DOI:
10.1039/C8NH00043C
(Communication)
Nanoscale Horiz., 2018,
3, 505-516
Realizing the nanoscale quantitative thermal mapping of scanning thermal microscopy by resilient tip–surface contact resistance models
Received
19th February 2018
, Accepted 24th April 2018
First published on 24th April 2018
Abstract
Quantitative assessment of thermal properties by scanning thermal microscopy (SThM) is a demanded technology, but still not yet available due to the presence of unpredictable thermal contact resistance (TCR) at the tip/substrate interface. The TCR is mainly affected by three major interfacial characteristics including surface roughness, hardness and contacting force. In this work, the TCR is mathematically derived into linear and non-linear models based on the interfacial micro-characteristics. The models have the capability to predict the TCR for both rough and smooth surfaces with satisfactory accuracy. With a predictable TCR, the heat transport across the tip/substrate nanointerface can be precisely described and thus quantitative thermal properties can be predicted from SThM measurements. The models are tested in three polymeric material systems, PDMS, epoxy and PVA. The thermal conductivity from the model prediction matches very well (<10% error) with the measured values from bulk polymer samples. Such models use general surface features as inputs, so they have wide applicability to other similar materials, especially polymers. Moreover, the models have been shown to be valid in doped PVA samples when extrapolated to predict thermal conductivity beyond the range of model development. This work extends the capability of SThM in quantitative measurement and enables a unique platform for thermal conductivity measurement at nanometer spatial resolution.
Conceptual insights
The existing scanning thermal microscopy (SThM) has the unique capability to probe the qualitative thermal properties of surfaces but quantitative techniques are not yet available due to the presence of unpredictable thermal contact resistance (TCR) at the tip/substrate interface. In this work, we developed two mathematical models, linear and non-linear, that can be used to quantitatively describe the TCR for a variety of surfaces. The developed models bridge heat transfer across the tip/surface interface and enable continuum thermal analysis. This work extends the capability of SThM in quantitative measurement and enables a unique platform for thermal measurement at nanometer spatial resolution, which opens up opportunities to study quantified thermal properties across composite interfaces, multi-layer structures, photovoltaic devices, microelectronics, etc.
|
1. Introduction
The rise of nanotechnology and the rapid development of the microelectronics industry pose the ever-increasing challenge of heat dissipation at the micro-/nano-scale.1,2 Effective cooling demands materials with excellent heat conduction capabilities. Despite the wide spread technologies for making thermally conductive materials, the precise and convenient measurement of thermal conductivity is still a big challenge in the field, especially with materials down to the micro-/nano-scale. In general, thermal conductivity can be measured by two different methods: the steady state method and the transient method.3–5 The steady state techniques are typically useful for bulk materials, which are developed based on Fourier's law. The measurement requires an equilibrium system and thus it is usually time-consuming. The transient methods are less time consuming since the measurement is completed before system equilibrium.6 More importantly, the requirement of sample dimensions is less limited with transient techniques, which enables thermal conductivity measurement at the micron-scale. Over the past few decades, a few transient techniques have been developed including scanning thermal microscopy (SThM), time-domain thermoreflectance (TDTR), time-domain differential Raman (TDDR), and frequency resolved Raman (FRR).7–11 Among these technologies, SThM and TDTR are the dominating ones while only TDTR provides quantitative calculation results of thermal conductivity.7 The capability of SThM could be significantly improved once quantitative measurement becomes possible. For example, the thermal conductivity distribution of heterogeneous materials could be precisely mapped at nanometer resolution and thermal conduction across the nanointerface of composites could be quantified.
SThM was developed by Clayton C. Williams and H. Kumar Wickramasinghe in 1986.12 SThM measurement is usually carried out on an atomic force microscopy (AFM) platform with additional thermal measurement accessories including a thermal tip. During measurement, the thermal tip scans across the sample surface and captures the feedback signal to get analyzable information of local thermal properties.13–16 Because of a unique combination of high spatial resolution and thermal properties, SThM has been widely used to characterize the thermal properties of various materials during the past two decades.17–20 It is widely accepted that SThM results can be used to describe the relative thermal property difference of materials at different regions.21–25 However, the quantification profile of the measurement is still not yet available. By combining other techniques such as spatially resolved Raman spectroscopy or ultra-high vacuum scanning thermal microscopy, a quantitative profile of thermal conductivity can be achieved, but the experimental setup is much more complicated.26–29 To date, there is no established method to obtain thermal conductivity via SThM. Here, the major challenge comes from the varied thermal resistance (or so-called “air gap”) at the tip–sample interface, which greatly influences the overall reading of the thermal signal. Digging into the working principle of SThM, the thermal feedback signal is determined by the heat dissipation through the sample and heat dissipation across the tip–sample interface.8,26,28,30–32 The “air gap” existing between the thermal tip and the sample surface is formed due to the intrinsic roughness of the contacting surface, which is the major obstacle of the quantitative calculation of thermal conductivity using SThM.8,33 The “air gap” can be seen as a thermal resistor which is influenced by many factors at the interface including but not limit to surface roughness, hardness, intrinsic thermal conductivity of the sample, contact force, etc. The “air gap” is the key area that needs to be better understood for bridging the communication between the tip and the sample surface. However, a quantitative method is still not yet available to predict the thermal contact resistance (TCR) at the interface. Therefore, a correlation between the probe current and the thermal conductivity of materials cannot be established and thus SThM is mostly used for qualitative analysis. Once TCR at the interface can be assessed, a direct relationship between the thermal probe current and thermal conductivity becomes possible, which provides a unique tool for measuring the in-plane thermal conductivity distribution in the nanoscale domain.
