DOI:
10.1039/C8CS00317C
(Review Article)
Chem. Soc. Rev., 2018,
47, 4781-4859
Synergy between nanomaterials and volatile organic compounds for non-invasive medical evaluation†
Received
21st April 2018
First published on 11th June 2018
Abstract
This article is an overview of the present and ongoing developments in the field of nanomaterial-based sensors for enabling fast, relatively inexpensive and minimally (or non-) invasive diagnostics of health conditions with follow-up by detecting volatile organic compounds (VOCs) excreted from one or combination of human body fluids and tissues (e.g., blood, urine, breath, skin). Part of the review provides a didactic examination of the concepts and approaches related to emerging sensing materials and transduction techniques linked with the VOC-based non-invasive medical evaluations. We also present and discuss diverse characteristics of these innovative sensors, such as their mode of operation, sensitivity, selectivity and response time, as well as the major approaches proposed for enhancing their ability as hybrid sensors to afford multidimensional sensing and information-based sensing. The other parts of the review give an updated compilation of the past and currently available VOC-based sensors for disease diagnostics. This compilation summarizes all VOCs identified in relation to sickness and sampling origin that links these data with advanced nanomaterial-based sensing technologies. Both strength and pitfalls are discussed and criticized, particularly from the perspective of the information and communication era. Further ideas regarding improvement of sensors, sensor arrays, sensing devices and the proposed workflow are also included.

Yoav Y. Broza
| Yoav Y. Broza received his PhD in Biotechnology & Food Engineering in 2009 from the Technion – Israel Institute of Technology. He is a senior researcher, head of the biological and analytical team in the Laboratory for Nanomaterial-Based Devices, headed by Prof. Hossam Haick. Yoav served as a researcher and consultant in several biomed and food-tech startups in the field of nano-sensors. Additionally, Yoav holds an external lecturer position in the Technion. His current research interests include volatolomics studies, single cell analysis, identification of disease and infectious-agents' biomarkers through volatile gas analysis by analytical methods and nano-arrays, and the development of novel technologies for gas sampling applications. |

Rotem Vishinkin
| Rotem Vishinkin received her Bachelor's (2012) degree in Biochemical Engineering from the Technion – Israel Institute of Technology, Israel. Rotem joined the group of Professor Hossam Haick during her BSc research project on development and characterization of chemical sensors based on field effect transistors of Si nanowires. In August 2014, Rotem obtained her MSc degree in Chemical Engineering and currently continues her direct PhD studies on non-invasive tuberculosis detection based on volatile organic biomarker patterns from skin and breath. Her research interests include development of field effect transistors and gold nanoparticle-based sensors for sensing applications and detection of disease-related volatile biomarkers, especially those emitted from the skin, using mass spectrometry methods and nano-arrays of chemical sensors. |

Orna Barash
| Orna Barash has finished her PhD in the Laboratory for Nanomaterial-based Devices (LNBD) under the supervision of Prof. Hossam Haick in 2010. Her research focus was on “Detection and Characterization of Volatile Organic Compounds Exhaled by In Vitro Lung Cancer Cells”. Orna has been working as a Researcher with the LNBD group for 2 years and held responsibilities of research management. Orna has recently joined a spin-off company named NanoScent as the Director of Research. NanoScent uses the core technology developed by prof. Hossam Haick at the Technion for scent detection in various fields. |
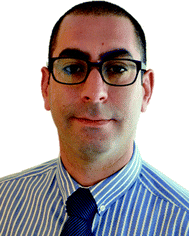
Morad K. Nakhleh
| Morad K. Nakhleh holds a BSc in Emergency Medicine (2006) and MSc in Medical Sciences (2011). In 2014, he received his PhD in Nanoscience and Nanotechnology, under the supervision of Prof. Hossam Haick, at the Russell Berrie Nanotechnology Institute, Technion-IIT, Haifa, Israel. Currently, Dr Nakhleh is performing his postdoctoral studies at INSERM UMR_S999, Le Plessis Robinson, France. In his current research, Dr Nakhleh focuses on the volatolomics associated with pulmonary arterial hypertension (PAH). His goal is to explore the underlying biological and molecular mechanisms of the disease by profiling the volatolome both on the vascular level and in exhaled breath, searching for early biomarkers associated with the diseases. |
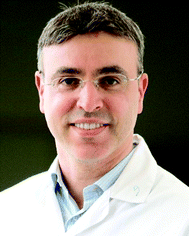
Hossam Haick
| Hossam Haick is a Full Professor in the Technion – Israel Institute of Technology and head of three major European consortia, has received numerous prestigious Prizes and Awards and was included in several important lists, including the list of the world's 35 leading young scientists published by MIT's Technology Review, the Nominet Trust 100 list (London), which includes the world's 100 most influential inventors and digital developments, and the Los Angeles-based GOOD Magazine's list of the 100 most influential people in the world. His research interests include nanomaterial-based chemical (flexible) sensors, electronic skin, nanoarray devices for screening, diagnosis, and monitoring of disease, breath analysis, volatile biomarkers, and molecular electronic devices. |
1. Introduction
Medical diagnostics, or disease diagnosis, is the identification of the presence of a specific disease, based on symptoms manifested in an individual. In order to save lives and provide patients with the optimum treatment, data classification is particularly important for comprehending the etiology and pathogenesis of a disease.1,2 Therefore, disease classification is based on the following steps: first, identifying as many candidate conditions as possible that can explain the expressed symptoms. Second, an elimination process to rule out the less probable conditions by additional medical tests and observations. And last, fine-tuning, i.e., allowing the physician to reach the stage where only one of the most probable disease candidates remains.1,2 Modern diagnostics relates to two main categories of diseases: non-infectious (non-transmissible) and infectious diseases.3,4 More than 60% of the worldwide disease-related deaths are due to non-infectious diseases; they could be slow developing chronic conditions, or more acute ones that result in rapid death.3 The likelihood of certain non-infectious diseases is influenced by different risk factors, such as genetic background, environment and lifestyle. Thus, related deaths in the next 12 to 15 years are expected to increase by more than 40%.3 The second most frequent cause of death worldwide is infectious diseases, which leads to >15 million deaths annually.5
For the medical world to cope with these growing numbers, novel diagnostic approaches and improved technologies are needed. A whole range of diagnostic methodologies and technologies are being used for diverse infectious and non-infectious diseases.3–5 It is gradually being accepted that better-quality diagnostic tools should be developed to give a personalized approach. Such an approach should take into full consideration detection of individuals at risk of developing diseases, data interpretation of the diagnostic tests, delivering prognostic data, and foreseeing and following the efficacy of therapies.6,7 Nowadays, diagnosis is based on a physical examination followed by a range of tests that include chemical, imaging, endoscopic, immunological, genomic and pathological procedures among others. Each has pros and cons, invasive vs. non-invasive; life threatening vs. safe; expensive vs. low-cost; complicated vs. simple; point of care vs. offline lab; fast vs. slow.
One rapidly developing strategy in the diagnostic field is based on volatolomic analysis, i.e. the combined analysis of volatile organic compounds (VOCs) emitted from different bodily sources (see below and ref. 8). Real-world implementation of this approach will benefit from a true point-of-care, non-invasive, easy to use and relatively low-cost system that can be an optimal screening tool for different diseases. VOC sampling, in general, and breath sampling, in particular, have high compliance because they are patient-friendly. The general concept addresses diseases in different organs by sampling VOCs from different bodily fluids or tissue (e.g. breath, skin, urine, and blood) and measuring them by different sensor techniques for point-of-care (POC) diagnostics (Fig. 1).
 |
| Fig. 1 VOC-based diagnostics approach is divided into several levels. Addressing diseases in different organs; VOC sampling from different bodily fluids/places (such as breath, skin, urine, blood etc.); sensor measurement using different sensor techniques; and POC diagnostics based on classification algorithms. | |
For the detection of disease-related VOCs, 2 main sensing strategies can be used. The first is based on a selective sensing mechanism, in which a chemical sensor is designated to interact and measure the presence of a single compound in a mixture (lock-and-key like). For this approach to be applied, one needs to have specific biomarker information on the medical condition being targeted. The second strategy is bio-inspired by the sense of smell and is based on semi-selective/cross-reactive sensors combined with pattern recognition methods. Since it is hard to find a single and unique VOC that could perform as a stand-alone diagnostic for a specific disease in the clinical world, a cross-reactive sensing approach is more favorable. Nevertheless, for this approach to mature, further progress in sensor technology – increased sensitivity, data-mining and pattern recognition power – is needed. We herein provide an updated overview of current advances in emerging technologies in the field of nanomaterial-based sensors, which are used, or have the potential, for disease and biomarker detection using VOCs. We also discuss new approaches in the field, such as hybrid sensors and future perspectives.
2. Origin and emission of volatile organic compounds from human body sources
During each cell's life span, extensive and continuous metabolic processes take place to sustain life, and adapt and react according to local and systemic stimuli.9 Among the main purposes of these chemical changes are energy production, anabolism (building up of cellular components), catabolism (breaking down of organic matter) and elimination of cellular waste.9 Under healthy and normal conditions, these processes ensure the well-being of the cells in maintaining vital functions. Metabolic reactions are facilitated mainly by enzymes that in turn interact with different biochemical compounds, including amino acids, proteins, lipids, carbohydrates, minerals and others.9
During metabolism, a wide range of byproducts are formed, including a family of organic chemicals characterized by high vapor pressure at both room and body temperatures, thus known as VOCs.10,11 For example, ketones are produced by cells in the glycolytic pathway of energy production, whereas acetone is synthesized by the decarboxylation of ketone bodies.12 Isoprene, on the other hand, is formed in the mevalonic pathway of cholesterol synthesis in the cytosol.11 Many molecular pathways and enzymes constantly produce dozens of other VOCs. Interestingly, due to their relatively small size, low molecular weight and their volatility, these compounds spread from their original microenvironment through blood circulation to distal organs, from which they may be excreted in different body fluids. VOCs are detectable at low concentrations in the peripheral blood, sweat (skin secretion), urine (kidney), feces (gastrointestinal tract) and exhaled breath (lungs). Therefore, VOCs can be highly informative regarding metabolic activity.12–14
When subjected to stress under systemic or local stimuli, cells usually respond by activation of different intracellular cascades involving multiple signaling pathways and enzymatic activity. These rapid metabolic reactions change baseline activities and/or the functions of the cells (e.g., accumulation of reactive oxygen species or hypoxia), adapting them to changing micro-environmental requirements.13,15,16 A wide spectrum of pathophysiological processes occur during disease states that are followed by adaptive and altered metabolism, ranging from the single cell level and up to whole organ response such as the liver and the kidney to maintain homeostasis. During these responses, extensive alterations in VOC production are reflected in the blood composition and excreted body fluids. Therefore, VOCs are informative non-invasive biomarkers, reflecting alterations in metabolism during disease.17
Hundreds of different VOC metabolites have been identified in human samples from different sources. VOCs in feces, for example, could be due to bacterial activity in the colon, as well as due to diffusion throughout the colon from both cellular activity and blood stream.18 In the kidney, on the other hand, VOCs diffuse throughout the glomerular filtrate and end up in the urine. Acids, alcohols, ketones, aldehydes, amines, N-heterocycles, O-heterocycles, VSCs and other VOCs have been detected and quantified in urine.19,20 A range of compound classes appear in skin VOC profiles, including aldehydes, carboxylic acids, alcohols, ketones, esters and amines.21 Skin VOCs are derived mainly from gland secretions and skin microbiota activity; therefore, skin VOC diversity and concentrations are directly correlated with the distribution of glands on the skin as well as cutaneous microorganisms.22,23
Aside from the systemic alterations in the rate of production of VOCs, their exhaled concentrations are influenced by the difference between the alveolar concentration (Calveolar) and ambient air concentration of a given compound. As summarized in the Farhi equation, Calveolar is positively correlated with the concentration in mixed venous blood.24 On the other hand, it is negatively correlated with the ratio between the alveolar ventilation and cardiac output. Thus, during stress/effort, for example, increase in alveolar ventilation leads to a decrease in Calveolar. The second factor negatively affecting the Calveolar is the ‘blood:air’ partition coefficient, reflecting the solubility of a given compound in the blood. Higher solubility in the blood results with higher binding of the VOC to blood proteins, thus negatively affecting Calveolar.17,24 The third factor is the ‘fat:blood’ partition coefficient, since a wide range of VOCs can be stored in the fat compartments. Therefore, the total fat tissue and the total blood volume of an individual should be taken into account as factors affecting the blood concentrations and, as a result, the final alveolar concentrations.17,24
3. Harnessing VOC data from analytical approaches towards sensor design
Two main approaches have been developed that are used to assess VOCs in biological samples. The goal of the first is to quantitatively profile each of the compounds in each mixture. Identification of the VOC spectrum allows one to study each compound separately and estimate the likelihood that it can serve as an individual biomarker of disease. This approach is based on variations of chromatography w/wo spectrometry, including, but not limited to, Gas Chromatography linked with Mass Spectrometry (GC-MS), proton transfer reaction mass spectrometry, and selected ion flow tube mass spectrometry. Due to these analytical variations and different sampling methods, it is difficult to reach a singular VOC pattern for each disease. On the other end of the spectrum, the second approach is based on different types of chemically-sensitive gas sensors that give a total assessment of the mixture/profile of VOCs in a sample.
Semi-selective/cross-reactive sensors combined with artificial intelligence algorithms have been adopted, which allows the identification of patterns of VOCs as conjoined markers of a disease. While this lacks the ability to identify each VOC present, the overall pattern can be detected and used in future to classify similar patterns. Therefore, in cases of multiple VOC alterations, such as in systemic diseases, this approach should be more compatible with assessment of any disease-associated patterns.14,23,25 In general, these 2 approaches are independent. However, to gain advantage when designing and developing novel sensing approaches, the knowledge and connection of specific molecules and their physiochemical properties are important. Towards this end, we updated a compilation of the current specific disease-related VOCs (patterns, as obtained from different studies) and their source of sampling, as summarized in Fig. 2, 3 and Table S1 (ESI†). This compilation shows the fraction and relation among the chemical family, disease and source; it allows researchers to focus and tune their potential gas-sensing system, based on currently available data. These figures can serve as a map that allows the reader to decipher the “disease-VOC-source” connection. This complete information can aid in choosing the correct (existing) sensing device for a clinical condition of interest, tuning the sensor development methodology based on chemical data of potential biomarkers, and developing suitable sampling methods based on the biomarkers’ source that fit real world clinical use.
 |
| Fig. 2 Relationships between chemical families (on the left side of each graph), disease states (the different diseases addressed, middle part of each graph) and sample source (on the right side of each graph) obtained by spectrometry methods. A specific color palette has been selected for each graph, according to (a) chemical families of VOCs and (b) sample source. Each figure is constructed from all the identified VOCs, while each line represents a single VOC. The more the lines the wider the occurrence of a specific VOC, which could also suggest that it was more thoroughly researched in the studies. | |
 |
| Fig. 3 Relationships between chemical families (on the left side of each graph), disease states (the different diseases addressed, middle part of each graph) and sample source (on the right side of each graph) obtained by spectrometry methods. A specific color palette has been selected for each graph, according to (a) all disease states and (b) malignant and non-malignant diseases. Each figure is constructed from all the identified VOCs, while each line represents a single VOC. The more the lines the wider the occurrence of a specific VOC, which could also suggest that it was more thoroughly researched in the studies. | |
Here are some examples of insights that can be deducted from the figures to improve sensing development for clinical use.
(1) Ester groups are frequently connected with fecal samples in gastrointestinal disease (Fig. 2a and 3b). Thus, in aiming to develop a sensing system for these diseases, it is preferable to focus on a semi-polar sensing layer that can react more strongly with esters that enhance sensitivity. Until recently, all studies that involved diagnosis of gastrointestinal diseases focused on fecal samples (Fig. 3a). Therefore, if such diseases are of interest, it would be preferable to design a sensor that is suitable for fecal samples, which needs to cope with the noisy VOC background interference that they are likely to possess.
(2) It would appear that malignant diseases (Fig. 3b) have been sampled from three main sources, namely breath, skin and in vitro culture. Therefore, to evaluate a proposed sensor system for detection of malignant disease, one should choose tentative biomarkers from either breath or skin.
(3) Halitosis is diagnosed only from breath, and most of the compounds are organosulfur, which means that a potential sensor system will require selective or semi-selective sensing layers for volatile sulfur compounds.
Development of disease diagnostic tools based on nanomaterials must be correlated with the potential pattern of VOCs reported in Fig. 2, 3 and Table S1 (ESI†). The following sections will introduce the VOC sensing abilities of many nanomaterial-based sensors, in both laboratory conditions and real clinical studies to determine those that are best in improving diagnostic accuracy.
4. Nanomaterial-based sensors for VOC detection
4.1. Metal oxide sensors
Semiconducting metal oxides (MOs) are broadly used in chemiresistors as a sensing layer for oxidizing or reducing gases; they usually operate at high temperatures (150–500 °C) compared to other semiconducting sensors.26–28 MOs also are used as sensing layers in field-effect transistor (FET) devices.29–31 MOs layers have a high affinity for negatively charged oxygen moieties (e.g. O2−, O−), which leads to the creation of either an electron-depletion or a hole-accumulation surface charge layer, according to the type of semiconductor, n-type and p-type, respectively.32 The gaseous phase reacts with the adsorbed negatively charged oxygen on the metal-oxide surface, altering the surface-trapped charge density, resulting in changes in resistance.32
MOs can be divided according to their electronic structures into 2 groups: transition-MOs (e.g. NiO, Cr2O3) and non-transition-MOs (e.g. ZnO, SnO2).33 Transition-MOs that have more than one preferred oxidation state are better suited for gas sensing applications.33 For sensitive detection at ppb levels, binary, ternary, quaternary or noble metal-decorated MOs are usually used.33,34 By controlling MO parameters – composition, size, shape, morphology, surface area, fabrication methods, doping levels, microstructures of sensing layers, humidity and operating temperature – their sensitivity and selectivity performance can be tuned to detect gases of interest under real-world conditions.27,34–49 Experimentally it has been found that as the particle size decreased for impurity-doped SnO2 and WO3 nanoparticles, the sensor's response towards H2, CO, and i-C4H10 or NO2 and NO improved, respectively.27 Furthermore, sensors with grain sizes less than twice the thickness of the surface charge layer were significantly more sensitive to the gases tested.44,45 Shape, morphology and surface area are also critical parameters for sensitivity enhancement of MO-based gas sensors, since they determine the number of atoms located at the edges of the nanocrystal structure.45,46 Pristine WO3 nanotubes (NTs) have a high and selective response to 5 ppm NO at 90% RH and 350 °C, and a small response towards toluene at the same concentration, whereas Pt- and Pd-loaded WO3 NT sensors have the opposite trend of responses to NO and toluene at 400 °C (Fig. 4).47 In order to increase the surface area to increase reaction sites, methods such as electrospinning are used for the generation of thin films of porous structures.45,50–52 Jin et al.46 compared the influence of various morphologies, synthesis routes, surface area and operating temperature on the performance of SnO2 MO-based sensors to ethanol exposure.46 In brief, SnO2 MO-based sensors with a large surface area gave stronger responses, whereas the operating temperature and morphology had less influence on their sensing performance.
 |
| Fig. 4 (a) Dynamic response of pristine WO3 NTs, Pt–WO3 NTs, and Pd–WO3NTs to NO in the range of 1–5 ppm at 350 °C, (b) temperature dependent NO response characteristic at 5 ppm in the range of 300–450 °C, (c) dynamic resistance transients of pristine WO3 NTs, Pt–WO3 NTs, and Pd–WO3 NTs to toluene in the range of 1–5 ppm at 400 °C, (d) temperature dependent toluene response characteristic at 5 ppm in the range of 300–450 °C. Reprinted from ref. 47 with permission from Elsevier, copyright 2016. | |
The use of MO-based gas sensors is limited by the ability to sense the gaseous phase of oxidizing or reducing species that can be both polar and nonpolar compounds. Such sensors are usually restricted for selective detection of a single gas of interest. The humidity dependence of the sensing properties emphasizes the biggest challenge for integration of sensors into real-world diagnostics, especially for exhaled breath as it contains at least 80% relative humidity (RH) under ambient conditions. Water adsorption reduces the sensitivity of MOs due to the prevention of electron donation to the surface charge layer, mostly for n-type MOs.46,48,53 The dependence mechanism might be explained by 2 models that describe the interaction between water vapor and oxygen found on the MO surface, as detailed elsewhere.54,55 Further investigation of these mechanisms will be critical for obtaining the underlying knowledge of how to generate humidity independence. Continuous exposure to humidity leads to the gradual formation of surface hydroxyls, leading to a progressive degradation that can be reversed by heating to temperatures >400 °C.45,56 Addition of surface agents with high affinity to water assists in trapping the water vapor and reduces the interaction with the sensing layer. CuO,48 NiO,57 Pd,58 Sb59 and CeO260 are potential absorbents to be integrated into MO sensors. Fig. 5 presents responses of MOs based on Ce–In2O3 to acetone at different RH levels.60 Optimization of the operating temperature must be balanced between the elimination of the humidity effects, magnitude of response, and recovery time, to the gas being investigated. Due to elevated operation temperatures, MOs – in comparison to other gas sensors – suffer from an inability to attach organic molecules or receptors to the sensor's surface in order to increase the cross-reactive selectivity to the gaseous phase.36 High operation temperature is a serious obstacle for room-temperature applications and for portable applications, although it has the advantage of rapid reversible recovery.27,36 However, detection of different gases has been possible by shifting the operation temperature.43,47,61,62 Even though some detrimental performance issues of MO sensors remain, due to wide investment of effort in tailoring the sensing behavior of MO sensors, they have been reported in the literature for successful detection and quantification of several single gases associated with potential diagnostic applications. It should be noted, however, that most of these studies were conducted in a dry atmosphere that does not mimic real-world conditions in which detection of health associated gaseous biomarkers is required. Furthermore, almost all the reports on sensors based on MOs for gas detection refer to a rigid substrate and only a few studies have presented flexible sensors for gas sensing purposes.62,63 To provide more specific details on the application of MO sensors for detection of VOC biomarkers, a general summary follows:
 |
| Fig. 5 Gas sensing transients of (a) pure, (b) 1.04, (c) 2.33, (d) 4.97, (e) 11.7, (f), 22.4, and (g) 39.9 Ce–In2O3 hollow spheres to 20 ppm acetone at 450 °C under dry and humid conditions (RH = 20, 50, and 80%). Reprinted from ref. 60 with permission from Wiley-VCH, copyright 2016. | |
4.1.1. Nitric oxide detection.
Nitric oxide (NO) is associated with asthmatic disorders, and is now being considered a potential diagnostic biomarker in exhaled breath.64,65 According to the literature, asthma patients exhale above 30 ppb NO, whereas healthy population exhales lower concentrations.65 Several studies have evaluated the performance of MO gas sensors regarding either NO or NO2, their response to both gases being similar. Moon et al.66 found high responses to 200–1000 ppb NO at 80% RH with villi-like WO3 nanostructures at 200 °C. This sensor had high selectivity for the NOx of interest by its high sensitivity to NO and NO2 with a detection limit of 88 ppt, whereas it responds negligibly to ethanol, ammonia, acetone and carbon monoxide.
4.1.2. Ammonia detection.
Ammonia is a potential biomarker in exhaled breath for renal, liver diseases and Helicobacter pylori infection.67–70 For end-stage renal disease patients, the concentration of NH3 ranges from 820 to 14
700 ppb, being lower for healthy volunteers.68 The majority of the studies reported high and selective responses under dry atmospheric conditions to ammonia at from 50 ppb to 4000 ppm compared to other gases at 200–500 °C operating temperatures.62,71–77 The majority of these sensors are based on MoO362,71–73,77 and WO3 with different doping,74–76 which are acidic oxides. Thus, acid–base interactions may explain the high NH3 responses as this is a basic gas. Güntner et al.78 reported on the operation of Si-doped α-MoO3 at 90% relative humidity that gave a selective response to 400 ppb NH3 at 400 °C.
4.1.3. Acetone detection.
Acetone is considered a potential biomarker for monitoring diabetes,79–82 with 1.8 ppm given as the lower concentration of acetone for diabetes patients, whereas the concentration <0.8 ppm was reported for healthy people.82 MOs have been investigated, to a large degree, for the selective detection of acetone in high humid atmospheres (>80% RH).52,60,83–97 The majority were based on catalyst-loaded WO383–88,91,98 and SnO252,89 for 0.1–5 ppm acetone concentrations at 250–450 °C. Righettoni et al.86 reported at least a 40% difference in 10 mol% Si-doped WO3-based sensor response between correlated acetone concentrations of healthy and diabetes subjects (Fig. 6). In a follow-up study, they assessed the performance of the sensors on 5 healthy volunteers, in whom they were able to detect acetone levels of 880–970 ppb.93 Choi et al.83 explored long-term stability tests of Pt-functionalized WO3 hemitubes, which, after 7 months in air, showed a decrease of 6% of the response to 2 ppm acetone at 300 °C. Functionalized WO3 hemitubes were used to sense acetone down to a detection limit of 120 ppb at 300 °C operating temperature.94 In 2 newer studies, the same group used the same semiconductor material to prepare metal functionalized mesoporous WO3 nanofibers.84,85 A catalytic nanoparticle was encapsulated into apoferritin. The functionalized proteins were then incorporated into the nanofibers to achieve a sensing layer. They estimated that the complex detection limit of acetone was 0.54 ppb by linear approximation of the data.85 The same concept was also investigated using SnO2 nanotubes that achieved a 10 ppb detection limit.52 Yet the latter sensor studies were only evaluated on pure standards, not in real-world breath samples, and their efficiency must be proven in the clinical scene. The most recent studies have explored Pd-loaded ZnO/ZnCo2O4 hollow spheres, giving 400 ppb detection;95 RuO2-loaded WO3 nanofibers with 100 ppb acetone detection;91 Rh2O3-loaded WO3 nanofibers giving 1–5 ppm,96 and PdO-loaded Co3O4 hollow nanocages with a calculated detection limit of 100 ppb.92 Sensors based on a single Fe2O3 nanowire evaluated for acetone detection achieved a 20 ppb detection limit for a nanowire of 25 nm diameter.97 However, it is noteworthy that many MO devices fabricated for acetone sensing are similarly sensitive to ethanol, which can hamper clinical breath applications.99 Recently, hollow In2O3 spheres decorated with CeO2-based sensors showed excellent very similar responses to 20 ppm of acetone under both dry and 80% RH conditions.60 Nevertheless, humidity remains a huge challenge for sensing acetone concentrations under real-life conditions.
 |
| Fig. 6 TEM images of flame-made (a) pure and (b) 10 mol% Si-doped WO3 nanoparticles collected downstream of the sensor substrate. (c) SEM image of a pure WO3 film directly deposited onto the sensor substrate after in situ annealing. (d) Sensor response for acetone detection (0–3 ppm) of a 10 mol% Si-doped WO3 sensor at 90% RH and 400 °C. Diabetic patients (1.8 ppm) can be clearly distinguished from healthy humans (0.9 ppm) by at least a 40% difference in sensor response. (e) WO3-Based sensor response to 600 ppb acetone at different RHs and doping levels as a function of Si-content (400 °C). Reprinted with permission from ref. 86. Copyright (2010) American Chemical Society. | |
4.1.4. Ethanol detection.
Similar responses were obtained from most of the MO sensors after exposure to acetone and ethanol,100,101 due to a similar intermediate product during oxidative sensing reactions of both compounds.102 Selectivity enhancement towards ethanol can be reached by adding a basic metal oxide, such as La2O3, to the sensor structure.102–104 On the other hand, acidic oxides are advantageous for increasing sensor selectivity to acetone. Selective detection of 10–500 ppm ethanol, mainly by α-Fe2O3-based sensors, has been explored103,105–107 under dry conditions and at temperatures from 240 to 350 °C. Shifting the operational temperature is another possibility for increasing the sensitivity to both acetone and ethanol, even in the presence of high humidity.53 A typical example to detect ethanol is by using ZnO nanorods and graphene hybrid architectures on a flexible substrate of stainless steel foils.63 Besides a desirable sensing response to 10–50 ppm ethanol at 300 °C in dry air, stability was particularly emphasized for this sensor. For instance, multiple (up to 100 times) bending tests showed that this hybrid architecture allowed deformation without electrical or mechanical failure for bending radius of <0.8 cm.
4.1.5. Hydrogen sulfide detection.
Detection of H2S is potentially important for diagnosis of halitosis.108,109 CuO-,43,110–121 MoO3-62,122–124 and Ag-125–128 based oxide sensors are most commonly used for such sensing application under dry or moderately humid conditions. Many of these sensors can be operated at low operating temperatures, even down to room temperature.110,111,114,122 CuO hollow spheres with hierarchical pores112 and CuO nanosheets113 were investigated for selective detection of 30–1200 ppb H2S at higher operating temperatures (190–240 °C) with a short response time and strong recovery abilities. A detection limit of 2 ppb was quoted in both studies.112,113 However, it was reported that a 3-month stability test of gas sensors based on CuO nanosheets yielded a constant response.113 Continuous exposure to H2S at low temperature might lead to the problem of modifying CuO to CuS; hence CuO-based sensor's recovery at low operating temperatures was obtained by pulse heating114 to reverse the oxidation of CuO to CuS, whereas this action was unnecessary at high temperature.114,115 In addition to the high sensitivity and low detection limit, Choi et al.48 reported that CuO-loaded hollow SnO2 spheres had excellent selective responses to 0.2–1 ppm H2S, with negligible dependence on the high humidity level (80% RH) at 300 °C. In contrast to CuO-based sensors, MoO3 is a nonreactive adsorption compound, such as MoO3·SH2, which has high reversibility at 70–300 °C.62,122,129 Li et al.62 reported on a flexible MoO3 nanopaper-based sensor for the detection of several gases, including H2S. When operated under dry conditions and at 250 °C, the sensor had a high selective response to 0.25–5 ppm H2S. The sensor became more selective towards NH3 after altering the operational temperature.
4.1.6. Detection of benzene ring-based (aromatic) VOCs.
Detection of less-reactive long chains (C4–C10) or benzene ring-based VOCs, related to different diseases, by a single selective MO-based sensor continues to pose many challenges. Some progress towards detection of benzene ring VOCs was achieved with p-type MOs of high catalytic activities; however, this was only under dry conditions.61,130–141 High selectivity was found with a Pd-loaded SnO2 yolk–shell sensing film coated with a thin catalytic Co3O4 overlayer by exposing to benzene and other indoor pollutants, such as toluene, xylene and ethanol.130 The sensor responded quickly to 5 ppm benzene and had negligibly low cross-responses to other indoor pollutants at 375–475 °C and dry air. Uncoated and coated (with a thin zeolitic imidazolate framework; ZIF-8) molecular sieve membrane ZnO nanowires were examined as gas sensors for detecting 10–30 ppm H2, toluene and benzene. The uncoated ZnO nanowires were markedly sensitive to toluene and benzene, whereas the coated sensors showed the highest selectivity to H2.142
4.1.7. Metal oxide-based nanoarrays.
MO-Based nanoarrays are useful for detecting gaseous mixtures with different properties, like real VOC patterns, which are associated with a variety of diseases.143–145 For example, 4 Pt-, Si-, Pd-, and Ti-doped SnO2 films were used for detecting formaldehyde in a gaseous mixture of acetone, ethanol and NH3 with 90% RH, mimicking real human breath. The detection limit of the array was 3 ppb formaldehyde at 400 °C, with low response times. A single sensor cannot detect and quantify formaldehyde at low concentration (0–180 ppb) in a humid gas mixture. Thus, the formaldehyde range of the 4 sensors was estimated accurately by multivariate linear regression, with an average error of ≤9 ppb in up to 4-analyte mixtures (Fig. 7).143 Moon et al.145 described a nanoarray of 9 MO-based sensors, including thin films, Au-functionalized thin films and villi-like nanostructures. The nanoarray was exposed to different gases at 80% RH. The array had enhanced sensitivity in the detection of H2S, NH3 and NO, with detection ranges of 534 ppt–2.87 ppb, 44542.29 ppb, and 206 ppt–2.06 ppb, respectively. Principal component analysis (PCA) strengthened the discriminatory abilities of the nanoarray.145
 |
| Fig. 7 (a) A microsensor substrate features a circular-shaped sensing area with a set of interdigitated Pt electrodes. This is surrounded by a meandered Pt electrode serving as a heater and a resistance temperature detector. (b and c) Flame-made sensing particles are accurately deposited by thermophoresis on such substrate packages (5 × 4 microsensors) using a shadow mask. (d) After package dicing, Pt:, Si:, Pd: and Ti:SnO2 microsensors are combined as an array (E-nose) and wire-bonded on a chip carrier. Suspending the sensors minimizes thermal losses and ensures a uniform temperature distribution. (e) E-nose estimation of formaldehyde at 90% RH and in breath-relevant (a) 3- (squares) and (b) 4-analyte mixtures (circles) consisting of formaldehyde (30–180 ppb), acetone (50–1800 ppb), NH3 (250–2000 ppb), and ethanol (50–600 ppb). Formaldehyde levels are estimated accurately with low error, εavg = 6 and 9 ppb, despite the significantly higher interferent gas concentrations. Symbols and error bars indicate the estimated average and the variability of different interferent levels. Reprinted with permission from ref. 143. Copyright (2016) American Chemical Society. | |
4.2. Chemiresistors based on monolayer-capped metal nanoparticles
Chemiresistors based on monolayer-coated metal nanoparticles (MCMNPs) are widely used for gas sensing due to their valuable advantages, including: large surface-to-volume ratio, low-output impedance, room temperature operation, (sub-)ppb detection limits for the VOCs of interest, low voltage operation, a wide dynamic range, fast response and recovery times, tolerance to humidity, the possibility of being deposited on both solid state and flexible substrates, and compatibility with the standard microelectronic industry.14,146–151 There are many possible fabrication methods for both solid-state and flexible sensors based on MCMNPs, including drop-casting,152–155 layer-by-layer (LbL) deposition,151,155–157 spraying,158,159 spin-casting,160 printing,161–164 self-assembly methods (including drain to-deposit165) and others.166–173 Selecting the fabrication method has a critical impact on the sensor properties. Bashouti et al.155 compared the performance of sensors prepared by 2 fabrication methods: drop casting and LbL (Fig. 8). Using the LbL method, the film was more homogeneous, and gradually increased in thickness and conductivity compared to the film prepared by the drop-casting method.155 The sensors were exposed to polar and nonpolar VOCs associated with health monitoring, including hexane, ethyl benzene, water and ethanol at p/p0 = 0.5 (p and p0 are the partial pressures of the VOC and the VOC vapor pressure, respectively). Interestingly, sensors prepared by the drop-casting method had a higher sensitivity compared to sensors prepared by the other method.155 Sensors fabricated using LbL methods may be more applicable to high-pressure sensing, where stability and reproducibility are mandatory.155
 |
| Fig. 8 Normalized resistance, ΔR/Rb, of MNPs prepared by LbL and DC methods. Upon exposure, hexane, ethylbenzene, water and ethanol in the vapor phase at pa/p0 = 0.5. (a) Shows both responses on the same scale bar, whereas (b) shows only the response of the LbL device. Reprinted with permission from ref. 155. Copyright (2014) American Chemical Society. | |
Selection of the substrate properties, especially when a flexible substrate is involved, provides additional opportunities to custom-made sensors with the desired flexibility, stability and sensing performance.154,156,174,175 Segev-Bar et al.174 reported on the contradictory effect between thickness and load sensitivity of the flexible substrate owing to bending and stretching. Direct control of the chemical and physical properties of nanoparticles (NPs) during synthesis allows significant diversity, and accurate tailoring of the sensing properties, such as sensitivity, selectivity and response time to the desired application.176 Among these parameters are inorganic particle type (i.e. pure metals, metal alloys, semiconducting materials),177–179 particle shape (i.e. sphere, cube, star, rod),146,180–184 and size (1–100 nm).185 Such control achieves nearly uniform nano-pores in the composite films.146,150,176,183,184 In addition, the sensing layer composition (i.e. alkylthiols, dialkyl disulfides, DNA, proteins, alkanethiols, and others) is critical, and numerous reports have been published on this topic.150,176,186–189 Another critical factor is film morphology, which affects sensing properties. The effect of morphology can be divided into several action-based types:148,190 (i) individual and/or clusters of MCMNPs located at a considerable distance from one another, and, as a result, contribute to resistance changes; (ii) island morphology that permits conductivity due to formation of a single dimensional percolation pathway and/or reduction of the island-to-island distance, and permittivity changes as a results of swelling; (iii) continuous 3-dimensional morphology with numerous percolation pathways,148,190 and (iv) perforated films that involve 2 simultaneous mechanisms of (a) ionic conduction on the SiO2 in the domains free from MCMNPs, and (b) tunneling conduction within the MCMNP domains.190
A change in the resistivity of the chemiresistor, during exposure to a vaporous phase, can be affected by one or a combination of the known mechanisms related to the activated tunneling model:149,176 (i) a steric change within the nanomaterial film, such as reversible swelling or aggregation, causing the interparticle distance to increase or decrease, thereby affecting the electrons' tunneling properties,17,25,36,149 and (ii) changes in the dielectric constant of the organic layer due to charge transfer from/to the layer once exposed to VOCs, especially if the dielectric constants of the sensing layer and the absorbed VOC differ significantly.25,183,191,192 Exposure to absorbed VOCs with low dielectric constants (e.g., toluene and n-hexane) results in decreased permittivity of the organic matrix, causing increased energy of tunneling activation, thereby leading to an increase in resistivity.192 In a similar manner, VOCs with high dielectric constants reduce the sensor's resistivity. MCMNPs can be integrated into single variable sensors, such as chemiresistors, or into multivariable sensors, such as resonant or non-resonant multivariable electrical sensors and material- or structure-based multivariable photonic sensors. More details about multivariable sensors have been described elsewhere.188
4.2.1. Solid state sensors based on monolayer-capped metal nanoparticles.
Many studies have been conducted to elucidate the sensing mechanism of solid-state chemiresistors based on MCMNPs.152,166,193–196 García-Berríos et al.193 investigated the response to various VOCs of sensors capped with a series of straight-chain alkanethiols, including 1-butanethiol, 1-pentanethiol, 1-hexanethiol and others. The VOCs explored that are associated with health conditions included hexane, heptane, n-octane, iso-octane, cyclohexane, toluene, ethyl acetate, methanol, ethanol, isopropanol and 1-butanol at room temperature and under dry conditions.193 The sensors gave rapid and reversible responses, and the resistance response sensitivity to hydrocarbons increased with the length of the capping alkanethiols, whereas with alcohols, negative sensitivities were measured as the alkanethiol length increased.193 For hydrocarbons and polar analytes, a dual response mechanism was observed, whereas the dielectric constant of the alcohol vapors was not weighty for the sensing mechanism compared to the steric effect.193 A more comprehensive study was later undertaken by measuring the responses of sensors to 20 different capping ligands to 13 VOCs.152 The capping ligands were divided into 4 groups: straight-chain alkanethiols (i.e. 1-butanethiol, 1-pentanethiol, 1-hexanethiol), branched alkanethiols (i.e. 2-methyl-1-propanethiol, 2-methyl-1-butanethiolm 3-methyl-1-butanethiol), aromatic thiols (i.e. 1-phenylthiol, 1-naphthalenethiol, 4-biphenylthiol) and composites of 2-phenylethanethiol-capped Au NPs with nonpolymeric aromatic materials (2-phenylethanethiol alone and with naphthalene, biphenyl, anthracene, or terphenyl).152 The VOCs included the following compounds at different concentrations and dry conditions: hexane, heptane, n-octane, iso-octane, cyclohexane, toluene, chloroform, tetrahydrofuran, ethyl acetate, methanol, ethanol, isopropanol and 1-butanol.152 As expected, the sensing responses for the straight-chain alkanethiol-capped Au-NPs affirmed the expected trends previously reported. According to the data for the branched alkanethiol-capped Au-NPs, the dual response mechanism was not shown, probably due to surface defects.152 Nevertheless, as the chain length increased, the average resistance-based sensitivities also increased. Sensors with aromatic thiols had higher selectivity to polar vapors due to the π-stacked islands that allowed for voids to polar analyte sorption.152 By applying PCA and Fisher's linear discriminant as pattern recognition algorithms, the sensor array gave an accurate classification of most vapors, except for those with similar polarity.152
As the MCMNP-based sensors are not specific and perform as cross-reactive sensors, each can be exposed to a variety of VOCs of interest in conjugation with pattern recognition algorithms distinguishing between them. Hubble et al.153 screened 132 different capping ligands based on thiols as the sensing layer for Au NP-based chemiresistors for the purpose of discrimination and quantitation of benzene, toluene, ethylbenzene, p-xylene, and naphthalene (BTEXN) mixtures at ppm levels in water. The sensors were screened on their baseline resistance values and the relative response to the BTEXN mixtures at 5 ppm.153 Combination of the sensor array data with random forests analysis also verified the predictive abilities for each individual component concentrations of 100 ppm in water in a mixture.153 Joseph et al.151 reported on the sensing performance of Pt- and Au-NPs functionalized with 1,9-nonanedithiol (NDT) and dodecylamine, to NH3, CO, toluene and vapors of water in the range from 300 ppb to 5000 ppm. Increased resistivity was seen with all the sensors upon exposure to VOCs. The sensitivity of the Pt NPs film was 2 to 8 times higher than that of the Au NP film, and gave a detection limit for NH3 of <100 ppb.151 Furthermore, response dynamics indicated that NH3 and CO bind significantly more strongly to the film compared to water and toluene.151 In another study, 3.5 nm Au NPs functionalized with 4-methylbenzenethiol, 1-hexanethiol and 1-dodecanethiol were examined upon exposure to NO2 gas and toluene vapors.197 Detection limits for NO2 were 0.5 ppm and 8.8 ppt toluene using a 4-methylbenzenethiol functionalized NP film at 22 °C.197 Detection of CO2 by NP-based chemiresistors was also reported.198 The conductivity of the unlinked film increased by up to 31 fold under increasing CO2 pressure (0–6.6 MPa) at room temperature, whereas it decreased with increasing CO2 pressure for the linked films with different ligands.198
The influence of humidity was also investigated by exposing the sensors to realistic humidity levels mimicking those of actual breath and skin samples. Dovgolevsky et al.183 examined the responses of cubic Pt NPs capped with oleylamine (ODA), 11-mercaptoundecanol, 11-mercaptoundecanoic acid, and benzylmercaptan to VOCs, including hexane, octane, decane, ethyl benzene, ethanol and water at p/p0 = 0.01–0.5 under humid conditions. Functionalized NPs with nonpolar ligands had a high response to nonpolar VOCs and a low sensitivity to polar ones, especially water.183 Moreover, sensing capabilities were shown for nonpolar analytes in high humidity environments by exposing an ODA-capped cubic Pt NP sensor to binary mixtures of low concentration octane and realistic humidity levels.183 Single-stranded DNA-functionalized Au NP-based sensors have also been reported.189 These sensors were exposed to ethanol, methanol, hexane, dimethyl methyl-phosphonate and toluene at p/p0 = 0.036 concentration under different relative humidity conditions at room temperature.189 Exposure to increasing level of humidity resulted in a non-monotonic resistance change that can be explained by the combined effects of hydration induced swelling and ionic conduction.189 Strong influence of humidity was also observed upon exposures to humid vapors.189 Homede et al.163 reported a novel printing technique termed self-propelled anti-pinning ink droplet that involves evaporative deposition during the path of motion. By changing the applied actuating forces, different deposition line patterns, thicknesses and morphologies were generated from a single droplet.163 The sensor array was based on Au NP-sensors capped with 1-hexanethiol, 1-dodecanethiol and 1-ocatadecanethiol prepared by either μ-revulet or spreading patterns.163,199 The array was exposed to 0–90% RH and a variety of VOCs, including 1-octanol, 1-hexanol, ethanol, decane, hexane, octane, dibutylether and mesitylene at 100–1000 ppb for each. Pattern recognition by discriminant function analysis (DFA) showed a respectable discrimination between the VOCs at all tested RH levels, including the 90% RH level that mimics real breath and skin samples (Fig. 9).163 Moreover, the results accurately quantified VOC concentrations.163 Such results indicate the vast potential of the novel printed NPs as a cost-effective technique (i.e., without the need for expensive printing equipment) for integration into multifunctional devices for health diagnosis and monitoring. Broza et al.200 and Karban et al.201 showed in their studies the capability of an array of MCMNPs in discriminating between simulants of multiple sclerosis (MS) and health, or between different bowel diseases, respectively.
 |
| Fig. 9 (a) DFA plots showing the separation between the different VOCs achieved by the sensor array at the 3 humidity levels: (I) 0, (II) 30, and (III) 90%. The arrow directions in (I) indicates increasing concentration of each VOC. (b) Discrimination accuracy between each pair of VOCs achieved by the chemisensor array at the 3 different humidity levels, i.e., (I)–(III) as above. Each square indicates the accuracy of separation between the VOCs from the x-axis and the y-axis. Reprinted from ref. 163 with permission from Wiley-VCH, copyright 2016. | |
4.2.2. Flexible sensors based on monolayer-capped metal nanoparticles.
Chemiresistors based on 4 nm (in diameter) Au NPs capped with NDT and pentaerythritol tetrakis(3-mercaptopropionate) on a flexible high-density polyethylene substrate were tested for detection of toluene, water, 1-propanol and 4-methyl-2-pentanone at from 50 to 10
000 ppm.157 The tested VOCs had similar vapor pressures, but different polarities.192 The sensors had higher sensitivity (∼30%) to these VOCs during a room temperature operation when 1% tensile strain was applied compared to the absence strain.157 Shan et al.202 investigated the sensing mechanism of Au NPs capped with decanethiol and NDT on flexible polyethylene terephthalate (PET) as a function of strain and its direction. The sensors were exposed to hexane (826–3300 ppm), ethanol (312–1300 ppm) and acetone (1300–5000 ppm) under ambient conditions and different radii of curvature of concave or convex strains.202 Response sensitivity analysis showed that the relative changes in response to a particular vapor were ruled by its nature and the sorption, and less by the applied strain.202 The influence of ligand length as well as the bending state was also elucidated for VOC sensing.154 Flexible sensors based on Au NPs were capped with alkanethiols, including 1-butanthiol, 1-hexanethiol, 1-decanethiol and 1-octadecanethiol, and were deposited on a Kapton substrate.154 These sensors were exposed to different VOCs at 3 bending states, including flat. The tested VOCs included compounds associated with health: cyclohexanol, octanol, decanol, mesitylene, octane and decane at increasing concentrations (p/p0 = 0.01, 0.1, 0.5). Combination of all the bending states increased the discriminatory ability to separate different VOCs in PCA analysis compared to a single bending state.154 The same report also included Au NPs capped with 1-hexanethiol sensors that were bent to radii of 0.21, 0.26 and 0.28 mm−1 as stretched and compressed deformations.154 These sensors were exposed to VOCs, including hexane, octane, toluene, hexanal, octanol, water and 2-ethylhexanol, at static bending states. PCA analysis, based on the sensing properties, showed an excellent separation between polar and non-polar VOCs, and between the individual VOCs. Furthermore, analysis indicated an orthogonal classification based on the bending groups (Fig. 10). Such a conclusion highlights the ability of a single sensor with multi-parametric sensing to identify the deformation status and its directions even in complex environments as well as the VOCs.154 Kahn et al.156 tested Au NP-based sensors capped with 2-nitro-4-flouromethylbenzenethiol, 2-naphthalenethiol, 4-chlorobenzenemethanethiol, 3-ethoxythiophenol and 4-tertbutylbenzenethiol on a flexible Kapton substrate. They were evaluated under the mutual effect of different strains, both dynamic and static, along with simulated VOC samples and real breath samples collected from ovarian cancer (OC) patients. The simulated VOC samples included both volatolomics linked with OC, such as styrene and nonanal, and possible confounding factors, such as ethanol and propioaniline at from 50 to 1000 ppb.156,203 The results show that the VOC-dependent strain response can be sensitive to low concentrations – down to 50 ppb – thus showing the potential for diagnostic applications using exhaled breath.156
 |
| Fig. 10 (a) Illustration of Au-MCNPs deposited on a flexible substrate while been bent to bending radii of 0.21, 0.26 and 0.28 mm−1. PCA plot based on 2 features extracted from the responses of Au-MCNP based sensors to 1-hexanethiol as the encapsulating ligand in response to 7 VOCs. (b) Orthogonal pattern displayed for stretching (Bend+), compressing (Bend−) and flat (Bend0) conditions of flexible sensors. (c) Polar vs. non-polar separation. (d) Specific VOC separation. Reprinted from ref. 154 with permission from Wiley-VCH, copyright 2017. | |
Paper-based sensors have also been reported.164 Two nm Au NPs capped with 11-mercaptoundecanoic acid were printed by aerosol jet printing on a 2-layer paper membrane of polyacrylonitrile (PAN)/PET with Ag electrodes.164 The paper-based sensor was exposed to ethanol at 200–800 ppm and 1-propanol at 50–275 ppm. In addition, methanol, benzene, acetone, hexane and 1-butanol were tested, but no data at the tested concentration were provided. The responses of the paper-based sensors were also compared to sensors based on a polyimide substrate, which is considered a 2D-nonporous substrate. The response sensitivity was higher, for almost all the tested VOCs, for the paper-based sensor compared to the polyimide-based sensor.164 The sensor was also exposed to human breath and responded to inhalation and exhalation.164
Self-healable sensors based on Au NPs for detecting VOC in the skin and breath were also reported.204 The sensor array included 3-ethoxythiophenol, benzylmercaptan, tert-dodecanethiol, hexanethiol, and decanethiol-functionalized NP films.204 The flexible NP-based sensor was built with 3 layers: a self-healable polymer substrate from self-healing disulfide cross-linked polyurethane (sh-crl-PU),205 a self-healable silver-polymer (μAg-polyurethane diol) composite (sh-μAgPU) and the sensing layer based on NP films.204 All the tested VOCs were reported as being emitted from breath and the skin, thus highlighting their importance for healthcare and diagnostics. This list included 2-hexanone, acetic acid, heptane, hexanal, hexane, 3-heptanone, heptanoic acid, hexanoic acid, heptanal, nonanal and nonane.204 The sensors had excellent healing properties at room temperature, had a fast self-healing within 3 h of being scratched.204 In addition, these self-healing sensors gave ultra-detection (limit of detection ranged at the ppb level) and classification of health-related VOCs by being combined with pattern recognition methods, both before and after scratching on to the fully healed state (Fig. 11).204 Nevertheless, all the tested VOCs were exposed under dry conditions, and the effect of humidity associated with real samples205,206 remains to be evaluated.
