DOI:
10.1039/C8RA00619A
(Paper)
RSC Adv., 2018,
8, 13945-13953
De novo assembly of Schizothorax waltoni transcriptome to identify immune-related genes and microsatellite markers†
Received
21st January 2018
, Accepted 9th April 2018
First published on 16th April 2018
Abstract
Schizothorax waltoni (S. waltoni) is one kind of the subfamily Schizothoracinae and an indigenous economic tetraploid fish to Tibet in China. It is rated as a vulnerable species in the Red List of China's Vertebrates, owing to overexploitation and biological invasion. S. waltoni plays an important role in ecology and local fishery economy, but little information is known about genetic diversity, local adaptation, immune system and so on. Functional gene identification and molecular marker development are the first and essential step for the following biological function and genetics studies. For this purpose, the transcriptome from pooled tissues of three adult S. waltoni was sequenced and analyzed. Using paired-end reads from the Illumina Hiseq4000 platform, 83
103 transcripts with an N50 length of 2337 bp were assembled, which could be further clustered into 66
975 unigenes with an N50 length of 2087 bp. The majority of the unigenes (58
934, 87.99%) were successfully annotated by 7 public databases, and 15 KEGG pathways of immune-related genes were identified for the following functional research. Furthermore, 19
497 putative simple sequence repeats (SSRs) of 1–6 bp unit length were detected from 14
690 unigenes (21.93%) with an average distribution density of 1
:
3.28 kb. We identified 3590 unigenes (5.36%) containing more than one SSR, providing abundant potential polymorphic markers in functional genes. This is the first reported high-throughput transcriptome analysis of S. waltoni, and it would provide valuable genetic resources for the functional genes involved in multiple biological processes, including the immune system, genetic conservation, and molecular marker-assisted breeding of S. waltoni.
Introduction
The Qinghai-Tibetan Plateau (QTP), 2.5 million km2 with an average altitude reaching 4000 m, is the highest and the largest, and also one of the youngest plateaus in the world. Known as the World's Roof, QTP has become a global hotspot for research on biodiversity, phylogeny, adaptation, and evolution.1–3 Accompanied by the uplift of QTP, the environment had undergone tremendous changes. Extreme environment, such as hypoxia, chilliness, high radiation, has almost certainly affected the component fauna of the plateau and the adjacent areas.3 The schizothoracine fishes are well adapted to the harsh conditions, and became the predominant group of endemic fishes on the QTP.4 There are 15 genera and over 100 species belonging to schizothoracine in the world, and about 76 species and subspecies belong to 11 genera on the QTP and the adjacent areas in China.5,6 The schizothoracine fishes are characterized by slow growth rates, late maturity, low fecundity, long-lived, and restricted distributions.5 Those characteristics of the schizothoracine fishes make them more affected by habitat modification, intense exploitation, and biological invasions.7–9 Therefore, the schizothoracine fishes can be used as the paragon models to study high altitude adaptation, historical and contemporary environmental changes, and evolutionary biology.10,11
Schizothorax waltoni, an indigenous economic tetraploid fish to Tibet in China, is one kind of the subfamily Schizothoracinae and only distributed in the middle reaches of the Yarlung Tsangpo River.12 It is rated as a vulnerable species in the Red List of China's Vertebrates.13 Owing to overexploitation and biological invasion, the population and catched individual size of S. waltoni have been declining rapidly in recent years.14 Although S. waltoni plays an important role in ecology and local fishery economy, little information is known about genetic diversity, local adaptation, immune system and so on. Existing related studies of S. waltoni were mainly focused on population recording, morphology, phylogeny, mitogenome, and several fundamental aspects of biology.5,9,12,15–17 In order to protect germplasm resources of S. waltoni, the research on artificial culture and breeding has been carried out in Tibet. However, the fishery industry is threatened by the multiple infectious pathogens, since S. waltoni is particularly vulnerable to pathogenic microorganism and environmental pollutants under artificial culture conditions. Prerequisite condition to prevention and treatment of diseases is understanding the functions of genes and pathways involved in the immune system. Meanwhile, microsatellite markers, the versatile and popular genetic marker with applications in conservation biology, population genetics, and evolutionary biology, can be used for the studies of marker-assisted selection in the improvement of economic traits.18,19 The large-scale development of microsatellite markers is necessary to carry out the research of marker-assisted selection, conservation biology, and population genetics. However, functional gene identification and marker development of S. waltoni still remain poorly explored.14,20
With the advent and development of next generation sequencing (NGS) technology, it is a cost- and time-efficiency method of transcriptome sequences to identify genes and develop genetic markers.21–23 In this study, we used the Illumina Hiseq 4000 platform to analyze the pooled tissues transcriptome of S. waltoni. The major objective of this study was to obtain a comprehensive S. waltoni transcriptome information from the multiple tissues, and provide an abundant resource for the following functional studies. Genes involved in immune system were annotated and emphasized since the general interest of immune response of fish species, including S. waltoni. A large number of microsatellite markers were also detected and analyzed for the conservation genetics studies and marker-assisted selection breeding in S. waltoni.
