DOI:
10.1039/C7RA12952A
(Paper)
RSC Adv., 2018,
8, 15831-15841
Metabolomics analysis of multidrug-resistant breast cancer cells in vitro using methyl-tert-butyl ether method†
Received
1st December 2017
, Accepted 21st April 2018
First published on 26th April 2018
Abstract
The comprehensive characterization of metabolome and lipidome to reveal unknown pathological conditions, are being used to investigate the molecular mechanisms of cancer, especially in the field of early diagnosis, treatment, and prognosis. The multidrug resistance (MDR) of tumor cells limits the therapeutic effect of anti-cancer drugs and is the main obstacle for chemotherapy. Here, we adopted a methyl-tert-butyl ether (MTBE)-based extraction method to simultaneously extract small polar molecules and lipophilic metabolites for nontargeted metabolomics of multidrug-resistant breast cancer cell line MCF-7/ADR and its parental cell line MCF-7/S by ultra-performance liquid chromatography coupled with quadrupole time-of-flight mass spectrometry. Distinctly different metabolic features were shown between MCF-7/ADR cells and MCF-7/S on the basis of multivariate analyses. 17 potential biomarkers were identified. And these potential biomarkers were mainly correlated with cell membrane lipids composition, cell signaling regulated by lipids, and anti-oxidation ability. The studies of cellular ultrastructure and morphology by in situ atomic force microscopy (AFM) also demonstrated the cellular membrane changed along with the MDR. We expect that this study could provide a new method for monitoring drug resistance during clinical chemotherapy and be useful for the development of drugs to overcome the MDR.
Introduction
Breast cancer is one of the most common malignancies in women. Along with surgery, radiotherapy, hormonal therapy and targeted antibodies, chemotherapy is often used to treat breast cancer and anthracyclines are widely used in the chemotherapy of breast cancer.1 Adriamycin (ADR) as anthracycline drug is most widely used in the chemotherapy of breast cancer.2,3 It belongs to cell cycle non-specific drugs and its action mechanisms have been known.4 But multidrug resistance (MDR) usually occurs, which is a major obstacle for breast cancer treatment. MDR possesses multifactorial natures. The main drivers for MDR include the increased levels of detoxification enzymes, the alterations of uptake and efflux of drugs, the changes of drug target enzymes, enhanced DNA repair and deregulation of survival/apoptosis signaling pathways.5–8 A complex network exists in multidrug-resistant cells to assist tumor progression. The most classical mechanism for MDR is the overexpression of drug efflux proteins, in which, P-glycoprotein (P-gp) is widely known. Although three generations of P-gp inhibitors have been developed, only a few were really used in clinical,9 so the development of inhibitors for MDR is gaining more and more attention. Understanding how these drivers responsible for MDR synergistically and systematically work is the prerequisite for effectively reversing MDR.
Although genomics and proteomics have been used to investigate the MDR mechanisms, and several related genes and proteins have been found, the investigation of alterations of global metabolites at the molecular level between the sensitive and resistant breast tumor cells is more needed. This is because the metabolome is the endpoint of omics cascade and the most closeness to the phenotypes.10 Lipidomics is a branch of metabolomics. Lipids participate in various biological processes, such as the constituent of cellular membranes, energy storage, cell motility and adhesion, and apoptosis.11 It has been demonstrated that human cancers are associated with disruption of lipid metabolism and/or lipids-related signaling pathways.12 Lipid-based biomarkers have been investigated as tools for tumor diagnosis,13 grade,14 subtyping,15 metastasis,16 and prognosis.17 For example, higher plasma levels of lysophosphatidylcholine 18:0 are related to a lower risk of common cancers.18 Therefore, the identification of metabolites and lipids as biomarkers plays critical roles in the tumor research.
The gold-standard Folch and Bligh–Dyer extraction procedure are two of the common methods based on a biphasic solvent system for lipids extraction. But both systems contain chloroform, which can be toxic and cause chemical modification of labile lipid species. In addition, both the Folch and Bligh–Dyer methods are easy to bring contamination for the collection of the lipids from the lower phase of the two-phase system because the insoluble materials are between the two phases, which is not beneficial for subsequent analysis.19 Matyash et al. have developed a halogenate-free method where lipids are enriched in the upper phase by using a lower density of methyl-tert-butyl ether (MTBE) in combination with methanol and water. And the precipitate and insoluble materials are at the bottom of the tube in this method, so that the lipids in the up layer are easily collected. By this method, we can accomplish the analysis of the two different types of compounds, lipophilic and water-soluble, in one experiment, thus which can save time and dosage of sample compared with the conventional methods.20
The first step that cytotoxic drugs enter the cell interior is the interaction of drugs and cell membrane. The changes of lipids or the proteins located in the cell membrane could affect the membrane permeability and fluidity. And it's been known that the overexpression of transmembrane protein P-glycoprotein (P-gp) plays a crucial role in the multidrug resistance. Therefore, investigating the microstructure of cell membrane is very useful for the research of the interaction of drugs and cell membrane. Atomic Force Microscopy (AFM) is a powerful tool for researching the structure of cellular membrane surface at the nanometer scale, providing the information of changes in the biophysical properties of the cell, such as roughness, elasticity, viscosity, and so on. It's a great advantage for studying cells including living and fixed cells that no complex sample preparations are needed.
Therefore, in this work, AFM was used to observe the microstructure of cellular membrane surface and the cell size. The cell surface roughness was detected by the topography to further understand the changes of the cell membrane surface induced by the MDR. And UPLC-Q-TOF-MS-based metabolomics and lipidomics were employed to profile intracellular metabolites and lipids in MCF-7/ADR and parental MCF-7/S cells by MTBE extraction. The potential biomarkers were identified by multivariate statistical analysis to explore the mechanism of MDR. By building the metabolic network correlated with potential biomarkers and analysing metabolic pathways, we investigated the relationship between MDR and metabolic perturbation on MCF-7/S cells in vitro. The results of this study are useful for understanding the molecular basis of MDR and even discovering new and effective targets to diagnose or reverse resistance.
Experimental
Reagents and materials
HPLC-grade chloroform, methanol, acetonitrile and formic acid (Fisher Scientific, Loughborough, UK) were used. Methyl-tert-butyl ether (MTBE) was purchased from Aladdin Co., Ltd. (Shanghai, China). Ammonium acetate was taken from Sigma-Aldrich (St. Louis, MO, USA). Ultrapure water was produced by a Milli-Q plus (Milford, MA, USA) water purification system. MCF-7/ADR cells were purchased from Keygen Biotech Co., Ltd. (Nanjing, China) and parental MCF-7/S cells were purchased from Chinese Academy of Medical Science. Adriamycin was purchased from Hisun Pharm Co., Ltd. (Taizhou, China). RPMI-1640 medium was taken from Hyclone, China. Fetal bovine serum was purchased from Hangzhou Sijiqing Co., Ltd. (Hangzhou, China). Penicillin and streptomycin were purchased from Beijing Dingguo Co. Ltd. (Beijing, China). Leucine enkephalin was obtained from Waters (Milford, USA).