Here, we developed a microscopic model that well describes the interfacial thermal resistance between the thermal probe and the sample surface in SThM measurement. By using this model, the probe current and thermal conductivity of the testing specimen can be well correlated and thus a quantitative thermal conductivity analysis by SThM becomes possible. This model is developed based on a comprehensive understanding of intrinsic interfacial features including sample roughness and micro-hardness, contacting force as well as the contacting area. Therefore, this model can be extended to study a wide range of material systems. In this work, two different models (linear and non-linear) are developed that quantitatively correlate the probe current and thermal conductivity with different material systems. Material surface features need to be considered for model selection. The thermal conductivity measurement of bulk samples was carried out to verify the predicted thermal conductivity from models based on the SThM probe current. Three polymeric systems, epoxy, polydimethylsiloxane (PDMS) and polyvinyl alcohol (PVA) were selected and tested to verify the effectiveness of the models in predicting thermal conductivity. The results of this work not only provide a good understanding of heat conduction across the nanointerface between the thermal probe and the sample surface, but also offer a reliable method to obtain quantified thermal properties at a scale down to the nanometer range.
2. Modeling part
2.1 Modeling of thermal contact resistance (TCR)
The main challenge of using SThM for thermal conductivity measurement is the quantification of TCR at the tip–sample contacting interface. The “air gap” between the thermal probe and the sample surface is the main reason for TCR during the scanning process, which behaves like a thermal contact resistor. Since the interfacial contact is affected by many factors, it is necessary to simplify the contacting mode and then quantitatively describe it. Scheme 1a shows the contacting mode of the thermal probe scanning on top of the sample surface. Such contacting mode is similar to the situation of two nonconforming rough surfaces described by M. Bahrami.34 Here, we have one rough surface and the other is a smooth thermal probe surface (Scheme 1b). The rough sample surface can be further simplified into two parts based on the heat transfer mode at the interface, i.e. heat spreading of the macro-contact and heat flux of the micro-contact. Macro- and micro-contact resistances are the two major forms of TCR at the interface of the two contact surfaces.35 The total contact resistance (Rcont) can be calculated by the summation of the macro-contact resistance (Rma) and micro-contact resistance (Rmi), eqn (1):Rma is majorly attributed to the thermal spreading resistance (Rs),34 which is formed due to the non-uniform distribution of heat at the heated side and consequently insufficient heat transfer from the heat source.36,37 It is defined as the temperature difference between the heat source and contact area divided by the heat flux, eqn (2):
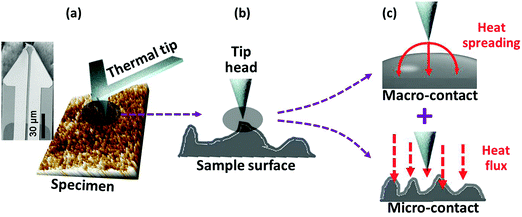 |
| Scheme 1 (a) “Air gap” between the thermal tip and the sample surface; (b) real contact situation between the tip head and the sample surface and (c) simplified contact modes: macro-contact and micro-contact. The cantilever is made from silicon nitride with a size of 150 × 60 × 0.4 um. The resistor metal is composed of NiCr and Pd and the track metal is composed of NiCr and Au. The radius of the thermal tip is ∼100 nm with a height of around 10 μm. The spring constant is 0.25 N m−1. | |
To calculate Rs, Cooper38 proposed a rigorous but simple method by using an isothermal flux tube model. In this model, Rs is a function of spreading resistance factor (φ), radius of macro-contact (a) and effective thermal conductivity (k), eqn (3):
where
φ(
ε) = (1 −
a/
b)
1.5,
b is the radius of the flux tube, and
ke =
2kkt/(
k +
kt). In our tip–sample system,
a is the tip radius,
b is the sample size and
ke is the effective thermal conductivity across the tip–sample interface. Due to the much lower thermal conductivity of the selected polymers compared to the tip (made of NiCr and Pd),
ke can be simplified as
ke = 2
k. Since the tip radius is much smaller than the sample size (
a ≪
b), the equation for
Rma can be further simplified,
eqn (4):
| 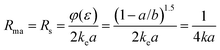 | (4) |
For micro-contact Rmi, Bahrami34 proposed a model by combining the Yovanovich39 empirical equation, eqn (5):
| 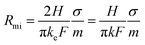 | (5) |
where
H is the micro-hardness,
σ is the effective roughness,
F is the contact force and
m is the effective slope of the tip and the sample. In this work,
H is calculated
via the Oliver and Pharr
40 model by an atomic force microscopy (AFM) based nanoindentation method.
41,42 16 local hardness values are measured, and the reported micro-hardness is the average of the 16 measurements.
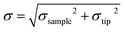
, where
σsample is the sample surface roughness measured by AFM and
σtip is considered to be 0 (assuming a smooth tip surface).
F is the contact force of the tip on the sample surface.
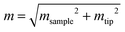
, where
msample is the sample slope obtained from the original AFM 3D topography images without the flatting process and
mtip is considered to be 0 (assuming a flat tip surface).
After combining the macro- and micro-contact thermal resistance, Rcont can be written in eqn (6), where Rcont is related to the thermal conductivity, micro-hardness, roughness of the prepared sample, slope of the sample surface and loading force of the tip during measurement.
| 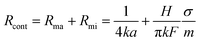 | (6) |
2.2 Modeling of SThM
SThM uses nanofabricated thermal probes with resistive elements to achieve high spatial and thermal resolution with a unique signal detection configuration. Based on the working principle, two different measurement modes can be used in SThM: a temperature contrast mode (TCM) and a conductivity contrast mode (CCM).43–46 In the TCM mode, the thermal probe is heated at a constant current and then it functions as a resistance thermometer. Specifically, as the thermal probe scans through a surface with non-uniform distribution of thermal conductivity, the probe temperature varies with the heat flux across the tip/surface interface that is determined by the thermal conductivity of the contacting area. In the CCM mode, the thermal probe is used as a resistive heater and sufficient energy is applied to keep a constant probe surface temperature. The probe current is measured as an index of thermal conductivity.19,47–49 In this work, the CCM mode was adopted for the systematic study.