Results and discussion
Transcriptome sequencing and de novo assembly
After sequencing using Illumina Hiseq 4000 platform, three cDNA libraries of S. waltoni generated 155
932
320 raw reads with a read length of 150 bp. After read quality evaluation, low quality trimming and length filtering, 147
852
684 clean reads were left. As a result for the trinity assembly, we obtained 83
103 transcripts ranging from 224 to 29
395 bp with average length of 1139 bp (Table 1). The transcripts were clustered into 66
975 unigenes with an average length of 956 bp (ranging from 224 to 29
395 bp) (Table 1). The N50 lengths of transcripts and unigenes were 2337 and 2087 bp, respectively, which is similar to that of Gymnodiptychus dybowskii (N50 of 2407 bp) and Schizothorax pseudaksaiensis (N50 of 2283 bp),24 and Gymnodiptychus pachycheilus (N50 of 2322 bp),10 and longer than that of Gymnocypris przewalskii (N50 of 1495 bp),25 G. przewalskii (N50 of 1836 bp),26 shorter than that of Schizothorax prenanti (N50 of 2539 bp).27 Irrespective of difference between organisms, several factors can affect the N50 length of transcripts and unigenes, including sequencing technique, read number, assembly software, and parameters. Among these unigenes, 42
890 (51.6%) unigenes were no more than 500 bp in length, 28
037 (33.7%) unigenes exceeded 1000 bp and 14
968 (18.0%) unigenes were longer than 2000 bp (Fig. 1). These proportions were also similar to that of S. prenanti.27 The detailed frequency distribution of the unigenes length is shown in Fig. 1.
Table 1 Assembled transcripts and unigenes obtained from transcriptome analysis
Terms |
Transcripts |
Unigenes |
Total number |
83 103 |
66 975 |
Shortest length (bp) |
224 |
224 |
Longest length (bp) |
29 395 |
29 395 |
Total length (bp) |
94 640 129 |
64 031 094 |
Average length (bp) |
1139 |
956 |
N50 length (bp) |
2337 |
2087 |
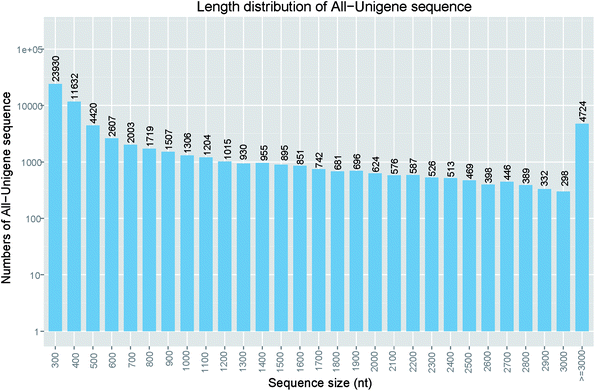 |
| Fig. 1 Length distribution of assembled unigenes. Assembled unigene numbers (y-axis) were plot against length interval (x-axis). | |
Functional annotation
Functional annotation of S. waltoni transcriptome was carried out by searching against several public nucleotide and protein databases. As a result, 57
301 (85.6%), 35
510 (53.0%), and 28
877 (43.1%) unigenes showed significant similarities (E-value < 10−5) to the NCBI nucleotide (NT), protein (NR), and Swiss-prot databases, respectively (Table 2). A total of 58
934 (88.0%) unigenes showed homologous matches in at least one database (Table 2). The annotation ratio of assembled unigenes higher than previously reported in other fish, such as Hyporthodus septemfasciatus (41.5%),28 G. przewalskii (77.8%),25 Cyprinus carpio (81.1%)29 and Ctenopharyngodon idella (82.8%),30 slightly lower than that of S. prenanti (94.4%).27 The high percentage of annotation ratio might be due to many long sequences obtained from transcriptome data and higher average length of unigenes, as well as the abundant sequence databases.31,32 The E-value distribution of unigenes which could be correctly annotated in the NR database showed that 28.1% of the unigenes had perfect matches, 30.3% of the unigenes showed significant homology to the previously stored sequences (less than 1 × 10−45), and 41.6% of the unigenes showed homology ranging from 1 × 10−45 to 1 × 10−5 (Fig. 2A). The similarity distribution of the top Blast hits for each sequence ranged from 17–100%. Among the similarity distribution, 16.5% of the unigenes had the similarity of 60–80%, and 69.8% of the unigenes obtained the similarity between 80–100% with the deposited sequences (Fig. 2B). According to the top-hit species distribution, we found that 17
611 (49.6%) unigenes exhibited homology hits in the NR search to the sequences of C. carpio, 10
484 (29.5%) to the sequences of Brachydanio rerio (Fig. 2C). This result show that evolutionary relationship is very close between S. waltoni, C. carpio, and B. rerio, consistent with the fact that three species belong to the Cyprinidae family.33
Table 2 Transcripts annotation by various databases
Database |
Hit number |
Percentage (%) |
Nr |
35 510 |
53.02% |
Nt |
57 301 |
85.56% |
Swiss-prot |
28 877 |
43.12% |
GO |
16 278 |
24.30% |
KEGG |
25 742 |
38.44% |
COG |
11 334 |
16.92% |
Pfam |
21 692 |
32.39% |
Total |
58 934 |
87.99% |
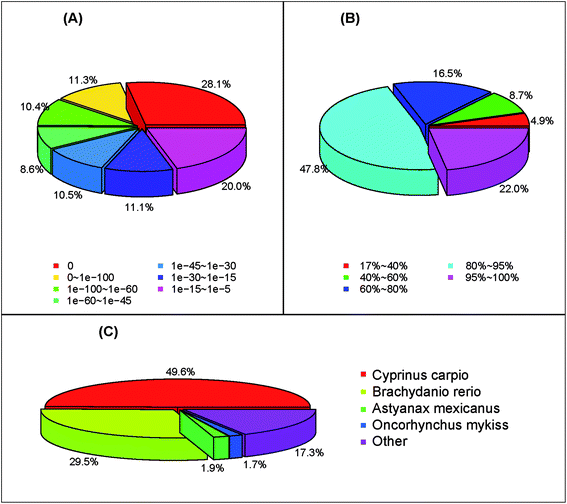 |
| Fig. 2 The length distribution of coding sequences (CDS). (A) E-value distribution, (B) similarity distribution, (C) species distribution. | |
The potential functions of all unigenes were predicted using the COG database. In all, 11
334 unigenes were grouped into 25 COG classifications (Fig. 3) in S. waltoni. The biggest category was the general function prediction only (4899, 43.2% of the matched unigenes), followed by the replication, recombination and repair (2170, 19.1%), the transcription (2127, 18.8%), the translation, ribosomal structure and biogenesis (1891, 16.7%), and the post-translational modification, protein turnover (1876, 16.6%) (Table S1,† Fig. 3). Furthermore, 106 unigenes were classified into defense mechanisms, implying that these unigenes might be related to immune defense in S. waltoni.
