DOI:
10.1039/C8RA01854E
(Paper)
RSC Adv., 2018,
8, 23629-23647
Design and virtual screening of novel fluoroquinolone analogs as effective mutant DNA GyrA inhibitors against urinary tract infection-causing fluoroquinolone resistant Escherichia coli†
Received
2nd March 2018
, Accepted 21st June 2018
First published on 29th June 2018
Abstract
Fluoroquinolones (FQs) belong to the class of quinolone drugs that are used to treat Urinary tract infections (UTIs) through inhibition of E. coli DNA gyrase. Resistance to FQs poses a serious problem in the treatment against resistant strains of E. coli which are associated with Ser83 to Leu and Asp87 to Asn mutations at the quinolone resistance determining region (QRDR) of the GyrA subunit of DNA gyrase. Mutant DNA GyrA (mtDNA GyrA) is deemed to be a significant target for the development of novel FQ drugs. Due to resistance to FQ drugs, discovery or development of novel FQs is crucial to inhibit the mtDNA GyrA. Hence, the present study attempts to design and develop novel FQs that are efficient against resistant E. coli strains. A three-dimensional structure of the mtDNA GyrA protein was developed by homology modeling, following which 204 novel FQ analogs were designed using target based SAR. The designed ligands were then screened using molecular docking studies, through which the pattern of interaction between the ligands and the target protein was studied. As expected, the results of the docking study revealed that the molecules FQ-147, FQ-151 and FQ-37 formed hydrogen bonding and Van der Waals interactions with Leu83 and Asn87 (mutated residues), respectively. Further, the wild-type (WT), mtDNA GyrA and docking complex were studied by molecular dynamics (MD) simulations. Subsequently, all the screened compounds were subjected to a structure and ligand based pharmacophore study followed by ADMET and toxicity (TOPKAT) prediction. Finally, eighteen hit FQ analogs which showed good results for the following properties, viz., best binding score, estimated activity (MIC value) and calculated drug-like properties, and least toxicity, were shortlisted and identified as potential leads to treat UTI caused by FQ resistant E. coli. Apart from development of novel drug candidates for inhibition of mtDNA GyrA, the present study also contributes towards a superior comprehension of the interaction pattern of ligands in the target protein. To a more extensive degree, the present work will be useful for the rational design of novel and potent drugs for UTIs.
Introduction
Urinary tract infections (UTIs) exemplify some of the most common bacterial contagions worldwide affecting 150 million people annually. Recurrence of UTI, occurring in ∼25–30% of women, is another major problem and has made treating UTIs particularly challenging; also, treatment options are limited owing to the emergence of multidrug-resistant strains of infection-causing bacteria, especially Escherichia coli (E. coli).1 Fluoroquinolones (FQ) are potent and powerful broad-spectrum antibiotics commonly used to treat UTIs caused by E. coli in hospitalized patients.2 E. coli DNA gyrase is one of the primary targets of FQs. They act by inhibiting DNA gyrase which subsequently controls DNA supercoiling, where FQ forms higher levels of FQ–gyrase-DNA complex in E. coli, thereby inhibiting its replication and transcription. Thus, elevated concentrations of these FQs prove to be lethal to the infection causing E. coli.3 Despite the fact, extensive and improper use of FQs by UTI patients has led to an increasing FQ resistance (FQR) in E. coli due to mutations in the GyrA subunit of DNA gyrase.2,4 Further, the increasing rate of FQR against UTIs in recent years is a major concern among the healthcare community, which projects a wide variation in the range of 6–75% from country to country.5 In 2015, Center for Disease Dynamics, Economics & Policy (CDDEP) reported FQR E. coli isolates from UTI patients in India (78%), United States (29%), South Africa (28%), United Kingdom (16%) and Australia (13%), which is indicative of the fact that UTI causing FQR E. coli is a serious problem worldwide, with India posing the risk of highest prevalence.6
Several previous studies on clinical isolates of E. coli have shown that majority of the resistant strains were mutated within the amino acid residues spanning from 67 and 106 (inclusive) of DNA GyrA which is termed as the quinolone resistant-determining region (QRDR).7 The spontaneous mutations at Ser83Leu and Asp87Asn in the QRDR region of GyrA are reported to be responsible for FQR in E. coli that were isolated from UTI patients.8,9 Mutation of these important residues results in ∼ten-fold decrease in binding affinity of FQs to the gyrase-DNA complex in E. coli and confers high level resistance to FQs10,11 due to which, management of serious UTIs with currently available FQs has become increasingly challenging. Emergence of FQR E. coli strains creates an increasing demand for development of novel and potent FQ derivatives for effective UTI therapeutics. However, there is no experimental evidence for the structure of mutant E. coli DNA GyrA (mtDNA GyrA) protein which makes development of target specific inhibitors even more complicated. With motivation from the above information, 3-D model of the mtDNA GyrA was developed using homology modeling, followed by which, novel FQ analogs were designed based on the structure–activity relationship (SAR) to study its interaction with the modeled mtDNA GyrA protein to develop drug candidates that can treat UTIs caused by resistant E. coli strains.
As a part of the present drug development study, in silico docking has been exploited with a goal to elucidate configurational information of mtDNA GyrA, determine its relative binding strength and the nature of interactions with the newly designed FQ analogs. Finally, the superlative FQ analogs among the designed compounds were identified through an integrated procedure of virtual screening and hit optimization with combined pharmacophore – ADMET-toxicity analysis.
Methodology
All the in silico studies (Homology modelling, Molecular dynamic simulation, Molecular docking, Pharmacophore, ADMET and TOPKAT) were carried out using BIOVIA Discovery Studio (DS) version 2017.12 The schematic view of this study is depicted in Fig. 1. All the figures in this manuscript are novel and developed by DS and OrginPro2015 software.
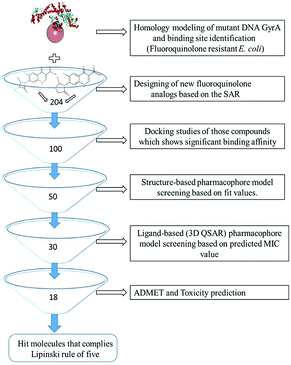 |
| Fig. 1 Virtual screening protocol for identification of novel mutant DNA GyrA inhibitor. | |
Homology modeling
The 3D-crystal structure of the target protein mutant GyrA of FQR E. coli was not available in the Protein Data Bank (PDB). Hence, it was determined using homology modeling technique. Mutant GyrA amino acid sequence (875aa) for FQR E. coli strain SMS-3-5 was retrieved from Uniport database with id B1LKX7. This strain exhibits mutations in both Ser83(TCG) to Leu(TTG), and Asp87(GAC) to Asn(AAC) in their GyrA sequence resulted in decreased susceptibility to FQs and significantly correlated with FQRs in UTI patents.8,13 Basic Local Alignment Search Tool (BLAST) protocol in DS against PDB was carried out to find an appropriate template, through which, wild-type (WT) E. coli DNA GyrA (PDB ID: 1AB4) was selected. The mutant target sequence was aligned with the template sequence (1AB4) using Align Multiple Sequences protocol. The Build Homology Modeling protocol was used to build the structure with the help of target sequence. Further, the cut overage limit was fixed as 4.5 Å to remove the terminal-unaligned residues in the model sequence. Additionally, disulfide bridges, cis-prolines and additional restraints were not included in order to simplify the homology model of mtDNA GyrA. Using high resolution discrete optimized protein energy (DOPE) method, the loop was refined at high optimized level. Finally, one best model was chosen according to the lowest DOPE score, where, lower DOPE score represents structures with superior stable 3D conformation.14
Model evaluation
The generated mtDNA GyrA model was superimposed with template structure by Align and Superimpose Protein protocol to calculate the root mean square deviation (RMSD) of coordinates between homology model and template structure. The model was then optimized by energy minimization technique using CHARMm force field with a gradient 0.1 kcal mol−1 Å−1 in order to remove any steric clash within the amino acid side chains. The accuracy of predicted model and its stereochemical properties were evaluated using Ramachandran plot and overall goodness factor (G-factor) using PROCHECK.15 In addition, the model was analysed by ProQ,16 ERRAT,17 Verify 3D18 and ProSA19 online servers.
Molecular dynamic simulation studies on modeled protein
Using the refined homology model (mtDNA GyrA) and its FQ complex as the starting structures in femto second (fs) molecular dynamics studies to generate a realistic model of a structure's motion. The above systems were solvated using explicit TIP3P water models to get accurate description. For explicit periodic boundary water simulation, an orthorhombic cell shape was set up and the molecules were positioned at 7 Å minimum distance from the cell boundary. Sodium and chloride ions were added to the systems to keep the system neutral. The Standard Dynamics Cascade protocol applies a set of simulation procedures to the input structure. Typically, it is used to minimize and equilibrate the molecular system and prepare for input to production dynamics using CHARMm algorithm. An initial minimization stage, typically using 1000 steps of the robust steepest descent algorithm and a second minimization stage, typically using the 2000 steps of conjugate gradient method to ensure that a low energy starting point is supplied to subsequent dynamics stages. Each system was energetically minimized and then slowly heated up from 50 to 300 K over a period of 2.0 fs with a harmonic constraint of 0.1 kcal mol−1 Å placed on all backbone atoms. The equilibration stage was performed to equilibrate the systems at a target temperature. A molecular dynamic production run in a suitable NVT thermodynamic ensemble at a given temperature based on the equilibrated system from the previous step. The results of the production stage are stored at 2 ps time intervals. Finally, trajectories generated for mtDNA GyrA and its FQ complex from the production run was analysed and compared.