Scheme 2 presents the diagram of the SThM CCM mode (Wheatstone bridge) and analysis of the thermal resistances in the system. Following on the fluctuation of electrical current, the Wheatstone bridge adjusts and balances the bridge voltage to measure the probe current. The fixed resistors R1 and R2 form one arm of the bridge while the rheostat Rx and the Rsystem form the other arm of the bridge. Since R1 and R2 are fixed, Rx can be adjusted to balance the bridge once Rsystem changes. The output voltage is in direct proportion to the current through the bridge and thus the probe current. Rsystem is the summation of Rprobe, Rsum and Rsample. Rprobe can be neglected since it is made of highly conductive metals and its thermal resistance is much smaller than the other two. Rsum is contributed by three resistance parts in parallel connection: gas conduction (Rgc), contact (Rcont) and convection and radiation (Rc&v). In most cases, Rgc and Rc&v are orders of magnitude higher than Rcont. Therefore, Rsum can be considered approximately the same as Rcont.
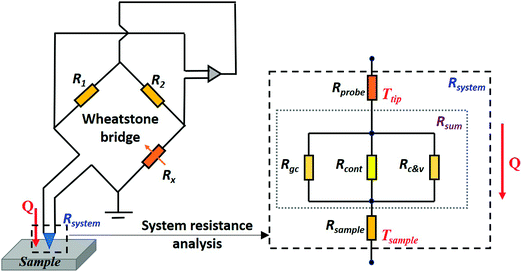 |
| Scheme 2 Diagram of the CCM mode of SThM and systematic analysis of the thermal resistance across the tip/sample surface interface. | |
Considering the total system energy balance, the energy (Q = UI, U is the voltage of the Wheatstone bridge and I is the probe current) in the electrical circuit system will be dissipated in two different ways: one is heat generation at the fixed resistors (QF) and the other is heat flux through the tip/surface interface (QR), eqn (7):
According to Fourier's law,
QR can be written as
eqn (8):
where Δ
T =
Ttip −
Tsample and
R =
Rsample +
Rcont (
Ttip is the temperature of the tip;
Tsample is the temperature of the sample surface).
Rsample can be obtained by the moving heat source method:
50,51
,
r0 is the radius of the heat source which is equal to the tip radius (100 nm) and
k is the thermal conductivity of the sample. Combining
eqn (7) and (8) into
eqn (9):
| 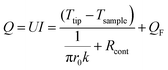 | (9) |
By substituting eqn (6) into eqn (9), eqn (10) can be obtained:
| 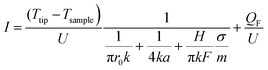 | (10) |
Since Ttip is maintained at a constant temperature during testing, Ttip − Tsample will remain a constant. This has also been verified from the experiment since the change of probe current versus the base input current is less than 0.56%. It is said that the temperature change of the Ttip can be neglected and therefore Ttip − Tsample. Meanwhile, the applied voltage (U) is fixed during the experiment. Under specified experimental conditions, eqn (10) can be rewritten into eqn (11):
| 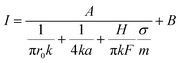 | (11) |
where
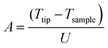
and

;
A and
B are constants relatedto the instrument parameters. From
eqn (11), the probe current
I is related to the thermal conductivity (
k), micro-hardness (
H), sample surface roughness (
σ), loading force of the tip (
F) and slope of the sample and the tip (
m).
H,
σ and
m can be characterized by AFM,
F is a set value that can be adjusted as desired. Thus, a relationship between the probe current
I and thermal conductivity
k can be described after identifying all other parameters in
eqn (11). In this work, three polymer systems, polydimethylsiloxane (PDMS), epoxy and polyvinyl alcohol (PVA), were employed to verify the model in correlating the probe current and thermal conductivity by considering the surface features at the nanoscale.
3. Experimental
3.1 Materials
PDMS (sylgard-184) was provided by Dow Corning. Epoxy resin (826 RS, 178–186 g/eq) was purchased from HEXION Inc. Curing agent (JEFFAMINE T403, max 0.25 wt% water) was provided by Huntsman Corporation. PVA powder (Mw: 14
600–18
600, 99+% hydrolyzed) was purchased from Sigma-Aldrich. Cobalt nitrate (Co(NO3)2, >98%), iron chloride (FeCl2, >97%) and zinc chloride (ZnCl2, >97%) were purchased from Sigma-Aldrich. All chemicals were used as received without further purification.
PDMS films were prepared by mixing different ratios of base monomer and curing agent. The percentage of the curing agent is fixed at 2, 5, 10, 20, 40 and 50 wt%. The PDMS base monomer and curing agent were stirred for 20 min at room temperature and then cured in a Petri dish for 4 hours at 80 °C. The PDMS samples are denoted as PDMS-X (X = 2, 5, 10, 20, 40 and 50%).
Epoxy films were prepared by mixing different loading percentages of the curing agent: 10, 20, 30, 40 and 50 wt%. The well mixed specimen was placed in a rubber mould for curing. Before curing, the samples were degassed in a vacuum oven for 30 min. Then they were cured at 80 °C for 4 hours in a regular oven. The epoxy samples are denoted as Epoxy-X (X = 10, 20, 30, 40 and 50%).
A PVA aqueous solution was prepared at a concentration of 8.0 wt%. PVA/salt solutions were prepared from a mixture of PVA solution and salt solution (Co(NO3)2, FeCl2, ZnCl2) at a fixed molar ratio of 1
:
0.05. All the solutions were placed in an oven and dried at 40 °C (3 days) for thin film preparation. The samples are denoted as PVA, PVA-Co, PVA-Fe and PVA-Zn, respectively.
3.2 Characterization
SThM characterization was performed using a Park System XE7 Atomic Force Microscope. The thermal tip is made of a silicon base and a thermally grown SiO2 cantilever. The base dimension is 2 × 3 mm and the cantilever dimension is 150 × 60 × 1 μm. The resistor metal is made of 5 nm NiCr and 40 nm Pd. The tip height is 12 μm and the tip radius is around 100 nm. The resistance of the tip is around 200–600 Ω. The thermal coefficient of resistivity is about 1 Ω per °C. The spring constant is 0.45 N m−1 and the resonance frequency is 48 KHz. The pre-setting probe current is 1.20 mA. Micro-hardness was characterized by nanoindentation with AFM. A thermal probe was used to collect the nanoindentation data that ensured the captured current signal and measured micro-hardness are from exactly the same region. The micro-hardness results were analyzed by the Olive and Pharr method.40 Surface roughness was analyzed from surface topography. The slope value was determined by the calculation of the line profile via an AFM 3D image without a flatting process. The loading force was set at 1.97, 3.97 and 5.97 nN. Thermal conductivity measurement of the polymer films was carried out at room temperature by using a TCi analyzer (C-Therm, Canada).
