DOI:
10.1039/C7RA12435J
(Paper)
RSC Adv., 2018,
8, 10665-10672
Heavy metal accumulation and its spatial distribution in agricultural soils: evidence from Hunan province, China
Received
14th November 2017
, Accepted 28th January 2018
First published on 16th March 2018
Abstract
The issue of heavy metal pollution in Hunan province, China, has attracted substantial attention. Current studies of heavy metal soil pollution in Hunan province mainly focus on medium and small scales, thus heavy metal pollution is rarely considered at the province scale in Hunan. In order to investigate the heavy metal pollution status in agricultural soils in Hunan province, literature related to heavy metal soil pollution in Hunan province was reviewed and organized from the following databases: Web of Science, China national knowledge infrastructure (CNKI), Wanfang Data, and China Science and Technology Journal Database (CQVIP). The literature data for the contents of Pb (122 soil sampling sites), Zn (103 sites), Cu (102 sites), Cd (105 sites), As (100 sites), Hg (85 sites), Cr (95 sites), and Ni (62 sites) in agricultural soils were obtained at the province scale. The spatial auto-correlation method was applied to reveal the spatial distribution of heavy metal accumulation. The average contents of the 8 heavy metals in agricultural soils of Hunan were all significantly (P < 0.05) higher than their background values and they were not distributed evenly across the Hunan province; the content of each heavy metal in eastern Hunan (including the cities of Yueyang, Changsha, Zhuzhou, and Chenzhou) was higher than that of other regions. The exceeding standard rate (the ratio of surveyed content to the background value) for Cu, Cd, As, and Hg had strongly positive spatial correlation, whereas Zn and Ni presented a negative spatial correlation. Overall, the higher exceeding standard rates of the 8 heavy metals were mainly distributed in the highly industrialized cities such as Changsha, Zhuzhou, Xiangtan, Chenzhou, and Hengyang, thus more attention should be paid to such areas to manage soil pollution.
1 Introduction
Soil is an important component of ecosystems, and is also the main location for biochemical reactions of environmental pollutants. Heavy metals in soil influence plant growth, and when accumulation of heavy metals exceeds the standard, soil contamination occurs that negatively affects the sustainable development of the ecological environment and social economy. In addition, heavy metals in soil can migrate and transfer into crops, water sources, and the atmosphere through multiple pathways and finally accumulate in human bodies through the food chain and thus severely threaten human health.1,2 In recent years, the contamination of heavy metals in soil has worsened under intensified industrial development and urbanization. According to the surveys of the Ministry of Land and Resources, Ministry of Environmental Protection, and Ministry of Agriculture, the total proportion of land exceeding the Chinese soil heavy metal standard3 of soil contamination all around the country reached 16.1%. Moreover, 64.8% of the 1.4 million hm2 of sewage-irrigated agricultural land suffers from heavy metal contamination and every year as much as 12 million t of grain is contaminated by heavy metals in China.4,5 Given the severe situation of soil heavy metal contamination, domestic and overseas scholars have introduced advanced mathematical statistics and spatial analysis methods to investigate the current status and sources of soil heavy metal contamination in different regions of China6–8 and under various land-use types9–11 as well as the forms, migration, and transformations of heavy metals.12,13 Overall, research into heavy metal contamination in soils has been continuously intensified and the research results are tending to be enriched, which have provided important grounds for the prevention and treatment of heavy metal contamination in China.
Hunan is characterized by rich nonferrous metal and nonmetallic mineral, and it is also the key area of soil heavy metal contamination in China. Scholars have carried out a great amount of studies on the soil heavy metals in various areas of Hunan province,14–18 for example, Ding et al.19 Yang et al.20 and Li et al.21 revealed the heavy metal accumulation status in Hunan soil based on the Changsha–Zhuzhou–Xiangtan area, and the cities of Changde, and Zhuzhou, respectively. However, current studies regarding heavy metals in Hunan province have mainly focused on typical areas at small and medium scales (e.g., municipal administrative units, sub-watersheds of basins, and experimental plots). Consequently, the status of heavy metal contamination in soils of entire Hunan province cannot therefore be revealed.5,6
Food is the basis of human survival and, as the medium for crop growth, soil is the basis of food. Therefore, heavy metal accumulation in agricultural land has the potential to enter human bodies through human intake of agricultural products. For instance, a sample survey of rice at market and in the mining areas of entire Hunan province showed that among the 100 rice samples collected from the entire Hunan province, only 15 samples met the requirement of national food hygiene standard for Cd, Pb, and As, while 86% of the rice in eastern Hunan was not suitable for human consumption.22 On account of the studies of heavy metal contamination status in Hunan province and the urgency of clearing the threat of heavy metal contamination in agricultural land to human health, this study organized the relevant literature focused on heavy metals in agricultural land of Hunan province in the Web of Science, CNKI, Wanfang Data, and CQVIP databases, and obtained the contents of 8 heavy metals in soil (Pb, Zn, Cu, Cd, As, Hg, Cr, and Ni) in agricultural land of Hunan province by applying statistical analysis at provincial scale. The spatial autocorrelation methods were employed to obtain the spatial distribution of heavy metals in agricultural land of Hunan province, which can provide a certain reference for integrated treatment of soil heavy metal contamination in Hunan.