SAR based design of new fluoroquinolone analogs
Several drugs have been pulled back from late-stage testing due to off-target impacts.20 Consequently, to accomplish selectivity and maintain a strategic distance from side effects, knowledge of related binding site is also important. About 204 new FQ analogs were designed based on the SAR of the standard FQ drug and binding site (QRDR region) of the modeled mtDNA GyrA protein. All the designed ligands including standard ciprofloxacin were drawn by using ChemDraw Professional 15.0 software. The structural data of the all the ligands were saved in .mol format for further studies. Novelty of the designed compounds was also evaluated using SciFinder (http://www.cas.org) and ChemSpider (http://www.chemspider.com).
Molecular docking
Molecular docking was performed to identify the biologically active hits among the designed ligands where the conformation with the lowest binding energy is considered to form stable complex inside the active site of mtDNA GyrA. This study was performed using CDOCKER, which is a grid-based molecular docking tool that employs CHARMm force field. The CDOCKER score is expressed as negative value (i.e., -CDOCKER_ENERGY) where higher value indicates a more favorable binding. The CDOCKER energy was calculated from the H-bonds, van der Waals and electrostatic interactions between the target protein and the ligand.
Binding site of the modeled protein was fixed based on the crystal data of the template protein. The binding site sphere center was set at 9 Å radius which would facilitate interaction of the ligands with mutated amino acids. Further, CHARMm force field was applied followed by energy minimization to identify local minima (lowest energy conformation) of the modeled mtDNA GyrA with an energy gradient of 0.1 kcal mol−1 Å−1, using smart minimizer algorithm.
The energy minimized receptor protein and the set of 204 designed structures with the binding site sphere radius set at X = 29.50, Y = −31.38 and Z = −38.79 were submitted to the CDOCKER parameter. The sphere encompassed 48 amino acids starting from 61st to 134th residue in the mtDNA GyrA including the mutated residues. Default setting was retained for the remaining scoring parameters. Ligands with lowest docking score were shortlisted for further pharmacophore analysis.
To get concord compounds, LibDock docking method was performed. This algorithm aligned the ligand conformation to hotspots (polar and apolar) of receptor interaction sites and the and retained best scoring poses of ligands.21 Ligand conformations can be pre-calculated and generated on the fly using catalyst algorithm. The Max Hits parameter was set as 1, which means, the highest LibDock score of each compound was saved. The remaining parameters were kept as default. Ligands with lowest-CDOCKER energy and highest LibDock score were shortlisted for further pharmacophore analysis.
Pharmacophore analysis
Pharmacophore models provide a rational explanation of how structurally diverse ligands could specifically bind to the active site of the target protein. In the present work, structure-based and ligand-based pharmacophore modeling approaches were applied to identify the functional groups and respective features which are responsible for the selective activity of the designed ligands against the mtDNA GyrA of the resistant E. coli strains.
Structure-based pharmacophore modeling
Structure-based pharmacophore (SBP) study analyzes the active site residues of mtDNA GyrA, extracts pharmacophore query from the Ludi interaction map which is created inside the active site sphere and assigns only three main features namely hydrogen bond acceptor (HBA), hydrogen bond donor (HBD) and hydrophobic (HY) features. In Interaction Generation protocol, mtDNA GyrA was used as input receptor with binding site sphere (9 Å radius) set at X = 16.13, Y = −35.25 and Z = −29.55. Subsequently, the density of polar sites and lipophilic sites values was fixed at 25 to specify the density of the vectors in the interaction site for hydrogen bonds and points in the interaction site for lipophilic atoms respectively. The Edit and Cluster Pharmacophore tool was used to cluster the common pharmacophore properties down to <30 features.
Screening of the designed compounds using feature map
The generated SBP model was used to screen the best hits among the selected compounds from docking studies. The screening was accomplished using Screen Library protocol which enumerates several possible pharmacophores from pharmacophore feature model using active training set. This protocol was performed with the flexible fit between designed compound conformations and pharmacophore model with default setup was maintained for other parameters. Geometric fit values were calculated for every hit based on how well the chemical structures of a compound map on to the location constrains of pharmacophore feature and their distance deviation from the feature centers. The fit value of molecules is computed by the equation,
Fit = ∑ mapped hypotheses features × W [1 − ∑(distance/tolerance)2] |
where, W is the weight associated with the feature sphere (default 1.0 Å). The weighting term included the distance between the feature centroid in the sphere and its corresponding chemical groups and tolerance is the radius of the feature sphere (default 1.6 Å).22 Hit compounds that satisfied all the screening tests were filtered and used for further ligand-based pharmacophore (LBP) modeling.
Ligand-based pharmacophore modeling
Data set preparation. For LBP modeling, a set of 10 standard FQ drugs and 20 other FQ derivatives which have been identified and reported to be active against E. coli were collected based on literature. The inhibitory activity of these compounds was expressed as minimum inhibitory concentration (MIC) in μg ml−1. For the data set, 10 standard FQ drugs were chosen as training set compounds and the remaining 20 compounds were considered as test set. The training set was chosen based on the structural diversity and extensive coverage of the activity values which spans a wide range transversely from 0.13 μg ml−1 to 0.0125 μg ml−1.All the training set and test set ligands were prepared using Prepare Ligands protocol in DS. The preparation steps involved conversion of 2D structures to 3D and addition of hydrogen atoms through which a reasonable 3D conformation can be generated followed by energy minimization. All the compounds were minimized using the smart minimizer algorithm with maximum steps set at 200 and RMS gradient value of 0.1 kcal mol−1. A principle attribute was added in the property of the molecule and the value was set as 2, 1 and 0 for most, moderate and least active molecules, respectively. Similarly, maximum omit feature (MaxomitFeat) value was assigned 0, 1 and 2 for most, moderate and least active molecules, respectively.
Pharmacophore model generation
An initial analysis of the training set compounds using the Common Feature Pharmacophore Generation protocol was performed, which revealed that HBA, HY and ring aromatic (RA) features of active training set compounds were crucial for antagonistic properties of DNA GyrA. On this basis, HBA, HY and RA were selected to generate standard pharmacophore model (Hypogen) by using 3D QSAR Pharmacophore Generation protocol. The maximum number of pharmacophore model was set to 5 followed by which minimum and the maximum numbers of selected features were set at 0 and 5, respectively. Simultaneously, best conformation method was selected from three methods viz., fast, best and caesar to generate multiple conformations for each compound present in training set to identify the best map. All other parameters used in this protocol were kept at their default settings. In this study, the top 5 hypothetical structures (Hypogen model) were generated with corresponding statistical values such as configuration, cost value, weight, root mean square deviation (RMSD) and fit values. This protocol also predicts the theoretical MIC value for each training set compounds. Out of the 5 models, 1 best Hypogen model (Hypo 1) was selected using cost analysis. Furthermore, the reliability of a pharmacophore model Hypo 1 was evaluated by superimposing the training set compounds on the Hypo 1 and assessed by correlating the geometric fit. Likewise, the predicted activity of training set compounds correlated with reported activity of the training set compounds.
Pharmacophore model evaluation
The main purpose of validating a quantitative pharmacophore model is to determine its capacity to identify active compounds, as well as, its predictive ability for corresponding molecules. It was performed with test set using Fischer randomization methods.
Test-set method
The prediction ability of Hypo 1 was validated by feature mapping and estimating the activity of 20 test set compounds. This technique is utilized to assess the reliability of the generated pharmacophore model to predict activity of the other compounds apart from the training set compounds and precisely classify them according to their activity scale. The test set compounds and Hypo 1 were subjected to Ligand Pharmacophore Mapping protocol with Flexible Fitting Method to estimate the MIC and the fit value of each test set compounds.
Activity of any compound can be estimated from a Hypogen through the equation,
log(estimated activity) = I + Fit |
where,
I = the intercept of the regression line obtained by plotting the log of the biological activity of the training or test compounds against the Fit values of the training or test set compounds.
22
Fischer randomization method
Significance of the best pharmacophore hypotheses was assessed using the Fischer validation (CatScramble test program) in DS. For the Fisher's randomization test, the desired confidence level was set as 95%, after which, 19 random spreadsheets were constructed.23 This method generates the hypotheses by randomly shuffling the activity value of the training set compounds after which it creates new spreadsheets to validate the strong correlation between chemical structure and biological activity of training set compounds.
Virtual screening of designed compounds
The validated Hypo 1 was used to predict the activity (MIC value) of hit compounds from the result of SBP modeling for which Ligand Pharmacophore Mapping protocol was used. Of the designed compounds, the compounds that are well mapped with the lowest predicted MIC values were shortlisted and subjected to further ADMET and toxicity prediction.
ADMET and toxicity predictions
The selected hit compounds were submitted to ADMET and TOPKAT protocol to assess pharmacokinetics and toxicity properties respectively. The various pharmacokinetic parameters such as, hepatotoxicity levels, aqueous solubility, cytochrome CYP2D6 inhibition, blood–brain-barrier penetration (BBB), plasma protein binding (PPB) and human intestinal absorption (HIA) of each designed compound were evaluated by using ADMET algorithm. These were considered as descriptors of drug-likeness. Further, potential toxicity and degradation products of designed compounds were performed by TOPKAT protocol. TOPKAT employs rigorously developed and validated Quantitative Structure Toxicity Relationship (QSTR) models to forecast toxicological end points regarding probability values.24
Results and discussion
Homology model of mtDNA GyrA
In recent years, homology modeling has become the main alternative to get a 3D representation of the target in the absence of crystal structures. As there was no crystal structure available for mtDNA GyrA of FQR E. coli, it was determined using a homology modeling in DS. The structure was built using WT E. coli DNA GyrA (PDB ID: 1AB4) as template with 98.7% sequence identity (Fig. 2). High sequence identity of the modeled structure with the template confirms precise connectivity of the secondary elements. The mutant model and the WT protein are similar except for minor variation in the loop regions that fall near the site of mutations.