Cell culture
The MCF-7/ADR cells were established by exposing parental MCF-7/S cells to increasing concentrations of adriamycin (from 0.01 to 1 μg mL−1). After cells could survive in 1 μg mL−1 adriamycin, they were maintained in medium containing 1 μg mL−1 adriamycin for two months to obtain MCF-7/ADR cells. Human breast cancer cell line MCF-7/ADR and MCF-7/S were maintained in RPMI-1640 medium containing 10% (v/v) fetal bovine serum, 100 U mL−1 penicillin, 100 μg mL−1 streptomycin. To maintain the drug-resistant phenotype, MCF-7/ADR cells were grown in RPMI-1640 medium supplemented with 1 μg mL−1 ADR and were grown in adriamycin-free culture medium for 1 week before the experiment. All cells were cultured at 37 °C in a humidified atmosphere of 5% CO2. The culture medium was changed every 2–3 days. The established MCF-7/ADR and parental MCF-7/S cells with the same passage number (the 35th generation) were used in our experiment.
Drug sensitivity assay
Drug sensitivity was detected by colorimetric MTT assay. When MCF-7/ADR and MCF-7/S cells grew to nearly 80% confluence, these cells were trypsinized and seeded in 96-well plates with 100 μL of cell suspension solution at a density of 5000 cells per well. After adhering overnight, the cells were treated with 100 μL of different concentrations of adriamycin solution (0, 1, 2, 4, 8, 16, 32, 64, and 128 μM, respectively) and incubated for an additional 48 h. After that time, the medium was replaced with fresh-prepared RPMI-1640 medium, followed by incubation with 20 μL of 5 mg mL−1 solution of MTT at 37 °C for 4 hours. Then the medium was completely removed and 150 μL of DMSO was added to each well to resolve the formazan product. Finally, the plate was incubated for 10 minutes on a shaker at 37 °C and the optical density (OD) at 570 nm was measured using a SpectraMax i3 (Molecular Devices, USA) microplate reader. The IC50 values were calculated by the GraphPad Prism 5 software (GraphPad Software, USA). Each concentration had 6 reduplicative wells and was repeated at least three times. Adriamycin was dissolved in water to prepare a 2 mM stock solution, further diluted with the RPMI-1640 medium to the desired concentrations.
The observations of cell ultrastructure alterations by AFM
The MCF-7/S cells and MCF-7/ADR cells were seeded in a 6-well plate with pre-placed sterile slides at a density of 2 × 105 cells per well. After 48 h, the medium was discarded, and the adherent cells were washed with cold PBS twice immediately, followed by dryness in the stream of nitrogen. The MCF-7/S cells and MCF-7/ADR cells were imaged on the Dimension Icon AFM (Veeco, Plainview, NY, USA) using silicon nitride probe (model SCANASYST-AIR, Veeco, USA) in the ScanAsyst mode, which is an imaging mode with automatic image optimization technology. The parameters of the AFM probe used in this experiment were as follows: a resonance frequency of 50–90 kHz, spring constant of 0.4 N m−1, and cantilever thickness of 0.55–0.75 μm. All AFM experiments were performed in air at ambient temperature. Twelve atomic force microscopic images were acquired for 6 pieces of glass slide where two single cells on each glass slide were selected to observe for each cell line. The 40 × 40 μm2 scan area was performed to obtain the single whole cell, and the 10 × 10 μm2 local area of the cell was continuously imaged. All the images were acquired with a 512 × 512 data point resolution. The shape, height and size of two cell lines were analyzed. And the roughness was obtained by analyzing four selected locations (5 × 5 μm2 area) at the centre and side regions of each cell from each cell line. The arithmetic mean roughness Ra and root mean square roughness Rq on the height image were used to assess the average roughness of a given area. The overall weighted mean surface roughness was calculated according to the previously reported method.21 The images were analyzed by the NanoScope analysis software 1.8 (Bruker, Santa Barbara, CA, USA).
Cell sample preparation and metabolite extraction
For metabolomics and lipidomics analyses, MCF-7/ADR and MCF-7/S cells were seeded in 6-well plates at a density of 2 × 105 cells per well for 48 h. After that, the medium was discarded, and the adherent cells were washed with cold PBS twice immediately, followed by addition of 200 μL of cold ultrapure water to each well. Finally, the plates were stored at −80 °C until extraction.
The polar metabolites and lipids extractions were based on a MTBE–methanol–H2O (20
:
6
:
5.8, v/v/v) extraction protocol (modified slightly).19,20,22 600 μL −20 °C methanol were added to each well, and cells were scraped and collected to centrifuge tubes. Then these tubes were vortexed. After that, 2 mL of MTBE was added and the mixtures were shaken for 1 h at room temperature. Phase separation was induced by adding 380 μL of water, standing for 10 min at room temperature and the samples were centrifuged at 1000 × g, 4 °C for 10 min. The upper phase containing nonpolar metabolites was collected, and the lower phase was re-extracted with 1 mL of the MTBE–methanol–water (10
:
3
:
2.5, v/v/v) mixture, that was corresponding to the ratio of the different compositions of the upper phase. The two phases were filtered through 0.22 μm membrane filter, and dried under a stream of nitrogen. Then the dried upper layers were resuspended in 200 μL of isopropanol
:
acetonitrile
:
water (2
:
1
:
1), and the lower layers were resuspended in 100 μL of initial mobile phase and then transferred to glass autosampler.
UPLC-MS conditions
Waters Acquity UPLC system equipped with a Q-TOF SYNAPT G2 High Definition Mass Spectrometer (HDMS) (Waters Crop., Manchester, UK) was used for the analysis of lipid-and water-soluble metabolites. Each sample was injected into a reversed phase BEH C18 column (2.1 mm × 50 mm, 1.7 μM, Waters Corp., Milford, MA) kept at 37 °C. For the metabolite analysis of bottom layer, acetonitrile (A) and 0.1% aqueous formic acid (v/v) (B) were used as mobile phase and the sample inject volume was 5 μL. The flow rate was 0.3 mL min−1. In the positive ion mode, the mobile phase gradient was as follows: 0–2 min, 5–35% A; 2–5 min, 35–45% A; 5–5.5 min, 45–54% A; 5.5–6 min, 54–67% A; 6–8.5 min 67–84% A; 8.5–14.5 min 84–90% A; 14.5–18 min, 90–100% A; 18–18.5 min, 100–5% A and kept at 5% A for 3 minutes. In the negative ion mode, the mobile phase gradient was as follows: 0–1 min, 5–50% A; 1–3.5 min, 50–80% A; 3.5–8.5 min, 85–98% A; 8.5–9 min, 98–100% A and maintained 100% A for 3 minutes, followed by equilibration with 5% A for 3 minutes. The mobile phases used for the lipidomic analysis were isopropanol–acetonitrile (90
:
10, v/v) (A) and acetonitrile–water (60
:
40, v/v) (B), both containing 10 mM ammonium formate and 0.1% formic acid. In the positive ion mode, the gradient elution was as follows: 0–3 min, 15–25% A; 3–5 min, 25–50% A; 5–11 min, 50–69% A; 11–17.5 min, 69–97% A; 17.5–21.5 min, 97–100% A; 21.5–22 min, 100–15% A, and held for 3 minutes. In the negative ion mode, the gradient elution was as follows: 0–1 min, 25% A; 1–2 min, 25–50% A; 2–9.5 min 50–97% A, followed by equilibration with 25% A for 3 minutes. The flow rate was 0.4 mL min−1. The injection volume was 2 μL, and the temperature of autosampler was maintained at 4 °C.