 |
| Fig. 11 PCA schemes of the data-set for the sensor array consisting of (a) 5 different sensor parts; (b) benzylmercaptan-, hexanethiol-, and decanethiol-functionalized GNP films; (c) tert-dodecanethiol-, hexanethiol-, and decanethiol-functionalized GNP films before scratching and after full healing. After manually scratching and complete healing, the sensor array retained its performance in sensing of VOCs within a satisfactory range. A desirable discrimination feature was also found after the sensor array had fully healed. Reprinted with permission from ref. 204. Copyright (2016) American Chemical Society. | |
The effect of humidity on sensor performance was also studied.154 Segev-Bar et al.154 reported sensing properties of Au NPs on a flexible Kapton substrate with (i) different capping layers including 1-hexanethiol, 1-decanethiol, 1-dodecanethiol, 1-octadecanethiol and 1-naphthalenethiol, and (ii) a set of Au NPs capped with 1-naphthalenethiol with different core diameters of 5, 10, 20 and 50 nm. Measurements were taken at a constant room temperature without applied strain and in the range from 5 to 30% RH.154 Response times for all the sensors were <20 seconds and there was only a minor drift with time.154 The results showed a negative response to the tested levels at these RH% for all the sensors. Furthermore, longer capping alkanethiol chains gave higher responses.154 An additional observation regarding the influence of core diameter gave the highest negative response for the smaller core diameter. The change in response direction for 1-naphthalenethiol sensors with different Au NP diameters suggests the possibility of controlling the diameter to avoid any response to humidity. These findings are consistent with the changes in the dielectric properties of the film once exposed to water vapor with a significantly higher dielectric constant, and which decreases the activation energy for charge transport.154
4.3. Carbon nanotube-based sensors
Carbon nanotubes (CNTs) are another promising nanomaterials for a wide range of VOC detection applications. CNT fabrication methods include mostly laser ablation,207–210 arc-discharge,211–216 and chemical vapor deposition (CVD).217–220 These nanomaterials can be divided into 2 groups according to their structure, single-walled (SWCNTs) and multi-walled carbon nanotubes (MWCNTs). CNTs attract the attention of many researchers especially due to their size range, large surface-area-to-volume ratio, electrical and mechanical properties, and their compatibility with other nanomaterials for the generation of enhanced properties. The size ranges 0.8–2 nm for SWCNTs, 5–100 nm for MWCNTs and the length of vertically aligned (VA) CNTs varies from microscale to milliscale.221,222 MWCNTs have a metallic behavior with a current carrying capability of up to 109 A cm−2,223 whereas the SWCNTs have either metallic or semiconducting behavior according to the chiral angle between the hexagons and tube axis.224,225 Semiconducting CNTs have excellent electrical properties,226 including high electron mobility (100
000 cm2 V−1 s−1), field effect mobility (79
000 cm2 V−1 s−1),227 electrical conductivity (104 S cm−1)228 and a band gap of ∼5 eV with opposite correlation with the diameter.229 The thermal conductance and thermal conductivity for metallic SWCNTs of 2.6 μm length and a diameter of 1.7 nm at room temperature are approximately 2.4 nW K−1 and 3500 W m−1 K−1, respectively.230 For a single tube, the thermal conductivity can reach 3000 W A−1 K−1.231 Both CNTs have excellent mechanical properties and the tensile strength ranges between 11 and 63 GPa for MWCNTs232 and between 13 and 52 GPa for SWCNTs.233 An outstanding tensile strength of 100 GPa has been recorded for both MWCNTs and SWCNTs.234,235 Furthermore, the maximum bending strength for SWCNTs was 28.5 GPa, whereas the range for the MWCNTs was 14.2 to 80 GPa.236 Not many studies focus on exposure to gas at real-world RH levels, and the present results based mainly on dry conditions. When exposed to water vapor, the conductivity of CNT-based sensors can be changed from p-type to n-type by humidity sorption as the water vapors act as electron donors.237 Gas sensors based on pristine CNTs may have some limitations, such as low sensitivity due to low adsorption energy or low affinity of the tested gas, lack of selectivity or long recovery time. Functionalization of CNTs with various materials may enhance the sensing abilities and overcome these limitations; however, low detection of nonpolar VOCs and increased sensitivity to water are problematic and limit the detection of gases in breath samples. These major limitations can be explained by several effects.238 Haick's group proposed that non-polymeric organic materials can act as coating layers that overcome these limitations and could be integrated into online devices for breath monitoring.238–244
Pristine and modified CNTs can be integrated into field-effect transistors238,245–269 and chemiresistors239–244,246,270–304 on a solid-state configuration with low fabrication costs and power consumption.305–307
4.3.1. Solid state carbon nanotube-based field effect transistors.
Sensors based on CNTs were investigated as FET (CNTFET) devices for gas sensing applications in many studies,238,245–268 mostly focused on 2 gases, NO2 and NH3, representing oxidizing and reducing gases, respectively. Kong et al.249 reported CNTFETs based on a single SWCNT that was operated under room conditions for the detection of 2–200 ppm NO2 and NH3 at 0.1–1% in Ar/air. The sensors had a fast response, 2 to 10 seconds, and fast recovery only at operating temperature pulses of 200 °C.249 A similar study was conducted with CNTFETs based on a single SWCNT including a polymethylmethacrylate (PMMA) resist as a center-covered configuration, or contact-covered configuration, also tested under ambient conditions.250 The sensor was exposed to 0–4 ppm NO2 and 0–400 ppm NH3. As expected, VON increased for NO2 and was reduced for NH3 exposures.249 The presence of a PMMA resistor influenced the subthreshold swing. For the center-covered configuration, exposure to NO2 led to a lower subthreshold swing, but a higher subthreshold swing when exposed to NH3. Opposite trends were observed for the contact-covered configuration.250 Sorption of gas molecules on the CNTs/metal interface has also been reported, which mostly affects the characteristics, consistent with the Schottky barrier transistor model on exposure to 0–10
000 ppm NH3 and 0–200 ppm NO2 at room temperature.251–253
CNTFET based on multi-CWCNTs were reported as room operated sensors for detecting NO2,254–257 NH3,254,256,257 ethanol,254 benzene254 and NO.257 Chang et al.254 found CNTFETs sensitive to NO2 at 200–1500 ppm, NH3 at 30–500 ppm, ethanol at 1000 ppm and benzene at 15 ppm. As expected, the threshold voltage was shifted to the negative gate voltage for reducing gases, such as NH3, ethanol and benzene, whereas a positive shift occurred on exposure to NO2. Memory effects were also measured in different atmospheres (Fig. 12).254 Recovery of the device was achieved by simply an inverse gate voltage for 30 seconds, a negative voltage for recovery from NO2 exposure and a positive voltage for NH3 recovery. In this manner, no degradation of the device can occur.254 A poly(9,9-dioctylfluorene) derivative-based semiconducting-SWCNTs were used as the sensing layer for printed thin-film transistors (TFTs) for detecting NO2.255 Printed SWCNT TFTs had superior electrical performance with high on/off ratios (∼106), highly effective hole mobility (∼29.8 cm2 V−1 s−1), small hysteresis, and a small subthreshold swing (142–163 mV dec−1) at a low operating voltage of ±2 V.255 Furthermore, the sensor was exposed to 40–60 ppm NO2 at room temperature. The sensing responses were fast (30 seconds), with high sensitivity and good stability. A fast recovery time of 30 seconds was also achieved by integrating a heating resistor applied at 2.5 V on the back of the gas sensor.255 Similarly, a heating element was integrated into an array of 12 SWCNT-based CNTFET sensors exposed to NO2 and NH3 concentrations of 0.4–10 ppm and 4–50 ppm, respectively.256 Chen et al.257 found an enhanced detection limit of CNTFETs by using ultraviolet illumination at room temperature. Detection limits of 1.51 ± 0.32 ppt, 27.8 ± 6.2 ppt, and 590 ± 120 ppq (parts-per-quadrillion) for NO2, NH3 and NO, respectively, were reported.257 Moreover, by controlling the gate bias, depending on its gate-dependent I–V characteristic profile, it can determine the sensitivity of the sensor to the gas being tested.257 Although the sensitivity of Au/SWCNT to H2S was strongly dependent upon the size and number of gold nanoparticles, the sensing mechanism was independent of these parameters.
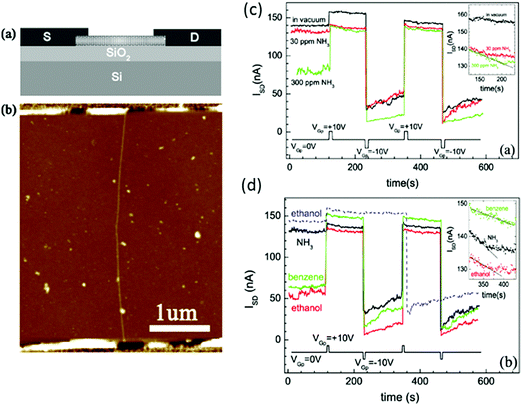 |
| Fig. 12 (a) Schematic diagram of the CNT-based gas sensor. A highly doped Si substrate served as a back gate. (b) AFM image of the sensor used in these experiments. Non-volatile memory effects measured in different atmospheres. (c) Vacuum, 30 ppm NH3, 300 ppm NH3; (d) 30 ppm NH3, 1000 ppm ethanol, 15 ppm benzene ISD is measured as a function of time with VSD = 50 mV and VG = 0 V, when VGp = 10 or −10 V is alternately applied. The dashed line in (b) shows ISD in 1000 ppm ethanol obtained from a different device. The insets show the temporal response from t = 120 to 240 s after applying VGp = 10 V under different conditions. Reprinted from ref. 254 with permission from IOP Publishing, copyright 2007. | |
Humidity effects on CNTFET that mimics real world conditions have also been investigated.258–261 Kim et al.259 reported on the humidity effects on CNTFET devices. Water molecules may shift the CNTFET's transfer characteristics as a function of gate voltage in terms of the hysteresis effect. The mechanisms for water trapping proposed were: (i) water molecules are weakly adsorbed onto the CNT surface and can easily be removed by applying a vacuum, and (ii) bonds creation between the water molecules and the SiO2 dielectric surface, proximal to the nanotube location. For eliminating undesired hysteresis, passivation of the devices with polymers that create hydrogen bonds with silanol groups, such as PMMA or octadecyltrichlorosilane, may be a solution.259,260
CNTFETs functionalized with other elements, such as metals,262–264 polymers265–267,269 and polycyclic aromatic hydrocarbons (PAH),239 were also investigated for enhanced sensing performance. CNTFETs decorated with metal NPs were reported for the detection of several gases and VOCs, including H2S at 500 ppm, NH3 at 200 ppm and NO2 at 5 ppm at room temperature.262 The authors explored 18 metallic NPs, including Mg, Pd, Pt, and Rh. A multivariate partial least-squares (PLS) regression model was also used to discriminate between the different gases. Mubeen et al.263 reported a Au/SWCNT-based FET device for detecting H2S at 2–1000 ppb. Au NPs act as nano-Schottky barriers and play a dominant part in hole mobility modulation by gas exposure.263 A correlation between the work function of the individual metal and the magnitude of electron transfer into the SWNT valence band has been reported in a study that explored the sensing properties of Pt, Pd, Au, and Ag NP decoration on the SWCNTs to 10 ppm of NO gas.264 Polymer/SWCNT-based CNTFET was also mentioned for increasing the sensitivity and selectivity to the tested gases.265–267 An et al.265 noted that modified CNTFETs with polypyrrole operated at room temperature for NO2 detection at 3000 ppm with n-type behavior. Functionalization with polyethyleneimine (PEI) resulted in ultra-sensing of NO2 down to 100 ppt and lack of sensitivity towards NH3, whereas functionalization with Nafion blocked NO2 and allowed selective sensing of NH3 down to 500 ppm.266 Recovery was achieved with UV light as in other studies.257,266,268 The influence of UV illumination on the polymer layer was not assessed. Functionalization of CNTFETs with specific DNA sequences for improving the sensitivity of the sensor in room operation has been published.267 After exposing the sensors to different vapors, including methanol and propionic acid, the authors found enhanced sensitivity compared to bare devices; this enhancement depended strongly on the DNA sequence used for a specific gas sensing.
Random networks of SWCNT FETs functionalized with tricosane (C23H48)239 and pentadecane (C15H32)/dioctyl phthalate (C24H38O4)239 were evaluated for detecting nonpolar VOCs associated with lung cancer, namely decane and 1,2,4-trimethylbenzene at p/p0 = 0.004 and room temperature.238 The pristine CNTFET had p-type behavior, as well as the C23H48/SWCNT FET. For the C15H32/C24H38O4/SWCNT sensor, n-type characteristics were revealed due to electron-rich functional groups.238 In addition, hysteresis in the transfer characteristics was significantly different between the devices. This has a significant role under humid conditions; for C23H48/SWCNT FET, an extended hysteresis occurred, indicating increased sensitivity to water molecules, whereas almost no hysteresis was seen for C15H32/C24H38O4/SWCNT FET and a decreased sensitivity to water molecules was expected.238
4.3.2. Solid state carbon nanotube-based chemiresistors.
CNT-based chemiresistors have also been thoroughly investigated as gas sensors.270–299 Pristine CNT-based chemiresistors were well characterized and exposed to several VOCs associated with health monitoring, including NO2,270–273 NH3274 and ethanol275 at room temperature. Li et al.270 obtained linear responses to 54–162 ppm NO2 and a detection limit of 44 ppb. Very slow recovery rates, due to high bonding energy between CNTs and NO2, were dramatically reduced by UV illumination. In addition, sensitivity variation was <6% for all of the devices tested.270 Electrokinetic fabrication was also reported for unmodified VA-CNT-based sensors for controlling trapped amounts of CNTs.271 Both MWCNTs and SWCNTs were fabricated and their initial conductance was proportional to the amount of trapped CNTs. The sensors were exposed to NO2 at 1–10 ppm, had a slow response time (∼50 minutes) and the relative conductance change of the sensors to NO2 exposure increased proportionally with the initial conductance of the sensors. Furthermore, SWCNT-based sensors had 2–6 times higher normalized sensitivity than those that were MWCNT-based, probably due to the greater abundance of the semiconducting tubes being correlated with the sensor's response.271 A VA-CNT-based sensor with a response time of <1 min was exposed to 0.5–1000 ppm NH3,274 but no details about the temperature of operation and the recovery process were provided. A recent comparison between a SWCNT random network and a SWCNT aligned network was reported for NO2 detection in the range of 0.5 to 20 ppm.272 Detection limits were 125 ppt and 165 ppt for the SWCNT-random network and the SWCNT aligned network, respectively. A decrease in the sensor's detection limit upon reduction in the aligned network-based density also occurred. Furthermore, sensors based on random networks of SWCNTs achieved not only a higher detection limit, but a better signal-to-noise ratio compared to the other investigated fabrication methods.272 In another study, VA-CNTs on a titanium/Au-coated glass plate were fabricated by electrophoresis and fissure formation techniques.273 This sensor had high sensitivity to NH3 and NO2 up to 100 ppm, with short recovery time within 40 seconds when a voltage of 5 V was applied for <30 seconds.273 Ethanol detection was achieved by a VA-MWCNT-based sensor with sensitivities of 0.18% to 1.67% for 50–800 ppm.275
For enhancement of sensing properties, CNTs modified with metal NPs,276–286,301 MOs,287–290,302–304 polymers292–296,300,301 and other nanomaterials238,240–244,297–299 have also been demonstrated. The stability and lifetime of the functionalized CNT-based sensors remain questionable, mainly due to the possibility of oxidation, which may degrade the sensing abilities.
Penza et al.276,277 compared the sensing performances of VA-CNTs276 and random networks of CNTs277 based on metal functionalization and bare MWCNTs over an operation range of 20–250 °C. The sensors were exposed to individual gases277 and mixtures276 of gases, such as NH3 and NO2. A temperature of 150 °C proved to be optimal for detecting NO2 and NH3.276,277 Metal/CNT-based sensors were more sensitive than unmodified sensors for 0.1–33 ppm NO2 and 5–1000 ppm NH3.276,277 Furthermore, random networks of CNTs achieved higher sensitivity compared to VA-CNTs.276,277 In order to increase the sensitivity, responses of the VA-CNT-based array were combined with PCA-based pattern recognition for clear separation of the responses to NO2 and NH3 from other compounds.276 Full recovery was achieved with temperature pulses.276 Several studies have explored the possibility of integrating different forms of CNTs as the basis of an acetone sensor used for potential diabetic diagnostics; however, none of them evaluated real-world breath samples. An array of 32 sensors based on nanotubes decorated with pristine metal (Pd, Au) and polymer was developed to selectively sense acetone and other gases at ppm levels.301 Abdelhalim et al.278 measured the sensing performance of an array with pristine and CNTs modified with Pd, Ag, Ti and Cr NPs and with different diameters to NH3, ethanol, CO and CO2 under ambient conditions. The optimal combination of the metallic NPs was 1.5 nm Au, 1.0 nm Cr and 0.2 nm Pd. Array responses were combined with PCA analysis for exposures to individual gases, as also to mixtures of 2 gases, NH3/ethanol and NH3/CO at different ratios in each mixture. PCA analysis showed that the response to a mixture of 2 gases lies in the plane between the data points of each gas separately.278 Penza et al.279 also reported on a room temperature operated sensor based on Ag/VA-MWCNTs with a selective response for 1 ppm NO2. Ag NP functionalization was also successfully reported for detection of 2 ppm H2S under ambient conditions, yet the recovery of the sensors has not been achieved due to the strong chemical interaction between H2S and Ag NPs.280 Benzene281 and NO2281,282 detection at room temperature was achieved with MWCNTs decorated with different metal NPs. Modified sensors were exposed to 50–500 ppb benzene and 0.5–6.5 ppm NO2. Selective detection of NO2 and insensitivity towards other gases, such as benzene281 and ethanol,282 were accomplished with Au-MWCNTs. Functionalization with Rh, Pd and Ni NPs responded oppositely for both VOCs that were tested.281 Full and rapid recovery was accomplished by temperature pulses.281,282 Further reports on NO2 detection by a Au/CNT layer have also been published.283–286 VA-CNTs decorated with 6 nm Au NPs of different lengths were exposed to NO2 0.5–1 ppm with 0 and 50% RH under operation at room temperature.283 The optimal length for NO2 sensing was 300 μm. When the RH was increased to 50%, all the sensors had increased responses regardless of the nanotube length. A heat-pulse of 150 °C was used for reducing the recovery time.283 Au/MWCNTs were recently investigated for detecting 0.1–10 ppm NO2, 5–1000 ppm NH3 and 0.1–10 ppm H2S over a temperature range of 100–200 °C.284 The optimal operational temperature was 150 °C, and by controlling the gold loading, it was possible to control the sensing responses and the selectivity to different gases. The lowest Au content (0.3 at%) gave the highest response to NO2, whereas MWCNTs functionalized with the highest Au content (1.1 at%) gave the highest responses to H2S gas (Fig. 13). Furthermore, binary gas mixtures, NO2/H2S and NO2/NH3, were tested for evaluating the detection of NO2 gas.284 Charlier et al.285 found that Au/MWCNTs with selective responses to 0.5–6.5 ppm NO2 at room temperature, had a responsiveness up to 6% for 500 ppb, and 12% for 6.5 ppm NO2. Nevertheless, 20 min were required to reach a steady state resistance value after gas injection. The response time can be reduced at a higher operational temperature, yet the sensitivity at 150 °C was not better than that at room temperature.285 Random networks of 5 nm Au modification of the sidewalls of the CNTs, operated at an optimal temperature of 200 °C, were exposed to several gases, including NO2 and NH3, at 0.5–10 and 5–1000 ppm, respectively.286 As expected, Au/CNTs gave a p-type response, namely an increase in resistance on exposure to reducing gases and a decrease in electrical resistance on exposure to NO2.286 MO nanomaterials are also excellent candidates for hybrid CNT sensors.287–290,302–304 An antimony–CNT–tin oxide (Sb–CNT–SnO2) thin film was fabricated and exposed to 100–1000 ppm of formaldehyde, ammonia, benzene and toluene.287 This sensor had high sensitivity along with short recovery and response times compared to a bare CNT device. Furthermore, the electric field on the surface of the Sb–CNT–SnO2 thin film was considered the reason for the rapid response to polar gases. Ethanol detection was reported by hybrid SWCNTs/Nb-Pt co-doped TiO2 thin films.288 Films with different SWCNT contents were exposed at a sequence of operating temperatures from 290 to 400 °C to 100–1000 ppm ethanol. The optimal content of SWCNTs was 0.01% by weight and the optimal operation temperate range lay between 290–320 °C. Detection of NO2 was demonstrated by a hybrid of SnO2/MWCNTs.289,290 This sensor was exposed to 100–500 ppm NO2 and had a high sensitivity, with total recovery after heating the chamber to 150 °C.290 Xiong et al.302 developed CNT cantilevers coated with WO3 for detecting a single acetone molecule based on changes in resonant frequency. Fabricated multi-wall CNT–SnO2 sensors enabled selective detection of ppm levels of acetone, and furthermore discriminating it from both ethanol and acetaldehyde.303 Ding et al.304 developed a photo-induced SWNT–TiO2 core/shell hybrid nanostructure that had a detection limit of several ppm to acetone vapors at room temperature.
 |
| Fig. 13 (a) TEM images of MWCNTs decorated with Au NPs. Scale bar is 50 nm on the left image, and 20 nm for the other images. Time response of chemiresistors based on pristine MWCNT films, and functionalized CNT films with Au loading of 0.3, 0.5 and 1.1 at%, exposed to 10 min pulses of decreasing concentrations of (b) NO2, (c) NH3 and (d) H2S at a sensor temperature of 150 °C. Reprinted from ref. 284 with permission from Elsevier, copyright 2016. | |
Polymer/CNT nanocomposites have also been reported for gas sensing applications.291–296 A VA-CNTs and poly(vinyl acetate)/polyisoprene (PVAc/PI) binary polymer composite film sensor was reported as being sensitive to cyclohexane and ethanol at high concentrations.291 Polyaniline (PANI)/MWCNTs were investigated for selective detection of NH3 gas at 0.05–150 ppm.292–294 A detection limit of 50 ppb was achieved, with a response time in the order of minutes and the recovery time of up to several hours.293 Another study reported a rapid response (6–24 seconds) and recovery time (35–62 seconds) along with high responses for the nanocomposite-based sensor compared to unmodified MWCNTs.294 Acetone detection was achieved by a PMMA/MWCNT-based sensor with a response time of 2–5 seconds at room temperature.295 Poly(m-aminobenzene sulfonic acid) (PABS)/SWNT random networks were sensitive to NH3, NO2 and water vapor.296 The sensor had excellent detection limits of 100 ppb for NH3 and 20 ppb for NO2. Adjustment of the initial resistance impacted on the sensitivity towards the gases tested. Upon exposure to RH levels of 10–70%, a response time of 2–3 min and full recovery time of <10 min were achieved after purging with dry air.296
Evans et al.297 reported zeolite/SWCNT nanocomposites for 10 ppm NO2 detection at room temperature and at different humidities related to real-world conditions. The choice of zeolites, which are known as molecular sieves, was due to their selective adsorption properties to targeted vapors, depending on their composition, hydrophilicity and loading. The results showed that zeolite/SWCNTs mixed as a layer configuration significantly reduced the sensor's responses to 25–75% RH. These findings show promise in that a composite of zeolite/SWCNTs can reduce the cross-sensitivity of the sensors to water vapor. Nevertheless, regeneration of the sensing layer after multiple exposures to water vapors required heat treatment at 150 °C.297 An array of metalloporphyrin/SWCNTs has been reported for gas detection at several RH levels.298 Several VOCs were tested, which included pentane, hexane, acetone, methanol, cyclohexane, ethanol, p-xylene and others at high ppm level concentrations. In combination with the PCA pattern recognition algorithm, the responses were accurately classified into 5 classes: alkanes, ketones, alcohols, aromatic hydrocarbons and amines. Furthermore, upon comparing the responses of [CoIII(tpp)]ClO4− and [CuII(tpp)]-based devices to VOCs at 2% RH in N2 and 10% RH in air, there were no substantial effects on sensor responses.298 Xie et al.299 used VA-MWCNTs modified with amino groups for the selective detection of formaldehyde from 20 to 200 ppb at room temperature. The sensors had high selectivity and fast response times to formaldehyde, and a negligible response to methanol, ethanol, acetone, ammonia and carbon dioxide each at 200 ppb.299
Haick's group demonstrated an essential focus in the detection of nonpolar VOCs under real humid conditions.238,240–244 An array of 10 random networks of SWCNTs coated with nonpolymeric organic materials was exposed to mixtures of VOCs found in the breath samples of lung cancer patients239 and of rats suffering from chronic renal failure240 at different RH levels. The nonpolymeric organic materials varied in their chain length, branching, aromatic configuration and functional groups.239,240 Due to this diversity, more interactions between the absorbed VOCs and the sensing layer became possible, and a combination with pattern recognition algorithms could discriminate between similar alkane compounds and their concentrations.308 At the first stage, the sensors were exposed to styrene, hexane, octane, trimethyl benzene and water at 0.01–100 ppm under ambient conditions.239 Based on pattern recognition, the sensor array discriminated well between different polar and nonpolar VOCs when exposed to one VOC at a time, and showed clear concentration dependence.239 Moreover, mixtures of VOCs, mimicking breath samples from healthy controls and lung cancer patients, were prepared at different RH levels: 1, 10 and 80%. PCA analysis showed a clearly opposite trend between the discriminative power and the RH level,239i.e. as the RH level decreased, the discriminative power increased. A preconcentration method for reducing RH 50 times resulted in excellent discrimination in a similar way to the simulated samples at 1% RH.239 Similar results were obtained based on simulated samples of chronic renal failure, including phenol, undecane, 2-ethyl hexanol and acetic acid at 10 and 80% RH.240 Zilberman et al.241 reported on additional organic nanomaterials, discotic hexa-peri-hexabenzocoronene (HBC) derivatives, used as a capping layer for random networks of SWCNTs. Two different derivatives were explored, namely HBC-C12 and HBC-C6,2, to both polar and nonpolar VOCs associated with lung cancer. The sensors were exposed to decane, octane, methanol and water at p/p0 = 1. A clear increase in the response to the nonpolar and methanol VOCs was observed for both HBC derivative-based sensors compared to the pristine SWCNT sensor (Fig. 14). Functionalization with films of HBC-C6,2 at much lower surface coverage compared to HBC-C12 improved sensing properties. The responses of pristine and HBC-C12/SWCNT sensor to water exposure were identical within the margin of error.241 In a further study, the sensitivity to different VOCs was investigated as a function of HBC-C12 coverage in the hybrid with SWCNTs.242 Comprehensive works about arrays of SWCNTs covered with different PAH have been published.243,244 These arrays were exposed to different polar and non-polar VOCs and water at a concentration range of p/p0 = 0.05–1 and at different RH levels of 5 to 80%.243,244 Such arrays gave 90.3%243 and 97.2%244 accuracy in discriminating between all the VOCs tested and water at 5% RH. For 80% RH conditions, 100%243 and 88.9%244 accuracies were achieved. These results demonstrate the potential of the PAH derivatives to critically affect the sensing properties by proper selection of the side-groups, concentration and the type of solvent.
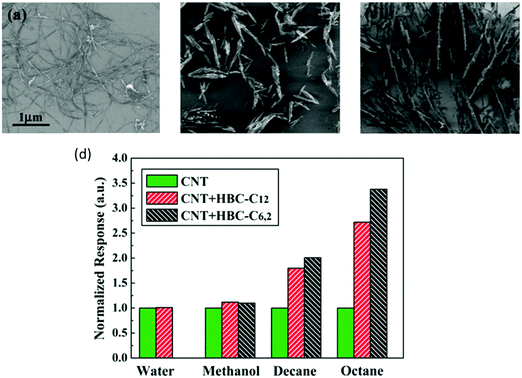 |
| Fig. 14 SEM of (a) RN-CNT cast from dimethylformamide (DMF) solution; (b) HBC-C6,2 structures cast from 10−3 M solution in xylene; and (c) HBC-C12 structures cast from 10−4 M solution in toluene. (d) Response of 2 kinds of HBC-functionalized RN-CNT sensors, normalized to the response of the corresponding pristine sensor, to water, methanol, decane, and octane at pa/p0 = 1. Reprinted with permission from ref. 241. Copyright (2009) American Chemical Society. | |
4.3.3. Flexible carbon nanotube-based chemiresistors.
CNT-based sensors are capable of withstanding high strains;309,310 nevertheless, a serious limitation is to detect small strains or pressures.310,311 Integration of CNTs into flexible sensors for gas sensing applications is well studied and many reports have been published, mostly on CNT-based chemiresistors.312–329 A large series of flexible substrates have been investigated, including polydimethylsiloxane (PDMS),312,313 plastic,314–316 paper,317,324 PET318–322,324,327 and polyimide.321,323,328 The majority focus on detection of NH3,314–316 H2S,316,318 NO,316,319 NO2,316,319–324 other VOCs and water.313,317,327,328 Rigoni et al.314,315 detected NH3 with sensors based on ITO/SWCNTs and pristine SWCNTs using flexible plastic substrates operating at room temperature. The ITO/SWCNT sensor had higher sensitivity towards concentrations >200 ppb, whereas similar responses were recorded for both sensors at lower concentrations. Detection limits of 13 and 3 ppb were estimated for ITO/SWCNTs and pristine SWCNT-based sensors, respectively. Upon exposure to 45–60% RH, opposite responses were recorded, and this behavior will allow, in the future, the combination of both sensors for discriminating the presence of water or ammonia under real-world conditions.314,315 Fe2O3/SWCNT composite films on plastic substrates had high sensitivity to several gases, including 10–200 ppm NH3, 1–100 ppm H2S, 10–100 ppm NO and 10–100 ppm NO2 at room temperature.316 The changes in the responses of a Fe2O3/SWNT composite film gas sensor under different bending angles up to 180° after exposure to 20 ppm H2S were negligible compared to a flat sensor. Asad et al.318 reported a flexible and selective H2S gas sensor based on CuNPs/SWCNTs on a PET substrate for detecting 5–100 ppm under both dry and humid conditions (40% RH) at room temperature (Fig. 15). A fast response and a recovery time of 10 and 15 seconds, respectively, were obtained for 5 ppm gas. A change of 5% in the response occurred upon bending up to 16 mm towards the gas compared to the flat position. Long-term evaluation of the sensor's performance to 5 ppm gas under ambient conditions resulted in a negligible change over a period of at least 30 days. Furthermore, the sensor was exposed to the gas under both dry and 40% RH conditions, and its response decreased ∼15% at 40% RH. WO3/MWCNT hybrid320 and reduced graphene oxide (RGO)/WO3/MWCNT hybrid321 had high sensitivity and selectivity to 0.5–10 ppm NO2 at room temperature with detection limits of 0.1 and 1 ppm, respectively. Shorter responses and recovery times were found with the RGO/WO3/MWCNT hybrid.321 Bending tests of up to 90° tested after exposure to gas showed <2% change in the response compared to the flat state. Further mechanical test using 106 bending cycles showed no significant degradation. In a humid environment, the baseline resistance shifted upward by 5%. Multilayers of a MWCNTs/PAH hybrid-based sensor on a PET substrate322 and a VA-CNTs/RGO hybrid film on a polyimide substrate323 were developed as NO2 sensors operating at room temperature. The concentration range tested was 0.5–20 ppm. Both the sensors were bent at different angles upon exposure to NO2; less than 5% change in the response was recorded in comparison to measurements on flat sensors. In order to achieve full recovery, UV radiation322 and heat treatment323 were used.
 |
| Fig. 15 (a) A schematic of the fabricated SWCNT-based gas sensors on a flexible substrate. (b) Sensor response to different H2S gas concentrations at room temperature. (c) Final response of the Cu-SWCNT based flexible sensor at 40% relative humidity and in a dry environment at room temperature. (d) Long-term evaluation of the sensor's response to 5 ppm H2S gas at room temperature. Reprinted from ref. 318 with permission from Elsevier, copyright 2015. | |
A sensor based on SWNTs on a flexible PET substrate was used for sensing high concentration organic vapors of hexane, toluene, acetone, chloroform, acetonitrile, methanol and water.327 The highest responses came from polar VOCs and water. A change of <4% in response occurred with bending downward 10 mm to saturated gas compared to the flat position. The sensing properties of a sensor based on AuNP and amine modified MWCNT on a polyimide substrate to 120–5800 ppm polar (propanol and ethanol) and non-polar (hexane, toluene, trichloroethylene and chloroform) VOCs and 4730–25
000 ppm water were also measured.328 PCA analysis showed discrimination between all these gases and their concentrations. Bending without exposure resulted in a negligible change in resistance.
4.3.4. Flexible carbon nanotube-based field effect transistors.
A FET sensor based on a AuNP/MWCNT hybrid on a PET substrate was able to detect down to 300 ppb ammonia with a limit of 255 ± 20 ppb at room temperature,330 but there was no full recovery. A bending test carried out without exposing the sensor to the gas, but significant change in the conductance was observed after 200 bending cycles.
4.4. Graphene-based sensors
Graphene (GR) is another potential material for gas sensing due to its numerous attractive properties. GR is a 2-D sheet with sp2 bonds between the carbon atoms, providing a very large surface area of 2630 m2 g−1. It is also compatible with chemical functionalization, and possesses extraordinary electrical, thermal and mechanical properties.331 GR is a p-type semiconductor in nature that does not contain metallic impurities as CNTs.332 It has high carrier mobility (200
000 cm2 V−1 s−1) with a carrier density of 1012 cm−2 and very low resistivity of 10−6 Ω at room temperature.333,334 High thermal conductivity (∼3000 W m−1 K−1) and mechanical strength (>1060 GPa) have also been reported.335,336 These properties, along with biocompatibility337 and high flexibility,338,339 make this nanomaterial suitable for many medical purposes. Nowadays, production of GR is simpler and bulk quantities can be produced by the CVD method,340–342 “unzipping” CNTs;343,344 and the most efficient method for producing GR monolayers is intercalation of small molecules in a graphite lattice and its exfoliation.345,346 However, not all the methods are suitable for deposition on flexible substrates.341,347 Preparation of GR oxide348–351 and RGO has been well described.352–354 By controlling GR production and its modification, an ultra-sensitive sensing performance is gained.355 GR and RGO were extensively reported as sensing materials for FETs,356–373 chemiresistors63,374–425 and other sensors426 in both solid state and flexible designs.
4.4.1. Solid state graphene-based field effect transistors.
Unfunctionalized GR and RGO-based FET sensors have been produced for detecting NO2,356–358,370 NH3,357,359–364 ethanol365 and humidity372 mostly at room temperature. Many fabrication parameters influence the sensing performance of the sensors, including substrate359 and gate type,360 GR/RGO preparation fabrication methods,357,358,361–364,370 and numbers of layers.356 GR gated by ionic liquid FET sensors had a sensitive and fast response to 9–2400 ppm NH3, with a calculated detection limit of 130 ppb.360 The current–voltage curve shifted 0.057 V per 10-fold increase in NH3 concentration. These results are similar to GR FET with a SiO2 gate, but with a lower operating gate voltage. A NO2 doped GR FET was tested for detection of 2–80 ppm ammonia with an estimated limit of 200 ppb (Fig. 16).364 Nevertheless, this doping method was not completely stable. Lu et al.363 reported an RGO-based FET that specifically, upon positive gate potential (n-type operation), possessed outstanding sensitivity to 1% NH3. Recovery time was 12 min at Vg = 40 V compared to hours or days for p-mode operated FETs. For detection of NO2, single- and multi-layered GR-based FETs were exposed to 2.5–50 ppm NO2.356 The single-layered GR-based device had a high sensitivity of up to 2.5 ppm, whereas the multi-layered device was of low sensitivity. Cui et al.370 reported a FET based on voltage-activated GR for detection of 0.05–1 ppm NO2. This process created more defects and oxygen functional groups that are primarily responsible for its sensing performance.
 |
| Fig. 16 (a) Sensitivity of as-fabricated graphene and NO2-doped graphene sensors to NH3 gas. (b) Sensitivity as a function of NH3 concentration. Id–Vg curves of (c) as-fabricated GR-FET and (d) NO2-doped GR-FET before and after exposure to NH3. Reprinted from ref. 364 with permission from American Institute of Physics, copyright 2016. | |
For enhancement of the sensing performance of different VOCs and gases, functionalization of GR or RGO with different nanomaterials, such as MOs,366,367 metals,368 polymers373 and DNA,369 was established under room temperature conditions. Mao et al.366 found a SnO2/RGO hybrid that selectively detected NO2. A SnO2/RGO FET was exposed to 1–100 ppm NO2 and a detection limit of 1 ppm NO2 was estimated. However, the recovery was slow, mainly due to the high-energy binding sites on the RGO possibly delaying recovery. Hybrids of Pd/RGO, with electrodes covered with grown graphene by the CVD method, sensitively detected 2–420 ppb NO with a response time of several hundred seconds for low concentrations (Fig. 17).368 The authors proposed that this ultra-sensitivity was due to the switch from the symmetric Schottky barrier to an asymmetric n-type barrier at the contact between Pd NPs and RGO after NO exposure. Cuong et al.367 successfully demonstrated detection of 2 ppm H2S with ZnO/GR composites. Detection of 0.25–25 ppm NH3 was achieved with a poly-3-hexylthiophene (P3HT)/GR nanocomposite based FET operating at room temperature, as the addition of GR to the polymer matrix provided a large surface area for absorption of gas molecules.373
 |
| Fig. 17 (a) Schematic illustration of the process for the preparation of Pd–RGO composites: (1) RGO synthesis and (2) Pd decoration of RGO. The inset photograph is the diluted Pd–RGO nanosheet suspension used for ac-DEP. (b) Schematics of graphene–Pd–RGO device fabrication and gas sensing test: (1) Ni electrode fabrication, (2) chemical vapor deposition (CVD) growth of graphene, (3) ac-DEP of Pd–RGO nanosheets, and (4) sensor measurement. (c) Relative change in conductance normalized by initial conductance (ΔG/G), real-time sensitivity dependence of device A of graphene–Pd–RGO exposed to several totally infused NO gas molecules with time durations of 1, 10, 60, 120, and 240 s corresponding to the concentrations of 2, 22, 132, 264, and 528 ppb, respectively. The inset demonstrates steady-state ΔG/G versus the concentration of total infused NO gas molecules ranging from 2 ppb to 1 ppm. (d) Typical relative changes in conductance of the devices versus time; the step-shaped conductance response corresponds to 1, 10, 60, and 120 s pulses of NO gas: 2, 22, 132, and 264 ppb, respectively. Reprinted with permission from ref. 368. Copyright (2011) American Chemical Society. | |
4.4.2. Solid-state graphene-based chemiresistors.
Many researchers of gas detection propose integration of both modified and pristine GRs and RGOs into chemiresistors.374–416,425 Pristine GRs and RGOs were thoroughly studied for detecting gases and VOCs, such as NO2,375–383,415 NH3,375,377,378,381,382 NO381 and toluene,384 mostly at room temperature. Hwang et al.377 explored the effect of GR's geometric characteristics on the chemical sensing properties of NO2 and NH3. These findings lead to the conclusion that the L/w ratio of an individual GR sheet dominated NH3 sensing, with no significant effect of the number of GR layers.377 The influence of the number of layers was measured as a function of 1–25 ppm NO2 detection, and a bilayer of GR proved to be optimal regarding the number of layers.415 A slow recovery was observed for all the structures.
Ethanol-based GR nanomesh sensors possessed sensitivities for both 1–10 ppm NO2 and 5–100 ppm NH3, with limits of detection of 15 and 160 ppb, respectively.378 A similar detection limit for NO2 was found with the epitaxial GR 6H-SiC based sensor.379 Ozone treated GR-based sensor showed a more sensitive response to 0.2–200 ppm NO2, with a detection limit of 1.3 ppb. Recovery was achieved with a dry air flow for 30 min.380 Extraordinary detection limits were obtained by pristine GR continuous illumination with UV light.381 During exposure, the sensor achieved detection limits of 158 ppq to NO, 2.06 ppt for NO2, 33.2 ppt for NH3 and 103 ppt for water. For NO sensing, the detection limit was 300% better than the sensitivity of CNTs under the same conditions. An 80% recovery was achieved within several min.381 300 ppb toluene was detected with a polycrystalline GR ribbon-based sensor.384 In some reports, full and fast recovery was enhanced by joule-heating382 and UV illumination.383
Further improvement of the sensing properties was attained by GR hybrids with other nanomaterials, including MO374,385–399,416 metals,400–405 polymers406–410,425 and organic materials.411–414 Many investigators have found several MO/GR hybrids for detecting NO2 in the range of 0.5–200 ppm.385–393 A 3.2 wt% WO3/GR nanocomposite film had high sensitivity to 0.5–20 ppm NO2 at room temperature and 40% RH. A response of 769% during the exposure to 5 ppm NO2 was achieved with response and recovery times of 9 and 18 min, respectively.391 The humidity influence on sensing performance was measured over the range of 30–80% RH upon exposure to 5 ppm NO2. As expected, the response of the sensor decreased as the RH increased. Long-term stability testing resulted in no significant change in the response for at least 45 days.391 Dong et al.392 reported α-Fe2O3/RGO nanocomposites exhibiting a high and selective response of 151% to 90 ppm NO2 at room temperature, with a detection limit down to 0.18 ppm. The recovery time was in the range of 44–1648 seconds.392 SnO2/RGO and In-doped SnO2(IDTO)/RGO were also prepared as selective NO2 gas sensors for 1–100 ppm concentration at room temperature, with a detection limit of 300 ppb.393 However, recovery times were very slow.393
Detection of other gases, such as NH3,394 acetone,395,396 H2S,395,397,416 formaldehyde374 and acetylene,398,399 was also examined by several composites with MO/GRs. For detection of 100–500 ppm NH3, Cu2O/RGO composites394 and an array of SnO2/RG and CuO/GR374 were investigated at room temperature. The Cu2O/RGO-based sensor achieved a high and selective response of 104% to 200 ppm NH3, with response and recovery times of 28 and 206 seconds, respectively.394 The sensor array of SnO2/RG and CuO/GR, in combination with neural network-based signal processing technologies, could recognize and predict the composition of NH3 and formaldehyde in a gas mixture, with a shorter recovery time of 110 seconds.374
Detection of acetone and H2S, which are associated with diabetes and halitosis, has been reported several times.395–397,416 Choi et al.395 showed the responses of SnO2/RGO to these gases as a function of RGO load and temperature at 85–95% RH. There was a sensitive response of 34% to 5 ppm hydrogen sulfide at an optimized temperature of 200 °C with 0.01 wt% RGO loading, whereas a sensitive response of 10% to 5 ppm acetone was achieved by increasing the RGO loading to 5 wt% and the operational temperature to 350 °C. For optimized temperatures, the detection limits were 1 ppm and 100 ppb for hydrogen sulfide and acetone, respectively.395 A similar detection limit of 120 ppb was found for acetone by the same researchers, demonstrating a selective response with a Co3O4/RGO/Ir NP composite at 300 °C and 90% RH.396 Combination of SnO2 quantum wires with RGO sheets showed a sensitive and selective detection of 10–100 ppm H2S at room temperature, with a fully reversible response and a detection limit of 43 ppb.416 A selective 1–1000 ppm acetylene detection was achieved with ZnO/Ag/GRO films.398,399 An optimum temperature of 150 °C with a 3 wt% Ag-loaded ZnO/GRO hybrid showed a sensitive response of 21.2% to 100 ppm acetylene gas,399 and a detection limit of 1 ppm at 11% RH and the optimized temperature.
A AuNPs/GR-based device was evaluated for detecting 15–58 ppm ammonia at room temperature.401 Recovery time was fast because of a combination of dry air and IR light during the entire recovery process; the detection limit was 6 ppm. Tran et al.402 compared the sensing performance between RGO/AgNWs and RGO/AgNPs to 15–100 ppm NH3 at room temperature. The RGO/AgNW sensor was more sensitive than RGO/AgNP and AgNP sensors. Cr/GR had a sensitive response to NO2 under ambient conditions with a detection limit of 90 ppb (Fig. 18).403 The sensor was exposed to 0.18–12.2 ppm NO2, with a 540 second recovery time following exposure to 0.732 ppm NO2. Composites of PIL-mediated Fe3O4/RGO and Ag/RGO,404 as also Gr functionalized with Ag or Au,405 had sensitive responses to several polar VOCs, including ethanol and acetone.
 |
| Fig. 18 (a) Low magnification and (b) high magnification SEM images of graphite oxide flakes. Response of (c) GO-Cs and GO based sensors towards NO2 with concentrations >1 ppm; (d) a GO-Cs based gas sensor after exposure to different concentrations of NO2 ranging from 0.091 to 1.44 ppm. Reprinted from ref. 403 with permission from Beilstein-Institut, copyright 2014. | |
Graphene oxide/polypyrene (GO/PPr) composite films were used for selective 24–500 ppm toluene detection at room temperature.406 Addition of PPr led to a more continuous and porous morphology that improved its sensing and mechanical properties. Fast response and recovery times were found. Polymeric modifications are also commonly used for detection of NH3.407–410 PANI/GR nanocomposites gave excellent responses to 1–6400 ppm NH3;408,409 however, the recovery process and time were inconsistent.408,409 A 30 day stability test for exposure to NH3 showed that the response of the PANI/GR to NH3 decreased and then stabilized after 9 days.409 A hybrid of P3HT/RGO was tested for selective detection of 10–50 ppm NH3 at room temperature;410 response and recovery times were relatively short, 141 and 488 seconds, respectively, following exposure to 10 ppm NH3.
RGO reduced by pyrrole sensors were fabricated and tested with NH3 at room temperature.412,413 The range used was 1 ppb to 100 ppm NH3, which gave a fast recovery under IR illumination.412,413 The sensor achieved fast response times, such as 1.4 seconds on exposure to 1 ppb NH3.412 Sulfonated RGO (S-G) and ethylenediamine-modified RGO (EDA-G)-based sensors were examined for the selective detection of 1–45 ppm NO2.414 The detection limits of these modified sensors were 3.6 ppm for S-G and 0.07 ppm for EDA-G, and full recovery was achieved in 30 min.