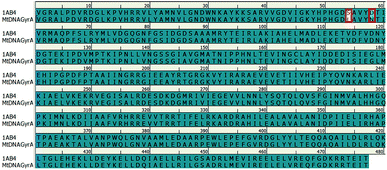 |
| Fig. 2 Pairwise sequence alignment between the amino acid sequence of mtDNA GyrA and that of template 1AB4. Mutated residues indicated in red square box. | |
It was observed that the cavity of mtDNA GyrA was found to be reduced with a size of 2597 Å compared to that of the WT DNA GyrA with cavity size of 2857 Å (Fig. 3), which is attributed to the increased hydrophobic surface area in mtDNA GyrA. The above information paves way for design of potent novel FQ analogs.
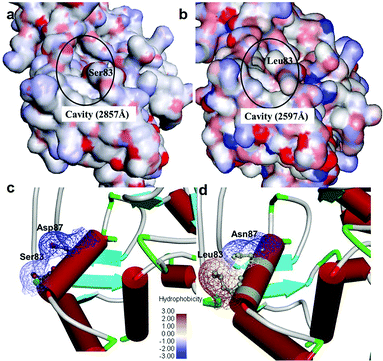 |
| Fig. 3 Cavity site of the (a) wild-type E. coli DNA GyrA and (b) resistant E. coli mtDNA GyrA. This site denoted as a quinolone resistance determining region (QRDR). Hydrophobic nature of the (c) wild-type type and (d) mtDNA GyrA (schematic view) active site. | |
Model evaluation
Modeled structure with the lowest DOPE score (−101452.2 kcal mol−1) was superimposed with template structure (1AB4) and RMSD value (1.32 Å) was calculated (Fig. 4a), the value of which is indicative of a greater backbone similarity between the modeled protein structure and the template. Further, the modeled structure was subjected to validation, where, PROCHECK-NMR-generated the overall G-factor value of 0.4 and Ramachandran plot (Fig. 4b) showed 90.3% of residues located in the most favored region, 8.0% in the additionally allowed region, 0.3% in the generously allowed region and 1.3% in disallowed regions. The mutated residues fell under the allowed region of the Ramachandran plot. These values strongly indicate that the model has good overall stereochemical quality and stability. The ProQ Levitt–Gerstein (LG) score (5.684) and verify 3D–1D average score (≥0.2) (Fig. S1†) showed that the model is an extremely high-quality 3D structure16 and good environmental profile, respectively. Moreover, according to ERRAT analysis, the overall quality factor of the model was 90.096 (Fig. S2†) and that of ProSA (protein structure analysis), the value of Z-score plot was −7.98 (Fig. S3†) which proved that generated model is of reliable quality. All the evidence suggested that the conformation of the backbone, non-bonded interaction and energy profile of the model was well within the range of the high-quality model.
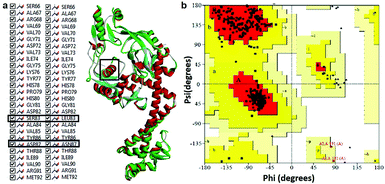 |
| Fig. 4 (a) Superimposition of mtDNA GyrA model (green) with template 1AB4 protein (red). (b) Ramachandran plot for the predicted model mtDNA GyrA. Color code: most favored (red), additionally allowed (yellow), generously allowed (pale yellow) and disallowed regions (white color). | |
SAR based design of new fluoroquinolone analogs
Generally, the bulky heterocyclic ring at the C-7th position in FQ structure has broad spectrum antibacterial activities and is poorly exported from bacterial cell.25 Also, its direct interaction with DNA gyrase affords more rigid drug–protein interactions and impedes the efflux mediated fluoroquinolone resistance.26 Recent studies revealed that substitutions at C-7th position of FQs overcome the protective effect of gyrase mutations in resistant E. coli.3 Based on the above information, 204 novel FQ analogs were designed with two different substitutions of azetidinone and thiazolidinone hetero rings at the C-7th position of FQ skeleton (Table S1†), general structure of the novel FQ analogs depicted in Fig. 5. Additionally, as per the SAR studies the carboxylic acid group and keto group substitution of the FQ core at C-3rd and C-4th position, respectively, is crucial for the interaction of FQ to the DNA GyrA.27
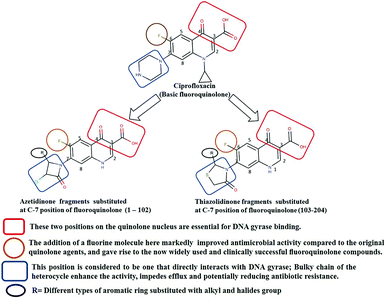 |
| Fig. 5 Design of new fluoroquinolone analogs based on the structure–activity relationship. | |
Any substitution at C-8 and N-1 positions would restrict the rotational freedom of the C-7 rings, which is critical for rigid FQ–gyrase-DNA interaction. Similarly, substitutions at N-1 and C-8 position are also reported to alter core FQ structure, thereby, affecting the FQ activity and lethality.28 Therefore, no alterations were made at C-3rd, C-4th, C-8th and N-1 positions in order to maintain the stereochemical integrity and subsequently property of the lead structure. It is to be noted that most of the mutated residues in the mtDNA GyrA are hydrophobic in nature. Therefore, different hydrophobic fragments (R) were substituted on the azetidinone and thiazolidinone ring at the C-7th position of designed FQs to improve the hydrophobic interaction with the mutated amino acids in the active site region. These data provide functional relevance towards binding of new designed FQ to mtDNA GyrA, thereby, opening a new avenue into understanding FQ–gyrase binding affinity and a novel platform for designing novel FQs that are capacitated to surpass existing FQR.
Molecular docking
Docking study was performed to analyse the binding pattern of the designed compounds with the mtDNA GyrA protein. Among the newly designed 204 FQ derivatives, 100 drug-like hit compounds were selected based on the lowest CDOCKER energy and their binding affinity in comparison with the standard ciprofloxacin (Fig. 6)
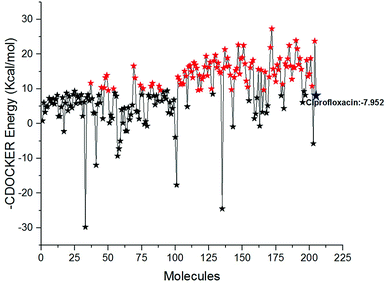 |
| Fig. 6 The-CDOCKER energy score (kcal mol−1) of the 204 newly designed FQ analogs. Color code: red stars indicates selected 100 hit compounds having lower-CDOCKER energy (−9.55 to (−27.324 kcal mol−1). Black stars indicated the higher-CDOCKER energy compounds (−9.47 to +29.7924 kcal mol−1). | |
Interestingly, from the selected 100 molecules, around 92 FQ molecules substituted with azetidinone fragment confined good binding affinity, which suggests that azetidinone fragment moiety at C-7th position in FQ formed more bonded and non-bonded interactions with the active site residues of the target protein, rather than thiazolidinone fragment. The molecule FQ-172 (6-fluoro-4-oxo-7-{4-oxo-2-[4-(pentyloxy) phenyl]-1,3-thiazolidin-3-yl}-1,4-dihydroquinoline-3-carboxylic acid) has four-fold higher affinity (−27.324 kcal mol−1) compared to standard ciprofloxacin (−7.952 kcal mol−1). This higher interaction is due to the 11th (C-4) and 19th (C-3) oxygen atoms of FQ basic nucleus forms two H-bond interactions with Arg32, with a bond length of 1.9 Å and 2.4 Å, respectively. Additionally, fluorine (C-6) atom of the FQ forms halogen interaction with Arg32. Halogen (fluorine) interactions are carbon-bound halogen interactions that have similar structural significance to weak hydrogen bonds.29 Moreover, FQ 172 forms van der Waals interaction with mutant residue Asn87. The other Pi–sulfur, Pi–Pi and Pi–alkyl interaction are clearly depicted in Fig. 7.
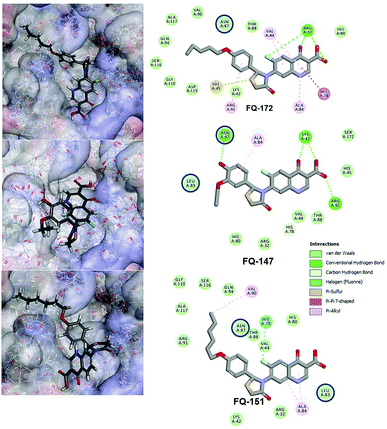 |
| Fig. 7 Interaction of molecule FQ-172, FQ-147 and FQ-151 with active site residues of mtDNA GyrA model. The blue circle indicated as a FQ resistant residues (mutant residues). | |
The CDOCKER energy of ciprofloxacin (−7.952 kcal mol−1) was found to be less compared with selected molecules due to the absence of strong H-bond interaction in active site residues of the mtDNA GyrA (Fig. 8b). Especially, molecule FQ-147 (−22.6919 kcal mol−1) forms strong H-bond with Asn87 and FQ-151 (−22.5215 kcal mol−1) forms van der Waals interaction with Leu83 and Asn87 (Fig. 7). The Leu83 and Asn87 is the one of most frequently mutated residue instigating high-level FQ resistance in E. coli among the UTI.8,9 Similarly, molecule FQ-177, FQ-137, FQ-131 and FQ-70 forms strong H-bond interaction with Asn87 (Fig. S4†). Additionally, the un-substituted –NH group and –COOH group of molecule FQ-37 forms two H-bond with Asn87 (1.9 Å) and Ala1171 (1.9 Å) residues (Fig. 8a) compared with that of the standard drug ciprofloxacin comprising –NH group substituted with propyl ring which clearly blocks the H-bond formation. This H-bond interaction of unsubstituted –NH group of the newly designed FQ analogs creates a novel binding site, thereby, reducing the steric hindrance in the mtDNA GyrA protein. From the above docking results, it is confirmed that the newly designed FQ analogues interact effectively with the mutated residues and, hence, serves as a novel mtDNA GyrA protein inhibitor.
