioxin mixture for 24 h (P < 0.05, one way ANOVA). For the cellular association and transport studies an exposure concentration of 0.2 ng mL−1 was used.
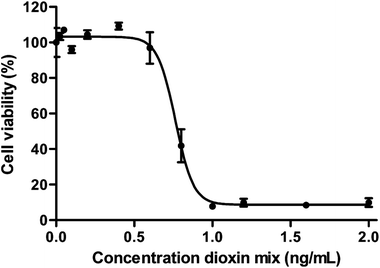 |
| Fig. 4 Cytotoxicity of the dioxin mixture in Caco-2 cells after 24 h exposure given as a percentage of viable cells (±SEM) of the total number of cells (n = 3). | |
After 24 hours of exposure the cells were harvested from the chip and Transwell membranes and the concentration of individual congeners in these fractions, as well as in the medium fractions from the apical and basolateral compartments of both the chip and Transwell, was determined using GC-HRMS. Also, hexane rinses of both the chip and Transwell were evaluated. The so-called mass balance was determined (Table 3), which is the sum of the amount of congeners detected in the AP and BL compartments and the cellular fraction. This indicated mass balances ranging from 75 to 99%, concluding the method and experimental setup suitable for dioxin transport studies.
Table 3 Mass balance from dioxin transport experiments analysed by GC-HRMS (n = 3)
Components |
Mass balance (%) |
Chip |
Transwell |
Congener |
2,3,7,8-TCDF |
82 ± 5 |
75 ± 1 |
1,2,3,7,8-PeCDF |
88 ± 5 |
86 ± 1 |
2,3,4,7,8-PeCDF |
93 ± 7 |
82 ± 2 |
1,2,3,4,7,8-HxCDF |
97 ± 7 |
85 ± 2 |
1,2,3,6,7,8-HxCDF |
94 ± 5 |
93 ± 1 |
2,3,4,6,7,8-HxCDF |
97 ± 7 |
87 ± 2 |
1,2,3,7,8,9-HxCDF |
91 ± 6 |
84 ± 2 |
1,2,3,4,6,7,8-HpCDF |
97 ± 5 |
93 ± 1 |
1,2,3,4,7,8,9-HpCDF |
97 ± 7 |
83 ± 2 |
OCDF |
96 ± 8 |
79 ± 2 |
2,3,7,8-TCDD |
85 ± 5 |
81 ± 2 |
1,2,3,7,8-PeCDD |
92 ± 6 |
85 ± 2 |
1,2,3,4,7,8-HxCDD |
98 ± 8 |
84 ± 2 |
1,2,3,6,7,8-HxCDD |
92 ± 5 |
92 ± 1 |
1,2,3,7,8,9-HxCDD |
93 ± 5 |
93 ± 1 |
1,2,3,4,6,7,8-HpCDD |
97 ± 7 |
83 ± 2 |
OCDD |
100 ± 8 |
82 ± 2 |
Transport of dioxin congeners across the two barrier models was low and ranged from 0.6% to 3.3% for OCDF and 1,2,3,7,8-PeCDF respectively in the dynamic model, and ranged from 0.2% to 4.4% in the static model for OCDF and 1,2,3,7,8-PeCDF respectively (Fig. 5A). Comparison of the transport and cellular association of the individual congeners between both models showed no significant differences. In addition, a similar trend in congener transport was observed (R2 = 0.96) between the two models (Fig. 5C). The larger error bars in the chip data compared to the Transwell data could be explained by the more complicated (technical) setup of the chip experiments compared to Transwells. In an earlier study the transport across a monolayer of Caco-2 cells of only 2,3,7,8-TCDD has been studied.56 The authors quantified the TCDD concentration using a luciferase HepG2 cell assay. They found that 15% of TCDD was transported across the Caco-2 cell monolayer in 24 h.56 This is higher than the 3% transported 2,3,7,8-TCDD observed by us for both models, which might be explained by differences in exposure concentration. In their study, a 10 fold higher concentration of 2.5 nM of 2,3,7,8-TCDD was used, whereas we used 0.26 nM of 2,3,7,8-TCDD as part of a mixture of dioxins.