The mass spectrometer with electrospray ionization (ESI) source was operated in positive and negative modes over the mass range from 50 to 1200 Da. In positive ion mode, the capillary voltage and cone voltage were set at 2.8 kV and 30 V, while at 2.2 kV and 30 V in negative ion mode, respectively. The source temperature and desolvation gas temperature were set at 110 °C and 350 °C, respectively. Nitrogen was respectively used as cone gas and desolvation gas. The flow rates of cone gas and desolvation gas were set at 50 L h−1 and 600 L h−1, respectively. Argon was used as the collision gas in the MS/MS experiments and collision energy was adjusted according the mass fragment spectrum. Data were acquired in centroid mode. Leucine enkephalin at a concentration of 2 ng μL−1 and with a flow rate of 5 μL min−1 was used as the lock mass.
Data processing and analysis
The acquired data were processed using MassLynx V4.1 software (Waters) to give a data matrix consisting of the retention time, m/z, and abundance value for each ion, and EZinfo 2.0 software was used to analyze the data matrices and identify statistically significant ions, where principal component analysis (PCA) and orthogonal partial least-squares discriminant analysis (OPLS-DA) were performed. The score plot and S-plot based on OPLS-DA model were taken for class separation and showed variables contributing to the classification. The potential metabolites were screened based on the variable importance in projection (VIP) value of VIP > 1 and p < 0.05 by using Student's t-test.
These potential ions were searched against the freely available metabolome databases, HMDB, Lipid MAPS, and METLIN. Once the potential metabolites had been identified, they were confirmed by matching fragmentation patterns and retention times. Only those that matched the known metabolite identities were conducted for further analysis. The metabolic pathways associated with these putative metabolites were evaluated with MetaboAnalyst 3.0, which is available online (http://www.metaboanalyst.ca). The metabolic network was visualized by MetScape, a plug-in CytoScape (v.3.4.0) software to provide the relationship between metabolites and genes and interpret the metabolomics data.
Results
Drug sensitivity of MCF-7/ADR cells to adriamycin
The IC50 value was an indicator of drug sensitivity. As shown in Fig. S1,† MCF-7/ADR cells had a significantly higher IC50 value for the adriamycin compared with parental MCF-7/S cells. The IC50 values of MCF-7/S and MCF-7/ADR cells were 6.22 ± 0.87 μM, 36.43 ± 1.52 μM, respectively. And the IC50 values of established MCF-7/ADR cells were also in comparison with that of the purchased MCF-7/ADR cells. The IC50 values of purchased MCF-7/ADR cells were 18.92 ± 1.55 μM. This means that higher levels of adriamycin are required to achieve same cytotoxic effects for MCF-7/ADR cells than that of MCF-7/S cells, i.e., MCF-7/ADR cells were resistant to adriamycin, and the established MCF-7/ADR cells were more resistant than purchased MCF-7/ADR cells.
AFM observations for surface morphology and nanomechanical properties of single MCF-7/S and MCF-7/ADR cell
Fig. 1(A1 and B1) show the morphologic information of the two cell lines. The AFM imaging revealed that the morphology of the MCF-7/ADR cell was spheroidal structure instead of the normal elongated shape of the MCF-7/S cell and the diameter of the MCF-7/ADR cell was 1.36 ± 0.24 μm instead of the 1.22 ± 0.35 μm of the MCF-7/S cell. The height of MCF-7/ADR cell was 25.27 ± 3.73 μm, and the MCF-7/S cell was 22.73 ± 3.52 μm, indicating that the average in height of cells increased slightly with the MDR, nevertheless the size and height of cell were not significantly different between the MCF-7/S cell and MCF-7/ADR cell (p > 0.05).
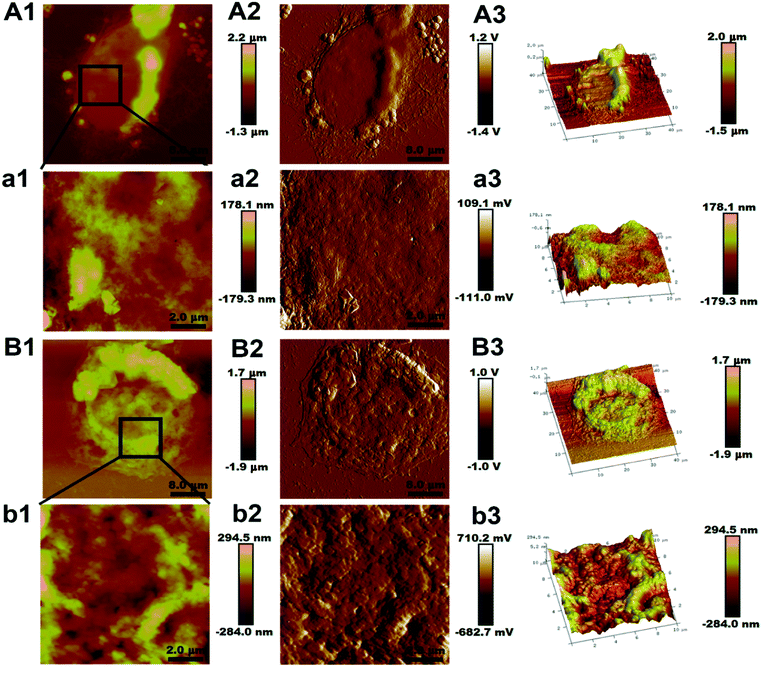 |
| Fig. 1 Representative AFM images of MCF-7/S and MCF-7/ADR cells. The first column (A1 and B1) and third column (A3 and B3) show height and three dimensional images of the entire MCF-7/S and MCF-7/ADR cells, respectively. The second column (A2, a2, B2 and b2) are peak force error images to highlight the fine features in the images. The first column (a1 and b1) and third column (a3 and b3) are the high-resolution zoomed height and three dimensional images (10 × 10 μm2) of the cells, respectively. The colors in the images indicate different heights with light and dark colors corresponding to higher and lower topography, respectively. | |
Fig. 1(a1 and b1) are the high-resolution magnified images to show the ultrastructure of the cell membrane surface. The cell membrane architecture of MCF-7/S cell was relatively smooth, fewer protrusions were on the cell membrane surface, but surface protrusions on the surface of MCF-7/ADR cell were higher and rougher. The surface roughness was used to quantify changes of the cancer cell topography. As shown in Table 1, the valves of Ra and Rq of two different areas on one cell show significant differences on the two cell lines. The Ra and Rq valves of MCF-7/S cells were minimum, indicating the smooth surface shown in Fig. 1(a1 and b1), but the Ra and Rq valves of MCF-7/ADR cells increased, indicating a rougher surface. Moreover, the valves of surface roughness at the edge region were higher than the ones at the central region, indicating the topographical heterogeneity of the membrane surface of cells.