2 Materials and methods
2.1 Study area
Hunan province is located in central China and in the middle reaches of Yangtze River with the geological location of 108°47′–114°15′E and 30°08′–24°38′N. Hunan is next to Jiangxi province in the east, adjacent to Chongqing and Guizhou province in the west, adjoining Guangdong and Guangxi province in the south, and connecting with Hubei province in the north. The land area of Hunan is 211
800 km2 and the total population is 67.372 million. Hunan province governs 14 prefectures and 122 counties (cities or districts). Mountain (including highland), hills, hillock farmland, plain, and waters account for 51.22%, 15.40%, 13.87%, 13.11%, and 6.39% of the total land area in the province, respectively. The province has a continental central Asian subtropical monsoon humid climate, which has an annual sunshine duration of 1300–1800 h, annual mean temperature of 15–18 °C, frost-free period of 260–310 days, and annual mean precipitation of 1200–1700 mm. Hunan is an agricultural province, which is famously known as the “granary of nine provinces” and “land of plenty”, in addition, it has rich nonferrous metal and nonmetallic minerals. In Hunan, 143 kinds of mineral products have been detected and the resource reserve of many minerals ranks in the top 5–10 in China. Moreover, the industrial areas of Hunan covers an extensive amount of land and there were many development areas at provincial and even national levels. These areas are famous for their mechanical engineering, electronic information, and new materials, hard alloy of Pb and Zn and further processing. There are four 100-billion level industrial parks including the Changsha economic developmental district, Changsha high-tech industrial zone, Zhuzhou high-tech industrial zone, and Xiangtan economic development zone, and 22 national-level new industrialization industry demonstration bases. These industrial and mining enterprises generate tremendous amounts of solid waste and discharge a large amount of waste gas and waste water, resulting in heavy metal accumulation in soil. Heavy metal accumulation in agricultural soils will do harm to human health through the food chain, for example, the cadmium-rice incident resulting from the heavy metal contamination in agricultural land has drawn much attention.22
2.2 Data collection and processing
The data in this study were mainly derived from the literature databases (Web of Science, CNKI, Wanfang Data, and CQVIP), and partial data were collected from the China Soil Database (http://www.soil.csdb.cn/) and national soil survey results. The heavy metal contents, the latitude and longitude data of study areas in relevant literature regarding the soil heavy metal (topsoil sample 0–20 cm, all mixed samples) in Hunan agricultural land during 2007–2016 were extracted and recorded from the literature databases mentioned above. The original layout of sampling sites of all the data from the literature were uniformly laid out based on the difference of land use status, soil type, and town regions. After removing the heavy metal outliers, Pb, Zn, Cu, Cd, As, Hg, Cr, and Ni samples were obtained from 122, 103, 102, 105, 100, 85, 95, and 62 sites, respectively (Fig. 1).