4. Results and discussion
4.1 Model testing and simplification
PDMS-20% and Epoxy-30% are selected to test the model and generate useful information for further model validation. Fig. 1 shows the microscopic characterization of both PDMS-20% and Epoxy-30% by AFM-SThM. Fig. 1(a1) shows the original SThM probe current image which is used to calculate the average probe current of the entire surface. The average probe current value is shown inside the scanning image. Fig. 1(b1) shows the 3D topography image without the flattening process and the corresponding height line profile with the calculated slope value m labeled inside the figure. Fig. 1(c1 and d1) show the topography and thermal mapping images of PDMS-20%. A similar characterization was carried out for Epoxy-30% and the results are presented in Fig. 1(a2–d2). All the parameters used for model testing and the calculated Rma and Rmi in both PDMS and epoxy are summarized in Table 1.
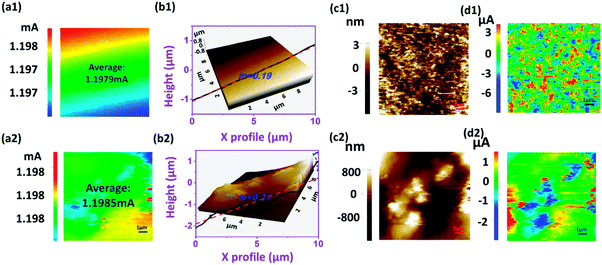 |
| Fig. 1 Characterization of (a1–d1) PDMS-20% and (a2–d2) epoxy-30%. (a1 and a2) Original SThM probe current images, (b1 and b2) AFM 3D images without the flatting process, (c1 and c2) AFM topography images and (d1 and d2) SThM thermal mapping images. | |
Table 1 Summarized parameters for the PDMS and epoxy systems
Materials |
k (Wm−1 K−1) |
a (nm) |
H (kPa) |
σ (nm) |
F (nN) |
m
|
R
ma (WK−1) |
R
mi (WK−1) |
PDMS-20% |
0.203 |
100 |
25.6 |
1.37 |
1.97 |
0.19 |
1.23 × 10−7 |
1.47 × 10−5 |
Epoxy-30% |
0.235 |
100 |
33.0 |
202.03 |
1.97 |
0.19 |
1.06 × 10−7 |
2.18 × 10−7 |
As seen in Table 1, Rma is about two orders of magnitude larger than Rmi in PDMS-20%. Thus, the contribution of Rma in the TCR can be neglected for PDMS. That is, the Rcont can be considered the same as Rma, which is only related to the thermal conductivity of the sample and tip radius. Then, eqn (11) can be rewritten into eqn (12) for PDMS, where a linear relationship between the probe current I and thermal conductivity k can be found:
| 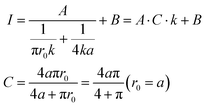 | (12) |
Epoxy-30% shows different surface features as compared to PDMS-20%, especially the surface roughness as summarized in Table 1. The Rmi is in direct proportion to the surface roughness (eqn (5)), which leads to a comparable Rma and Rmi. Therefore, none of these terms in eqn (10) can be neglected in the epoxy system.
Back to the heat transport principle between two solid materials: roughness, hardness and contact force are the three major factors influencing the TCR at the interface.52–55 In this work, the contact force is a pre-setting constant value during the test. The hardness value is on the same order of magnitude for both PDMS and epoxy systems. The varied surface roughness is the major reason for the dramatically different Rmi in both materials. These results indicate that the surface roughness could be the dominating factor for TCR. The sample surface with a small roughness leads to a linear relationship between the probe current and thermal conductivity. While for a surface with a large roughness, the probe current is related to thermal conductivity and other factors as well (mainly micro-hardness and roughness).
4.2 Model validation
In Section 4.1, two different models were developed for PDMS and epoxy systems, to correlate the probe current and thermal conductivity by analyzing the interfacial TCR. To verify the models, more specimens with varied base/curing agent ratios were tested. Fig. 2 and 3 summarize the features of PDMS and epoxy resin characterized by AFM and SThM. In general, the surface roughness of all the PDMS samples is much smaller than that of the epoxy ones, as evidenced by the scales of the topography images in Fig. 2(c1–c6) and 3(c1–c5). It is worth noting that the topography pattern of the samples shows a certain similarity to the pattern of probe current distribution. This is more obvious in epoxy samples where larger surface roughness was observed. Such a similar pattern of topography and the probe current indicates the remarkable influence of the surface geometry on interfacial thermal transport. According to the working principle of SThM, the topography capturing system and the probe current signal feedback loop are two independent channels and they are not supposed to interfere with each other. Because the probe current is a result of the combined action of sample thermal conductivity and Rcont, the only explanation for the topography-related probe current distribution is that the heat flux caused by the TCR is large enough and poses a great influence on the final observation in the thermal image. In other words, the feedback signal is mostly contributed by the heat flux of the tip–sample interface rather than from the sample itself. Such results are in good agreement with the literature reports from other researchers.28,56 Based on the proposed model in this work, a larger roughness value means higher TCR and thus lower probe current. For the PDMS system, the surface roughness is much smaller (<2 nm) and no obvious correlation can be found between the topography image and the thermal image. Thus, the probe current is randomly distributed without any specific patterns. All the samples show a similar slope value (m) of around 0.2. The small difference in m values is not expected to impact further calculation significantly.