Table 1 Average roughness of MCF-7/S and MCF-7/ADR cells in the different regions (the centre and edge region of the cell)
Cell line |
Edge region |
Central region |
Ra (nm) |
Rq (nm) |
Ra (nm) |
Rq (nm) |
MCF-7/S |
52.52 ± 1.75 |
70.95 ± 2.28 |
24.62 ± 0.80 |
29.17 ± 0.98 |
MCF-7/ADR |
76.45 ± 1.06 |
105.83 ± 1.35 |
44.52 ± 0.92 |
60.18 ± 1.51 |
The changes of polar and non-polar metabolites following MTBE extraction in MCF-7/ADR and parental cells
The untargeted lipidomics and metabonomics were carried out using UPLC-Q-TOF-MS in positive and negative ion modes. The typical base-peak ion (BPI) chromatograms from the non-polar and polar metabolites of MCF-7/ADR are shown in Fig. S2.† The further observation of global metabolic differences between the drug-resistant and parental MCF-7/S cells was performed by pattern recognition analysis. A principal component analysis (PCA) was initially used to obtain a comprehensive comparison and identify outliers of the LC-MS data from different groups. PCA scores plot based on polar and non-polar metabolites in both positive and negative ion modes (Fig. 2 and S3†) all showed good separations among different groups, which indicates the endogenous substances were disturbed significantly due to the emergence of drug resistance.
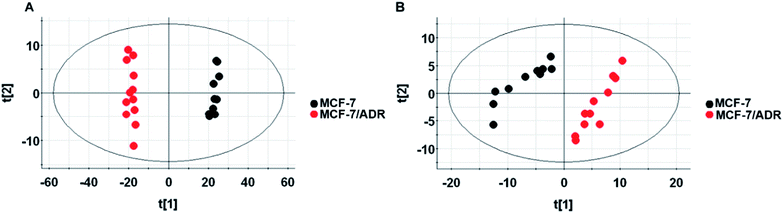 |
| Fig. 2 Scores plot of lipid metabolites in positive ion mode (A) and negative ion mode (B). Scores plot of PCA model with MS data of metabolites from the MCF-7/S (black) and MCF-7/ADR (red) cells. | |
Identification of potential biomarkers
To identify the main metabolites responsible for the separation between drug-resistant and drug-sensitive groups, S-plots obtained from OPLS-DA analysis was used (Fig. 3, S4 and S5†). In the S-plots, the distance of variables from the origin is positively related to their importance for the group separation, which means variables far away from the origin would have greater contribution to group discrimination. Moreover, the variable importance in the projection (VIP) value was used to quantify the contribution. In this paper, variables with VIP values of >1 were selected. The selected variables were confirmed by Student's t-test (p < 0.05). Finally, 4 polar metabolites and 13 lipids based on the conditions above (Table 2) were considered as the potential biomarkers for further study. The accurate molecular weights and fractional isotope abundance of these potential biomarkers provided by Q-TOF-MS were used to presume their molecular formulas. And the structures of these possible compounds were obtained by searching freely accessible databases such as Lipid MAPS and HMDB. The potential biomarkers were identified finally according to the mass fragments and retention time present in commercially available mass spectral database or literatures. The identification of Cer(d18:0/16:0) or Cer(d16:0/18:0) was taken as an example. The m/z 540.5350 at retention time (Rt) 9.04 min was targeted and monitored. Fig. 4A is the extracted ion chromatogram (EIC) of 540.5350, and Fig. 4B is the MS2 spectrum. The m/z 540.5350 was inputed to databases in the forms of [M + H]+, [M + Na]+, [M + NH4]+ adducts in the positive mode within mass error of 10 ppm, to which many known metabolites corresponded, so the possible molecular formula C34H69NO3 would be assigned to the potential biomarker based on elemental composition and fractional isotope abundance. Based on the obtained MS/MS spectrum, fragment ions were primarily observed at 311.2950, 284.2935, 283.2636, 274.2752, and 256.2639. The most abundant ion at m/z 256.2639 ([M + H − 284]+) corresponded to the fatty acyl moiety as a protonated amide ion ([C15H31CONH3]+, 256.2635), indicating the fatty acid substituent. And the less abundant product ion at m/z 311.2950 ([M + H − 229]+) was also individually observed in the MS2 spectra of m/z 512.5037 and 484.4726. So it's inferred that the ion was irrelevant to the the fatty acid substituent. The ion at m/z 311 might arise from the neutral loss of H2O and the fatty acid substituent. The loss of the fatty acyl substituent as a ketene (C16H33CH
CO) from the m/z 540.5350 yielded the m/z 274.2752, indicating the backbone of ceramide may be amino alcohol containing 16 carbon atoms. Moreover, the less abundant product ion at m/z 284.2935 ([M + H − 256]+) is the characteristic fragment ion of dihydroceramides or the protonated amide ion ([C17H35CONH3]+). Given these fragments, the Cer(d18:0/16:0) or Cer(d16:0/18:0) might be assigned to the m/z 540.5350. The other peaks were also identified by this method.