4.4.3. Flexible graphene-based chemiresistors.
Flexible sensors based on GR and RGO are relatively new, and only a few reports have been published on their gas sensing applications. Detection of NO2,417–423 NH3422–424 and ethanol63 by flexible Gr-based chemiresistors has been reported. A multilayer GR film on a PI substrate has an enhanced sensitivity to 0.2–5 ppm NO2 at room temperature.417 Less than 5% change in the resistance upon exposure to NO2 was seen compared to the flat state. In order to improve the recovery time, heating elements418,419 and UV radiation420 were used. Flexible GR gas sensors integrated with an internal heater418,419 and a RGO film on a PET substrate with external UV radiation420 could detect 0.5–200 NO2. During the bending test, <5% change in the response of the GR film upon exposure to 1 ppm NO2 was observed compared to an unbent sensor.419 In contrast, the RGO film showed 12–26% change in resistance as compared to flat measurement.420 Addition of Pd and Al NPs was investigated in terms of sensing performance of flexible GR-based sensors at 150 °C.422 A Pd/GR device was sensitive to 5–100 ppm NH3, whereas Al/GR was sensitive to 1.2–5 ppm NO2. Pd NPs accumulated the hole carriers of GR and Al depleted hole carriers. An Al/GR sensor was exposed to 1.2 ppm NO2 with bending for up to 104 cycles. Resistance changes were negligible in comparison to the flat sensor even after 3 months. RGO decorated with Ag NPs has also been reported for detecting 0.5–100 ppm NO2 and 13 ppt NH3 at room temperature, with fast response and recovery times of 12 and 20 seconds, respectively.423 An ink-jet printed poly(3,4-ethylenedioxythiophene):poly(styrenesulfonate) (PEDOT:PSS)/GR gas sensor had a high selective response to 25–1000 ppm NH3 and diethylamine at room temperature compared to a PEDOT:PSS-based sensor (Fig. 19).424 Upon bending to up to 70° angle under an exposure to 500 ppm NH3, the gas response to NH3 increased as the bending angle increased; for an angle of 70°, a 6.2% change in the resistance occurred, probably due to enhanced swelling due to the bending extension. A ZnO nanorod/GR hybrid based on a metal foil was reported to be sensitive to 10–50 ppm ethanol at room temperature and possessed excellent mechanical or electrical properties on flexural deformation to a bending radius of <0.8 cm for up to 100 times.63 Yang et al.371 demonstrated a 200 ppm NO2 detection at room temperature by GR-FET on a paper substrate under a strain of 0.5%, with <7% change in the response compared to relaxed conditions.
 |
| Fig. 19 (a) Schematic diagram of the gas sensor fabrication process. Gas responses of flexible printed (b) PEDOT:PSS and (c) graphene–PEDOT:PSS gas sensors to different concentrations of VOCs at room temperature. Reprinted from ref. 424 with permission from Elsevier, copyright 2014. | |
4.5. Silicon nanowire-based sensors
Silicon nanowires (SiNWs) are excellent candidates for gas sensing applications because they have several advantages, including large carrier mobility,427,428 tunable properties afforded by controlling the doping levels429 and/or chemical functionalization,430,431 compatible with very-large-scale integration (VLSI) processes, and compatible with complementary metal-oxide-semiconductor (CMOS) technologies.432,433 Furthermore, SiNW synthesis can be prepared by several methods, including CVD, pulsed laser deposition (PLD), thermal evaporation, template-assisted growth, and reactive ion etching (RIE).434–436 Although the sensing capabilities of bare SiNWs to polar VOCs are excellent, detection of non-polar VOCs remains problematic. Functionalization of the SiNWs with different coating layers overcomes this obstacle. Even though there are many reports on SiNW based devices in sensing application, only a small portion sampled in the gas phase.436–467
4.5.1. Solid-state silicon nanowire-based field effect transistors.
Han et al.443 showed a chemically gated FET based on hybrid integration of 1D SiNWs and 2D SnO2 thin films. This sensor was exposed to several gases, including 100 ppm NO and 10–100 ppm NH3. In 2011, Niskanen et al.444 reported on an unmodified SiNW that can be used in combination with pattern recognition algorithms as versatile chemical vapor sensors without additional functionalization. They exposed the sensors to acetone, ethanol and water with 100% accuracy, whereas methanol, ethanol and 2-propanol were classified with 96% accuracy under ambient conditions. Haick's research group has published a number of systematic studies on the fundamentals and applications of functionalized SiNW-based FETs for both polar and non-polar VOC detection.445–453 Paska et al.445 reported on the sensing properties of SiNW functionalized with hexyltrichlorosilane (HTS) to both polar (water, ethanol, 1-butanol, 1-hexanol, 1-octanol and 1-decanol) and nonpolar (n-hexane, n-octane and n-decane) VOCs at ppm levels in 15% RH air. HTS modification reduced the Si–O–Si bond fraction between adjacent molecules and greatly enhanced sensitivity to both polar and nonpolar VOCs. The sensing mechanism of the non-polar ones can be explained in terms of an indirect interaction in which nonpolar VOC molecules induce conformational changes in the organic monolayer. The sensing mechanism of polar VOCs is based on direct interaction via VOC-induced changes in the SiNW charge carriers, most probably due to electrostatic interaction between the SiNW and polar VOCs. In a subsequent article, Paska et al.446 modeled the detection process based on changes in the carrier mobility, voltage threshold, off-current, off-voltage and subthreshold swing of the devices to non-polar VOCs. The devices were modified with alkyl trichlorosilanes with different alkyl lengths to explore the interactive effect of hysteresis and surface chemistry upon exposure to both polar and non-polar VOCs.447 The density of the unpassivated Si–OH groups (trap states) on the SiNW surface had a crucial effect on the hysteresis characteristics of the gated silicon nanowire sensors relative to the effect of the hydrophobicity or molecular density of the organic monolayer. Wang et al.448,449 investigated the effects of chain length and functional groups on the sensing properties of silicon nanowires to different various VOCs at 0.01–0.08 p0/p concentration (p0 and p represent VOC's partial pressure and the total vapor pressure, respectively). Functionalization of SiNW FETs with longer monolayers of alkane-backbone silane enhanced the selectivity of the sensors to non-polar VOCs.448
Conjugation of an array of modified SiNWs with pattern recognition algorithms for discrimination of a variety of VOCs has also been reported.450,451 Ermanok et al.451 found by a combination with DFA, the potential to discriminate between polar and nonpolar VOCs at 0.01–0.08 p0/p concentration, as also between the individual VOCs within each group. Combination with an artificial intelligence algorithm on SiNW FET device parameters provided high selectivity to specific VOCs in both single-component and multi-component environments, even in mixtures that contained counteracting compounds of similar structures or chemical properties to those of the target VOC. Concentration was also estimated (Fig. 20).450 Shehada et al.452,453 reported successful discrimination of simulated samples based on related VOCs to gastric cancer (GC) and lung cancer and environmental confounding factors using an array of modified SiNW FETs.
 |
| Fig. 20 (a) Schematic illustration of an ANN model for VOC recognition. (b) Hot plots of logarithm Euclidean distance at VOC concentration of pa/p0 = 0.08. (c) Euclidean distance of ANN outputs using sensor S3 to identify hexane, hexanol, octane, and their binary and ternary mixtures. All the VOC concentrations are at pa/p0 = 0.08. Inset: Schematics of the relationship between single VOCs and their binary and ternary mixtures in ANN outputs. Reprinted with permission from ref. 450. Copyright (2014) American Chemical Society. | |
4.5.2. Solid-state silicon nanowire-based chemiresistors.
Bare SiNWs, fabricated by the vapor–liquid–solid mechanism, were used to detect 175–700 ppm NH3.455 SiNWs-decorated with TeNPs lowered the detection range to 10–400 ppm NH3.456 Bare SiNWs, fabricated by tri-layer nanoimprinting were reported as chemiresistors for the detection of NO2457,458 and NH3.457 The authors fabricated 2 nanowire sensors with different line-widths and a thin-film device for comparison. The sensors were sensitive to 250 ppm NO2457,458 and NH3457 at room temperature, especially for the narrower device due to its larger surface to volume ratio.457 SiNWs coated with thinner layers of AuNPs gave bigger sensor responses to samples at 30–95% RH at room temperature.454 As RH increased, a monotonic decrease of the current occurred. Modification of peptides on SiNW enhanced sensitivity to 100 ppm NH3 and acetone at room temperature.459 Gao et al.460 described a device based on bare SiNWs fabricated by bilayer nanoimprint and angle deposition that had higher sensitivity (155% at 60 nm width of SNW) to 250 ppm NO2 compared to previous publications.457,458 In 2012, Cuscunà et al.461 showed an alternative on-chip fabrication method for NO2 sensors. The authors exploited SiNWs directly grown onto a selected area, over and between pre-patterned interdigitated electrodes defined on oxidized silicon wafers and exposed to ammonia plasma. This sensor was ultrasensitive to 10 ppb gas at room temperature. A similar detection level for NO2 in humidified air was achieved by a device based on periodically porous top electrodes on a vertical SiNW array (Fig. 21).461 Furthermore, this device had a sensitive response to 1 ppb NH3.
 |
| Fig. 21 (a) Sketch of the interdigitated structure used to realize the sensor and the designed Si NW sensor. (b) SEM images of the finite chemoresistive sensor, and (c) Si NW network details for the interdigitated structure with L = 6 μm. (d) Response curve of a typical sensor with a finger gap value of L = 21 μm. The sensor response is the current change due to NO2 concentration variation at a bias voltage of 1 V. The inset shows the response and recovery behaviors of the device at 20 and 10 ppb. (e) Normalized current variation, defined as S = 100ΔI/I0 = 100(I − I0)/I0, as a function of NO2 concentration at a selected response time of 3 min. I and I0 are the electrical current in NO2/humid nitrogen mixture and only humid nitrogen, respectively. Reprinted from ref. 461 with permission from AIP Publishing, copyright 2012. | |
Sensors based on ZnO nanorod/porous SiNWs463 and SiNW/WO3NW nanocomposites with a cactus-like structure464 were used for 0.25–50 ppm NO2 detection at room temperature. A WO3 nanowires/porous silicon composite proved to be sensitive and selective to NO2 at sub-ppm levels, with a fast response-recovery characteristic at an operating temperature of 100 °C.465 Bare porous SiNWs prepared by metal-assisted chemical etching were sensitive to 500 ppb–5000 ppm NO at room temperature, with a fast response and excellent reversibility.466
4.5.3. Flexible silicon nanowire-based field effect transistors.
Only a single study was found in the literature describing the use of flexible FET devices based on SiNWs, even though many such sensors have been described, using the same solid-state structures. McAlpine et al.467 showed that SiNWs on flexible plastic form a sensitive FET device for detecting 20 ppb–20 ppm NO2 under ambient conditions. The array of 4r FETs is based on modified (alkane-, aldehyde- and amino-silanes) and unmodified SiNW fabricated on a flexible plastic and exposed to 1000 ppm acetone and hexane. This nanoarray was capable of distinguishing between the gases.
4.6. Surface acoustic wave sensors
Surface acoustic wave (SAW) sensors are based on frequency modulation of waves that are traveling along the surface of an elastic substrate. They are sensitive to physical phenomenon as their amplitudes decay exponentially with substrate depth.468 The sensing mechanism is based on a frequency shift of the sensing layer, which can be caused by changes in conductivity, stress effect, mass loading and viscoelastic effect as a result of gas absorption.468,469 Selection of a proper sensing layer is critical in order to enhance good sensitivity and selectivity to the target gas. Atmospheric conditions may influence the propagation of waves. These conditions include temperature, pressure and humidity.468 Several materials have been used as sensing layers for a variety of gases and VOCs, including MOs,470–476 GR,477–479 polymers480–484 and CNTs.485–489
4.6.1. Metal oxide-based surface acoustic wave.
Tang et al.470 described a room temperature SAW sensor for the selective detection of NH3 based on Co3O4/SiO2 composite films. A 50% Co3O4 loading into the film gave the best sensing properties to 1–60 ppm NH3. They recorded the sensor's stability and reproducibility at room temperature. ZnO/SiO2 bi-layer porous nanofilms on a ST-cut quartz SAW sensor successfully detected 5–120 ppm NH3 at room temperature.471 The sensing performance was dependent on the value of sheet conductivity of the films. As a result, the bi-layer nanofilms were more sensitive to NH3 compared to single layer films, and depended on the thickness of the top ZnO layer. A 60 nm layer of ZnO resulted in a frequency shift of 2000 Hz at 30 ppm of gas, with good repeatability and stability. Pristine SiO2, TiO2 and composite SiO2/TiO2 films of 200 nm thickness were coated on quartz SAW sensors.472 Upon exposure to 1–40 ppm, NH3, TiO2 and SiO2/TiO2 films gave positive frequency shifts, whereas the SiO2 film gave a negative frequency shift. According to these results, the negative frequency shift was mainly due to the increase of NH3 mass loading on the sensing film, whereas the positive frequency shift upon exposure to NH3 gas was related to the condensation of the hydroxyl groups on the film leading to its stiffer and lighter nature. Humidity was also explored, as it has a significant effect on the sensing performance because the water absorbed on the film surface may provide an active site to absorb NH3, thus enhancing the sensitivity of the sensors. A SAW sensor, based on a composite SiO2/TiO2 film, was much more sensitive to NH3 at low concentration levels, with a response of 2 kHz while displaying fast response and recovery, excellent selectivity and stability.472 A ferroelectric lead zirconium titanate (PZT)-based SAW sensor was explored as an NO2 sensor473 by being exposed to 80–250 ppm gas at room temperature. A linear calibration curve was obtained with a sensitivity of 9.6 Hz ppm−1. Ultrasensitive detection of NO2 was achieved with a ZnO-based quartz SAW resonator.474 This sensor exposed to 0.4–16 ppm gas at room temperature was highly sensitive and selective. Ippolito et al.475 exposed an optimized SAW sensor based on ZnO/WO3 to 10–500 ppm ethanol under both dry and humid conditions. The magnitude of the response decreased with increasing RH levels and decreasing operating temperatures (Fig. 22). Frequency shifts of 119, 90 and 86 kHz upon exposure to 500 ppm ethanol in synthetic air at room temperature were observed for 0, 25 and 50% RH, respectively. The largest response to 500 ppm of ethanol was seen after the operating temperature had increased to 300 °C. Recently, an amino-functionalized iron oxide NP-based SAW sensor was explored as a VOC that was sensitive at room temperature.476 It was exposed to 25–150 ppm butanol, 400–1200 ppm isopropanol, 200–1000 ppm toluene and 50–200 ppm xylene over a low concentration range. Sensitive responses to these VOCs were demonstrated, together with fast response times of a few min with good reproducibility. The calculated limits of detection for each one of the VOCs was ∼1 ppm for butanol, 12 ppm for isopropanol, 3 ppm for toluene and 0.5 ppm for xylene.
 |
| Fig. 22 (a) Sensitivity of a layered structure. (b) Response of ethanol at an operating temperature of 300 °C RH of 0, 25 and 50% (measured at 20 °C). Reprinted from ref. 475 with permission from Elsevier, copyright 2006. | |
4.6.2. Graphene-based surface acoustic wave.
GR-Based SAWs have also featured in the literature.477–479 Thomas et al.478 investigated a GR-based SAW sensor for detecting ppm-levels of NO2. The sensor had a sensitive response of 25 Hz ppm−1. A GR-like nano-sheet/LiTaO3 SAW sensor was fabricated and investigated for detecting 60–1000 ppm CO gas.479 A GR/LiNbO3-based SAW device was used to detect a relative humidity in the range of 25 to 85% RH at room temperature.490 In the low RH range (RH < 50%), a frequency downshift of 1.38 kHz/1% RH change occurred, whereas for the high RH range (RH > 50%), a 2.6 kHz/1% RH frequency downshift occurred.
4.6.3. Polymer-based surface acoustic wave.
An array of SAW sensors based on 20 different polymers was evaluated for the detection of a variety of VOCs, including toluene, acetone, ethanol and methanol in dry air each at 120–1300 ppm.480 The array was most sensitive and selective to toluene exposure. Furthermore, PLS regression was applied to the sensor responses, being obtained from ternary mixtures of the analytes. Stahl et al.481 reported on an array of 8 surface transverse wave (STW) sensors coated with different polymers, which were used at semi-annual intervals for a 3 year period for detecting 3 different VOCs, chloroform, octane and xylene. Among the polymers used as sensing layers were poly(butyl methacrylate), polyepichlorohydrin (PECH) and PDMS, etc. The following concentrations were used of each VOC: 2.3–11.6 ppm chloroform, 11.5–34.4 ppm octane and 0.8–4.5 ppm xylene. Radar charts were plotted based on the sensors' responses to obtain characteristic patterns for each VOC. Furthermore, no significant time-related changes were seen over the test period.481 Liu et al.482 demonstrated a high-frequency SAW (1.56 GHz) humidity sensor based on electrospun CeO2 NPs/polyvinylpyrrolidone (PVP) nanofibers. The sensor was exposed to 11–95% RH; due to an additional acoustoelectric loading effect arising from the enlarged electrical conductivity of nanofibers at high RH levels, the frequency response was improved compared to a SAW sensor based on pure PVP nanofibers. Addition of inorganic CeO2 NPs into PVP also enhanced this humidity sensor, with an excellent frequency response and superior long-term stability. A polypyrrole nanofiber surface acoustic wave gas sensor was tested for 2.1–8.5 ppm NO2 at room temperature.483 The frequency shift due to the sensor's responses measured 4.5 kHz at 2.1 ppm NO2. The sensor's performance was also assessed during a 5 day period. A SAW sensor based on a PANI/In2O3 nanofiber nanocomposite was NO2 and CO sensitive at room temperature.484 This sensor was exposed to 0.51–8.5 ppm NO2 and 60–1000 ppm CO in dry air; fast response and recovery times with good repeatability were observed.
4.6.4. Carbon nanotube-based surface acoustic wave.
SAW sensors based on CNT have also been reported.485–488 Penza et al.486,487 investigated SAW sensors coated with either SWCNTs or MWCNTs dispersed in ethanol or toluene for detecting VOCs at room temperature. The VOCs included ethanol, ethylacetate and toluene. Selectivity to VOCs can be controlled by the type of organic solvent used to disperse the CNTs, as also the number of layers of the CNTs. For example, the highest sensitivity to ethanol was obtained with SWCNTs in ethanol, whereas for the other VOCs it was with SWCNTs in toluene.486 The calculated limits of detection were 1.3 ppm for ethanol, 1.6 ppm for ethylacetate and 1.2 ppm for toluene.486 SAWs, based on nanocomposites of PECH and polyetherurethane with different percentages of MWCNTs, were tested on exposure to octane and toluene at room temperature.488 The sensors gave a high selective response to toluene and octane, but no response to other gases, such as H2, NH3, NO2 and CO. Addition of small percentages of MWCNTs to the polymer increased the sensitivity to toluene, but hardly changed the response to octane.
4.7. Piezoelectric sensors
Piezoelectric sensors are commonly used for gas-phase detection in a variety of applications.491,492 The sensor measures fundamental oscillating frequency changes of a quartz crystal resonator as a result of molecule adsorption from the gaseous phase onto its surface. The performance of a quartz crystal microbalance (QCM) system can be controlled by different coating layers selected from a wide range of MOs,493–497 polymers498–513 and other nanomaterials.514–517 Environmental parameters, including temperature and humidity, must be taken into account as they affect the resonant frequency.501,512,518
Detection of humidity was demonstrated by several QCM sensors.512,513,516 A QCM sensor, based on mesoporous silicate SBA-15 with monodisperse hexagonal lamelliform, was prepared as a sensitive humidity sensor.512 The sensitivity of the sensor exposed to 10–100% RH at room temperature was directly proportional to the film's thickness. A SBA-15 film of 20 nm thickness had the best sensing properties giving 12.32 Hz/RH% with a response time of ∼12 seconds and a recovery time of 16 seconds (Fig. 23). The long-term stability of the sensor was tested at 97% RH for 42 days. A fibrous composite of polyacrylic acid (PAA)/PVA membrane was developed as a sensitive coating layer of a QCM sensor to detect humidity.513 This sensor was exposed to 6–95% RH range and had high sensitivity especially in the range of 20–95% RH compared to a flat PAA/PVA film. The nanofiber PAA membranes were remarkably sensitive to humidity compared to fibrous PAA/PVA membranes. Long-term stability of a PAA nanofiber-based QCM was also measured over 30 days and a good stability was observed. Yao et al.516 investigated the performance of a chemically derived graphene oxide (GO) thin film coating deposited at different thicknesses on a QCM sensor for humidity detection. The sensor was exposed to 6.4–93.5% RH environments at room temperature. As the thickness of the film increased, the sensor became less sensitive to humidity compared with thinner coating layers. Long-term stability was measured for 3 weeks.
 |
| Fig. 23 SEM (a and b) images and TEM (c and d) images of as-made hexagonal lamelliform mesoporous SBA-15. (e) Frequency response curves of S1 from low (RH = 11.3%) to high, then high to low, relative humidity levels. Reprinted from ref. 512 with permission from Elsevier, copyright 2010. | |
4.7.1. Metal oxide-based quartz crystal microbalance.
ZnO NW-based QCM sensors with different thicknesses of the sensing layer were prepared and tested for detecting 40–1000 ppm NH3 at room temperature.493 A fast response time of 5 seconds was observed for each gas concentration tested. The sensors showed good frequency stability and reproducibility. ZnO nanorods were also explored for selective detection of NH3 at room temperature.494 QCM-based ZnO nanorods were exposed to 50–200 ppm NH3 with a 9.1 Hz frequency shift to 50 ppm. The response and recovery times varied with NH3 concentration. These gas sensors also showed good reproducibility and high stability. Thin ITO film-based QCMs were demonstrated as an NO detection sensor;495–497 they were exposed to 295–1770 ppm NO and had an approximately linear negative frequency shift when exposed to an NO-doped atmosphere.495 Hu et al.496 found good sensitivity of an ITO-coated QCM sensor to 60–1180 ppm at room temperature. Furthermore, a distinct negative frequency shift of 110 Hz within 600 seconds was observed upon exposure to 60 ppm gas. However, the responses of all these reported sensors were irreversible.495–497
4.7.2. Polymer-based quartz crystal microbalance.
Electrospun nanofibers of cross-linkable PAA and PVA with different weight ratios of PAA/PVA were deposited on the surface of QCM for detecting 50–200 ppm NH3 at room temperature and 50–60% RH.498 The sensing performance was affected mainly by the content of the PAA component in the nanofibers, the relative humidity and the concentration of NH3. Palaniappan et al.499 detected NO with phthalocyanine/silica hybrid films on a QCM at room temperature. Their results showed sensitivities up to 25 ppb Hz−1. Cross-sensitivity to CO was also evaluated. A QCM coated with cross-linked poly(styrene-co-chloromethyl styrene) was fabricated to develop an NO2 gas sensor.500 The results indicate a sensitivity of 1200 Hz h−1 for 50 ppm NO2, yet gas exposure irreversibly decreased the oscillation frequencies of all the devices tested.
Formaldehyde detection has also been demonstrated with QCM sensors.503,504 Zhang et al.503 tested a QCM sensor coated with 3D polystyrene (PS) fibers functionalized with polyethyleneimine with 3–140 ppm gas at room temperature. The sensor showed selective and sensitive responses to the gas. PEI/PVA nanofibers at a weight ratio of 1.6/1, formed from the cosolvent of water and ethanol, were also reported to be formaldehyde sensitive.504 QCM-based PEI/PVA nanofibers were found to selectively respond to 10–255 ppm gas at room temperature. During exposure to formaldehyde, the sensor was exposed to humidity conditions from 10 to 70% RH. Exposure to 255 ppm formaldehyde at 10% RH gave no response, whereas responses of the QCM sensor were augmented in increasing relative humidity (Fig. 24).
 |
| Fig. 24 FE-SEM images of fibrous membranes at different weight ratios of PEI/PVA: (a) 0.8/1; (b) 1.6/1; and (c) 3.2/1. (d) Response of the fibrous membrane coated QCM sensor with a PEI/PVA weight ratio of 1.6/1 formed from the cosolvent of water and ethanol, exposed to 10, 30, 80, 155, and 255 ppm formaldehyde at different RHs. Reprinted from ref. 504 with permission from Elsevier, copyright 2010. | |
Ayad et al.505 described a PANI-coated QCM sensor for detecting alcohol vapors, such as ethanol, methanol, 2-propanol and 1-propanol, in the ppm range at room temperature. A linear correlation was noted between concentrations of alcohol vapors in the ppm range and the frequency shift. Increasing film thickness led to more hydrogen bond formation with the PANI chains.
Si et al.506 fabricated and tested 8 QCM sensors coated with different conducting polymers of thiophene and its derivatives with a variety of VOCs, including toluene at 200–5000 ppm, and p-xylene, acetic acid, acetone, ethanol, 1-octanol, acetonitrile and water vapor at 5000 ppm. By combining with PCA analysis, the array discriminated between polar and non-polar VOCs. Calixarene film-coated QCM sensors were used for detecting VOCs, including aromatics, chlororganics, ketones, and alcohols.507 The calixarene molecule acts as the host whereas different VOCs act as guests. Structural changes of the numbers of aryl fragments and the binding of different functional groups to upper and lower rims lead to sensing of various VOCs with different sensitivity and selectivity. Phosphorous-containing calixarenes showed maximal sensitivity to the tested VOCs with a limit of detection in the ppm range. PEGylated lipids (polyethylene glycol) containing disulphide as supports have also been used as QCM coating layers.508 These sensors were exposed to a wide range of VOCs, including alcohols, esters, acids and aldehydes each at 100 ppm. PCA analysis showed separation between the different VOCs into 10 distinct groups. QCM-coated with a PVC blended lipid membrane was exposed to methanol, ethanol, chloroform, acetone and benzene at high ppm levels.509 By combining with PCA analysis, the sensor showed good separation and classification of VOCs. An array of 8 phthalocyanine-coated QCM sensors together with an artificial neural network (ANN) was used to find the composition of gas mixtures.510 Three binary systems were used in this study, namely: ethanol–acetone, ethanol–trichloroethylene, and acetone–trichloroethylene. By using the ANN algorithm, the success rate of the system in identifying the component concentrations was >84.5%, with overall average prediction error of 10.6%. Toluene and p-xylene molecular imprinted polymers, composed of methyl methacrylate as a monomer and divinylbenzene as a cross-linking agent, were prepared in the presence of toluene and p-xylene.511 By adding the solvent molecules, specific recognition sites inside the polymer matrix were created. Each sensor reacted with the preferred VOC in the range of 170–540 ppm, but with slow response rates.
4.7.3. Other nanomaterial-based quartz crystal microbalance.
TiO2/poly(acrylic acid) ultrathin film QCM sensors were fabricated and exposed to 0.3–15 ppm NH3 at different humidities.514 There was a linear response to NH3 concentration. Moreover, no frequency changes were recorded upon exposure to NH3 over a 30–70% RH range. The limit of detection was estimated at 0.1 ppm. PVP/RGO nanocomposites were sprayed on a QCM sensor for selective NO2 sensing at room temperature.515 The sensor exposed to 20–100 ppm had a higher sensitivity and a shorter recovery time than a PVP-based QCM sensor. Tao et al.517 developed a QCM-based sensor for detecting acetone in the breath of diabetic patients. The authors used a room temperature ionic liquid (1-butyl-3-methylimidazolium tetrafluoroborate) as a selective sensing material coating the QCM. The solubilization of acetone in the ionic liquid leads to a decrease in density and viscosity of the film, resulting in a positive frequency shift of the QCM. A linear response to varying acetone concentrations was achieved, with a detection limit of 5 ppm. Confounding VOCs commonly present in breath (e.g., isoprene) did not interfere with the response.517
4.8. Colorimetric sensors
Colorimetric sensors are based on chemo-responsive indicators, which chemically react and change their color differentially upon exposure to different chemical species.519,520 Color changes are measurable by scanning the sensor array before and after an exposure, thereby identifying and quantifying the species. Chemo-responsive indicators include (i) pH indicators that respond to Brønsted acidity/basicity (i.e., hydrogen bonding), (ii) metal salts that respond to redox reactions, (iii) metal ion-containing dyes that respond to Lewis basicity (i.e., electron pair donation); (iv) dyes with large permanent dipoles (i.e., solvatochromic dyes), and (v) nucleophilic indicators that respond to electrophilic analytes.519,520 A colorimetric sensor array has many advantages, such as being a fast, small and portable device with the possibility for customization. Nevertheless, many challenges need to be overcome; for example, an array is operated in an irreversible manner, meaning it has to be a disposable single-use device. Another challenge is reproducibility of the printing and imaging, in addition to difficulties in determining individual components in a mixture.521 Yet another involves the sensing non-polar VOCs compared to the abilities of other sensors.519
Suslick et al.522 described an array of 24 colorimetric sensors based on a variety of chemo-responsive dyes for detection of a wide range of VOCs at room temperature. These chemo-responsive dyes included Lewis acid/base dyes, Brønsted acidic or basic dyes, and dyes with large permanent dipoles. This array was exposed to alcohols, aldehydes, esters, ketones, and other compounds. Linear combinations of the RGB responses to the 24 dyes, in combination with PCA analysis, could discriminate between different VOCs. Furthermore, the selected dyes were essentially unresponsive to water vapor. Sensitivity at the ppb-level was demonstrated for VOCs, such as thiols, amines and carboxylic acids. In further investigations, colorimetric arrays were tested with up to 100 different VOCs and different humidities (Fig. 25),523–526 and similar results were obtained.
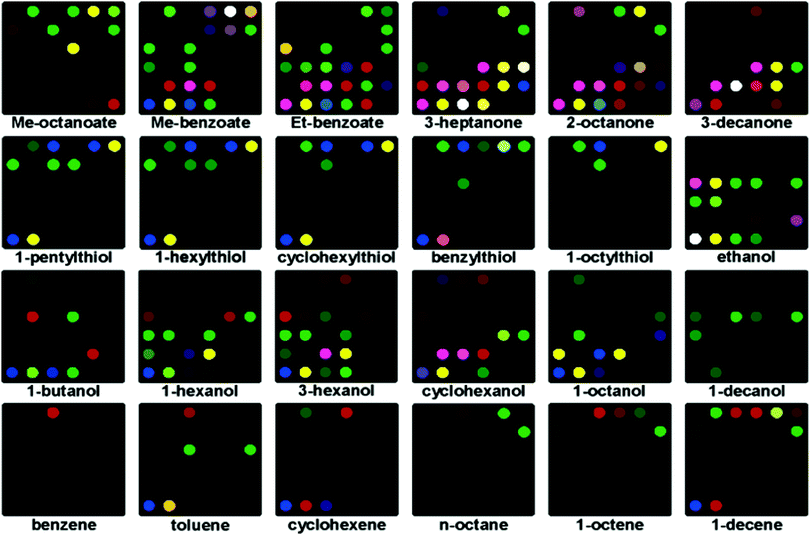 |
| Fig. 25 Colorimetric array response for 24 representatives of less responsive organic compounds at their vapor pressure at 295 K; color range expanded from 4 to 8 bits per color (8–17 expanded to 0–255). Reprinted with permission from ref. 523. Copyright (2006) American Chemical Society. | |
Detection and quantification of NH3, NO2 and H2S was investigated by a colorimetric array with different chemo-responsive dyes.527,528 Significant color changes upon exposure to defined concentrations of each analyte were observed. The extrapolated limits of detection for NH3, NO2 and H2S were 0.08, 0.03 and 0.06 ppm, respectively,527 values significantly below the permissible exposure limits of the gases. Sen et al.529 detected 0.05–50 ppm H2S at room temperature with an array of chemo-responsive dyes, with an exposure time of up to 30 min to reach equilibrium. PCA analysis gave a 100% classification at all concentrations tested. A polymeric optical waveguide based on PET foils for detection of 5–20 ppm NH3 has been recorded.530 The planar waveguide configuration was intended to improve the transducer sensitivity and simplify the fabrication process. The sensor had fast response times and good sensitivity to the gas. Endo et al.531 have described selective detection of benzene, toluene, acetone and xylene by a colorimetric sensor based on a glass substrate with a 3D colloidal crystal and PDMS elastomer. A polydiacetylene/GR-stacked composite film-based colorimetric sensor was tested with 0.01–10% of a variety of VOCs, including methanol.532
Humidity detection by a colorimetric sensor has also been reported.533 A carbon-coated copper/polymer porous hybrid film was synthesized for humidity sensing resulting from plasmon resonance and interference. No change in color was observed up to 50% RH. At approximately 65% RH, the color of the film shifted to green and the absorption peak also shifted. At 70% RH, the color of the film shifted to blue. The absorption shifted by 50 nm per 1% increase in RH from 70 to 80% RH.
4.9. Optical sensors
Optical fibers seem to be promising gas sensors.534,535 These sensors can be characterized by the location at which the transduction between light and measurement takes place, namely inside or outside the fiber, termed intrinsic and extrinsic, respectively.534 Intrinsic optical fibers are more frequently used for detection of gases and VOCs, as a passive layer of the fiber is replaced with a small segment of gas-sensitive nanomaterials, such as polymers,536–543 GR544–547 and MOs.534,535,548 Upon exposure to gases, optical and/or structural changes occur in the reactive layer of the fiber, which evoke a change in the reflective index of the optical fiber changing its transmission properties.534 Sensitivity of optical fibers to gases can be increased by bending or using hollow core fibers, resulting in an increase in the penetration depth in the sensing area by increasing the coupling of the optical power in the evanescent field.534,536,547,549 Optical fibers have short response and recovery times, along with on-line monitoring possibilities.534,535,538
4.9.1. Metal oxide-based optical fiber.
Bismuth oxide (Bi2O3)-clad modified optical fibers were used to detect 100–500 ppm methanol, acetone, NH3 and ethanol at room temperature.548 There was gas selectivity for methanol, ethanol and NH3, with sensitivities of 212 × 10−3 kPa−1, 109 × 10−3 kPa−1 and 15 × 10−3 kPa−1, respectively.
4.9.2. Polymer-based optical fiber.
A U-bent optical fiber sensor modified with an organic–inorganic hybrid of PAH/silica NPs and infused with tetrakis (4-sulfophenyl)porphine (TPSS) was tested as a vapor sensor (Fig. 26).536 The sensor was exposed to different alcoholic vapors and water. As expected, TPSS worked as a selective absorption site for methanol, leading to the highest response. Silva et al.537 reported on an optical fiber coated with a sensitive film of poly[methyl(3,3,3-trifluoropropyl)siloxane] for detection of pentane, heptane, octane, decane, benzene, toluene and other VOCs in human breath at room temperature. Detection limits ranged from 0.8 pmol L−1, for heptane to 9.5 pmol L−1 for decane. The fiber responses also had high linearity and stability. In a further study, a portable optical fiber coated with the same polymer was used to detect benzene, toluene, ethylbenzene, p-xylene, m-xylene and o-xylene in real-time monitoring.538 The fiber had high sensitivity to the vapors tested, with high linearity and detection limits in the range of a few nanograms. In yet another study, an optical fiber was coated with a vapochromic material for sensing acetone and dichloromethane.550 Changes in the transmitted optical power were up to 13.5 dB as a result of exposures to different concentrations of the vapors. Elosúa et al.540 could detect ethanol, methanol and isopropanol with a thin layer of a different vapochromic material on an optical fiber. Changes up to 3 dB in the reflected optical power were found upon exposure to 21.5–125 mmol L−1 gas. A lutetium bisphthalocyanine thin layer was also used as a sensitive layer on an optical fiber for detection of a variety of vapors at room temperature.541 The fiber was exposed to acetic acid, ethanol, n-butyl acetate and hexanal at different concentrations in the range from 4 to 88 mmol L−1. As expected, the highest attenuation of the reflected optical power was seen with acetic acid, the most oxidant compound, with a variation of 9.5 dB at the highest concentration. For sensitive detection of aromatic vapors, an optical fiber coated with calixarene has been tested.542 Once the nanoscale cavity in the calixarene has entrapped a VOC molecule, there is a change in the refractive index. This fiber had been exposed to toluene, benzene, cyclohexane and hexane. As expected, the fiber gave the highest response to toluene and the lowest to hexane. Sensitivity to toluene of 231 ppm was reached, while ensuring a reversible response with a fast recovery time. An electrospun polypyrrole–polyethylene oxide coated optical fiber was fabricated and exposed to different VOCs, including 0.1–10 ppm NH3, 6–10 ppm acetone and 2–10 ppm methanol and ethanol.543 The sensor was highly sensitivity to ammonia, methanol and ethanol vapors, but less to acetone.
 |
| Fig. 26 (a) Schematic of a U-bent optical fiber and the coating process of a TSPP-infused PAH/SiO2 alternate thin film. (b) Changes in the transmission spectra of the U-bent OF with a TSPP-infused (PAH/SiO2)10 film after exposure to water and alcohol vapors. (c) Dynamic responses at 700 nm of the sensor after exposure to water and alcohol vapors. Reprinted from ref. 536 with permission from Wiley-VCH, copyright 2017. | |
4.9.3. Graphene-based optical fiber.
A reduced GR oxide coated hollow core fiber exposed to 30–90% RH547 showed sensitivity of up to 0.22 dB/% RH, with good repeatability and a fast response time. A reduced graphene oxide coated PMMA optical fiber proved sensitive to 0–500 ppm ethanol and methanol at room temperature.544 The sensor had good sensitivities of 0.065 and 0.038 counts per ppm ethanol and methanol, respectively. Optical fibers based on Pt NP-incorporated graphene oxide possessed high sensitivity to 0–120 ppm NH3 at room temperature.545 The Pt-decorated sensor had a sensitivity of 10.2 pm ppm−1, 3 times higher than the sensitivity of a fiber based on reduced Gr alone. An optimal concentration of Pt NPs was 185.2 mg L−1. A linear response was obtained for NH3 concentrations <80 ppm. In another study, optical-fibers based on different concentrations of Ag NPs on exfoliated graphene oxide sheets were tested for detection of 0–500 ppm NH3.546 The optimal concentration was 0.1 M AgNP, which yielded 1.5 and 2.2 times higher sensitivity than 0.6 M AgNP/Gr oxide composite and reduced graphene oxide coated sensors, respectively.
5.
In vitro-based disease diagnostics
5.1. Cancerous diseases
5.1.1. Lung cancer.
5.1.1.1. Monolayer-capped metal nanoparticle- and carbon nanotube-based sensors.
Many studies have reported the ability of VOCs to serve as biomarkers for lung cancer based on histologic and genetic classification after analysis of the headspace of cancer cell lines by different analytical methods.551–557 However, not many have dealt with the ability of cross-reactive nanomaterial-based devices to discriminate between lung cancer cell lines with different histologies and genetic mutations. Comprehensive in vitro studies conducted by Barash et al.558 reported 100% accuracy for discriminating between 7 different non-small cell lung cancer (NSCLC) cell lines from their growth medium using an array of cross reactive nanomaterial sensors based on organically modified GNPs. A volatolomic signature obtained by applying the PCA algorithm on the multidimensional responses of the nanoarray also successfully discriminated between breath prints of LC patients and healthy controls.558 In a complementary study, the same NSCLC cell lines could be successfully discriminated from immortal bronchial epithelium (IBE) cell lines, which served as healthy controls, with 96% sensitivity, 86% specificity and 93% accuracy. NSCLC cell lines were also distinguished from small cell lung cancer (SCLC) cell lines with 100% sensitivity, 75% specificity and 96% accuracy. Furthermore, an artificial intelligence nanoarray could discriminate sub-histologies of NSCLC cell lines with 90% accuracy by applying support vector machine (SVM) cross-validation (Fig. 27).559
 |
| Fig. 27 SVM analysis from the GNPs’ multidimensional data output for optimal separation between headspace samples from: (A) LC and IBE cells; (B) SCLC and NSCLC; and from (C) subcategories of NSCLC (i.e., adenocarcinoma and squamous cell carcinoma cells). The pseudo-3D representation of the selected features illustrates the separation between histologically different LC subtypes. F1 is the ΔRstart, F2 is the ΔRmid, F3 is the ΔRend, F4 is the ΔR/R0, and F5 is the area under the normalized response signal (note: ΔR is the net resistance change upon exposure and R0 is the baseline resistance). S1–S4 refers to the sensors being tested. Reprinted from ref. 559 with permission from Elsevier, copyright 2012. | |
In a different study, DFA analysis of the same cross-reactive nanomaterial-based device resulted in accuracies varying between 84 and 96% for genetic mapping of 3 selected genes related to targeted therapy in LC: EGFR, KRAS and EML4-ALK fusion.560 Headspace samples of 6 cell lines representing EGFRmut oncogene, 4 representing mutant KRAS, one cell line with EML4-ALK fusion and 7 lung cancer cell lines of wild type (WT) to all 3 tested genes were collected. Nine volatolomic signatures of the GNPs’ responses were constructed using a DFA model by comparing each mutation with a large group of WT cells, between every 2 mutations, and between each mutation and a small group of lung cancer cell lines, which were WT to all. Classification success of the global 9 test array was calculated by global leave-one-out cross validation, giving 76% accuracy (Fig. 28).560
 |
| Fig. 28 DFA plots of CV1 calculated from the responses of GNP sensors to the headspace samples of NSCLC cell-lines having EGFRmut, KRASmut or EML4-ALK oncogenes, or genetic mutations that are WT to the former 3. The standard deviation of the CV1 values is represented by the error bars. The boxes represent the 95% CI of the CV1 values, corresponding to 1.96 × SE. Subpanels A.1–A.3 represent the 3 primary general DFA models applied simultaneously to the data and contain all 37 headspace samples. Each point represents one sample. The samples were obtained from multiple cell-lines having the oncogenes of interest. Reprinted from ref. 560 with permission from Elsevier, copyright 2013. | |
The above-mentioned studies were conducted on lung cancer cell lines, which provide an excellent model for simulating LC diversity; however, in aiming to assign specific volatolomic signatures to distinct genetic mutation, a more precise and delicate model is required. For this purpose Davies et al.561 have developed a human bronchial epithelial cell (HBEC) model. HBEC provides a more traceable system to follow the effect of minimal genetic alterations by using the parental cell line in which each additional genetic lesion can be studied. With this in mind, cancer cells bearing either KRAS mutation knock-down of T53 or both were compared. Headspace samples were analyzed by means of artificial intelligence nanoarray in combination with DFA, resulting in accuracies above 77% in multiple comparison analysis.561 To increase the discriminative power in this study, a combined array of organic-coated GNPs and organic-modified CNTs was used. DFA results demonstrate eNOSE ability to differentiate between lung cancer cells with minimal genetic alterations based on volatiles emitted into their microenvironment. The volatolomic signature can now be translated into barcodes to provide an easy and accurate tool for genetic mapping of LC, which can be used in the clinics for biopsy classification, testing tumor margins and other purposes.
5.1.1.2. Polymer composite.
In a different study, LC sub histologies, including 4 types of NSCLC, 1 type of metastatic squamous cell carcinoma of the lung and 1 mesothelioma, were successfully classified and discriminated from each other as well as from normal fibroblasts and smooth muscle cells by applying PCA analysis on responses obtained from a polymer composite electronic nose.562 The electronic nose classification success was estimated by calculating Mahalanobis distances, which were in most cases >3, indicating distinct volatolomic signatures of the different aerodigestive track malignant cell lines.562
5.1.2. Breast cancer.
5.1.2.1. Metal oxide sensors.
Many studies have proven the efficiency of breast cancer screening by the analysis of breath VOCs. However, only one in vitro study was reported on breast cancer cell lines, suggesting that VOC detection might provide an insight into the biochemical and tumorigenic characteristics of the disease.563 By using a self-temperature modulated metal oxide semiconductor gas sensor, researchers could distinguish between the headspace VOCs of non-transformed breast control cells MCF10a, 5 transformed breast cancer cell lines MDA231, culture media without cells and distilled water (Fig. 29). Breast control cell lines were also discriminated from 5 different types of cancer cell lines (MDA231, MCF-7, ZR751, BT474, SKBR3) by applying a PCA model. The PLS-DA classification model resulted in 88% sensitivity and 80% specificity for the discrimination between cancer cells and non-transformed controls.563 Interestingly, the PLS-DA model could detect breast cancer cell lines with low cell doubling times and those positive to estrogen receptor with 88% and 85% accuracy, respectively. Breast cancer cell lines expressing progesterone receptor (PgR) and HER2 overexpression were classified with lower accuracies (<75%). These results suggest the possibility that headspace analysis provides cost-effective prognostic and therapeutic molecular information that might have a huge impact on the clinical outcomes due to accurate and fast tumor genetic mapping.563
 |
| Fig. 29 (A) Schematic of the self-modulation sensor. (B) Circuit implementation used in this work; (C) from top to bottom, examples of output signal, temperature modulation signal and sensor temperature. Reprinted from ref. 563 with permission from Nature Publishing Group, copyright 2015. | |
5.1.3. Prostate cancer.
5.1.3.1. Metal oxide sensors.
Since prostate cancer is the most common cancer in men worldwide and as the performance of prostate specific antigen tests is not optimal, there is a need for a new biomarker. An in vitro study of telomerase-immortalized prostate epithelial cell line EP-156T and prostate adenocarcinoma cell line LNCaP was conducted to test the ability of an electronic nose to distinguish between benign and malignant prostate cell lines. The e-nose device consists of an ion mobility cell, which consists of 8 electrode strips and a metal oxide semiconductor cell.564 PCA analysis was used for dimension reduction and visualization of the sensor's responses. Linear discriminate classifiers were used to distinguish between cell lines and water, cell lines from media, and between benign and cancer cells. M-fold cross validation was used to calculate misclassification rates (MCRs), which ranged from 1.4 to 1.7%. Discrimination between the malignant and nonmalignant cell lines resulted in 2.6–3.6% MCRs. Testing of cell lines and empty media gave a definite conclusion, i.e. that cells modify the volatolomic signature of their media.564
5.1.4. Hepatocellular carcinoma.
5.1.4.1. Monolayer-capped metal nanoparticle- and carbon nanotube-based sensors.
In a headspace study of 36 cell cultures with high metastatic potential (HMP) and low metastatic potential (LMP), and from normal cells, samples were collected and analyzed by means of nanomaterial based sensors with DFA as the pattern recognition algorithm. Classification success was determined by leave-one-out cross-validation. The artificial intelligence nanoarray used was composed of cross-reactive chemiresistors based on organic functionalized GNPs and SWCNTs.565 Resistance recording from different combinations of sensors in the array served as the input to the DFA model, which resulted in 97% accuracy for the discrimination of HCC cell lines from normal cell headspace samples. Accuracy was >96% for the comparisons of HCC and LMP compared to normal cells. The comparison between HCC-LMP and HCC-HMP was 96.7% accuracy, with 83% sensitivity and 100% specificity.565
5.1.5. Gastric cancer.
5.1.5.1. Multi-walled carbon nanotube/Au–Ag electrochemical biosensor.
Headspace VOCs of MGC-803 gastric cancer (GC) cells and GSE-1 gastric mucus cells were sampled and analyzed by a MWCNTs/Au–Ag electrochemical biosensor with different modified electrodes (MWNTs/Au–Ag/glass carbon electrode (GCE), MWNTs/Ag/GCE, MWNTs/Au/GCE, MWNTs/GCE and bare GCE).566 Preliminary experiments with the different electrodes suggested that the MWCNTs/Au–Ag nanocomposites formed a more efficient electrode sensing film than other nanomaterials, which can be explained by the improved absorbing properties of MWCNTs/Au–Ag/GCE due to a synergistic effect. The different I–V curves from the GC cells, the mucosa cells, and the cell-free medium were recorded and compared; clear differences were seen between the curves. The investigators also exposed the electrochemical sensors to 3-octanone and butanone, found only in the headspace of the GC cell lines. Results of the simulated gas exposure might explain the excellent separation between the I–V curves of the 2 types of cell lines, implying that butanone and 3-octanone had more electrochemical activity compared to other compounds, as detected by GC-MS.566
5.1.6. Brain cancers.
5.1.6.1. Polymer–carbon black composite films.
Odor signatures of organs taken from chicken, such as liver and heart, as well as human glioblastoma (U251) and human melanoma tumor (A2058) cell lines, have been investigated. Headspace VOCs were analyzed by an electronic nose composed of 16 sensors with different coated polymer–carbon black composite films.567 Comparison between glioblastoma and melanoma tumor cell lines and their medium resulted in 44% and 77% accuracies, respectively. Comparing the initial number of cultured cells, melanoma cell lines did not produce any significant difference in volatile signature, whereas there was a 49% variation for the glioblastoma cells. However, successful variation of the headspace signature was achieved when comparing the 2 types of cancer cells, as also in comparing the 2 types of fresh organ tissues.567
5.1.7. Melanoma.
Skin cancer is the most common form of cancer in the United States. Melanoma is the deadliest of skin cancers, as it can rapidly spread to distant parts of the body. The incidence of melanoma has risen rapidly over the past 30 years; the rate of diagnosis has increased by 2–3% per year from 2004 to 2013 among both men and women aged 50 or older,568 with an associated economic burden estimated at $932.5 million per year across all age groups.569 Early detection of melanoma is critical for improving survival rate: if detected when still confined to the primary site, the melanoma 5 year survival rate is 98.2%.570
5.1.7.1. Quartz crystal microbalance sensors.
An array of 7 QCM chemical sensors coated with different metalloporphyrins has been used for identifying unique fingerprints for nevus and melanoma based on headspace samples.571–573 Three melanoma cancer cell lines, a synovial sarcoma and a thyroid cancer cell line were grown on a Petri dish covered with stainless-steel cylinders for headspace collection.571 Score plots of the first 2 principal components (PCs) obtained from the PCA model based on responses of the QCM sensors to the headspace discriminated well between melanomas and other tumor cell lines (Fig. 30). In a different study by this group, 4 QMB sensors coated with different metalloporphyrins could distinguish melanoma cell lines from different tumor cells by applying the PCA algorithm.572 These results imply that there is a unique volatolomic signature produced by melanoma cells that elicits response from the metalloporphyrin-coated sensors and suggests the potential use of these sensors for mole detection straight from the skin. To establish their hypothesis, these investigators also ran in vitro trials by smelling melanoma xerographs from nude mice in an in vivo experiment.573In vivo data led to the conclusion that tumor xerographs produce a distinctive VOC pattern that can be differentiated from the volatolomic signature of a tumor-free control. Thus, an appropriate and well-tuned sensor array is capable of detecting and monitoring tumor growth by continually analyzing headspace VOCs.