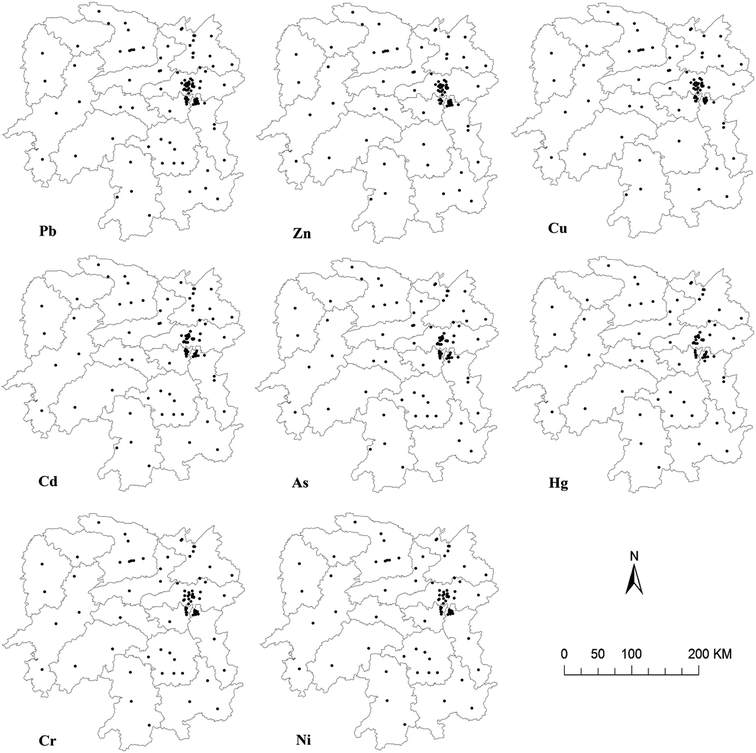 |
| Fig. 1 Distribution of sampling sites of soil Pb, Zn, Cu, Cd, As, Hg, Cr, and Ni in Hunan province. | |
2.3 Spatial autocorrelation method
Spatial autocorrelation analysis is one of the major methods used in geographic studies. The first law of geography states that the spatial autocorrelation among objects exists objectively.23 Global spatial autocorrelation and local spatial autocorrelation index can be used to measure the spatial autocorrelation characteristics among objects. Correspondingly, global spatial autocorrelation could comprehensively reflect the spatial autocorrelation of the entire region and local spatial autocorrelation is used to further reflect the level of correlation of attribute eigenvalues between each geographic unit and the adjacent spatial unit. The global Moran's I index and local Moran's I index24 were adopted to conduct spatial autocorrelation analysis on the exceeding standard rate (ESR, the ratio of heavy metal content to background value) in Geodata. The range of Moran's I index is [−1, 1], and Moran's I > 0 denotes a positive spatial correlation; the greater the index and closer to 1, the stronger the spatial correlation. Moran's I < 0 denotes a negative spatial correlation; the smaller the value and closer to −1, the greater the spatial difference. Otherwise, Moran's I = 0 denotes the spatial randomness.25,26 The significance of spatial distribution is denoted with Z(I), and Z(I) > 1.96 indicates that the distribution of a certain phenomenon within the study area has a significant correlation.27 The calculation formula for global Moran's I index is: |
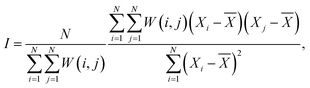 | (1) |
and the calculation formula of local Moran's I index is: |
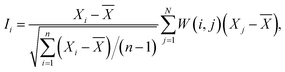 | (2) |
where N denotes the amount of study objectives, Xi denotes the observed value,
denotes the mean value of Xi, and W(i, j) denotes the spatial connection matrix between objects i and j.
The spatial weight is the prerequisite and foundation of conducting spatial autocorrelation analysis.28 The adjacency relation can be classified into three types of adjacency criteria: Rook, Queen, and Bishop and the adjacency criterion used in this study was Rook.
The local spatial autocorrelation can be represented by the Local Indicators of Spatial Association (LISA) map. The High–High (HH) type of spatial cluster and Low–Low (LL) type of spatial cluster indicate higher and lower spatial cluster characteristics, respectively. Whereas the High–Low (HL) and Low–High (LH) type spatial variation indicate a negative spatial correlation (a region with discrete characteristics).24
2.4 Statistical methods
The maximum value, minimum value, mean, standard error, skewness–kurtosis, and coefficient of variation (CV) were calculated in SPSS 20.0 (IBM, Chicago, USA) and Microsoft Excel 2007 (Microsoft, Washington, USA). The spatial distribution of the 8 soil heavy metals in Hunan agricultural land soils was processed in ArcGIS 10.2 (Esri, California, USA) to generate the diagram map. Moreover, the spatial autocorrelation of soil heavy metals was operated in GEODA™ 0.9.5 (Luc Anselin, Urbana, USA).
3 Results
3.1 The statistical analysis of heavy metal contents in soil of Hunan province
The statistical analysis of heavy metal contents in soil of Hunan province was shown in Table 1. The Pb, Zn, Cu, Cd, As, Hg, Cr, and Ni contents were within the ranges 15.20–233.56, 43.28–403.08, 13.85–111.60, 0.06–8.87, 4.00–77.42, 0.04–1.04, 6.72–169.47, and 3.49–66.59 mg kg−1, respectively, and their mean values followed the trend: Zn (147.28 mg kg−1) > Cr (74.96 mg kg−1) > Pb (56.06 mg kg−1) > Cu (38.85 mg kg−1) > Ni (26.83 mg kg−1) > As (21.05 mg kg−1) > Cd (0.85 mg kg−1) > Hg (0.25 mg kg−1). Compared with the background values of Hunan soil,3 the average values of Pb, Zn, Cu, Cd, As, Hg, and Cr were 2.05, 1.66, 1.53, 10.76, 1.55, 1.75, and 1.16 times higher than the average background values, while the Ni content was lower than the background value. Moreover, the maximum values of Pb, Zn, Cu, Cd, As, Hg, Cr, and Ni were 8.56, 4.55, 4.39, 112.28, 5.69, 7.17, 2.61, and 2.26 times higher than the soil background values. Zn had the greatest standard errors followed by Pb and Cr and those of Cd and Hg were the smallest, indicating that, among the 8 studied soil heavy metals in Hunan, the variation of Zn content was the greatest, while those of Cd and Hg were the smallest.