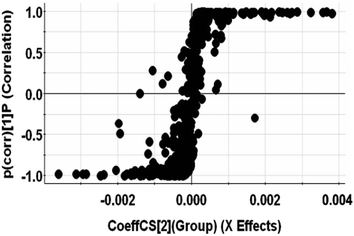 |
| Fig. 3 S-plot of OPLS-DA model with MS data of non-polar metabolites in positive ion mode. | |
Table 2 Potential biomarkers identified from metabolites of MCF-7/ADR cells compared to MCF-7/S cells and their content changes in two groups. Fold change value was the ratio of average value of the metabolite in the MCF-7/ADR cells to that of the MCF-7 cells
Phase |
Rt (min) |
Measured mass |
Ion mode |
Identification |
Formular |
VIP value |
Error (ppm) |
Fold change |
Fragment ions |
Organic phase |
1.57 |
302.3062 |
[M + H]+ |
Sphinganine |
C18H39NO2 |
1.35 |
+2.8 |
1.6 |
284.2858, 88.0731, 74.0958 |
2 |
314.3423 |
[M + NH4]+ |
Thromboxane |
C20H40O |
1.38 |
+1.8 |
10 |
297.3366, 256.3004, 187.1117, 171.0805, 139.1144, 121.1013, 93.0705 |
2.19 |
398.3632 |
[M + H]+ |
Behenoylglycine |
C24H47NO3 |
1.17 |
+0.8 |
5.2 |
338.3423 |
2.84 |
330.3375 |
[M + NH4]+ |
Phytanic acid |
C20H40O2 |
2.57 |
+2.6 |
5.6 |
312.3240, 286.3156, 88.0733 |
5.93 |
391.2849 |
[M + H]+ |
9′-Carboxy-alpha-chromanol |
C24H38O4 |
1.27 |
+1.6 |
0.5 |
149.0197 |
6 |
621.3105 |
[M + H]+ |
1-Arachidonoylglycerophosphoinositol |
C29H49O12P |
1.92 |
+11.4 |
0.7 |
509.1788, 338.3338, 284.2907 |
6.77 |
484.4726 |
[M + H]+ |
Cer(d18:0/12:0) or Cer(d14:0/16:0) |
C30H61NO3 |
4.49 |
+0.4 |
7.9 |
311.2950, 283.2634, 228.2325, 200.2015, |
7.85 |
512.5037 |
[M + H]+ |
Cer(d18:0/14:0) or Cer(d16:0/16:0) |
C32H65NO3 |
3.34 |
0 |
2.9 |
311.2949, 283.2639, 256.2644, 228.2328 |
9.04 |
540.5350 |
[M + H]+ |
Cer(d18:0/16:0) or Cer(d16:0/18:0) |
C34H69NO3 |
2.33 |
0 |
3.2 |
311.2950, 284.2935, 283.2636, 274.2752, 256.2639 |
9.37 |
732.5523 |
[M + H]+ |
PC(14 : 1/18 : 0) |
C40H78NO8P |
1.08 |
−2.0 |
0.2 |
591.5342, 448.2855, 380.3184, 184.0713 |
9.4 |
758.5681 |
[M + H]+ |
PC(16:1/18:1) |
C42H80NO8P |
1.26 |
−1.8 |
0.3 |
617.5326, 504.3477, 478.3283, 184.0691 |
10.28 |
760.5838 |
[M + H]+ |
PC(18:1/16:0) |
C42H82NO8P |
1.64 |
−1.7 |
0.3 |
673.5298, 619.5351, 504.3477, 478.3283, 184.0716 |
3.39 |
423.3259 |
[M − H]− |
Alpha-tocotrienol |
C29H44O2 |
7.59 |
−2.3 |
8.2 |
256.0334, 205.8767 |
Aqueous phase |
6.04 |
516.3043 |
[M + H]+ |
LysoPC(18:4) |
C26H46NO7P |
1.53 |
−8.1 |
0.6 |
498.2974, 258.1108, 184.0720 |
7.43 |
246.2438 |
[M + NH4]+ |
Myristic acid |
C14H28O2 |
3.9 |
+4.3 |
2.1 |
228.2303, 202.2483, 184.2173, 88.0771 |
1.17 |
128.0354 |
[M − H]− |
5-Oxoprolinate |
C5H7NO3 |
1.13 |
+0.6 |
0.6 |
110.0220, 84.0446 |
2.13 |
165.0557 |
[M − H]− |
Guaiacyl acetate |
C9H10O3 |
1.28 |
0 |
0.3 |
123.0430, 109.0320 |
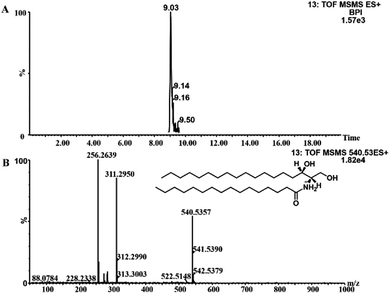 |
| Fig. 4 The MS/MS experiment of m/z 540.5350 in positive ion. (A) is the extracted ion chromatogram (EIC) of m/z 540.5350, (B) is the MS2 spectrum mode. | |
Heat map of all the differential metabolites was conducted to visualize the relative concentration of these potential biomarkers in each sample. On the heat map (Fig. 5), the up-regulation or down-regulation of the potential biomarkers in the drug-resistant groups compared to the drug-sensitive groups was expressed with different colors, thereby the changes of the patterns in metabolite concentrations across samples are observed easily. The higher levels of dihydroceramide and the lower levels of phosphatidylcholines (PCs) and lysophosphatidyl-cholines (LPCs) were shown clearly in the drug resistant MCF-7/ADR cells.
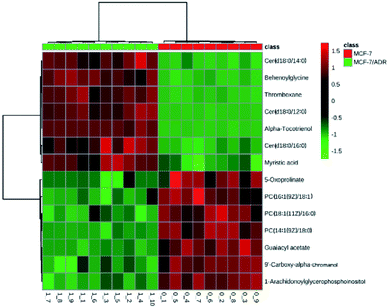 |
| Fig. 5 The heat map of metabolite levels in MCF-7/ADR and parental cells. The columns represent samples in different experimental conditions, and the rows represent different biomarkers. Different colors represent the concentrations in different samples. | |
Metabolic pathway analysis on biomarkers
The metabolic pathways related to drug resistance were determined by MetaboAnalyst 3.0. The most relevant pathways existed in the present experimental condition could be identified based on the results obtained by using the pathway analysis, integrating results from powerful pathway enrichment analysis with the pathway topology analysis. By inputting the potential biomarkers that were significantly influenced by drug resistance, all the matched pathways were then presented graphically in Fig. 6 as well as in Table S1.† From Fig. 6, we can see that the sphingolipid metabolism, glycerophospholipid metabolism, arachidonic acid metabolism and glutathione metabolism were recognized as the most important metabolic pathways associated with drug resistance. To reveal the biochemical relationship among these potential biomarkers, the pathway-based network was visualized by Metascape (Fig. 7). Ten of seventeen potential biomarkers were recognized by Metascape and were built a metabolic pathway network. It is noted that the compound–reaction–enzyme–gene network was not fully connected. And we input two key metabolites, arachidonate and pyruvate, and expanded the isolated network components by Metscape expanding function to make the network to be fully connected. We then used CentiScape, a plugin within Cytoscape software, to estimate the node importance according to the centroid and betweenness centrality scores. The node with the centroid and betweenness centrality values that were larger than the network average was considered as the most important node. As shown in Fig. 7, four of ten potential biomarkers, including sphinganine, phosphatidylcholine, lysophosphatidylcholine, and phosphatidylinositol were in the critical position, and they were associated with many cellular metabolic processes. Moreover, the result of pathway analysis showed that the pathway with high impact value influenced by MDR were the sphingolipid and glycerophospholipid metabolism pathways. Sphingolipid metabolism pathway is involved into sphinganine, ceramide, ceramide 1-phosphate, sphingosine 1-phosphate, sphinganine 1-phosphate, dihydroceramide, and other metabolites. And phosphatidylcholine, lysophosphatidylcholine, and phosphatidylinositol are correlated with glycerophospholipid metabolism pathway. And there were discriminable differences in the levels of dihydroceramide, sphinganine, phosphatidylcholine, lysophosphatidylcholine, and phosphatidylinositol between the MCF-7/ADR cells and the control group. These indicated that sphingolipids and glycerophospholipids played critical roles in the MDR. These key nodes in the metabolic network represent potential therapeutic targets that could be utilized for the reversal of MDR for breast cancer.