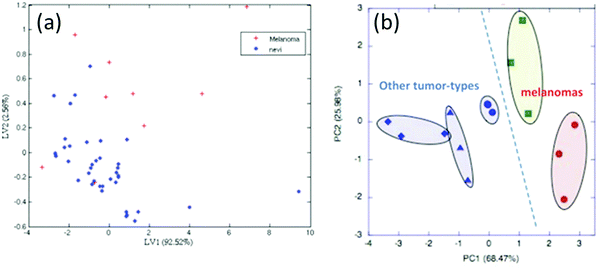 |
| Fig. 30 (a) Score plot of the first 2 latent variables (LVs) of the PLS model built on the data collected from in vivo control and melanoma skin lesions. Good discrimination between the 2 groups I seen, and is confirmed by a correct classification percentage of 87%; (b) score plot of the first 2 PCs of the PCA model built on the cell culture headspace measurements. A good discrimination can be seen between melanomas and other tumors along the principal component 1 (PC1) (68.5% of the total variance). Reprinted from ref. 571 with permission from Elsevier, copyright 2009. | |
5.2. Detection of bacteria
5.2.1. Metal-oxide sensors.
Accurate and fast bacterial identification may be of considerable importance both in medicine and industry. Clinician prescriptions of appropriate and the most suitable antibiotic treatment are dependent on bacterial identification, which can take a long time. During this delay, the infection may spread and as a consequence sepsis continues to be one of the leading causes of death in USA and Europe. In industry, regulatory processes may cause unnecessary delays due to bacterial infections.574–576 This is the reason for many studies using different electronic noses for headspace detection of different pathogenic bacteria.575,577–585 In one study, headspace samples (360 samples per bacterium) of 2 pathogenic microorganisms, Escherichia coli (E. coli) and Staphylococcus aureus (S. aureus), were successfully discriminated using an array of 6 different metal oxide semiconducting gas sensors selected to target hydrocarbons, alcohols, aldehydes/heteroatoms, polar and non-polar molecules.586 Samples were classified by applying multi-layer perceptron (MLP) with a back-propagation (BP) learning algorithm. The best performance of the MLP resulted in correct classification of 96.1% of all test vectors, with only 2.2% incorrectly classified and 1.7% unknown.
5.2.2. Electroconductive polymers.
Furthermore, an array of 14 electroconductive polymer, semi-micro chemoresistive sensors could differentiate between 12 different bacteria and one pathogenic yeast with a 93.4% classification success by applying a neutral network.580 An array of 8 QCMs, 8 MOs and 4 electrochemical gas sensors was used to discriminate between E. coli and Enterobacter aerogenes. PCA analysis was applied to discriminate between the different bacteria and between different periods during bacterial growth.582
5.2.3. Colorimetric sensor array.
In another study, E. coli, Pseudomonas aeruginosa, S. aureus and Moraxella catarrhalis were discriminated by means of a colorimetric sensor array with 100% accuracy using a logistic regression classification algorithm for the sensor's responses to headspace samples.581 These results imply that different bacteria produce distinct volatile metabolites due to different enzymatic pathways, resulting in unique volatolomic signatures in their headspace samples. In a further study using disposable colorimetric sensors, researchers discriminated not only between different bacterial species, but between different strains of the same species. All strains and their antibiotic resistant forms were successfully classified with 98.8% accuracy within 10 h (Fig. 31).577 Whereas in most studies a single headspace sample taken from a closed environment is examined, in this research the VOCs produced by the bacterium were also tested over time, indicating different phases of the bacterial growth.
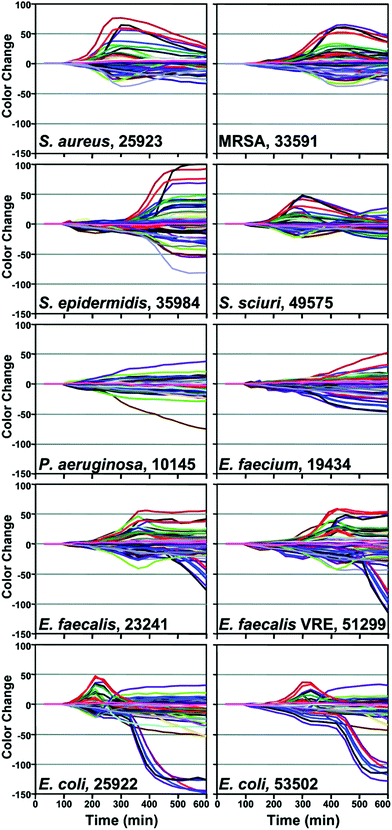 |
| Fig. 31 Time response profiles for 10 different bacterial strains (name and American Type Culture Collection number given in each panel). For each bacterial strain, the color change values versus time are plotted for all color channels at each time-point. Reprinted (adapted) with permission from ref. 577. Copyright (2011) American Chemical Society. | |
5.3. Tuberculosis
5.3.1. Electroconductive polymer.
Few studies have reported the ability of electronic noses to identify Tuberculosis (TB) through detection of headspace VOCs in an in vitro setup. It was reported elsewhere that 37 headspace samples of TB-positive and TB-negative cultures were analyzed by means of an electronic nose composed of 14 conducting polymers.587 Sixty-one samples previously clinically diagnosed as positive or negative by conventional microbiology methods were analyzed after headspace sampling. A neural network differentiated between M. tuberculosis and a sterile culture with a 100% prediction rate. DFA analysis was applied to check the model's ability to detect blind samples, which also resulted in 100% accuracy. In a second trial, discrimination of 96% between 61 samples with M. tuberculosis and related species was achieved by applying the neural network algorithm. DFA analysis was also applied to check the model on a blind sample, which gave absolute identification of the blind samples. Applying the neural network to sensor parameters obtained from exposures to sputum samples resulted in a prediction rate of 96%.
In a different study by Fend et al.588 a gas sensor array composed of 14 conducting polymers detected 3 different Mycobacterium species (M. tuberculosis, M. avium, and M. scrofulaceum) and Pseudomonas aeruginosa in the headspace of cultures, and could differentiate between them and empty medium. Furthermore, applying the DFA model on the first 4 PCs obtained through PCA analysis resulted in clear differentiation between the 3 Mycobacterium spp. Blind analysis of 15 samples gave 100% accuracy.
In a complementary study in which negative pooled sputum samples were spiked with the 3 different bacteria, the electronic nose discriminated between them with 100% success. For sensitivity calculation, 6 different concentrated M. tuberculosis suspensions were analyzed. In this experiment, 2 of the 14 blind samples were incorrectly classified, resulting in a high detection limit of 1 × 104 mycobacteria per mL. In a complementary spiked sputum trial, all 12 blind samples were correctly identified. The performance of the electronic nose was validated from 330 clinical samples of 50 positive control samples, which consisted of non-TB sputum samples spiked with 108M. tuberculosis cells per mL, 50 negative non-TB sputum samples from Netherlands, and 280 samples from Africa. Good separation was obtained between TB-positive and TB-negative samples by applying PCA analysis.588
6. Breath-based disease diagnostics
6.1. Cancerous diseases
6.1.1. Lung cancer.
6.1.1.1. Monolayer-capped metal nanoparticle- and carbon nanotube-based sensors.
Many studies have shown the ability of an artificial intelligence nanoarray composed of GNP chemiresistors in the screening, detection, classification and genetic mapping of lung cancer through extended breath analysis from hundreds of volunteers. A series of studies conducted by Haick et al. on a monolayer gold nanoparticle sensor array have proven the ability of an electronic nose to discriminate between early and late stages of LC with 88% accuracy, and between different histologies (e.g. SCLC and NSCLC) and sub-histologies (e.g. adenocarcinoma and squamous cell carcinoma) of LC with 93% and 88% accuracy, respectively.7,17,191,589 In a recent study of Shlomi et al.590 19 LC patients harboring the EGFR mutation were successfully discriminated from 34 LC patients who were EGFR wild type with 83% accuracy, 79% sensitivity and 85% specificity. Furthermore, early LC patients (n = 16) were discriminated from patients with benign nodules (n = 30) with 87% accuracy, positive predictive (PPV) and negative predictive values (NPV), using an array of 40 cross-reactive chemiresistors, based on organically functionalized GNPs, as well as SWCNTs, capped with PAHs.590 In another study, 144 breath samples were collected from 39 advanced LC patients. The investigators could discriminate between LC patients after surgery and monitoring response to therapy using only one GNP sensor with only 59% accuracy. However, DFA analysis of the collective responses obtained from the nanoarray had the ability to monitor changes in tumor response across therapy and also indicate lack of any further response to therapy with 85% success.591 Using the same array of GNPs, the researchers could detect LC at early onset and monitor breath volatolomics after LC resection. DFA maps clearly differentiated between LC patients and volunteers with benign nodules before and after surgery. Breath prints of LC volunteers before resection were clearly distinguished from those of patients after surgery. Moreover, clear discrimination was obtained between patients with benign nodules pre- and post-surgery (Fig. 32).7 Furthermore, 4 of the most common cancers in developed countries – lung, breast, colorectal and prostate – were compared and could be discriminated from each other, as also from healthy states, regardless of some confounding factors (including age, gender, and smoking habits). PCA analysis of GNPs’ responses to 5 breath fingerprints with minimal overlap between them indicates a high potential of these sensors to serve as first-line tools in cancer screening.592
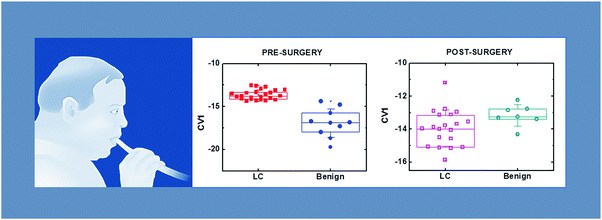 |
| Fig. 32 DFA models that discriminated between lung cancer (LC) and benign states before surgery, and LC and benign states after surgery. Each point represents a single breath sample; 1–2 breath samples were collected per patient. Reprinted from ref. 7 with permission from Elsevier, copyright 2013. | |
6.1.1.2. Polymer composite sensors.
Breath signatures of LC patients obtained from the PCA plot of the Cyranose 320 responses could successfully be distinguished from those of subjects with chronic obstructive pulmonary disease (COPD).593,594 About 85% of the samples were correctly classified by linear canonical discriminate analysis with 3.73 Mahalanobis distance between the group means.593 Furthermore, breath signatures of LC patients could be discriminated from healthy controls with 90% accuracy and 2.96 Mahalanobis distance. In another study, breath signatures of 14 individuals with bronchogenic carcinoma were discriminated from those of 45 healthy controls using an electronic nose composed of 32 polymer composite sensors.594 The applied mathematical algorithm used for analyzing the sensor responses was PCA in combination with Mahalanobis distance for discrimination between the classes. A separate group of 76 volunteers (14 with cancer and 62 tumor-free) was used for constructing a cancer prediction model by the SVM algorithm, which gave 71.4% sensitivity and 91.9% specificity.594 However, since the data were collected and validated on patients with relatively advanced LC, there is a need to modify the model to be more suitable for a wider population.
6.1.1.3. Quartz crystal microbalance sensors.
In a separate study, a total of 42 breath samples were collected from patients with different types of lung cancer. An electronic nose composed of 8 QCM sensors coated with different metalloporphyrins was used to detect cancer-diseased patients, and distinguish between cancer-diseased and post-surgery patients, by applying PLS-DA on the sensor's response.595 A total accuracy of 90.3% was achieved with 100% of the patients, with LC being correctly identified. In following research, the same set of porphyrins coating 8 QCM sensors together with the PLS-DA algorithm for data classification was used.596 For this purpose, breath samples from 36 healthy controls, 28 patients with LC and 28 patients with diverse lung diseases were collected. Classification between LC patients and healthy controls, as well as between lung cancer and other lung disease patients, as also between LC and lung disease together with healthy controls was successfully achieved with 85, 92.8 and 89.3% sensitivity, respectively. Artificial cancerous breath samples were also generated by adding 3 suspected volatile biomarkers to the breath of 2 healthy controls. In this case, the sensor's response to the healthy volunteers drifted significantly towards the values obtained for the lung cancer signatures, but they did not overlap. In a separate study, QCM sensors were used to analyze the content of the breath collected from 30 subjects who consented to the breath test.597 Among them, 10 formed the control group and 20 subjects with radiological lesions were divided into adenocarcinoma (ADK) and squamous cell carcinoma (SCC) groups. Breath samples were collected both in the traditional manner using collection bags and by pulling excess air during bronchoscopy directly to the electronic nose. Two PLS-DA models were built, aiming to differentiate between LC patients and healthy volunteers, as also between LC histologies, i.e. ADK and SCC. Good capability of the sensor array for the discrimination of LC from healthy controls was achieved, both using the breath from the bags and the breath taken straight from the lungs, with the latter having better performance in terms of classification success. For LC histologic classification the PLS-DA model, based on breath taken from the lungs, provided a classification rate of 75%, which is much higher compared to that achieved by analyzing data collected from a breath bag.
6.1.1.4. Surface acoustic wave sensors.
An electronic nose system composed of 2 SAW sensors working together as the detector, out of which one was coated with a polymer film of poly isobutylene and the other worked as a reference, in combination with improved ANN, was used for noninvasive diagnosis of lung cancer.598 For this purpose, breath samples were collected from 42 volunteers, including 15 healthy subjects, 20 LC patients and 7 chronic bronchitis patients. SAW sensors were calibrated using 11 selected VOCs representative of LC detection as follows: a sample with none of the 11 VOCs corresponds to healthy subjects; if one of these VOCs appears in the sample, it is defined as suspected, and if more than one of the VOCs appear in the sample, the output is lung cancer. Breath samples were collected into 2 Tedlar bags, and were then absorbed on Solid-Phase Microextraction (SPME) fibers for pre-concentration. The extracted VOCs from the TD column were absorbed on the polymer-coated SAW sensor one by one, followed by recording 2 parameters: the frequency response (Hz) and the corresponding retention time. Results from the SAW sensors were translated into 6000 virtual sensor array responses. For patient diagnosis, an image method that combines frequency responses and retention times was applied together with BP-ANN (Fig. 33). For prediction purposes, breath samples from 5 LC patients and 5 healthy volunteers were collected and analyzed by BP-ANN. Results of this blind test showed that 8 of the patients were correctly classified, with one LC patient and one healthy subject being classified as suspect.
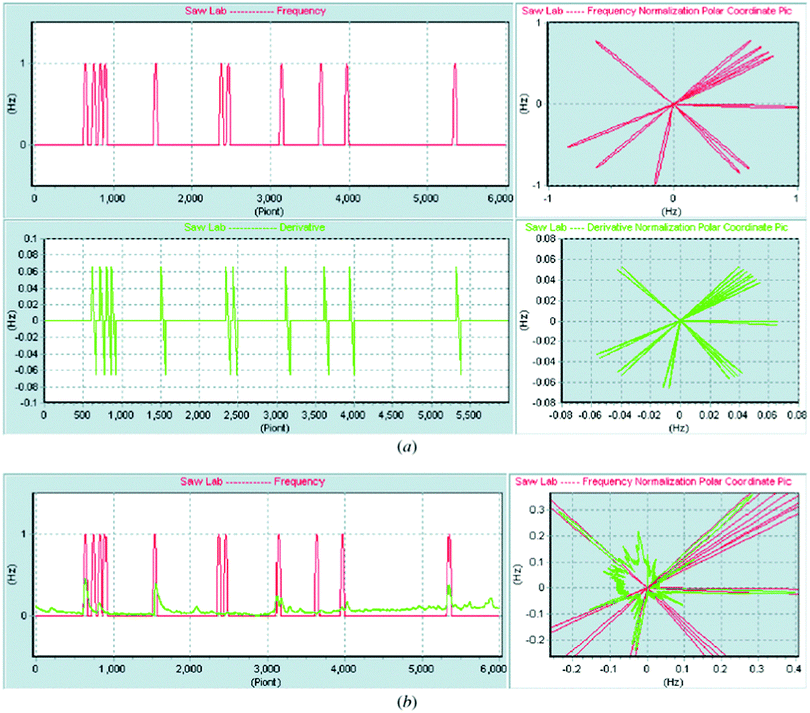 |
| Fig. 33 An image method for lung cancer diagnosis based on a virtual SAW gas sensor array. (a) Ideal response of lung cancer patients, and (b) image response of a lung cancer patient overlapped with the ideal response. Reprinted from ref. 598 with permission from IOP Publishing, copyright 2005. | |
6.1.1.5. Colorimetric sensor array.
In Mazzone et al.,599 a colorimetric sensor array was designed for noninvasive LC detection through exhaled breath. NSCLC patients (n = 49), controls bearing different pulmonary diseases (n = 73) including COPD, sarcoidosis, pulmonary arterial hypertension and IPF, and healthy volunteers (n = 21) were enrolled. Each colorimetric sensor array was composed of 36 chemically sensitive spots with different sensitivities to VOCs. Each sensor in the array responded in a change in color, which was further analyzed into its RGB components and then to numerical values. Data collected from the sensor array were analyzed by the random forest method using 70% of the data as training, which resulted in 73.3% sensitivity and 72.4% specificity for the diagnosis of LC. In a following study, the contents of exhaled breath taken from 229 volunteers, divided into biopsy-proven untreated LC patients, a control group of individuals at high risk to develop the disease, and individuals with indeterminate lung nodules, were examined using a colorimetric sensor array.600 Breath signatures of 8 binary groups were compared for identification and characterization of lung cancer with high sensitivities and specificities of >70%. Models developed for discriminating between LC histologies had higher accuracy (90% sensitivity) than those aiming to compare LC patients and healthy volunteers (70% sensitivity). Furthermore, patient characteristics had no influence on the accuracies of the models comparing different features of LC (histology, stage and survival).
6.1.2. Mesothelioma.
6.1.2.1. Polymer composite sensors.
In a study using Cyranose 320, the detection of mesothelioma (an aggressive cancer mainly related to asbestos exposure) was reported.601 For this purpose, breath samples were collected from 42 healthy people, 20 volunteers with malignant mesothelioma (MM) approved on the basis of immunohistological diagnosis, 5 volunteers with asbestosis, and 13 with other pleural diseases. The study was conducted in 2 phases: a training phase used to build the model based on PCA analysis, and a blind phase to validate the strength of the model. The Mahalanobis distance between the mean of the groups was also calculated. The results of the training set, which included 10 MM and 10 controls, showed 95% accuracy and a Mahalanobis distance of 4.59. Blind test results (including 10 MM and 32 healthy controls) reached 90% sensitivity and 91% specificity.
6.1.3. Breast cancer.
6.1.3.1. Monolayer-capped metal nanoparticle- and carbon nanotube-based sensors.
Using an array of cross-reactive GNPs, it was possible to discriminate between 36 female volunteers: 7 were with negative mammography, 16 with benign breast conditions, and 13 women with malignant breast lesions.602 The first principal component could discriminate between healthy volunteers and women with breast cancer, as well as between women who were negative in mammography and women with benign or malignant lesions. Statistical differences in the tested group were also evaluated by applying Student's t-test for PC1, giving p < 0.05 for all comparisons. SVM classification provided 94% sensitivity and 80% specificity for breast cancer screening. In a complementary study from the same group, 276 breast samples were collected from healthy volunteers, women with benign breast lesions and women with breast cancer, characterized by different molecular sub-types.603 DFA analysis of responses obtained from the GNP sensor array indicated 83% accuracy in the blind test for discriminating breast cancer from non-cancer cases (including healthy volunteers, benign lesions and DCIS). The DFA model sensitivities and specificities for breast cancer molecular sub-types (viz. Luminal A, Luminal B, Triple Negative, HER2+, HER2 equivocal) vary from 81 to 88%, and 76 to 96%, respectively.
6.1.4. Ovarian Cancer.
6.1.4.1. Monolayer-capped metal nanoparticle-based sensors.
An array of 6 modified GNP sensors was used to discriminate between 48 women bearing positive OC, 86 women with benign genital tract neoplasia and 48 tumor-free controls.203 In this study, the DFA algorithm was applied for multiple binary comparisons of OC compared to non-cancer subjects, and to OC compared to tumor-free cases. Furthermore, early stages of OC were compared to advanced OC, as also to tumor-free volunteers. Classification success of the DFA models for the nanoarray analysis provided accuracies varying between 75 and 90% for the training run, and between 58 and 89% for the blind tests. Furthermore, diagnosis of OC from exhaled breath has recently been accomplished using a dynamic integrated array of flexible sensors based on modified GNPs.156 For this purpose, breath samples were collected from 43 subjects: 26 were healthy controls and 17 were women previously diagnosed with epithelial OC. Since each sensor in the array can be bent multiple times, it was possible to extract numerous bending-related features from each sensor separately; hence, each sensor acted as a stand-alone array. As a result 82.3% sensitivity, 84.6% specificity and 83.7% accuracy were achieved by applying leave-one-out cross-validation on the DFA model calculated from only single sensor responses. Further verification of the results was obtained by applying k-Fold (k = 4) analysis, which gave sensitivity, specificity and accuracy >80%.
6.1.5. Gastric cancer.
6.1.5.1. Monolayer-capped metal nanoparticle- and carbon nanotube-based sensors.
Monolayer capped GNPs were used to distinguish between GC patients (n = 37) and benign gastric conditions (n = 93), and between early (n = 17) and advanced stage (n = 18) GC.604 Among the benign conditions, patients suffering from ulcers (n = 32) were distinguished from those with less severe conditions (n = 61). For the first comparison, DFA analysis of the responses obtained from the GNP array resulted in 89% sensitivity and 90% specificity. Comparison of the different stages of GC gave 89% sensitivity and 96% specificity in a blind test. Finally, the volatolomic signature of the benign conditions was 86% accurate. In a following research exercise, an array of artificially intelligent GNPs and SWCNTs coated with different organic ligands successfully distinguished binary comparisons of breath signatures collected from GC patients (n = 99; 36 at stages I–II, 59 at stages III–IV), pre-malignant lesion patients (n = 53), 7 patients with low grade dysplasia, and 325 cases with the following operative link on gastric intestinal metaplasia (OLGIM) distribution: 155-OLGIM 0, 136-OLGIM I–II and 34 OLGIM III–IV.605 ROC curves based on canonical variables of the DFA model were plotted and gave calculated AUCs of 76–98% in the different binary comparisons. Potential confounding factors – including H. pylori, gender, age, smoking habits and alcohol consumption – were also tested to validate the strength of the calculated DFA model. TP, FN, TN and FP were also validated based on blind experiment. The high accuracies and specificities of this study demonstrated the ability of the artificially intelligent nanoarray based on GNPs and SWCNTs to serve as a clinical tool for GC detection and screening.
6.1.5.2. Silicon nanowire-based field effect transistor.
Breath samples were collected and analyzed from 30 GC patients divided into patients at early stage of the disease (n = 6), late stage (n = 13), and unknown stages of the disease (n = 11).452 The control group included 77 breath samples from 23 patients with early intestinal metaplasia, 9 with ulcer and 45 volunteers with healthy stomachs. Breath signatures were compared by means of molecularly modified SiNW FET. A training set of only one sensor resulted in 87% sensitivity, 81% specificity and 83% accuracy after applying the DFA algorithm for discriminating GC from the control group. The blind test resulted in 71% sensitivity, 89% specificity and 85% accuracy. For the discrimination between early and advanced stages, sensitivity and specificity of 92% and 67%, respectively, were achieved. The influence of the presence of H. pylori and smoking habits on breath signatures was also tested, but found to be negligible.
In another study, breath samples collected from 374 volunteers included 129 healthy control, 149 LC patients that were further divided into early and advanced stages, 40 GC patients also divided into early and advanced stages, and 56 patients having asthma and COPD (AC).453 Signals from the SiNW FET array were analyzed by both the ANN and DFA algorithms. ANN analysis for the comparison of LC and the control group yielded high sensitivity, specificity and accuracy (all >80%). Comparison of GC and the control group gave 87% sensitivity, 98% specificity and 95% accuracy using a different SiNW. Furthermore, the third group of AC that served as either rule-in or rule-out to LC, resulted in 81% specificity and 75% sensitivity. For discrimination between LC and AC a high sensitivity of 92% was observed. An exceptionally high sensitivity of 98% was achieved for discrimination between GC and AC. ANN results were further validated by applying DFA to the same set of binary comparisons.
6.1.6. Colorectal cancer.
6.1.6.1. Monolayer-capped metal nanoparticle-based sensors.
For the purpose of using GNPs for detecting colorectal cancer (CRC), breath samples were collected from 122 controls, 65 volunteers having CRC that had been confirmed histologically, and 22 classified with advanced adenoma (AA), having adenomatous polyps.606 Five different DFA models were calculated for 5 separate comparisons: (1) CRC vs. control; (2) CRC vs. AA; (3) AA vs. control; (4) non-advanced AA vs. advanced AA; and (5) AA vs. the control group. Accuracies in all 5 comparisons were >90% both in the training and the blind set. The effects of age, gender, smoking habits and fasting state were examined, but had no influence on the breath signature.
6.1.7. Squamous cell carcinoma of the head and neck.
6.1.7.1. Monolayer-capped metal nanoparticle- and carbon nanotube-based sensors.
For the purpose of detection, screening and staging of squamous cell carcinoma of the head and neck (HNSCS), breath samples were collected from 87 volunteers and analyzed by means of an artificial intelligence nanoarray composed of chemically modified GNPs.607 The study population included 22 patients diagnosed with HNSCC, divided into early and late staging, and also into different tumor locations, i.e. larynx or pharynx. Breath samples were also collected from 21 volunteers with benign lesions and 19 healthy subjects. Sensor responses were further analyzed by the DFA model for the design of 5 volatolomic maps. For HNSCC detection and screening sensitivity, specificity and accuracy of 77, 90 and 83%, respectively, were achieved. For HNSCC differential staging of early HNSCC compared to late HNSCC as well as for the comparison based on tumor location, high sensitivities, specificities and accuracies of >90% were reported.
6.1.7.2. Metal oxide sensors.
In a different study aimed at enrolling metal oxide-based sensors for HNSCC screening applications, breath samples were collected from 36 cancerous patients and 23 volunteers with different benign conditions.608 MOs, consisting of triplicates of 4 sensor types (CH4, CO, NOx and Pt) were used. Data collected from the sensors analyzed by logistic regression modeling gave 3 false negative and 4 false positive classifications. The model achieved a sensitivity of 90% and a specificity of 80%.
6.2. Pulmonary arterial hypertension
Accumulating evidence points to survival rates and clinical outcomes being significantly enhanced when pulmonary arterial hypertension is diagnosed at its early stages. Nevertheless, it remains a major clinical challenge and relies on an invasive right heart catheterization procedure. The complex pathologies involved in pulmonary arterial hypertension could result in a wide range of biomarkers in the breath. Indeed, there are alterations in the breath volatolome among PAH patients.
6.2.1. Monolayer-capped metal nanoparticle-based sensors.
It has been reported that a proof-of-concept study in which an array of GNP-based sensors was used for breath volatolome analysis and successfully detected and classified pulmonary arterial hypertension cases (22 patients and 23 healthy controls).609 The combined signals obtained from the sensors after exposure were significantly different in samples from each group. After a supervised DFA analysis, a receiver operating characteristic (ROC)-based classifier discriminated between the samples (pulmonary arterial hypertension vs. controls) with an accuracy of 92%. A continuation analysis aimed at detecting heritable pulmonary arterial hypertension cases diagnosed a mutation in the bone morphogenetic protein receptor type 2 (BMPR2) gene.610 The classifier successfully pointed out heritable pulmonary arterial hypertension (n = 7) compared to idiopathic PAH (n = 15), scoring an overall accuracy of 87%. A third model showed a correlation between the signals obtained from the sensor array and the severity of the patient's disease. With 91% accuracy, the samples were rated according to the New York Heart Association severity classification. However, the sample size in this case was relatively small and validation will be required. Currently, a clinical trial for validation (and expansion) is ongoing with a goal of recruiting 400 volunteers, including idiopathic pulmonary arterial hypertension patients, heritable pulmonary arterial hypertension patients, high risk subjects without pulmonary arterial hypertension (asymptomatic relatives of familial pulmonary arterial hypertension cases carrying a BMPR2 mutation) and chronic thromboembolic pulmonary hypertension patients (ClinicalTrials.gov Identifier NCT02782026).
6.2.2. Polymer composite sensors.
With similarity in concept, pulmonary arterial hypertension patients were included among a wide group of controls (lung diseases) in a study testing the feasibility of the Cyranose 320 system to detect LC and discriminate it from other common lung diseases.594 The system was composed of 32 polymer composite sensors, a sampling system, a data acquisition system and a processor (Smiths Detection, Pasadena, CA). For the training phase, the exhaled breath samples of 14 individuals with bronchogenic carcinoma and 45 healthy control subjects were analyzed. PCA and SVM models were obtained and optimized for discrimination between cancer and non-cancer cases. The models were applied to a second independent set of 76 individuals, 14 with and 62 without cancer (including pulmonary arterial hypertension patients). In the validation study, the electronic nose gave 71.4% sensitivity and 91.9% specificity for detecting lung cancer. Positive and negative predictive values were 66.6 and 93.4%, respectively. In this population with a lung cancer prevalence of 18%, positive and negative predictive values were 66.6 and 94.5%, respectively. The authors used GCMS only on 8 LC patients to understand the nature of the composition of the VOC profiles. A similar study, however, was not conducted for other groups, and quantitative comparison between the diseases was not carried out.
6.2.3. Colorimetric sensors.
Mazzone et al.599 enrolled 20 pulmonary arterial hypertension patients as a control group to test the feasibility of a chemical-colorimetric sensor array for volatolome collective assessment in the diagnosis of lung cancer (LC). This array is composed of group of 36 spots, containing different chemically sensitive compounds (e.g., metalloporphyrins) impregnated on a disposable cartridge. The colors of these spots change based on the chemicals with which they come into contact. After exposure to breath samples, the changes in color are converted into numerical values for the red, green and blue components of each spot for each scan. This resulted in a 108-dimensional vector (36 spots, 3 values per spot). Random forest analysis was used to explore the discriminative feasibility of the method. The results indicated that the collective breath volatolome of LC patients (n = 49) differed from pulmonary arterial hypertension, idiopathic pulmonary fibrosis (IPF) (n = 15), COPD (n = 18), sarcoidosis (n = 20) and healthy controls (n = 21). Once trained, the sensor array could discriminate between the diseases with a sensitivity of 73.3% and a specificity of 72.4%. The results were not influenced by gender, age, histology or smoking history. In this case, the authors used no complementary analysis for the identification and quantification of the volatile biomarkers that they measured.
6.3. Heart failure
6.3.1. Metal oxide sensors.
In a study focused on heart failure (HF), an array of 3 MO-based gas sensors was used to analyze the exhaled breath of 13 patients with decompensated HF (DHF), 16 patients of compensated HF (CHF), and 13 controls without HF (CON).611 Each of the sensors was modified with a different thin oxide layer with different sensitivity and selectivity for certain gas molecules at different temperatures. For each patient, 10 measurements over 35 min were taken from a 500 mL single breath sample collected from each patient. Three binary DFA classifiers were calculated from the normalized sensor responses. DHF and CHF could be discriminated with an accuracy of 91%, and between CON and CHF it was 97%. The best result was in the discrimination between CON and DHF (100%), but it is of a lesser medical significance.
6.4. Intestinal diseases
6.4.1. Monolayer-capped metal nanoparticle-based sensors.
An array of GNP based sensors was trained and programmed for the detection of inflammatory bowel diseases and irritable bowel syndrome using breathprint.201 Two breath samples were collected from 36 Crohn's disease (CD) and 35 ulcerative colitis (UC) patients – a total of 71 inflammatory bowel disease (IBD) samples – and 26 irritable bowel syndrome (IBS) patients. The first set of samples was analyzed by GCMS to pinpoint the VOCs with discriminative power between the different groups. The “artificial samples” were generated by a gas generator system; these samples, consisting of mixtures of the VOCs corresponding to the significant differences between the study groups, were used as the artificially intelligent training phase for the sensor array. After exposure to the artificial breath mixtures, the most sensitive sensors were chosen for ANN analysis as a training pre-clinical phase. The clinical performance of the chosen sensors was put to the test by analysis of 97 clinical breath samples. In the 6-fold validation method, 92% sensitivity (PPV of 85%), 53% specificity and a total accuracy of 81% when targeting the IBD group were achieved. The area under ROC test was 70%. The same planning and execution strategies were used to discriminate between CD and UC cases. After the training phase with artificial mixtures resulted in an accuracy of 96%, the model was tested with clinical breath samples, giving 75% sensitivity (targeting CD group), 47% specificity and 75% total accuracy, with an area under ROC of 72%.
6.5. Renal diseases
6.5.1. Monolayer-capped metal nanoparticle- and carbon nanotube-based sensors.
Four functionalized spherical GNP sensors (out of an array of 20 different sensors) were used to detect chronic kidney disease and disease progression from an analysis of exhaled breath from 62 volunteers.612 These sensors were chemically modified with 2-ethylhexanethiol, tert-dodecanethiol, hexanethiol or dibutyl disulfide as sensing layers, and were used to analyze the exhaled breath of 20 healthy controls and 42 chronic kidney disease (CKD) patients diagnosed at different stages (severity) of the disease, ranging from early stages to end stage failure. Using PCA followed by SVM and leave-one-out cross-validation (LOOCV), the authors used 4 sensing features extracted from 2 sensors to discriminate between healthy controls and early stage CKD patients, giving an accuracy of 79%. Employing a second algorithm, based on using 5 sensing features extracted from 3 sensors, discrimination between advanced stages and end stage CKD was achieved with an accuracy of 85%. Moreover, the authors showed a clear correlation between the sensing signal of a single 2-ethylhexanethiol sensor and the estimated glomerular filtration rate, one of the main clinical indications for renal function. These signals significantly correlated with transition from early-stage to late-stage CKD. This work was supported by quantitative analysis by GCMS indicating disease and/or severity associated volatolomic alterations in the exhaled breath of the patients. Fifteen different compounds were identified in the disease, or in specific stages of the disease progression.
In a continuation study, these authors tested the feasibility of the sensor array, this time including an additional set of random networks of single-walled carbon nanotubes capped with organic films for identifying patterns of exhaled VOCs of end-stage renal disease (ESRD) and studying the impact of hemodialysis (HD).613 Exhaled breath samples of the 37 participants (26 ESRD patients treated with HD and 11 healthy subjects) were analyzed using the sensor array, and DFA algorithms were obtained to specify the informative volatolomic patterns. First the DFA model was applied on 80% of the data-base as a training phase, whereas the remaining samples were used for the validation of the developed model. Including the responses of the 3 sensors (Au NPs with dodecanethiol, gold nanoparticles with tert-dodecanethiol, and SWCNTs with sulfated β-cyclodextrin), the model showed that the breath pattern of ESRD patients was distinguishable from controls with an accuracy of 80%. Moreover, the responses of the sensors showed significant variance during HD therapy. Seventeen to twenty breath samples were tested before and 1, 2, 3 and 4 h after the beginning of HD, and showed significant changes along with treatment. Once again, the authors supported their results with quantitative analysis of a second set of breath samples obtained from each patient during the same time intervals, showing that 7 VOCs were altered in patients at ESRD, whereas the concentrations of 2 of them were significantly reduced after 4 h of treatment.
In parallel to the previous 2 clinical studies, the feasibility of the sensor array for the early detection of acute and chronic kidney failure was also tested in an animal model.240,614 In one study, the volatolomic signature was assessed as a function of time after inducing kidney failure in an ischemic injury model in rats. This was compared to blood and urine biomarkers routinely used in the clinical world. The signature obtained from 3 GNP-based sensors with 3 different organic functionalities – dodecanthiol, octadecanethiol, and 4-chlorobenzenemethanethiol – was altered 1 h after AKI induction, whereas urinary neutrophil gelatinase-associated lipocalin and serum creatinine levels were increased after 2 h. These trends were explained by GCMS analysis showing an accumulation in a specific range of VOCs in the exhaled breath shortly (T1–T4) after disease induction.614 In a second pre-clinical study, a combination of 10 chemiresistive random network of SWCNTs coated with organic materials, in combination with pattern recognition methods, was used to detect the pattern changes in rats that had undergone induction of CKD. First, the sensors were trained and optimized by exposure to artificial gas mixtures corresponding to the statistically validated VOCs identified by GCMS analysis. The sensors were exposed to 10 breath samples of sham control rats and 7 CKD rats. PCA analysis showed a clear separation between the 2 clusters, corresponding to the groups and indicating 100% detection accuracy.240
6.6. Neurological diseases
6.6.1. Parkinson's and Alzheimer's diseases.
Diagnosis of Parkinson's disease (PD) is based mainly on the presence of clinical motor symptoms of the disease, which usually appear after the loss of 50–70% of the target dopaminergic neurons in the substantia nigra pars compacta. Non-invasive and objective early detection could reduce misdiagnosis rates and promote early neuroprotective therapy, thus improving clinical outcomes.
6.6.1.1. Monolayer-capped metal nanoparticle- and carbon nanotube-based sensors.
To investigate whether damage to the dopaminergic neurons could produce specific alterations in the volatolomic signature, presymptomatic PD in rats was induced and their exhaled breath analyzed by an array of organically functionalized random networks of SWCNTs (RN-CNTs).615 Dopaminergic neurons were destroyed by the neurotoxin, 6-hydroxydopamine (6-OHDA), which is taken up selectively by neurons expressing a catecholamine transporter, resulting in loss of 55% of dopamine in the striatal tissue homogenate compared to control animals (10 lesioned and 9 sham-operated controls). LOOCV analysis of the DFA algorithm on the output of RN-CNTs functionalized with a layer of α-cyclodextrin or a layer of β-cyclodextrin resulted in a sensitivity, specificity, and accuracy of 80, 100 and 90%, respectively.
A further investigation of a similar model compared rats with mild and severe lesions of dopamine neurons (50 and 70% dopaminergic denervation; n = 8–11 rats per group).616 In this study, an array of sensors composed of those based on GNPs or SWCNTs, organically functionalized with 4-methoxy-toluenethiol or dibutyl-disulfide, was used to qualitatively analyze breath samples. DFA applied to the responses to exhaled breath samples obtained from a training set of 6-OHDA-lesioned rats showed a clear difference between all the 6-OHDA rats and sham-operated controls (sensitivity, specificity, and accuracy >80%). A difference was also seen between the responses to exhaled air of 5,7-dihydroxytryptamine (DHT)-lesioned rats and controls, which was less clear than that between 6-OHDA and controls (sensitivity 70, specificity 100, and accuracy 84%), as well as between the 5,7-DHT-lesioned rats and all the 6-OHDA lesioned rats (sensitivity 89, specificity 100, accuracy 93%). The exhaled breath of the Sprague-Dawley transgenic rat (Tg) model carrying the human A53T SNCA mutation (n = 8) was analyzed as an additional Parkinsonian model. An array of CNT functionalized with β-cyclodextrin sensors was used to compare the breath signatures of Tg rats and WT controls (n = 10). The analysis gave a distinctive power of sensitivity, specificity, and accuracy of 100, 90 and 94%, respectively (LOOCV). These last results were supported by complementary analysis by GCMS of VOCs obtained from both blood and striatal homogenate samples, which showed a wide range of significant differences in their abundance of VOCs.
A case–control study assessed the use of an array of spherical GNPs (core diameter 3–4 nm) and SWCNTs to detect and classify Parkinsonian cases based on analysis of their exhaled breath.617 The GNP sensors were organically functionalized with different sensing layers, such as dodecanethiol, 1-dodecanethiol, 4-chlorobenzene methanethiol, whereas the SWCNT-based sensors were organically functionalized with PAH derivatives. In this study, breath samples of 44 idiopathic PD (iPD), 16 non-iPD patients (atypical Parkinsonism) and 37 healthy controls were collected and analyzed. First, comparison of non-iPD with iPD states yielded 88% sensitivity, 88% accuracy and 88% ROC-AUC in the training set samples with known identity. K-Fold cross-validation analysis of this comparison scored 81% sensitivity and accuracy, and a 92% negative predictive value. A second comparison between atypical Parkinsonism states and healthy subjects scored 94% sensitivity and 85% accuracy in the training set samples with known identity. The validation set of this comparison scored 81% sensitivity and 78% accuracy. The results were unaffected by L-dopa or monoamine oxidase B (MAO-B) inhibitor treatment (AUC = 56% and 59%, respectively). The results were explained by GCMS analysis, which showed significant variations in the concentrations of 4 VOCs in the exhaled breath of these groups.
In a second study, the same system was tested for the potential use of the discrimination between 2 major neurodegenerative disorders, Parkinson's and Alzheimer's disease.618 Similarly, an array of 2-mercapto-benzoxazole-capped GNPs, 3-mercapto-propionate-capped GNPs and α-cyclodextrin, β-cyclodextrin, carboxy-methylated β-cyclodextrin, hydroxypropyl-β-cyclodextrin or heptakis(2,3,6-tri-O-methyl)-β-cyclodextrin modified SWCNTs was used to analyze alveolar breath collected from 57 volunteers (AD patients, PD patients and healthy controls). DFA applied to the responses of different combinations of the sensors could clearly distinguish AD from healthy states, PD from healthy states, and AD from PD states, with a classification accuracy of 85, 78 and 84%, respectively. Supportive GCMS analysis of a second set of breath samples from each subject showed that a range of 24 different VOCs was statistically varied between the study groups.
6.6.1.2. Polymer composite sensors.
With the same goal, Bach et al. used the Cyranose 320 (C-320) eNose (Smiths Detection Group Ltd, Watford, UK) composed of 32 conducting chemiresistors made from carbon black nanocomposites that change their resistance in response to VOCs.619 Breath samples were collected from 39 AD, 16 PD, 35 controls and several binary classifiers were obtained by pattern recognition algorithms. Using LOOCV, a sensitivity of 50% and a specificity of 77% were calculated in discriminating between AD and controls. Moreover, the authors reported that a second model was built to discriminate between all 3 groups, in which, after a training phase, an independent group of samples (including 21 AD and 16 controls) were used as a validation set. This resulted in a sensitivity of 69.8% and a specificity of 68.7%. They also used ion mobility spectrometry to explore the quantitative shifts in the composition of exhaled breath. Using a decision tree with 4 VOCs, the method showed a considerably higher accuracy of 94% (95%; CI 88–100) in which only 3 patients were misclassified out of a total of 53 participants.
6.6.1.3. Metal oxide sensors.
Other studies have focused on the detection of Alzheimer's disease by breath analysis as a potential method of early diagnosis. For example, using an array of metal oxide semiconductor sensors (iAQ-2000 by Applied-Sensor, Warren, NJ), Mazzatenta et al.620 analyzed the exhaled breath of 59 volunteers, divided into neurodegenerative (n = 15, age range 59–95 years) and healthy (n = 44, age range 19–105 years) subjects. The VOC fingerprint of AD is significantly different from the healthy ones, as was the frequency distribution of VOCs in both AD and control subjects. The fit of the VOC frequency distribution returned 2 distinct Gaussian peaks for the AD (r2 = 0.56) and healthy subjects (r2 = 0.98) (Fig. 34a and b). In another study, 11 metal oxide semiconductor sensors were used to analyze 60 breath samples from 40 AD and PD patient groups, and 20 healthy individuals, collected at Kyungpook National University Hospital.621 Separated into 2 arrays, the authors used the following MOX based gas sensors: TGS 2600, TGS 2602, TGS 2611, TGS 2620, TGS 2610 (Figaro, Osaka, Japan), and MICS 5135, MICS 5132, MICS 2610, and MICS 2610 (e2V Technologies, Chelmsford, Essex, UK). After exposure of the breath samples to the sensors, it was clear that the normalized responses of the sensitivity characteristics for the gas sensors individually in each system were significantly different between the AD, the PD, and the control groups (Fig. 34c). Using different combinations of sensors, clustering analysis of the exhaled samples showed that the AD, PD, and control groups fell into 3 clusters by both sensor arrays. The results demonstrate that the control group could be distinguished from the AD and PD patients based on the PCA and Sammon's mapping methods, with a confidence limit of 95%. A complementary analysis by GCMS analysis of breath samples, to explain the performance of the sensors, showed significant alterations in 5 VOCs, associated with these diseases.
 |
| Fig. 34 VOC fingerprint of Alzheimer's disease (AD) and Parkinson's disease (PD) using 2 different MO sensor systems. (a and b) Characterized by a cluster of signals (dark gray) generated by VOCs’ relative abundance significantly different from the healthy one (light gray), p < 0.5. (a) VOCs’ frequency in both AD (fit r2 = 0.56) and controls (fit r2 = 0.98); (b) frequency distribution of VOCs in AD and control. Fit of the VOC frequency distribution shows 2 distinct Gaussian peaks for the AD (r2 = 0.56) and healthy subjects (r2 = 0.98). Reprinted from ref. 620 with permission from Elsevier, copyright 2015. (c) Normalized responses of the sensitivity characteristics for different types of gas sensors. Response sensitivity of exhaled breath samples from the patients with AD, PD and healthy controls. Reprinted from ref. 621 with permission from MDPI, copyrights 2017. | |
6.6.2. Multiple sclerosis.
Multiple Sclerosis (MS) is the most widespread chronic neurological disease affecting young adults. Commonly the disease outbreaks at 20–50 years of age, and is approximately 3 times more frequent in women than in men.622,623 MS diagnostics is based on clinical characteristics and commonly supported by Magnetic Resonance Imaging (MRI) as a complementary test. A number of VOCs are believed to be present in breath samples, including acetophenone, heptadecane, nonanal, n-decanal, and sulfur dioxide.200 One explanation for the pathogenesis of MS is the toxic effect on the central nervous system of oxidative stress, which is one of the main causes of VOC formation in diseased states.200
6.6.2.1. Monolayer-capped metal nanoparticle- and carbon nanotube-based sensors.
In two separate follow-up studies, researchers have reported on a homemade e-nose system (NaNose) for MS diagnosis from breath analysis.200,624 The first study used only sensors based on bilayers of PAHs and SWCNTs (Fig. 35).624 Data analysis of 51 breath samples showed 80.4% accuracy in classification of MS and healthy people based on a 4-sensor analysis. The second study used a combination of 6 gold nanoparticle sensors combined with 2 PAH-based carbon nanotube sensors.200 In the latter study, 204 people were sampled in 2 phases (training set and blind set), and subcategories were evaluated for diagnostic purposes. Using ANN analysis, the sensor data allowed discrimination between MS patients and controls, with accuracies up to 90% in the training sets. Blinded sets showed a 95% positive predictive value between MS-remission and control, 86% NPV between relapse and control, and 100% sensitivity with 100% NPV between MS untreated and controls.200 The authors tested the effect of confounding factors, such as smoking and gender; the results show that the sensor system was not influenced by them. Furthermore, potential biomarkers identified in parallel by mass spectrometry were used to evaluate the sensor response. The same array of sensors that achieved classification presented a linear correlated response to changing concentrations of the 3 tested biomarkers.200
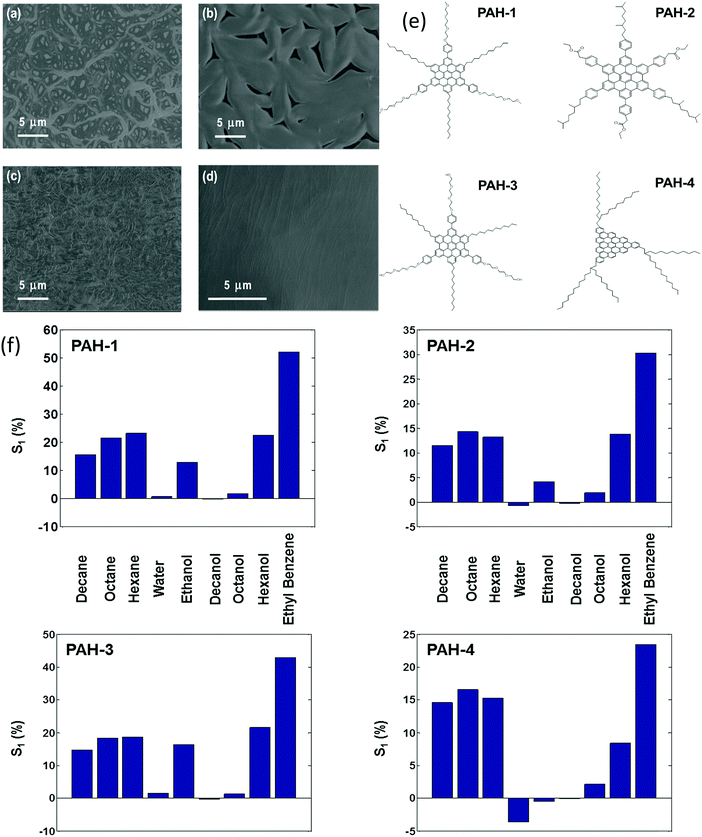 |
| Fig. 35 Field emission scanning electron microscopy (FE-SEM) images of the sensing films made of PAH derivatives on top of random networks of SWCNTs: (a) PAH-1/SWCNT; (b) PAH-2/SWCNT; (c) PAH-3/SWCNT; and (d) PAH-4/SWCNT. (e) Discotic polycyclic aromatic hydrocarbon (PAH) derivatives employed in the study; (f) normalized change of sensor resistance in the middle of the exposure (S1) calculated from sensor responses to the 9 VOCs analyzed at pa/p0 = 0.5. Reprinted with permission from ref. 624. Copyright (2011) American Chemical Society. | |
6.7. Diabetes mellitus
Diabetes mellitus (DM) is an important chronic metabolic disease increasing globally and is truly endangering the social order. A greater number of people are afflicted every year worldwide by this disease due to high-calorie diet and sedentary lifestyle.625 Based on a World Health Organization (WHO) global report, 422 million adults suffered from diabetes in 2014, compared to 108 million in 1980.626 Eventually, if not controlled, DM could lead to heart attack, stroke, kidney failure, blindness, and amputations.626 Due to the everyday discomfort of glucose blood sampling, researchers have been trying to find a non-invasive way for diagnosing diabetes. Different VOCs can act as possible markers related to diabetes; for example, ketones are abundant in urine being the result of excessive fat oxidation by the liver; they are at high levels in diabetic patients.8,25 In spite of the great interest, only a few sensor-based studies have tested urine VOCs for diabetes, whereas most used spectrometric-based methods.627–629
Mass spectrometry studies have shown that breath acetone can serve as a VOC biomarker of diabetes type I.630 GC-MS analysis of breath samples from diabetic patients contained acetone breath levels at >1.71 ppmv, as opposed to acetone levels of healthy people (<0.76 ppmv).82 However, other studies have clearly shown wide variations in breath acetone concentration due to diet and natural intra-individual biological variability. This places uncertainty in the use of acetone alone to monitor blood glucose.631 Nevertheless, many different sensor studies examined acetone in breath sampling using sensors.