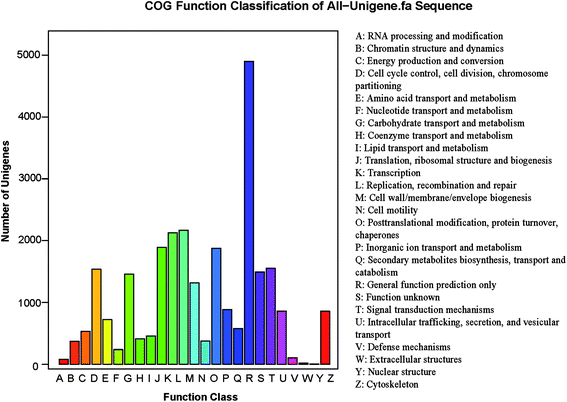 |
| Fig. 3 COG functional classification of putative protein for Schizothorax waltoni transcriptome. Out of 66 975 unigenes, 11 334 unigenes were grouped into 25 COG categories. The letters on the x-axis represent different COG assortments. | |
To further functionally classify S. waltoni transcripts, GO terms were assigned to each unigenes. Among the 35
510 annotated unigenes against NR database, a total of 16
278 unigenes were categorized into 64 level-2 GO terms in three major GO categories (Fig. 4). The most enriched components in Biological Process (BP) terms were cellular process (10
088 unigenes, GO: 0009987), single-organism process (8768 unigenes, GO: 0044699), and metabolic process (8116 unigenes, GO: 0008152). For Cellular Component (CC) terms, a large number of unigenes were involved in cell (8777 unigenes, GO: 0005623), cell part (8718 unigenes, GO: 0044464), and organelle (5836 unigenes, GO: 0043226). In the Molecule Function (MF) category, a high percentage of unigenes were related to the terms binding (8606 unigenes, GO: 0005488), and catalytic activity (5904 unigenes, GO: 0003824), followed by the transporter activity (1071 unigenes, GO: 0005215).
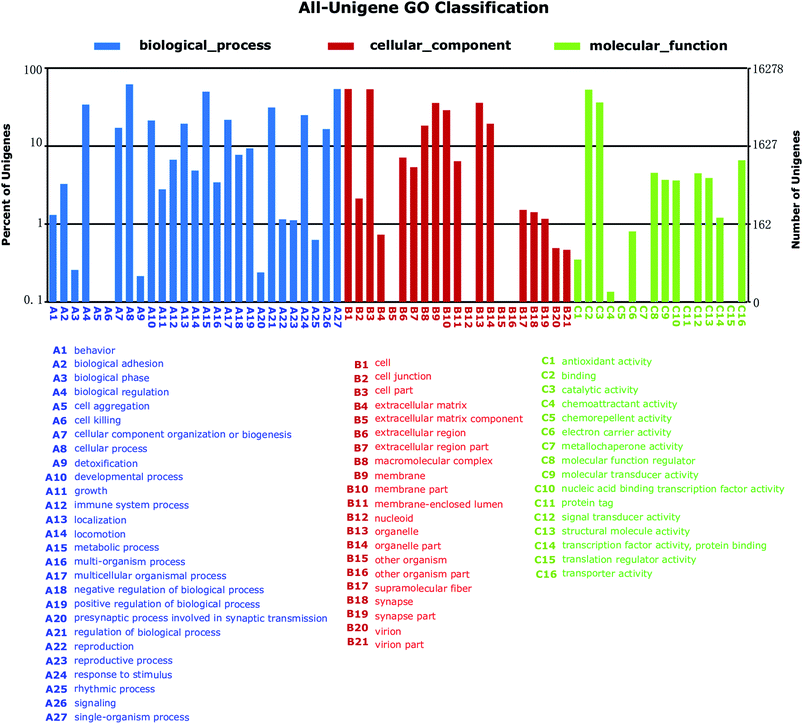 |
| Fig. 4 Gene ontology (GO) annotation (2nd level GO terms) of Schizothorax waltoni transcriptome. Unigenes were annotated by gene ontology (GO) terms which belong to three main categories: biological process, cellular component, or molecular function. | |
To further identify biological pathways of assembled unigenes in S. waltoni, we mapped these unigenes to the reference canonical pathways in the KEGG database. A total of 25
742 unigenes were hit to KEGG Orthology (KO) terms and grouped into 259 different pathways, and the number of unigenes in different pathways ranged from 2 to 2880 (Table S2†). These pathways were grouped into six level-1 KO terms: cellular process, environmental information processing, genetic information processing, human diseases, metabolism, and organismal systems. Among these unigenes, 23
677 were mapped to human diseases groups, mostly involving in pathways in cancer (1127, ko05200), influenza A (1092, ko05164), tuberculosis (855, ko05152), and dilated cardiomyopathy (848, ko05414). The second largest level-1 KO terms is the organismal systems (17
107), involving vascular smooth muscle contraction (721, ko04270), cardiac muscle contraction (707, ko04260), and NOD-like receptor signaling pathway (585, ko04621). In the third largest level-1 KO terms, the metabolic pathways was the largest pathway, which contained 2880 unigenes, followed by purine metabolism (643, ko00230), and pyrimidine metabolism (467, ko00240).