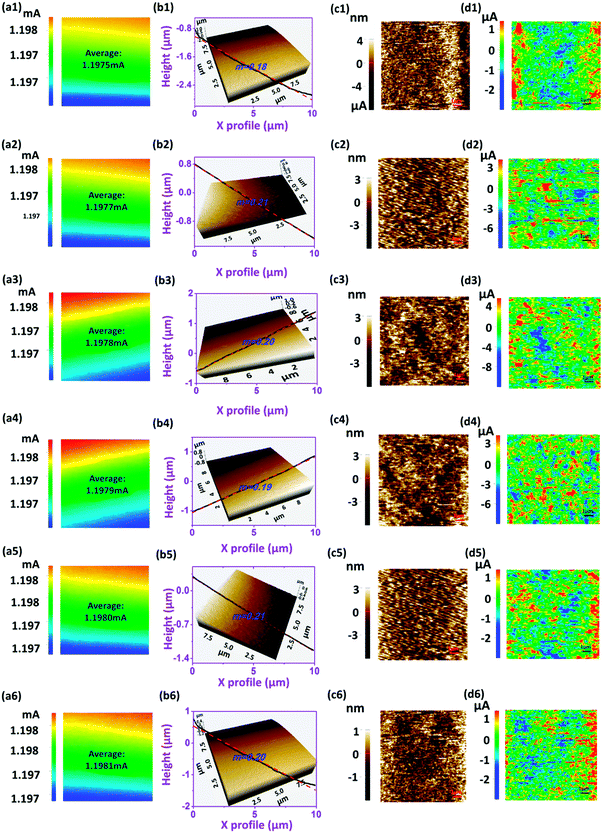 |
| Fig. 2 AFM and SThM characterization of PDMS. (a1–a6) Original SThM probe current images, (b1–b6) AFM 3D images without the flatting process, (c1–c6) AFM topography images and (d1–d6) SThM thermal mapping images. 1–6 means the increasing loading of the curing agent in PDMS: 2, 5, 10, 20, 40 and 50 wt%. | |
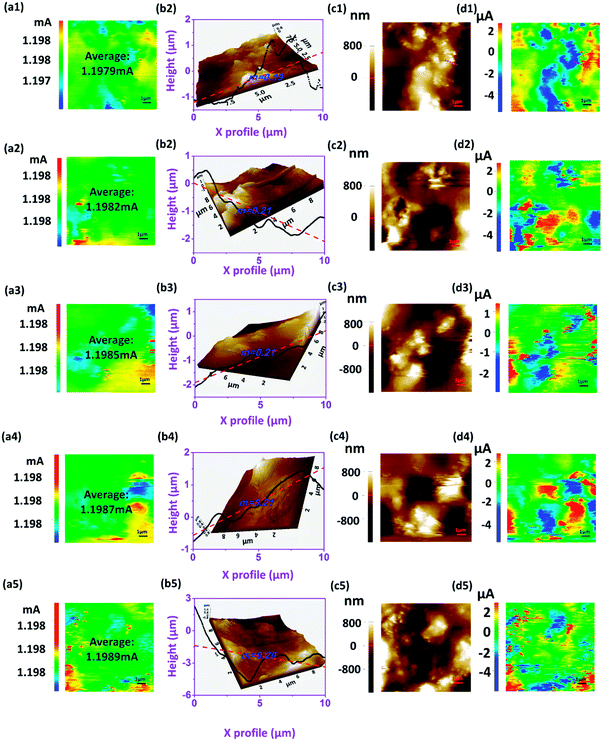 |
| Fig. 3 AFM and SThM characterization of epoxy. (a1–a5) Original SThM probe current images, (b1–b5) AFM 3D images without the flatting process, (c1–c5) AFM topography images and (d1–d5) SThM thermal mapping images. 1–6 means the increasing loading of the curing agent in epoxy: 10, 20, 30, 40 and 50 wt%. | |
The probe current and the bulk thermal conductivity of PDMS are measured by SThM CCM mode and TCi, respectively, Fig. 4(a and b). Both of them increase with the increasing load percentage of curing agent. Fig. 4(c and d) show the micro-hardness and surface roughness as a function of the percentage of curing agent, where a gradual increase of both parameters was observed. It should be noticed that the variation of the hardness and roughness are still at the same level of magnitude. With that, eqn (12) can be used for all six PDMS samples and thus it is expected that the probe current and thermal conductivity of the materials should follow a linear relationship. To verify the model, the probe current against thermal conductivity was plotted in Fig. 4(e). Since the variation of probe current is very small, the differentiation of the readings becomes difficult. Therefore, a revised probe current (I*) was used in this work and the data are replotted in Fig. 4(f):
Fig. 4(f) shows a highly linear relationship (
R2 > 0.99) between
I* and thermal conductivity. Such results demonstrate a solid linear correlation between the microscale probe current and macroscale thermal conductivity. The proposed model well describes such relationship and can be extended to predict thermal conductivity based on microscale measurements.
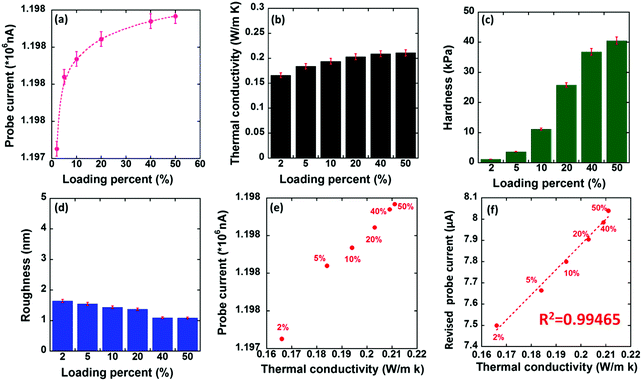 |
| Fig. 4 Parameters used for calculation in PDMS systems. (a) Probe current, (b) thermal conductivity, (c) micro-hardness, (d) roughness, (e) probe current vs. thermal conductivity and (f) revised probe current vs. thermal conductivity and its linear fitting. | |
Similar to the PDMS system, Fig. 5(a–d) summarize the characterization results of the epoxy system. Compared to the 30% curing agent system, both the micro-hardness and roughness values of the other 4 epoxy samples are at the same order of magnitude. Therefore, the model cannot be simplified into a linear form and eqn (11) is used to model the tip–surface thermal transport for epoxy. Fig. 5(e) provides a relationship between the probe current and thermal conductivity, which does not follow a linear pattern. Since micro-contact resistance cannot be neglected in eqn (11), the probe current I is not only related to thermal conductivity; other parameters should be considered as well. Taking the instrument parameters A and B from the PDMS model (A is the slope, and B is the intercept from the linear fitting) into the epoxy model, a simplified relationship can be constructed between the probe current and thermal conductivity. A good relationship between the measured and calculated thermal conductivity was found with less than 5% error, Fig. 5(f).