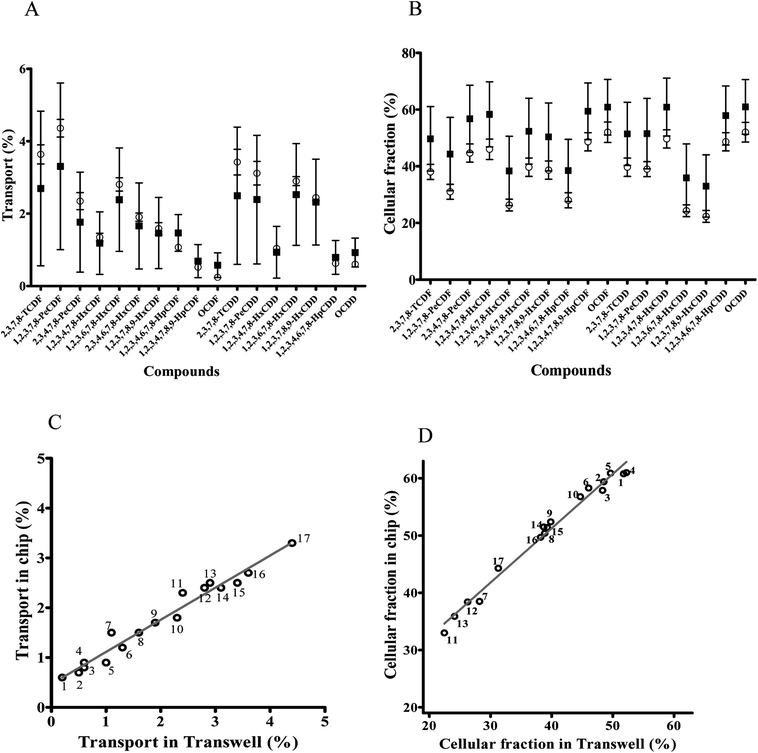 |
| Fig. 5 Comparison of dioxin congeners in the cellular fraction and transported over the cell barrier between a dynamic ( ) versus a static ( ) model. (A) Transport of dioxin congeners through the monolayer of cells cultured in chips and Transwells given as a percentage (±SEM) of dioxin congeners in the basal side compared to the total exposed amount. (B) Fraction of dioxin congeners in the cell fraction given as a percentage (±SEM) of dioxin congeners in the cellular fraction compared to the total exposed amount. (C) Correlation between transported dioxin congeners in the dynamic versus static model. (D) Correlation between dioxin congeners in the cellular fraction in the dynamic versus static model, 1 = OCDF; 2 = 1,2,3,4,7,8,9-HpCDF; 3 = 1,2,3,4,6,7,8-HpCDD; 4 = OCDD; 5 = 1,2,3,4,7,8-HxCDD; 6 = 1,2,3,4,7,8-HxCDF; 7 = 1,2,3,4,6,7,8-HpCDF; 8 = 1,2,3,7,8,9-HxCDF; 9 = 2,3,4,6,7,8-HxCDF; 10 = 2,3,4,7,8-PeCDF; 11 = 1,2,3,7,8,9-HxCDD; 12 = 1,2,3,6,7,8-HxCDF; 13 = 1,2,3,6,7,8-HxCDD; 14 = 1,2,3,7,8-PeCDD; 15 = 2,3,7,8-TCDD; 16 = 2,3,7,8-TCDF; 17 = 1,2,3,7,8-PeCDF. | |
Upon 24 h of exposure to the mixture of dioxins the cellular fraction contained relative amount of each congener ranging from 33% for 1,2,3,7,8,9-HxCDD to 61% for OCDD of the total exposure concentration (based on a mass balance calculation) in the dynamic model and relative amount ranging from 22.5% for 1,2,3,7,8,9-HxCDD to 51.8% for OCDD in the static model (Fig. 5B). While the fraction of the dioxin mixture in the cellular fraction seems to be increased in cells cultured under dynamic conditions this was not significantly different. Cellular association and accumulation of dioxins is likely caused by their lipophilicity as reported in the previous studies.57,58 Under both culture conditions individual congeners were present in the cellular fraction in a comparable pattern, as a strong correlation between the chip and Transwell cellular amount was observed for the whole series of test compounds (R2 = 0.98; Fig. 5D).