6.7.1. Metal oxide sensors.
Some studies, including chemiresistors based on MO, were used to explore diabetes, although the sensors used were commercial ones not based on nanomaterials. Yan et al.632 used a chamber with 11 sensors, comprising 6 ordinary MOS sensors, 3 temperature modulated MO sensors, a carbon dioxide sensor and a temperature–humidity sensor. First, a simulation experiment with acetone at 0.1 to 20 ppm was evaluated, along with spiked breath samples. The 295 healthy breath samples and 279 diabetic samples were collected and analyzed, and the sensitivity and specificity of diabetes screening were ∼92% and 91%, respectively. Prediction of blood glucose levels by the breath sample also showed moderate correlations.632 Jaisutti et al.633 developed room-temperature operable gas sensors based on Na-doped p-type ZnO nanoflowers as a potential sensor for diabetes detection. The sensing layer was activated by ultraviolet illumination and the sensors responded to acetone gas (100 ppm, UV intensity of 5 mW cm−2) with a detection limit of 0.2 ppm at room temperature.633 A FET sensor based on a 10 nm thick indium nitride (InN) layer as an acetone sensor achieved a detection limit of 400 ppb acetone at 200 °C.31 This sensor was evaluated in the presence of varying concentrations of oxygen, which was done to simulate a response in real breath samples; it showed a good response to a mixture of oxygen and 2.4 ppm of acetone.31 The sensors were not tested on real breath samples.
6.7.2. Conducting polymer sensors.
A portable gas system using a conducting polymer (polypyrrole) sensor array was used as a potential system to analyze diabetic patients’ breath.634 Four polypyrrole thin film sensors of different thicknesses were used to detect acetone. Baseline was calibrated from dry air and voltage variation rates were measured after exposure to acetone. The sensors, initially exposed to acetone concentrations of 50 to 700 ppm, showed increasing responses, but these concentrations were higher than those expected in breath samples. Following this, a breath test was given to six individuals, 3 diabetics and 3 non-diabetics. Discrimination between their breath samples was achieved using the PCA algorithm.634
6.7.3. Optical fiber based sensors.
Researchers are now developing a sensor device for acetone and isopropanol breath sampling based on an optical fiber probe.635 Acetone and oxidized nicotinamide adenine dinucleotide (NADH to NAD+) can be reduced in a weak acid environment by secondary alcohol dehydrogenase. Excitation of NADH by 340 nm light will subsequently result in fluorescence emission at 490 nm. Thus, monitoring the decrease in NADH fluorescence intensity should measure acetone.635 The sensor was tested on breath samples from 25 diabetic patients and 55 healthy controls (Fig. 36). The breath acetone in healthy people had a mean value of 750.0 ± 434.4 ppb, whereas diabetic patients showed a mean concentration value of 1207.7 ± 689.5 ppb. The acetone levels were not influenced by confounding factors, such as gender and age.635
 |
| Fig. 36 (A) Schematic diagram of acetone and IPA (isopropanol) biosniffers. The inserted chart shows the structure of the flow-cell. (B) Schematic diagram of breath measurement using biosniffers. The sample breath was delivered to the biosniffer by an air pump, and the flow rate was 200 mL min−1, which was the same as in the standard gas measurement. (C) Selectivity of acetone and IPA biosniffers. N.D.: none detected. *: 2-butanol was not measured by the acetone biosniffer. Reprinted (adapted) with permission from ref. 635. Copyright (2017) American Chemical Society. | |
6.8. Tuberculosis
Diagnosis of TB remains a major global public health challenge. Although sophisticated and accurate diagnostics are now available, they are often non-accessible in TB stricken regions, where old-fashioned and non-accurate tests are still in use. Several reports have pointed out that alteration in exhaled VOCs is found both in cultures and TB patients, indicating that exhaled breath analysis holds the potential for non-invasive diagnostics of TB. Several nano-based sensor technologies have developed and put to the test.
6.8.1. Monolayer-capped metal nanoparticle- and carbon nanotube-based sensors.
In a case–control study, 198 breath samples were analyzed by an array of sensors, including MCMNPs and molecularly-modified SWCNTs.636 The study population consisted of 3 main groups: active TB (n = 64), controls (that included a group of healthy subjects (n = 67), and a group of suspected with TB, yet ruled out by negative smear microscopy, culture and GeneXpert MTB/RIF, n = 67). About 70% of the samples were used as a training set, with the remainder being used as a blind validation set for the sensors’ analysis. Interestingly, several sensors gave a discriminative response to the different groups, yet a chemiresistor based on dodecanethiol-capped gold nanoparticles correctly classified 121 of the 138 training samples, with an accuracy of 88%, a sensitivity of 85%, and a specificity of 89%. In the validation set, the same sensor scored a sensitivity of 90%, a specificity of 93% and an accuracy of 92%. The sensor's function was unaffected by possible confounding factors including smoking habits, HIV infection and antibiotic treatment (for up to 2 weeks prior to testing; Fig. 37a). In another study of Mycobacterium bovis infected cattle, 6 GNP-based sensors were used to analyze breath samples obtained from 8 TB infected and 4 TB-negative from an infected dairy, and 10 animals from non-infected dairies. The sensors were functionalized with octadecanethiol, decanethiol, 2-naphthalenethiol, 2-mercaptobenzoazole or 2-nitro-4-trifluoromethylbenzenethiol as sensing layers. Applying the DFA algorithm to the responses gave sensitivity and specificity of 100% and 79%, respectively.637
 |
| Fig. 37 (a) Dot-plots of the response of a single dodecanethiol-capped gold nanoparticle. Each circle/star represents a single sample. The central dashed line represents Youden's cut-point, and the outer dashed lines represent the cut-points to rule-in and rule-out tuberculosis. Samples from the validation set with responses lower than the threshold were classified as tuberculosis-positive (open stars) or non-tuberculosis-positive (closed stars) according to Youden's cut-point. Reproduced with permission from the ©ERS 2014.636 (b) Distribution of quartz microbalance (QMB) gas sensors’ signals among cases with pulmonary tuberculosis at baseline and on days 2, 7, 14 and 30 after treatment. Reprinted from ref. 638 with permission from Elsevier, copyright 2017. | |
6.8.2. Quartz crystal microbalance sensors.
In another study, a sensor array made of 8 metalloporphyrin-coated QCM sensors was used to assess the exhaled volatolomic signature of TB patients during their treatment.638 Exhaled breath samples of 51 TB patients and 20 controls were analyzed before and 2, 7, 14, and 30 days after therapy, which were correlated with clinical and microbiological measurements on sputum samples. Significant differences in the frequency shifts were measured by all 8 sensors when comparing between TB patients and controls at day 0. Pattern recognition analysis that included the signals of all sensors scored 93% accuracy (Fig. 37b). Nanomaterial-based sensors were also examined in cases of bovine TB.639 An array of 9 MO-based sensors was used to analyze the headspace of cattle sera samples obtained from 11 TB infected and 10 TB-free cattle. DFA and PCA models were obtained using data from 4 sensors that had higher selectivity to oxidizing and flammable compounds, such as nitric oxide, hydrocarbons and alcohols. Both statistical models resulted in absolute discrimination between the samples.
7. Urine-based disease diagnostics
Urine is a dominant bodily fluid used for diagnostics. Urine diagnostics can be used for detection of bacterial infections, metabolic disorders, protein degradation products and renal dysfunction. It is a complex biofluid containing many components that are influenced by a number of factors, such as diet, age, gender, physical activity and health status.640–642 Traditionally, clinical urine analysis involves detection of large metabolites directly in the liquid phase, for example, neutrophil gelatinase-associated lipocalin and kidney injury molecule-1 (KIM-1) in the case of acute kidney injury (AKI),643 or leukocyte esterase urinary tract infection (UTI).644 However, urine odors have been used for diagnostics for >2000 years ago640 and recently analysis of VOCs from urine is regaining attention as diagnostic biomarkers.8 Approximately 280 VOCs have been reported in different studies on healthy people.21 Most VOCs in the urine are water-soluble and depend on renal filtration.8 VOCs in urine include a number of chemical families e.g., ketones, aldehydes, acids, amines and others. Other exogenous molecules arising from medical and non-medical drugs are secreted in urine and affect the VOC profile.8
7.1. Diabetes mellitus
7.1.1. Metal oxide sensors.
Siyang et al.645 reported a homemade e-nose for diabetes diagnosis, using a combination of 8 off-the-shelf MOS sensors. Sensors were commercially made for detection of different compounds, such as alcohol, ammonia and others. The study used 6 pure urine samples that were spiked with different concentrations of glucose from 0 to 2000 mg dL−1 and heated to different temperatures between 30 and 45 °C. Using PCA analysis, they showed separation of the control vs. high concentration samples at 45 °C, with the ammonia sensor being the most sensitive.
7.2. Malignant diseases of the urinary and genital systems
As discussed above, cancer diseases are widely studied and numerous researches have worked on the field of VOC analysis in cancer. Recent statistics from the United States estimate prostate cancer to be the leading in new cancer cases in 2017, with 19% of the new cases out of all the estimated cancer cases in 2017.568 Urinary bladder and kidney cancers are the fourth and the sixth new cancer cases, 7% and 5%, respectively.568 Cancers related to the urinary tract system, bladder and prostate have been conventionally detected by classical imaging techniques, such as ultrasound, computed tomography (CT), positron emission tomography and MRI. Recently there has been a rapid advance in those urological imaging techniques, such as MRI and fusion biopsy.646 However, these techniques continue to be limited by tissue contrast resolution in MRI and determination of the optimal radiotracer in the case of positron emission tomography.646 Most cancer cases lack early clinical symptoms and continue asymptomatic until late stages, when therapy options become limited.647 In recent years, such cancers have been the target for VOC based analysis from urine samples using GC-MS and sensor systems.647–651
7.2.1. Metal oxide sensors.
Weber et al. used a set of commercial 12 MOS and 10 MOS-FET sensors incorporated into a NST 3320 Lab Emission Analyser.649 The system includes a capacitance-based humidity sensor and an infrared-based CO2 sensor. The trial included urine samples from 30 patients with bladder cancer (transitional cell carcinoma) prior to surgical intervention, and 59 control people. Data were analyzed using PLS-DA, initially on a training set, that resulted in the best classification between cancerous samples and healthy samples with 70% accuracy. Discrimination of bladder cancer from a control group which included healthy and non-cancerous bladder diseases resulted in a lower accuracy of 65%. Finally, the authors concluded that the tested commercial e-nose is not the best choice for these diagnostics.649
A pilot study done by Horstmann et al. tested an e-nose with 3 different MOS sensors at 3 temperatures. They tested urine samples from 15 patients with suspected bladder cancer and 21 controls with non-cancerous urological conditions.652 Using PCA and DA, the authors achieved an overall sensitivity of 75% and specificity of 86% in classifying cancerous vs. non-cancerous samples.652 Since this reference is published in short-note format, the authors did not provide any detailed information on the sensors used or their set-up, making it difficult to evaluate their findings in the current context.652
Roine et al.653 used a commercial e-nose system (ChemPro 100) in which detection is based on a combination of an ion mobility chamber and a set of MOS sensors to differentiate cancer from benign cases using headspace of urine samples (Fig. 38). They tested 50 patients with confirmed prostate cancer and 15 patients with benign prostatic hyperplasia.653 Urine samples were taken prior to operation, and another sample was taken post operation in the case of the control group. DA with leave-one-out cross-validation was used to analyze measurements and reduce the chance for over fitting of the data, followed by ROC curve analysis. Sensitivity and specificity were 78% and 67%, respectively, and AUC was 0.77 between the tested groups.653
 |
| Fig. 38 Combined result of an e-nose MOS and ion mobility system. Total average of all data represents typical smell print of urine. Characteristics of average smell prints of benign prostatic hyperplasia (BPH) and prostate cancer (PCa) groups are represented by deviation from the total average. Reprinted from ref. 653 with permission from Public Library of Science, copyright 2014. | |
7.2.2. Quartz crystal microbalance sensors.
QCM sensors are based on the piezoelectric properties that, in general, rely on the sensor's response to an applied mechanical stress.8 The adsorption of molecules from the gas phase on the sensors’ surface results in variations in the fundamental oscillating frequency of a thin quartz crystal.8 This variation can be measured and analyzed for VOC classification. A number of QCM studies involved cancer detection from urine samples. All of them used the same set-up based on 8 different QCM sensors, coated by sensing layers of metalloporphyrins and integrated in an e-nose system developed at the University of Rome Tor Vergata.648,651,654,655 Bernabei et al.648 measured the headspace of urine samples from 113 patients with different urological pathologies. Using PCA and discriminant analysis combined with partial least square (PLS-DA), they could differentiate between healthy and sick people, and achieved gradual distinction between prostate and bladder cancer.648 In follow-up experiments on prostate cancer, the same group used the same electronic nose set-up on a varying small clinical set of 21 to 41 samples. Using the same PLS-DA methodology, the authors showed fair discrimination between prostate cancer patients and the control group based on first urine samples. The authors reported 93% specificity in the last test.651,654,655
7.3. Bacterial and urinary tract infections
Microbial detection and analysis based on odor or VOCs produced by microorganism populations have been widely investigated.25 Different microorganisms have unique metabolic pathways and can divide into very high numbers that can reach an average of 108–109 cell per mL culture fluid. As a result, different molecules are produced and degraded at high rates so that they can potentially be detected by analytical and sensor technologies, particularly for clinical applications.656–659 UTI is a very common bacterial infection at all ages, and one of the most common reasons for hospitalizations due to infections among elderly individuals.644 The clinical symptoms of UTI are often atypical or asymptomatic, making their diagnosis and management very challenging due to their high prevalence.644 UTI diagnosis is currently based on the existence of clinical symptoms together with a nitrite strip test and partial measurement of white blood cell count in the urine. Even though urine culture is the gold standard test for UTI detection, it is both costly and time-consuming.644
7.3.1. Carbon black polymer sensors.
Each sensor element is constructed from films consisting of carbon black particles dispersed in insulating organic polymers. The film's electrical conductivity is provided by the carbon black, whereas the different polymers provide the chemical diversity among elements in the sensor array.660 Yates et al.661 studied VOCs emitted from urine and blood samples using a commercial electronic nose (Cyrano Sciences C320) together with an Agilent 4440 Chemosensor. The e-nose system consisted of 32 carbon black polymer sensors. Samples of urine from routine hospital screening for UTI were evaluated, with the goal of classifying positive vs. negative UTI samples. The authors tested several different classification models and the best accuracy was 80%, using a nonlinear model with Kernel width parameter optimization.661 In another trial using the polymer-based Cyranose 320 system, they tested urine samples from 13 patients.662 Using the 32 sensors and PCA analysis, the authors checked only 9 of the 13 samples, out of which only one sample was identified correctly with UTI, 6 samples tested abnormal with mucus, and 2 were classified as healthy.662
7.3.2. Conducting polymer sensors.
Sensors are constructed using conducting polymers with a specific selection of polymers and doping materials, resulting in responsiveness to different odor groups measured by changes in resistance. Pavlou et al.663 used a commercial electronic nose (BH-114: Bloodhound Sensors, Leeds, UK), which employed 14 conducting polymer (polyaniline) sensors. In a 2-set series experiment, 25 and 45 urine samples from patients were analyzed by incubation in a VOC generation test tube system for 4–5 h. Data were interpreted using neural network analysis.663 In both experiments the authors correctly identified all but one of the samples compared to standard culture methods. They were able to distinguish among the 4 tested subgroups: normal urine, Escherichia coli infected, Proteus spp. and Staphylococcus spp.663 Kodogiannis et al.664 used the same commercial system to test urine samples from 45 UTI suspected patients. They collected 5 mL of urine to which an appropriate culture medium was added to a final volume of 20 mL, which was incubated for 5 h at 37 °C. Using an extended normalized radial basis function based on neural networks, they correctly classified all samples using the overall model.664
7.4. Intestinal diseases
Inflammatory bowel disease (IBD) relates to a number of inflammatory disorders of the colon and small intestine; most notable are Crohn's disease (CD) and ulcerative colitis (UC).665 Environmental factors (diet, smoking and personal hygiene), ethnicity, immune system problems, genetic susceptibility, and microbial infections can result in mucosal inflammation.665 Bacterial diversity in the gastrointestinal tract is immense and difficult to study due to many of the bacteria being non-culturable in vitro.666 The effect of this bacterial colonization, mainly through fermentation-derived VOCs, has been studied for diagnostic proposes.666
7.4.1. Metal oxide sensors.
A commercial “electronic nose” (Fox 4000; AlphaMOS, Toulouse, France) was used to analyze the chemical signature of urine samples of 24 patients with UC, 24 patients with CD and 14 controls.666 The system comprises an array of 18 metal oxide gas sensors, with the resistance being modulated in the presence of a target gas/vapor. The system uses 2.5 mL of headspace from the sample bottle, which is directly injected into the electronic nose. The change in resistance of the sensors was measured from an injection time of 120 seconds at a sampling rate of 1 Hz. PCA analysis applied to the signals obtained from the sensors showed clear variance between the 3 groups. A DFA classifier was developed, scoring 70% accuracy in separating the studied groups. A second analytical method was also tested, both as a complementary method and for a comparison between the 2 analytical methods. They used Field Asymmetric Ion Mobility Spectroscopy (FAIMS, Lonestar; Owlstone, UK). After the sample had been ionized, fragments of different sizes and types were introduced between 2 metal plates, and an asynchronous high-voltage waveform was applied. The ionized molecules are then subjected to these high electric fields. The difference in movement of these molecules in this high electric field can be measured, resulting in separation of the complex mixture. The results of FDA on the FAIMS data for patients with CD, UC, and controls scored an accuracy that exceeded 75%.
Using a similar technology, Covington et al. tested another bowel condition, Bile Acid Diarrhea (BAD).667 BAD is the most common cause of chronic diarrhea that affects ∼5% of the world population. The same AlphaMOS Fox 4000 electronic nose, in combination with an Owlstone Lonestar Field Asymmetric Ion Mobility Spectrometer, was used to sample urine headspace (Fig. 39). They sampled a total of 110 patients: 23 with BAD, 42 with UC and 45 controls.667 One mL headspace from each 5 mL urine sample was examined using a HS100 autosampler after heating them for 10 min at 60 °C. The sensor's resistance change was measured over 180 seconds from injection at a sampling rate of 1 Hz. Results analyzed by DA gave 79 to 83% accuracy in classification of the tested groups. A complementary GC-MS analysis revealed 2 VOCs, 2-propanol and acetamide, which were significantly elevated in the BAD sample.667
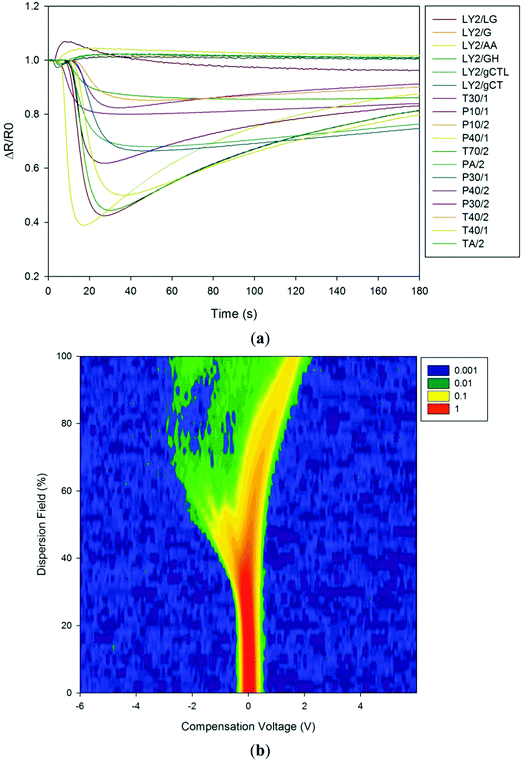 |
| Fig. 39 (a) Raw electronic nose results showing sensor responses to Bile Acid Diarrhea (BAD) of a patient's urine sample. (b) Raw data from the FAIMS instrument response to a BAD patient urine sample. Intensity is in arbitrary units of ion count. Reprinted from ref. 667 with permission from MDPI, copyright 2013. | |
7.5. Other medical conditions
7.5.1. Quartz crystal microbalance sensors.
An electronic nose-based on QCM technology was used to analyze urine samples containing blood. In different injuries, such as kidney injuries, trace amounts of blood might appear in urine.668 Here authors used a QCM-based electronic nose with 8 sensors coated with different metalloporphyrins. Urine samples were collected from children affected by kidney diseases with blood in urine and age-matched healthy children.668 Samples were sealed in vials for 30 min at 30 °C. No data were provided regarding the volume of urine and headspace samples, or the number of participants. Their results suggest the ability of the system to correctly identify blood-containing urine samples.668 They also claimed that by using ANN they could correctly determine the pH.668
8. Feces-based disease diagnostics
Feces have been gaining interest lately in scientific and clinical studies, mainly due to the recent explosion in microbiome research.669 The human intestine harbors a variety of microorganisms that can reach over trillions of cells, consequently creating a microbiome within the human body some 100 times bigger than the human genome.669,670 Host–microbe relations are essential to many aspects of normal human physiology, such as metabolic activity and immune homeostasis, affecting the balance between health and disease.671,672 Many fecal metabolomics studies on gut-related issues use Nuclear Magnetic Resonance spectroscopy (NMR) and mass-spectrometry techniques.673 Dedicated studies on VOCs report on ∼500 compounds emitted from stool samples.8 Colonic fermentation of different molecules, e.g., amino acids, is responsible for the production of typical fecal odors, such as methane, aliphatic amines, ammonia, branched chain fatty acids, derivatives of phenol or indole, and volatile sulfur-containing compounds. Other VOCs found in fecal headspace, e.g., chloroform, are possibly the outcome of contaminants absorbed from food, water or pollution.8
8.1. Malignant diseases
8.1.1. Carbon black polymer sensors.
In a proof of concept study done on CRC patients, some authors used the commercial Cyranose 320 system composed of 32 polymer-based sensors to examine volatiles from fecal samples.674 The study population included 40 patients with CRC, 60 patients with advanced adenomas, and 57 healthy controls. Each stool sample contained about 2 g frozen feces in a sealed vacutainer that was heated to 37 °C for 1 h. Thereafter, the heated vacutainers were connected to the Cyranose in an air-tight closed loop system.674 The results showed that fecal VOC profiles of CRC patients significantly differed from controls with 85% sensitivity and 87% specificity. Similarly, VOC profiles of advanced adenoma patients differed from controls with 62% sensitivity and 86% specificity.674
8.2. Intestinal diseases
8.2.1. Metal oxide sensors.
A single metal oxide sensor combined with a GC system was used to differentiate patients with IBD from those with IBS.675 Here the researchers used a tin and zinc oxide paste to create a MOS sensor that served as the detector of the GC system. Interdigitated gold electrodes with 8 interpenetrating bars were constructed and operated at 450 °C. Sensor measurement was continuous and measured the change in electrical resistance. A GC with a static headspace was used to introduce feces samples using a sulfur column after 10 min of incubation at 50 °C (Shepherd SF 2014). An in-house ANN was used to differentiate between the groups. The authors sampled 181 patients from 3 groups (IBS, IBD, healthy). They achieved a distinction of 76% accuracy between IBS and IBD, whereas IBD vs. control and IBS vs. control gave mean accuracies of 79% and 54%, respectively.675 Covington et al.676 studied the plausibility of an electronic nose system based on both MOS and FAIMS technologies. The e-nose contained an array of 6 metal oxide-based sensors, as well as 6 electrochemical sensors, a pellistor (catalytic sensor), and a nondispersive infrared sensor (optical, to monitor CO2). The MOS sensors operated under a constant current source (100 μA), the voltage across the sensor being amplified and monitored. The system was used to classify patients receiving pelvic radiotherapy, which frequently causes gastrointestinal side-effects.676 Stool samples were taken before and 4 weeks after radiotherapy from 23 patients with the highest and lowest level of toxicity. Ten mL samples were heated for 1 h at 40 °C and, after dilution with 5 mL water, the sample was divided for e-nose and FAIMS analyses. Samples were introduced into the sensor chamber at 500 mL min−1 flow rate with 20% RH lab air. The VOCs emitted were products of fermentation caused by gut microflora.676 Using PCA of the electronic nose data (and wavelet transform followed by Fisher discriminant analysis of FAIMS), they showed that it was possible to differentiate patients after treatment by their toxicity levels. Furthermore, differences were also identified in their pre-treatment samples and could be correctly classified in 22 of the 23 samples.676
Aggio et al.677 used a platform composed of a gas chromatography column coupled to metal oxide gas sensors (OdoReader) to analyze fecal VOC patterns of 152 patients: 33 patients with active inflammatory bowel disease; 50 patients with inactive IBD; 28 patients with IBS and 41 healthy donors. Two mL fecal headspace were collected and injected into the GC column-metal oxide gas sensor system. The sensor was controlled by an electronic circuit monitored by computer software, which records the electrical resistance of the sensor at 0.5 s intervals during each 40 min machine run. The resistance profile of each sample generated by the system was used as the input for pattern recognition algorithms, namely SVM and PLS. Several cross-validation methods were used, including leave-one-out cross-validation, 10-fold cross-validation, double cross-validation and their Monte Carlo variations. The most clinically important findings after double cross-validation were the accuracy of active CD vs. irritable bowel syndrome (87%; CI 84–89%) and irritable bowel syndrome vs. controls (78%; CI 76–80%; Fig. 40). The same GC-sensor system was also used to investigate whether fecal VOC levels are associated with a response to dietary interventions in patients with IBS.678 In this study, 93 IBS patients were randomly assigned to a group counselled to follow a diet low in fructans, galacto-oligosaccharides, lactose, fructose and polyols (low-FODMAP diet, n = 46) or a group that received placebo dietary advice (sham diet, n = 47) for 4 weeks. Fecal samples were collected and analyzed at baseline, and at the end of the 4 week study period. The PLS model to classify responders and non-responders on baseline samples showed very high accuracy for both interventions. The PLS model from the low FODMAP diet group predicted responses to the diet with median accuracy, sensitivity and specificity of 100%. However, the same PLS model showed low accuracy when classifying baseline samples from the patients in the control group, indicating that the model was specific for the responses to dietary interventions. In both these last studies, the authors did not identify or report the specific VOCs that contributed to the alterations in the profile shifts.
 |
| Fig. 40 VOCs were extracted from fecal samples and analyzed using an in-house-developed gas chromatography-sensor device. The profiles of VOCs were further analyzed using an in-house-developed computer algorithm that identified resistance patterns associated with specific medical conditions. This figure shows the selected features used to differentiate 19 samples from patients with active Crohn's disease (Act-CD) and 28 patients with irritable bowel syndrome (IBS), and a 2-component principal component plot based on the selected features. Reprinted from ref. 677 with permission from Wiley-Blackwell, copyright 2017. | |
8.2.2. Polymer composite sensors.
de Meij et al.679 tested the ability of Cyranose 320 to discriminate between CD and UC by the volatolomic signature from fecal samples of children. In this case–control study, a total of 83 children (29 CD, 26 UC, and 28 age-matched controls) were enrolled. Two g frozen feces from each child were thawed in a sealed container and placed at 37 °C for 1 h, allowing the headspace to fill with VOCs prior to exposure to the sensors. Using internal cross-validated canonical discriminant analysis, the fecal VOC profiles of patients with UC and CD differed from controls; in active disease (sensitivity, specificity: 100%, 100%, 86% and 67%) and in clinical remission (94%, 94%) and (94%, 94%), respectively. Furthermore, CD-patients differed from UC-patients during active disease (97%, 92%), and after reaching clinical remission (88%, 72%). Quantitative analysis was not performed to address the specific differences in the concentrations of fecal VOCs. In another study by de Meij et al., they tested the use of the Cyranose 320 system to detect necrotizing enterocolitis (NEC) by VOCs, emitted from fecal samples.680 Samples from infants born at a gestational age of ≤30 weeks were collected daily, up to 28 days of life. The infants were assigned to 3 subgroups: NEC n = 13, sepsis n = 31, and matched controls n = 14.680 Approximately 0.5 g feces were used in a sealed vacutainer that was heated to 37 °C for 1 h. PCA was used to analyze the results. The authors showed that fecal VOC prints of infants with NEC could be differentiated from controls 2–3 days prior to the onset of clinical symptoms. NEC vs. control at days 2 and 3 gave 83% sensitivity, 75% specificity and 89% sensitivity, 89% specificity, respectively.680
8.3. Infectious diseases
8.3.1. Metal oxide sensors.
A set-up for the detection of C. difficile infection by VOC profiling was prepared using a MOS sensor connected to a GC system.681 Here the authors used a tin and zinc oxide paste to create a MOS sensor that served as the detector in the GC system.681 An interdigitated gold electrode with 8 interpenetrating bars was constructed and operated at 450 °C. Sensor measurement was continuous and measured the change in electrical resistance. A GC with a static headspace was used to introduce feces after 10 min of incubation at 50 °C. An in-house standard stool sample was used throughout the study, and all samples were from people presenting with diarrhea. Fifty C. difficile positive and 50 negative samples were tested. An ANN was used to differentiate between groups. The results showed comparable capability in discriminating between positive and negative samples with sensitivity and specificity of 85% and 80%, respectively.681
8.3.2. Carbon black polymer sensors.
Similar to the work described in Sections 7.1 and 7.2, the authors used the same Cyranose 320 device, containing 32 polymer-based sensors. The possibility of the e-nose to diagnose late-onset sepsis (LOS) in preterm infants was explored.682 In a multi-center study, fecal samples from infants at gestational age of ≤30 weeks were collected daily. Samples collected up to 5 days prior to LOS diagnosis were analyzed using the e-nose and compared to matched controls. Approximately 0.5 g feces were used in a sealed vacutainer that was heated to 37 °C for 1 h. Based on the sensors’ response and combined with the PCA method, the method could discriminate between fecal VOC-profiles of infants with LOS (n = 36) and controls (n = 40) at 3, 2 and 1 day before the onset of LOS.682 Sensitivity and specificity were moderate at best and spanned between 57.1% to 75% and 61.5% to 70.8%, respectively.
9. Saliva-based disease diagnostics
Saliva samples have been studied in different clinical and non-clinical applications.21 VOCs are assumed to reach the saliva by passive diffusion from the blood.8 Several studies have evidence of a correlation between VOCs in the blood and saliva.683 In addition to VOCs from blood, saliva can potentially contain VOCs coming from other endogenous sources, such as gingival exudate, the nasal cavity, gastrointestinal reflux, oral cavity microorganisms and exogenous sources, such as food debris, commercial products and environmental pollution.8
10. Skin-based disease diagnostics
Skin is the largest human organ being 12–15% of body weight.684 Skin, in particular sweat, could be informative in a number of clinical observations and diagnosis. For example, by utilizing tattoo-based sensors on the skin, quantitative chemical analysis of the sweat, pH, glucose and sodium levels monitoring is possible.685–687 An additional approach is based on sampling the gaseous phase, the skin headspace. The skin emits a wide range of VOCs as metabolism products that contribute to a person's body odor. The difference between the secretion glands (eccrine, sebaceous and apocrine glands) and their heterogeneous distribution across the body results in different VOC profiles and thus different odors from assorted body regions. Even though different body regions share a considerable number of compounds, there are significant quantitative and qualitative differences in their VOC profiles. An additional factor comes from different bacterial populations, mostly aerobic, localized in different body regions. Many of these populations decompose long-chain body metabolism products into short-chain VOCs, including ketones, aldehydes, alcohols, and acids.688 Most of the studies concentrate on the VOC signature from the axillary area, which is rich in apocrine glands and the primary source of human body odor. The emitted odors are of great interest for an almost unlimited number of potential applications, including forensic, biological,689 diagnostic tools, search for entrapped humans690 and many others. Diseases or disorders that interfere with the homeostatic harmony of the body result in changes in the skin VOC pattern both in quality and quantity. These changes result in characteristic odors.25,640,691,692 For example, scarlet fever infection is characterized by a distinctive foul odor from the skin.693 Investigation of healthy human skin has been reported from many studies.21,684,688,694–701 de Lacy Costello et al. examined 532 different skin VOCs collected from healthy subjects.21 Despite the enormous potential of the skin VOC pattern for diagnosing diseases, not many studies have been conducted on the subject. One of the major obstacles is there being no protocol standardization nowadays for skin VOC collection and analysis in terms of sampling location, duration, cleaning procedure, sampling methods and spectrometric analysis.684,690,696,697,699,700,702–712 The most common sampling method is the SPME directly from the skin702 or from absorbing materials which have been in direct contact with the body.703,704 The majority of skin-based diagnosis studies focused on a spectrometric analysis of skin-related diseases, such as skin cancer,703 wounds640,713–715 and VOCs associated with mosquito attraction.711,712,716 The implementation of gas sensor technology for disease diagnosis from the skin is still premature and includes reports for only a few diseases.571,573,703,704,717,718 There is also a tremendous potential for diabetes monitoring by detecting the disease-related VOCs from the skin;705,706 however, no report on a medical device for this monitoring has been published. With the rapid advancement in flexible gas sensors,23,46 we hope to see more activity in this field.
10.1. Melanoma cancer
Many efforts have been invested in sampling the headspace of melanoma biopsies, as mentioned in Section 4.1. However, only one study703 has investigated the VOC pattern associated with melanoma directly from skin headspace. This sampling should reduce the diagnosis period, allowing early screening and detection. D'Amico et al.703 investigated VOC pattern differences emitted by melanoma lesions, nevi and healthy skin, from the same subject by sampling with a gauze pad.
10.1.1. Quartz crystal microbalance sensors.
D'Amico et al.703 also reported a gas sensor array for discriminating between nevi and melanomas by using 7 QCM sensors with different metalloporphyrins.719,720 A metal cylinder with 4 cm diameter was used to isolate and sample the suspected lesions and nevi from 40 subjects. Comparing the responses of each sensor to melanoma lesions and nevi, a large variance in the former samples was observed. Only 2 sensors from the array showed a significantly higher mean value of the response to the melanoma samples compared to nevi. Additional assessment of the array performance was studied in a sub-group of 7 subjects before and after surgical removal of the lesion or nevi. Based on the collected responses, a PLS-DA resulted in 87% correct differentiation between melanoma and nevi samples. Pennazza et al.571 reported the use of a gas sensor array based on 7 QCMs with different metalloporphyrins721 for the detection of melanoma in both skin and headspace of biopsy samples (as mentioned in Section 4.1). A 4 cm diameter stainless-steel cylinder was used to isolate the investigated lesions among 40 subjects, 10 of whom had melanoma lesions. Each subject was sampled before and after surgical removal. The analysis demonstrated a significant change in the response for each sensor between those taken before and after surgical removal of the melanoma. A further PLS-DA showed a correct classification of 87% among the tested samples with sensitivity and specificity of 70% and 90%, respectively.571 In a further study, Pennaza et al.573 demonstrated the additional use of the gas sensor array in detecting melanoma development in both mice skin and headspace of biopsy samples (as mentioned in Section 4.1.7). Xenografted mice from 2 melanoma cell lines and tumor-free mice were sampled periodically with a metallic calendric chamber by a gas sensor array based on QCM with different metalloporphyrins: Cu(TBuOPP); Zn(TBuOPP); Fe(TBuOPP); Sn(TBuOPP); Ru(TBuOPP) and Cr(TBuOPP).721 In a PCA analysis, they showed discrimination between control and xenografted skin headspace samples. Pattern changes as a function of sampling time in coordination with the tumor growth phase were also observed.573
10.2. Schizophrenia
Skin-related diseases are not the only diseases associated with detection of VOCs from the skin; studies on schizophrenia detection have also been reported. Schizophrenia is a severe mental disorder characterized by hallucinations, delusions and cognitive deficits. The life-risk of the disorder is ∼1% and it is assumed that it is caused by multiple factors, including hereditary and environmental factors. The current methods of diagnosis are based mostly on behavioral signs and neuropsychological testing.
10.2.1. Quartz crystal microbalance sensors.
In addition to GC-MS findings, Di Natale et al.704 described a gas sensor analysis of skin odor collected with a cotton compress applied for 30 min on the upper side of the forearm. This study included 27 individuals, subjects with schizophrenia, other mental disorders and healthy volunteers. All patients were treated with anti-psychotic drugs. Prior to sampling, a strict cleaning protocol was used on each subject. After the sampling, the compress cotton was closed in a sealed vial, held at 25 °C for 30 min and then exposed to the sensor chamber. An array of 7 QCM sensors with different metalloporphyrins was used. Metalloporphyrin-based sensors have good sensitivity to carboxylic acids, as the potential candidate for detecting trans-3-methyl-2-hexenoic acid, based on previous GC-MS studies.704,709,710 In a linear discriminant analysis of the gas sensor array, more than 80% correct classifications were achieved.
10.3. Heart failure
10.3.1. Metal oxide sensors.
Voss et al.717 reported the sensors’ ability to detect heart failure, this time by analyzing skin emitted VOCs. The sensor head was placed in the crook of the arm. Ten measurements were taken for every patient over 35 min in the same air-conditioned room to avoid any environmental influences. In this study, 27 patients with DHF, 25 patients with CHF and 28 controls were involved. This time, DFA analysis of compensated vs. decompensated HF resulted in 87% discrimination accuracy and 85% discrimination accuracy between CHF and controls. These authors also reported on significant correlations between the calculated sensors’ responses and clinical laboratory parameters, such as blood bicarbonate and pH levels. However, they did not report on the quantitative identification of the VOCs that affected the sensors’ performance.
10.4. Renal diseases
10.4.1. Metal oxide sensors.
Voss et al.718 assessed the skin/body odor of renal failure patients with an array of 3 thick-film MO-based gas sensors with heater elements. Each of the sensors had a slightly different sensitivity to odorant molecules. Molecular components from the skin of 42 dialysis patients, 20 CKD patients (stages 3–5) and 11 healthy controls were allowed to pass directly to the sensors. Sensor resistance was measured in the range of 200–385 °C, which lasted approximately 2 min. Quadratic discriminant analysis on the corrected signals (normalized to the calibration mixture) of the 1st and 2nd PC from the 2 groups of renal patients gave a correct classification of 95.2% (3 patients were misclassified), whereas correct classification increased to 98.4% (only 1 patient being misclassified) using 1st–3rd PC. The authors also tested the correlation between the PC1 and PC2 for several clinical parameters. For example, they found a significant correlation between the principal components and blood albumin (r = −0.69), pH (r = −0.55) and creatinine (r = −0.52).
11. Hybrid sensor array
Previous sections (5–10) provided detailed references on technological achievements in the VOC diagnostic field. To take diagnostics to the next level, we should use this vast database to improve sensing technology. In this section, we address this issue and provide possible solution and explain how to use the available data of VOC disease-print for improving the technology. The rapid technological development, together with ongoing necessities, drives researchers to develop new nano-based sensors. Currently, as thoroughly discussed above, sensors based on different technologies are being used for VOC sampling e.g., MOS, metal nanoparticles, CNTs, optic, piezoelectric, conducting polymers, and others in a range of applications. Yet in most cases, studies have concentrated on a single array technology and single transduction technology, such as conductance, mass, current and absorption changes. However, in terms of data analysis and pattern recognition, when analyzing electronic sensor array system applications (such as electronic noses), maximum orthogonality is required in the selection of many independent features.722 Consequently, basing an array on a single transducer clearly leads to a limited power of resolution of such sensor arrays.722 Therefore, the concept of Hybrid Sensor Arrays (multi-transducer arrays) has been developed, in which different construction techniques are used, leading to lower correlations between the responses of the different sensor types, which in turn strengthens the power of data analysis.723 Thus, a hybrid array involves multivariable transducers with carefully selected excitation settings to deliver independent outputs allowing recognition of different responses to VOCs (Fig. 41).
 |
| Fig. 41 Hybrid sensors are composed of a variety of sensing layer and different transducers to create multidimensional orthogonal responses. | |
While such hybrid arrays have been frequently advocated, they are scarcely used for VOC detection in general, and disease detection in particular. More than 20 years ago, Holmberg et al. described the use of a hybrid sensor array based on 3 approaches, MOSFET, chemiresistors and optical sensors for determining the quality of paper by its odor. Using 2 MOFSET and 2 chemiresistors, they achieved the best classification.724 Ulmer et al. showed that an array of 4 different types of transducers could potentially operate better than one.725 They used a combination of MOS, QCM, electrochemical and calorimetric sensors in the array. System performance was evaluated on food samples, and the results clearly showed a higher resolution power of the tested samples when using different transducers rather than just one.725 The use of hybrid arrays, however, does not always ensure better classification. Moreover, a weak visible grouping based on PCA plots or cluster analysis does not necessarily indicate poor classification. The important factor would be to lower the actual quantitative indicator of performance, i.e., the classifiers’ test-set error.723 High dimensionality spaces in pattern recognition can often lead to a bad classification, therefore another important part in the analysis would be the selection of an appropriate feature (sensor). In some cases, it could be that all the sensors together do not lower significantly the test-set error compared to a single class of sensors, but a correctly chosen subset does.723 Pardo et al. evaluated the latter points using an array based on 7 QCM, 8 semiconductor and 4 electrochemical sensors on different data-sets of VOC emitting sources. After analyzing the data based on all sensors and using single or combined technology, they concluded the following among other factors: (1) feature selection increases the operation of any given sensor class by 3 to 5%, even when using just 2 or 3 sensors; (2) the performance achieved with the whole hybrid array (no feature selection) is superior to the performance of the top chosen sensor subsets for any single sensor type; (3) when selecting the best sensor subsets from the hybrid array, the performance increases, leading to a 35% reduction in the classification error in the tested case.723 Jin et al. evaluated the use of 3 different transducers, cantilevers, capacitors, and calorimeters, coated with 5 different sorptive-polymer films.726 They evaluated VOCs and VOC mixtures with single-transducer arrays of up to 5 sensors and hybrid arrays of up to 15 sensors. They showed that the best hybrid arrays constantly outperform the best single-transducer arrays of similar size. Furthermore, recognition rates were not improved significantly by using more than 5 sensors in a hybrid array for any particular analysis, regardless of difficulty.726 In a following study, they evaluated the recognition limit for binary and ternary VOC mixtures with a similar hybrid array.727 A few important conclusions were drawn using a hybrid array; first, the VOCs’ relative concentration ratios are considerably more significant factors of performance (recognition of each) than are their absolute concentrations. Second, when performing a targeted-VOC analysis in a mixture, as long as the sensitivity to that VOC is better than its interfering VOCs, it should be identifiable over a range of concentrations even if it is the marginal component.727 In an in vitro study monitoring anti-fungal efficacy based on VOC analysis, researchers used a sensor array hybrid.728 The hybrid consisted of MOS and MOSFET sensors, but no clear data were provided regarding the separate performance of each type. The use of the hybrid array could possibly discriminate between effective and non-effective concentrations of anti-fungal compounds based on VOCs print.728 Originally, each component of a hybrid sensor array is not connected, but each is constructed differently, as described above. Yet in some cases, researchers use the same base technology for the receptor part of the sensor while using different technologies for the transducer part. One such example is described in Li et al.729 in which they used the same 4 gold nanoparticle covered ligands as a receptor and connected it to 2 different transducers. In the first case, they placed it as a sensing layer in chemiresistors, and in the second as a sensing layer in QCM, thus creating a hybrid array with a similar receptor and different transducers. They tested 15 different VOCs and their gas evaluation result showed an increase in classification when using the hybrid technologies rather than using just one technology.729 Similarly, the VOC response to a surface modified SWCNT (as the receptor) and 2 different transducer platforms, chemiresistors (CR) and QCM, was evaluated (Fig. 42a).730 The study showed that even when using the same coating layer (receptor) on both QCM and CR, the QCM response reached equilibrium faster than CR. The proposed explanation is that vapor molecules take longer to intercalate between the junctions of 2 nanotubes, thus delaying the increase in resistance in the CR (Fig. 42b).730 With respect to response patterns, this additional mechanism involved in changes of resistance directed the differences between QCM and chemiresistor patterns; thus the same set of SWCNTs will prompt diverse response patterns on 2 diverse sensor array transducers.730
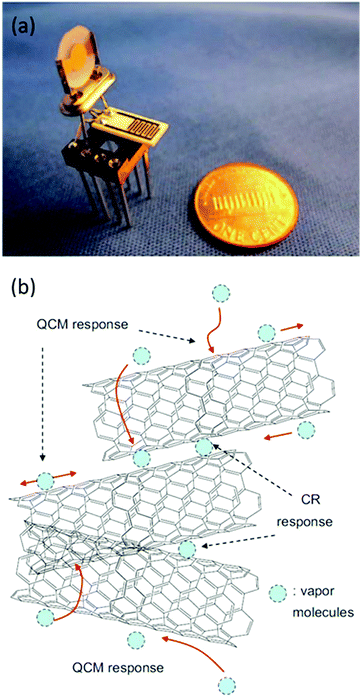 |
| Fig. 42 Hybrid sensing: (a) photo of a hybrid QCM and chemiresistor, (b) scheme for the response mechanism of pristine SWCNT-coated QCMs and chemiresistors. Reprinted from ref. 730 with permission from Elsevier, copyright 2015. | |
In yet another work comparing the performance of 2 types of transducers (QCM, CR) for VOC detection, the authors concluded that response diversity in the hybrid (multi-transducer) array is modestly greater than a similar dimensional (i.e., same number of sensors) single transducer array.731 The hybrid approach can allow multidimensional measurement that can react to the VOC mixture in a complementary manner, thus providing higher sensitivity and consequently better resolution. Using different transducer platforms on top of different sensing layers introduces an additional degree of freedom for selection and analysis of data. Novel hybrid arrays will bring new ways of thinking about measurements of interfering compounds. Instead of trying to avoid their measurement, the hybrid array will measure them but in a different orthogonal direction than the VOC of interest, allowing clearer observation. Alternatively, studies also refer to an adaptable chemosensor approach in which operating parameters of the system can adapt and change based on application needs and ambient changes.732 Such adaptation could be on the tunable level of the device itself, such as internal sensing alteration of operation voltages and frequencies, as well as adaptation of post-data analysis with different filters, machine learning and statistical tools that might tune in real-time sensing factors.732 One example would be using temperature-programmed metal oxide sensors, in which heating operating element changes during analysis, resulting in different responses overcoming complex environmental measurements.733 Such an approach can provide a single sensing transducer array with the power of a hybrid array.