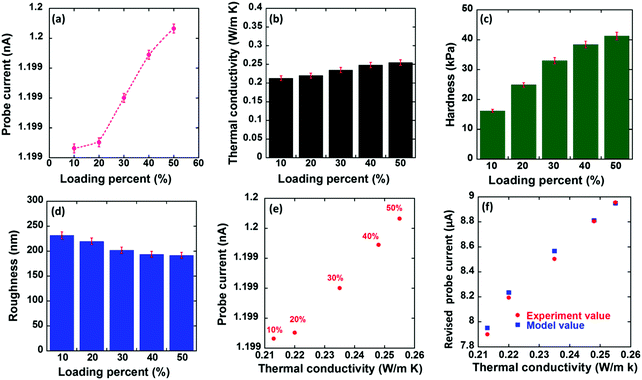 |
| Fig. 5 Parameters used for calculation in the epoxy systems. (a) Probe current, (b) thermal conductivity, (c) micro-hardness, (d) roughness, (e) probe current vs. thermal conductivity and (f) comparison of the modeling and experiment results for revised probe current. | |
All the previous measurements were carried out at a loading force of 1.97 nN. The variation of loading force will change the interfacial contact and thus heat transport across the contacting interface. The robustness of the developed models needs to be tested under different loading force conditions. In this work, two other sets of loading forces are selected: 3.97 and 5.97 nN. The probe currents are collected under specified loading force conditions on all the samples. At the same time, the probe current is calculated from the developed models by substituting the force value. Fig. 6(a) shows the comparison of the revised probe current from both the experiment and model prediction for PDMS under three different loading force conditions. It is observed that the majority of the data points are overlapped at each loading percent indicating the negligible influence of the loading force on the probe current measurement of PDMS as well as the robustness of the model under different testing conditions. These results are expected since the loading force only affects the Rmi in the model and the Rmi can be neglected for the PDMS system. As a result, the changes of the loading force F do not influence the probe current measurement. Fig. 6(b) shows the comparison of the experiment/model probe current values of the epoxy models. In general, the model and experimental results follow a very similar trend at different loading forces indicating the good adaptability of the epoxy model. However, the amplitude of the probe current increases with increasing loading force. By increasing the loading force, a better interfacial contact can be expected especially on a rough surface. As a result, the thermal resistance at the interface can be reduced by a tight contact and thus a larger probe current across the interface. In the developed epoxy model, the increase of loading force F decreases the term Rmi and this increases the probe current I. Based on these results, it can be concluded that the two models developed in this work are reliable to correlate the probe current and thermal conductivity of polymeric materials with different surface features (low roughness and high roughness).
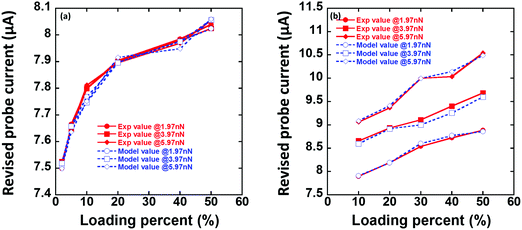 |
| Fig. 6 (a) Comparison of the revised probe current by experiment and the revised probe current by model under different loading forces for the PDMS system, and (b) comparison of the revised probe current by experiment and the revised probe current by model under different loading forces for the epoxy system. | |
4.3 Model extrapolation with PVA and doped PVA
To further verify the effectiveness of the model to be used for other types of polymeric materials, PVA and doped PVA (PVA-Co, PVA-Fe and PVA-Zn) samples were employed in this work. The AFM and SThM characterization results are summarized in Fig. 7(a–d). It is apparent that the PVA system follows a similar pattern to PDMS; both of their surface roughness are around a few nanometers. Therefore, the linear model (eqn (11)) can be applied for the PVA system. Fig. 8(a–e) show the characterization results of the PVA based samples. Since the thermal conductivity of the PVA samples is much larger than that of the PDMS samples, the extrapolation method is used here to predict the thermal conductivity. The predicted thermal conductivity obtained from the model is marked with a cross symbol on the extrapolated line and the corresponding experimental results are plotted as well. The experimental results are all closely distributed near the predicted values as shown in Fig. 8(f). All these results confirm the robustness of the developed model in predicting the thermal conductivity in a wide range of polymeric materials.