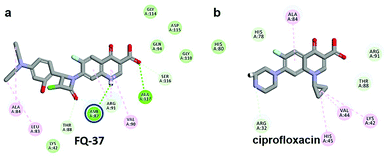 |
| Fig. 8 (a) Hydrogen bond interaction of unsubstituted –NH group in molecule FQ-37 with mutant residue Asn87 (b) interactions of substituted –NH group in ciprofloxacin. | |
Docking studies performed using LibDock
The LibDock docking software was used to confirm the reliability ranking of novel FQ analogous. The results showed that among the 204 molecules only 150 FQ molecules were docked and the remaining molecules failed to form proper orientation in the active site. The LibDock score of each compound are graphically shown in Fig. 9. The molecule FQ-182 shows a highest LibDock score of 108.48 compared to other molecules. Which forms strong hydrogen bonds, van der Waals, Pi–sulfur and Pi–alkyl interactions with active site residues. Notably, the mutant residue Asn 87 interacts with unsubstituted –NH group of FQ-181 molecule by strong hydrogen bond (Fig. 10).
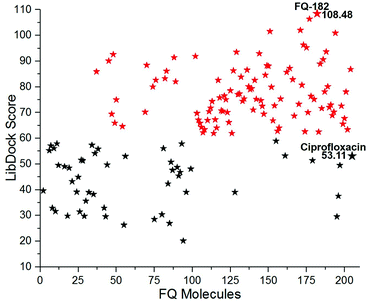 |
| Fig. 9 The LibDock score of the 150 FQ molecules. Color code: red stars indicates selected 100 hit compounds having highest score (61.96 to 108.48). Black stars indicated the lower score (20.15 to 52.92). | |
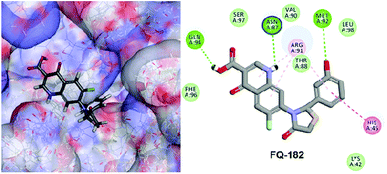 |
| Fig. 10 The docked pose of FQ-182 (highest LibDock score) with active site residues of mtDNA GyrA model. | |
The combined CDOCKER and LibDock results provide a more reliable ranking of 100 best molecules. The 100 hit molecules screened from both the docking results had good interaction with active site residues of mtDNA GyrA through H-bonds, Pi–alkyl and van der Waals interactions. It primes a docking score ranging from −23.9069 to −9.55468 (kcal mol−1) (Fig. 6) and 61.96 to 108.48 (Fig. 9.) as listed out in Table S2.† The binding affinity assessment of the docked compounds revealed higher affinity of azitidinone fragment substitution when compared with thiazolidinone fragment substitution, from which, it can be inferred that the fragments with hydrophobic alkyl group performed better than those with aromatic ring substitutions. The highest dock score value of all the 100 hit molecules indicates good molecular level interactions into binding site and these molecules were further subjected to pharmacophore screening and toxicity prediction.
Molecular dynamic simulation studies
MD simulation was focused on analysing the pattern, strength, properties of protein behaviour, drug receptor complex stability and conformation changes by using comprehensive empirical energy function. The solvation process added 15
161 water, 54 sodium and 40 chloride ion in the explicit periodic boundary cell to determine the molecular conformation, electronic properties and binding energies. Initial coordinates have bad contacts, causing high energies and forces. The protein stability during MD simulation is monitored through the time evolution of the root mean square deviation (RMSD). MD simulations were carried out up to 2200 ps for the mtDNA GyrA and its FQ complex systems. Fig. 11 shows that the total energy variation and stabilization temperature for the systems. All the system found stabilized at the 1100 ps time at 290 K temperature and the energy of the system at the stabilized state was 625, 570 and 510 (kcal mol−1) for WT, mtDNA GyrA protein and FQ complex respectively. Fig. 12 displays the distributional probability of RMSD from 120 to 420 ns trajectories. The mean RMSD values of the three systems were 0.7 Å (WT), 1.0 Å (mtDNA GyrA) and 1.3 Å (FQ-complex). The RMSD analysis shows that the structural stabilities in MD simulations are consistent with the experimental binding affinity data. Notably, the RMSD value of the mtDNA GyrA system is higher than that of WT. In the WT system, the polar non-charged residue Ser83 was mutated to non-polar Leu residue and negative charged amino acid Asp87 was mutated neutral residues of Asn. Thus, this mutation caused the steric repulsion increased the fluctuation of the protein structure remarkably. The flexibility of each residue is calculated by its root mean square fluctuation (RMSF) value. Fig. 12 showed the RMSF values of residues of three systems calculated from 120 to 420 ns trajectories. The mutated part of the system showed relatively low fluctuation values. Hence it is not affected the stability of the protein, but this mutation causes hydrophobic repulsion of the old FQ drugs.
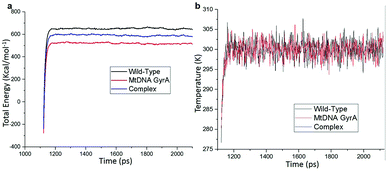 |
| Fig. 11 (a) Time based total energy changes graph in MD simulation for three systems. (b) Equilibrium state effect of temperature changes in different time scale of all the three system in equilibrium state. | |
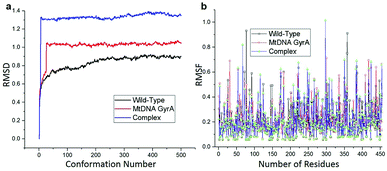 |
| Fig. 12 (a) RMSD of various conformation generate in production stage. (b) RMSF of amino acid residues between this three system in production stage run. | |
Docking studies performed with different conformations
The MD simulation study report of 500 conformations for mtDNA GyrA protein was performed. After clearly analyzing the results for each conformation of mtDNA GyrA, it was observed that there was no significant conformational changes in the active site cavity, with the only minor disparity in the other sites. To analyses stability of the above docking results, additionally, four conformations (conformations no. 49, 194, 372, and 500) were selected based on the stable RMSD (0.75, 0.84, 0.92, and 0.90 respectively) values for the docking process. The docking results using LibDock algorithm revealed that all the four conformations formed a stable complex with the screened 100 FQ analogs (Fig. 13). The detailed LibDock score of each conformation are listed out in Table S3.† It concludes that the docking results generated are not by chance.
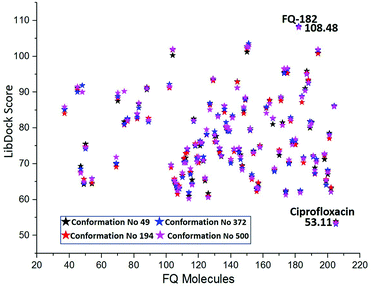 |
| Fig. 13 LibDock scores of the virtually screened 100 FQ analogous in four conformations. | |
Pharmacophore modeling
Pharmacophore modeling, in total, is collective information of the steric and electronic features necessary for optimal supra-molecular interactions with a target protein structure and block/triggers its response.30
Structure-based pharmacophore modeling
SBP is a kind of pharmacophore derived from protein' active site information which pertains to identification of hydrophobic (HY), H-bond donors (HBD) or H-bond acceptors (HBA) of target protein. In this study, the generated pharmacophore model of mtDNA GyrA protein contains a total of 26 features such as ten HY, nine HBD and seven HBA (Fig. 14a). As a result of mapping, 100 hit molecules from docking results were mapped with the generated SBP model to identify the geometric fit values of the compounds. Based on their higher fit values, 50 compounds were carefully chosen for further ligand-based pharmacophore modeling. The fit value of the 50 molecules ranged from 3.225 to 2.0161 and that of the relative energy ranged from 19.577 to 0.125 (kcal mol−1) (Fig. 15). All the selected molecules were well fitted with the active site features of mtDNA GyrA pharmacophore. It was observed that the molecule FQ-151 mapped well with six features of SBP model as depicted in Fig. 14b. The phenyl ring of the FQ-151 nucleus and long alky chain of the azitidinone fragment mapped with four HY features. Also, the –NH position of the phenyl ring and azitidinone (O-atom) formed good orientation fit with HBD and HBA.
