DOI:
10.1039/C8RA05042B
(Paper)
RSC Adv., 2018,
8, 25456-25467
Dynamics revelation of conformational changes and binding modes of heat shock protein 90 induced by inhibitor associations†
Received
12th June 2018
, Accepted 10th July 2018
First published on 16th July 2018
Abstract
Heat shock protein 90 (Hsp90) has been an attractive target of potential drug design for antitumor treatment. The current work integrates molecular dynamics (MD) simulations, calculations of binding free energy, and principal component (PC) analysis with scanning of inhibitor–residue interaction to probe the binding modes of inhibitors YK9, YKJ and YKI to Hsp90 and identify the hot spot of the inhibitor–Hsp90 binding. The results suggest that the introductions of two groups G1 and G2 into YKJ and YKI strengthen the binding ability of YKJ and YKI to Hsp90 compared to YK9. PC analysis based MD trajectories prove that inhibitor bindings exert significant effects on the conformational changes, internal dynamics and motion modes of Hsp90, especially for the helix α2 and the loops L1 and L2. The calculations of residue-based free energy decomposition and scanning of the inhibitor–Hsp90 interaction suggest that six residues L107, G108, F138, Y139, W162 and F170 construct the common hot spot of the inhibitor–residue interactions. Moreover the substitutions of the groups G1 and G2 in YKJ and YKI lead to two additional hydrogen bonding interactions and multiple hydrophobic interactions for bindings of YKJ and YKI to Hsp90. This work is also expected to contribute theoretical hints for the design of potent inhibitors toward Hsp90.
1 Introduction
Heat shock protein 90 (Hsp90), a main member of the heat shock protein family, is richly expressed in organisms ranging from bacteria to humans. Hsp90 is an ATP-dependent molecular chaperone and plays important roles in maintaining conformation, stability and function of extensive signaling proteins acting as pathways of cell proliferation, cell cycle progression, angiogenesis, invasion and metastasis.1,2 The previous studies proved that many client proteins of Hsp90 are involved in multiple key oncogenic proteins, such as Her2, AKT, CDK4, VEGF, MET and ALK.3–7 Moreover, Hsp90's broad client proteins are known disease-related peptides and implicate the chaperone in the progression of several pathologies relating with various protein misfolding disorders, cancer, and neurological diseases.8–10 Thus, Hsp90 has been an attractive target of potential drug design for antitumor treatment.
According to the previous studies, inhibitors of Hsp90 can produce simultaneous effects on several abnormal signaling pathways in tumor cells, which may conquer different issues related to cancer resistance.11–14 Since the first Hsp90 inhibitor (17-AAG, tanespimycin) was identified,15 extensive efforts have been paid in rational drug design and drug discovery targeting Hsp90. For example, Biamonte et al. applied the structure-based drug design technology to develop the first totally synthesized Hsp90 inhibitor PU3 (ref. 16 and 17) and the following inhibitors CNF-2024 and BIIB021 entering clinical trials in 2005 were also developed based on the pharmacophore model derived from PU3.18,19 Yi et al. employed MD simulations and binding free energy calculations to probe binding modes of pyrazole-based inhibitors to Hsp90 and their results revealed that van der Waals interaction is primarily responsible for binding of inhibitors to Hsp90.20 Recently, some different inhibitors have also entered clinical trials and displayed certain efficiency in inhibiting the activity of Hsp90.21–27 In despite of high efficiency of these inhibitors targeting the N-terminal ATP pocket of Hsp90 in clinical trials, side effects were commonly observed in Hsp90 inhibitors, which heavily limits the clinical studies of some novel inhibitors.28–31 Thus it is of significance to further probe binding modes of inhibitors to Hsp90 at atomic level for design of potent inhibitors targeting Hsp90.
It is well known that efficient Hsp90 chaperone cycles depend on extremely flexible structure of Hsp90, which is in concert with cochaperones and adaptor proteins.32 The previous work demonstrated that conformational changes of the N-terminal ATP site of Hsp90 play key role in inhibition of ATP hydrolysis and client processing.33–35 In fact, bindings of substrates and inhibitors really induce large-scale conformational changes of Hsp90.8,36–38 Moreover, probing drug–protein binding mechanisms and their thermodynamics and kinetics of conformational changes are prerequisites to develop efficient drugs toward targeting proteins.39 Therefore, it is essential to explore the conformational changes of Hsp90 induced by inhibitor bindings for design of potent inhibitor targeting Hsp90.
Recently, MD simulations40–48 and principal component (PC) analysis49–53 have been powerful tools to study the conformational changes of proteins. Binding free energy calculations54–60 were also an important approach to reveal binding modes of inhibitors to proteins. Moreover, the works from Colombo et al. proved that MD simulations have been successfully used to study binding modes of inhibitors to Hsp90 and revealed multiple binding poses in the binding pocket of Hsp90.61–63 In this work, we selected three inhibitors YK9, YKJ and YKI to probe binding mechanisms of inhibitors to Hsp90. The structure of inhibitor–Hsp90 complex and binding pocket are depicted in Fig. 1A and B, while the structures of three inhibitors were displayed in Fig. 1C–E. The cause why we selected these inhibitors is because they have different binding ability to Hsp90 and the IC50 values of YK9, YKJ and YKI are 8100, 610 and 30 nM, respectively.64 We expect that three aims can be realized through this work: (1) MD simulations followed by PC analysis were performed to probe the conformational changes of Hsp90 induced by inhibitor bindings, (2) binding free energies were calculated by using molecular mechanics Poisson–Boltzmann surface area (MM-PBSA) and molecular mechanics generalized Born surface area (MM-GBSA)65–71 to comparatively evaluate the binding modes of inhibitors to Hsp90, (3) decomposition of residue-based free energies and scanning of inhibitor–residue interactions were carried out to reveal the hot spot of interactions of inhibitors with Hsp90. This study was also expected to theoretically contribute significant dynamics information and molecular basis to design of efficient inhibitors targeting Hsp90.
 |
| Fig. 1 Molecular structures. (A) The YKI-Hsp90 complex is shown in cartoon modes, (B) binding pocket of YKI to Hsp90 is displayed in surface modes and the key residues around the pocket are shown in stick modes. (C), (D) and (E) are the structures of inhibitors YK9, YKJ and YKI. | |
2 Methods
2.1 Construction of initial systems
The initial coordination used in MD simulations were taken from protein data bank (PDB): 2YK9, 2YKJ and 2YKI separately correspond to the YK9-, YKJ- and YKI-Hsp90 complexes.64 The apo Hsp90 was generated by removing YK9 from 2YK9. The protonated state of residues in Hsp90 were checked by using the program PROPKA.72,73 All crystal water molecules were kept in the initial model and all missing hydrogen atoms were connected with heavy atoms by using the Leap module in Amber 16.74 The parameters of Hsp90 were generated with ff14SB force field of Amber75 and that of water molecules were assigned using the TIP3P model.76 Molecular structure of three inhibitors were optimized using the Gaussian 09 program at the HF/6-31G* level, and then restrained electrostatic potential (RESP) charges were assigned to atoms of inhibitors.77 Meanwhile, the general Amber force field (GAFF) were applied to produce the force field parameters of three inhibitors.78 Each complex was placed in a truncated octahedral box of TIP3P water molecules, extending at least 12.0 Å along each dimension from each complex. The total of seven counterions Na+ were added to neutralize each system.