Identification of immune-related genes
To further study the immune system of S. waltoni in the following research, we identified a great deal of candidate genes involving in immune function. There are two subcategories which are closely related to immune function in GO classification: response to stimulus and immune system process, containing 4064 and 1088 unigenes, respectively. In addition, KEGG pathways were often used to identify genes associated with immune processes and their interactions. In all, 3332 immune-related unigenes were identified in 15 KEGG immune pathways (Fig. 5). Many of these immune-related unigenes are reported for the first time in S. waltoni. Top two most abundant pathways of the immune system were NOD-like receptor signaling pathway (ko04621, 585 unigenes) and leukocyte transendothelial migration (ko04670, 566 unigenes). Other immune pathways with plenty number of unigenes included chemokine signaling pathway (ko04062, 522 unigenes), complement and coagulation cascades (ko04610, 509 unigenes), and Fc gamma R-mediated phagocytosis (ko04666, 496 unigenes). In addition, important pattern recognition receptors pathways were also identified, and Toll-like receptor signaling pathway and the RIG-I-like receptor signaling pathway both contained 218 unigenes (Table S2†). With the help of these signaling pathways, we got more comprehensive and systematic information for the understanding of the S. waltoni immune system and their regulatory network.
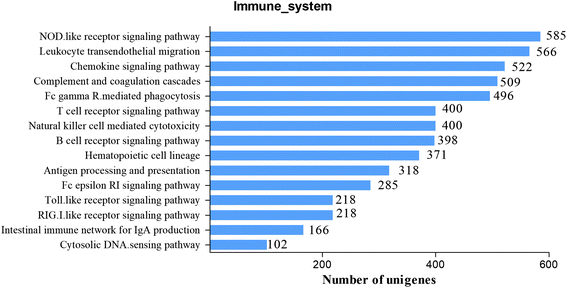 |
| Fig. 5 Identified immune-related KEGG pathways of assembled unigenes of Schizothorax waltoni. | |
NOD-like receptors are cytoplasmic pattern-recognition receptors, expressed intracellularly and have been proved to respond to a wide range of classes of bacterial wall component, ligands, toxin, and host-derived ligands, such as uric acids, damaged membrane.34 They are intracellular sentinels of cytosolic sanctity, which able to orchestrate innate immunity and inflammatory responses ensuring the detection of noxious signals within the cell.35 In this study, we identified several members in the NOD-like receptor family, including NOD1, NOD2, NLRP1, NLRP3, and NLRP12. NOD1 and NOD2 are critical receptors of minimal peptidoglycan motifs of Gram-postive bacteria and Gram-negative bacteria,36,37 which play a vital role in protecting the host against invasion by microbial pathogens.34 NLRP1, NLRP3, and NLRP12 are the key components of inflammasome.35 NLRP1 and NLRP3 can activate the inflammasome, which further activates caspase-1 giving rise to the processing and release of IL-1β and IL-18 and other targets.34,38 NLRP12 is shown as a negative regulator of immune response by brushing with NF-κB activation.39 Information on unigenes involved in NOD-like receptor signaling pathway was listed in Fig. S1 and Table S2.†
Neither the adaptive nor innate immune system responds unless leukocytes cross blood vessels.40 The migration of leukocytes is indispensable to drive immune responses, including immune surveillance, chronic and acute inflammatory.41 This process includes several distinct steps: firstly, the leukocytes are mediated by adhesion molecules and roll over the endothelial cells. When the leukocytes get close to endothelium, chemotactic cytokines activate the leukocytes. Finally, the activated leukocytes will firmly adhere to the endothelium and migrate in an ameboid fashion through the intercellular clefts between the endothelial cells and, in some cases, through the endothelial cell itself to the proper locations.40,41 In this study, we identified a large amount of important unigenes in leukocyte transendothelial migration pathway, such as intercellular cell adhesion molecule 1 (ICAM-1), vascular cell adhesion molecule 1 (VCAM-1), CD11b/CD18, CD29, CD99, junctional adhesion molecule 1 (JAM-1), junctional adhesion molecule 2 (JAM-2), integrin alpha L (ITGAL), integrin alpha M (ITGAM), integrin beta 1 (ITGB1), and integrin beta 2 (ITGB2). ICAM-1 and VCAM-1 are not involved in diapedesis per se, however, they seem to be involved in processes that directly precede diapedesis.40 They are both recruited to the endothelial cell border during transmigration in mammal. ICAM-1 is involved in the firm adhesion of leukocytes to the apical surface of endothelial cells, and VCAM-1 is involved in the firm adhesion of leukocytes and monocytes.40 Integrins play a prominent role in the control of trafficking of leukocytes during transendothelial migration.42 In addition, we have identified several negative regulators during the transendothelial migration, such as vascular endothelial cell-specific cadherin (VE-cadherin), the main adhesion molecule of the endothelial adherens junction.40,43 Although the functions of mammalian and human genes in the leukocyte transendothelial migration pathway have been studied adequately.40,41 The study of JAM-1, an important gene involved in the pathway, in grass carp (Ctenopharyngodon idellus) indicated that JAM-1 have similar expression patterns and similar functions to that in mammalian, however, similar studies on fish is rarely reported.44 These sequences information will benefit functional researches of important genes in S. waltoni. All unigenes involved in the leukocyte transendothelial migration were included in Fig. S2 and Table S2.†
Microsatellites discovery