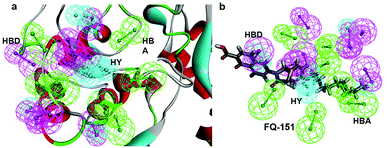 |
| Fig. 14 (a) Structure-based pharmacophore model generated from the mtDNA GyrA protein and (b) molecule FQ-151 mapped on the generated pharmacophore features. The identified pharmacophoric features are shown in green, cyan and magenta for HA, HY and HD features, respectively. | |
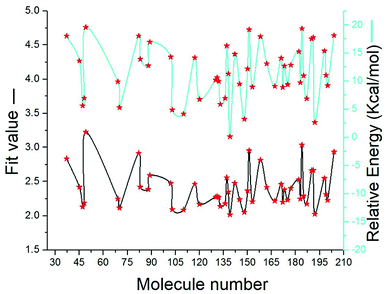 |
| Fig. 15 The predicted fit values and relative energy (kcal mol−1) of the screened 50 molecules using structure-based pharmacophore model. | |
Ligand-based pharmacophore modeling
In this process, 5 set of pharmacophore hypotheses (Hypogen) were generated from the 10 training set compounds by using 3D QSAR pharmacophore generation protocol. Hypogen is the quantitative hypotheses used to identify the features that are present in the training set compounds. Generated hypotheses comprised of three chemical features: HBA, HY and RA (Fig. 16a) from which it could be proposed that these three features are crucial factors for inhibition of the E. coli DNA gyrase. Inhibition of a target protein is not only decided by the chemical features of the training set compounds, it also required to have an exact shape to fit into the active site of the protein. Thus, we had carefully evaluated the Hypogen by statistical parameters like cost analysis, correlation coefficient and RMS deviation. The statistical parameters such as cost values, correlation and RMSD are summarized in Table 1. The total cost of each hypotheses was much closer to the fixed cost value, thus, satisfying the potentials of a good hypotheses. Among the total cost values of five pharmacophore models, Hypo 1 scored the closest value (98.15) to the fixed cost value (107.15) than other models. Hypo 1 had the highest cost difference between null cost (217.81) and total cost (98.15) of 119.66 bits. The large difference between these costs suggests that Hypo 1 has more than 90% statistical significance. High correlation coefficient and low RMSD value of Hypogen indicated higher ability to predict the activity of the training set compounds.31 The developed Hypo 1 had the correlation coefficient value of 0.921 Å and RMSD deviation of <1.12 Å than other four models, which suggests that the Hypo 1 model is more effective for prediction of the activity of the training set compounds, predictive activity and average fit values listed in Table 2. The geometric fit values for every molecule was determined by their map on the feature location. In Fig. 16c and d, the highest active compound (gemifloxacin MIC = 0.01 μg ml−1) exhibits a good fit with all the features of the Hypo 1, whereas, in the least active compound (enoxacin MIC = 0.22 μg ml−1) the HBA feature mapped away from the fit. On this basis, it can be concluded that Hypo 1 is a reliable model that accurately estimates the MIC values of the training set compounds. Further, the best pharmacophore model Hypo 1 was validated by Fisher's randomization and test set method.
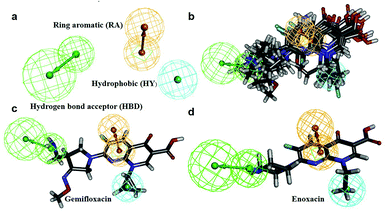 |
| Fig. 16 (a) Hypo 1 (best pharmacophore) generated by Hypogen. (b) Total training set compounds mapped on Hypo 1. (c) Mapping of most active and (d) least active compounds. | |
Table 1 Statistical results of the generated pharmacophore models
Hypogen no |
Total cost (bits) |
Cost difference (total cost-null cost) (bits)a |
RMSb (Å) |
Correlation |
Featuresb |
Max fit |
The cost difference between null cost and total cost; null cost is 217.816 bits; fixed cost is 107.15 bits; configuration cost is 0.921 bits. Abbreviation: RMS, root mean square deviation; HBA, hydrogen bond acceptor; HBD, hydrogen bond donor, HY, hydrophobic and RA, ring aromatic. |
Hypo 1 |
98.15 |
119.666 |
1.12 |
0.921 |
1HBA lipid,1Hy,1RA |
9.12 |
Hypo 2 |
106.85 |
110.966 |
1.59 |
0.916 |
1HBA lipid,1Hy,1RA |
8.11 |
Hypo 3 |
133.45 |
84.366 |
1.41 |
0.930 |
2HBA lipid,1Hy |
10.02 |
Hypo 4 |
129.27 |
88.546 |
1.70 |
0.867 |
3HBA lipid |
3.20 |
Hypo 5 |
122.74 |
95.076 |
1.66 |
0.883 |
1HBA lipid, 2Hy, 1RA |
5.29 |
Table 2 Experimental and estimated MIC values of the training set compounds based on the best pharmacophore hypotheses Hypo 1
Training set compounds |
MIC value (μg ml−1) |
Fit value |
Errorb |
Structure |
Name |
Experimentala |
Estimated |
Ref. 3,32–37. Positive value indicates that the estimated MIC value is higher than the experimental MIC value; the negative value indicates that the estimated MIC value is lower than the experimental MIC value. |
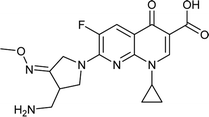 |
Gemifloxacin |
0.01 |
0.0097976 |
5.96258 |
−1.02065 |
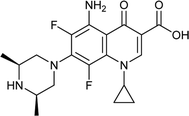 |
Sparfloxacin |
0.0125 |
0.0189725 |
5.67557 |
1.5178 |
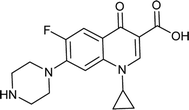 |
Ciprofloxacin |
0.015 |
0.0289285 |
5.49237 |
1.92857 |
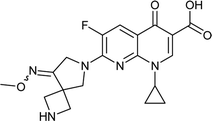 |
Zabofloxacin |
0.015 |
0.0152749 |
5.76972 |
1.01833 |
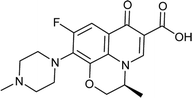 |
Levofloxacin |
0.022 |
0.011427 |
4.8766 |
−1.84213 |
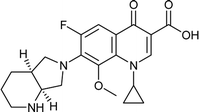 |
Moxifloxacin |
0.025 |
0.0172101 |
5.71792 |
−1.45264 |
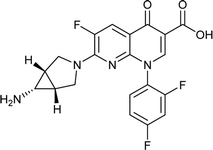 |
Trovafloxacin |
0.025 |
0.0238815 |
5.57564 |
−1.04684 |
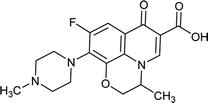 |
Ofloxacin |
0.062 |
0.0719427 |
4.8766 |
1.92624 |
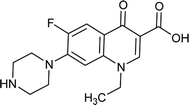 |
Norfloxacin |
0.094 |
0.0637224 |
5.14941 |
−1.47515 |
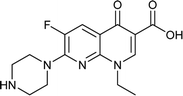 |
Enoxacin |
0.1 |
0.074975 |
5.07878 |
−1.33378 |
Pharmacophore model evaluation
Fisher's randomization. Fischer's randomization test was used to validate the statistical significance of Hypo 1. The confidence level was fixed at 95% and a total 19 random spreadsheets were created to produce the hypotheses (Fig. 17). The formula S = [1 − 19 + X)/Y] × 100 was used to calculate the significance level of hypotheses at 95% (S), where, X is the total number of hypotheses having a total cost lower than the original hypotheses and Y is the total number of Hypogen runs. Here, X = 0 and Y= (1 + 19), hence, 95% = {1 − [(1 + 0)/(19 + 1)]} × 100. All 19 random spreadsheets have high-cost values (121.968–322.813) than total cost (98.15) and that of the correlation value (0.301–0.894) is less than the Hypo 1 (0.918) (Table S4†).
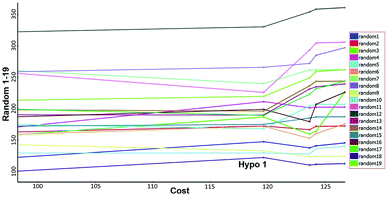 |
| Fig. 17 Results of Fischer randomization test for 95% confidence level. | |
It clearly shows that Hypo 1 was far more superior to the 19 random hypotheses, suggesting that Hypo 1 was not generated by mere chance. Finally, the Fischer's randomization test confirmed that Hypo 1 was statistically robust.
Test-set method. Cross-validation was performed by test set method to check the predictability power and accuracy of Hypo 1. Test set contains 20 structurally distinct compounds with different activity range. All the test set compounds were classified relatively into three sets based on their activity values: highly active (MIC < 0.04 μM, +++), moderately active (0.04 μM ≤ MIC < 0.08, ++) and low active (MIC > 0.08, +). The experimental and estimated MIC value of both training and test set compounds were plotted in Fig. 18 and listed out in Table S5.† It was observed that estimated MIC value of test set compounds using Hypo 1 was similar to that of the reported experimental values, as supported by the correlation coefficient value (0.92). From the results, it is evident that Hypo 1 has a precise predictive ability which is capacitated to produce the most reliable hits among the designed compounds can be retrieved.
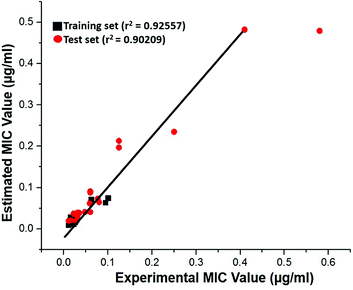 |
| Fig. 18 The correlation graph between experimental and estimated activity values based on Hypo 1. | |
Pharmacophore screening. The precisely validated pharmacophore model, Hypo 1, was used as query to predict the MIC value for the 50 molecules that were screened from SBP modeling. In order to find out hit compounds with the lowest MIC values, the Ligand Pharmacophore Mapping protocol was performed with best search option. Finally, 30 compounds with lowest MIC values (0.0080 to <0.020 μg ml−1), higher fit values (Fig. 19) and necessary pharmacophore features were shortlisted (Table 3). All the compounds were exactly mapped on to the 1 HBA, 1 HY and 1 RA with the greater orientation fit. These well-mapped molecules with lowest MIC values were preferred for ADMET and toxicity studies.