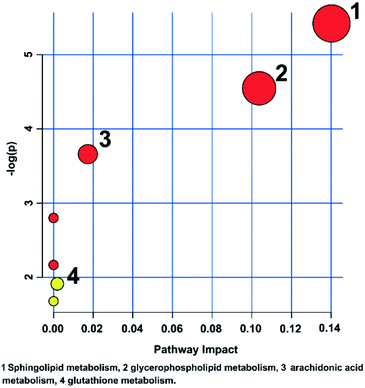 |
| Fig. 6 Metabolic pathway analysis of breast cancer cells affected by the MDR. The color and size of each circle is based on the p level for Student's t-test and pathway impact value, respectively. | |
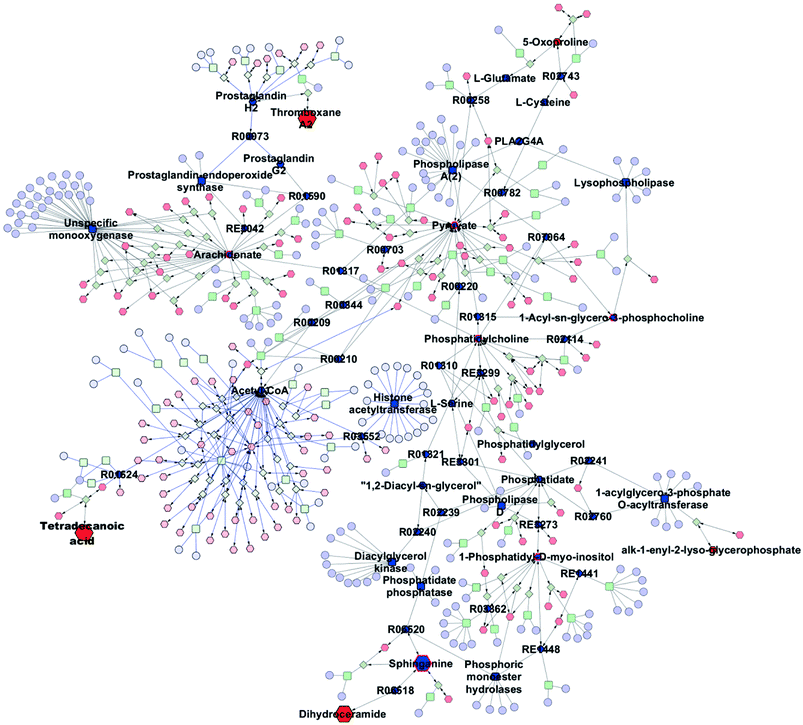 |
| Fig. 7 Network of the identified potential biomarkers associated with MDR. The metabolites, genes, enzymes, and reactions are represented by a hexagon, ellipse, round rectangle, and diamond. The size of the node represents the direction of the change of metabolite in the MCF-7/ADR cells compared with the parental cells. The input compounds are filled with red. Node with centrality values (centroid and betweenness) greater than the threshold value is filled with dark blue. The potential biomarker with centrality values greater than the threshold value is shown in red border. | |
Discussion
Lipids are known to possess diverse biological functions such as energy storage, structural composition of cell membranes, and signal transduction.23 It's demonstrated that the disorder of lipids is related to a variety of diseases including cancer, obesity, diabetes and neurodegenerative diseases.24 So, in this study, we adopt a MTBE-based method to profile intracellular metabolites and lipids in MCF-7/ADR and parental MCF-7 cells by UPLC-Q-TOF-MS. The metabolic pathways related to lipid metabolism involving sphingolipid metabolism, glycerophospholipid metabolism and arachidonic acid metabolism as well as antioxidant pathway were revealed as the most important altered metabolic pathways in terms of the changes of metabolites in these pathways.
Meanwhile, AFM was used to observe the changes of microstructure of cellular membrane surface and the cell size. The cell surface roughness was applied to further understand the changes of the cell membrane surface induced by the MDR. The data indicated that the topographical heterogeneity existed on the membrane surface of cells, due to the higher valves of surface roughness at the edge region than the ones at the centre region. Moreover, the cell membrane architecture of MCF-7/S cell was relatively smooth, less protrusions were on the cell membrane surface than MCF-7/ADR cell, may be attributed to the overexpression of the ATP-binding cassette (ATP) proteins pumping the drugs out of the cells. It has been reported that the protruding particles correspond to membrane proteins or to protein-lipid complexes.25 Therefore, the cellular membrane, affecting the permeability to drugs and protein exposure, could serve a target for reversal of drug resistance.
Sphingolipid metabolism
Sphingolipids are the major lipid constituents of cellular membranes. Sphingolipids contain sphingomyelins and glycosphingolipids (cerebrosides, sulfatides, globosides and gangliosides). The intermediates of sphingolipids metabolism, such as ceramide, dihydroceramide, sphinganine, and sphingosine, have various effects on cellular processes including cell growth, cell invasion, apoptosis and the others, but the effects were different according to the type of fatty acids attached to the sphingosine backbone.26
Dihydroceramide, an intermediate of sphingolipid metabolic pathway, is generated by de novo synthesis of ceramide in the endoplasmic reticulum (ER). It plays roles in autophagy, hypoxia, and cellular proliferation and has been associated with many diseases.27 It can inhibit mitochondrial ceramide channel formation and reduce the permeabilisation of the outer membrane and apoptosis, which is favorable for tumor cells.28,29 So, dihydroceramide is a type of antiapoptotic substance, but the role of dihydroceramide in apoptosis is controversial, which may be associated with the chain length and degree of unsaturation of fatty acid linked to dihydroceramide. Moreover, reactive oxygen species (ROS) generation could trigger dihydroceramide production, as the oxidative stressors hydrogen peroxide, menadione, or tert-butyl-hydroperoxide could increase dihydroceramide levels in various immortalized cell lines.27 Furthermore, increased ROS levels in cancer cells have been found and are related to tumor resistance to chemotherapy by regulating P-gp and other multidrug resistant proteins expression, but the correlation between ROS and drug resistant proteins lacks enough evidence.30
Glycerophospholipids metabolism
It can be seen that one of the most significant alterations resulted from drug resistance is the decrease in PCs contents.
Glycerophospholipids are also the main components of the cell membranes, directly affecting the physiological function of cells. Changes in the concentrations of phospholipids indicate the changes of cell membrane composition and permeability, which will affect the normal physiological function of cells. So the change of phospholipid content well reflects the disorders of lipid metabolism in vivo, and is a very important biological indicator. Lysolipids and fatty acids are the natural products of lipid hydrolysis by phospholipases. Moreover, the intramitochondrial oxidation of fatty acids is an important energy source for growth and proliferation of cells.
The lower contents of phosphatidylcholines (PCs) and lysophosphatidylcholines (LPCs) were found in the MCF-7/ADR cells compared with the MCF-7/S cells. PCs are the major and important components of cell membrane, and lysoPCs and fatty acids are the hydrolysates of glycerophospholipids in the reaction catalyzed by phospholipase A2 (PLA2). Decreased levels of PCs indicated that the metabolism of this membrane phospholipid PCs could be enhanced, and increased activity of PLA2 may be responsible for this phenomenon. Meanwhile, the changes of PC contents could affect the structure and properties of cell membranes. The fluidity of cell membrane is proportional to the cell permeability of the drug. The higher levels of long-chain saturated fatty acids in the biological membrane could decrease the cell membrane fluidity, because those fatty acids are more firmly packed and form stronger van der Walls interactions with each other, resulting in the increased rigidity of the cell membranes.31 In addition, the increase of cholesterol and sphingomyelin (SM) could also cause the decrease of cell membrane fluidity.32 Furthermore, functional alterations may be caused by protein position changes, arising from changes in structural cell membrane induced by modification in cell fluidity, like an alteration in exposure or function of the P-gp, thereby mediating drug resistance.33 So the decrease of PC and the increase of dihydroceramide associated with SM may be responsible for the decreased cell permeability to drug. And the ultrastructure analysis of the membrane surface also demonstrated that the membrane did change along with the MDR.