Arguably, the hybrid approach is indeed the next promising step of these sensors. In sections above (5–10), we have thoroughly provided examples of the specific sensor technologies that have been successfully used until now in the field of medical diagnostics. These sections, together with the “maps” of VOC-prints we build in (Fig. 2 and 3) and detailed information summarized in Table S1 (ESI†), should serve as basic information, tutorial-like, for designing and developing novel sensor arrays. For example, gastric cancer diagnostics has until now successfully been analyzed from breath by monolayer capped metal nanoparticles, carbon nanotubes and silicon nanowires. This is further supported by in vitro data that used carbon nanotubes and electrochemical sensors. Thus, hybrid arrays combining these 3 technologies would be the logical approach for new breath-based gastric cancer systems. From another example in Sections 5–10, one can see that, for diabetes mellitus diagnostics, a metal oxide sensor can be used successfully for urine samples, and also metal oxides, conducting polymers and optical fibers can be used for breath samples. This information can direct a metal oxide sensor towards hybrid sampling of both urine and breath specimens; cross-validation from 2 sources will likely provide improved accuracy and a better sensing system.
12. Disease sense in the ICT era
Disease management is becoming more and more sophisticated, part of which is due to the fast-technological communication leap. With that, many diseases still suffer from problems and challenges for proper diagnosis, as illustrated in the case of rare diseases (Fig. 43).
 |
| Fig. 43 This illustration presents the main problems in diagnosing diseases, the challenges and part of the web-based solutions that might contribute considerably to patient diagnosis. Reprinted from ref. 734 with permission from Elsevier, copyright 2017. | |
The information and communication (ICT) era relates to the spatiotemporal integration of data collected by a variety of entities, such as telecommunication, computers, software and databases. Today's globalization makes the data available in seconds. These latter factors together with developing technologies open the options for future high impact point-of-care (POC) diagnostics. POC diagnostics relate to medical tests that can be carried out at the bedside with online immediate results, thereby directing immediate clinical treatment.735 As a result, no unnecessary delays occur due to preparation, logistics and analysis, thereby providing fast economical care, particularly in developing countries, or in places that lack laboratory infrastructure and trained healthcare personal.736 Currently a variety of tests are available as POC in clinical applications, such as enzymes, hematology, diabetes mellitus, infectious agents, fertility, metabolites and others.737 Entering the new era of ICT, 3 main aspects will take important roles – miniaturization, parallel analyses and data networking by ICT.737 Miniaturization by chip technology, microfluidics and nano-based sensors will reduce sample volume (liquid or gas) and associated procedures.737 Furthermore, it will enable connection and direct integration into current ICT platforms. Parallel analysis and lab-on-chip approaches enable laboratory scale measurements in a single system. Hybrid system sensor arrays, discussed in the previous section operating on orthogonal platforms, will allow measurements of a range of parameters for general screening purposes using portable systems. ICT platforms, cellphones or other portable devices and potentially human imbedded chips are direct result of these miniaturization and parallelization. Clinical data will be immediately uploaded to cloud-based servers, comparing and supporting deep online analysis. Indeed, for such ideas to be realized, many of the technologies still need standardization for cross-reference analysis and adequate handling of pre- and post-sampling issues; moreover, it will require the clinical community to address personal data security, ethics and political hurdles. Cloud-based servers provide a huge data storage capacity, but constitute a menace to privacy and security of clinical data that potentially can be catastrophic in the wrong hands. Therefore, closed systems are required that can cope with this issue. Moreover, as the future draws us towards online and cloud storage, a new issue arises, namely losing medically relevant data no longer backed up by hardcopy files.
While many sensor technologies are being developed (as we have extensively reviewed above) showing high potential in lab simulations as well as clinical trials, only a few achieve real-world applicability. This is probably the result of the underestimation of the difficulty of the sensing task together with overestimation of sensor array abilities, and the absence of proper data validation.738 Still, many clinical conditions involve numerous parameters. While parallel analysis can be achieved for some, such as in the hybrid system, others are more problematic and in many cases sensor technologies are not sufficiently sensitive, or do not possess the required resolution power to correctly identify and quantify potential biomarkers or conditions. The long-term function of chemical sensing systems is unknown, and thus changing environments will require adaptive techniques to compensate for changes and reduce the system's power requirements.732 Therefore, any development and progression of sensors and sensor arrays aimed for clinical use should follow a complex procedure. Such a workflow is based on individual steps that are constantly validated and reanalyzed to achieve optimal results, and will require years of research and development to mature (Fig. 44). The first step is based on chemical and material engineering work; in this part the basis of the sensing system is established, selecting the correct sensor platform, followed by the selection of an appropriate sensing layer and constructing them together. The second step includes electrical engineering work to integrate different sensors to a cohesive single or multi-array platform. The third step includes both software engineering and analytical chemistry for developing analysis software for single and then after multiple pattern samples; this step is continuously checked by single and multiplex analytical standards and varying concentration to evaluate accuracy, precision, sensitivity, selectivity, LOD, dynamic range, linearity, resolution, response time and life cycle. The fourth step includes clinical, analytical and statistical steps, and mainly involves the performance and data analysis of suitable clinical studies, including blind analysis. The fifth step is the ICT integrating system to a cloud-based database. The last step (a multistep) is post-development scale-up that includes testing the system for manufacturability, stability, reproducibility and regulatory function.
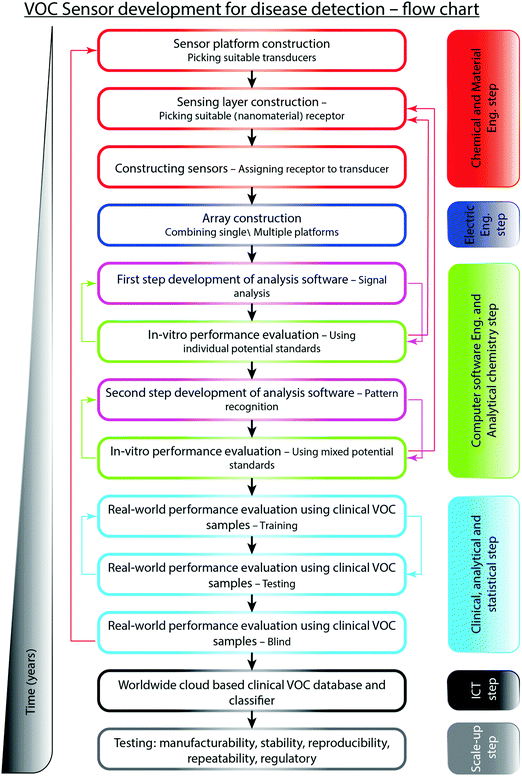 |
| Fig. 44 Workflow process of sensor/sensor array development for clinical use. The process is based on individual steps that are constantly validated and reanalyzed to achieve optimal results. Such a procedure will require years of research and development to mature. | |
13. Conclusion
Clinical diagnostics is one field that does not rest and requires constant improvement in order to cope with new conditions and new diseases. It is well established that efficient screening programs, particularly for diseases, such as cancer, neurological or similar, are crucial for saving and extending life. For example, prognostic data show that early detection can increase survival chance by >50% in 5 year terms for some cancers. In turn, it will also reduce direct and indirect medical cost, allowing governments and health insurance companies to allocate funding for developing novel drugs and improving current treatments. For these to happen, technological improvement is needed and key players would be superior diagnostic sensor arrays that could be easily integrated into POC systems. More specifically, sensor arrays for volatolomic analysis, which is a promising non-invasive diagnostic approach based on the metabolic changes within the body, can provide the means to achieve this improvement. Many new sensors are being studied using a variety of transducers and an even wider variety of sensing layers. However, most of them are at very preliminary phases of construction and low-complexity in vitro evaluation, and serve as proof-of-concept that in most cases do not reflect the real world requirements for applicable diagnostics.
Therefore, the following recommendations are most important:
(1) Multidisciplinary collaborations must be encouraged already from the early stages of the sensor development in order to bridge the vast gap of knowledge transfer between the field of engineering science and the clinical field.
(2) Testing and scaling-up of such systems for real-world experiments using worldwide representative populations.
(3) Evaluating the performance of the sensors under ‘non-sterile’ conditions, which includes consideration of different confounding factors from within the body as well as from the environment. This should be followed by adjustments of suitable techniques in order to test sensors within the true complex settings.
(4) Current empirical and metabolic data estimate the concentrations of medically relevant VOCs to be in the range of ppt and up to hundreds of ppb. Nevertheless, many sensors still do not possess the required sensitivity to detect such levels. Thus new (nano-)materials and approaches, such as hybrid arrays, should be developed to achieve the required sensitivity levels.
(5) Other issues, such as sampling, standardization, logistics and regulation, will as well need further attention to achieve future cohesive diagnostic systems.
The medical world will have to be convinced that VOC detection and identification is valuable for healthcare. The next few years will be critical for such an approach, in which scientists and engineers will need to present the real plausibility of this approach. This will require scale-up, proof of precision and accuracy of the technology. At the same time, we, the researchers of the technology, must be open-minded and listen to the requirements of the medical profession and industry that will bring the products to the market place. Vast funding together with worldwide commercial support could drive this methodology as the next best approach to diagnostics.
Abbreviations
A
| Area |
AA | Advanced adenoma |
AC | Asthma and chronic obstructive pulmonary disease |
ac-DEP | Alternating current dielectrophoresis |
AD | Alzheimer's disease |
ADK | Adenocarcinoma |
AKI | Acute kidney injury |
ANN | Artificial neural network |
AUC | Area under curve |
BAD | Bile acid diarrhea |
BP | Back-propagation |
BTEXN | Benzene, toluene, ethylbenzene, p-xylene, and naphthalene |
C
alveolar
| Alveolar concentration |
CD | Crohn's disease |
CHF | Compensated heart failure |
CI | Confidence interval |
CKD | Chronic kidney disease |
cm | Centimeter |
CMOS | Complementary metal-oxide-semiconductor |
CNTFET | Carbon-nanotube field effect transistor |
CNT | Carbon nanotube |
COPD | Chronic obstructive pulmonary disease |
CR | Chemiresistor |
CRC | Colorectal cancer |
CT | Computed tomography |
CVD | Chemical vapor deposition |
DA | Discriminant analysis |
dB | Decibel |
DC | Drop-casting |
DFA | Discriminant function analysis |
DHF | Decompensated heart failure |
DHT | Dihydroxytryptamine |
DM | Diabetes mellitus |
DMF | Dimethylformamide |
DNA | Deoxyribonucleic acid |
E. coli
|
Escherichia coli
|
EDA-G | Ethylenediamine-modified reduced graphene oxide |
EGFR | Epidermal growth factor receptor |
EML4-ALK | Echinoderm microtubule-associated protein-like 4 fused to the anaplastic lymphoma kinase gene |
ESRD | End-stage renal disease |
eV | Electron volt |
FAIMS | Field asymmetric ion mobility spectroscopy |
FET | Field effect transistor |
FN | False negative |
FODMAP | Fermentable, oligo-, di-, mono-saccharides and polyols |
FP | False positive |
GC | Gastric cancer |
GC-MS | Gas chromatography linked with mass spectrometry |
GCE | Glass carbon electrode |
GNP | Gold nanoparticle |
GO | Graphene oxide |
GPa | Gigapascal |
GR | Graphene |
HBC | Hexa-peri-hexa-benzocoronene |
HBEC | Human bronchial epithelial cell |
HCC | Hepatocellular carcinoma |
HD | Hemodialysis |
HER2 | Human epidermal growth factor receptor 2 |
HF | Heart failure |
HiPco | High-pressure carbon monoxide disproportionation |
HIV | Human immunodeficiency virus |
HMP | High metastatic potential |
HNSCS | Head and neck squamous cell carcinoma |
HTS | Hexyltrichlorosilane |
IBD | Inflammatory bowel disease |
IBE | Immortal bronchial epithelium |
IBS | Irritable bowel syndrome |
ICT | Information communications technology |
IPA | Isopropanol |
iPD | Idiopathic PD |
IPF | Idiopathic pulmonary fibrosis |
K | Kelvin |
kHz | Kilo hertz |
KIM-1 | Kidney injury molecule-1 |
KRAS | Kirsten rat sarcoma |
LbL | Layer-by-layer |
LC | Lung cancer |
LMP | Low metastatic potential |
LOOCV | Leave one out cross validation |
LOS | Late-onset sepsis |
LV | Latent variable |
m | Meter |
M | Molar |
MAO-B | Monoamine oxidase B |
MCMNP | Monolayer-coated metal nanoparticle |
MCNP | Monolayer-coated nanoparticle |
MCR | Misclassification rate |
MLP | Multi-layer perceptron |
MM | Malignant mesothelioma |
MO | Metal oxide |
MOFSET | Metal-oxide-semiconductor field-effect transistor |
MPa | Megapascal |
MRI | Magnetic resonance imaging |
MS | Multiple sclerosis |
MTB | Mycobacterium tuberculosis |
MWCNT | Multi-walled carbon nanotube |
NAD+ | Oxidized nicotinamide adenine dinucleotide |
NADH | Reduced nicotinamide adenine dinucleotide |
NDT | 1,9-Nonanedithiol |
NEC | Necrotizing enterocolitis |
nm | Nanometer |
NMR | Nuclear magnetic resonance spectroscopy |
NP | Nanoparticle |
NPV | Negative predictive value |
NSCLC | Non-small cell lung cancer |
NT | Nanotube |
NW | Nanowire |
OC | Ovarian cancer |
ODA | Oleylamine |
OF | Optic fiber |
OHDA | Hydroxydopamine |
OLGIM | Operative link on gastric intestinal metaplasia |
P
| Pressure |
P3HT | Poly-3-hexylthiophene |
PAA | Polyacrylic acid |
PABS | Poly(m-aminobenzene sulfonic acid) |
PAH | Polycyclic aromatic hydrocarbons |
PAN | Polyacrylonitrile |
PANI | Polyaniline |
PC1 | Principal component 1 |
PCA | Principal component analysis |
PD | Parkinson's disease |
PDMS | Polydimethylsiloxane |
PECH | Polyepichlorohydrin |
PEDOT:PSS | Poly(3,4-ethylenedioxythiophene):poly(styrenesulfonate) |
PEG | Polyethylene glycol |
PEI | Polyethyleneimine |
PET | Polyethylene terephthalate |
PgR | Progesterone receptor |
pH | Potential of hydrogen |
PI | Polyisoprene |
PLD | Pulsed laser deposition |
PLS | Partial least squares |
PMMA | Polymethylmethacrylate |
POC | Point-of-care |
ppb | Parts per billion |
ppm | Parts per million |
ppq | Parts per quadrillion |
PPr | Polypyrene |
ppt | Parts per trillion |
PS | Polystyrene |
PSL-DA | Discriminant analysis combined with partial least square |
PVA | Poly(vinyl acetate) |
PVDF | Poly(vinylidene fluoride) |
PVP | Polyvinylpyrrolidone |
PPV | Positive predictive value |
PZT | Ferroelectric lead zirconium titanate |
QCM | Quartz crystal microbalance |
RGO | Reduced graphene oxide |
RH | Relative humidity |
RIF | Rifampicin |
RN | Random network |
ROC | Receiver operating characteristic |
s | Second |
S | Siemens |
SAW | Surface acoustic wave |
SCC | Squamous cell carcinoma |
SCLC | Small cell lung cancer |
SD | Source-drain |
SEM | Scanning electron microscope |
S-G | Sulfonated reduced graphene oxide |
sh-crl-PU | Self-healing disulfide-cross-linked polyurethane |
sh-μAgPU | Self-healable silver-polymer (μag-polyurethane diol) composite |
SiNW | Silicon nanowire |
STW | Surface transverse wave |
SPME | Solid-phase microextraction |
SVM | Support vector machine |
SWCNT | Single-walled carbon nanotube |
TB | Tuberculosis |
TEM | Transmission electron microscopy |
TFT | Thin-film transistor |
Tg | Transgenic rat |
TN | True negative |
TP | True positive |
TPSS | Tetrakis(4-sulfophenyl)porphine |
UC | Ulcerative colitis |
UTI | Urinary tract infection |
UV | Ultraviolet |
V | Voltage |
VA | Vertically aligned |
VOC | Volatile organic compound |
W | Watt |
WHO | World health organization |
WT | Wild type |
wt% | Weight percentage |
ZIF | Zeolitic imidazolate framework |
Ω | Ohm |
Conflicts of interest
There are no conflicts to declare.
Acknowledgements
This work received funding from the Horizon 2020 ICT Program under the SNIFFPHONE (grant agreement no. 644031). This work received funding from the Phase-II Grand Challenges Explorations award of the Bill and Melinda Gates Foundation (Grant ID: OPP1109493). This work received funding from the Britain Israel Research and Academic Exchange Partnership (BIRAX; Grant 62BX14RBHH). The authors acknowledge Prof. Jin Han for fruitful discussions, Dr Viki Kloper for help with artwork production, Ms Shifaa Bdarneh for help with constructing the disease-related VOC table and Dr Falk Fish for a detailed review of the manuscript. R. V. acknowledges The Ariane de Rothschild Foundation for a PhD scholarship.
References
- T. McKeown, BMJ, 1983, 287, 594 CrossRef PubMed.
- W. M. Patterson, Family Practice, 1985, 2, 1–3 CrossRef PubMed.
- World Health Organization (WHO), Introduction in WHO global status report on noncommunicable diseases, Geneva, Switzerland, 2010.
-
M. Kuhn, W. Goebel, D. J. Philpott and P. J. Sansonetti, Overview of the bacterial pathogens, 2002 Search PubMed.
- World Health Organization (WHO), Communicable disease alert and response for mass gathering: key considerations, 2008.
- J. Karnon, E. Goyder, P. Tappenden, S. T. McPhie, I. Towers, J. Brazier and J. Madan, Health Technol Assess, 2007, 11, 1–145 Search PubMed.
- Y. Y. Broza, R. Kremer, U. Tisch, A. Gevorkyan, A. Shiban, L. A. Best and H. Haick, Nanomedicine: NBM, 2013, 33, 92 Search PubMed.
- Y. Y. Broza, P. Mochalski, V. Ruzsanyi, A. Amann and H. Haick, Angew. Chem., Int. Ed., 2015, 54, 11036–11048 CrossRef PubMed.
- K. Rohlenova, K. Veys, I. Miranda-Santos, K. De Bock and P. Carmeliet, Trends Cell Biol., 2017, 16, 30201–30205 Search PubMed.
- A. Amann, M. Corradi, P. Mazzone and A. Mutti, Expert Rev. Mol. Diagn., 2011, 11, 207–217 CrossRef PubMed.
- W. Miekisch, J. K. Schubert and G. F. Noeldge-Schomburg, Clin. Chim. Acta, 2004, 347, 25–39 CrossRef PubMed.
- M. Hakim, Y. Y. Broza, O. Barash, N. Peled, M. Phillips, A. Amann and H. Haick, Chem. Rev., 2012, 112, 5949–5966 CrossRef PubMed.
- B. Buszewski, M. Kesy, T. Ligor and A. Amann, Biomed. Chromatogr., 2007, 21, 553–566 CrossRef PubMed.
- M. K. Nakhleh, Y. Y. Broza and H. Haick, Nanomedicine, 2014, 9, 1991–2002 CrossRef PubMed.
- B. J. Hunt and K. M. Jurd, BMJ, 1998, 316, 1328–1329 CrossRef PubMed.
- C. Michiels, Am. J. Pathol., 2004, 164, 1875–1882 CrossRef PubMed.
- H. Haick, Y. Y. Broza, P. Mochalski, V. Ruzsanyi and A. Amann, Chem. Soc. Rev., 2014, 43, 1423–1449 RSC.
- D. K. Chan, C. L. Leggett and K. K. Wang, World J. Gastroenterol., 2016, 22, 1639–1649 CrossRef PubMed.
- P. Trefz, S. Kamysek, P. Fuchs, P. Sukul, J. K. Schubert and W. Miekisch, J. Breath Res., 2017, 11, 1752–7163 CrossRef PubMed.
- G. A. Mills and V. Walker, J. Chromatogr. B: Biomed. Sci. Appl., 2001, 753, 259–268 CrossRef.
- B. de Lacy Costello, A. Amann, H. Al-Kateb, C. Flynn, W. Filipiak, T. Khalid, D. Osborne and N. M. Ratcliffe, J. Breath Res., 2014, 8, 1–29 CrossRef PubMed.
- L. Dormont, J. M. Bessiere and A. Cohuet, J. Chem. Ecol., 2013, 39, 569–578 CrossRef PubMed.
- R. Vishinkin and H. Haick, Small, 2015, 11, 6142–6164 CrossRef PubMed.
- A. Amann, P. Mochalski, V. Ruzsanyi, Y. Y. Broza and H. Haick, J. Breath Res., 2014, 8, 016003 CrossRef PubMed.
- Y. Y. Broza and H. Haick, Nanomedicine, 2013, 8, 785–806 CrossRef PubMed.
- N. Barsan and U. Weimar, J. Electroceram., 2001, 7, 143–167 CrossRef.
- M. E. Franke, T. J. Koplin and U. Simon, Small, 2006, 2, 36–50 CrossRef PubMed.
- P. I. Gouma, A. K. Prasad and K. K. Iyer, Nanotechnology, 2006, 17, S48 CrossRef PubMed.
- E. N. Dattoli, A. V. Davydov and K. D. Benkstein, Nanoscale, 2012, 4, 1760–1769 RSC.
- Z. Fan and J. G. Lu, IEEE Trans. Nanotechnol., 2006, 5, 393–396 CrossRef.
- K.-W. Kao, M.-C. Hsu, Y.-H. Chang, S. Gwo and J. A. Yeh, Sensors, 2012, 12, 7157–7168 CrossRef PubMed.
- H. J. Kim and J. H. Lee, Sens. Actuators, B, 2014, 192, 607–627 CrossRef.
- G. Korotcenkov, Mater. Sci. Eng., B, 2007, 139, 1–23 CrossRef.
- C. M. Hangarter, N. Chartuprayoon, S. C. Hernández, Y. H. Choa and N. V. Myung, Nano Today, 2013, 8, 39–55 CrossRef.
- P. C. Chen, F. N. Ishikawa, H. K. Chang, K. Ryu and C. Zhou, Nanotechnology, 2009, 20, 125503 CrossRef PubMed.
- U. Tisch and H. Haick, MRS Bull., 2010, 35, 797–803 CrossRef.
- S. Chakraborty, A. Sen and H. S. Maiti, Sens. Actuators, B, 2006, 115, 610–613 CrossRef.
- J. Tamaki, T. Maekawa, N. Miura and N. Yamazoe, Sens. Actuators, B, 1992, 9, 197–203 CrossRef.
- S. J. Kim, I. S. Hwang, C. W. Na, I. D. Kim, Y. C. Kang and J. H. Lee, J. Mater. Chem., 2011, 21, 18560–18567 RSC.
- Y. H. Cho, Y. N. Ko, Y. C. Kang, I. D. Kim and J. H. Lee, Sens. Actuators, B, 2014, 195, 189–196 CrossRef.
- T. Sahm, W. Rong, N. Bârsan, L. Mädler and U. Weimar, Sens. Actuators, B, 2007, 127, 63–68 CrossRef.
- Y. J. Hong, J. W. Yoon, J. H. Lee and Y. C. Kang, Chem. – Eur. J., 2014, 20, 2737–2741 CrossRef PubMed.
- S. J. Kim, C. W. Na, I. S. Hwang and J. H. Lee, Sens. Actuators, B, 2012, 168, 83–89 CrossRef.
- H. Zhao, B. Rizal, G. McMahon, H. Wang, P. Dhakal, T. Kirkpatrick, Z. Ren, T. C. Chiles, M. J. Naughton and D. Cai, ACS Nano, 2012, 6, 3171–3178 CrossRef PubMed.
- C. X. Wang, L. W. Yin, L. Y. Zhang, D. Xiang and R. Gao, Sesnors, 2010, 10, 2088–2106 Search PubMed.
- H. Jin, Y. S. Abu-Raya and H. Haick, Adv. Healthcare Mater., 2017, 6, 1700024–1700044 CrossRef PubMed.
- W. T. Koo, S. J. Choi, N. H. Kim, J. S. Jang and I. D. Kim, Sens. Actuators, B, 2016, 223, 301–310 CrossRef.
- K. I. Choi, H. J. Kim, Y. C. Kang and J. H. Lee, Sens. Actuators, B, 2014, 194, 371–376 CrossRef.
- P. M. Perillo and D. F. Rodríguez, J. Alloys Compd., 2016, 657, 765–769 CrossRef.
- Z. P. Li, Q. Q. Zhao, W. L. Fan and J. H. Zhan, Nanoscale, 2011, 3, 1646–1652 RSC.
- Y. Zhang, J. P. Li, G. M. An and X. L. He, Sens. Actuators, B, 2010, 144, 43–48 CrossRef.
- J. S. Jang, S. J. Choi, S. J. Kim, M. Hakim and I. D. Kim, Adv. Funct. Mater., 2016, 26, 4740–4748 CrossRef.
- R. Xing, L. Xu, J. Song, Q. L. Zhou, D. Liu and H. W. Song, Sci. Rep., 2015, 5, 10717 CrossRef PubMed.
- D. Koziej, N. Bârsan, U. Weimar, J. Szuber, K. Shimanoe and N. Yamazoe, Chem. Phys. Lett., 2005, 410, 321–323 CrossRef.
- K. Großmann, S. Wicker, U. Weimar and N. Barsan, Phys. Chem. Chem. Phys., 2013, 15, 19151–19158 RSC.
- P. Shankar and J. B. B. Rayappa, Sci. Lett. J., 2015, 4, 1–18 Search PubMed.
- H. R. Kim, A. Haensch, I. D. Kim, N. Barsan, U. Weimar and J. H. Lee, Adv. Funct. Mater., 2011, 21, 4456–4463 CrossRef.
- N. Ma, K. Suematsu, M. Yuasa, T. Kida and K. Shimanoe, ACS Appl. Mater. Interfaces, 2015, 7, 5863–5869 Search PubMed.
- K. Suematsu, M. Sasaki, N. Ma, M. Yuasa and K. Shimanoe, ACS Sens., 2016, 1, 913–920 CrossRef.
- J. W. Yoon, J. S. Kim, T. H. Kim, Y. J. Hong, Y. C. Kang and J. H. Lee, Small, 2016, 12, 4229–4240 CrossRef PubMed.
- C. S. Lee, Z. Dai, S. Y. Jeong, C. H. Kwak, B. Y. Kim, D. H. Kim, H. W. Jang, J. S. Park and J. H. Lee, Chem. – Eur. J., 2016, 22, 7102–7107 CrossRef PubMed.
- H. Y. Li, L. Huang, X. X. Wang, C. S. Lee, J. W. Yoon, J. Zhou, X. Guo and J. H. Lee, RSC Adv., 2017, 7, 3680–3685 RSC.
- J. Yi, J. M. Lee and W. I. Park, Sens. Actuators, B, 2011, 155, 264–269 CrossRef.
- K. Alving, E. Weitzberg and J. M. Lundberg, Eur. Respir. J., 1993, 6, 1368–1370 Search PubMed.
- J. Morton, R. L. Henry and P. S. Thomas, Pediatr Pulmonol, 2006, 41, 929–936 CrossRef PubMed.
- H. G. Moon, Y. R. Choi, Y. S. Shim, K. I. Choi, J. H. Lee, J. S. Kim, S. J. Yoon, H. H. Park, C. Y. Kang and H. W. Jang, ACS Appl. Mater. Interfaces, 2013, 5, 10591–10596 Search PubMed.
- S. T. Krishnan, J. P. Devadhasan and S. Kim, Anal. Bioanal. Chem., 2017, 409, 21–31 CrossRef PubMed.
- S. Davies, P. Spanel and D. Smith, Kidney Int., 1997, 52, 223–228 CrossRef PubMed.
- S. DuBois, S. Eng, R. Bhattacharya, S. Rulyak, T. Hubbard, D. Putnam and D. J. Kearney, Dig. Dis. Sci., 2005, 50, 1780–1784 CrossRef PubMed.
- D. J. Kearney, T. Hubbard and D. Putnam, Dig. Dis. Sci., 2002, 47, 2523–2530 CrossRef PubMed.
- D. Mutschall, K. Holzner and E. Obermeier, Sens. Actuators, B, 1996, 36, 320–324 CrossRef.
- C. Imawan, F. Solzbacher, H. Steffes and E. Obermeier, Sens. Actuators, B, 2000, 64, 193–197 CrossRef.
- S. S. Sunu, E. Prabhu, V. Jayaraman, K. I. Gnanasekar, T. K. Seshagiri and T. Gnanasekaran, Sens. Actuators, B, 2004, 101, 161–174 CrossRef.
- V. Srivastava and K. Jain, Sens. Actuators, B, 2008, 133, 46–52 CrossRef.
- I. Jimenez, M. A. Centeno, R. Scotti, F. Morazzoni, J. Arbiol, A. Cornet and J. R. Morante, J. Mater. Chem., 2004, 14, 2412–2420 RSC.
- C. Zamani, O. Casals, T. Andreu, J. R. Morante and A. Romano-Rodriguez, Sens. Actuators, B, 2009, 140, 557–562 CrossRef.
- P. Gouma, K. Kalyanasundaram, X. Yun, M. Stanacevic and L. Wang, IEEE Sens. J., 2010, 10, 49–53 CrossRef.
- A. T. Güntner, M. Righettoni and S. E. Pratsinis, Sens. Actuators, B, 2016, 223, 266–273 CrossRef.
- P. Galassetti, B. Novak, D. Nemet, C. Rose-Gottron, D. M. Cooper, S. Meinardi, R. Newcomb, F. Zaldivar and D. R. Blake, Diabetes Technol. Ther., 2005, 7, 115–123 CrossRef PubMed.
- S. J. Kim, S. J. Choi, J. S. Jang, H. J. Cho and I. D. Kim, Acc. Chem. Res., 2017, 50, 1587–1596 CrossRef PubMed.
- J. King, A. Kupferthaler, K. Unterkofler, H. Koc, S. Teschl, G. Teschl, W. Miekisch, J. Schubert, H. Hinterhuber and A. Amann, J. Breath Res., 2009, 3, 027006 CrossRef PubMed.
- C. Deng, J. Zhang, X. Yu, W. Zhang and X. Zhang, J. Chromatogr. B: Anal. Technol. Biomed. Life Sci., 2004, 810, 269–275 CrossRef.
- S. J. Choi, I. Lee, B. H. Jang, D. Y. Youn, W. H. Ryu, C. O. Park and I. D. Kim, Anal. Chem., 2013, 85, 1792–1796 CrossRef PubMed.
- S. J. Choi, S. J. Kim, H. J. Cho, J. S. Jang, Y. M. Lin, H. L. Tuller, G. C. Rutledge and I. D. Kim, Small, 2016, 12, 911–920 CrossRef PubMed.
- S. J. Kim, S. J. Choi, J. S. Jang, N. H. Kim, M. Hakim, H. L. Tuller and I. D. Kim, ACS Nano, 2016, 10, 5891–5899 CrossRef PubMed.
- M. Righettoni, A. Tricoli and S. E. Pratsinis, Anal. Chem., 2010, 82, 3581–3587 CrossRef PubMed.
- M. Righettoni, A. Tricoli and S. E. Pratsinis, Chem. Mater., 2010, 22, 3152–3157 CrossRef.
- M. Righettoni and A. Tricoli, J. Breath Res., 2011, 5, 037109 CrossRef PubMed.
- J. Shin, S. J. Choi, I. Lee, D. Y. Youn, C. O. Park, J. H. Lee, H. L. Tuller and I. D. Kim, Adv. Funct. Mater., 2013, 23, 2357–2367 CrossRef.
- W. T. Koo, S. J. Choi, J. S. Jang and I. D. Kim, Sci. Rep., 2017, 7, 45074 CrossRef PubMed.
- K. H. Kim, S. J. Kim, H. J. Cho, N. H. Kim, J. S. Jang, S. J. Choi and I. D. Kim, Sens. Actuators, B, 2017, 241, 1276–1282 CrossRef.
- W. T. Koo, S. Yu, S. J. Choi, J. S. Jang, J. Y. Cheong and I. D. Kim, ACS Appl. Mater. Interfaces, 2017, 9, 8201–8210 Search PubMed.
- M. Righettoni, A. Tricoli, S. Gass, A. Schmid, A. Amann and S. E. Pratsinis, Anal. Chim. Acta, 2012, 738, 69–75 CrossRef PubMed.
- S.-J. Choi, I. Lee, B.-H. Jang, D.-Y. Youn, W.-H. Ryu, C. O. Park and I.-D. Kim, Anal. Chem., 2013, 85, 1792–1796 CrossRef PubMed.
- W.-T. Koo, S.-J. Choi, J.-S. Jang and I.-D. Kim, Sci. Rep., 2017, 7, 45074 CrossRef PubMed.
- N.-H. Kim, S.-J. Choi, S.-J. Kim, H.-J. Cho, J.-S. Jang, W.-T. Koo, M. Kim and I.-D. Kim, Sens. Actuators, B, 2016, 224, 185–192 CrossRef.
- O. Lupan, V. Postica, N. Wolff, O. Polonskyi, V. Duppel, V. Kaidas, E. Lazari, N. Ababii, F. Faupel and L. Kienle, Small, 2017, 13, 1602868 CrossRef PubMed.
- N. H. Kim, S. J. Choi, S. J. Kim, H. J. Cho, J. S. Jang, W. T. Koo, M. K. Kim and I. D. Kim, Sens. Actuators, B, 2016, 224, 185–192 CrossRef.
- J.-W. Yoon and J.-H. Lee, Lab Chip, 2017, 17, 3537–3557 RSC.
- J. Rao, A. Yu, C. Shao and X. Zhou, ACS Appl. Mater. Interfaces, 2012, 4, 5346–5352 Search PubMed.
- S. Xiong, J. Xu, D. Chen, R. Wang, X. Hu, G. Shen and Z. L. Wang, CrystEngComm, 2011, 13, 7114–7120 RSC.
- T. Jinkawa, G. Sakai, J. Tamaki, N. Miura and N. Yamazoe, J. Mol. Catal. A: Chem., 2000, 155, 193–200 CrossRef.
- H. Fan, T. Zhang, X. Xu and N. Lv, Sens. Actuators, B, 2011, 153, 83–88 CrossRef.
- M. Siemons and U. Simon, Sens. Actuators, B, 2007, 126, 181–186 CrossRef.
- L. Wang, Z. Lou, J. Deng, R. Zhang and T. Zhang, ACS Appl. Mater. Interfaces, 2015, 7, 13098–13104 Search PubMed.
- Q. Hao, S. Liu, X. Yin, Z. Du, M. H. Zhang, L. Li, Y. Wang, T. Wang and Q. Li, CrystEngComm, 2011, 13, 806–812 RSC.
- F. Zhang, H. Yang, X. Xie, L. Li, L. Zhang, J. Yu, H. Zhao and B. Liu, Sens. Actuators, B, 2009, 141, 381–389 CrossRef.
- A. Tangerman, Int. Dent. J., 2002, 52, 201–206 CrossRef PubMed.
- M. K. Nakhleh, M. Quatredeniers and H. Haick, Oral Dis., 2017, 1–11 Search PubMed.
- N. S. Ramgir, S. K. Ganapathi, M. Kaur, N. Datta, K. P. Muthe, D. K. Aswal, S. K. Gupta and J. V. Yakhmi, Sens. Actuators, B, 2010, 151, 90–96 CrossRef.
- X. Xue, L. Xing, Y. Chen, S. Shi, Y. Wang and T. Wang, J. Phys. Chem. C, 2008, 112, 12157–12160 Search PubMed.
- Y. Qin, F. Zhang, Y. Chen, Y. Zhou, J. P. Li, A. Zhu, Y. Luo, Y. Tian and J. Yang, J. Phys. Chem. C, 2012, 116, 11994–12000 Search PubMed.
- F. Zhang, A. Zhu, Y. Luo, Y. Tian, J. Yang and Y. Qin, J. Phys. Chem. C, 2010, 114, 19214–19219 Search PubMed.
- X. Liang, T. H. Kim, J. W. Yoon, C. H. Kwak and J. H. Lee, Sens. Actuators, B, 2015, 209, 934–942 CrossRef.
- H. S. Woo, C. H. Kwak, I. D. Kim and J. H. Lee, J. Mater. Chem. A, 2014, 2, 6412–6418 Search PubMed.
- I. Giebelhaus, E. Varechkina, T. Fischer, M. Rumyantseva, V. Ivanov, A. Gaskov, J. R. Morante, J. Arbiol, W. Tyrra and S. Mathur, J. Mater. Chem. A, 2013, 1, 11261–11268 Search PubMed.
- J. Chen, K. Wang, L. Hartman and W. Zhou, J. Phys. Chem. C, 2008, 112, 16017–16021 Search PubMed.
- S. Steinhauer, E. Brunet, T. Maier, G. C. Mutinati, A. Köck, O. Freudenberg, C. Gspan, W. Grogger, A. Neuhold and R. Resel, Sens. Actuators, B, 2013, 187, 50–57 CrossRef.
- J. Tamaki, K. Shimanoe, Y. Yamada, Y. Yamamoto, N. Miura and N. Yamazoe, Sens. Actuators, B, 1998, 49, 121–125 CrossRef.
- I. S. Hwang, J. K. Choi, S. J. Kim, K. Y. Dong, J. H. Kwon, B. K. Ju and J. H. Lee, Sens. Actuators, B, 2009, 142, 105–110 CrossRef.
- S. Steinhauer, E. Brunet, T. Maier, G. C. Mutinati and A. Köck, Sens. Actuators, B, 2013, 186, 550–556 CrossRef.
- X. Gao, C. Li, Z. Yin and Y. Chen, RSC Adv., 2015, 5, 37703–37709 RSC.
- H. L. Yu, L. Li, X. M. Gao, Y. Zhang, F. Meng, T. S. Wang, G. Xiao, Y. J. Chen and C. L. Zhu, Sens. Actuators, B, 2012, 171, 679–685 CrossRef.
- S. Kabcum, N. Tammanoon, A. Wisitsoraat, A. Tuantranont, S. Phanichphant and C. Liewhiran, Sens. Actuators, B, 2016, 235, 678–690 CrossRef.
- J. W. Yoon, Y. J. Hong, Y. C. Kang and J. H. Lee, RSC Adv., 2014, 4, 16067–16074 RSC.
- C. H. Liu, L. Zhang and Y. J. He, Thin Solid Films, 1997, 304, 13–15 CrossRef.
- S. Ma, J. Jia, Y. Tian, L. Cao, S. Shi, X. Li and X. Wang, Ceram. Int., 2016, 42, 2041–2044 CrossRef.
- Y. Wang, Y. Wang, J. Cao, F. Kong, H. Xia, J. Zhang, B. Zhu, S. Wang and S. Wu, Sens. Actuators, B, 2008, 131, 183–189 CrossRef.
- S. Matsuda, T. Kamo, J. Imahashi and F. Nakajima, Ind. Eng. Chem. Fundam., 1982, 21, 18–22 Search PubMed.
- S. Y. Jeong, J. W. Yoon, T. H. Kim, H. M. Jeong, C. S. Lee, Y. C. Kang and J. H. Lee, J. Mater. Chem. A, 2017, 5, 1446–1454 Search PubMed.
- H. M. Jeong, H. J. Kim, P. Rai, J. W. Yoon and J. H. Lee, Sens. Actuators, B, 2014, 201, 482–489 CrossRef.
- H. J. Kim, J. W. Yoon, K. I. Choi, H. W. Jang, A. Umar and J. H. Lee, Nanoscale, 2013, 5, 7066–7073 RSC.
- J. W. Yoon, Y. J. Hong, G. D. Park, S. J. Hwang, F. Abdel-Hady, A. A. Wazzan, Y. C. Kang and J. H. Lee, ACS Appl. Mater. Interfaces, 2015, 7, 7717–7723 Search PubMed.
- S. J. Hwang, K. I. Choi, J. W. Yoon, Y. C. Kang and J. H. Lee, Chem. – Eur. J., 2015, 21, 5872–5878 CrossRef PubMed.
- H. S. Woo, C. H. Kwak, J. H. Chung and J. H. Lee, Sens. Actuators, B, 2015, 216, 358–366 CrossRef.
- H. S. Woo, C. H. Kwak, J. H. Chung and J. H. Lee, ACS Appl. Mater. Interfaces, 2014, 6, 22553–22560 Search PubMed.
- K. Xu, J. Zou, S. Tian, Y. Yang, F. Zeng, T. Yu, Y. Zhang, X. Jie and C. Yuan, Sens. Actuators, B, 2017, 246, 68–77 CrossRef.
- B. Y. Kim, J. H. Ahn, J. W. Yoon, C. S. Lee, Y. C. Kang, F. Abdel-Hady, A. A. Wazzan and J. H. Lee, ACS Appl. Mater. Interfaces, 2016, 8, 34603–34611 Search PubMed.
- H. M. Jeong, J. H. Kim, S. Y. Jeong, C. H. Kwak and J. H. Lee, ACS Appl. Mater. Interfaces, 2016, 8, 7877–7883 Search PubMed.
- J. H. Kim, H. M. Jeong, C. W. Na, J. W. Yoon, F. Abdel-Hady, A. A. Wazzan and J. H. Lee, Sens. Actuators, B, 2016, 235, 498–506 CrossRef.
- S. Liu, Z. Wang, H. Zhao, T. Fei and T. Zhang, Sens. Actuators, B, 2014, 197, 342–349 CrossRef.
- M. Drobek, J.-H. Kim, M. Bechelany, C. Vallicari, A. Julbe and S. S. Kim, ACS Appl. Mater. Interfaces, 2016, 8, 8323–8328 Search PubMed.
- A. T. Güntner, V. Koren, K. Chikkadi, M. Righettoni and S. E. Pratsinis, ACS Sens., 2016, 1, 528–535 CrossRef.
- S. J. Choi, K. H. Ku, B. J. Kim and I. D. Kim, ACS Sens., 2016, 1, 1124–1131 CrossRef.
- H. G. Moon, Y. H. Jung, S. D. Han, Y. S. Shim, B. Shin, T. Lee, J. S. Kim, S. Lee, S. C. Jun and H. H. Park, ACS Appl. Mater. Interfaces, 2016, 8, 20969–20976 Search PubMed.
- E. Dovgolevsky, U. Tisch and H. Haick, Small, 2009, 5, 1158–1161 Search PubMed.
- L. Y. Wang, J. Luo, M. J. Schadt and C. J. Zhong, Langmuir, 2010, 26, 618–632 CrossRef PubMed.
- Y. Joseph, B. Guse, T. Vossmeyer and A. Yasuda, J. Phys. Chem. C, 2008, 112, 12507–12514 Search PubMed.
- U. Tisch and H. Haick, Rev. Chem. Eng., 2010, 26, 171–179 Search PubMed.
- M. C. Daniel and D. Astruc, Chem. Rev., 2004, 104, 293–346 CrossRef PubMed.
- Y. Joseph, B. Guse, A. Yasuda and T. Vossmeyer, Sens. Actuators, B, 2004, 98, 188–195 CrossRef.
- E. García-Berríos, T. Gao, J. C. Theriot, M. D. Woodka, B. S. Brunschwig and N. S. Lewis, J. Phys. Chem. C, 2011, 115, 6208–6217 Search PubMed.
- L. J. Hubble, J. S. Cooper, A. Sosa-Pintos, H. Kiiveri, E. Chow, M. S. Webster, L. Wieczorek and B. Raguse, ACS Comb. Sci., 2015, 17, 120–129 CrossRef PubMed.
- M. Segev-Bar, N. Bachar, Y. Wolf, B. Ukrainsky, L. Sarraf and H. Haick, Adv. Carbon Mater. Technol., 2017, 2, 1600206 CrossRef.
- M. Y. Bashouti, A. S. de la Zerda, D. Geva and H. Haick, J. Phys. Chem. C, 2014, 118, 1903–1909 Search PubMed.
- N. Kahn, O. Lavie, M. Paz, Y. Segev and H. Haick, Nano Lett., 2015, 15, 7023–7028 CrossRef PubMed.
- N. Olichwer, E. W. Leib, A. H. Halfar, A. Petrov and T. Vossmeyer, ACS Appl. Mater. Interfaces, 2012, 4, 6151–6161 Search PubMed.
- H. Wohltjen and A. W. Snow, Anal. Chem., 1998, 70, 2856–2859 CrossRef.
- Q.-Y. Cai and E. T. Zellers, Anal. Chem., 2002, 74, 3533–3539 CrossRef PubMed.
- H. L. Zhang, S. D. Evans, J. R. Henderson, R. E. Miles and T. H. Shen, Nanotechnology, 2002, 13, 439 CrossRef.
- W. Zhao, T. Rovere, D. Weerawarne, G. Osterhoudt, N. Kang, P. Joseph, J. Luo, B. Shim, M. Poliks and C.-J. Zhong, ACS Nano, 2015, 9, 6168–6177 CrossRef PubMed.
- J. Herrmann, K. H. Muller, T. Reda, G. R. Baxter, B. Raguse, G. J. J. B. de Groot, R. Chai, M. Roberts and L. Wieczorek, Appl. Phys. Lett., 2007, 91, 183105 CrossRef.
- E. Homede, M. Abo Jabal, R. Ionescu and H. Haick, Adv. Funct. Mater., 2016, 26, 6359–6370 CrossRef.
-
J. Lombardi, M. D. Poliks, W. Zhao, S. Yan, N. Kang, J. Li, J. Luo, C.-J. Zhong, Z. Pan and M. Almihdhar, IEEE 67th Electronic Components and Technology Conference (ECTC), Orlando, FL, 2017, pp. 764–771 Search PubMed.
- G. Yang and D.
T. Hallinan Jr, Nanotechnology, 2016, 27, 225604 CrossRef PubMed.
- L. Wang, L. Wang, X. Shi, N. N. Kariuki, M. Schadt, G. R. Wang, Q. Rendeng, J. Choi, J. Luo and S. Lu, J. Am. Chem. Soc., 2007, 129, 2161–2170 CrossRef PubMed.
- H. Moreira, J. Grisolia, N. M. Sangeetha, N. Decorde, C. Farcau, B. Viallet, K. Chen, G. Viau and L. Ressier, Nanotechnology, 2013, 24, 095701 CrossRef PubMed.
- C. Farcau, N. M. Sangeetha, H. Moreira, B. Viallet, J. Grisolia, D. Ciuculescu-Pradines and L. Ressier, ACS Nano, 2011, 5, 7137–7143 CrossRef PubMed.
- C. Farcau, H. Moreira, B. Viallet, J. Grisolia, D. Ciuculescu-Pradines, C. Amiens and L. Ressier, J. Phys. Chem. C, 2011, 115, 14494–14499 Search PubMed.
- N. M. Sangeetha, N. Decorde, B. Viallet, G. Viau and L. Ressier, J. Phys. Chem. C, 2012, 117, 1935–1940 Search PubMed.
- L. Y. Wang, J. Luo, J. Yin, H. Zhang, J. M. Wu, X. Shi, E. Crew, Z. Xu, Q. Rendeng and S. Lu, J. Mater. Chem., 2010, 20, 907–915 RSC.
- C. Xu and X. Wang, Small, 2009, 5, 2212–2217 CrossRef PubMed.
- Y. G. Sun and H. H. Wang, Appl. Phys. Lett., 2007, 90, 213107 CrossRef.
- M. Segev-Bar, A. Landman, M. Nir-Shapira, G. Shuster and H. Haick, ACS Appl. Mater. Interfaces, 2013, 5, 5531–5541 Search PubMed.
- M. Segev-Bar and H. Haick, ACS Nano, 2013, 7, 8366–8378 CrossRef PubMed.
- H. Haick, J. Phys. D: Appl. Phys., 2007, 40, 7173–7186 CrossRef.
- O. Masala and R. Seshadri, Annu. Rev. Mater. Res., 2004, 34, 41–81 CrossRef.
- B. L. Cushing, V. L. Kolesnichenko and C. J. O'Connor, Chem. Rev., 2004, 104, 3893–3946 CrossRef PubMed.