2.2 MD simulations in waters
To relieve high-energy contacts between atoms and highly repulsive orientations of protein-solvent system, each system were minimized by the steepest descent minimization of 2000 steps followed by the conjugate gradient minimization of 3000 steps. Then, a gentle heating process of 1 ns were performed to heat each system from 0 to 300 K at constant volume and another 1 ns process of temperature equilibrium at 300 K was adopted to further equilibrate the simulated system. Finally, a 200 ns MD simulation without restriction was conducted to fully relax each system and the coordinates of atoms were recorded by at a time intervals of 4 ps. During MD simulations, the SHAKE algorithm79 was employed to constrain the chemical bond involving hydrogen atoms so that a time step of 2 fs was adopted. The temperature of systems was controlled by the Langevin thermostat80 with a collision frequency of 2.0 ps−1. The long-range electrostatic interactions were computed by using the particle mesh Ewald (PME) method with an appropriate cut-off value of 10 Å. The same cutoff as calculations of the electrostatic interaction was adopted to calculate van der Waals interactions. PC analysis was performed on MD trajectories using the program CPPTRAJ in Amber81 and the details concerning PC analysis have been described in our previous studies.50,82 The software PyMOL83 and VMD84 were applied to analyze MD trajectories and depict pictures.
2.3 Calculations of binding free energies
Binding free energies between inhibitors and Hsp90 were calculated by using MM-PBSA and MM-GBSA methods to comparatively evaluate binding ability of inhibitors to Hsp90. The total of 200 snapshots extracted from the last 100 ns of MD trajectories at a time interval of 500 ps were used to perform calculations of binding free energies based on the following equation. |
ΔGbind = Gcomp − Gpro − Glig = ΔEele + ΔEvdW + ΔGpol + ΔGnonpol − TΔS
| (1) |
in which Gcomp, Gpro and Glig indicate free energies of the complex, protein and inhibitor, respectively. ΔEele and ΔEvdW represent electrostatic and van der Waals interactions of inhibitors with proteins, independently. ΔGpol and ΔGnonpol are the polar and nonpolar solvation free energies, respectively. In the current work, ΔGpol can be separately solved by using the PB and GB model developed by Onufriev et al.85 and ΔGnonpol is determined by the following empirical equation: |
ΔGnonpol = γ × ΔSASA + β
| (2) |
where the parameter γ and ΔSASA separately represent the surface tension and the difference in the solvent accessible surface areas induced by inhibitor bindings. The parameter β indicates a regression offset of the linear relationship. In the PB calculations, γ and β were respectively set to 0.00542 kcal mol Å−2 and 0.92 kcal mol−1, while in the GB calculations, γ and β were set to 0.0072 kcal mol Å−2 and 0 kcal mol−1, separately.86 The last term −TΔS indicates the contributions of the entropy changes to inhibitor bindings, which is calculated by using the mmpbsa_py_nabnmode program based on 50 snapshots.87
2.4 Calculations of cross-correlation map
By now, the cross-correlation map has been an effective approach to probe internal dynamics of proteins and can efficiently reveal details of movement modes between residues in proteins. The cross-correlation coefficient Cij of each pair of Cα atoms i and j can be generated using MD trajectories based on the following equation.88,89 |
 | (3) |
where Δri represents the displacement from the averaged position of the ith Cα atom. The values of the cross-correlation coefficient Cij range from −1 to 1. The positive Cij are indicator of the correlated motions between residues i and j, while the negative Cij characterize the anticorrelated movements of residue i relative to j. In this work, the CPPTRAJ module in AMBER 16 was applied to compute the cross-correlation map. The extent of correlated motions between residues is displayed in color-coded modes.
3 Results and discussion
3.1 Structural stability and flexibility of MD simulations
To evaluate equilibrium and stability of MD simulations, root-mean-square deviations (RMSDs) of backbone atoms in Hsp90 relative to the first structure were computed based on MD trajectories. The function of RMSD for backbone atoms vs. the simulated time is depicted in ESI Fig. S1.† It is observed that all four systems basically reach the equilibrium after 100 ns of MD simulations. The RMSD values of the apo Hsp90, YK9-, YKJ- and YKI-Hsp90 compounds are 2.37, 1.99, 1.51 and 1.52 Å after the equilibrium of systems, respectively, and inhibitor bindings decrease the RMSD values of Hsp90 complexed with inhibitors compared to the apo system. Furthermore, the fluctuation of RMSDs in four systems are lower than 0.6 Å. This result suggests that the equilibrium of systems is reliable for the following analysis of conformational changes for Hsp90 and calculations of binding free energies.
To check the stability of three inhibitors in the binding pocket of Hsp90 during MD simulations, RMSDs of non-hydrogen atoms in inhibitors relative to the first structure were calculated through the entire MD simulations (Fig. S2A†). It is seen that the RMSD values of three inhibitors are lower than 1.35 Å, which suggests that three inhibitors are stable in the binding pocket of Hsp90. The previous studies proved that structural cluster analysis can better reveal the binding poses of inhibitors in the binding pocket of Hsp90.61,62 Thus we performed the structural cluster analyses of inhibitors on the RMSDs with a cut-off of 0.5 Å using the CPPTRAJ module in Amber (Fig. S2B–D†). The results indicate that YK9 has a binding pose, while YKJ and YKI have two binding poses, which implies that YK9 should have different binding modes from YKJ and YKI.
Root-mean-square fluctuations (RMSFs) of residues are usually used to evaluate structural flexibility of proteins. In this work, RMSFs of Cα atoms in Hsp90 were computed using the equilibrated MD trajectories (Fig. 2). The RMSFs of the N-terminus, the residues 100–110 and the region near the residue 180 are increased relative to the apo Hsp90, which shows that the structural flexibility of these residues are strengthened by bindings of three inhibitors. However, the RMSFs of the domains aside from the N-terminus, the residues 100–110 and the region near the residue 180 are decreased by inhibitor bindings compared to the apo Hsp90, which suggests that the flexibility of the above domains are weakened due to the presence of three inhibitors. This result may imply that the hot spot of inhibitor–residue interactions occurs in the domains of the obvious changes in the structural flexibility.
 |
| Fig. 2 Root-mean-square fluctuations (RMSFs) of Cα atoms in Hsp90. | |
3.2 Changes in internal dynamics of Hsp90 induced by inhibitor bindings
The changes in conformations and internal dynamics of targeting proteins are of significance for drug designs. In this work, the cross-correlation maps of residues in Hsp90 were computed based on the equilibrated MD trajectories to probe the changes in the motion modes of Hsp90 caused by inhibitor bindings (Fig. 3). The strongly correlated motions between specific residues were reflected in red and yellow, while the strongly anticorrelated movements were indicated in blue or dark blue. It is observed from Fig. 3 that inhibitor bindings really induce obvious changes in the motion modes of Hsp90.
 |
| Fig. 3 Cross-correlation maps of the fluctuations of the coordinates for Cα atoms of Hsp90 around the mean positions after the equilibration of MD simulations: (A) the apo Hsp90, (B) the YK9-bounded Hsp90, (C) the YKJ-bounded Hsp90 and (D) the YKI-bounded Hsp90. | |
For the apo Hsp90 (Fig. 3A), the strongly correlated motions occur in the regions R1, R2, R3 and R4 (yellow and red). The regions R1 and R2 reflect the correlated motions of residues 48–87 and 144–178 relative to themselves. The motions in the region R3 represent the correlated motions of residues 85–100 relative to residues 43–78, and the region R4 describes the correlated movements between residues 178–186 and 138–156. In addition, the obviously anticorrelated motions are observed in the regions R5 and R6 (blue), in which the region R5 reflects the anticorrelated movements between residues 110–145 and 40–68, and the region R6 characterizes the anticorrelated motions of the C-terminus of Hsp90 relative to residues 50–80. Compared to the apo Hsp90, bindings of three inhibitors obviously weaken the correlated motions occurring in the regions R1, R2, R3 and R4 (Fig. 3B–D). Differently, the YK9 binding weakens the anticorrelated movements of the region R5 (Fig. 3B), but the bindings of YKJ and YKI highly strengthen the anticorrelated motion in the region R5. In addition, the presence of YKI in Hsp90 also increases the anticorrelated movement of the region R7 compared to the apo Hsp90 (Fig. 3D).