LysoPCs can be further metabolized by lysophospholipase to fatty acids and choline, suggesting that lysoPCs may act as carriers of fatty acids in addition to being glycerophospholipid metabolism intermediates. The decreased levels of lysoPCs indicated that a higher metabolism rate existed in the drug-resistant MCF-7/ADR cells. Moreover, lysoPCs have cytolytic and membrane perturbing properties,34 and are believed to be rapidly acylated with acetyl-CoA to maintain normal membrane composition,35 so the levels of lysoPCs must be strictly controlled.
Arachidonic acid metabolism
The arachidonic acid metabolism was disturbed according to the drug resistance effect on thromboxane metabolite in the MCF-7/ADR cells. Thromboxane (TX) belonging to the category of prostanoids, which also include prostaglandin (PG), is an important bioactive lipid mediator that is responsible for various physiological and pathological processes including inflammation and cancer. Thromboxane (TX) is synthesized from the prostaglandin H2 (PGH2) by thromboxane synthase (TXAS), and PGH2 is biosynthesized from arachidonic acid via the cyclooxygenase-2 (COX-2). And thromboxane is released to the extracellular space to work in an autocrine or paracrine manner by binding to cell surface receptors. The release process was regulated by multidrug resistance-associated proteins (MRPs).36 Previous research also found that COX-2 could up-regulate the expression of P-glycoprotein in drug-resistant human cancer cells, and the selective COX-2 inhibitors could reverse the drug resistance.37 In addition, overexpression of TXAS has been found in both squamous cell carcinoma and transitional cell carcinoma of the bladder and TXAS inhibition could enhance cells sensitivity to the chemotherapeutic agents.38
Antioxidant pathway (5-oxoproline and alpha-tocotrienol)
5-oxoproline (pyroglutamate) is converted to glutamate by 5-oxoprolinase, which is related with glutathione (GSH) recycling. Although, the levels of GSH were not detected, it could be speculated that the lower 5-oxoproline levels observed in the MCF-7/ADR cells were related to the changes of glutathione concentrations. One of mechanisms involved in adriamycin action is the free oxygen radical generation.39 And GSH plays an important role in removing these free radicals.40 Furthermore, GSH can react with a wide range of reactive electrophiles including antineoplastic drugs such as vincristine (VCR) and adriamycin (ADR) under the catalyzation of glutathione-s-transferase (GST) to form GSH conjugates and these GSH conjugates may be pumped out by the multidrug resistance-associated protein (MRP), whereby causing a lower intracellular level than that necessary for cytotoxicity in the tumor cells.41 Thisis also the most widely investigated MDR mechanism, which drugs are pumped out of the tumor cells by one or more energy-dependent transporters, such as glycoprotein P (P-gp/ABCB1) and MRP. Accordingly, GSH is consider as an important antioxidant and scavenger of toxicant. Therefore, the levels of 5-oxoproline are correlated with anti-oxidative and detoxifying capacity, which affect effectiveness of adriamycin treatment.
Alpha-tocotrienol is a member of the vitamin E family, which comprises α-, β-, γ-, and δ-tocopherols as well as α-, β-, γ-, and δ-tocotrienols. And tocotrienols are currently receiving more attention because of the antioxidative, hypocholesterolemic, and anti-cancer activities. However, in low concentrations α-tocotrienol had little effect on proliferation and apoptosis of human breast cancer cells.42
According to the above-mentioned discussion, adriamycin-resistant cells were more energetic in the anti-oxidation, detoxification and the regulation of proliferation and apoptosis-related signaling than adriamycin-sensitive cells.
Conclusion
In this study, significant metabolic changes were observed between multidrug resistant cells and drug sensitive cells by using an UPLC-Q/TOF-MS-based metabolomic technique, which were mainly involved in some important metabolic pathways, such as glycerophospholipid metabolism, sphingolipid metabolism, arachidonic acid metabolism as well as antioxidant pathways. The adriamycin-resistant cells are better in the anti-oxidation, the regulation of proliferation and apoptosis-related signaling and the detoxification than the adriamycin-sensitive cells. And the results were also interpreted by MTT assay and AFM assay, showing that MCF-7/ADR cells developed MDR and the cellular membrane also changed due to the MDR. Moreover, it has been demonstrated that the alterations in phospholipid composition of cell membrane, especially glycerophospholipids and sphingolipids could change the fluidity and structure of cell membrane, so lipids are vital in the formation of MDR. Targeting the glycerophospholipids and sphingolipids metabolism could be an approach to reverse MDR. Additionally, the metabolic changes observed in the present study may help us to reveal the molecular mechanism of multidrug resistance and serve for early diagnosis.
Conflicts of interest
There are no conflicts to declare.
Acknowledgements
The work was supported by the National Natural Science Foundation of China (No. 81773690, 81373952).
Notes and references
- H. Wang, T. Vo, A. Hajar, S. Li, X. Chen, A. M. Parissenti, D. N. Brindley and Z. Wang, BMC Cancer, 2014, 14, 37–51 CrossRef PubMed.
- R. Misra, M. Das, B. S. Sahoo and S. K. Sahoo, Int. J. Pharm., 2014, 475, 372–384 CrossRef CAS PubMed.
- A. T. Lucas, S. K. O'Neal, C. M. Santos, T. F. White and W. C. Zamboni, J. Pharm. Biomed. Anal., 2016, 119, 122–129 CrossRef CAS PubMed.
- R. Zhang, X. Zhuang, L. Zong, S. Liu, Z. Liu and F. Song, Anal. Bioanal. Chem., 2016, 408, 5843–5854 CrossRef CAS PubMed.
- G. Kibria, H. Hatakeyama and H. Harashima, Arch. Pharmacal Res., 2014, 37, 4–15 CrossRef CAS PubMed.
- L. Galluzzi, I. Vitale, J. Michels, C. Brenner, G. Szabadkai, A. Harel-Bellan, M. Castedo and G. Kroemer, Cell Death Dis., 2014, 5, e1257–e1274 CrossRef CAS PubMed.
- M. Videira, R. L. Reis and M. A. Brito, Biochim. Biophys. Acta, 2014, 1846, 312–325 CAS.
- C. A. Vilanova-Costa, H. K. Porto, L. C. Pereira, B. P. Carvalho, W. B. Dos Santos and P. Silveira-Lacerda Ede, Biol. Trace Elem. Res., 2015, 163, 39–47 CrossRef CAS PubMed.
- S. Karthikeyan and S. L. Hoti, Anti-Cancer Agents Med. Chem., 2015, 15, 605–615 CrossRef CAS PubMed.