Table 1 Descriptive statistical analysis of contents of 8 heavy metal in soil in Hunan province
Items |
Sample size |
Minimum (mg kg−1) |
Maximum (mg kg−1) |
Mean (mg kg−1) |
Standard error |
Skewness |
Kurtosis |
Coefficient of variation (%) |
Background values for Hunan (mg kg−1) |
Pb |
122 |
15.20 |
233.56 |
56.06 |
32.07 |
2.08 |
8.25 |
57.21 |
27.300 |
Zn |
103 |
43.28 |
403.08 |
147.28 |
76.56 |
1.23 |
1.53 |
51.98 |
88.600 |
Cu |
102 |
13.85 |
111.60 |
38.85 |
17.22 |
1.30 |
2.92 |
44.32 |
25.400 |
Cd |
105 |
0.06 |
8.87 |
0.85 |
1.25 |
4.22 |
21.98 |
147.06 |
0.079 |
As |
100 |
4.00 |
77.42 |
21.05 |
10.82 |
2.43 |
9.20 |
51.40 |
13.600 |
Hg |
85 |
0.04 |
1.04 |
0.25 |
0.19 |
1.95 |
4.44 |
76.00 |
0.145 |
Cr |
95 |
6.72 |
169.47 |
74.96 |
31.55 |
0.03 |
0.57 |
42.09 |
64.900 |
Ni |
62 |
3.49 |
66.59 |
26.83 |
11.45 |
1.63 |
3.99 |
42.68 |
29.400 |
The CV is an indicator reflecting sample data fluctuation characteristics, which can indicate the degree to which samples are influenced by human activities.29 Table 1 shows that the coefficient of variation (CV) of the 8 heavy metals were consistently greater than 0.3, indicating strong variation. The statistical results also showed that skewness and kurtosis of the 8 heavy metal contents were all positive and large, among which the skewness decreased in the order Cd > As > Pb > Hg > Ni > Cu > Zn > Cr, while Kurtosis decreased in the order Cd > As > Pb > Hg > Ni > Cu > Zn > Cr.
3.2 Distribution of heavy metals
Fig. 2 showed the distribution of 8 heavy metals. The high Pb contents occurred in southeastern Hunan, including the Zhuzhou, Chenzhou, as well as the Tujia–Miao autonomous prefecture of Xiangxi, and the high Pb contents were also found in Xiangtan, Yongzhou, Yiyang, Changsha, and Huaihua. There were also several areas with high Zn content, such as the Hengyang in the central and southern part, Loudi in the central part, Yongzhou in the southern part, Huaihua and the Tujia–Miao autonomous prefecture of Xiangxi in the western part of Hunan. The zones with low Zn content were located in Zhangjiajie and Shaoyang. Areas with high Cu content were found in Changsha in the east of Hunan province, such as Zhuzhou, Xiangtan, and Chenzhou; however, the Cu contents in Huaihua, the Tujia–Miao autonomous prefecture of Xiangxi, and Loudi were lower. Zones with high Cd content were concentrated in Xiangtan, Yueyang, and Zhuzhou in the eastern part of Hunan province; moreover, the Cd content in Changsha, Hengyang, and Chenzhou were also high. Yueyang, Yiyang, and Hengyang were found to have higher As contents. In addition, the distribution of Hg was similar to that of Zn, higher content were located in Yongzhou in southern Hunan, Shaoyang, Changsha, and Hengyang. The distribution of high Cr contents was in Zhuzhou, Changsha, Yueyang, and Yiyang in the central and northeast of Hunan, and the high Cr contents were also found in Hengyang, Chenzhou, and Loudi. The distribution of Ni was similar to that of Cd, with zones of high Cd content located in northwestern Yueyang, Xiangtan, and the southern part of Hunan province. Several areas of low Ni content were found in Huaihua, Changde, Loudi, and Changsha.