To further probe the conformational changes of Hsp90 due to inhibitor bindings, PC analysis was performed to obtain eigenvalues and eigenvectors by constructing a covariance matrix using the atom coordinates from MD trajectories. Fig. S3† gives the function of the eigenvalues vs. the eigenvector indices, which reflects the motion strength of Hsp90 in the apo and bounded states. The first three principal components account for 78.21%, 70.02%, 64.58% and 63.61% of the total motion observed in the last 100 ns of MD simulations. It is seen that inhibitor bindings highly weaken the motion strength of Hsp90 in the bounded state. The changes in the direction of motions can be captured by the eigenvectors obtained from PC analysis, which are depicted in four porcupine plots (Fig. 4). It is noted that inhibitor bindings produce significant effect on the motions of the helixes α1, α3 and the loops L1 and L2. In the apo Hsp90, α1 has a strong tendency of upward and outward motions, meanwhile the helix α2 and the loop L2 have weak tendency of outward motions. On the contrary, the helix α3 and the loop L1 produce strong tendency of inward movements. Compared to the apo Hsp90, the YK9 binding heavily inhibits the motion strength of α1 (Fig. 4B), while the bindings of YKJ and YKI extremely change the motion direction of α1 (Fig. 4C and D). Commonly, the bindings of all three inhibitors make L1 and α3 produce the outward motion direction. According to Fig. 4B–D, the presences of three inhibitors in Hsp90 not only change the motion direction of the loop L2, but also strengthen the movement of L2 compared to the apo Hsp90. In addition, the association of YKI with Hsp90 also strengthens the motion of the helix α2 (Fig. 4D).
 |
| Fig. 4 Collective motions corresponding to the first principal component PC1 obtained by performing principal component analysis (PCA) on the equilibrated MD trajectories: (A) the unbounded Hsp90, (B) the YK9-bounded Hsp90, (C) the YKJ-bounded Hsp90 and (D) the YKI-bounded Hsp90. | |
To better understand the conformational changes of Hsp90 induced by inhibitor bindings, free energy landscapes, contributing important information of different conformations sampled by proteins, were constructed by using projections of MD trajectories on the eigenvectors of the first two principal components PC1 and PC2 (Fig. 5). It is seen that the bindings of three inhibitors induce obvious redistribution of the conformation of Hsp90. Fig. 5A suggests that the apo Hsp90 spans six different subspaces and energy basins, which shows that Hsp90 has the conformation diversity in the unbounded state. According to Fig. 5B–D, the associations of three inhibitors with Hsp90 lead to the conformational convergence. In the bounded state of YK9 and YKJ, Hsp90 tends to have four main conformational subspaces (Fig. 5B and C), while the presence of YKI makes Hsp90 redistribute in three conformational subspaces (Fig. 5D). To better understand the conformational changes, the cluster analysis was performed on MD trajectories using the RMSD values of Cα atoms in Hsp90 and the CPPTRAJ program (Fig. S4†) and the cutoff used this analysis is 2.0 Å. The results indicate that the apo, YK9-, YKJ- and YKI-Hsp90 have six, four, four and three clusters, which agrees basically with our current PC analysis. The above analyses suggest that the YKI binding induces the most obvious conformational changes among three inhibitors.
 |
| Fig. 5 Free energy landscapes of Hsp90 constructed using projection of MD trajectories on the first two principal components PC1 and PC2 from the diagonalization of covariance matrix: (A) the apo Hsp90, (B) the YK9-bounded Hsp90, (C) the YKJ-bounded Hsp90 and (D) the YKI-bounded Hsp90. | |
Based on the above analysis, the bindings of YK9, YKJ and YKI produce extremely different effect on the motion modes, internal dynamics and conformations of Hsp90. According to Fig. 1C and D, the main difference in the structures of three inhibitors comes from the substitutions of two groups G1 and G2 with polar nitrogen atom. These two substitutions increase the size of YKJ and YKI relative to YK9, which must lead to more interaction contacts with the residues in the binding pocket of Hsp90, further induce the conformational changes of Hsp90 and affect the bindings of inhibitors to Hsp90.
3.3 Calculations of binding free energies
To evaluate binding ability of three inhibitors to Hsp90, MM-PBSA and MM-GBSA methods were applied to compute binding free energies of YK9, YKJ and YKI to Hsp90. The results calculated by MM-PBSA were listed in Table 1 and that computed by MM-GBSA were given in Table S1.† Although the binding free energies predicted by two methods are extremely higher than the experimentally determined values, it is encouraging that the rank of our predicted binding free energies using two methods is in agreement with the one determined by the experimental data. For two methods, the results calculated by MM-GBSA are higher than that computed by MM-PBSA.