Microsatellites, or simple sequence repeats (SSRs), are useful molecular markers for population genetic studies, genetic linkage map construction, and breeding studies.45,46 They are composed of arrays of tandemly repeated short nucleotide motifs of 1 to 6 bases, and are separately appointed to mono-, di-, tri-, tetra-, penta- and hexa-nucleotide repeats.46 Up to now, only a few microsatellite markers are available for S. waltoni.14,16,20 In this study, we detected potential microsatellites markers by the MISA package (http://pgrc.ipk-gatersleben.de/m-isa/) from all of assembled unigenes. In total, 19
497 SSRs of 1 to 6 bp unit length were identified (Table 3) in 14
690 unigenes (21.93%), and 3590 unigenes (5.36%) contained more than one SSR. This number of SSRs corresponds to a frequency of about one SSR per 3.28 kb of expressed sequences (one SSR per 5.16 kb after eliminating the mono-nucleotide repeats). This distribution density was higher than previously reported for other fish, including S. prenanti (one SSR per 9.60 kb),27 Larimichthys polyactis (one SSR per 7.50 kb),47 Paramisgurnus dabryanus (one SSR per 6.99 kb),32 comparable to Scophthalmus maximus (one SSR per 3.36 kb),48 nevertheless lower than Haliotis midae (one SSR per 0.76 kb).49 Without regard to species per se, several decisive factors can affect the distribution density of SSRs, including database-mining software, the parameters for identification of SSRs, SSRs detection standard, and dataset size.50,51
Table 3 Repeat numbers and unit length distribution of putative SSR markers in the transcriptome
Repeat numbers |
Motif length |
Total |
Percent (%) |
Mono |
Di |
Tri |
Tetra |
Penta |
Hexa |
4 |
0 |
0 |
0 |
0 |
150 |
82 |
232 |
1.19 |
5 |
0 |
0 |
1480 |
214 |
27 |
4 |
1725 |
8.85 |
6 |
0 |
2331 |
635 |
127 |
6 |
6 |
3105 |
15.93 |
7 |
0 |
1274 |
385 |
14 |
4 |
4 |
1681 |
8.62 |
8 |
0 |
785 |
221 |
13 |
4 |
2 |
1025 |
5.26 |
9 |
0 |
604 |
37 |
7 |
0 |
2 |
650 |
3.33 |
10 |
0 |
503 |
44 |
7 |
2 |
0 |
556 |
2.85 |
11 |
0 |
801 |
30 |
3 |
0 |
1 |
835 |
4.28 |
12 |
1213 |
421 |
14 |
4 |
3 |
2 |
1657 |
8.50 |
13 |
846 |
192 |
12 |
3 |
3 |
0 |
1056 |
5.42 |
14 |
668 |
191 |
12 |
1 |
1 |
0 |
873 |
4.48 |
15 |
477 |
200 |
7 |
5 |
2 |
0 |
691 |
3.54 |
16 |
374 |
159 |
6 |
8 |
2 |
0 |
549 |
2.82 |
17 |
287 |
161 |
1 |
1 |
1 |
1 |
452 |
2.32 |
18 |
238 |
114 |
0 |
3 |
0 |
0 |
355 |
1.82 |
19 |
162 |
97 |
1 |
6 |
1 |
0 |
267 |
1.37 |
≥20 |
2813 |
943 |
10 |
18 |
2 |
2 |
3788 |
19.43 |
Total |
7078 |
8776 |
2895 |
434 |
208 |
106 |
19 497 |
100 |
Percent (%) |
36.30 |
45.01 |
14.85 |
2.23 |
1.07 |
0.54 |
100 |
|
Among those SSRs, we identified 7078 (36.30%) mono-nucleotide repeats, 8776 (45.01%) di-nucleotide repeats, 2895 (14.85%) tri-nucleotide repeats, and 748 (3.84%) tetra-/penta-/hexa- nucleotide repeats (Table 3). The SSRs number of S. waltoni was obviously higher than other two Schizothorax fishes, S. prenanti (7998 SSRs) and Schizothorax biddulphi (1379 SSRs).27,52 In the study of S. prenanti, the samples came from cultured population, less quantity of SSRs possibly because that artificial culture reduced the genetic diversity. On the other hand, this might be partly due to the effective assembly of the S. waltoni transcriptome. The difference of the SSRs number between S. waltoni and S. biddulphi mainly due to use two kinds of different next generation sequencing techniques.
The copy number of repeat motifs ranged from 4 to 127. 15.93% of SSRs had the copy number of six, followed by those with five copy number (8.85%), seven copy number (8.62%), and twelve copy number (8.50%). The copy number of different repeats in the SSR sequences was distributed unequally, it is consistent with the previous studies of teleosts, such as S. prenanti,27 and P. dabryanus.32 Without regard to the mono-nucleotide repeats, 132 types of repeats motifs were found among the S. waltoni transcriptome, and di-, tri-, tetra-, penta-, and hexa-nucleotide repeats had 4, 10, 23, 46, and 49 types, respectively. The most frequent type was (AC/GT)n (5101, 41.07%), followed by (AT/AT)n (1880, 15.14%), (AG/CT)n (1766, 14.22%), (AAT/ATT)n (852, 6.86%), (ATC/ATG)n (537, 4.32%), and (AGG/CCT)n (498, 4.01%). In addition, the most abundant type in tetra- and penta-nucleotide SSRs was (AGAT/ATCT)n (105, 0.85%), and (AAAAC/GTTTT)n (31, 0.25%) (Fig. 6) respectively. Di-nucleotide repeats of S. waltoni accounted for 45.01%. (AC/GT)n motif was the most abundant repeat in di-nucleotide SSRs, consistent with previously reported in vertebrate animal species.50
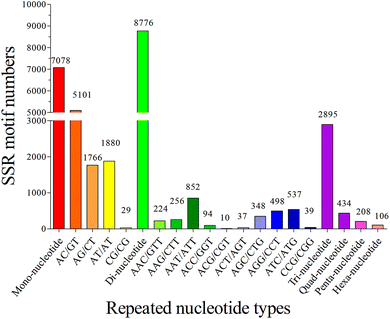 |
| Fig. 6 Frequency distribution of the top twenty most abundant SSRs based on repeated nucleotide types. Each histogram represented one detected SSR type in transcriptome of Schizothorax waltoni. | |
Materials and methods
Fish sampling
All animal procedures were performed in accordance with the guidelines for laboratory animal care and use committee of Southwest University, and approved by the Animal Ethics Committee of Southwest University (no. 20150602). In order to reduce stress, fish were anesthetized using tricaine methanesulfonate (MS222) before dissection. Three wild S. waltoni were sampled from Yarlung Tsangpo River, Tibet, China. To get the majority of expressed genes including tissue-specific ones, seven organs (heart, brain, liver, kidney, spleen, gill, and intestine) were sampled and stored in RNAlater (QIAGEN) immediately. After 4 °C overnight, the samples were transferred to a −80 °C ultra-low freezer until preparation of RNA.