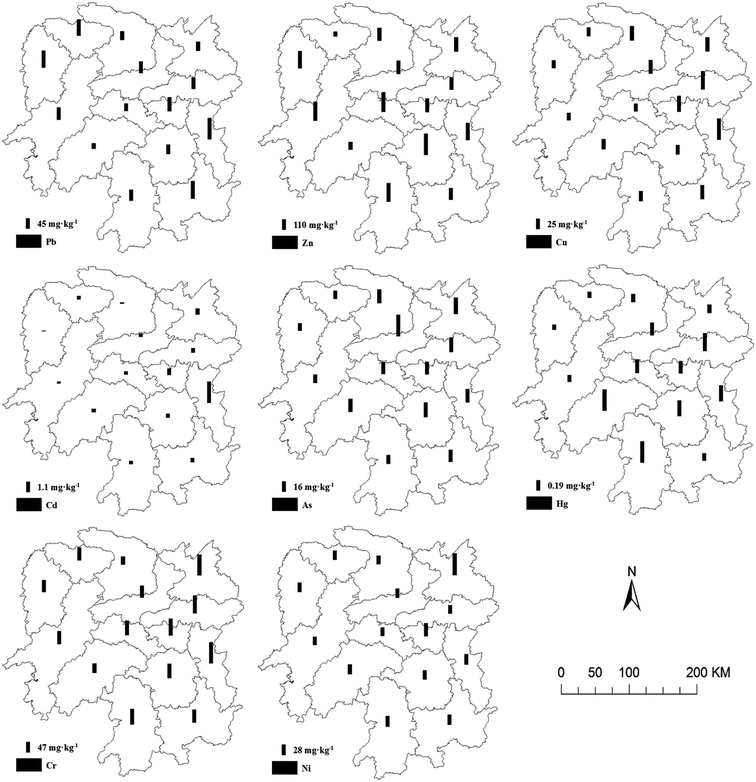 |
| Fig. 2 Contents of 8 heavy metal in soil, Hunan province. | |
Overall, the heavy metal contents were higher in eastern parts of Hunan province including Yueyang, Changsha, Zhuzhou, and Chenzhou, while the heavy metals contents in Yongzhou, Hengyang, and Changde were lower. The distributions of Pb and Zn contents were similar and those of Cu and Cr were also similar.
3.3 Spatial autocorrelation analysis of soil heavy metals ESR
The global Moran's I indexes of each heavy metal's ESR decreased in the order Cu (0.3649) > As (0.3138) > Hg (0.3071) > Pb (0.2020) > Cr (0.2139) > Cd (0.0986) > Ni (−0.1013) > Zn (−0.2913) (Table 2). This indicates that the ESR of Cu, Cd, As, Hg, Pb, and Cr had a positive spatial auto-correlation and ESR of Zn and Ni had a negative spatial auto-correlation. At the significance level of 5%, Z(I) (an index to test whether or not the subject has significant spatial auto-correlation, if Z(I) >1.96, it is significant and vice versa) of Cu, Cd, As, and Hg were greater than 1.96 indicating that Cu, Cd, As, and Hg had a significant positive spatial auto-correlation.
Table 2 The global autocorrelation analysis of the ESR of the 8 soil heavy metals in Hunan province
Items |
Pb |
Zn |
Cu |
Cd |
As |
Hg |
Cr |
Ni |
Moran's I |
0.2020 |
−0.2913 |
0.3649 |
0.0986 |
0.3138 |
0.3071 |
0.2139 |
−0.1013 |
Z(I) |
1.6938 |
−1.3151 |
2.6341 |
2.0204 |
2.5082 |
2.1811 |
1.8202 |
−0.2296 |
The LISA map of ESR of each heavy metal in Hunan province is shown in Fig. 3. Fig. 3 showed that, in Changsha, the HH cluster zones of ESR of heavy metal Cu, As, and Cr appeared simultaneously. In Xiangtan, the HH cluster zones of ESR of heavy metal Cd and Cr appeared simultaneously. The HH cluster zones of ESR of Cu, As, and Hg occurred in Zhuzhou, Yueyang, and Hengyang, respectively. The LL spatial clustering zones of ESR of Cu, Cd, Cr, and Ni appeared simultaneously in Huaihua. In addition, in Tujia–Miao autonomous prefecture of Xiangxi, the LL cluster zones of ESR of Cu, As, and Hg also occurred. The LL cluster zones of ESR of Cu and Hg were found in Shaoyang, Zhangjiajie, Changde. There were relatively fewer isolated zones of HL and LH of ESR. The ESR of Cd had HL isolated zone in Zhuzhou. The LH isolated zone occurred with the presence of HH cluster zone, for instance, the ESR of Hg in Chenzhou had an LH isolated zone, whereas in the adjacent Hengyang a HH spatial cluster zone was found. The ESR of Cd in Changsha had an LH isolated zone, whereas in the adjacent Xiangtan a HH spatial cluster zone was found. The ESR of Zn had LH isolated zones in Shaoyang and Chenzhou, whereas that of Ni had an LH isolated zone in Changsha. The heavy metal Pb did not show any obvious spatial clustering or isolation phenomena.