Table 1 Binding free energies of inhibitors to Hsp90 calculated by MM-PBSA method
aComponents |
YKC-Hsp |
YKJ-Hsp |
YKI-Hsp |
Average |
bSTD |
Average |
STD |
Average |
STD |
All components of free energies are in kcal mol−1. Standard errors. ΔGele+pol = ΔEele+ΔGpol. The experimental values were derived from the experimental Ki values in reference using the equation ΔGexp = −RT ln Ki. |
ΔEele |
−14.75 |
3.01 |
−16.98 |
4.30 |
−35.95 |
4.16 |
ΔEvdW |
−45.10 |
2.74 |
−46.09 |
3.02 |
−60.70 |
2.62 |
ΔGpol |
35.28 |
2.42 |
32.71 |
3.44 |
−6.37 |
0.10 |
ΔGnonpol |
−4.84 |
0.07 |
−4.94 |
0.10 |
61.54 |
4.16 |
cΔGele+pol |
20.53 |
2.85 |
15.73 |
3.32 |
25.59 |
4.33 |
−TΔS |
18.97 |
1.98 |
21.29 |
1.99 |
26.90 |
2.12 |
ΔGbind |
−10.44 |
|
−14.01 |
|
−15.48 |
|
dΔGexp |
−6.96 |
|
−8.50 |
|
−10.31 |
|
According to Table 1, binding free energies of YK9, YKJ and YKI to Hsp90 are −10.44, −14.01 and −15.48 kcal mol−1, respectively. It is found that binding free energies of inhibitors to Hsp90 consist of van der Waals interactions (ΔEvdW), electrostatics interactions (ΔEele), polar solvation energies (ΔGpol), nonpolar solvation energies (ΔGnonpol) and the entropy changes (−TΔS). The van der Waals interactions and nonpolar solvation energies contribute advantageous forces to inhibitor bindings, but the entropy changes impair the association of inhibitors with Hsp90. Although the electrostatics interaction favors inhibitor bindings, this favorable factor is fully encountered by the polar solvation energies to produce unfavorable forces (ΔEele+pol) for inhibitor bindings to Hsp90. The results from the MM-GBSA calculations (Table S1†) also give similar analysis to the MM-PBSA calculations. As shown in Table 1, binding free energies of YKJ and YKI to Hsp90 are increased by 3.57 and 5.04 kcal mol−1 compared to that of YK9 to Hsp90, respectively. According to Table S1,† binding affinities of YKJ and YKI are 3.24 and 4.96 kcal mol−1 stronger than YK9, separately. The computational comparison of two methods not only suggest that our current results are reliable, but also prove that binding ability of YKJ and YKI to Hsp90 is stronger than YK9. The comparison of separate free energy components from Table 1 further reveals that van der Waals interaction of YKJ and YKI with Hsp90 are increased by 0.99 and 15.6 kcal mol−1 relative to that of YK9 with Hsp90, respectively, and the electrostatic interactions of YKJ and YKI with Hsp90 are strengthened by 2.23 and 21.20 kcal mol−1 relative to YKJ. In addition, the nonpolar solvation energies caused by the bindings of YKJ and YKI are increased by 0.10 and 1.53 kcal mol−1 compared to YK9, respectively. According to Fig. 1C–E, the hydrophobic rings in the G1 and G2 groups of YKJ and YKI may increase the hydrophobic contacts with the hydrophobic residues in Hsp90 relative to YK9, and the polar nitrogen atoms in the G1 and G2 groups may bring new hydrogen bonding interactions with Hsp90 compared to YK9, which improves the van der Waals interactions and electrostatics interactions of YKJ and YKI with Hsp90. This result also agrees well with the experimental results from Vallée et al.64
3.4 Identification of inhibitor–residue interaction hot spot
To identify the hot spot of the inhibitor–residue interaction and probe the contributions of separate residues to inhibitor bindings, the residue-based free energy decomposition method was applied to compute the interactions of inhibitors with separate residues of Hsp90 (Fig. 6). To compare with the calculations of inhibitor–residue interactions, scanning of hydrophobic contacts and hydrogen bond contacts of inhibitors with Hsp90 was performed on the entire MD trajectories by using the LIGPLOT program.90 In our current scanning, the default parameters from LIGPLOT were applied to define hydrogen bond and hydrophobic contacts. The function of hydrophobic contacts and hydrogen bond contacts vs. the simulation time were depicted in Fig. 7 and S5,† respectively. The statistical results of hydrophobic contacts and hydrogen bond contacts were displayed in Fig. 8 and S6.† Meanwhile, the CPPTRAJ program was employed to analyze the hydrogen bonding interactions of inhibitors with Hsp90 and the corresponding information was listed in Table 2. In addition, the geometry position involving hydrophobic contacts and hydrogen bonding interactions are separately displayed in Fig. 9 and S7† by using the lowest energy structures extracted from MD trajectories.
 |
| Fig. 6 Inhibitor–residue interactions calculated by using residue-based free energy decomposition: (A) YK9, (B) YKJ and (C) YKI. | |
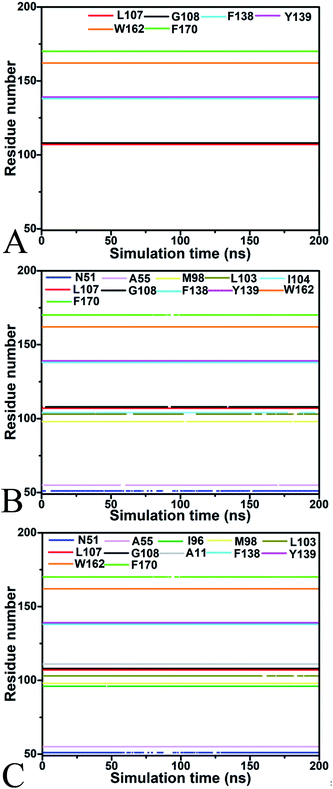 |
| Fig. 7 The function of hydrophobic contacts between inhibitors and Hsp90 vs. the simulation time: (A) YK9, (B) YKJ and (C) YKI. | |
 |
| Fig. 8 Statistical analyses of hydrophobic contacts between inhibitors and separate residues of Hsp90. In the current work, the occupancy is defined as the number of conformations forming the C–C atom contacts accounting for the percentage of the total number of conformations recorded in MD trajectories. | |
Table 2 Main hydrogen bonding interactions of inhibitors with Hsp90
Hydrogen bonds |
aDistance (Å) |
aAngle (°) |
bOccupancy (%) |
Hydrogen bonds are determined by the acceptor⋯donor distance of <3.5 Å and acceptor⋯H-donor angle of >120°. Occupancy (%) is defined as the percentage of simulation time that a specific hydrogen bond exists. The full lines indicate chemical bonds, and the dotted lines describe hydrogen bonding interactions. |
cYK9-N12⋯Y139-OH-HH |
3.07 ± 0.31 |
137.29 ± 7.8 |
94.37 |
YKJ-N6-H6⋯G97-O |
3.32 ± 0.24 |
121.26 ± 10.1 |
23.61 |
YKJ-N2-H3⋯L103-O |
3.01 ± 0.28 |
153.72 ± 9.6 |
67.60 |
YKJ-N3⋯Y139-OH-HH |
2.96 ± 0.35 |
158.38 ± 11.2 |
92.22 |
YKI-O13⋯N51-ND2-HD22 |
3.02 ± 0.21 |
132.61 ± 8.4 |
43.28 |
YKI-N2-H3⋯L103-O |
3.02 ± 0.44 |
147.93 ± 8.9 |
64.31 |
YKI-N3⋯Y139-OH-HH |
2.92 ± 0.26 |
159.52 ± 11.6 |
98.80 |
 |
| Fig. 9 Key residues of Hsp90 forming hydrophobic contacts with three inhibitors. Key residues and inhibitors are shown in stick modes. A pair of carbon atoms following the default parameters of LIGPLOT in distances are defined as hydrophobic contacts. | |
For the inhibitor YK9, Fig. 6A suggests that YK9 can produce interactions stronger than 1.0 kcal mol−1 with six residues of Hsp90, including L107, G108, F138, Y139, W162 and F170. Scanning of hydrophobic contacts between YK9 and Hsp90 also shows that YK9 forms stable hydrophobic contacts with these six residues of Hsp90 (Fig. 7A). The interaction energy of L107 with YK9 are −4.10 kcal mol−1 (Fig. 6A). This interaction structurally comes from the CH–π interaction of the alkyl in L107 with the hydrophobic ring R1 (Fig. 9A), which is supported by the hydrophobic contact occupancy of 90.9% between L107 and YK9 (Fig. 8). According to Fig. 9A and S7A,† Y139 structurally generates two classes of interactions, one stems from the π–π interaction between the ring R1 of YK9 and the phenyl of Y139, another origins from the hydrogen bonding interaction with the occupancy of 94.37% between the nitrogen atom N12 of YK9 and the oxygen atom OH of Y139 (Table 2, Fig. S5A† and S6A). These two interactions totally contribute the interaction energy of −2.13 kcal mol−1 to the YK9 binding (Fig. 6A). Scanning of hydrophobic contacts finds that the ring R3 of YK9 forms the stable π–π contacts with F138 and W162 though MD simulations (Fig. 7A and 9A). The number of conformations detecting the hydrophobic contacts of YK9 with F38 and W162 separately account for 90.3% and 90.4% of the total conformations from MD trajectories (Fig. 8), which provides the energy contributions of −2.32 and −2.62 kcal mol−1 for the YK9 binding (Fig. 6A). As shown in Fig. 9A, the carbon atom of G108 and the phenyl of F170 structurally close the hydrophobic ring R2 of YK9, which are easy to respectively produce the CH–π and π–π interactions between them. These two interactions are not only supported by scanning of hydrophobic contacts (Fig. 7A and 8), but also contribute the interaction energies of −1.22 and −1.15 kcal mol−1 to the YK9 binding (Fig. 6A).