Dioxins have been investigated extensively in vitro with most studies focusing on their potential toxicity and metabolism, focussing specifically on 2,3,7,8-TCDD-aryl hydrocarbon receptor (AhR) interactions. 2,3,7,8-TCDD can induce expression of cytochrome P450 1A1 (CYP1A1), a phase I drug metabolism enzyme, and UDP-glucuronosyltransferase (UGT) isoforms UGT1A6 and UGT1A9, phase II drug metabolism enzymes, in Caco-2 cells.59–62 On the contrary, intestinal uptake and transport of dioxins has only very limitedly been studied in vitro before. We identified only one study that reports cellular association, in this study only a single dioxin congener has been used. For 2,3,7,8-TCDD, a steady-state amount of approximately 13% cellular association has been reported following 90 minutes of exposure to 3.4 × 10−2 μCi mL−1 U-14C-TCDD and less than 1.5% of apical concentration was transported to the basal compartment.63 Our data illustrates an amount of 2,3,7,8-TCDD in the cellular fraction of approximately 39 and 56% in Transwell and chip model, respectively. We observed that the dioxin mixture was more retained in the cellular fraction than passed through the monolayer to the basal side under both experimental conditions. This can be explained by the high lipophilicity of the dioxin congeners and its influence on their partition coefficient, so they are likely to remain in phospholipid bilayers and only slowly diffuse across it.64 This has been reported also for polychlorinated biphenyls, PCB#52, #118, #153 and #180, which have an extremely high hydrophobicity (log
P > 5).65
3.4. QSPR modelling for PCDDs and PCDFs
To further explore the relationship between the physicochemical properties of the used dioxins and their uptake and/or cellular association we used QSPR modelling. QSPR modelling is used to identify correlations between structure and biological effects. In order to build a QSPR model experimental (chip) data is necessary, once a QSPR model is validated it could be used for prioritization of (new) specific compounds for (on-chip) testing based on their molecular structure. Combinations of in vitro studies and computational approaches have been identified as a powerful approach to develop alternative testing strategies without the use of animals.66,67 For each model equation we chose 2 descriptors, to avoid their overfitting.37 The chosen descriptors are presented in the Table 4.
Table 4 Molecular descriptor pairs for the developed QSPR models
Pair of models |
Descriptors |
|
Cell QSPR models |
ATSC3v |
Centred Broto–Moreau autocorrelation of lag 3 weighted by van der Waals volume |
GATS5v |
Geary autocorrelation of lag 5 weighted by van der Waals volume |
Transport QSPR models |
ATS5m |
Centred Broto–Moreau autocorrelation of lag 5 weighted by mass |
MATS5m |
Moran autocorrelation of lag 5 weighted by mass |
We have developed four statistically significant QSPR models describing relationship between the chemical structures of PCDDs and PCDFs and their uptake and/or cellular association and transport across the monolayer of cells. In the process of model calibration we obtained the following eqn (3)–(6):
|
Model 1 (Uptake-chip): Y = −9.9324 ATSC3v −52.8616 GATS5v + 121.637
| (4) |
|
Model 2 (Uptake-Transwells): Y = −12.7950 ATSC3v − 57.3662 GATS5v + 118.7720
| (5) |
|
Model 3 (Transport-chip): log Y = −1.0760 ATS5m − 0.6038 MATS5m + 4.4399
| (6) |
|
Model 4 (Transport-Transwells): log Y = −1.7296 ATS5m − 0.5969 MATS5m + 7.0661
| (7) |
The values of determination coefficients (R2), internal and external validation coefficients (QCV2 and Q2EXT) and root mean square errors (RMSEC, RMSECV, RMSEEXT) presented in Table 5 indicate good quality, stability and predictive capabilities of the models.
Table 5 Parameters of quality evaluation for developed models
|
R2 |
RMSEC |
QCV2 |
RMSECV |
QEXT2 |
RMSEEXT |
Model 1 |
0.83 |
3.83 |
0.73 |
4.83 |
0.87 |
3.11 |
Model 2 |
0.86 |
3.60 |
0.80 |
4.39 |
0.72 |
4.31 |
Model 3 |
0.91 |
0.07 |
0.86 |
0.08 |
0.87 |
0.08 |
Model 4 |
0.92 |
0.01 |
0.14 |
0.14 |
0.96 |
0.07 |
This evaluation is additionally confirmed by high correlation between experimental and predicted values of endpoints presented at the Fig. 6.