RNA extraction and sequencing
For each tissue, total RNA was extracted using TRIzol reagent (Invitrogen, USA) according to the manufacturer's instructions, and incubated for 1 h at 37 °C with 10 units of DNase I (TaKaRa, Dalian, China) to eliminate genomic DNA. RNA degradation and contamination were monitored on 1% agarose gels. RNA purity, concentration, and integrity were analyzed using the NanoPhotometer® spectrophotometer (IMPLEN, CA, USA), Qubit® RNA Assay Kit in Qubit® 2.0 Fluorometer (Life Technologies, CA, USA), and RNA Nano 6000 Assay Kit of the Agilent Bioanalyzer 2100 system (Agilent Technologies, CA, USA), respectively. Equal amounts of the extracted RNA from different tissues of each fish were pooled to create a cDNA library construction. Three libraries were constructed using NEBNext® Ultra™ RNA Library Prep Kit for Illumina® (NEB, USA) with 1.5 μg of pooled total RNA following manufacturer's recommendations and index codes were added to attribute sequences to each sample. The clustering of the index-coded samples was performed on cBot Cluster Generation System using HiSeq 4000 PE Cluster Kit (Illumina) according to the manufacturer's instructions. After cluster generation, each library was sequenced on an Illumina Hiseq 4000 in 150 PE mode (Illumina Inc., San Diego, CA, USA). Short reads were deposited in the NCBI Sequence Read Archive (SRA) under accession numbers PRJNA412935.
Transcriptome de novo assembly and annotation
In order to ensure reliable assembly results, raw reads of fastq format were firstly filtered through in-house perl scripts. In this step, clean reads were obtained by removing low-quality reads (quality score lower than 20), reads containing adapter, and reads containing poly-N from raw reads. At the same time, Q20, Q30, GC-content and sequence duplication level of the clean reads were calculated. All the clean reads were assembled into transcripts by Trinity software (trinityrnaseq-2.0.6) using default parameters.53 The SOAPaligner software (Release 2.21, http://soap.genomics.org.cn/soapa-ligner.html) for short oligonucleotide alignment to remove sequences which were not covered by any sample reads, and interrupt sequences which no reads across its region. To generate effective unigenes, the assembled transcripts were processed through the TGICL (v2.1, Linux ×86) to eliminate sequence redundancy and assemble sequences with default parameters.54 Finally, the unigenes were generated, which were then used for functional annotation. All unigenes were first annotated by searching sequence homologies against the National Center for Biotechnology Information (NCBI) non-redundant protein (NR) database (released on March 14, 2016), Swiss-Prot database (released on July, 2016), Kyoto Encyclopedia of Genes and Genomes (KEGG) database (released on 59.3), Pfam databases (http://pfam.xfam.org/), and Clusters of Orthologous Groups (COG) database (released on March 31, 2009) with Blastx package (E-value < 10−5).55 Whereafter, Blastn package (E-value < 10−5) of all unigenes were performed in the NCBI non-redundant nucleotide sequence (NT) database (released on May 14, 2014).55 Gene ontology (GO) terms were extracted from the best hits using Blast2GO (v2.5.0, released on April, 2016).56 Additionally, COG and KEGG databases also used to predict and classify functions based upon Blast against NR database.57
Microsatellites discovery
Microsatellites (SSRs) with repeat unit lengths from mono- to hexa-nucleotides were detected using Microsatellite identification tool (MISA, http://pgrc.ipk-gatersleben.de/misa/misa.html). The parameters were set to identify mono-, di-, tri-, tetra-, penta-, and hexa-nucleotide motifs with a minimum of twelve, six, five, five, four, and four repeats, respectively.
Conclusion
In this work, we characterized the comprehensive transcriptome profile of S. waltoni, and identified enormous immune-related genes and microsatellite markers. The transcriptome sequences of S. waltoni will provide a valuable resource for further functional studies of important gene and its genome annotation. We identified a large amount of functional genes related to immunity, and provided the solid foundation for deep insights into the molecular mechanisms and regulatory network of the immune system in S. waltoni. In particular, the complement system, as a vital component of innate immunity in teleosts, its activation eventually leads to the direct killing pathogens, and it is worth further research. The detected SSRs markers could be used for population genetics, conservation and linkage maps construction.
Conflicts of interest
There are no conflicts to declare.
Acknowledgements
This research was supported by the Fundamental Research Funds for the Central Universities (XDJK2015C034, XDJK2017B008, XDJK2017C035), National Natural Science Foundation of China (31402302), Scientific research initiation project aided by special fund, Southwest University Rongchang Campus (20700208, 20700502), and the Youth Foundation of Southwest University Rongchang Campus (20700937, 20700938).
References
- D. Qi, Y. Chao, S. Guo, L. Zhao, T. Li, F. Wei and X. Zhao, PLoS One, 2012, 7, e34070 CAS.