Similar to YK9, YKJ also generates favorable interactions with the residues L107, G108, F138, Y139, W162 and F170 of Hsp90 (Fig. 6B), which is supported by scanning of hydrophobic contacts between YKJ and Hsp90 as well as statistical analysis of hydrophobic contacts (Fig. 7B and 8). The interaction modes of these six residues with YKJ are also similar to YK9 (Fig. 9B). A hydrogen bond with the occupancy of 92.22% is also identified between the oxygen atom OH of Y139 and the nitrogen atom N3 of YKJ (Table 2, Fig. S5B, S6 and S7B†). Due to the substitution of the group G1 (Fig. 1D), several additional interactions of YKJ with N51, A55, G97, M98 and I104 are detected (Fig. 6B, 7B and S5B†). The CPPTRAJ analysis shows that YK9 constructs two hydrogen bonding interactions with G97 and L103 (Table 2), which agrees well with scanning of hydrogen bond contacts (Fig. S5B, S6 and S7B†). In addition, L103 also forms stable hydrophobic contacts with YKJ (Fig. 7B, 8 and 9B). Totally, L103 provides the energy contribution of −2.28 kcal mol−1 for the YKJ binding to Hsp90 (Fig. 6B). Structurally, the carbon atom C of N51 and the alkyls of A55 and M98 are located near the ring R4 of YKJ (Fig. 9B) and are easy to generate the CH–π interactions between them, which is supported by scanning of hydrophobic contacts of YKJ with Hsp90 (Fig. 7B and 8). Thus, N51, A55 and M98 independently contribute the interaction energies of −2.11, −0.68 and −2.38 kcal mol−1 to the YKJ binding to Hsp90 (Fig. 6B). It is observed that an additional interaction of −1.17 kcal mol−1 occurs between YK9 and I104 (Fig. 6B and 8), which is in consistence with scanning of hydrophobic contacts on MD trajectories (Fig. 7B). This interaction structurally comes from the CH–π interaction of the alkyl in I104 with the ring R2 of YKJ.
By comparison with YK9, YKI also generates similar interactions with six residues L107, G108, F138, Y139, W162 and F170 (Fig. 6C), which agree well with scanning of hydrophobic contacts between YKI and Hsp90 (Fig. 7C and 8). A common hydrogen bond with the high occupancy of 98.80% is detected between the oxygen atom OH of Y139 and the nitrogen atom N3 of YKI (Table 2, Fig. S5C and S6†). It is found that the substitution of the group G2 (Fig. 1E) brings several additional interactions of YKI with five residues N51, A55, I96, M98 and L103. Among these five residues, the carbon atom of N51 and the alkyl of L103 separately produce the CH–π interactions with the rings R4 and R3 (Fig. 9C), moreover the nitrogen atom ND2 of N51 and the oxygen atom O of L103 form the hydrogen bonding interactions with the nitrogen atoms N3 and N2 of YKI (Fig. S5C, S6 and S7C†), respectively, corresponding to the occupancy of 43.28% and 64.31% (Table 2). Totally, N51 and L103 independently contribute the interaction energies of −1.33 and −3.02 kcal mol−1 to the YKI binding to Hsp90 (Fig. 6C). As shown in Fig. 9C, the alkyls of A55, I96 and M98 structurally close the G2 group (namely the hydrophobic ring R4) and are easy to produce the CH–π interactions between them. A55, I96 and M98 respectively provide the energy contributions of −1.00, −1.25 and −2.77 kcal mol−1 to the YKI association with Hsp90 (Fig. 6C). The current analysis based on identifying the hot spot of the inhibitor–residue interactions basically agrees with the experimental results from Vallée et al.64
Based on the above analyses of the residue-based free energy decomposition and scanning of hydrophobic contacts and hydrogen bond contacts between inhibitors and Hsp90, the CH–π, π–π and hydrogen bonding interactions play significant role in bindings of inhibitors to Hsp90. Six common residues L107, G108, F138, Y139, W162 and F170 are identified as the hot spot involving the interactions of YK9, YKJ and YKI with Hsp90. Compared to YK9, the substitution of the group G1 in YKJ not only leads to hydrogen bonding interactions with G97 and L103, but also produces hydrophobic interactions with N51, A55, M98 and I104. Similar to YKJ, the introduction of the Group G2 into YKI also brings two hydrogen bonding interactions with N51 and L103, meanwhile generates hydrophobic interactions with N51, A55, M98 and L103. These additional interactions induced by the substitutions of G1 and G2 can be used as new drug target toward Hsp90.
4 Conclusions
In this work, 200 ns MD simulations were performed on the apo Hsp90 and three inhibitor–Hsp90 complexes to explore binding modes of inhibitors to Hsp90. The RMSFs demonstrate that the associations of inhibitors heavily affect the flexibility of Hsp90. The equilibrated MD trajectories were used for PC analysis and the results suggest that inhibitor bindings produce important effect on the conformational changes, internal dynamics and motion modes of Hsp90, especially for the helix α1 and the loops L1 and L2. Binding affinities of inhibitors to Hsp90 were computed based on 200 snapshots extracted from the equilibrated MD trajectories and the results show that binding ability of YKJ and YKI is stronger than YK9. Furthermore, the substitutions of two groups G1 and G2 in YKJ and YKI not only increase the van der Waals interactions of YKJ and YKI with Hsp90 compared to YK9, but also improve the electrostatics interactions of YKJ and YKI with Hsp90. According to scanning of inhibitor–residue interactions and residue-based on free energy decomposition, the substitution of the group G1 in YK9 not only leads to two additional hydrogen bonding interactions with G97 and L103, but also produces hydrophobic interactions with N51, A55, M98 and I104 compared to YK9. The introduction of the Group G2 into YKI also brings two hydrogen bonding interactions with N51 and L103, meanwhile generates hydrophobic interactions with N51, A55, M98 and L103. We also expect that this study can contribute theoretical hints for design of potent inhibitors toward Hsp90.
Conflicts of interest
There are no conflicts to declare.
Acknowledgements
This work is supported by the National Key Research and Development Program (Grant 2016YFA0502301), the National Natural Science Foundation of China (21403283, 11274206, 11504206, 81273435, and 81573350). Shandong Province Key Research and Development Program (No. 2016GGX102043) and Shandong Provincial Natural Science Foundation (ZR2017MA040 and ZR2015AL022). A Project of Shandong Province Higher Educational Science and Technology Program (No. J18KA040, J17KA045), major development projects of Shandong Jiaotong University and CAS Key Laboratory of Receptor Research (SIMM1706YKF-03).