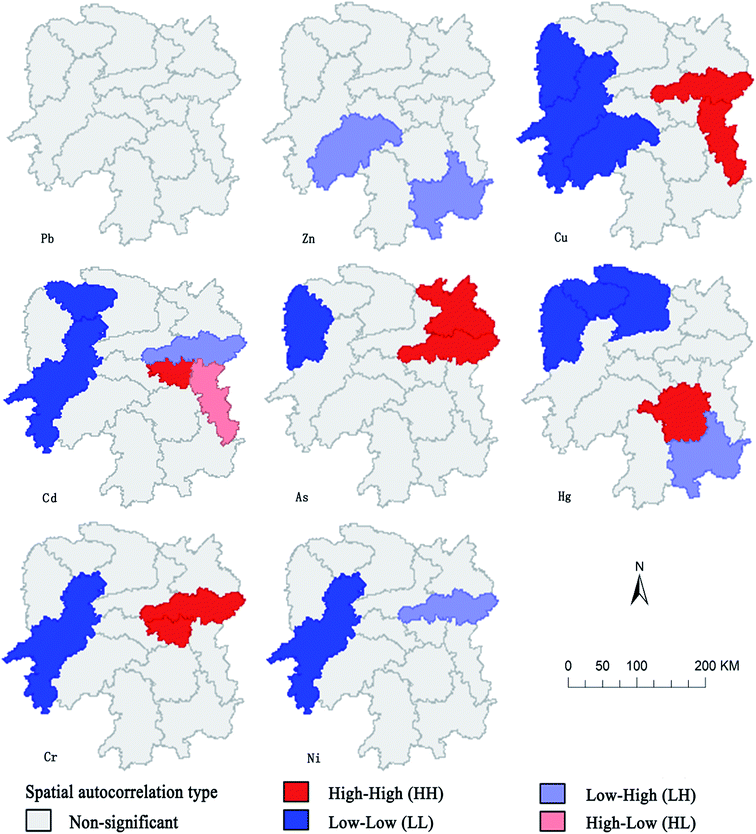 |
| Fig. 3 LISA map of ESR of the 8 soil heavy metals in Hunan province. | |
Overall, the HH and LH spatial cluster zones of the ESR for each heavy metal were mainly distributed in the highly industrialized Changsha, Zhuzhou, Xiangtan, Chenzhou, and Hengyang.
4 Discussion
In this paper, the heavy metal contents in agricultural land of Hunan province was analyzed based on data from the literature, and the results showed clear spatial differentiation characteristics of soil heavy metal contents in Hunan. The Pb, Zn, Cu, Cd, As, Hg, and Cr contents were all higher than the background values, indicating that each heavy metal has been disturbed by human activities to varying degrees. The differences in economic development modes and industry types in different cities in Hunan province caused variations in the spatial accumulation of heavy metals in soil, and hence led to differences in their spatial distribution.
The increase of soil heavy metal contents was closely related with the human activities such as heavy industries, mining and exploitation. Hunan is characterized by rich nonferrous metal and nonmetallic mineral where development of high-tech industry areas is continuous. The developed industry resulted in soil heavy metal pollution. Thus, we clarified the distribution of 8 heavy metal across Hunan province and the global/local spatial autocorrelation were employed to reveal the distribution of ESR of soil heavy metals. The results showed that global spatial autocorrelation can well determine whether there are spatial cluster zones and isolated zones in the spatial distribution of ESR of soil heavy metals. Moreover, the local spatial autocorrelation indicators can well reveal the spatial distribution pattern of ESR of soil heavy metals and we can obtain the specific locations of spatial clustering and isolated zones of heavy metal pollution.30 For example, Cd and Hg contamination in Xiangtan and Changsha was prominent while Xiangtan and Changsha include the national level high-tech development zone which is an industrial region, hence, the pollution can be resulted from industrial wastes and sewage irrigation.31,32 In this study, the highly clustered zone of Cd was located in the eastern part of Hunan province, therefore, industrial pollution was an important source of Cd contamination in soil. In addition, the contents of Pb, Zn, and Cu in agricultural soils of Zhuzhou, Chenzhou, and Yongzhou were higher than other areas, and studies have shown that the heavy metal Pb, Zn, and Cu contamination in farm land around mining areas is severe. The correlation analysis indicated that most heavy metal sources were relatively closer, which might be from anthropogenic pollution such as smelting,33,34 which was closely related with the smelting plant in Zhuzhou and Pb–Zn mines in Chenzhou and Yongzhou. The content of As in agricultural soils of Changde was high, and studies had shown that it would be related to the exploitation of the realgar in this area.35–37 The highly clustered zone of Ni was located in Yueyang, which would be affected by local agricultural production such as fertilizers, pesticides and sewage irrigation.38,39
Therefore, for the difference in pollutant sources in different areas, different measures should be taken and we should comprehensively prevent and treat heavy metal contamination in soil by adjusting measures to local conditions. Firstly, in the heavy-metal-contaminated regions (i.e. industrial agglomeration areas such as Changsha, Xiangtan, and Zhuzhou) the level of supervision of machine, electronics, and material factories that generate pollution needs to be increased, so that treated waste water can be discharged into the environment only after meeting corresponding standards. In the meantime, the focus should be on the introduction of high-tech industries that reduces the level of heavy metal contaminant release or prevents it altogether. Secondly, different pollution control areas can be classified based on the correlation strength of different heavy metals, combined with the characteristics and soil contamination degrees of different heavy metals, and adopt physical, chemical, and biological restoration measures to reduce contamination, such as the soil replacement method, applying ameliorants, and using hyper-accumulation plants for phytoremediation. Such approaches would realize effective, precise, and targeted comprehensive partitioning control of the areas affected by soil heavy metal contamination.