- Y. Li, Z. Ren, A. M. Shedlock, J. Wu, L. Sang, T. Tersing, M. Hasegawa, T. Yonezawa and Y. Zhong, Gene, 2013, 517, 169–178 CrossRef CAS PubMed.
- S. Yang, H. Dong and F. Lei, Prog. Nat. Sci., 2009, 19, 789–799 CrossRef CAS.
- W. Cao, Y. Chen, Y. Wu and S. Zhu, in The comprehensive scientific expedition to the Qinghai-Xizang Plateau, studies on the period, amplitude and type of the uplift of the Qinghai-Xizang Plateau, Science Press, Beijing, 2000, pp. 273–388 Search PubMed.
- Y. Chen and W. Cao, in Fauna Sinica, Osteichthyes, Cypriniformes III, Science Press, Beijing, 2000, pp. 273–388 Search PubMed.
- M. R. Mirza, Pak. J. Zool., 1991, 23, 339–341 Search PubMed.
- C. Buxton, Environ. Biol. Fishes, 1993, 36, 47–63 CrossRef.
- B. Huo, C.-X. Xie, B.-S. Ma, X.-F. Yang and H.-P. Huang, Zool. Stud., 2012, 51, 185–194 Search PubMed.
- X. Zhou, C. Xie, B. Huo, Y.-J. Duan, X. Yang and B.-S. Ma, J. Appl. Anim. Res., 2017, 45, 346–354 CrossRef CAS.
- L. Yang, Y. Wang, Z. Zhang and S. He, Genome Biol. Evol., 2014, 7, 251–261 CrossRef PubMed.
- J. Wu, F. Hou, Y. Wang, B. Wu, Q. Wei and Z. Song, Conserv. Genet. Resour., 2013, 5, 891–894 CrossRef.
- Bureau of aquartic products, Tibet, China, Fishes and fish resources in Xizang, China, China Agriculture Press, Beijing, 1995 Search PubMed.
- Z. Jiang, J. Jiang, Y. Wang, E. Zhang, Y. Zhang, L. Li, F. Xie, C. Bo, L. Cao, G. Zheng, L. Dong, Z. Zhang, P. Ding, Z. Luo, C. Ding, Z. Ma, S. Tang, W. Cao, C. Li, H. Hu, Y. Ma, Y. Wu, Y. Wang, K. Zhou, S. Liu, Y. Chen, J. Li, Z. Feng, Y. Wang, B. Wang, C. Li, X. Song, L. Cai, C. Zhang, Y. Zeng, Z. Meng, H. Fang and X. Ping, Biodiversity Sci., 2016, 24, 500–551 CrossRef.
- X. Guo, G.-R. Zhang, K.-J. Wei, W. Ji, R.-B. Yang, J. P. A. Gardner and Q.-W. Wei, Conserv. Genet. Resour., 2014, 6, 413–415 CrossRef.
- Y. Chen, Q. Cheng, H. Qiao, Y. Zhu and W. Chen, Mitochondrial DNA, 2013, 24, 642–644 CrossRef CAS PubMed.
- S. S. Guo, G. R. Zhang, X. Z. Guo, K. J. Wei, W. Ji and Q. W. Wei, Russ. J. Genet., 2014, 50, 105–109 CrossRef CAS.
- H. Qiu and Y. Chen, Ichthyol. Res., 2009, 56, 260–265 CrossRef.
- P. M. Abdul-Muneer, Genet. Res. Int., 2014, 2014, 691759 CAS.
- T. Sjakste, N. Paramonova and N. Sjakste, Medicina, 2013, 49, 505–509 Search PubMed.
- X. Guo, G. Zhang, K.-J. Wei, S.-S. Guo, J. P. A. Gardner and C.-X. Xie, Biochem. Syst. Ecol., 2013, 51, 259–263 CrossRef CAS.
- R. Che, Y. Sun, R. Wang and T. Xu, PLoS One, 2014, 9, e87940 Search PubMed.
- S. Xiao, Z. Han, P. Wang, F. Han, Y. Liu, J. Li and Z. Y. Wang, PLoS One, 2015, 10, e0124432 Search PubMed.
- J. Seong, S. W. Kang, B. B. Patnaik, S. Y. Park, H. J. Hwang, J. M. Chung, D. K. Song, M. Y. Noh, S.-H. Park, G. J. Jeon, H. S. Kong, S. Kim, U. W. Hwang, H. S. Park, Y. S. Han and Y. S. Lee, Genes, 2016, 7, 114 CrossRef PubMed.
- W. Chi, X. Ma, J. Niu and M. Zou, BMC Genomics, 2017, 18, 310 CrossRef PubMed.
- R. Zhang, A. Ludwig, C. Zhang, C. Tong, G. Li, Y. Tang, Z. Peng and K. Zhao, Sci. Rep., 2015, 5, 9780 CrossRef CAS PubMed.
- C. Tong, C. Zhang, R. Zhang and K. Zhao, Fish Shellfish Immunol., 2015, 46, 366–377 CrossRef CAS PubMed.
- H. Luo, S. Xiao, H. Ye, Z. Zhang, C. Lv, S. Zheng, Z. Wang and X. Wang, PLoS One, 2016, 11, e0152572 Search PubMed.
- J.-O. Kim, J.-O. Kim, W.-S. Kim and M.-J. Oh, Genes, 2017, 8, 31 CrossRef PubMed.
- G. Li, Y. Zhao, Z. Liu, C. Gao, F. Yan, B. Liu and J. Feng, Fish Shellfish Immunol., 2015, 44, 420–429 CrossRef CAS PubMed.
- X. Song, X. Hu, B. Sun, Y. Bo, K. Wu, L. Xiao and C. Gong, Sci. Rep., 2017, 7, 40777 CrossRef CAS PubMed.