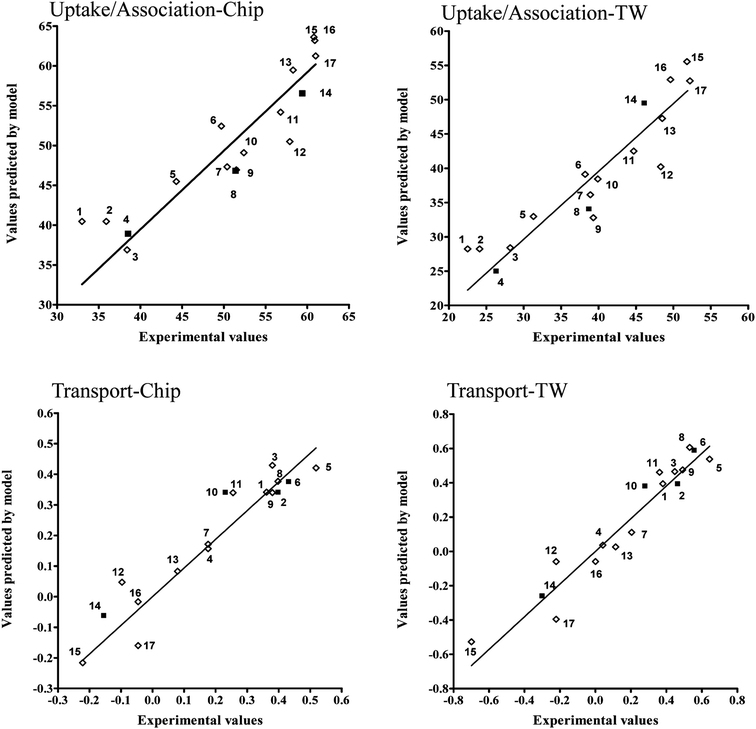 |
| Fig. 6 Experimental and predicted values of endpoint for each developed model, the training (◊) and validation set (■). 1 = 1,2,3,7,8,9-HxCDD; 2 = 1,2,3,6,7,8-HxCDD; 3 = 1,2,3,6,7,8-HxCDF; 4 = 1,2,3,4,6,7,8-HpCDF; 5 = 1,2,3,7,8-PeCDF; 6 = 2,3,7,8-TCDF; 7 = 1,2,3,7,8,9-HxCDF; 8 = 2,3,7,8-TCDD; 9 = 1,2,3,7,8-PeCDD; 10 = 2,3,4,6,7,8-HxCDF; 11 = 2,3,4,7,8-PeCDF; 12 = 1,2,3,4,6,7,8-HpCDD; 13 = 1,2,3,4,7,8-HxCDF; 14 = 1,2,3,4,7,8,9-HpCDF; 15 = OCDF; 16 = 1,2,3,4,7,8-HxCDD; 17 = OCDD. | |
To verify models applicability domains (AD) we applied Williams plots11 (values of standardized residuals versus the leverage values). AD is limited by the critical values of standardized residuals (three standard deviation units-3σ) and the critical leverage value (h*). The developed Williams plots are presented in Fig. 7.
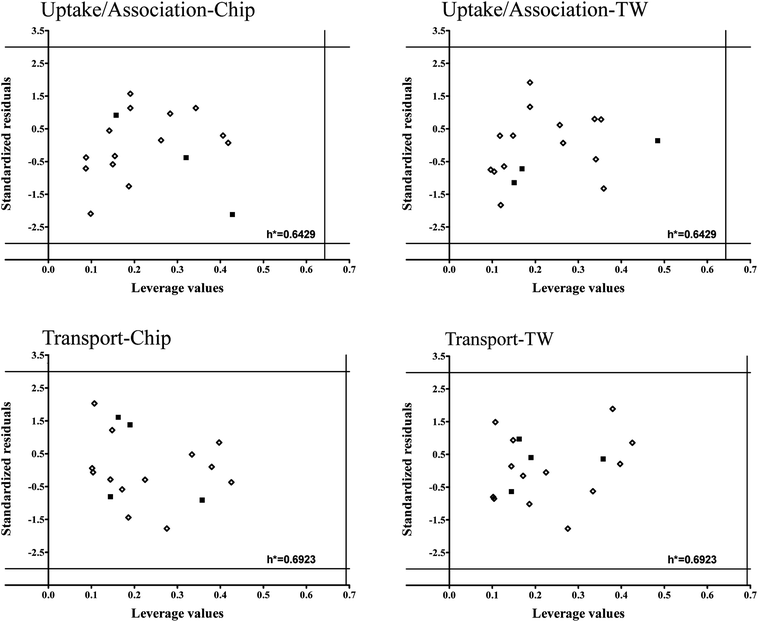 |
| Fig. 7 Williams plots for developed models. The lines on the plot (±3σ and h*) define the applicability domain of the model, the training (◊) and validation set (■). | |
All the compounds are situated in the range of ±3 standard deviations from 0 and none of them exceeded the critical leverage value (h*). It means that all the compounds used to develop the models are structurally similar and the uncertainly of model predictions for them is acceptable. Moreover, it confirms the assumption that PCDDs and PCDFs may belong to the common AD.