Notably, data extracted from published papers (from Web of Science, CNKI, Wanfang Data, and CQVIP) was applied in the study. Data collected from the eastern of Hunan were more dense than that from the west, due to more studies and sample sites were found in the eastern. This to some extent results in the deviation in estimating the status of soils in Hunan, thus a comprehensive soil survey across Hunan province was expected to reveal the distribution of heavy metals at provincial scale.
5 Conclusion
(1) The Pb, Zn, Cu, Cd, As, Hg, and Cr contents in topsoil of agricultural land in Hunan province were all considerably higher than the background values, and different metals presented different degrees of accumulation. Comparison with the background values indicated that the accumulation of Cd was the most severe, followed by Pb, Zn, As, and Hg.
(2) The distribution characteristics for each heavy metal showed that a clear spatial distribution pattern of soil heavy metal contents in each region of Hunan province. In the eastern part of Hunan (including Yueyang, Changsha, Zhuzhou, and Chenzhou), the heavy metal contents were all relatively high, whereas those in other regions were relatively low. The highest Pb contents were found in Changsha, Zhuzhou, Chenzhou, and Yongzhou, whereas high Zn contents occurred in Hengyang, Loudi, and Yongzhou. Clear accumulation of Cu, Cd, Hg, and Cr was found in Zhuzhou. The accumulation of As was also large in Changde, Yueyang, and Yiyang, and the Ni content was highest in Yueyang and Yiyang.
(3) The ESR of Cu, Cd, As, and Hg contents in each city of Hunan province showed a clear positive spatial correlation, where as those of Zn and Ni contents presented negative spatial correlation. The HH and LH cluster zones of each heavy metal's ESR were mainly distributed in highly industrialized cities such as Changsha, Zhuzhou, Xiangtan, Chenzhou, and Hengyang.
Conflicts of interest
There are no conflicts to declare.
Acknowledgements
This study was supported by the Foundation for Public Welfare of the Ministry of Land and Resources of China (No. 201511082). The suggestions from Hengfei Song during the preparation of the manuscript are also highly appreciated.
References
- H. Zhao and X. Li, Environ. Pollut., 2013, 174(5), 297–304 CrossRef CAS PubMed.
- L. Wang, X. Lu, C. Ren, X. Li and C. Chen, Environ. Earth Sci., 2014, 71(5), 2095–2104 CrossRef CAS.
- Environmental Monitoring Of China, in the Soil Baseline of China, 1990.
- P. Zhu, Z. Zhao, Y. Chen, Q. L. I. M. Yang, P. Zhang and S. Liu, Chin. J. Environ. Eng., 2017, 11(4), 2535–2541 Search PubMed.
- X. Zhang, X. Zhang, T. Zhong and H. Jiang, Environ. Sci., 2014, 35(2), 692–703 Search PubMed.
- Y. Gu and F. Gao, Environ. Chem., 2017, 36(1), 62–71 CAS.
- X. Liang, J. Liu, S. Shi and Z. Jiang, Clean: Soil, Air, Water, 2014, 42(1), 81–87 CrossRef.
- X. Wang, X. Zeng, C. Liu, X. Xu and Y. Lv, Environ. Monit. Assess., 2016, 188(8), 1–12 CrossRef CAS PubMed.
- D. Liu, Y. Li, J. Ma, C. Li and X. Chen, Water, Air, Soil Pollut., 2016, 227(5), 1–10 CAS.
- B. Peng, Z. Song, X. Tu and H. Lv, Environ. Geol., 2004, 45(8), 1137–1147 CrossRef CAS.
- G. Kandpal, P. Srivastava and B. Ram, Water Air & Soil Pollution, 2005, 161(1–4), 353–363 CAS.
- X. Sun, P. Ning, X. Tang and K. Li, Procedia Environ. Sci., 2012, 16, 758–763 CrossRef CAS.