References
- M. Taipale, D. F. Jarosz and S. Lindquist, Nat. Rev. Mol. Cell Biol., 2010, 11, 515–528 CrossRef PubMed.
- T. Didenko, A. M. S. Duarte, G. E. Karagöz and S. G. D. Rüdiger, Biochim. Biophys. Acta, Mol. Cell Res., 2012, 1823, 636–647 CrossRef PubMed.
- M. E. Feder and G. E. Hofmann, Annu. Rev. Physiol., 1999, 61, 243–282 CrossRef PubMed.
- C. Garrido, S. Gurbuxani, L. Ravagnan and G. Kroemer, Biochem. Biophys. Res. Commun., 2001, 286, 433–442 CrossRef PubMed.
- L. Neckers and P. Workman, Clin. Cancer Res., 2012, 18, 64–76 CrossRef PubMed.
- L. H. Pearl and C. Prodromou, Annu. Rev. Biochem., 2006, 75, 271–294 CrossRef PubMed.
- A. Maloney and P. Workman, Expert Opin. Biol. Ther., 2002, 2, 3–24 CrossRef PubMed.
- D. L. Penkler, C. Atilgan and Ö. Tastan Bishop, J. Chem. Inf. Model., 2018, 58, 383–404 CrossRef PubMed.
- F. H. Schopf, M. M. Biebl and J. Buchner, Nat. Rev. Mol. Cell Biol., 2017, 18, 345–360 CrossRef PubMed.
- T. Taldone, S. O. Ochiana, P. D. Patel and G. Chiosis, Trends Pharmacol. Sci., 2014, 35, 592–603 CrossRef PubMed.
- K. Moulick, J. H. Ahn, H. Zong, A. Rodina, L. Cerchietti, E. M. Gomes DaGama, E. Caldas-Lopes, K. Beebe, F. Perna, K. Hatzi, L. P. Vu, X. Zhao, D. Zatorska, T. Taldone, P. Smith-Jones, M. Alpaugh, S. S. Gross, N. Pillarsetty, T. Ku, J. S. Lewis, S. M. Larson, R. Levine, H. Erdjument-Bromage, M. L. Guzman, S. D. Nimer, A. Melnick, L. Neckers and G. Chiosis, Nat. Chem. Biol., 2011, 7, 818 CrossRef PubMed.
- V. C. H. Da Silva and C. H. I. Ramos, J. Proteomics, 2012, 75, 2790–2802 CrossRef PubMed.
- J. Travers, S. Sharp and P. Workman, Drug Discovery Today, 2012, 17, 242–252 CrossRef PubMed.
- H. Misun, M. Luigi and L. Bo, Curr. Med. Chem., 2009, 16, 3081–3092 CrossRef.
- S. M. Roe, C. Prodromou, R. O'Brien, J. E. Ladbury, P. W. Piper and L. H. Pearl, J. Med. Chem., 1999, 42, 260–266 CrossRef PubMed.
- M. A. Biamonte, J. Shi, K. Hong, D. C. Hurst, L. Zhang, J. Fan, D. J. Busch, P. L. Karjian, A. A. Maldonado, J. L. Sensintaffar, Y.-C. Yang, A. Kamal, R. E. Lough, K. Lundgren, F. J. Burrows, G. A. Timony, M. F. Boehm and S. R. Kasibhatla, J. Med. Chem., 2006, 49, 817–828 CrossRef PubMed.
- M. A. Biamonte, R. Van de Water, J. W. Arndt, R. H. Scannevin, D. Perret and W.-C. Lee, J. Med. Chem., 2010, 53, 3–17 CrossRef PubMed.
- J. R. Porter, C. C. Fritz and K. M. Depew, Curr. Opin. Chem. Biol., 2010, 14, 412–420 CrossRef PubMed.
- S. R. Kasibhatla, K. Hong, M. A. Biamonte, D. J. Busch, P. L. Karjian, J. L. Sensintaffar, A. Kamal, R. E. Lough, J. Brekken, K. Lundgren, R. Grecko, G. A. Timony, Y. Ran, R. Mansfield, L. C. Fritz, E. Ulm, F. J. Burrows and M. F. Boehm, J. Med. Chem., 2007, 50, 2767–2778 CrossRef PubMed.
- C.-H. Yi, J.-Z. Chen, S.-H. Shi, G.-D. Hu and Q.-G. Zhang, Mol. Simul., 2010, 36, 454–460 CrossRef.
- P. A. Brough, W. Aherne, X. Barril, J. Borgognoni, K. Boxall, J. E. Cansfield, K.-M. J. Cheung, I. Collins, N. G. M. Davies, M. J. Drysdale, B. Dymock, S. A. Eccles, H. Finch, A. Fink, A. Hayes, R. Howes, R. E. Hubbard, K. James, A. M. Jordan, A. Lockie, V. Martins, A. Massey, T. P. Matthews, E. McDonald, C. J. Northfield, L. H. Pearl, C. Prodromou, S. Ray, F. I. Raynaud, S. D. Roughley, S. Y. Sharp, A. Surgenor, D. L. Walmsley, P. Webb, M. Wood, P. Workman and L. Wright, J. Med. Chem., 2008, 51, 196–218 CrossRef PubMed.
- K. H. Huang, J. M. Veal, R. P. Fadden, J. W. Rice, J. Eaves, J.-P. Strachan, A. F. Barabasz, B. E. Foley, T. E. Barta, W. Ma, M. A. Silinski, M. Hu, J. M. Partridge, A. Scott, L. G. DuBois, T. Freed, P. M. Steed, A. J. Ommen, E. D. Smith, P. F. Hughes, A. R. Woodward, G. J. Hanson, W. S. McCall, C. J. Markworth, L. Hinkley, M. Jenks, L. Geng, M. Lewis, J. Otto, B. Pronk, K. Verleysen and S. E. Hall, J. Med. Chem., 2009, 52, 4288–4305 CrossRef PubMed.
- P.-P. Kung, B. Huang, G. Zhang, J. Z. Zhou, J. Wang, J. A. Digits, J. Skaptason, S. Yamazaki, D. Neul, M. Zientek, J. Elleraas, P. Mehta, M.-J. Yin, M. J. Hickey, K. S. Gajiwala, C. Rodgers, J. F. Davies and M. R. Gehring, J. Med. Chem., 2010, 53, 499–503 CrossRef PubMed.
- P.-P. Kung, L. Funk, J. Meng, M. Collins, J. Z. Zhou, M. Catherine Johnson, A. Ekker, J. Wang, P. Mehta, M.-J. Yin, C. Rodgers, J. F. Davies, E. Bayman, T. Smeal, K. A. Maegley and M. R. Gehring, Bioorg. Med. Chem. Lett., 2008, 18, 6273–6278 CrossRef PubMed.
- C. W. Murray, M. G. Carr, O. Callaghan, G. Chessari, M. Congreve, S. Cowan, J. E. Coyle, R. Downham, E. Figueroa, M. Frederickson, B. Graham, R. McMenamin, M. A. O'Brien, S. Patel, T. R. Phillips, G. Williams, A. J. Woodhead and A. J. A. Woolford, J. Med. Chem., 2010, 53, 5942–5955 CrossRef PubMed.