- C. J. Murphy, Anal. Chem., 2002, 74, 520A–526A CrossRef PubMed.
- J. Yin, P. P. Hu, J. Luo, L. Wang, M. F. Cohen and C. J. Zhong, ACS Nano, 2011, 5, 6516–6526 CrossRef PubMed.
- X. H. Qian and H. S. Park, J. Mech. Phys. Solids, 2010, 58, 330–345 CrossRef.
- X. H. Qian and H. S. Park, Nanotechnology, 2010, 21, 365704 CrossRef PubMed.
- E. Dovgolevsky, G. Konvalina, U. Tisch and H. Haick, J. Phys. Chem. C, 2010, 114, 14042–14049 Search PubMed.
- E. Dovgolevsky and H. Haick, Small, 2008, 4, 2059–2066 CrossRef PubMed.
- F. Xiao, J. Song, H. Gao, X. Zan, R. Xu and H. Duan, ACS Nano, 2011, 6, 100–110 CrossRef PubMed.
- T. Yonezawa, K. Yasui and N. Kimizuka, Langmuir, 2001, 17, 271–273 CrossRef.
- D. Rautaray, A. Kumar, S. Reddy, S. R. Sainkar and M. Sastry, Cryst. Growth Des., 2002, 2, 197–203 Search PubMed.
- R. A. Potyrailo, Chem. Soc. Rev., 2017, 46, 5311–5346 RSC.
- K. Fu, S. Li, X. Jiang, Y. Wang and B. G. Willis, Langmuir, 2013, 29, 14335–14343 CrossRef PubMed.
- M. Segev-Bar, G. Shuster and H. Haick, J. Phys. Chem. C, 2012, 116, 15361–15368 Search PubMed.
- G. Peng, U. Tisch, O. Adams, M. Hakim, N. Shehada, Y. Y. Broza, S. Billan, R. Abdah-Bortnyak, A. Kuten and H. Haick, Nat. Nanotechnol., 2009, 4, 669–673 CrossRef PubMed.
- Y. Joseph, A. Peic, X. Chen, J. Michl, T. Vossmeyer and A. Yasuda, J. Phys. Chem. C, 2007, 111, 12855–12859 Search PubMed.
- E. García-Berríos, T. Gao, M. D. Woodka, S. Maldonado, B. S. Brunschwig, M. W. Ellsworth and N. S. Lewis, J. Phys. Chem. C, 2010, 114, 21914–21920 Search PubMed.
- Y. Joseph, I. Besnard, M. Rosenberger, B. Guse, H. G. Nothofer, J. M. Wessels, U. Wild, A. Knop-Gericke, D. Su and R. Schlögl, J. Phys. Chem. B, 2003, 107, 7406–7413 CrossRef.
- N. Krasteva, Y. Fogel, R. E. Bauer, K. Müllen, Y. Joseph, N. Matsuzawa, A. Yasuda and T. Vossmeyer, Adv. Funct. Mater., 2007, 17, 881–888 CrossRef.
- N. Krasteva, B. Guse, I. Besnard, A. Yasuda and T. Vossmeyer, Sens. Actuators, B, 2003, 92, 137–143 CrossRef.
- M. D. Hanwell, S. Y. Heriot, T. H. Richardson, N. Cowlam and I. M. Ross, Colloids Surf., A, 2006, 284, 379–383 CrossRef.
- J. P. Choi, M. M. Coble, M. R. Branham, J. M. DeSimone and R. W. Murray, J. Phys. Chem. C, 2007, 111, 3778–3785 Search PubMed.
- M. A. Jabal, E. Homede, L. M. Pismen, H. Haick and A. M. Leshansky, Eur. Phys. J.-Spec. Top., 2017, 226, 1307–1324 CrossRef.
- Y. Y. Broza, L. Har-Shai, R. Jeries, J. C. Cancilla, L. Glass-Marmor, I. Lejbkowicz, J. S. Torrecilla, X. Yao, X. Feng, A. Narita, K. Mullen, A. Miller and H. Haick, ACS Chem. Neurosci., 2017, 8, 2402–2413 CrossRef PubMed.
- A. Karban, M. K. Nakhleh, J. C. Cancilla, R. Vishinkin, T. Rainis, E. Koifman, R. Jeries, H. Ivgi, J. S. Torrecilla and H. Haick, Adv. Healthcare Mater., 2016, 5, 2339–2344 CrossRef PubMed.
- S. Shan, W. Zhao, J. Luo, J. Yin, J. C. Switzer, P. Joseph, S. Lu, M. Poliks and C. J. Zhong, J. Mater. Chem. C, 2014, 2, 1893–1903 RSC.
- H. Amal, D. Y. Shi, R. Ionescu, W. Zhang, Q. L. Hua, Y. Y. Pan, L. Tao, H. Liu and H. Haick, Int. J. Cancer, 2015, 136, E614–E622 CrossRef PubMed.
- H. Jin, T. P. Huynh and H. Haick, Nano Lett., 2016, 16, 4194–4202 CrossRef PubMed.
- T. P. Huynh and H. Haick, Adv. Mater., 2015, 28, 138–143 CrossRef PubMed.
- G. Konvalina and H. Haick, ACS Appl. Mater. Interfaces, 2012, 4, 317–325 Search PubMed.
- R. L. van der Waals, G. M. Berger and T. M. Ticich, Appl. Phys. A: Mater. Sci. Process., 2003, 77, 885–889 CrossRef.
- C. D. Scott, S. Arepalli, P. Nikolaev and R. E. Smalley, Appl. Phys. A: Mater. Sci. Process., 2001, 72, 573–580 CrossRef.
- N. Braidy, M. M. El Khakani and G. G. Botton, J. Mater. Res., 2002, 17, 2189–2192 CrossRef.
- S. Arepalli, J. Nanosci. Nanotechnol., 2004, 4, 317–325 CrossRef PubMed.
- S. Iijima and T. Ichihashi, Nature, 1993, 363, 603–605 CrossRef.
- M. V. Antisari, R. Marazzi and R. Krsmanovic, Carbon, 2003, 41, 2393–2401 CrossRef.
- C. Journet, W. K. Maser, P. Bernier and A. Loiseau, Nature, 1997, 388, 756 CrossRef.
- H. Li, L. Guan, Z. Shi and Z. Gu, J. Phys. Chem. B, 2004, 108, 4573–4575 CrossRef.
- Z. Shi, Y. Lian, X. Zhou, Z. Gu, Y. Zhang, S. Iijima, L. Zhou, K. T. Yue and S. Zhang, Carbon, 1999, 37, 1449–1453 CrossRef.
- J. L. Hutchison, N. A. Kiselev, E. P. Krinichnaya, A. V. Krestinin, R. O. Loutfy, A. P. Morawsky, V. E. Muradyan, E. D. Obraztsova, J. Sloan and S. V. Terekhov, Carbon, 2001, 39, 761–770 CrossRef.
- H. Dai, Carbon Nanotubes, 2001, 29–53 Search PubMed.
- L. C. Qin, D. Zhou, A. R. Krauss and D. M. Gruen, Appl. Phys. Lett., 1998, 72, 3437–3439 CrossRef.
- V. K. Varadan and J. Xie, Smart Mater. Struct., 2002, 11, 610 CrossRef.
- K. Mukhopadhyay and G. N. Mathur, J. Nanosci. Nanotechnol., 2002, 2, 197–201 CrossRef PubMed.
- R. Masud, I. Dauda Sh, M. Asyraf, S. Jarin and A. Tomal, Sens. Rev., 2017, 37, 127–136 CrossRef.
- K. Hata, D. N. Futaba, K. Mizuno, T. Namai, M. Yumura and S. Iijima, Science, 2004, 306, 1362–1364 CrossRef PubMed.
- B. Q. Wei, R. Vajtai and P. M. Ajayan, Appl. Phys. Lett., 2001, 79, 1172–1174 CrossRef.
- M. J. Treacy, T. W. Ebbesen and J. M. Gibson, Nature, 1996, 381, 678 CrossRef.
- W. Zhang, Z. Zhu, F. Wang, T. Wang, L. Sun and Z. Wang, Nanotechnology, 2004, 15, 936 CrossRef.
- P. Avouris, Z. Chen and V. Perebeinos, Nat. Nanotechnol., 2007, 2, 605–615 CrossRef PubMed.
- T. Dürkop, S. A. Getty, E. Cobas and M. S. Fuhrer, Nano Lett., 2004, 4, 35–39 CrossRef.
- T. W. Ebbesen, H. J. Lezec, H. Hiura, J. W. Bennett, H. F. Ghaemi and T. Thio, Nature, 1996, 382, 54–56 CrossRef.
-
M. S. Dresselhaus, G. Dresselhaus and P. C. Eklund, Science of fullerenes and carbon nanotubes: their properties and applications, Elsevier, Orlando, Florida, 1996 Search PubMed.
- E. Pop, D. Mann, Q. Wang, K. Goodson and H. Dai, Nano Lett., 2006, 6, 96–100 CrossRef PubMed.
- P. Kim, L. Shi, A. Majumdar and P. L. McEuen, Phys. Rev. Lett., 2001, 87, 215502 CrossRef PubMed.
- M.-F. Yu, O. Lourie, M. J. Dyer, K. Moloni, T. F. Kelly and R. S. Ruoff, Science, 2000, 287, 637–640 CrossRef PubMed.
- M.-F. Yu, B. S. Files, S. Arepalli and R. S. Ruoff, Phys. Rev. Lett., 2000, 84, 5552 CrossRef PubMed.
- B. Peng, M. Locascio, P. Zapol, S. Li, S. L. Mielke, G. C. Schatz and H. D. Espinosa, Nat. Nanotechnol., 2008, 3, 626–631 CrossRef PubMed.
- J.-P. Salvetat, G. A. D. Briggs, J.-M. Bonard, R. R. Bacsa, A. J. Kulik, T. Stöckli, N. A. Burnham and L. Forró, Phys. Rev. Lett., 1999, 82, 944 CrossRef.
- E. W. Wong, P. E. Sheehan and C. M. Lieber, Science, 1997, 277, 1971–1975 CrossRef.
- A. Zahab, L. Spina, P. Poncharal and C. Marliere, Phys. Rev. B: Condens. Matter Mater. Phys., 2000, 62, 10000 CrossRef.
- G. Peng, U. Tisch and H. Haick, Nano Lett., 2009, 9, 1362–1368 CrossRef PubMed.
- G. Peng, E. Trock and H. Haick, Nano Lett., 2008, 8, 3631–3635 CrossRef PubMed.
- H. Haick, M. Hakim, M. Patrascu, C. Levenberg, N. Shehada, F. Nakhoul and Z. Abassi, ACS Nano, 2009, 3, 1258–1266 CrossRef PubMed.
- Y. Zilberman, U. Tisch, W. Pisula, X. Feng, K. Müllen and H. Haick, Langmuir, 2009, 25, 5411–5416 CrossRef PubMed.
- Y. Zilberman, U. Tisch, G. Shuster, W. Pisula, X. Feng, K. Mullen and H. Haick, Adv. Mater., 2010, 22, 4317–4320 CrossRef PubMed.
- Y. Zilberman, R. Ionescu, X. Feng, K. Müllen and H. Haick, ACS Nano, 2011, 5, 6743–6753 CrossRef PubMed.
- N. Bachar, L. Mintz, Y. Zilberman, R. Ionescu, X. Feng, K. Müllen and H. Haick, ACS Appl. Mater. Interfaces, 2012, 4, 4960–4965 Search PubMed.
- J. Zhao, A. Buldum, J. Han and J. P. Lu, Nanotechnology, 2002, 13, 195 CrossRef.
- T. Zhang, S. Mubeen, N. V. Myung and M. A. Deshusses, Nanotechnology, 2008, 19, 332001 CrossRef PubMed.
- P. Bondavalli, P. Legagneux and D. Pribat, Sens. Actuators, B, 2009, 140, 304–318 CrossRef.
- M. Magno, V. Jelicic, K. Chikkadi, C. Roman, C. Hierold, V. Bilas and L. Benini, IEEE Sens. J., 2016, 16, 8329–8337 Search PubMed.
- J. Kong, N. R. Franklin, C. Zhou, M. G. Chapline, S. Peng, K. Cho and H. Dai, Science, 2000, 287, 622–625 CrossRef PubMed.
- X. Liu, Z. Luo, S. Han, T. Tang, D. Zhang and C. Zhou, Appl. Phys. Lett., 2005, 86, 243501 CrossRef.
- I. Dube, D. Jiménez, G. Fedorov, A. Boyd, I. Gayduchenko, M. Paranjape and P. Barbara, Carbon, 2015, 87, 330–337 CrossRef.
- J. Zhang, A. Boyd, A. Tselev, M. Paranjape and P. Barbara, Appl. Phys. Lett., 2006, 88, 123112 CrossRef.
- N. Peng, Q. Zhang, C. L. Chow, O. K. Tan and N. Marzari, Nano Lett., 2009, 9, 1626–1630 CrossRef PubMed.
- Y. W. Chang, J. S. Oh, S. H. Yoo, H. H. Choi and K.-H. Yoo, Nanotechnology, 2007, 18, 435504 CrossRef.
- C. Zhou, J. Zhao, J. Ye, M. Tange, X. Zhang, W. Xu, K. Zhang, T. Okazaki and Z. Cui, Carbon, 2016, 108, 372–380 CrossRef.
- J. Li, Y. Lu, Q. Ye, L. Delzeit and M. Meyyappan, Electrochem. Solid-State Lett., 2005, 8, H100–H102 CrossRef.
- G. Chen, T. M. Paronyan, E. M. Pigos and A. R. Harutyunyan, Sci. Rep., 2012, 2, 1–7 Search PubMed.
- M. Rinkiö, M. Y. Zavodchikova, P. Törmä and A. Johansson, Phys. Status Solidi B, 2008, 245, 2315–2318 CrossRef.
- W. Kim, A. Javey, O. Vermesh, Q. Wang, Y. Li and H. Dai, Nano Lett., 2003, 3, 193–198 CrossRef.
- S. A. McGill, S. G. Rao, P. Manandhar, P. Xiong and S. Hong, Appl. Phys. Lett., 2006, 89, 163123 CrossRef.
- D. Sung, S. Hong, Y.-H. Kim, N. Park, S. Kim, S. L. Maeng and K.-C. Kim, Appl. Phys. Lett., 2006, 89, 243110 CrossRef.
- A. Star, V. Joshi, S. Skarupo, D. Thomas and J.-C. P. Gabriel, J. Phys. Chem. B, 2006, 110, 21014–21020 CrossRef PubMed.
- S. Mubeen, J. H. Lim, A. Srirangarajan, A. Mulchandani, M. A. Deshusses and N. V. Myung, Electroanalysis, 2011, 23, 2687–2692 CrossRef.
- D. R. Kauffman and A. Star, Nano Lett., 2007, 7, 1863–1868 CrossRef PubMed.
- K. H. An, S. Y. Jeong, H. R. Hwang and Y. H. Lee, Adv. Mater., 2004, 16, 1005–1009 CrossRef.
- P. Qi, O. Vermesh, M. Grecu, A. Javey, Q. Wang, H. Dai, S. Peng and K. J. Cho, Nano Lett., 2003, 3, 347–351 CrossRef.
- C. Staii, A. T. Johnson, M. Chen and A. Gelperin, Nano Lett., 2005, 5, 1774–1778 CrossRef PubMed.
- R. J. Chen, N. R. Franklin, J. Kong, J. Cao, T. W. Tombler, Y. Zhang and H. Dai, Appl.
Phys. Lett., 2001, 79, 2258–2260 CrossRef.
- S. Badhulika, N. V. Myung and A. Mulchandani, Talanta, 2014, 123, 109–114 CrossRef PubMed.
- J. Li, Y. Lu, Q. Ye, M. Cinke, J. Han and M. Meyyappan, Nano Lett., 2003, 3, 929–933 CrossRef.
- J. Suehiro, G. Zhou, H. Imakiire, W. Ding and M. Hara, Sens. Actuators, B, 2005, 108, 398–403 CrossRef.
- D. Kumar, P. Chaturvedi, P. Saho, P. Jha, A. Chouksey, M. Lal, J. S. B. S. Rawat, R. P. Tandon and P. K. Chaudhury, Sens. Actuators, B, 2017, 240, 1134–1140 CrossRef.
- S. M. Jung, H. Y. Jung and J. S. Suh, Sens. Actuators, B, 2009, 139, 425–428 CrossRef.
-
Y. Jiang, P. Wang and L. Lin, IEEE 24th International Conference on Micro Electro Mechanical Systems, Cancun, 2011, pp. 396–399 Search PubMed.
- S. J. Young and Z. D. Lin, Microsyst. Technol., 2016, 1–4 Search PubMed.
- M. Penza, R. Rossi, M. Alvisi and E. Serra, Nanotechnology, 2010, 21, 105501 CrossRef PubMed.
- M. Penza, G. Cassano, R. Rossi, M. Alvisi, A. Rizzo, M. A. Signore, T. Dikonimos, E. Serra and R. Giorgi, Appl. Phys. Lett., 2007, 90, 173123 CrossRef.
- A. Abdelhalim, M. Winkler, F. Loghin, C. Zeiser, P. Lugli and A. Abdellah, Sens. Actuators, B, 2015, 220, 1288–1296 CrossRef.
- M. Penza, R. Rossi, M. Alvisi, M. A. Signore, G. Cassano, D. Dimaio, R. Pentassuglia, E. Piscopiello, E. Serra and M. Falconieri, Thin Solid Films, 2009, 517, 6211–6216 CrossRef.
- D. W. H. Fam, A. I. Y. Tok, A. Palaniappan, P. Nopphawan, A. Lohani and S. G. Mhaisalkar, Sens. Actuators, B, 2009, 138, 189–192 CrossRef.
- R. Leghrib, A. Felten, F. Demoisson, F. Reniers, J.-J. Pireaux and E. Llobet, Carbon, 2010, 48, 3477–3484 CrossRef.
- E. H. Espinosa, R. Ionescu, C. Bittencourt, A. Felten, R. Erni, G. Van Tendeloo, J.-J. Pireaux and E. Llobet, Thin Solid Films, 2007, 515, 8322–8327 CrossRef.
- P. R. Mudimela, M. Scardamaglia, O. González-León, N. Reckinger, R. Snyders, E. Llobet, C. Bittencourt and J.-F. Colomer, Beilstein J. Nanotechnol., 2014, 5, 910 CrossRef PubMed.
- E. Dilonardo, M. Penza, M. Alvisi, C. Di Franco, R. Rossi, F. Palmisano, L. Torsi and N. Cioffi, Sens. Actuators, B, 2016, 223, 417–428 CrossRef.
- J.-C. Charlier, L. Arnaud, I. V. Avilov, M. Delgado, F. Demoisson, E. H. Espinosa, C. P. l. Ewels, A. Felten, J. Guillot and R. Ionescu, Nanotechnology, 2009, 20, 375501 CrossRef PubMed.
- M. Penza, R. Rossi, M. Alvisi, G. Cassano and E. Serra, Sens. Actuators, B, 2009, 140, 176–184 CrossRef.
- J. Liu, Z. Guo, F. Meng, Y. Jia and J. Liu, J. Phys. Chem. C, 2008, 112, 6119–6125 Search PubMed.
- N. Van Hieu, N. Van Duy, P. T. Huy and N. D. Chien, Physica E, 2008, 40, 2950–2958 CrossRef.
- R. Ionescu, E. H. Espinosa, R. Leghrib, A. Felten, J. J. Pireaux, R. Erni, G. Van Tendeloo, C. Bittencourt, N. Canellas and E. Llobet, Sens. Actuators, B, 2008, 131, 174–182 CrossRef.
- E. H. Espinosa, R. Ionescu, B. Chambon, G. Bedis, E. Sotter, C. Bittencourt, A. Felten, J.-J. Pireaux, X. Correig and E. Llobet, Sens. Actuators, B, 2007, 127, 137–142 CrossRef.
- C. Wei, L. Dai, A. Roy and T. B. Tolle, J. Am. Chem. Soc., 2006, 128, 1412–1413 CrossRef PubMed.
- L. He, Y. Jia, F. Meng, M. Li and J. Liu, Mater. Sci. Eng., B, 2009, 163, 76–81 CrossRef.
- T. Zhang, M. B. Nix, B. Y. Yoo, M. A. Deshusses and N. V. Myung, Electroanalysis, 2006, 18, 1153–1158 CrossRef.
- S. Abdulla, T. L. Mathew and B. Pullithadathil, Sens. Actuators, B, 2015, 221, 1523–1534 CrossRef.
- J. K. Abraham, B. Philip, A. Witchurch, V. K. Varadan and C. C. Reddy, Smart Mater. Struct., 2004, 13, 1045 CrossRef.
- T. Zhang, S. Mubeen, E. Bekyarova, B. Y. Yoo, R. C. Haddon, N. V. Myung and M. A. Deshusses, Nanotechnology, 2007, 18, 165504 CrossRef.
- G. P. Evans, D. J. Buckley, A.-L. Adedigba, G. Sankar, N. T. Skipper and I. P. Parkin, ACS Appl. Mater. Interfaces, 2016, 8, 28096–28104 Search PubMed.
- S. F. Liu, L. C. H. Moh and T. M. Swager, Chem. Mater., 2015, 27, 3560–3563 CrossRef.
- H. Xie, C. Sheng, X. Chen, X. Wang, Z. Li and J. Zhou, Sens. Actuators, B, 2012, 168, 34–38 CrossRef.
- S. Sharma, S. Hussain, S. Singh and S. S. Islam, Sens. Actuators, B, 2014, 194, 213–219 CrossRef.
- Y. Lu, C. Partridge, M. Meyyappan and J. Li, J. Electroanal. Chem., 2006, 593, 105–110 CrossRef.
-
X. Xiong and M. Xia, Carbon nanotube-based ultra-sensitive breath acetone sensor for non-invasive diabetes diagnosis, University of Bridgeport, 2012.
- S. Ahmadnia-Feyzabad, A. A. Khodadadi, M. Vesali-Naseh and Y. Mortazavi, Sens. Actuators, B, 2012, 166, 150–155 CrossRef.
- M. Ding, D. C. Sorescu and A. Star, J. Am. Chem. Soc., 2013, 135, 9015–9022 CrossRef PubMed.
- C. Gao, Z. Guo, J.-H. Liu and X.-J. Huang, Nanoscale, 2012, 4, 1948–1963 RSC.
- E. Llobet, Sens. Actuators, B, 2013, 179, 32–45 CrossRef.
- A. Goldoni, L. Petaccia, S. Lizzit and R. Larciprete, J. Phys.: Condens. Matter, 2009, 22, 013001 CrossRef PubMed.
- T. Gao, M. D. Woodka, B. S. Brunschwig and N. S. Lewis, Chem. Mater., 2006, 18, 5193–5202 CrossRef.
- E. T. Thostenson and T. W. Chou, Adv. Mater., 2006, 18, 2837–2841 CrossRef.
- D. J. Lipomi, M. Vosgueritchian, B. C. Tee, S. L. Hellstrom, J. A. Lee, C. H. Fox and Z. Bao, Nat. Nanotechnol., 2011, 6, 788–792 CrossRef PubMed.
- T. Yamada, Y. Hayamizu, Y. Yamamoto, Y. Yomogida, A. Izadi-Najafabadi, D. N. Futaba and K. Hata, Nat. Nanotechnol., 2011, 6, 296–301 CrossRef PubMed.
- C. S. Woo, C. H. Lim, C. W. Cho, B. Park, H. Ju, D. H. Min, C. J. Lee and S. B. Lee, Microelectron. Eng., 2007, 84, 1610–1613 CrossRef.
- B. Li, M. G. Hahm, Y. L. Kim, H. Y. Jung, S. Kar and Y. J. Jung, ACS Nano, 2011, 5, 4826–4834 CrossRef PubMed.
- F. Rigoni, S. Tognolini, P. Borghetti, G. Drera, S. Pagliara, A. Goldoni and L. Sangaletti, Procedia Eng., 2014, 87, 716–719 CrossRef.
- F. Rigoni, G. Drera, S. Pagliara, A. Goldoni and L. Sangaletti, Carbon, 2014, 80, 356–363 CrossRef.
- C. Hua, Y. Shang, Y. Wang, J. Xu, Y. Zhang, X. Li and A. Cao, Appl. Surf. Sci., 2017, 405, 405–411 CrossRef.
- A. Arena, N. Donato, G. Saitta, A. Bonavita, G. Rizzo and G. Neri, Sens. Actuators, B, 2010, 145, 488–494 CrossRef.
- M. Asad, M. H. Sheikhi, M. Pourfath and M. Moradi, Sens. Actuators, B, 2015, 210, 1–8 CrossRef.
- D. Fu, H. Lim, Y.-F. Shi, X. Dong, S. G. Mhaisalkar, Y. Chen, S. Moochhala and L. J. Li, J. Phys. Chem. C, 2008, 112, 650–653 Search PubMed.
- U. Yaqoob, D.-T. Phan, A. S. M. I. Uddin and G.-S. Chung, Sens. Actuators, B, 2015, 221, 760–768 CrossRef.
- U. Yaqoob, A. S. M. I. Uddin and G.-S. Chung, Sens. Actuators, B, 2016, 224, 738–746 CrossRef.
- P. G. Su, C. T. Lee, C. Y. Chou, K. H. Cheng and Y. S. Chuang, Sens. Actuators, B, 2009, 139, 488–493 CrossRef.
- H. Y. Jeong, D. S. Lee, H. K. Choi, D. H. Lee, J. E. Kim, J. Y. Lee, W. J. Lee, S. O. Kim and S. Y. Choi, Appl. Phys. Lett., 2010, 96, 213105 CrossRef.
- S. Ammu, V. Dua, S. R. Agnihotra, S. P. Surwade, A. Phulgirkar, S. Patel and S. K. Manohar, J. Am. Chem. Soc., 2012, 134, 4553–4556 CrossRef PubMed.
- K. Cattanach, R. D. Kulkarni, M. Kozlov and S. K. Manohar, Nanotechnology, 2006, 17, 4123 CrossRef PubMed.
- Y. Wang, Z. Yang, Z. Hou, D. Xu, L. Wei, E. S. W. Kong and Y. Zhang, Sens. Actuators, B, 2010, 150, 708–714 CrossRef.
- K. Parikh, K. Cattanach, R. Rao, D. S. Suh, A. Wu and S. K. Manohar, Sens. Actuators, B, 2006, 113, 55–63 CrossRef.
- C. Tasaltin and F. Basarir, Sens. Actuators, B, 2014, 194, 173–179 CrossRef.
- P. Wan, X. Wen, C. Sun, B. K. Chandran, H. Zhang, X. Sun and X. Chen, Small, 2015, 11, 5409–5415 CrossRef PubMed.
- K. Lee, V. Scardaci, H.-Y. Kim, T. Hallam, H. Nolan, B. E. Bolf, G. S. Maltbie, J. E. Abbott and G. S. Duesberg, Sens. Actuators, B, 2013, 188, 571–575 CrossRef.
- M. Pumera, A. Ambrosi, A. Bonanni, E. L. K. Chng and H. L. Poh, TrAC, Trends Anal. Chem., 2010, 29, 954–965 CrossRef.
- M. Pumera, Chem. – Eur. J., 2009, 15, 4970–4978 CrossRef PubMed.
- W. Yuan and G. Shi, J. Mater. Chem. A, 2013, 1, 10078–10091 Search PubMed.
- D. S. L. Abergel, V. Apalkov, J. Berashevich, K. Ziegler and T. Chakraborty, Adv. Phys., 2010, 59, 261–482 CrossRef.
- A. K. Geim and K. S. Novoselov, Nat. Mater., 2007, 6, 183–191 CrossRef PubMed.
- A. H. C. Neto, F. Guinea, N. M. R. Peres, K. S. Novoselov and A. K. Geim, Rev. Mod. Phys., 2009, 81, 109 CrossRef.
- S. Agarwal, X. Zhou, F. Ye, Q. He, G. C. K. Chen, J. Soo, F. Boey, H. Zhang and P. Chen, Langmuir, 2010, 26, 2244–2247 CrossRef PubMed.
- Y. Wang, J. Liu, L. Liu and D. D. Sun, Nanoscale Res. Lett., 2011, 6, 241 CrossRef PubMed.
- Z. Chen, W. Ren, L. Gao, B. Liu, S. S. Pei and H.-M. Cheng, Nat. Mater., 2011, 10, 424–428 CrossRef PubMed.
- N. G. Shang, P. Papakonstantinou, M. McMullan, M. Chu, A. Stamboulis, A. Potenza, S. S. Dhesi and H. Marchetto, Adv. Funct. Mater., 2008, 18, 3506–3514 CrossRef.
- A. Reina, X. Jia, J. C. Ho, D. Nezich, H. C. Son, V. Bulovic, M. S. Dresselhaus and J. Kong, Nano Lett., 2009, 9, 30–35 CrossRef PubMed.
- X. Li, W. Cai, J. An, S. Kim, J. Nah, D. Yang, R. Piner, A. Velamakanni, I. Jung, E. Tutuc, S. K. Banerjee, L. Colombo and R. S. Ruoff, Science, 2009, 324, 1312–1314 CrossRef PubMed.
- D. V. Kosynkin, A. L. Higginbotham, A. Sinitskii, J. R. Lomeda, A. Dimiev, B. K. Price and J. M. Tour, Nature, 2009, 458, 872 CrossRef PubMed.
- L. Jiao, L. Zhang, X. Wang, G. Diankov and H. Dai, Nature, 2009, 458, 877 CrossRef PubMed.
- P. K. Ang, S. Wang, Q. Bao, J. T. L. Thong and K. P. Loh, ACS Nano, 2009, 3, 3587–3594 CrossRef PubMed.
- J. T. Robinson, F. K. Perkins, E. S. Snow, Z. Wei and P. E. Sheehan, Nano Lett., 2008, 8, 3137–3140 CrossRef PubMed.
- Q. Yu, J. Lian, S. Siriponglert, H. Li, Y. P. Chen and S. S. Pei, Appl. Phys. Lett., 2008, 93, 113103 CrossRef.
- W. S. Hummers and R. E. Offeman, J. Am. Chem. Soc., 1958, 80, 1339 CrossRef.
- D. C. Marcano, D. V. Kosynkin, J. M. Berlin, A. Sinitskii, Z. Sun, A. Slesarev, L. B. Alemany, W. Lu and J. M. Tour, ACS Nano, 2010, 4, 4806–4814 CrossRef PubMed.
- D. Li, M. B. Muller, S. Gilje, R. B. Kaner and G. G. Wallace, Nat. Nanotechnol., 2008, 3, 101–105 CrossRef PubMed.
- C. D. Zangmeister, Chem. Mater., 2010, 22, 5625–5629 CrossRef.
- S. Stankovich, D. A. Dikin, R. D. Piner, K. A. Kohlhaas, A. Kleinhammes, Y. Jia, Y. Wu, S. B. T. Nguyen and R. S. Ruoff, Carbon, 2007, 45, 1558–1565 CrossRef.
- Y. Shao, J. Wang, M. Engelhard, C. Y. Wang and Y. Lin, J. Mater. Chem., 2010, 20, 743–748 RSC.
- L. J. Cote, R. Cruz-Silva and J. Huang, J. Am. Chem. Soc., 2009, 131, 11027–11032 CrossRef PubMed.
- F. Schedin, A. K. Geim, S. V. Morozov, E. Hill, P. Blake, M. I. Katsnelson and K. S. Novoselov, Nat. Mater., 2007, 6, 652–655 CrossRef PubMed.
- R. Pearce, T. Iakimov, M. Andersson, L. Hultman, A. L. Spetz and R. Yakimova, Sens. Actuators, B, 2011, 155, 451–455 CrossRef.
- K. Yu, P. Wang, G. Lu, K.-H. Chen, Z. Bo and J. Chen, J. Phys. Chem. Lett., 2011, 2, 537–542 CrossRef.
- G. Lu, L. E. Ocola and J. Chen, Nanotechnology, 2009, 20, 445502 CrossRef PubMed.
- Z. Ben Aziza, Q. Zhang and D. Baillargeat, Appl. Phys. Lett., 2014, 105, 254102 CrossRef.
- A. Inaba, K. Yoo, Y. Takei, K. Matsumoto and I. Shimoyama, Sens. Actuators, B, 2014, 195, 15–21 CrossRef.
- M. Gautam and A. H. Jayatissa, J. Appl. Phys., 2012, 112, 064304 CrossRef.
- J. Zhang, P. Hu, R. Zhang, X. Wang, B. Yang, W. Cao, Y. Li, X. He, Z. Wang and W. O'Neill, J. Mater. Chem., 2012, 22, 714–718 RSC.
- G. Lu, K. Yu, L. E. Ocola and J. Chen, Chem. Commun., 2011, 47, 7761–7763 RSC.
- S. M. Mortazavi Zanjani, M. M. Sadeghi, M. Holt, S. k. F. Chowdhury, L. Tao and D. Akinwande, Appl. Phys. Lett., 2016, 108, 033106 CrossRef.
- B. Chen, H. Liu, X. Li, C. Lu, Y. Ding and B. Lu, Appl. Surf. Sci., 2012, 258, 1971–1975 CrossRef.
- S. Mao, S. Cui, G. Lu, K. Yu, Z. Wen and J. Chen, J. Mater. Chem., 2012, 22, 11009–11013 RSC.
- T. V. Cuong, V. H. Pham, J. S. Chung, E. W. Shin, D. H. Yoo, S. H. Hahn, J. S. Huh, G. H. Rue, E. J. Kim and S. H. Hur, Mater. Lett., 2010, 64, 2479–2482 CrossRef.
- W. Li, X. Geng, Y. Guo, J. Rong, Y. Gong, L. Wu, X. Zhang, P. Li, J. Xu and G. Cheng, ACS Nano, 2011, 5, 6955–6961 CrossRef PubMed.
- Y. Lu, B. R. Goldsmith, N. J. Kybert and A. T. C. Johnson, Appl. Phys. Lett., 2010, 97, 083107 CrossRef.
- S. Cui, H. Pu, E. C. Mattson, Z. Wen, J. Chang, Y. Hou, C. J. Hirschmugl and J. Chen, Anal. Chem., 2014, 86, 7516–7522 CrossRef PubMed.
- G. Yang, C. Lee, J. E. Kim, F. Ren and S. J. Pearton, Phys. Chem. Chem. Phys., 2013, 15, 1798–1801 RSC.
-
T. Hayasaka, Y. Kubota, Y. Liu and L. Lin, 19th International Conference on Solid-State Sensors, Actuators and Microsystems (TRANSDUCERS), Kaohsiung, 2017, pp. 2103–2106 Search PubMed.
- S. Tiwari, A. K. Singh, S. K. Balasubramanian, W. Takashima and R. Prakash, J. Nanosci. Nanotechnol., 2016, 16, 9634–9641 CrossRef.
- D. Zhang, J. Liu, C. Jiang, A. Liu and B. Xia, Sens. Actuators, B, 2017, 240, 55–65 CrossRef.
- J. D. Fowler, M. J. Allen, V. C. Tung, Y. Yang, R. B. Kaner and B. H. Weiller, ACS Nano, 2009, 3, 301–306 CrossRef PubMed.
- T. H. Han, Y.-K. Huang, A. T. L. Tan, V. P. Dravid and J. Huang, J. Am. Chem. Soc., 2011, 133, 15264–15267 CrossRef PubMed.
- S. J. Hwang, J. Lim, H. G. Park, W. K. Kim, D.-H. Kim, I. S. Song, J. H. Kim, S. Lee, D. H. Woo and S. C. Jun, Curr. Appl. Phys., 2012, 12, 1017–1022 CrossRef.
- R. K. Paul, S. Badhulika, N. M. Saucedo and A. Mulchandani, Anal. Chem., 2012, 84, 8171–8178 CrossRef PubMed.
- M. W. K. Nomani, R. Shishir, M. Qazi, D. Diwan, V. B. Shields, M. G. Spencer, G. S. Tompa, N. M. Sbrockey and G. Koley, Sens. Actuators, B, 2010, 150, 301–307 CrossRef.
- M. G. Chung, D. H. Kim, H. M. Lee, T. Kim, J. H. Choi, D. k. Seo, J.-B. Yoo, S.-H. Hong, T. J. Kang and Y. H. Kim, Sens. Actuators, B, 2012, 166, 172–176 CrossRef.
- G. Chen, T. M. Paronyan and A. R. Harutyunyan, Appl. Phys. Lett., 2012, 101, 053119 CrossRef.
- F. Yavari, Z. Chen, A. V. Thomas, W. Ren, H.-M. Cheng and N. Koratkar, Sci. Rep., 2011, 1, 166 CrossRef PubMed.
- G. Ko, H.-Y. Kim, J. Ahn, Y.-M. Park, K.-Y. Lee and J. Kim, Curr. Appl. Phys., 2010, 10, 1002–1004 CrossRef.
- A. Salehi-Khojin, D. Estrada, K. Y. Lin, M. H. Bae, F. Xiong, E. Pop and R. I. Masel, Adv. Mater., 2012, 24, 53–57 CrossRef PubMed.
- S. Srivastava, K. Jain, V. N. Singh, S. Singh, N. Vijayan, N. Dilawar, G. Gupta and T. D. Senguttuvan, Nanotechnology, 2012, 23, 205501 CrossRef PubMed.
- L. T. Hoa, H. N. Tien, V. H. Luan, J. S. Chung and S. H. Hur, Sens. Actuators, B, 2013, 185, 701–705 CrossRef.
- S. Liu, B. Yu, H. Zhang, T. Fei and T. Zhang, Sens. Actuators, B, 2014, 202, 272–278 CrossRef.
- X. Liu, J. Cui, J. Sun and X. Zhang, RSC Adv., 2014, 4, 22601–22605 RSC.
- F. Gu, R. Nie, D. Han and Z. Wang, Sens. Actuators, B, 2015, 219, 94–99 CrossRef.
- H. Zhang, J. Feng, T. Fei, S. Liu and T. Zhang, Sens. Actuators, B, 2014, 190, 472–478 CrossRef.
- P.-G. Su and S.-L. Peng, Talanta, 2015, 132, 398–405 CrossRef PubMed.
- Y.-l. Dong, X.-f. Zhang, X.-l. Cheng, Y.-m. Xu, S. Gao, H. Zhao and L.-h. Huo, RSC Adv., 2014, 4, 57493–57500 RSC.
- S. Cui, Z. Wen, E. C. Mattson, S. Mao, J. Chang, M. Weinert, C. J. Hirschmugl, M. Gajdardziska-Josifovska and J. Chen, J. Mater. Chem. A, 2013, 1, 4462–4467 Search PubMed.
- H. Meng, W. Yang, K. Ding, L. Feng and Y. Guan, J. Mater. Chem. A, 2015, 3, 1174–1181 Search PubMed.
- S.-J. Choi, B.-H. Jang, S.-J. Lee, B. K. Min, A. Rothschild and I.-D. Kim, ACS Appl. Mater. Interfaces, 2014, 6, 2588–2597 Search PubMed.
- S.-J. Choi, W.-H. Ryu, S.-J. Kim, H.-J. Cho and I.-D. Kim, J. Mater. Chem. B, 2014, 2, 7160–7167 RSC.
- Z. Zhang, R. Zou, G. Song, L. Yu, Z. Chen and J. Hu, J. Mater. Chem., 2011, 21, 17360–17365 RSC.
- A. S. M. I. Uddin, K.-W. Lee and G.-S. Chung, Sens. Actuators, B, 2015, 216, 33–40 CrossRef.
- A. S. M. I. Uddin, D.-T. Phan and G.-S. Chung, Sens. Actuators, B, 2015, 207, 362–369 CrossRef.
- S. Cui, S. Mao, Z. Wen, J. Chang, Y. Zhang and J. Chen, Analyst, 2013, 138, 2877–2882 RSC.
- M. Gautam and A. H. Jayatissa, Solid-State Electron., 2012, 78, 159–165 CrossRef.
- Q. T. Tran, H. T. M. Hoa, D.-H. Yoo, T. V. Cuong, S. H. Hur, J. S. Chung, E. J. Kim and P. A. Kohl, Sens. Actuators, B, 2014, 194, 45–50 CrossRef.
- C. Piloto, M. Notarianni, M. Shafiei, E. Taran, D. Galpaya, C. Yan and N. Motta, Beilstein J. Nanotechnol., 2014, 5, 1073 CrossRef PubMed.
-
T. T. Tung, M. Castro and J.-F. Feller, 12th IEEE International Conference on Nanotechnology (IEEE-NANO), Birmingham, 2012, pp. 1–5 Search PubMed.
- M. Gautam and A. H. Jayatissa, J. Appl. Phys., 2012, 112, 114326 CrossRef.
- L. Zhang, C. Li, A. Liu and G. Shi, J. Mater. Chem., 2012, 22, 8438–8443 RSC.
- X. Huang, N. Hu, L. Zhang, L. Wei, H. Wei and Y. Zhang, Synth. Met., 2013, 185, 25–30 CrossRef.
- X. Huang, N. Hu, R. Gao, Y. Yu, Y. Wang, Z. Yang, E. S.-W. Kong, H. Wei and Y. Zhang, J. Mater. Chem., 2012, 22, 22488–22495 RSC.
- Z. Wu, X. Chen, S. Zhu, Z. Zhou, Y. Yao, W. Quan and B. Liu, Sens. Actuators, B, 2013, 178, 485–493 CrossRef.
- Z. Ye, Y. Jiang, H. Tai and Z. Yuan, Integr. Ferroelectr., 2014, 154, 73–81 CrossRef.
- X. L. Huang, N. T. Hu, Y. Y. Wang and Y. F. Zhang, Adv. Mat. Res., 2013, 669, 79–84 Search PubMed.
- N. Hu, Z. Yang, Y. Wang, L. Zhang, Y. Wang, X. Huang, H. Wei, L. Wei and Y. Zhang, Nanotechnology, 2014, 25, 025502 CrossRef PubMed.
- Y. Wang, L. Zhang, N. Hu, Y. Wang, Y. Zhang, Z. Zhou, Y. Liu, S. Shen and C. Peng, Nanoscale Res. Lett., 2014, 9, 251 CrossRef PubMed.
- W. Yuan, A. Liu, L. Huang, C. Li and G. Shi, Adv. Mater., 2013, 25, 766–771 CrossRef PubMed.
- Y. Seekaew, D. Phokharatkul, A. Wisitsoraat and C. Wongchoosuk, Appl. Surf. Sci., 2017, 404, 357–363 CrossRef.
- Z. Song, Z. Wei, B. Wang, Z. Luo, S. Xu, W. Zhang, H. Yu, M. Li, Z. Huang and J. Zang, Chem. Mater., 2016, 28, 1205–1212 CrossRef.
- H. K. Choi, H. Y. Jeong, D.-S. Lee, S.-Y. Choi and C.-G. Choi, Carbon Lett., 2013, 14, 186–189 CrossRef.
- M. W. Jung, S. Myung, W. Song, M.-A. Kang, S. H. Kim, C.-S. Yang, S. S. Lee, J. Lim, C.-Y. Park and J.-O. Lee, ACS Appl. Mater. Interfaces, 2014, 6, 13319–13323 Search PubMed.
- H. Choi, J. S. Choi, J. S. Kim, J. H. Choe, K. H. Chung, J. W. Shin, J. T. Kim, D. H. Youn, K. C. Kim and J. I. Lee, Small, 2014, 10, 3685–3691 CrossRef PubMed.
- V. Dua, S. P. Surwade, S. Ammu, S. R. Agnihotra, S. Jain, K. E. Roberts, S. H. Park, R. S. Ruoff and S. K. Manohar, Angew. Chem., Int. Ed., 2010, 49, 2154–2157 CrossRef PubMed.
- C. Lee, J. H. Ahn, K. B. Lee, D. S. Kim and J. E. Kim, Thin Solid Films, 2012, 520, 5459–5462 CrossRef.
- B. Cho, J. Yoon, M. G. Hahm, D.-H. Kim, A. R. Kim, Y. H. Kahng, S.-W. Park, Y.-J. Lee, S.-G. Park and J.-D. Kwon, J. Mater. Chem. C, 2014, 2, 5280–5285 RSC.
- L. Huang, Z. L. Wang, J. Zhang, J. Pu, Y. Lin, S. Xu, L. Shen, Q. Chen and W. Shi, ACS Appl. Mater. Interfaces, 2014, 6, 7426–7433 Search PubMed.
- Y. Seekaew, S. Lokavee, D. Phokharatkul, A. Wisitsoraat, T. Kerdcharoen and C. Wongchoosuk, Org. Electron., 2014, 15, 2971–2981 CrossRef.
- M.-S. Kim, S. Kim, H. J. Kong, O. S. Kwon and H. Yoon, J. Phys. Chem. C, 2016, 120, 18289–18295 Search PubMed.
- X. Peng, J. Chu, A. Aldalbahi, M. Rivera, L. Wang, S. Duan and P. Feng, Appl. Surf. Sci., 2016, 387, 149–154 CrossRef.
- Y. Cui, Z. Zhong, D. Wang, W. U. Wang and C. M. Lieber, Nano Lett., 2003, 3, 149–152 CrossRef.
- D. Braga and G. Horowitz, Adv. Mater., 2009, 21, 1473–1486 CrossRef.
- Y. Cui, X. Duan, J. Hu and C. M. Lieber, J. Phys. Chem. B, 2000, 104, 5213–5216 CrossRef.
- R. M. Penner, Annu. Rev. Anal. Chem., 2012, 5, 461–485 CrossRef PubMed.
- M. Y. Bashouti, K. Sardashti, S. W. Schmitt, M. Pietsch, J. Ristein, H. Haick and S. H. Christiansen, Prog. Surf. Sci., 2013, 88, 39–60 CrossRef.
- M. Mescher, L. C. P. M. De Smet, E. J. R. Sudhölter and J. H. Klootwijk, J. Nanosci. Nanotechnol., 2013, 13, 5649–5653 CrossRef PubMed.
- E. Stern, J. F. Klemic, D. A. Routenberg, P. N. Wyrembak, D. B. Turner-Evans, A. D. Hamilton, D. A. LaVan, T. M. Fahmy and M. A. Reed, Nature, 2007, 445, 519–522 CrossRef PubMed.
- X. Liu, P. R. Coxon, M. Peters, B. Hoex, J. M. Cole and D. J. Fray, Energy Environ. Sci., 2014, 7, 3223–3263 Search PubMed.
- R. Dussart, T. Tillocher, P. Lefaucheux and M. Boufnichel, J. Phys. D: Appl. Phys., 2014, 47, 123001 CrossRef.
- R. Ghosh and P. K. Giri, Nanotechnology, 2016, 28, 012001 CrossRef PubMed.
- A. Cao, E. Sudhölter and L. de Smet, Sensors, 2014, 14, 245 CrossRef PubMed.
-
T. Yoshizumi and Y. Miyahara, in Different Types of Field-Effect Transistors – Theory and Applications, ed. M. M. Pejovic and M. M. Pejovic, InTech, Rijeka, 2017, ch. 08 Search PubMed.
- X. Chen, C. K. Y. Wong, C. A. Yuan and G. Zhang, Sens. Actuators, B, 2013, 177, 178–195 CrossRef.
- S. Clavaguera, A. Carella, L. Caillier, C. Celle, J. Pécaut, S. Lenfant, D. Vuillaume and J.-P. Simonato, Angew. Chem., Int. Ed., 2010, 49, 4063–4066 CrossRef PubMed.
- V. Passi, F. Ravaux, E. Dubois, S. Clavaguera, A. Carella, C. Celle, J.-P. Simonato, L. Silvestri, S. Reggiani and D. Vuillaume, IEEE Electron Device Lett., 2011, 32, 976–978 CrossRef.
- S. Clavaguera, N. Raoul, A. Carella, M. Delalande, C. Celle and J.-P. Simonato, Talanta, 2011, 85, 2542–2545 CrossRef PubMed.
- J. W. Han, T. Rim, C.-K. Baek and M. Meyyappan, ACS Appl. Mater. Interfaces, 2015, 7, 21263–21269 Search PubMed.
- A. O. Niskanen, A. Colli, R. White, H. W. Li, E. Spigone and J. M. Kivioja, Nanotechnology, 2011, 22, 295502 CrossRef PubMed.
- Y. Paska, T. Stelzner, S. Christiansen and H. Haick, ACS Nano, 2011, 5, 5620–5626 CrossRef PubMed.
- Y. Paska, T. Stelzner, O. Assad, U. Tisch, S. Christiansen and H. Haick, ACS Nano, 2011, 6, 335–345 CrossRef PubMed.
- Y. Paska and H. Haick, ACS Appl. Mater. Interfaces, 2012, 4, 2604–2617 Search PubMed.
- B. Wang and H. Haick, ACS Appl. Mater. Interfaces, 2013, 5, 5748–5756 Search PubMed.