- N. Jarvis, Eur. J. Soil Sci., 2010, 58(3), 523–546 CrossRef.
- T. Nagata, H. Shimura and T. Terashima, J. Food Hyg. Soc. Jpn., 2010, 11(1), 41–45 CrossRef.
- M. Lei, B. Tie, Z. Song, B. Liao, E. Joe and Y. Huang, Food Secur., 2015, 7(1), 45–54 CrossRef.
- L. Wang, Z. Guo, X. Xiao, B. Tong, X. Liao, J. Song and B. Wu, J. Geogr. Sci., 2008, 18(3), 353–362 CrossRef.
- H. Dai, X. Song, B. Huang and J. Xin, Hum. Ecol. Risk Assess., 2016, 22(8), 1636–1650 CrossRef CAS.
- H. Kim, K. Kim, H. Kim and K. Kim, Environ. Earth Sci., 2015, 74(2), 1249–1259 CrossRef CAS.
- C. Ding, Z. Chen, H. Li, X. Peng, H. Feng, Y. Zhang, G. Lei and S. Zhao, Ecol. and Environ. Sci., 2012, 21(12), 2002–2006 Search PubMed , in Chinese.
- J. Yang, W. Li, S. Zhou, S. Luo and C. Liu, Chin. J. Soil Sci., 2015, 46(2), 489–493 CAS , in Chinese.
- X. Li and X. Chen, J. Guilin Univ. Technol., 2016, 36(3), 545–549 Search PubMed , in Chinese.
- P. Williams, M. Lei, G. Sun, Q. Huang, Y. Lu, C. Deacon, A. Meharg and Y. Zhu, Environ. Sci. Technol., 2009, 43(3), 637–642 CrossRef CAS PubMed.
- Y. Chen, Geographical Research, 2009, 28(6), 1449–1463 Search PubMed , in Chinese.
- W. Li, C. Zhu, H. Wang and B. Xu, Trans. Chin. Soc. Agric. Eng., 2016, 32(23), 239–245 Search PubMed , in Chinese.
- S. Zhang and K. Zhang, Acta Sci. Nat. Univ. Sunyatseni, 2007, 46(4), 93–97 Search PubMed , in Chinese.
- S. Zhang and K. Zhang, Geodesy and Geodynamics, 2007, 27(3), 31–34 Search PubMed , in Chinese.
- Y. Yuan, X. Shi, S. Niu, C. Zhang and S. Yan, Econ. Geogr., 2015, 35(7), 148–155 Search PubMed , in Chinese.
- S. Wei, C. Xiong, Q. Luan and Y. Hu, Trans. Chin. Soc. Agric. Eng., 2014, 30(18), 249–256 Search PubMed , in Chinese.
- B. Dai, J. Lv, J. Zhan, Z. Zhang, Y. Liu and R. Zhou, Environ. Sci., 2015, 36(2), 507–515 Search PubMed , in Chinese.
- Q. Yang, J. Yang, S. Yu, B. Huang and W. Sun, Chin. J. Appl. Environ. Biol., 2011, 17(3), 393–397 CrossRef CAS , in Chinese.
- X. Wang, M. He, J. Xie and X. Lu, J. Soils Sediments, 2010, 10(5), 827–837 CrossRef CAS.
- M. Lei, B. Tie, Z. Song and Y. Huang, Food Secur., 2015, 7(1), 45–54 CrossRef.
- L. Wang, Z. Guo, X. Xiao and B. Wu, J. Geogr. Sci., 2008, 18(3), 353–362 CrossRef.
- X. Zhao, J. Liu, X. Xia and Z. Jiang, Environ. Sci. Pollut. Res. Int., 2014, 21(7), 5076–5085 CrossRef CAS PubMed.
- M. Ceng, B. Liao, Q. Zeng, Y. Zhang, Q. Luo and B. Ouyang, J. Agro-Environ. Sci., 2006, 25(2), 418–421 Search PubMed , in Chinese.
- W. She, Y. Jie, H. Xing, Y. Lu, W. Kang and D. Wang, Acta Ecol. Sin., 2011, 31(3), 874–881 CAS , in Chinese.
- M. Lei, M. Zeng, Y. Zheng, B. Liao and Y. Zhu, Acta Sci. Circumstantiae, 2008, 28(6), 1212–1220 CAS , in Chinese.
- Q. Zhu, D. Huang, R. Fan, C. He and S. Liu, J. Agro-Environ. Sci., 2007, 26(s1), 22–26 Search PubMed , in Chinese.
- L. Yi and Q. Zeng, Chin. J. Soil Sci., 2015, 46(6), 1509–1513 CAS , in Chinese.
|
This journal is © The Royal Society of Chemistry 2018 |
Click here to see how this site uses Cookies. View our privacy policy here.