- A. J. Woodhead, H. Angove, M. G. Carr, G. Chessari, M. Congreve, J. E. Coyle, J. Cosme, B. Graham, P. J. Day, R. Downham, L. Fazal, R. Feltell, E. Figueroa, M. Frederickson, J. Lewis, R. McMenamin, C. W. Murray, M. A. O'Brien, L. Parra, S. Patel, T. Phillips, D. C. Rees, S. Rich, D.-M. Smith, G. Trewartha, M. Vinkovic, B. Williams and A. J. A. Woolford, J. Med. Chem., 2010, 53, 5956–5969 CrossRef PubMed.
- D. A. Proia and R. C. Bates, Cancer Res., 2014, 74, 1294–1300 CrossRef PubMed.
- H.-P. Sun, J.-M. Jia, F. Jiang, X.-L. Xu, F. Liu, X.-K. Guo, B. Cherfaoui, H.-Z. Huang, Y. Pan and Q.-D. You, Eur. J. Med. Chem., 2014, 79, 399–412 CrossRef PubMed.
- E. Tzanetou, S. Liekens, K. M. Kasiotis, G. Melagraki, A. Afantitis, N. Fokialakis and S. A. Haroutounian, Eur. J. Med. Chem., 2014, 81, 139–149 CrossRef PubMed.
- E. Casale, N. Amboldi, M. G. Brasca, D. Caronni, N. Colombo, C. Dalvit, E. R. Felder, G. Fogliatto, A. Galvani, A. Isacchi, P. Polucci, L. Riceputi, F. Sola, C. Visco, F. Zuccotto and F. Casuscelli, Bioorg. Med. Chem., 2014, 22, 4135–4150 CrossRef PubMed.
- D. Audisio, D. Methy-Gonnot, C. Radanyi, J.-M. Renoir, S. Denis, F. Sauvage, J. Vergnaud-Gauduchon, J.-D. Brion, S. Messaoudi and M. Alami, Eur. J. Med. Chem., 2014, 83, 498–507 CrossRef PubMed.
- J. Trepel, M. Mollapour, G. Giaccone and L. Neckers, Nat. Rev. Cancer, 2010, 10, 537 CrossRef PubMed.
- M. Mickler, M. Hessling, C. Ratzke, J. Buchner and T. Hugel, Nat. Struct. Mol. Biol., 2009, 16, 281 CrossRef PubMed.
- K. Richter, J. Soroka, L. Skalniak, A. Leskovar, M. Hessling, J. Reinstein and J. Buchner, J. Biol. Chem., 2008, 283, 17757–17765 CrossRef PubMed.
- M. Hessling, K. Richter and J. Buchner, Nat. Struct. Mol. Biol., 2009, 16, 287–293 CrossRef PubMed.
- T. O. Street, L. A. Lavery and D. A. Agard, Mol. Cell, 2011, 42, 96–105 CrossRef PubMed.
- T. O. Street, K. A. Krukenberg, J. Rosgen, D. W. Bolen and D. A. Agard, Protein Sci., 2010, 19, 57–65 Search PubMed.
- M. Amaral, D. B. Kokh, J. Bomke, A. Wegener, H. P. Buchstaller, H. M. Eggenweiler, P. Matias, C. Sirrenberg, R. C. Wade and M. Frech, Nat. Commun., 2017, 8, 2276 CrossRef PubMed.
- S. Lu, H. Jang, S. Muratcioglu, A. Gursoy, O. Keskin, R. Nussinov and J. Zhang, Chem. Rev., 2016, 116, 6607–6665 CrossRef PubMed.
- P. Wang, T. Fu, X. Zhang, F. Yang, G. Zheng, W. Xue, Y. Chen, X. Yao and F. Zhu, Biochim. Biophys. Acta, Gen. Subj., 2017, 1861, 2766–2777 CrossRef PubMed.
- G. Li, H. Shen, D. Zhang, Y. Li and H. Wang, J. Chem. Theory Comput., 2016, 12, 676–693 CrossRef PubMed.
- T. Hou, W. A. McLaughlin and W. Wang, Proteins: Struct., Funct., Genet., 2008, 71, 1163–1174 CrossRef PubMed.
- G. Hu, A. Ma, X. Dou, L. Zhao and J. Wang, Int. J. Mol. Sci., 2016, 17, 819 CrossRef PubMed.
- L. Duan, G. Feng, X. Wang, L. Wang and Q. Zhang, Phys. Chem. Chem. Phys., 2017, 19, 10140–10152 RSC.
- M. Yang, X. Zhang and K. Han, Proteins: Struct., Funct., Genet., 2010, 78, 2222–2237 CrossRef PubMed.
- H. Sun, Y. Li, M. Shen, D. Li, Y. Kang and T. Hou, J. Chem. Inf. Model., 2017, 57, 1895–1906 CrossRef PubMed.
- L. Shao-Yong, H. Zhi-Min, H. Wen-Kang, L. Xin-Yi, C. Ying-Yi, S. Ting and Z. Jian, Proteins, 2013, 81, 740–753 CrossRef PubMed.
- E. L. Wu, K.-Y. Wong, X. Zhang, K. Han and J. Gao, J. Phys. Chem. B, 2009, 113, 2477–2485 CrossRef PubMed.
- T. Ichiye and M. Karplus, Proteins: Struct., Funct., Genet., 1991, 11, 205–217 CrossRef PubMed.
- J. Chen, J. Wang and W. Zhu, Phys. Chem. Chem. Phys., 2017, 19, 3067–3075 RSC.
- J. Su, X. Liu, S. Zhang, F. Yan, Q. Zhang and J. Chen, J. Biomol. Struct. Dyn., 2018, 36, 1212–1224 CrossRef PubMed.
- J. Chen, RSC Adv., 2016, 6, 58573–58585 RSC.
- L.-Q. Yang, P. Sang, R.-P. Zhang and S.-Q. Liu, RSC Adv., 2017, 7, 42094–42104 RSC.
- L. Duan, X. Liu and J. Z. H. Zhang, J. Am. Chem. Soc., 2016, 138, 5722–5728 CrossRef PubMed.
- J. Chen, J. Wang and W. Zhu, Phys. Chem. Chem. Phys., 2017, 19, 30239–30248 RSC.
- D. Shi, Q. Bai, S. Zhou, X. Liu, H. Liu and X. Yao, Proteins: Struct., Funct., Genet., 2018, 86, 43–56 CrossRef PubMed.
- G. Hu, A. Ma and J. Wang, J. Chem. Inf. Model., 2017, 57, 918–928 CrossRef PubMed.
- T. Hou, J. Wang, Y. Li and W. Wang, J. Comput. Chem., 2011, 32, 866–877 CrossRef PubMed.
- L. L. Duan, G. Q. Feng and Q. G. Zhang, Sci. Rep., 2016, 6, 31488 CrossRef PubMed.
- G. Hu, S. Xu and J. Wang, Chem. Biol. Drug Des., 2015, 86, 1351–1359 CrossRef PubMed.
- G. Colombo, G. Morra, M. Meli and G. Verkhivker, Proc. Natl. Acad. Sci. U. S. A., 2008, 105, 7976–7981 CrossRef PubMed.
- G. Vettoretti, E. Moroni, S. Sattin, J. Tao, D. A. Agard, A. Bernardi and G. Colombo, Sci. Rep., 2016, 6, 23830 CrossRef PubMed.