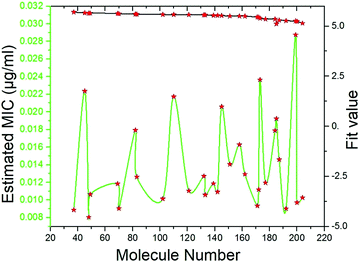 |
| Fig. 19 Estimated MIC (μg ml−1) and fit values of the 30 screened molecules by using best pharmacophore model Hypo 1. | |
Table 3 Estimated MIC values of 30 hit molecules from ligand-based pharmacophore modeling
Molecules name |
Estimate MIC (μg ml−1) |
Features |
Fit value |
FQ-37 |
0.00887224 |
HBA, Hy, RA |
5.65967 |
FQ-48 |
0.00804618 |
HBA, Hy, RA |
5.70211 |
FQ-49 |
0.0106329 |
HBA, Hy, RA |
5.58105 |
FQ-70 |
0.0090439 |
HBA, Hy, RA |
5.65134 |
FQ-83 |
0.0126287 |
HBA, Hy, RA |
5.50634 |
FQ-111 |
0.0118406 |
HBA, Hy, RA |
5.53433 |
FQ-113 |
0.017929 |
HBA, Hy, RA |
5.35414 |
FQ-120 |
0.0110452 |
HBA, Hy, RA |
5.56453 |
FQ-131 |
0.0101417 |
HBA, Hy, RA |
5.60159 |
FQ-132 |
0.0126948 |
HBA, Hy, RA |
5.50407 |
FQ-133 |
0.0105782 |
HBA, Hy, RA |
5.58329 |
FQ-137 |
0.0109687 |
HBA, Hy, RA |
5.29398 |
FQ-139 |
0.011871 |
HBA, Hy, RA |
5.53321 |
FQ-142 |
0.0109171 |
HBA, Hy, RA |
5.56959 |
FQ-145 |
0.0205929 |
HBA, Hy, RA |
5.29398 |
FQ-147 |
0.011372 |
HBA, Hy, RA |
5.27077 |
FQ-149 |
0.012864 |
HBA, Hy, RA |
5.25821 |
FQ-151 |
0.0140471 |
HBA, Hy, RA |
5.46011 |
FQ-158 |
0.01628 |
HBA, Hy, RA |
5.39605 |
FQ-162 |
0.0129325 |
HBA, Hy, RA |
5.49602 |
FQ-171 |
0.00934395 |
HBA, Hy, RA |
5.63717 |
FQ-172 |
0.0111819 |
HBA, Hy, RA |
5.55918 |
FQ-177 |
0.0119603 |
HBA, Hy, RA |
5.52996 |
FQ-182 |
0.010761 |
HBA, Hy, RA |
5.23391 |
FQ-183 |
0.011389 |
HBA, Hy, RA |
5.14912 |
FQ-185 |
0.0192373 |
HBA, Hy, RA |
5.11541 |
FQ-187 |
0.0145925 |
HBA, Hy, RA |
5.44357 |
FQ-192 |
0.00901582 |
HBA, Hy, RA |
5.65269 |
FQ-200 |
0.00969673 |
HBA, Hy, RA |
5.62107 |
FQ-204 |
0.0102625 |
HBA, Hy, RA |
5.59645 |
ADMET study. In silico ADMET assessment is a beneficial stride for predictive quantitative structure-property studies that can be applied in drug discovery. It decreases the requirement for expensive and expansive in vitro pharmacokinetics and toxicity screening.38 ADMET descriptors were used to calculate and filter out the FQ molecules with undesired pharmacokinetic properties among the 30 screened molecules. The pharmacokinetics profile of all the 30 compounds and the standard ciprofloxacin under analysis were predicted by means of six recalculated ADMET models. The pharmacokinetic analysis results are shown in Fig. 20. After examination of ADMET biplot, it was observed that the molecules FQ-171 and FQ-204 fall outside the ADMET ellipses due to the octyloxy and nonyloxy substitutions. These substitutions increased the lipophilic nature of the molecules FQ-171 and FQ-204 leading to reduced oral bioavailability and hence are shown to possess poor HIA and BBB penetration property as per the biplot. The remaining 28 molecules and the standard drug ciprofloxacin fall into the 95% and 99% confidence ellipses for the HIA and BBB models, respectively.
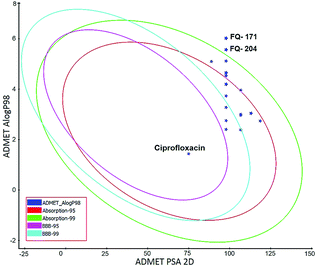 |
| Fig. 20 Plot of PSA versus A log P for selected molecules showing 95% and 99% confidence limit ellipses corresponding to the blood–brain barrier (BBB) and human intestinal absorption (HIA) models. | |
The screened 28 molecules did not inhibit CYP450 2D6 enzyme, which confirms that the FQ analogs had no selectivity towards the metabolic enzyme. Additionally, these molecules had excellent absorption through the cell membrane, PSA < 140 Å2 (polar surface area) and A
log
P 98 < 5 which satisfied the reported criteria.39 It exposed that the selected molecules had overall good BBB, absorption, solubility, hepatotoxicity, CYP2D and PPB level (Table 4). These hit compounds were subjected to further filtering by toxicity prediction analysis.
Table 4 ADMET result of selected 28 compounds and standard ciprofloxacin druga
Molecules name |
BBB penetration level |
Absorption level |
Solubility level |
Hepato toxicity |
CYP2D6 inhibitor |
PPB level |
A log P 98 |
PSA 2D |
Abbreviations: BBB- blood–brain barrier, CYP2D6- cytochrome P450 2D6, PPB- plasma protein binding, A log P 98- the logarithm of the partition coefficient between n-octanol and water, PSA- polar surface area. |
FQ-37 |
Low |
Good |
Good |
No |
No |
<90% |
3.051 |
113.04 |
FQ-48 |
Low |
Moderate |
Good |
No |
Yes |
<90% |
5.114 |
97.81 |
FQ-49 |
Medium |
Moderate |
Good |
No |
No |
<90% |
4.658 |
97.81 |
FQ-70 |
Low |
Good |
Good |
No |
Yes |
<90% |
4.202 |
97.81 |
FQ-83 |
Low |
Good |
Good |
No |
No |
<90% |
3.004 |
106.74 |
FQ-121 |
Medium |
Good |
Good |
No |
No |
<90% |
3.166 |
88.88 |
FQ-124 |
Medium |
Good |
Good |
No |
No |
<90% |
2.623 |
88.88 |
FQ-120 |
Undefined |
Good |
Good |
No |
Yes |
<90% |
2.401 |
97.81 |
FQ-131 |
Low |
Good |
Good |
No |
Yes |
<90% |
3.985 |
97.81 |
FQ-132 |
Low |
Good |
Good |
No |
Yes |
<90% |
3.73 |
97.81 |
FQ-133 |
Medium |
Good |
Good |
No |
Yes |
<90% |
2.385 |
106.74 |
FQ-137 |
Low |
Moderate |
Good |
No |
No |
<90% |
1.934 |
130.51 |
FQ-139 |
Low |
Good |
Good |
No |
No |
<90% |
3.035 |
113.04 |
FQ-142 |
Low |
Good |
Good |
No |
No |
<90% |
2.963 |
106.74 |
FQ-145 |
Undefined |
Good |
Good |
No |
No |
<90% |
2.75 |
97.81 |
FQ-147 |
Low |
Good |
Good |
No |
No |
<90% |
2.508 |
118.62 |
FQ-149 |
Medium |
Good |
Good |
No |
No |
<90% |
1.818 |
101.43 |
FQ-151 |
Low |
Moderate |
Good |
No |
No |
<90% |
4.642 |
97.81 |
FQ-158 |
Low |
Good |
Good |
No |
No |
<90% |
2.737 |
118.62 |
FQ-162 |
Low |
Moderate |
Good |
No |
Yes |
<90% |
3.961 |
106.74 |
FQ-172 |
Low |
Good |
Good |
No |
Yes |
<90% |
4.186 |
97.81 |
FQ-177 |
Medium |
Good |
Good |
No |
Yes |
<90% |
3.274 |
97.81 |
FQ-182 |
Low |
Good |
Good |
No |
No |
<90% |
2.176 |
109.69 |
FQ-183 |
Low |
Good |
Good |
No |
No |
<90% |
2.176 |
109.69 |
FQ-185 |
Low |
Good |
Good |
No |
No |
<90% |
2.988 |
106.74 |
FQ-187 |
Low |
Moderate |
Moderate |
No |
No |
<90% |
4.537 |
97.81 |
FQ-192 |
Undefined |
Moderate |
Moderate |
No |
No |
<90% |
5.096 |
88.88 |
FQ-200 |
Low |
Moderate |
Moderate |
No |
No |
<90% |
4.537 |
97.81 |
Cipro |
Low |
Good |
Good |
No |
No |
<90% |
1.435 |
74.932 |
Toxicity risk assessment. The USFDA (US FDA, United States Food and Drug Administration) standard toxicity risk predictor software TOPKAT (toxicity risk assessment screening) was used to locate potential threat and toxicity risk fragments within the compound.40Twenty-eight hit molecules screened from ADMET were assessed using TOPKAT. About 18 molecules resulted in positive response to carcinogenicity, ames mutagenicity, skin sensitization, ocular irritancy and skin irritancy. Other details of the predicted toxicity parameters such as rat oral LD50, developmental toxicity potential, rat inhalational LC50, rat maximum tolerated dose, fathead minnow LC50 and aerobic biodegradability are summarized in Table 5. These molecules consist of the most satisfactory results than other molecules compared with standard ciprofloxacin. The chemical structure and properties of the selected 18 molecules are described in Table 6. Remarkably, all the selected lead molecules complied with the Lipinski rule of five,41 thus having better drug-likeness.