Interpretation of QSPR models can be used to hypothesize on mechanism of cellular uptake (and/or association) and subsequent transport of dioxins across the monolayer of cells. Molecular descriptors chosen for the model equation indicate which structural features determine the values of the endpoint. For our models we selected four autocorrelation descriptors: ATSC3v and GATS5v for uptake (and/or association) models, ATS5m and MATS5m for transport models. Autocorrelation descriptors can encode not only the structure of the molecule but also physico-chemical properties attributed to atoms, therefore descriptors of this type are effectively used for modelling compounds' interactions with membranes or their cytotoxicity.68–71 For the cellular association models we developed (Model 1 and 2) there is a clear relationship between values of the ATSC3v descriptor and the number of chlorine atoms in the compound. These quantities are inversely proportional, but this descriptor is preceded in model equations by negatives coefficients. Increasing number of chlorine atoms in the molecule is therefore associated with increasing value of PCDDs and PCDSs cellular association. The second descriptor used in uptake models-GATS5v-is connected to the substitution pattern. It takes on higher values when the distribution of chlorine atoms in the molecule is less symmetrical. Thus the GATS5v descriptor describes the distribution of the charge in the compound. This means that the uptake of PCDDs and PCDFs is higher in the case of less symmetrical molecules. This conclusion is also confirmed by the fact that GATS5v descriptor assumes higher values for PCDFs compared to PCDDs.
The results are relatively similar in case of models developed for the transport of PCDDs and PCDFs: first selected descriptor (ATS5m) is also inversely proportional to the number of chlorine atoms in a molecule. It shows, that compounds with higher number of chlorine atoms are more easily transported. The values of the second descriptor, MATS5m, depend on the substitution pattern.
Conclusion
Here we have implemented a dynamic flow gut-on-a-chip model that can be used to study the transport and the cellular accumulation of lipophilic compounds like dioxins. The experimental conditions were optimized to avoid uncontrollable adsorption. By using a very sensitive, dioxin congener specific GC-HRMS detection method, we show that the transport and cellular accumulation profile of dioxins of Caco-2 cells grown under continuous flow and static conditions is comparable. Physicochemical properties, of the dioxin congeners allowed us to predict their transport profiles in vitro.
Caco-2 cell models have been used to study the absorption and local effects of a variety of compounds, and are recognized as usefully predictive in vitro models for the study of passage of substances through the gut wall. However, their conventional culture methods provide some disadvantages, for example, long term cultures time (∼3 weeks), not fully mimicking in vivo conditions and inability to do on-line measurement. Therefore, a gut-on-a-chip system has been developed and proposed to be used as an in vitro alternative model that recapitulates not only in vivo fluid flows, but also the structure, transport, of the gut epithelium.
The results illustrate that Caco-2 cells cultured in a dynamic system for 10 days shows similar polarisation and morphological properties as cells cultured for 21 days in a static system. Furthermore, the gut-on-a-chip system also showed transport properties similar to the static system indicating its applicability for transport studies. Thus it now is possible to use an advanced gut-on-a-chip models next to the traditional Transwell model as a screening assay to study effects of compounds on dioxin absorption in order to prevent dioxin ingestion in human. Clearly, there are remaining technical challenges to be addressed in working with organ-on-chips, to allow an easier implementation of these models into routine testing. Precisely controlled injection systems, with flow and pressure control, and easy to use inert chip materials, are the most important technical challenges.72 This is the first report comparing the transport of 17 dioxin congener using an in vitro system and revealing their structure–transport relationship. Interestingly, using quantitative structure–property relationship modelling we have been able to reveal the relationship between the degree of chlorination and the chlorination patterning on the cellular association and transport of the individual dioxin congeners across a monolayer of Caco-2 cells.
The implemented gut-on-a-chip system can be used for dioxin transport screening which might be useful for other lipophilic compound study as well. Moreover, the microfluidic organ-on-a-chip model can be coupled to downstream analysis systems, such as mass spectrometry to measure the transported amount of drugs or metabolites in the system in real-time.73–75
Conflicts of interest
There are no conflicts of interest to declare.
Acknowledgements
This research was financed by the Dutch Ministry of Agriculture, Nature and Food Quality and K. K. is supported by the Royal Thai government Scholarship.