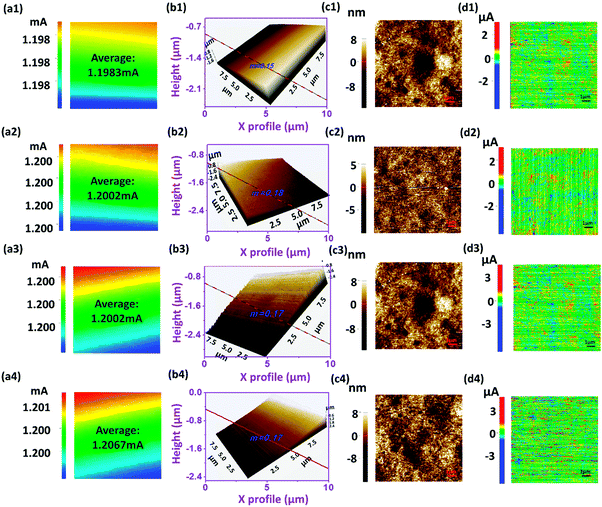 |
| Fig. 7 AFM and SThM characterization of PVA–salt systems. (a1–a4) Original SThM probe current images, (b1–b4) AFM 3D images without the flatting process, (c1–c4) AFM topography images and (d1–d4) SThM thermal mapping images. 1–4 correspond to PVA, PVA-Co, PVA-Fe and PVA-Zn, respectively. | |
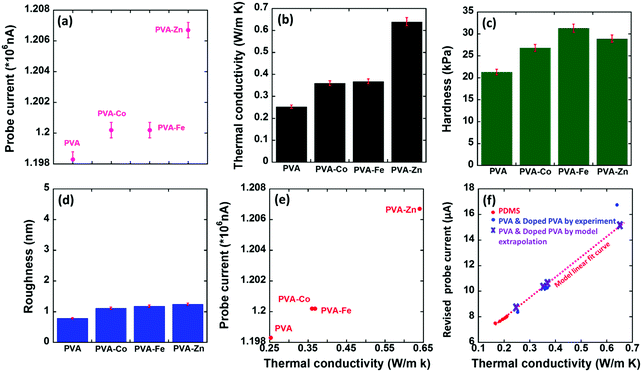 |
| Fig. 8 Parameters used for calculation in the PVA–salt systems. (a) Probe current, (b) thermal conductivity, (c) micro-hardness, (d) roughness, (e) probe current vs. thermal conductivity and (f) comparison of the revised probe current values of the PVA systems by experiment and model extrapolation. | |
5. Conclusions
In summary, this work derives a mathematical model that well correlates the SThM probe current and bulk thermal conductivity of polymeric materials. The key challenge of this work, quantification of thermal contact resistance (TCR), is addressed by implementing micro-structural features at the interface such as surface roughness, hardness, etc. Two different models, a linear model and a non-linear model, were successfully developed which can be used to predict the thermal conductivity of materials by measuring the SThM surface probe current. Surface roughness is the major criterion for model selection. That is, the linear model fits samples with a smooth surface while the non-linear model applies to samples with a rough surface. Both models provide satisfactory prediction results in three polymeric material systems: PDMS, epoxy and PVA. Changing the loading force does not affect the effectiveness of the model, which expands the applicability of the developed models to a wider range of materials. Moreover, the model has been shown to be valid when extrapolated to predict thermal conductivity beyond the range of model development. This work developed a simple but rigorous method to mathematically describe the thermal contact resistance at the tip/surface interface and offer reliable models to enable a quantitative characterization of thermal conductivity by SThM. This unique feature endows SThM with a new capability in quantitative thermal analysis with spatial resolution down to nanometers, which is promising to quantify the thermal conduction across the interfaces within composites, multi-layer membranes, microelectronics, etc.
Conflicts of interest
The authors declare no conflict of interest.
Acknowledgements
We acknowledge the Donors of the American Chemical Society Petroleum Research Fund (#55570-DNI10) and the National Science Foundation (CBET-1603264). Partial support from the start-up fund of The University of Akron is also acknowledged.
References
- B. Song, Y. Ganjeh, S. Sadat, D. Thompson, A. Fiorino, V. Fernández-Hurtado, J. Feist, F. J. Garcia-Vidal, J. C. Cuevas and P. Reddy, Nat. Nanotechnol., 2015, 10, 253–258 CrossRef CAS PubMed
.
- Y. Zhang, H. Han, N. Wang, P. Zhang, Y. Fu, M. Murugesan, M. Edwards, K. Jeppson, S. Volz and J. Liu, Adv. Funct. Mater., 2015, 25, 4430–4435 CrossRef CAS
.
- R. Von Herzen and A. Maxwell, J. Geophys. Res., 1959, 64, 1557–1563 CrossRef
.
- K. A. Borup, J. De Boor, H. Wang, F. Drymiotis, F. Gascoin, X. Shi, L. Chen, M. I. Fedorov, E. Müller and B. B. Iversen, Energy Environ. Sci., 2015, 8, 423–435 CAS
.
- H. Ji, D. P. Sellan, M. T. Pettes, X. Kong, J. Ji, L. Shi and R. S. Ruoff, Energy Environ. Sci., 2014, 7, 1185–1192 CAS
.
- J. D. Richards, IEEE Electr. Insul. Mag., 1988, 4, 23–32 CrossRef
.
- M. D. Losego, M. E. Grady, N. R. Sottos, D. G. Cahill and P. V. Braun, Nat. Mater., 2012, 11, 502–506 CrossRef CAS PubMed
.
- K. Kim, B. Song, V. Fernández-Hurtado, W. Lee, W. Jeong, L. Cui, D. Thompson, J. Feist, M. H. Reid and F. J. García-Vidal, Nature, 2015, 528, 387–391 CrossRef CAS PubMed
.
- C. Li, S. Xu, Y. Yue, B. Yang and X. Wang, Carbon, 2016, 103, 101–108 CrossRef CAS
.
- L. Su and Y. Zhang, Appl. Phys. Lett., 2015, 107, 071905 CrossRef
.
- S. Wheaton, R. M. Gelfand and R. Gordon, Nat. Photonics, 2015, 9, 68–72 CrossRef CAS
.
- C. Williams and H. Wickramasinghe, Microelectron. Eng., 1986, 5, 509–513 CrossRef
.
- D. Halbertal, J. Cuppens, M. B. Shalom, L. Embon, N. Shadmi, Y. Anahory, H. Naren, J. Sarkar, A. Uri and Y. Ronen, Nature, 2016, 539, 407–410 CrossRef CAS PubMed
.
- S. Huxtable, D. G. Cahill, V. Fauconnier, J. O. White and J.-C. Zhao, Nat. Mater., 2004, 3, 298–301 CrossRef CAS PubMed
.
- A. Böker, Y. Lin, K. Chiapperini, R. Horowitz, M. Thompson, V. Carreon, T. Xu, C. Abetz, H. Skaff and A. Dinsmore, Nat. Mater., 2004, 3, 302–306 CrossRef PubMed
.
- L. Cui, W. Jeong, V. Fernández-Hurtado, J. Feist, F. J. García-Vidal, J. C. Cuevas, E. Meyhofer and P. Reddy, Nat. Commun., 2017, 8, 14479 CAS
.
- Y. Li, N. Mehra, T. Ji, X. Yang, L. Mu, J. Gu and J. Zhu, Nanoscale, 2018, 10, 1695–1703 RSC
.
- L. Shi, S. Plyasunov, A. Bachtold, P. L. McEuen and A. Majumdar, Appl. Phys. Lett., 2000, 77, 4295–4297 CrossRef CAS
.