- A. Zhang, H. Sun, G. Yan, P. Wang and X. Wang, BioMed Res. Int., 2015, 2015, 354671–354676 Search PubMed.
- S. Wang, X. Chen, H. Luan, D. Gao, S. Lin, Z. Cai, J. Liu, H. Liu and Y. Jiang, Rapid Commun. Mass Spectrom., 2016, 30, 533–542 CrossRef CAS PubMed.
- G. van Meer, EMBO J., 2005, 24, 3159–3165 CrossRef CAS PubMed.
- L. Yang, X. Cui, N. Zhang, M. Li, Y. Bai, X. Han, Y. Shi and H. Liu, Anal. Bioanal. Chem., 2015, 407, 5065–5077 CrossRef CAS PubMed.
- K. A. Ahmed and P. Chinnaiyan, Metabolites, 2014, 4, 740–750 CrossRef PubMed.
- C. M. Rocha, A. S. Barros, B. J. Goodfellow, I. M. Carreira, A. Gomes, V. Sousa, J. Bernardo, L. Carvalho, A. M. Gil and I. F. Duarte, Carcinogenesis, 2015, 36, 68–75 CrossRef CAS PubMed.
- C. D. Hart, L. Tenori, C. Luchinat and A. Di Leo, Adv. Exp. Med. Biol., 2016, 882, 217–234 CrossRef CAS PubMed.
- M. L. Doria, A. S. Ribeiro, J. Wang, C. Z. Cotrim, P. Domingues, C. Williams, M. R. Domingues and L. A. Helguero, FASEB J., 2014, 28, 4247–4264 CrossRef CAS PubMed.
- T. Kuhn, A. Floegel, D. Sookthai, T. Johnson, U. Rolle-Kampczyk, W. Otto, M. von Bergen, H. Boeing and R. Kaaks, BMC Med., 2016, 14, 13–21 CrossRef PubMed.
- V. Matyash, G. Liebisch, T. V. Kurzchalia, A. Shevchenko and D. Schwudke, J. Lipid Res., 2008, 49, 1137–1146 CrossRef CAS PubMed.
- S. Chen, M. Hoene, J. Li, Y. Li, X. Zhao, H. U. Haring, E. D. Schleicher, C. Weigert, G. Xu and R. Lehmann, J. Chromatogr. A, 2013, 1298, 9–16 CrossRef CAS PubMed.
- A. K. Adya, E. Canetta and G. M. Walker, FEMS Yeast Res., 2006, 6, 120–128 CrossRef CAS PubMed.
- C. Z. Ulmer, R. A. Yost, J. Chen, C. E. Mathews and T. J. Garrett, J. Proteomics Bioinf., 2015, 8, 126–132 Search PubMed.
- S. B. Breitkopf, S. J. H. Ricoult, M. Yuan, Y. Xu, D. A. Peake, B. D. Manning and J. M. Asara, Metabolomics, 2017, 13, 30–50 CrossRef PubMed.
- C. Y. Wang, M. Wang and X. L. Han, Mol. BioSyst., 2015, 11, 698–713 RSC.
- H. Bai, H. Jin, F. Yang, H. Zhu and J. Cai, Scanning, 2014, 36, 622–631 CrossRef CAS PubMed.
- T. Sassa, S. Suto, Y. Okayasu and A. Kihara, Biochim. Biophys. Acta, 2012, 1821, 1031–1037 CrossRef CAS PubMed.
- M. M. Siddique, Y. Li, B. Chaurasia, V. A. Kaddai and S. A. Summers, J. Biol. Chem., 2015, 290, 15371–15379 CrossRef CAS PubMed.
- P. Breen, N. Joseph, K. Thompson, J. M. Kraveka, T. I. Gudz, L. Li, M. Rahmaniyan, J. Bielawski, J. S. Pierce, E. Van Buren, G. Bhatti and D. Separovic, Anticancer Res., 2013, 33, 77–84 CAS.
- J. Shi, J. Zhou, H. Ma, H. Guo, Z. Ni, J. Duan, W. Tao and D. Qian, Anal. Bioanal. Chem., 2016, 408, 1413–1424 CrossRef CAS PubMed.
- S. Galadari, A. Rahman, S. Pallichankandy and F. Thayyullathil, Free Radical Biol. Med., 2017, 104, 144–164 CrossRef CAS PubMed.
- A. M. Campos, E. Maciel, A. S. Moreira, B. Sousa, T. Melo, P. Domingues, L. Curado, B. Antunes, M. R. Domingues and F. Santos, J. Cell. Physiol., 2016, 231, 1024–1032 CrossRef CAS PubMed.
- I. N. Todor, N. Y. Lukyanova and V. F. Chekhun, Exp. Oncol., 2012, 34, 97–100 CAS.
- J. Leibovici, O. Klein, Y. Wollman, N. Donin, T. Mahlin and M. Shinitzky, Biochim. Biophys. Acta, 1996, 1281, 182–188 CrossRef.
- X. Gao, M. Guo, Q. Li, L. Peng, H. Liu, L. Zhang, X. Bai, Y. Wang, J. Li and C. Cai, PLoS One, 2014, 9, e100017–100026 Search PubMed.
- K. Klavins, T. Koal, G. Dallmann, J. Marksteiner, G. Kemmler and C. Humpel, Alzheimer's Dementia, 2015, 1, 295–302 Search PubMed.
- A. Furugen, H. Yamaguchi, N. Tanaka, N. Shiida, J. Ogura, M. Kobayashi and K. Iseki, Prostaglandins Other Lipid Mediators, 2013, 106, 37–44 CrossRef CAS PubMed.
- H. B. Xu, F. M. Shen and Q. Z. Lv, Eur. J. Pharmacol., 2016, 776, 1–8 CrossRef CAS PubMed.
- O. Moussa, J. M. Riker, J. Klein, M. Fraig, P. V. Halushka and D. K. Watson, Oncogene, 2008, 27, 55–62 CrossRef CAS PubMed.
- B. Cao, M. Li, W. Zha, Q. Zhao, R. Gu, L. Liu, J. Shi, J. Zhou, F. Zhou, X. Wu, Z. Wu, G. Wang and J. Aa, Metabolomics, 2013, 9, 960–973 CrossRef CAS PubMed.
- Y. Liu, T. Chen, M. H. Li, H. D. Xu, A. Q. Jia, J. F. Zhang and J. S. Wang, Chemosphere, 2015, 138, 537–545 CrossRef CAS PubMed.
- G. Rocha Gda, R. R. Oliveira, M. A. Kaplan and C. R. Gattass, Eur. J. Pharmacol., 2014, 741, 140–149 CrossRef PubMed.
- R. Loganathan, K. R. Selvaduray, K. Nesaretnam and A. K. Radhakrishnan, Cell Proliferation, 2013, 46, 203–213 CrossRef CAS PubMed.
Footnote |
† Electronic supplementary information (ESI) available. See DOI: 10.1039/c7ra12952a |
|
This journal is © The Royal Society of Chemistry 2018 |
Click here to see how this site uses Cookies. View our privacy policy here.