Table 5 Probability values of different toxicity models of the selected 18 compounds by TOPKAT analysisa
Name |
FDA |
AMU |
DTP |
SI |
SS |
OC |
ABD |
Rate |
Fathead minnow LC50 (ng l−1) |
Daphnia EC50 (ng l−1) |
FRC |
MRC |
FMC |
MMC |
Oral LD50 (g kg−1) |
Max. tolerated dose (mg kg−1) |
Inhalation LC50 (mg m−3 H−1) |
Abbreviations: FDA- Food and Drug Administration, EC50- effective concentration 50%, LD50- lethal dose 50%, LOAEL- lowest adverse effect level, TD50- tumorigenic dose 50%, FRC- female rat carcinogenicity, MRC- male rat carcinogenicity, C- carcinogenicity, NC- non carcinogenicity, FMC- female mouse carcinogenicity, MMC- male mouse carcinogenicity, MU- Ames mutagenicity, NM- non mutagenicity, DTP -development toxicity potential, T- toxicity, SI- skin irritation, NSI-non skin irritation, SS- skin sensitization, NS- non skin sensitization, I- intermediate, OC-ocular irritancy, NOC- non ocular irritancy, BD- aerobic biodegradable. |
FQ-37 |
C |
C |
NC |
NC |
NM |
T |
NSI |
NS |
NOC |
BD |
4.8 |
81.5 |
9.9 |
2.5 |
3.3 |
FQ-49 |
C |
C |
I |
NC |
NM |
T |
SI |
NS |
NOC |
BD |
1.8 |
83.9 |
189.6 |
457.0 |
258.9 |
FQ-70 |
C |
I |
NC |
NC |
NM |
T |
SI |
NS |
NOC |
BD |
3.3 |
86.2 |
46.9 |
903.3 |
260.0 |
FQ-121 |
C |
C |
NC |
NC |
NM |
T |
NSI |
NS |
NOC |
BD |
2.6 |
69.24 |
86.9 |
23.3 |
56.8 |
FQ-124 |
C |
C |
I |
NC |
NM |
T |
NSI |
NS |
NOC |
BD |
11.5 |
223.6 |
834.0 |
33.6 |
48.3 |
FQ-131 |
C |
C |
NC |
NC |
NM |
T |
NSI |
NS |
NOC |
BD |
7.3 |
135.8 |
12.7 |
1.0 |
48.2 |
FQ-132 |
C |
C |
NC |
NC |
NM |
T |
SI |
NS |
NOC |
BD |
10 |
450 |
538 |
1.7 |
77.2 |
FQ-133 |
C |
C |
NC |
NC |
NM |
T |
SI |
NS |
NOC |
BD |
7.5 |
46.7 |
155.5 |
3.0 |
19.6 |
FQ-137 |
C |
C |
I |
NC |
NM |
T |
NSI |
NS |
NOC |
BD |
3.4 |
102.2 |
4.3 |
15.8 |
603.2 |
FQ-139 |
C |
C |
NC |
NC |
NM |
T |
SI |
NS |
NOC |
BD |
10 |
679 |
1.3 |
2.4 |
16.7 |
FQ-147 |
C |
C |
NC |
NC |
NM |
T |
NSI |
NS |
NOC |
`BD |
6.8 |
60.1 |
1.4 |
3.6 |
86.4 |
FQ-149 |
C |
C |
I |
NC |
NM |
T |
NSI |
NS |
NOC |
BD |
2.4 |
47.8 |
106.9 |
97.1 |
93.8 |
FQ-151 |
C |
C |
NC |
NC |
NM |
T |
SI |
NS |
NOC |
BD |
4.3 |
111.6 |
1.762 |
428.2 |
94.9 |
FQ-172 |
C |
I |
NC |
NC |
NM |
T |
SI |
NS |
NOC |
BD |
8.0 |
136.7 |
2.0 |
846.5 |
85.4 |
FQ-177 |
C |
C |
NC |
NC |
NM |
T |
SI |
NS |
NOC |
BD |
10 |
191.1 |
162.5 |
3.3 |
69.4 |
FQ-182 |
C |
C |
I |
NC |
NM |
T |
NSI |
NS |
NOC |
BD |
15.1 |
87.1 |
148.0 |
29.1 |
33.7 |
FQ-183 |
C |
C |
NC |
NC |
NM |
T |
NSI |
NS |
NOC |
BD |
13.8 |
69.7 |
145.8 |
28.8 |
47.5 |
FQ-185 |
C |
NC |
NC |
NC |
NM |
T |
I |
NS |
NOC |
BD |
10 |
34.0 |
2.8 |
432.9 |
25.8 |
Cipro |
C |
C |
NC |
NC |
NM |
I |
NSI |
NS |
NOC |
BD |
0.5 |
10 |
1.7 |
220.0 |
1.1 |
Table 6 Details of shortlisted potent new fluoroquinolone analogs against resistant E. coli
Molecule number |
Chemical structure |
Molecular formula |
Mol. weight (g mol−1) |
H-bond donor/acceptor |
log P value |
FQ-37 |
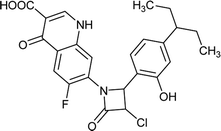 |
C23H21ClFN3O5 |
473.8 |
3/6 |
3.051 |
FQ-49 |
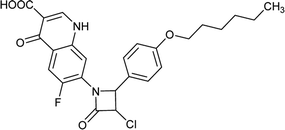 |
C25H24ClFN2O5 |
486.9 |
2/6 |
4.658 |
FQ-70 |
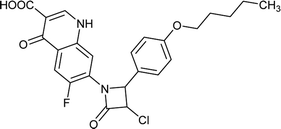 |
C24H22ClFN2O5 |
472.8 |
2/6 |
4.202 |
FQ-121 |
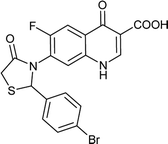 |
C19H12BrFN2O4S |
463.2 |
2/6 |
3.166 |
FQ-124 |
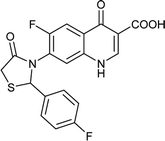 |
C19H12F2N2O4S |
402.3 |
2/6 |
2.623 |
FQ-131 |
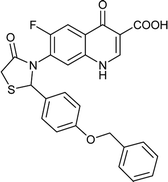 |
C26H19FN2O5S |
490.5 |
2/7 |
3.985 |
FQ-132 |
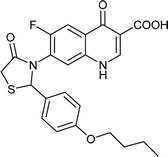 |
C23H21FN2O5S |
456.4 |
2/7 |
3.73 |
FQ-133 |
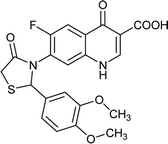 |
C21H17FN2O6S |
444.4 |
2/8 |
2.385 |
FQ-137 |
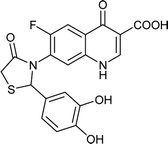 |
C19H13FN2O6S |
416.3 |
4/8 |
1.934 |
FQ-139 |
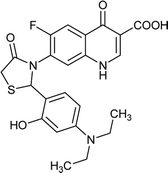 |
C23H22FN3O5S |
471.5 |
3/8 |
3.035 |
FQ-147 |
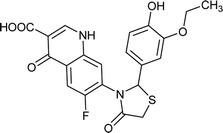 |
C21H17FN2O6S |
444.4 |
3/8 |
2.508 |
FQ-149 |
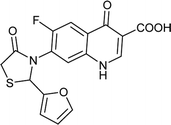 |
C17H11FN2O5S |
374.3 |
2/6 |
1.818 |
FQ-151 |
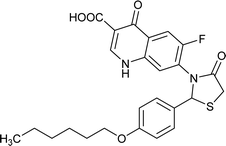 |
C25H25FN2O5S |
484.5 |
2/7 |
4.642 |
FQ-172 |
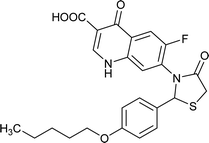 |
C24H23FN2O5S |
470.5 |
2/7 |
4.186 |
FQ-177 |
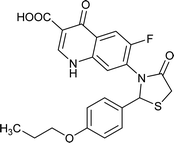 |
C22H19FN2O5S |
442.4 |
2/7 |
3.274 |
FQ-182 |
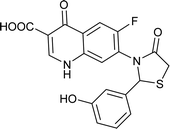 |
C19H13FN2O5S |
400.3 |
3/7 |
2.176 |
FQ-183 |
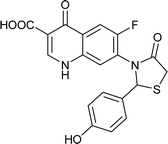 |
C19H13FN2O5S |
400.3 |
3/7 |
2.176 |
FQ-185 |
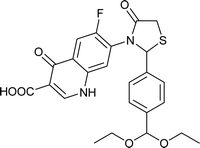 |
C24H23FN2O6S |
486.5 |
2/8 |
2.988 |
Conclusions
In the present study, the 3D structure of mtDNA GyrA of FQR E. coli was predicted using homology modeling followed by structure validation. The modeled protein encompassed mutation at Ser83Leu and Asp87Asn, it's reported for FQR E. coli and was used for screening of 204 novel FQ analogs that were designed based on SAR. Followed by docking and interpretation, it was observed that the newly designed FQ analogs formed van der Waals interactions with Leu83 and H-bond with Asn87 of mtDNA GyrA, from which it is clearly understood that the novel FQ analogs possess higher inhibitory efficacy towards mtDNA GyrA. Based on the binding energy, compounds with the least energy values were filtered and used for further studies. The mtDNA GyrA and the docked complex structures were validated by RMSD and RMSF with help of MD simulations. It proved that both structures were stable and no conformational variations were observed when compare with the MD results of WT protein. Predicted SBP model was used to screen the designed compounds by fit values, followed by which, the LBP model Hypo 1 was used to predict the MIC value for screened compounds. Our study proved that the multiple pharmacophore modeling approaches are crucial for identification of the pharmacophore features required for identification of compounds that are close to standard FQ drugs. The molecules with lowest predicted MIC value were filtered subsequently by ADMET and TOPKAT techniques, using which, the unwanted toxic fragments containing FQ molecules and the numbers of false positive results were eliminated. Finally, 18 molecules were shortlisted for further synthesis by green technology and drug development to curb the UTIs caused by FQR E. coli. On the whole, this study provides an insight on the binding site properties of the mtDNA GyrA that can be utilized as a guide for future studies for designing various structurally diverse compounds from FQ family. Overall, the present work enriches the repository of FQ drugs that can be successfully used against FQR pathogens.