- L. Mu, Y. Li, N. Mehra, T. Ji and J. Zhu, ACS Appl. Mater. Interfaces, 2017, 9, 12138–12145 CAS
.
- N. Mehra, L. Mu, T. Ji, Y. Li and J. Zhu, Compos. Sci. Technol., 2017, 151, 115–123 CrossRef CAS
.
- V. Gorbunov, N. Fuchigami, J. Hazel and V. Tsukruk, Langmuir, 1999, 15, 8340–8343 CrossRef CAS
.
- X. Yan, Y. Imai, D. Shimamoto and Y. Hotta, Polymer, 2014, 55, 6186–6194 CrossRef CAS
.
-
J. Christofferson, K. Maize, Y. Ezzahri, J. Shabani, X. Wang and A. Shakouri, 2007 International Conference on Thermal Issues in Emerging Technologies: Theory and Application, 2007, 3-9.
- P. D. Tovee and O. V. Kolosov, Nanotechnology, 2013, 24, 465706 CrossRef PubMed
.
- I. Jo, I.-K. Hsu, Y. J. Lee, M. M. Sadeghi, S. Kim, S. Cronin, E. Tutuc, S. K. Banerjee, Z. Yao and L. Shi, Nano Lett., 2010, 11, 85–90 CrossRef PubMed
.
- G. B. M. Fiege, A. Altes, R. Heiderhoff and L. J. Balk, J. Phys. D: Appl. Phys., 1999, 32, L13 CrossRef CAS
.
- A. Soudi, R. D. Dawson and Y. Gu, ACS Nano, 2010, 5, 255–262 CrossRef PubMed
.
- K. Kim, J. Chung, G. Hwang, O. Kwon and J. S. Lee, ACS Nano, 2011, 5, 8700–8709 CrossRef CAS PubMed
.
- K. Kim, W. Jeong, W. Lee and P. Reddy, ACS Nano, 2012, 6, 4248–4257 CrossRef CAS PubMed
.
- A. Majumdar, Annu. Rev. Mater. Sci., 1999, 29, 505–585 CrossRef CAS
.
- K. Kim, W. Jeong, W. Lee, S. Sadat, D. Thompson, E. Meyhofer and P. Reddy, Appl. Phys. Lett., 2014, 105, 203107 CrossRef
.
-
A. Assy, S. Lefèvre and S. Gomès, 21st International Workshop on Thermal Investigations of ICs and Systems (THERMINIC), 2015, 1–5.
- K. Yoon, G. Hwang, J. Chung, H. goo Kim, O. Kwon, K. D. Kihm and J. S. Lee, Carbon, 2014, 76, 77–83 CrossRef CAS
.
- M. Bahrami, J. Culham, M. Yovanovich and G. Schneider, J. Thermophys. Heat Transfer, 2004, 18, 218–227 CrossRef CAS
.
- R. S. Prasher and P. E. Phelan, J. Appl. Phys., 2006, 100, 063538 CrossRef
.
- Y. Muzychka, M. Yovanovich and J. Culham, J. Thermophys. Heat Transfer, 2006, 20, 247–255 CrossRef CAS
.
- Y. Muzychka, M. Yovanovich and J. Culham, J. Thermophys. Heat Transfer, 2004, 18, 45–51 CrossRef CAS
.
- M. Cooper, B. Mikic and M. Yovanovich, Int. J. Heat Mass Transfer, 1969, 12, 279–300 CrossRef
.
- M. Yovanovich, AIAA Paper, 1982, 81, 83–95 Search PubMed
.
- G. Pharr and W. Oliver, MRS Bull., 1992, 17, 28–33 CrossRef
.
- T. Young, M. Monclus, T. Burnett, W. Broughton, S. Ogin and P. Smith, Meas. Sci. Technol., 2011, 22, 125703 CrossRef
.
- K. Miyahara, N. Nagashima, T. Ohmura and S. Matsuoka, Nanostruct. Mater., 1999, 12, 1049–1052 CrossRef
.
- K. Kim, B. Song, V. Fernández-Hurtado, W. Lee, W. Jeong, L. Cui, D. Thompson, J. Feist, M. H. Reid and F. J. García-Vidal, Nature, 2015, 528, 387–391 CrossRef CAS PubMed
.
- L. Gross, Nat. Chem., 2011, 3, 273–278 CrossRef CAS PubMed
.
- F. Guo, N. Trannoy and J. Lu, Mater. Sci. Eng., A, 2004, 369, 36–42 CrossRef
.
- F. Guo, N. Trannoy and J. Lu, Superlattices Microstruct., 2004, 35, 445–453 CrossRef CAS
.
- A. Dawson, M. Rides, A. S. Maxwell, A. Cuenat and A. R. Samano, Polym. Test., 2015, 41, 198–208 CrossRef CAS
.
- A. Dawson, M. Rides, A. Cuenat and L. Winkless, Int. J. Thermophys., 2013, 1–18 Search PubMed
.
- Y. Bellouard, M. Dugan, A. A. Said and P. Bado, Appl. Phys. Lett., 2006, 89, 161911 CrossRef
.
- P. Dawson and S. Malkin, J. Eng. Ind., 1984, 106, 179–186 CrossRef
.
- Y. Gu, X. Ruan, L. Han, D. Zhu and X. Sun, Int. J. Thermophys., 2002, 23, 1115–1124 CrossRef CAS
.
- M. Grujicic, C. Zhao and E. Dusel, Appl. Surf. Sci., 2005, 246, 290–302 CrossRef CAS
.
- R. S. Prasher, J. Heat Transfer, 2001, 123, 969–975 CrossRef CAS
.
- M. Bahrami, M. M. Yovanovich and J. R. Culham, Int. J. Heat Mass Transfer, 2005, 48, 3284–3293 CrossRef
.
- C. Fieberg and R. Kneer, Int. J. Heat Mass Transfer, 2008, 51, 1017–1023 CrossRef CAS
.
- L. Shi and A. Majumdar, J. Heat Transfer, 2002, 124, 329–337 CrossRef CAS
.
|
This journal is © The Royal Society of Chemistry 2018 |
Click here to see how this site uses Cookies. View our privacy policy here.