References
- C. Pereira, J. Costa, B. Sarmento and F. Araújo, in Concepts and Models for Drug Permeability Studies, Woodhead Publishing, 2016, DOI:10.1016/B978-0-08-100094-6.00005-5, pp. 57–81
. - R. B. van Breemen and Y. Li, Expert Opin. Drug Metab. Toxicol., 2005, 1, 175–185 CrossRef PubMed
. - A. M. Marino, M. Yarde, H. Patel, S. Chong and P. V. Balimane, International journal of pharmaceutics, 2005, 297, 235–241 CrossRef PubMed
. - M. J. Briske-Anderson, J. W. Finley and S. M. Newman, Proc. Soc. Exp. Biol. Med., 1997, 214, 248–257 CrossRef PubMed
. - H. J. Kim and D. E. Ingber, Integr. Biol., 2013, 5, 1130–1140 RSC
. - H. J. Kim, D. Huh, G. Hamilton and D. E. Ingber, Lab Chip, 2012, 12, 2165–2174 RSC
. - M. Gori, M. C. Simonelli, S. M. Giannitelli, L. Businaro, M. Trombetta and A. Rainer, PLoS One, 2016, 11, e0159729 CrossRef PubMed
. - E. W. Esch, A. Bahinski and D. Huh, Nat. Rev. Drug Discovery, 2015, 14, 248–260 CrossRef PubMed
. - K. J. Jang and K. Y. Suh, Lab Chip, 2010, 10, 36–42 RSC
. - N. S. Bhise, J. Ribas, V. Manoharan, Y. S. Zhang, A. Polini, S. Massa, M. R. Dokmeci and A. Khademhosseini, J. Controlled Release, 2014, 190, 82–93 CrossRef PubMed
. - J. D. Wang, E. Khafagy, K. Khanafer, S. Takayama and M. E. H. Elsayed, Mol. Pharmaceutics, 2016, 13, 895–906 CrossRef PubMed
. - J. H. Yeon and J. K. Park, Anal. Chem., 2009, 81, 1944–1951 CrossRef PubMed
. - G. J. Mahler, M. B. Esch, R. P. Glahn and M. L. Shuler, Biotechnol. Bioeng., 2009, 104, 193–205 CrossRef PubMed
. - W. H. Organization, Exposure to Dioxins and Dioxin-like Substances: A Major Public Health Concern, World Health Organization, Geneva, 2010 Search PubMed
. - E. F. S. Authority, Dioxin and PCBs, (accessed 30 January 2018) Search PubMed.
- M. O. Milbrath, Y. Wenger, C. W. Chang, C. Emond, D. Garabrant, B. W. Gillespie and O. Jolliet, Environ. Health Perspect., 2009, 117, 417–425 CrossRef PubMed
. - G. Charnley and J. Doull, Food Chem. Toxicol., 2005, 43, 671–679 CrossRef PubMed
. - J. K. Huwe and G. L. Larsen, Environ. Sci. Technol., 2005, 39, 5606–5611 CrossRef PubMed
. - A. K. Liem, P. Furst and C. Rappe, Food Addit. Contam., 2000, 17, 241–259 CrossRef PubMed
. - H. Poiger and C. Schlatter, Chemosphere, 1986, 15, 1489–1494 CrossRef
. - K. Kitamura, M. Nagahashi, M. Sunaga, S. Watanabe and M. Nagao, J. Health Sci., 2001, 47, 591–59 Search PubMed
. - E. F. S. Authority, EFSA J., 2012, 10, 2832 Search PubMed
. - M. Vandenberg, J. Dejongh, H. Poiger and J. R. Olson, Crit. Rev. Toxicol., 1994, 24, 1–74 CrossRef PubMed
. - R. Hoogenboom, G. Ten Dam, M. van Bruggen, S. M. F. Jeurissen, S. P. J. van Leeuwen, R. M. C. Theelen and M. J. Zeilmaker, Chemosphere, 2016, 150, 311–319 CrossRef PubMed
. - S. Kim, P. A. Thiessen, E.