- L. Huang, G. Li, Z. Mo, P. Xiao, J. Li and J. Huang, PLoS One, 2015, 10, e0117642 Search PubMed.
- C. Li, Q. Ling, C. Ge, Z. Ye and X. Han, Gene, 2015, 557, 201–208 CrossRef CAS PubMed.
- Y. Wu and C. Wu, The fishes of the Qinghai-Xizang plateau, Sichuan Science and Technology Publishing House, Chengdu, Sichuan, China, 1991 Search PubMed.
- V. Motta, F. Soares, T. Sun and D. J. Philpott, Physiol. Rev., 2015, 95, 149–178 CrossRef PubMed.
- F. Barbé, T. Douglas and M. Saleh, Cytokine Growth Factor Rev., 2014, 25, 681–697 CrossRef PubMed.
- S. E. Girardin, I. G. Boneca, L. A. M. Carneiro, A. Antignac, M. Jéhanno, J. Viala, K. Tedin, M.-K. Taha, A. Labigne, U. Zähringer, A. J. Coyle, P. S. DiStefano, J. Bertin, P. J. Sansonetti and D. J. Philpott, Science, 2003, 300, 1584–1587 CrossRef CAS PubMed.
- S. E. Girardin, I. G. Boneca, J. Viala, M. Chamaillard, A. Labigne, G. Thomas, D. J. Philpott and P. J. Sansonetti, J. Biol. Chem., 2003, 278, 8869–8872 CrossRef CAS PubMed.
- F. Martinon, K. Burns and J. Tschopp, Mol. Cell, 2002, 10, 417–426 CrossRef CAS PubMed.
- I. C. Allen, J. E. Wilson, M. Schneider, J. D. Lich, R. A. Roberts, J. C. Arthur, R.-M. T. Woodford, B. K. Davis, J. M. Uronis, H. H. Herfarth, C. Jobin, A. B. Rogers and J. P.-Y. Ting, Immunity, 2012, 36, 742–754 CrossRef CAS PubMed.
- W. A. Muller, Annu. Rev. Pathol.: Mech. Dis., 2011, 6, 323–344 CrossRef CAS PubMed.
- J. D. van Buul and P. L. Hordijk, Arterioscler., Thromb., Vasc. Biol., 2004, 24, 824–833 CrossRef CAS PubMed.
- T. M. Carlos and J. M. Harlan, Blood, 1994, 84, 2068–2101 CAS.
- U. Gotsch, E. Borges, R. Bosse, E. Böggemeyer, M. Simon, H. Mossmann and D. Vestweber, J. Cell Sci., 1997, 110(Pt 5), 583–588 CAS.
- F. Du, J. Su, R. Huang, L. Liao, Z. Zhu and Y. Wang, Fish Shellfish Immunol., 2013, 34, 1476–1484 CrossRef CAS PubMed.
- K. A. Selkoe and R. J. Toonen, Ecol. Lett., 2006, 9, 615–629 CrossRef PubMed.
- A. Grover and P. C. Sharma, Crit. Rev. Biotechnol., 2016, 36, 290–302 CrossRef CAS PubMed.
- L. Liu, Y. Sui, W.-B. Zhu, A. Guo, K.-D. Xu and Y.-D. Zhou, Mar. Genom., 2017, 33, 27–29 CrossRef PubMed.
- D. Ma, A. Ma, Z. Huang, G. Wang, T. Wang, D. Xia and B. Ma, PLoS One, 2016, 11, e0149414 Search PubMed.
- P. Franchini, M. van der Merwe and R. Roodt-Wilding, BMC Res. Notes, 2011, 4, 59 CrossRef CAS PubMed.
- G. Tóth, Z. Gáspári and J. Jurka, Genome Res., 2000, 10, 967–981 CrossRef.
- R. K. Varshney, A. Graner and M. E. Sorrells, Trends Biotechnol., 2005, 23, 48–55 CrossRef CAS PubMed.
- W. Luo, Z. Nie, F. Zhan, J. Wei, W. Wang and Z. Gao, Int. J. Mol. Sci., 2012, 13, 14946–14955 CrossRef CAS PubMed.
- M. G. Grabherr, B. J. Haas, M. Yassour, J. Z. Levin, D. A. Thompson, I. Amit, X. Adiconis, L. Fan, R. Raychowdhury, Q. Zeng, Z. Chen, E. Mauceli, N. Hacohen, A. Gnirke, N. Rhind, F. di Palma, B. W. Birren, C. Nusbaum, K. Lindblad-Toh, N. Friedman and A. Regev, Nat. Biotechnol., 2011, 29, 644–652 CrossRef CAS PubMed.
- G. Pertea, X. Huang, F. Liang, V. Antonescu, R. Sultana, S. Karamycheva, Y. Lee, J. White, F. Cheung, B. Parvizi, J. Tsai and J. Quackenbush, Bioinformatics, 2003, 19, 651–652 CrossRef CAS PubMed.
- S. F. Altschul, T. L. Madden, A. A. Schäffer, J. Zhang, Z. Zhang, W. Miller and D. J. Lipman, Nucleic Acids Res., 1997, 25, 3389–3402 CrossRef CAS PubMed.
- A. Mortazavi, B. A. Williams, K. McCue, L. Schaeffer and B. Wold, Nat. Methods, 2008, 5, 621–628 CrossRef CAS PubMed.
- M. Kanehisa, M. Araki, S. Goto, M. Hattori, M. Hirakawa, M. Itoh, T. Katayama, S. Kawashima, S. Okuda, T. Tokimatsu and Y. Yamanishi, Nucleic Acids Res., 2008, 36, D480–D484 CrossRef CAS PubMed.
Footnote |
† Electronic supplementary information (ESI) available. See DOI: 10.1039/c8ra00619a |
|
This journal is © The Royal Society of Chemistry 2018 |