Conflicts of interest
There are no conflicts to declare.
Acknowledgements
We gratefully thank TEQIP II for funding and the facility offered to author Sakthivel Balasubramaniyan.
References
- C. Hamilton, L. Tan, T. Miethke and P. K. Anand, Nat. Rev. Urol., 2017, 14, 284–295 CrossRef PubMed.
- Y. Betitra, V. Teresa, V. Miguel and T. Abdelaziz, Asian Pac. J. Trop. Med., 2014, 7, 462–467 CrossRef PubMed.
- M. Malik, A. Mustaev, H. A. Schwanz, G. Luan, N. Shah, L. M. Oppegard, E. C. De Souza, H. Hiasa, X. Zhao, R. J. Kerns and K. Drlica, Nucleic Acids Res., 2016, 44, 3304–3316 CrossRef PubMed.
- S. Conrad, M. Oethinger, K. Kaifel, G. Klotz, R. Marre and W. V Kern, J. Antimicrob. Chemother., 1996, 38, 443–455 CrossRef PubMed.
- S. Bouchillon, D. Hoban, R. Badal and S. Hawser, Open Microbiol. J., 2012, 6, 74–78 CrossRef PubMed.
- CDDEP, Resistance Map, Center for Disease Dynamics, Economics & Policy, Washington DC, 2015 Search PubMed.
- F. M. Barnard and A. Maxwell, J. Antimicrob. Chemother., 2001, 45, 1994–2000 CrossRef PubMed.
- S. Krishnan, D. Balasubramaniyan, B. A. Raju and B. S. Lakshmi, J. Antimicrob. Chemother., 2012, 2, 366–371 Search PubMed.
- K. Wada, R. Kariyama, R. Mitsuhata, S. Uehara, T. Watanabe, K. Monden and H. Kumon, Acta Med. Okayama, 2009, 63, 263–272 Search PubMed.
- J. Ruiz, J. Antimicrob. Chemother., 2003, 51, 1109–1117 CrossRef PubMed.
- E. I. Parkinson, J. S. Bair, B. A. Nakamura, H. Y. Lee, H. I. Kuttab, E. H. Southgate, G. W. Lau and P. J. Hergenrother, Nat. Commun., 2015, 6, 6947 CrossRef PubMed.
- Dassault Systèmes BIOVIA, Discovery Studio Modeling Environment, Release 2017, Dassault Systèmes, San Diego, 2016 Search PubMed.
- T. Yasufuku, K. Shigemura, T. Shiralawa, Y. Nakano, K. Tanaka, S. Arakawa, S. Kinoshita, K. Nishimura and M. Fujisawa, Scand. J. Infect. Dis., 2011, 43, 83–88 CrossRef PubMed.
- M. Shen and A. Sali, Protein Sci., 2006, 15, 2507–2524 CrossRef PubMed.
- R. A. Laskowski, M. W. MacArthur, D. S. Moss and J. M. Thornton, J. Appl. Crystallogr., 1993, 26, 283–291 CrossRef.
- B. Wallner and A. Elofsson, Protein Sci., 2003, 12, 1073–1086 CrossRef PubMed.
- C. Colovos and T. O. Yeates, Protein Sci., 1993, 2, 1511–1519 CrossRef PubMed.
- D. Eisenberg, R. Lüthy and J. U. Bowie, Methods Enzymol., 1997, 277, 396–404 Search PubMed.
- M. Wiederstein and M. J. Sippl, Nucleic Acids Res., 2007, 35, 407–410 CrossRef PubMed.
- H. Wieman, K. Tondel, E. Anderssen and F. Drabløs, Mini-Rev. Med. Chem., 2004, 4, 793–804 Search PubMed.
- D. Kang, X. Pang, W. Lian, L. Xu, J. Wang, H. Jia, B. Zhang and G. H. Du, RSC Adv., 2008, 8, 5286 RSC.
- M. Taha, Virtual Screening, 2012, p. 100 Search PubMed.
- M. Niu, J. Qin, C. Tian, X. Yan, F. Dong, Z. Cheng, G. Fida, M. Yang, H.-Y. Chen and Y.-Q. Gu, Acta Pharmacol. Sin., 2014, 35, 967–979 CrossRef PubMed.
- P. D. Kalariya, M. Sharma, P. Garg, J. R. Thota, S. Ragampeta and M. V. N. K. Talluri, RSC Adv., 2015, 5, 31024–31038 RSC.
- E. Pestova, J. J. Millichap, G. a Noskin and L. R. Peterson, J. Antimicrob. Chemother., 2000, 45, 583–590 CrossRef PubMed.
- L. R. Peterson, Clin. Infect. Dis., 2001, 33, S180–S186 CrossRef PubMed.
- D. T. Chu and P. B. Fernandes, J. Antimicrob. Chemother., 1989, 33, 131 CrossRef.
- M. Malik, K. R. Marks, H. A. Schwanz, N. German, K. Drlica and R. J. Kerns, J. Antimicrob. Chemother., 2010, 54, 5214–5221 CrossRef PubMed.
- C. Bissantz, B. Kuhn and M. Stahl, J. Med. Chem., 2010, 53, 5061–5084 CrossRef PubMed.
- T. Kaserer, K. R. Beck, M. Akram, A. Odermatt, D. Schuster and P. Willett, Molecules, 2015, 20, 22799–22832 CrossRef PubMed.
- N. Kandakatla and G. Ramakrishnan, Adv. Bioinf., 2014, 2014, 812148 Search PubMed.
- M. Kumar, S. Dahiya, P. Sharma, S. Sharma, T. P. Singh, A. Kapil and P. Kaur, PLoS One, 2015, 10, 1–23 Search PubMed.
- K. Vinothkumar, G. N. Kumar and A. K. Bhardwaj, Front Microbiol, 2016, 7, 1–10 CrossRef PubMed.
- K. Credito, K. Kosowska-Shick, P. McGhee, G. A. Pankuch and P. C. Appelbaum, J. Antimicrob. Chemother., 2010, 54, 673–677 CrossRef PubMed.
- M. Kolar, J. Bardon, P. Sauer, M. Kesselova, L. Cekanova, I. Vagnerova, D. Koukalova and P. Hejnar, Acta Vet. Brno, 2005, 74, 249–253 CrossRef.
- L. B. Boyd, M. J. Maynard, S. K. Morgan-Linnell, L. B. Horton, R. Sucgang, R. J. Hamill, J. R. Jimenez, J. Versalovic, D. Steffen and L. Zechiedrich, J. Antimicrob. Chemother., 2009, 53, 229–234 CrossRef PubMed.
- C. G. Gloria, A. Parra, L. Aguilar, C. Ponte, M. J. Gimenez, A. Carcas, M. Kinzig Schippers and F. Soriano, J. Antimicrob. Chemother., 2001, 45, 1876–1878 CrossRef PubMed.
- B. Billones, M. C. O. Carrillo, V. G. Organo, S. J. Y. Macalino, J. B. A. Sy, I. A. Emnacen, N. A. B. Clavio and G. P. Concepcion, Drug Des., Dev. Ther., 2016, 10, 1147–1157 CrossRef PubMed.
- A. Puratchikody, D. Sriram, A. Umamaheswari and N. Irfan, Chem. Cent. J., 2016, 10, 24 CrossRef PubMed.
- A. Shukla, P. Sharma, O. Prakash, M. Singh, K. Kalani, F. Khan, D. U. Bawankule, S. Luqman and S. K. Srivastava, PLoS One, 2014, 9, e100797 CrossRef PubMed.
- C. A. Lipinski, F. Lombardo, B. W. Dominy and P. J. Feeney, Adv. Drug Delivery Rev., 1997, 23, 3–25 CrossRef.
Footnote |
† Electronic supplementary information (ESI) available: Data Fig. S1–S4 and Table S1–S4. See DOI: 10.1039/c8ra01854e |
|
This journal is © The Royal Society of Chemistry 2018 |