E. Bolton, J. Chen, G. Fu, A. Gindulyte, L. Y. Han, J. E. He, S. Q. He, B. A. Shoemaker, J. Y. Wang, B. Yu, J. Zhang and S. H. Bryant, Nucleic Acids Res., 2016, 44, D1202–D1213 CrossRef PubMed
. - R. Hoogenboom, T. Bovee, W. Traag, R. Hoogerbrugge, B. Baumann, L. Portier, G. van de Weg and J. de Vries, Mol. Nutr. Food Res., 2006, 50, 945–957 CrossRef PubMed
. - A. Tropsha, Mol. Inf., 2010, 29, 476–488 CrossRef PubMed
. - M. Haranczyk, T. Puzyn and P. Sadowski, QSAR Comb. Sci., 2008, 27, 826–833 CrossRef
. - A. Rybinska, A. Sosnowska, M. Barycki and T. Puzyn, J. Comput.-Aided Mol. Des., 2016, 30, 165–176 CrossRef PubMed
. - T. Puzyn, N. Suzuki, M. Haranczyk and J. Rak, J. Chem. Inf. Model., 2008, 48, 1174–1180 CrossRef PubMed
. - A. Mauri, V. Consonni, M. Pavan and R. Todeschini, MATCH, 2006, 56, 237–248 Search PubMed
. - X. J. Yao, A. Panaye, J. P. Doucet, R. S. Zhang, H. F. Chen, M. C. Liu, Z. D. Hu and B. T. Fan, J. Chem. Inf. Model., 2004, 44, 1257–1266 CrossRef PubMed
. - T. Ghafourian and M. T. D. Cronin, SAR QSAR Environ. Res., 2005, 16, 171–190 CrossRef PubMed
. - P. Gramatica, N. Chirico, E. Papa, S. Cassani and S. Kovarich, J. Comput. Chem., 2013, 34, 2121–2132 CrossRef
. - S. E. Haupt and R. L. Haupt, Practical Genetic Algorithms, John Wiley & Sons, Inc., New Jersey, 2nd edn, 2004 Search PubMed
. - A. Cherkasov, E. N. Muratov, D. Fourches, A. Varnek, I. I. Baskin, M. Cronin, J. Dearden, P. Gramatica, Y. C. Martin, R. Todeschini, V. Consonni, V. E. Kuz'min, R. Cramer, R. Benigni, C. H. Yang, J. Rathman, L. Terfloth, J. Gasteiger, A. Richard and A. Tropsha, J. Med. Chem., 2014, 57, 4977–5010 CrossRef PubMed
. - OECD, Principles for the validation, for regulatory purposes, of (quantitative) structure-activity relationship models, OECD, Paris, 2004 Search PubMed
. - J. H. Wu, J. A. Mei, S. X. Wen, S. Y. Liao, J. C. Chen and Y. Shen, J. Comput. Chem., 2010, 31, 1956–1968 CrossRef PubMed
. - P. Gramatica, QSAR Comb. Sci., 2007, 26, 694–701 CrossRef
. - N. Chirico and P. Gramatica, J. Chem. Inf. Model., 2011, 51, 2320–2335 CrossRef PubMed
. - T. Cseh, S. Sanschagrin, J. Hawari and R. Samson, Appl. Environ. Microbiol., 1989, 55, 3150–3154 Search PubMed
. - J. K. Unger, G. Kuehlein, A. Schroers, J. C. Gerlach and R. Rossaint, Biomaterials, 2001, 22, 2031–2037 CrossRef PubMed
. - J. C. McDonald, D. C. Duffy, J. R. Anderson, D. T. Chiu, H. K. Wu, O. J. A. Schueller and G. M. Whitesides, Electrophoresis, 2000, 21, 27–40 CrossRef PubMed
. - A. L. Thangawng, R. S. Ruoff, M. A. Swartz and M. R. Glucksberg, Biomed. Microdevices, 2007, 9, 587–595 CrossRef PubMed
. - C. W. Tsao, Micromachines, 2016, 7(12), 225 CrossRef
. - E. Berthier, E. W. K. Young and D. Beebe, Lab Chip, 2012, 12, 1224–1237 RSC
. - K. Y. Chumbimuni-Torres, R. E. Coronado, A. M. Mfuh, C. Castro-Guerrero, M. F. Silva, G. R. Negrete, R. Bizios and C. D. Garcia, RSC Adv., 2011, 1, 706–714 RSC
. - M. W. Toepke and D. J. Beebe, Lab Chip, 2006, 6, 1484–1486 RSC
. - B. J. van Meer, H. de Vries, K. S. A. Firth, J. van Weerd, L. G. J. Tertoolen, H. B. J. Karperien, P. Jonkheijm, C. Denning, I. J. AP and C. L. Mummery, Biochem. Biophys. Res. Commun., 2017, 482, 323–328 CrossRef PubMed
. - N. Li, M. Schwartz and C. Ionescu-Zanetti, Journal of biomolecular screening, 2009, 14, 194–202 CrossRef PubMed