- G. Morra, G. Verkhivker and G. Colombo, PLoS Comput. Biol., 2009, 5, e1000323 CrossRef PubMed.
- F. Vallée, C. Carrez, F. Pilorge, A. Dupuy, A. Parent, L. Bertin, F. Thompson, P. Ferrari, F. Fassy, A. Lamberton, A. Thomas, R. Arrebola, S. Guerif, A. Rohaut, V. Certal, J.-M. Ruxer, C. Delorme, A. Jouanen, J. Dumas, C. Grépin, C. Combeau, H. Goulaouic, N. Dereu, V. Mikol, P. Mailliet and H. Minoux, J. Med. Chem., 2011, 54, 7206–7219 CrossRef PubMed.
- J. Wang, P. Morin, W. Wang and P. A. Kollman, J. Am. Chem. Soc., 2001, 123, 5221–5230 CrossRef PubMed.
- W. Wang and P. A. Kollman, J. Mol. Biol., 2000, 303, 567–582 CrossRef PubMed.
- W. Wang and P. A. Kollman, Proc. Natl. Acad. Sci. U. S. A., 2001, 98, 14937–14942 CrossRef PubMed.
- C. Wang, D. A. Greene, L. Xiao, R. Qi and R. Luo, Front. Mol. Biosci., 2018, 4, 1–18 Search PubMed.
- H. Sun, Y. Li, M. Shen, S. Tian, L. Xu, P. Pan, Y. Guan and T. Hou, Phys. Chem. Chem. Phys., 2014, 16, 22035–22045 RSC.
- H. Sun, Y. Li, S. Tian, L. Xu and T. Hou, Phys. Chem. Chem. Phys., 2014, 16, 16719–16729 RSC.
- J. Wang, Q. Shao, B. P. Cossins, J. Shi, K. Chen and W. Zhu, J. Biomol. Struct. Dyn., 2016, 34, 163–176 CrossRef PubMed.
- H. Li, A. D. Robertson and J. H. Jensen, Proteins: Struct., Funct., Genet., 2005, 61, 704–721 CrossRef PubMed.
- D. C. Bas, D. M. Rogers and J. H. Jensen, Proteins: Struct., Funct., Genet., 2008, 73, 765–783 CrossRef PubMed.
- D. A. Case, R. M. Betz, D. S. Cerutti, T. E. Cheatham, T. A. Darden, R. E. Duke, T. J. Giese, H. Gohlke, A. W. Goetz, N. Homeyer, S. Izadi, P. Janowski, J. Kaus, A. Kovalenko, T. S. Lee, S. LeGrand, P. Li, C. Lin, T. Luchko, R. Luo, B. Madej, D. Mermelstein, K. M. Merz, G. Monard, H. Nguyen, H. T. Nguyen, I. Omelyan, A. Onufriev, D. R. Roe, A. Roitberg, C. Sagui, C. L. Simmerling, W. M. Botello-Smith, J. Swails, R. C. Walker, J. Wang, R. M. Wolf, X. Wu, L. Xiao and P. A. Kollman, 2016, AMBER 2016, University of California, San Francisco Search PubMed.
- J. A. Maier, C. Martinez, K. Kasavajhala, L. Wickstrom, K. E. Hauser and C. Simmerling, J. Chem. Theory Comput., 2015, 11, 3696–3713 CrossRef PubMed.
- W. L. Jorgensen, J. Chandrasekhar, J. D. Madura, R. W. Impey and M. L. Klein, J. Chem. Phys., 1983, 79, 926–935 CrossRef.
- M. J. Frisch, G. W. Trucks, H. B. Schlegel, G. E. Scuseria, M. A. Robb, J. R. Cheeseman, G. Scalmani, V. Barone, B. Mennucci, G. A. Petersson, H. Nakatsuji, M. Caricato, X. Li, H. P. Hratchian, A. F. Izmaylov, J. Bloino, G. Zheng, J. L. Sonnenberg, M. Hada, M. Ehara, K. Toyota, R. Fukuda, J. Hasegawa, M. Ishida, T. Nakajima, Y. Honda, O. Kitao, H. Nakai, T. Vreven, J. A. Montgomery Jr, J. E. Peralta, F. Ogliaro, M. Bearpark, J. J. Heyd, E. Brothers, K. N. Kudin, V. N. Staroverov, R. Kobayashi, J. Normand, K. Raghavachari, A. Rendell, J. C. Burant, S. S. Iyengar, J. Tomasi, M. Cossi, N. Rega, J. M. Millam, M. Klene, J. E. Knox, J. B. Cross, V. Bakken, C. Adamo, J. Jaramillo, R. Gomperts, R. E. Stratmann, O. Yazyev, A. J. Austin, R. Cammi, C. Pomelli, J. W. Ochterski, R. L. Martin, K. Morokuma, V. G. Zakrzewski, G. A. Voth, P. Salvador, J. J. Dannenberg, S. Dapprich, A. D. Daniels, O. Farkas, J. B. Foresman, J. V. Ortiz, J. Cioslowski and D. J. Fox, Gaussian 09, revision C.01, Gaussian, Inc., Wallingford, CT, 2009 Search PubMed.
- J. Wang, R. M. Wolf, J. W. Caldwell, P. A. Kollman and D. A. Case, J. Comput. Chem., 2004, 25, 1157–1174 CrossRef PubMed.
- J.-P. Ryckaert, G. Ciccotti and H. J. C. Berendsen, J. Comput. Phys., 1977, 23, 327–341 CrossRef.
- J. A. Izaguirre, D. P. Catarello, J. M. Wozniak and R. D. Skeel, J. Chem. Phys., 2001, 114, 2090–2098 CrossRef.
- D. R. Roe and T. E. Cheatham, J. Chem. Theory Comput., 2013, 9, 3084–3095 CrossRef PubMed.
- J. Chen, J. Wang, W. Zhu and G. Li, J. Comput.-Aided Mol. Des., 2013, 27, 965–974 CrossRef PubMed.
- E-mail: http://www.pymol.org.
- W. Humphrey, A. Dalke and K. Schulten, J. Mol. Graphics, 1996, 14, 33–38 CrossRef PubMed.
- A. Onufriev, D. Bashford and D. A. Case, Proteins: Struct., Funct., Genet., 2004, 55, 383–394 CrossRef PubMed.
- H. Gohlke, C. Kiel and D. A. Case, J. Mol. Biol., 2003, 330, 891–913 CrossRef PubMed.
- B. R. Miller, T. D. McGee, J. M. Swails, N. Homeyer, H. Gohlke and A. E. Roitberg, J. Chem. Theory Comput., 2012, 8, 3314–3321 CrossRef PubMed.
- T. Ichiye and M. Karplus, Proteins: Struct., Funct., Genet., 1991, 11, 205–217 CrossRef PubMed.
- C. Jianzhong, Chem. Biol. Drug Des., 2017, 89, 548–558 CrossRef PubMed.
- A. C. Wallace, R. A. Laskowski and J. M. Thornton, Protein Eng., Des. Sel., 1995, 8, 127–134 CrossRef.
Footnote |
† Electronic supplementary information (ESI) available. See DOI: 10.1039/c8ra05042b |
|
This journal is © The Royal Society of Chemistry 2018 |
Click here to see how this site uses Cookies. View our privacy policy here.