. - J. D. Wang, N. J. Douville, S. Takayama and M. ElSayed, Ann. Biomed. Eng., 2012, 40, 1862–1873 CrossRef PubMed
. - S. Noria, F. Xu, S. McCue, M. Jones, A. I. Gotlieb and B. L. Langille, Am. J. Pathol., 2004, 164, 1211–1223 CrossRef PubMed
. - S. Miura, K. Sato, M. Kato-Negishi, T. Teshima and S. Takeuchi, Nat. Commun., 2015, 6 Search PubMed
. - K. R. Groschwitz and S. P. Hogan, J. Allergy Clin. Immunol., 2009, 124, 3–20 CrossRef PubMed
, quiz 21–22.. - J. Konig, J. Wells, P. D. Cani, C. L. Garcia-Rodenas, T. MacDonald, A. Mercenier, J. Whyte, F. Troost and R. J. Brummer, Clin. Transl. Gastroenterol., 2016, 7, e196 CrossRef PubMed
. - Y. Natsume, H. Satsu, Y. Hatsugai, H. Watanabe, R. Sato, H. Ashida, R. H. Tukey and M. Shimizu, Food Sci. Technol. Res., 2003, 9, 364–366 CrossRef
. - M. Casalegno, G. Raos and G. Sello, Phys. Chem. Chem. Phys., 2016, 18, 17731–17739 RSC
. - M. Laznicek and A. Laznickova, J. Pharm. Biomed. Anal., 1995, 13, 823–828 CrossRef PubMed
. - M. Hamada, H. Satsu, Y. Natsume, S. Nishiumi, I. Fukuda, H. Ashida and M. Shimizu, J. Agric. Food Chem., 2006, 54, 8891–8898 CrossRef PubMed
. - P. A. Munzel, S. Schmohl, H. Heel, K. Kalberer, B. S. Bock-Hennig and K. W. Bock, Drug Metab. Dispos., 1999, 27, 569–573 Search PubMed
. - M. Daujat, S. Charrasse, I. Fabre, P. Lesca, Y. Jounaidi, C. Larroque, L. Poellinger and P. Maurel, Eur. J. Biochem., 1996, 237, 642–652 CrossRef PubMed
. - W. J. de Waard, J. M. M. J. G. Aarts, A. A. C. M. Peijnenburg, H. Baykus, E. Talsma, A. Punt, T. M. C. M. de Kok, F. J. van Schooten and L. A. P. Hoogenboom, Toxicol. in Vitro, 2008, 22, 396–410 CrossRef PubMed
. - S. Cavret, C. Feidt and F. Laurent, J. Agric. Food Chem., 2005, 53, 2773–2777 CrossRef PubMed
. - H. Lodish, A. Berk and S. L. Zipursky, et al., in Molecular Cell Biology, W. H. Freeman, New York, 4th edn, 2000, ch. 15.1 Search PubMed
. - A. G. Oomen, J. Tolls, M. Kruidenier, S. S. D. Bosgra, A. J. A. M. Sips and J. P. Groten, Environ. Health Perspect., 2001, 109, 731–737 CrossRef PubMed
. - T. Hartung, Nature, 2009, 460, 208–212 CrossRef PubMed
. - T. Hartung, T. Luechtefeld, A. Maertens and A. Kleensang, Alternatives to Animal Experimentation, 2013, 30, 3–18 Search PubMed
. - M. Wagener, J. Sadowski and J. Gasteiger, J. Am. Chem. Soc., 1995, 117, 7769–7775 CrossRef
. - H. Bauknecht, A. Zell, H. Bayer, P. Levi, M. Wagener, J. Sadowski and J. Gasteiger, J. Chem. Inf. Model., 1996, 36, 1205–1213 CrossRef PubMed
. - L. Saiz-Urra, M. P. Gonzalez and M. Teijeira, Bioorg. Med. Chem., 2007, 15, 3565–3571 CrossRef PubMed
. - B. Bordas, I. Belai and T. Komives, J. Agric. Food Chem., 2011, 59, 2863–2869 CrossRef PubMed
. - C. Probst, S. Schneider and P. Loskill, Curr. Opin. Biomed. Eng., 2018, 6, 33–41 CrossRef
. - S. F. Mao, D. Gao, W. Liu, H. B. Wei and J. M. Lin, Lab Chip, 2012, 12, 219–226 RSC
. - D. Gao, H. Li, N. Wang and J. M. Lin, Anal. Chem., 2012, 84, 9230–9237 CrossRef PubMed
. - D. Gao, H. X. Liu, J. M. Lin, Y. N. Wang and Y. Y. Jiang, Lab Chip, 2013, 13, 978–985 RSC
.
Footnotes |
† Electronic supplementary information (ESI) available. See DOI: 10.1039/c8ra05430d |
‡ These authors jointly directed this work. |
|
This journal is © The Royal Society of Chemistry 2018 |