- B. Wang and H. Haick, ACS Appl. Mater. Interfaces, 2013, 5, 2289–2299 Search PubMed.
- B. Wang, J. C. Cancilla, J. S. Torrecilla and H. Haick, Nano Lett., 2014, 14, 933–938 CrossRef PubMed.
- R. Ermanok, O. Assad, K. Zigelboim, B. Wang and H. Haick, ACS Appl. Mater. Interfaces, 2013, 5, 11172–11183 Search PubMed.
- N. Shehada, G. Bronstrup, K. Funka, S. Christiansen, M. Leja and H. Haick, Nano Lett., 2015, 15, 1288–1295 CrossRef PubMed.
- N. Shehada, J. C. Cancilla, J. S. Torrecilla, E. S. Pariente, G. Bronstrup, S. Christiansen, D. W. Johnson, M. Leja, M. P. Davies, O. Liran, N. Peled and H. Haick, ACS Nano, 2016, 10, 7047–7057 CrossRef PubMed.
- H. T. Hsueh, T. J. Hsueh, S. J. Chang, F. Y. Hung, W. Y. Weng, C. L. Hsu and B. T. Dai, IEEE Sens. J., 2011, 11, 3036–3041 CrossRef.
- L. Ni, E. Jacques, R. Rogel, A.-C. Salaün, L. Pichon and G. Wenga, Procedia Eng., 2012, 47, 240–243 CrossRef.
- L. Yang, H. Lin, Z. Zhang, L. Cheng, S. Ye and M. Shao, Sens. Actuators, B, 2013, 177, 260–264 CrossRef.
- J. Wan, S.-R. Deng, R. Yang, Z. Shu, B.-R. Lu, S.-Q. Xie, Y. Chen, E. Huq, R. Liu and X.-P. Qu, Microelectron. Eng., 2009, 86, 1238–1242 CrossRef.
- C. Gao, S.-R. Deng, J. Wan, B.-R. Lu, R. Liu, E. Huq, X.-P. Qu and Y. Chen, Microelectron. Eng., 2010, 87, 927–930 CrossRef.
- M. C. McAlpine, H. D. Agnew, R. D. Rohde, M. Blanco, H. Ahmad, A. D. Stuparu, W. A. Goddard Iii and J. R. Heath, J. Am. Chem. Soc., 2008, 130, 9583–9589 CrossRef PubMed.
- C. Gao, Z.-C. Xu, S.-R. Deng, J. Wan, Y. Chen, R. Liu, E. Huq and X.-P. Qu, Microelectron. Eng., 2011, 88, 2100–2104 CrossRef.
- M. Cuscunà, A. Convertino, E. Zampetti, A. Macagnano, A. Pecora, G. Fortunato, L. Felisari, G. Nicotra, C. Spinella and F. Martelli, Appl. Phys. Lett., 2012, 101, 103101 CrossRef.
- H. J. In, C. R. Field and P. E. Pehrsson, Nanotechnology, 2011, 22, 355501 CrossRef PubMed.
- J. Liao, Z. Li, G. Wang, C. Chen, S. Lv and M. Li, Phys. Chem. Chem. Phys., 2016, 18, 4835–4841 RSC.
- W. Zhang, M. Hu, X. Liu, Y. Wei, N. Li and Y. Qin, J. Alloys Compd., 2016, 679, 391–399 CrossRef.
- S. Ma, M. Hu, P. Zeng, M. Li, W. Yan and Y. Qin, Sens. Actuators, B, 2014, 192, 341–349 CrossRef.
- K.-Q. Peng, X. Wang and S.-T. Lee, Appl. Phys. Lett., 2009, 95, 243112 CrossRef.
- M. C. McAlpine, H. Ahmad, D. Wang and J. R. Heath, Nat. Mater., 2007, 6, 379 CrossRef PubMed.
- W. P. Jakubik, Thin Solid Films, 2011, 520, 986–993 CrossRef.
- M. C. Horrillo, M. J. Fernández, J. L. Fontecha, I. Sayago, M. Garcıa, M. Aleixandre, J. P. Santos, L. Arés, J. Gutiérrez and I. Gracia, Thin Solid Films, 2004, 467, 234–238 CrossRef.
- Y.-L. Tang, Z.-J. Li, J.-Y. Ma, H.-Q. Su, Y.-J. Guo, L. Wang, B. Du, J.-J. Chen, W. Zhou and Q.-K. Yu, J. Hazard. Mater., 2014, 280, 127–133 CrossRef PubMed.
- Y.-L. Tang, Z.-J. Li, J.-Y. Ma, Y.-J. Guo, Y.-Q. Fu and X.-T. Zu, Sens. Actuators, B, 2014, 201, 114–121 CrossRef.
- Y. Tang, D. Ao, W. Li, X. Zu, S. Li and Y. Q. Fu, Sens. Actuators, B, 2018, 254, 1165–1173 CrossRef.
- L. Rana, R. Gupta, R. Kshetrimayum, M. Tomar and V. Gupta, Surf. Coat. Technol., 2018, 343, 89–92 CrossRef.
- L. Rana, R. Gupta, M. Tomar and V. Gupta, Sens. Actuators, B, 2017, 252, 840–845 CrossRef.
- S. J. Ippolito, A. Ponzoni, K. Kalantar-Zadeh, W. Wlodarski, E. Comini, G. Faglia and G. Sberveglieri, Sens. Actuators, B, 2006, 117, 442–450 CrossRef.
- D. Matatagui, O. Kolokoltsev, J. M. Saniger, I. Gràcia, M. J. Fernández, J. L. Fontecha and M. d. C. Horrillo, Sensors, 2017, 17, 2624 CrossRef PubMed.
- I. Sayago, D. Matatagui, M. J. Fernández, J. L. Fontecha, I. Jurewicz, R. Garriga and E. Muñoz, Talanta, 2016, 148, 393–400 CrossRef PubMed.
- S. Thomas, M. Cole, A. De Luca, F. Torrisi, A. C. Ferrari, F. Udrea and J. W. Gardner, Procedia Eng., 2014, 87, 999–1002 CrossRef.
- R. Arsat, M. Breedon, M. Shafiei, P. G. Spizziri, S. Gilje, R. B. Kaner, K. Kalantar-zadeh and W. Wlodarski, Chem. Phys. Lett., 2009, 467, 344–347 CrossRef.
- D. Amati, D. Arn, N. Blom, M. Ehrat, J. Saunois and H. M. Widmer, Sens. Actuators, B, 1992, 7, 587–591 CrossRef.
- U. Stahl, A. Voigt, M. Dirschka, N. Barié, C. Richter, A. Waldbaur, F. J. Gruhl, B. E. Rapp, M. Rapp and K. Länge, Sensors, 2017, 17, 2529 CrossRef PubMed.
- Y. Liu, H. Huang, L. Wang, D. Cai, B. Liu, D. Wang, Q. Li and T. Wang, Sens. Actuators, B, 2016, 223, 730–737 CrossRef.
- L. Al-Mashat, H. D. Tran, W. Wlodarski, R. B. Kaner and K. Kalantar-Zadeh, Sens. Actuators, B, 2008, 134, 826–831 CrossRef.
- A. Z. Sadek, W. Wlodarski, K. Shin, R. B. Kaner and K. Kalantar-Zadeh, Nanotechnology, 2006, 17, 4488 CrossRef.
- M. David, M. Arab, C. Martino, L. Delmas, F. Guinneton and J.-R. Gavarri, Thin Solid Films, 2012, 520, 4786–4791 CrossRef.
- M. Penza, F. Antolini and M. V. Antisari, Sens. Actuators, B, 2004, 100, 47–59 CrossRef.
- M. Penza, F. Antolini and M. Vittori-Antisari, Thin Solid Films, 2005, 472, 246–252 CrossRef.
- I. Sayago, M. J. Fernández, J. L. Fontecha, M. C. Horrillo, C. Vera, I. Obieta and I. Bustero, Sens. Actuators, B, 2012, 175, 67–72 CrossRef.
-
M. N. Hamidon and Z. Yunusa, Progresses in Chemical Sensor, ed. W. Wang, InTech, Rijeka, 2016, ch. 8 Search PubMed.
- Y. J. Guo, J. Zhang, C. Zhao, P. A. Hu, X.-T. Zu and Y. Q. Fu, Optik, 2014, 125, 5800–5802 CrossRef.
- S. K. Vashist and P. Vashist, Sensors, 2011, 1–13 Search PubMed.
- F. L. Dickert, O. Hayden and M. E. Zenkel, Anal. Chem., 1999, 71, 1338–1341 CrossRef PubMed.
- X. Wang, J. Zhang and Z. Zhu, Appl. Surf. Sci., 2006, 252, 2404–2411 CrossRef.
- N. Van Quy, V. A. Minh, N. Van Luan, V. N. Hung and N. Van Hieu, Sens. Actuators, B, 2011, 153, 188–193 CrossRef.
- J. Zhang, J. Q. Hu, F. R. Zhu, H. Gong and S. J. O'shea, Sens. Actuators, B, 2002, 87, 159–167 CrossRef.
- J. Hu, F. Zhu, J. Zhang and H. Gong, Sens. Actuators, B, 2003, 93, 175–180 CrossRef.
- J. Zhang, J. Hu, Z. Q. Zhu, H. Gong and S. J. O’Shea, Colloids Surf., A, 2004, 236, 23–30 CrossRef.
- B. Ding, J. Kim, Y. Miyazaki and S. Shiratori, Sens. Actuators, B, 2004, 101, 373–380 CrossRef.
- A. l. Palaniappan, S. Moochhala, F. E. H. Tay, X. Su and N. C. L. Phua, Sens. Actuators, B, 2008, 129, 184–187 CrossRef.
- M. Matsuguchi, Y. Kadowaki and M. Tanaka, Sens. Actuators, B, 2005, 108, 572–575 CrossRef.
- Z. Ying, Y. Jiang, X. Du, G. Xie, J. Yu and H. Wang, Sens. Actuators, B, 2007, 125, 167–172 CrossRef.
- Z. Ying, Y. Jiang, X. Du, G. Xie, J. Yu and H. Tai, Eur. Polym. J., 2008, 44, 1157–1164 CrossRef.
- C. Zhang, X. Wang, J. Lin, B. Ding, J. Yu and N. Pan, Sens. Actuators, B, 2011, 152, 316–323 CrossRef.
- X. Wang, B. Ding, M. Sun, J. Yu and G. Sun, Sens. Actuators, B, 2010, 144, 11–17 CrossRef.
- M. M. Ayad, G. El-Hefnawey and N. L. Torad, J. Hazard. Mater., 2009, 168, 85–88 CrossRef PubMed.
- P. Si, J. Mortensen, A. Komolov, J. Denborg and P. J. Møller, Anal. Chim. Acta, 2007, 597, 223–230 CrossRef PubMed.
- I. A. Koshets, Z. I. Kazantseva, Y. M. Shirshov, S. A. Cherenok and V. I. Kalchenko, Sens. Actuators, B, 2005, 106, 177–181 CrossRef.
- B. Wyszynski, P. Somboon and T. Nakamoto, Sens. Actuators, B, 2008, 130, 857–863 CrossRef.
- A. K. M. S. Islam, Z. Ismail, M. N. Ahmad, B. Saad, A. R. Othman, A. Y. M. Shakaff, A. Daud and Z. Ishak, Sens. Actuators, B, 2005, 109, 238–243 CrossRef.
- A. Özmen, F. Tekce, M. A. Ebeoğlu, C. Taşaltın and Z. Z. Öztürk, Sens. Actuators, B, 2006, 115, 450–454 CrossRef.
- M. Matsuguchi and T. Uno, Sens. Actuators, B, 2006, 113, 94–99 CrossRef.
- Y. Zhu, H. Yuan, J. Xu, P. Xu and Q. Pan, Sens. Actuators, B, 2010, 144, 164–169 CrossRef.
- X. Wang, B. Ding, J. Yu, M. Wang and F. Pan, Nanotechnology, 2009, 21, 055502 CrossRef PubMed.
- S.-W. Lee, N. Takahara, S. Korposh, D.-H. Yang, K. Toko and T. Kunitake, Anal. Chem., 2010, 82, 2228–2236 CrossRef PubMed.
-
J. L. Huang, G. Xie, Y. Zhou, T. Xie, H. Tai and G. J. Yang, 7th International Symposium on Advanced Optical Manufacturing and Testing Technologies: Smart Structures and Materials for Manufacturing and Testing, 2014, vol. 9285, pp. 92850B Search PubMed.
- Y. Yao, X. Chen, H. Guo and Z. Wu, Appl.
Surf. Sci., 2011, 257, 7778–7782 CrossRef.
- W. Tao, P. Lin, S. Liu, Q. Xie, S. Ke and X. Zeng, Sensors, 2017, 17, 194 CrossRef PubMed.
- F. Pascal-Delannoy, B. Sorli and A. Boyer, Sens. Actuators, A, 2000, 84, 285–291 CrossRef.
- V. S. A. Piriya, P. Joseph, S. C. G. K. Daniel, S. Lakshmanan, T. Kinoshita and M. Sivakumar, Mater. Sci. Eng., C, 2017, 78, 1231–1245 CrossRef PubMed.
- J. R. Askim, M. Mahmoudi and K. S. Suslick, Chem. Soc. Rev., 2013, 42, 8649–8682 RSC.
- M. J. Kangas, R. M. Burks, J. Atwater, R. M. Lukowicz, P. Williams and A. E. Holmes, Crit. Rev. Anal. Chem., 2017, 47, 138–153 CrossRef PubMed.
- K. S. Suslick, N. A. Rakow and A. Sen, Tetrahedron, 2004, 60, 11133–11138 CrossRef.
- M. C. Janzen, J. B. Ponder, D. P. Bailey, C. K. Ingison and K. S. Suslick, Anal. Chem., 2006, 78, 3591–3600 CrossRef PubMed.
- C. Zhang and K. S. Suslick, J. Am. Chem. Soc., 2005, 127, 11548–11549 CrossRef PubMed.
- N. A. Rakow and K. S. Suslick, Nature, 2000, 406, 710–713 CrossRef PubMed.
- K. S. Suslick, MRS Bull., 2004, 29, 720–725 CrossRef.
- L. Feng, C. J. Musto, J. W. Kemling, S. H. Lim and K. S. Suslick, Chem. Commun., 2010, 46, 2037–2039 RSC.
- S. H. Lim, L. Feng, J. W. Kemling, C. J. Musto and K. S. Suslick, Nat. Chem., 2009, 1, 562–567 CrossRef PubMed.
- A. Sen, J. D. Albarella, J. R. Carey, P. Kim and W. B. McNamara, Sens. Actuators, B, 2008, 134, 234–237 CrossRef.
- J. Courbat, D. Briand, J. Wöllenstein and N. F. D. Rooij, Procedia Chem., 2009, 1, 576–579 CrossRef.
- T. Endo, Y. Yanagida and T. Hatsuzawa, Sens. Actuators, B, 2007, 125, 589–595 CrossRef.
- X. Wang, X. Sun, P. A. Hu, J. Zhang, L. Wang, W. Feng, S. Lei, B. Yang and W. Cao, Adv. Funct. Mater., 2013, 23, 6044–6050 CrossRef.
- N. A. Luechinger, S. Loher, E. K. Athanassiou, R. N. Grass and W. J. Stark, Langmuir, 2007, 23, 3473–3477 CrossRef PubMed.
- C. Elosua, I. R. Matias, C. Bariain and F. J. Arregui, Sensors, 2006, 6, 1440–1465 CrossRef.
- M. Hernaez, C. Zamarreño, S. Melendi-Espina, L. Bird, A. Mayes and F. Arregui, Sensors, 2017, 17, 155 CrossRef PubMed.
- H. Okuda, T. Wang and S. W. Lee, Electron. Comm. JPN, 2017, 100, 43–49 Search PubMed.
- L. I. B. Silva, A. C. Freitas, T. A. P. Rocha-Santos, M. E. Pereira and A. C. Duarte, Talanta, 2011, 83, 1586–1594 CrossRef PubMed.
- L. I. B. Silva, T. A. P. Rocha-Santos and A. C. Duarte, Talanta, 2009, 78, 548–552 CrossRef PubMed.
- C. Bariáin, I. R. Matías, I. Romeo, J. Garrido and M. Laguna, Appl. Phys. Lett., 2000, 77, 2274–2276 CrossRef.
- C. Elosúa, C. Bariáin, I. R. Matías, F. J. Arregui, A. Luquin and M. Laguna, Sens. Actuators, B, 2006, 115, 444–449 CrossRef.
- C. Bariain, I. R. Matıas, C. Fernandez-Valdivielso, F. J. Arregui, M. L. Rodrıguez-Méndez and J. A. De Saja, Sens. Actuators, B, 2003, 93, 153–158 CrossRef.
- S. M. Topliss, S. W. James, F. Davis, S. P. J. Higson and R. P. Tatam, Sens. Actuators, B, 2010, 143, 629–634 CrossRef.
- S. Bagchi, R. Achla and S. K. Mondal, Sens. Actuators, B, 2017, 250, 52–60 CrossRef.
- T. Kavinkumar, D. Sastikumar and S. Manivannan, Proc. SPIE, 2014, 9270 DOI:10.1117/12.2071841.
- C. Yu, Y. Wu, X. Liu, F. Fu, Y. Gong, Y.-J. Rao and Y. Chen, Sens. Actuators, B, 2017, 244, 107–113 CrossRef.
- T. Kavinkumar and S. Manivannan, Ceram. Int., 2016, 42, 1769–1776 CrossRef.
- R. Gao, D.-F. Lu, J. Cheng, Y. Jiang, L. Jiang and Z.-M. Qi, Sens. Actuators, B, 2016, 222, 618–624 CrossRef.
- M. Manjula, B. Karthikeyan and D. Sastikumar, Opt. Lasers Eng., 2017, 95, 78–82 CrossRef.
-
W. S. Wong and A. Salleo, Flexible electronics: materials and applications, Springer Science & Business Media, 2009 Search PubMed.
- C. Bariain, I. R. Matıas, I. Romeo, J. Garrido and M. Laguna, Appl. Phys. Lett., 2000, 77, 2274–2276 CrossRef.
- X. Chen, F. Xu, Y. Wang, Y. Pan, D. Lu, P. Wang, K. Ying, E. Chen and W. Zhang, Cancer, 2007, 110, 835–844 CrossRef PubMed.
- W. Filipiak, A. Sponring, A. Filipiak, C. Ager, J. Schubert, W. Miekisch, A. Amann and J. Troppmair, Cancer Epidemiol., Biomarkers Prev., 2010, 19, 182–195 CrossRef PubMed.
- W. Filipiak, A. Sponring, T. Mikoviny, C. Ager, J. Schubert, W. Miekisch, A. Amann and J. Troppmair, Cancer Cell Int., 2008, 8, 17 CrossRef PubMed.
- D. Smith, T. Wang, J. Sulé-Suso, P. Španěl and A. E. Haj, Rapid Commun. Mass Spectrom., 2003, 17, 845–850 CrossRef PubMed.
- A. Sponring, W. Filipiak, C. Ager, J. Schubert, W. Miekisch, A. Amann and J. Troppmair, Cancer Biomarkers, 2010, 7, 153–161 CrossRef PubMed.
- A. Sponring, W. Filipiak, T. Mikoviny, C. Ager, J. Schubert, W. Miekisch, A. Amann and J. Troppmair, Anticancer Res., 2009, 29, 419–426 Search PubMed.
- J. Sulé-Suso, A. Pysanenko, P. Španěl and D. Smith, Analyst, 2009, 134, 2419–2425 RSC.
- O. Barash, N. Peled, F. R. Hirsch and H. Haick, Small, 2009, 5, 2618–2624 CrossRef PubMed.
- O. Barash, N. Peled, U. Tisch, P. A. Bunn Jr., F. R. Hirsch and H. Haick, Nanomedicine, 2012, 8, 580–589 CrossRef PubMed.
- N. Peled, O. Barash, U. Tisch, R. Ionescu, Y. Y. Broza, M. Ilouze, J. Mattei, P. A. Bunn, Jr., F. R. Hirsch and H. Haick, Nanomedicine, 2013, 9, 758–766 CrossRef PubMed.
- M. Davies, O. Barash, R. Jeries, N. Peled, M. Ilouze, R. Hyde, M. Marcus, J. Field and H. Haick, Br. J. Cancer, 2014, 111, 1213 CrossRef PubMed.
- K. B. Gendron, N. G. Hockstein, E. R. Thaler, A. Vachani and C. W. Hanson, Otolaryngol.–Head Neck Surg., 2007, 137, 269–273 CrossRef PubMed.
- L. Lavra, A. Catini, A. Ulivieri, R. Capuano, L. B. Salehi, S. Sciacchitano, A. Bartolazzi, S. Nardis, R. Paolesse, E. Martinelli and C. D. Natale, Sci. Rep., 2015, 5, 13246 CrossRef PubMed.
- A. Roine, M. Tolvanen, M. Sipilainen, P. Kumpulainen, M. A. Helenius, T. Lehtimaki, J. Vepsalainen, T. A. Keinanen, M. R. Hakkinen, J. Koskimaki, E. Veskimae, A. Tuokko, T. Visakorpi, T. L. Tammela, T. Sioris, T. Paavonen, J. Lekkala, H. Helle and N. K. J. Oksala, Future Oncol., 2012, 8, 1157–1165 CrossRef PubMed.
- H. Amal, L. Ding, B. B. Liu, U. Tisch, Z. Q. Xu, D. Y. Shi, Y. Zhao, J. Chen, R. X. Sun, H. Liu, S. L. Ye, Z. Y. Tang and H. Haick, Int. J. Nanomed., 2012, 7, 4135–4146 Search PubMed.
- Y. X. Zhang, G. Gao, H. J. Liu, H. L. Fu, J. Fan, K. Wang, Y. S. Chen, B. J. Li, C. L. Zhang, X. Zhi, L. He and D. X. Cui, Theranostics, 2014, 4, 154–162 CrossRef PubMed.
- B. Kateb, M. Ryan, M. Homer, L. Lara, Y. Yin, K. Higa and M. Y. Chen, NeuroImage, 2009, 47, T5–T9 CrossRef PubMed.
- R. L. Siegel, K. D. Miller and A. Jemal, Ca-Cancer J. Clin., 2017, 67, 7–30 CrossRef PubMed.
- G. P. Guy, D. U. Ekwueme, F. K. Tangka and L. C. Richardson, Am. J. Prev. Med., 2012, 43, 537–545 CrossRef PubMed.
- T. Abaffy, M. G. Möller, D. D. Riemer, C. Milikowski and R. A. DeFazio, Metabolomics, 2013, 9, 998–1008 CrossRef PubMed.
- G. Pennazza, M. Santonico, A. Bartolazzi, R. Paolesse, C. Di Natale, R. Bono, V. Tamburrelli, S. Cristina and A. D'Amico, Procedia Chem., 2009, 1, 995–998 CrossRef.
- A. Bartolazzi, M. Santonico, G. Pennazza, E. Martinelli, R. Paolesse, A. D'Amico and C. D. Natale, Sens. Actuators, B, 2010, 146, 483–488 CrossRef.
- G. Pennazza, M. Santonico, E. Martinelli, R. Paolesse, V. Tamburrelli, S. Cristina, A. D’Amico, C. Di Natale and A. Bartolazzi, Sens. Actuators, B, 2011, 154, 288–294 CrossRef.
- F. R. Coelho and J. O. Martins, Rev. Assoc. Med. Bras., 2012, 58, 498–504 CrossRef PubMed.
- L. S. Jackson, J. Agric. Food Chem., 2009, 57, 8161–8170 CrossRef PubMed.
- S. Riedel and K. C. Carroll, J. Infect. Chemother., 2010, 16, 301–316 CrossRef PubMed.
- J. R. Carey, K. S. Suslick, K. I. Hulkower, J. A. Imlay, K. R. Imlay, C. K. Ingison, J. B. Ponder, A. Sen and A. E. Wittrig, J. Am. Chem. Soc., 2011, 133, 7571–7576 CrossRef PubMed.
- M. Holmberg, F. Gustafsson, E. G. Hörnsten, F. Winquist, L. E. Nilsson, L. Ljung and I. Lundström, Biotechnol. Tech., 1998, 12, 319–324 CrossRef.
- M. Holmberg, F. Gustafsson, E. Hörnsten, F. Winquist, L. Nilsson, L. Ljung and I. Lundström, Biotechnol. Tech., 2004, 12, 319–324 CrossRef.
- T. Gibson, O. Prosser, J. Hulbert, R. Marshall, P. Corcoran, P. Lowery, E. Ruck-Keene and S. Heron, Sens. Actuators, B, 1997, 44, 413–422 CrossRef.
- C. L. Lonsdale, B. Taba, N. Queralto, R. A. Lukaszewski, R. A. Martino, P. A. Rhodes and S. H. Lim, PLoS One, 2013, 8, e62726 Search PubMed.
- C. McEntegart, W. Penrose, S. Strathmann and J. Stetter, Sens. Actuators, B, 2000, 70, 170–176 CrossRef.
- M. Moens, A. Smet, B. Naudts, J. Verhoeven, M. Ieven, P. Jorens, H. Geise and F. Blockhuys, Lett. Appl. Microbiol., 2006, 42, 121–126 CrossRef PubMed.
- J. Karasinski, S. Andreescu, O. A. Sadik, B. Lavine and M. N. Vora, Anal. Chem., 2005, 77, 7941–7949 CrossRef PubMed.
- I. A. Casalinuovo, D. Di Pierro, M. Coletta and P. D. Francesco, Sensors, 2006, 6, 1428–1439 CrossRef.
- J. Gardner, M. Craven, C. Dow and E. Hines, Meas. Sci. Technol., 1998, 9, 120 CrossRef.
- A. K. Pavlou, N. Magan, J. M. Jones, J. Brown, P. R. Klatser and A. P. Turner, Biosens. Bioelectron., 2004, 20, 538–544 CrossRef PubMed.
- R. Fend, A. H. Kolk, C. Bessant, P. Buijtels, P. R. Klatser and A. C. Woodman, J. Clin. Microbiol., 2006, 44, 2039–2045 CrossRef PubMed.
- N. Peled, M. Hakim, P. A. Bunn, Y. E. Miller, T. C. Kennedy, J. Mattei, J. D. Mitchell, F. R. Hirsch and H. Haick, J. Thorac. Oncol., 2012, 7, 1528–1533 CrossRef PubMed.
- D. Shlomi, M. Abud, O. Liran, J. Bar, N. Gai-Mor, M. Ilouze, A. Onn, A. Ben-Nun, H. Haick and N. Peled, J. Thorac. Oncol., 2017, 12, 1544–1551 CrossRef PubMed.
- I. Nardi-Agmon, M. Abud-Hawa, O. Liran, N. Gai-Mor, M. Ilouze, A. Onn, J. Bar, D. Shlomi, H. Haick and N. Peled, J. Thorac. Oncol., 2016, 11, 827–837 CrossRef PubMed.
- G. Peng, M. Hakim, Y. Y. Broza, S. Billan, R. Abdah-Bortnyak, A. Kuten, U. Tisch and H. Haick, Br. J. Cancer, 2010, 103, 542–551 CrossRef PubMed.
- S. Dragonieri, J. T. Annema, R. Schot, M. P. C. van der Schee, A. Spanevello, P. Carratu, O. Resta, K. F. Rabe and P. J. Sterk, Lung Cancer, 2009, 64, 166–170 CrossRef PubMed.
- R. F. Machado, D. Laskowski, O. Deffenderfer, T. Burch, S. Zheng, P. J. Mazzone, T. Mekhail, C. Jennings, J. K. Stoller, J. Pyle, J. Duncan, R. A. Dweik and S. C. Erzurum, Am. J. Respir. Crit. Care Med., 2005, 171, 1286–1291 CrossRef PubMed.
- C. Di Natale, A. Macagnano, E. Martinelli, R. Paolesse, G. D'Arcangelo, C. Roscioni, A. Finazzi-Agro and A. D'Amico, Biosens. Bioelectron., 2003, 18, 1209–1218 CrossRef PubMed.
- A. D'Amico, G. Pennazza, M. Santonico, E. Martinelli, C. Roscioni, G. Galluccio, R. Paolesse and C. Di Natale, Lung Cancer, 2010, 68, 170–176 CrossRef PubMed.
- M. Santonico, G. Lucantoni, G. Pennazza, R. Capuano, G. Galluccio, C. Roscioni, G. La Delfa, D. Consoli, E. Martinelli, R. Paolesse, C. Di Natale and A. D'Amico, Lung Cancer, 2012, 77, 46–50 CrossRef PubMed.
- X. Chen, M. F. Cao, Y. Li, W. J. Hu, P. Wang, K. J. Ying and H. M. Pan, Meas. Sci. Technol., 2005, 16, 1535–1546 CrossRef.
- P. J. Mazzone, J. Hammel, R. Dweik, J. Na, C. Czich, D. Laskowski and T. Mekhail, Thorax, 2007, 62, 565–568 CrossRef PubMed.
- P. J. Mazzone, X. F. Wang, Y. M. Xu, T. Mekhail, M. C. Beukemann, J. Na, J. W. Kemling, K. S. Suslick and M. Sasidhar, J. Thorac. Oncol., 2012, 7, 137–142 CrossRef PubMed.
- E. A. Chapman, P. S. Thomas, E. Stone, C. Lewis and D. H. Yates, Eur. Respir. J., 2012, 40, 448–454 CrossRef PubMed.
- G. Shuster, Z. Gallimidi, A. H. Reiss, E. Dovgolevsky, S. Billan, R. Abdah-Bortnyak, A. Kuten, A. Engel, A. Shiban, U. Tisch and H. Haick, Breast Cancer Res. Treat., 2011, 126, 791–796 CrossRef PubMed.
- O. Barash, W. Zhang, J. M. Halpern, Q. L. Hua, Y. Y. Pan, H. Kayal, K. Khoury, H. Liu, M. P. A. Davies and H. Haick, Oncotarget, 2015, 6, 44864–44876 CrossRef PubMed.
- Z. Xu, Y. Y. Broza, R. Ionsecu, U. Tisch, L. Ding, H. Liu, Q. Song, Y. Pan, F. Xiong and K. Gu, Br. J. Cancer, 2013, 108, 941 CrossRef PubMed.
- H. Amal, M. Leja, K. Funka, R. Skapars, A. Sivins, G. Ancans, I. Liepniece-Karele, I. Kikuste, I. Lasina and H. Haick, Gut, 2016, 65, 400–407 CrossRef PubMed.
- H. Amal, M. Leja, K. Funka, I. Lasina, R. Skapars, A. Sivins, G. Ancans, I. Kikuste, A. Vanags, I. Tolmanis, A. Kirsners, L. Kupcinskas and H. Haick, Int. J. Cancer, 2016, 138, 229–236 CrossRef PubMed.
- M. Gruber, U. Tisch, R. Jeries, H. Amal, M. Hakim, O. Ronen, T. Marshak, D. Zimmerman, O. Israel and E. Amiga, Br. J. Cancer, 2014, 111, 790–798 CrossRef PubMed.
- N. Leunis, M. L. Boumans, B. Kremer, S. Din, E. Stobberingh, A. G. Kessels and K. W. Kross, Laryngoscope, 2014, 124, 1377–1381 CrossRef PubMed.
- S. Cohen-Kaminsky, M. Nakhleh, F. Perros, D. Montani, B. Girerd, G. Garcia, G. Simonneau, H. Haick and M. Humbert, Am. J. Respir. Crit. Care Med., 2013, 188, 756–759 CrossRef PubMed.
- M. K. Nakhleh, H. Haick, M. Humbert and S. Cohen-Kaminsky, Eur. Respir. J., 2017, 49, 01897 Search PubMed.
- K. Witt, C. Fischer, S. Reulecke, V. Kechagias, R. Surber, H. R. Figulla and A. Voss, Biomed. Tech., 2013, 58, 1–2 Search PubMed.
- O. Marom, F. Nakhoul, U. Tisch, A. Shiban, Z. Abassi and H. Haick, Nanomedicine, 2012, 7, 639–650 CrossRef PubMed.
- S. Assady, O. Marom, M. Hemli, R. Ionescu, R. Jeries, U. Tisch, Z. Abassi and H. Haick, Nanomedicine, 2014, 9, 1035–1045 CrossRef PubMed.
- M. K. Nakhleh, H. Amal, H. Awad, A. Gharra, N. Abu-Saleh, R. Jeries, H. Haick and Z. Abassi, Nanomedicine, 2014, 10, 1767–1776 CrossRef PubMed.
- U. Tisch, Y. Aluf, R. Ionescu, M. Nakhleh, R. Bassal, N. Axelrod, D. Robertman, Y. Tessler, J. P. Finberg and H. Haick, ACS Chem. Neurosci., 2012, 3, 161–166 CrossRef PubMed.
- J. P. M. Finberg, Y. Aluf, Y. Loboda, M. K. Nakhleh, R. Jeries, M. Abud-Hawa, S. Zubedat, A. Avital, S. Khatib, J. Vaya and H. Haick, ACS Chem. Neurosci., 2018, 9, 291–297 CrossRef PubMed.
- M. K. Nakhleh, S. Badarny, R. Winer, R. Jeries, J. Finberg and H. Haick, Parkinsonism Relat. Disord., 2015, 21, 150–153 CrossRef PubMed.
- U. Tisch, I. Schlesinger, R. Ionescu, M. Nassar, N. Axelrod, D. Robertman, Y. Tessler, F. Azar, A. Marmur, J. Aharon-Peretz and H. Haick, Nanomedicine, 2013, 8, 43–56 CrossRef PubMed.
- J. P. Bach, M. Gold, D. Mengel, A. Hattesohl, D. Lubbe, S. Schmid, B. Tackenberg, J. Rieke, S. Maddula, J. I. Baumbach, C. Nell, T. Boeselt, J. Michelis, J. Alferink, M. Heneka, W. Oertel, F. Jessen, S. Janciauskiene, C. Vogelmeier, R. Dodel and A. R. Koczulla, PLoS One, 2015, 10, e0132227 Search PubMed.
- A. Mazzatenta, M. Pokorski, F. Sartucci, L. Domenici and C. D. Giulio, Respir. Physiol. Neurobiol., 2015, 209, 81–84 CrossRef PubMed.
- H. C. Lau, J. B. Yu, H. W. Lee, J. S. Huh and J. O. Lim, Sensors, 2017, 17, 1783 CrossRef PubMed.
- R. Milo and A. Miller, Autoimmun. Rev., 2014, 13, 518–524 CrossRef PubMed.
- J. H. Noseworthy, C. Lucchinetti, M. Rodriguez and B. G. Weinshenker, N. Engl. J. Med., 2000, 343, 938–952 CrossRef PubMed.
- R. Ionescu, Y. Broza, H. Shaltieli, D. Sadeh, Y. Zilberman, X. Feng, L. Glass-Marmor, I. Lejbkowicz, K. Mullen, A. Miller and H. Haick, ACS Chem. Neurosci., 2011, 2, 687–693 CrossRef PubMed.
- J. Banerjee, V. Nema, Y. Dhas and N. Mishra, Biochimie, 2017, 139, 9–19 CrossRef PubMed.
- World Health Organization (WHO), Global report on diabetes, World Health Organization, 2016.
- K. Krönert, M. Künzel, B. Reutter, C. Zimmermann, H. M. Liebich, D. Luft and M. Eggstein, Diabetes Res. Clin. Pract., 1990, 10, 161–165 CrossRef.
- H. M. Liebich, J. Chromatogr. B: Biomed. Sci. Appl., 1983, 273, 67–75 CrossRef.
- S. Esfahani, N. M. Sagar, I. Kyrou, E. Mozdiak, N. O’Connell, C. Nwokolo, K. D. Bardhan, R. P. Arasaradnam and J. A. Covington, Biosensors, 2016, 6, 4 CrossRef PubMed.
- D. Smith, P. Španěl, A. A. Fryer, F. Hanna and G. A. A. Ferns, J. Breath Res., 2011, 5, 022001 CrossRef PubMed.
- P. Španěl, K. Dryahina, A. Rejšková, T. W. E. Chippendale and D. Smith, Physiol. Meas., 2011, 32, N23 CrossRef PubMed.
- K. Yan, D. Zhang, D. Wu, H. Wei and G. Lu, IEEE Trans. Biomed. Eng., 2014, 61, 2787–2795 CrossRef PubMed.
- R. Jaisutti, M. Lee, J. Kim, S. Choi, T.-J. Ha, J. Kim, H. Kim, S. K. Park and Y.-H. Kim, ACS Appl. Mater. Interfaces, 2017, 9, 8796–8804 Search PubMed.
- J.-B. Yu, H.-G. Byun, M.-S. So and J.-S. Huh, Sens. Actuators, B, 2005, 108, 305–308 CrossRef.
- P.-J. Chien, T. Suzuki, M. Tsujii, M. Ye, I. Minami, K. Toda, H. Otsuka, K. Toma, T. Arakawa and K. Araki, Anal. Chem., 2017, 89, 12261–12268 CrossRef PubMed.
- M. K. Nakhleh, R. Jeries, A. Gharra, A. Binder, Y. Y. Broza, M. Pascoe, K. Dheda and H. Haick, Eur. Respir. J., 2014, 43, 1522–1525 CrossRef PubMed.
- N. Peled, R. Ionescu, P. Nol, O. Barash, M. McCollum, K. VerCauteren, M. Koslow, R. Stahl, J. Rhyan and H. Haick, Sens. Actuators, B, 2012, 171, 588–594 CrossRef.
- N. M. Zetola, C. Modongo, O. Matsiri, T. Tamuhla, B. Mbongwe, K. Matlhagela, E. Sepako, A. Catini, G. Sirugo, E. Martinelli, R. Paolesse and C. Di Natale, J. Infect., 2017, 74, 367–376 CrossRef PubMed.
- Y. S. Cho, S. C. Jung and S. Oh, Lett. Appl. Microbiol., 2015, 60, 513–516 CrossRef PubMed.
- M. Shirasu and K. Touhara, J. Biochem., 2011, 150, 257–266 CrossRef PubMed.
-
B. de Lacy Costello, N. M. Ratcliffe and D. Smith, Volatile organic compounds (VOCs) found in urine and stool, Elsevier, Amsterdam, The Netherlands, 2013 Search PubMed.
- D. Samudrala, B. Geurts, P. A. Brown, E. Szymańska, J. Mandon, J. Jansen, L. Buydens, F. J. M. Harren and S. M. Cristescu, Metabolomics, 2015, 11, 1656–1666 CrossRef PubMed.
- E. V. Schrezenmeier, J. Barasch, K. Budde, T. Westhoff and K. M. Schmidt-Ott, Acta Physiol., 2017, 219, 556–574 CrossRef PubMed.
- A. Masajtis-Zagajewska and M. Nowicki, Clin. Chim. Acta, 2017, 471, 286–291 CrossRef PubMed.
-
S. Siyang, C. Wongchoosuk and T. Kerdcharoen, The 5th 2012 Biomedical Engineering International Conference, Ubon Ratchathani, 2012, pp. 1–4 Search PubMed.
- A. Srivastava, L. M. Douglass, V. Chernyak and K. L. Watts, Curr. Neurol. Neurosci. Rep., 2017, 18, 69 Search PubMed.
- M. Monteiro, N. Moreira, J. Pinto, A. S. Pires-Luís, R. Henrique, C. Jerónimo, M. d. L. Bastos, A. M. Gil, M. Carvalho and P. Guedes de Pinho, J. Cell. Mol. Med., 2017, 21, 2092–2105 CrossRef PubMed.
- M. Bernabei, G. Pennazza, M. Santonico, C. Corsi, C. Roscioni, R. Paolesse, C. Di Natale and A. D’Amico, Sens. Actuators, B, 2008, 131, 1–4 CrossRef.
- C. M. Weber, M. Cauchi, M. Patel, C. Bessant, C. Turner, L. E. Britton and C. M. Willis, Analyst, 2011, 136, 359–364 RSC.
- A. Roine, E. Veskimäe, A. Tuokko, P. Kumpulainen, J. Koskimäki, T. A. Keinänen, M. R. Häkkinen, J. Vepsäläinen, T. Paavonen and J. Lekkala, J. Urol., 2014, 192, 230–235 CrossRef PubMed.
- M. Santonico, G. Pennazza, A. D. Asimakopoulos, D. Del Fabbro, R. Miano, R. Capuano, E. Finazzi-Agrò and A. D’Amico, Procedia Eng., 2014, 87, 320–323 CrossRef.
- M. Horstmann, D. Steinbach, C. Fischer, A. Enkelmann, M.-O. Grimm and A. Voss, J. Urol., 2015, 193, e560–e561 CrossRef.
- A. Roine, T. Saviauk, P. Kumpulainen, M. Karjalainen, A. Tuokko, J. Aittoniemi, R. Vuento, J. Lekkala, T. Lehtimäki and T. L. Tammela, PLoS One, 2014, 9, e114279 Search PubMed.
- A. D’Amico, M. Santonico, G. Pennazza, R. Capuano, G. Vespasiani, D. Del Fabbro, R. Paolesse, C. Di Natale, E. Martinelli and E. F. Agrò, Procedia Eng., 2012, 47, 1113–1116 CrossRef.
- A. D. Asimakopoulos, D. Del Fabbro, R. Miano, M. Santonico, R. Capuano, G. Pennazza, A. D'amico and E. Finazzi-Agro, Prostate Cancer Prostatic Dis., 2014, 17, 206–211 CrossRef PubMed.
- J.-L. Kim, L. Elfman, Y. Mi, G. Wieslander, G. Smedje and D. Norbäck, Indoor Air, 2007, 17, 153–163 CrossRef PubMed.
- S. Zehm, S. Schweinitz, R. Würzner, H. P. Colvin and J. Rieder, Curr. Microbiol., 2012, 64, 271–275 CrossRef PubMed.
- T. Nawrath, G. F. Mgode, B. Weetjens, S. H. E. Kaufmann and S. Schulz, Beilstein J. Org. Chem., 2012, 8, 290 CrossRef PubMed.
- M. Nakhleh, R. Jeries, A. Gharra, A. Binder, Y. Y. Broza, M. Pascoe, K. Dheda and H. Haick, Eur. Respir. J., 2014, 43, 1522–1525 CrossRef PubMed.
- M. C. Lonergan, E. J. Severin, B. J. Doleman, S. A. Beaber, R. H. Grubbs and N. S. Lewis, Chem. Mater., 1996, 8, 2298–2312 CrossRef.
- J. W. T. Yates, M. J. Chappell, J. W. Gardner, C. S. Dow, C. Dowson, A. Hamood, F. Bolt and L. Beeby, Comput. Methods Programs Biomed., 2005, 79, 259–271 CrossRef PubMed.
-
T. M. A. Sabeel, F. K. CheHarun, S. E. Eluwa and S. M. A. Sabeel, International conference on computing, electrical and electronic engineering (ICCEEE), Khartoum, 2013, pp. 1–4 Search PubMed.
- A. K. Pavlou, N. Magan, C. McNulty, J. M. Jones, D. Sharp, J. Brown and A. P. F. Turner, Biosens. Bioelectron., 2002, 17, 893–899 CrossRef PubMed.
- V. S. Kodogiannis, J. N. Lygouras, A. Tarczynski and H. S. Chowdrey, IEEE Transactions on Information Technology in Biomedicine, 2008, 12, 707–713 CrossRef PubMed.
- D. C. Baumgart and S. R. Carding, Lancet, 2007, 369, 1627–1640 CrossRef.
- R. P. Arasaradnam, N. Ouaret, M. G. Thomas, N. Quraishi, E. Heatherington, C. U. Nwokolo, K. D. Bardhan and J. A. Covington, Inflammatory Bowel Dis., 2013, 19, 999–1003 CrossRef PubMed.
- J. A. Covington, E. W. Westenbrink, N. Ouaret, R. Harbord, C. Bailey, N. O'Connell, J. Cullis, N. Williams, C. U. Nwokolo and K. D. Bardhan, Sensors, 2013, 13, 11899–11912 CrossRef PubMed.
- C. Di Natale, A. Mantini, A. Macagnano, D. Antuzzi, R. Paolesse and A. D'Amico, Physiol.
Meas., 1999, 20, 377 CrossRef PubMed.
- O. Deda, H. G. Gika, I. D. Wilson and G. A. Theodoridis, J. Pharm. Biomed. Anal., 2015, 113, 137–150 CrossRef PubMed.
- A. Vrieze, F. Holleman, E. G. Zoetendal, W. M. De Vos, J. B. L. Hoekstra and M. Nieuwdorp, Diabetologia, 2010, 53, 606–613 CrossRef PubMed.
- L. Dethlefsen, M. McFall-Ngai and D. A. Relman, Nature, 2007, 449, 811–818 CrossRef PubMed.
- E. Holmes, J. V. Li, T. Athanasiou, H. Ashrafian and J. K. Nicholson, Trends Microbiol., 2011, 19, 349–359 CrossRef PubMed.
- K. S. Smirnov, T. V. Maier, A. Walker, S. S. Heinzmann, S. Forcisi, I. Martinez, J. Walter and P. Schmitt-Kopplin, Int. J. Med. Microbiol., 2016, 306, 266–279 CrossRef PubMed.
- T. G. Meij, I. B. Larbi, M. P. Schee, Y. E. Lentferink, T. Paff, J. S. Terhaar sive Droste, C. J. Mulder, A. A. Bodegraven and N. K. Boer, Int. J. Cancer, 2014, 134, 1132–1138 CrossRef PubMed.
- S. F. Shepherd, N. D. McGuire, B. P. J. de Lacy Costello, R. J. Ewen, D. H. Jayasena, K. Vaughan, I. Ahmed, C. S. Probert and N. M. Ratcliffe, J. Breath Res., 2014, 8, 026001 CrossRef PubMed.
- J. A. Covington, L. Wedlake, J. Andreyev, N. Ouaret, M. G. Thomas, C. U. Nwokolo, K. D. Bardhan and R. P. Arasaradnam, Sensors, 2012, 12, 13002–13018 CrossRef PubMed.
- R. B. Aggio, P. White, H. Jayasena, B. de Lacy Costello, N. M. Ratcliffe and C. S. Probert, Aliment. Pharmacol. Ther., 2017, 45, 82–90 CrossRef PubMed.
- M. Rossi, R. Aggio, H. M. Staudacher, M. C. Lomer, J. O. Lindsay, P. Irving, C. Probert and K. Whelan, Clin. Gastroenterol. Hepatol., 2017, 6, 31201–31206 Search PubMed.
- T. G. de Meij, N. K. de Boer, M. A. Benninga, Y. E. Lentferink, E. F. de Groot, M. E. van de Velde, A. A. van Bodegraven and M. P. van der Schee, J. Crohns Colitis, 2014 DOI:10.1016/j.crohns.2014.09.004.
- T. G. J. de Meij, M. P. C. van der Schee, D. J. C. Berkhout, M. E. van de Velde, A. E. Jansen, B. W. Kramer, M. M. van Weissenbruch, A. H. van Kaam, P. r. Andriessen and J. B. van Goudoever, J. Pediatr., 2015, 167, 562–567 CrossRef PubMed.
- N. D. McGuire, R. J. Ewen, B. de Lacy Costello, C. E. Garner, C. S. J. Probert, K. Vaughan and N. M. Ratcliffe, Meas. Sci. Technol., 2014, 25, 065108 CrossRef PubMed.
- D. J. C. Berkhout, H. J. Niemarkt, M. Buijck, M. M. Van Weissenbruch, P. Brinkman, M. A. Benninga, A. H. van Kaam, B. W. Kramer, P. r. Andriessen and N. K. H. de Boer, J. Pediatr. Gastroenterol. Nutr., 2017, 65, e47–e52 CrossRef PubMed.
- R. M. Nagler, O. Hershkovich, S. Lischinsky, E. Diamond and A. Z. Reznick, J. Invest. Med., 2002, 50, 214–225 CrossRef PubMed.
- M. Gallagher, C. J. Wysocki, J. J. Leyden, A. I. Spielman, X. Sun and G. Preti, Br. J. Dermatol., 2008, 159, 780–791 CrossRef PubMed.
- A. Koh, D. Kang, Y. Xue, S. Lee, R. M. Pielak, J. Kim, T. Hwang, S. Min, A. Banks, P. Bastien, M. C. Manco, L. Wang, K. R. Ammann, K.-I. Jang, P. Won, S. Han, R. Ghaffari, U. Paik, M. J. Slepian, G. Balooch, Y. Huang and J. A. Rogers, Sci. Transl. Med., 2016, 8, 366ra165 CrossRef PubMed.
- A. J. Bandodkar, V. W. S. Hung, W. Jia, G. Valdés-Ramírez, J. R. Windmiller, A. G. Martinez, J. Ramírez, G. Chan, K. Kerman and J. Wang, Analyst, 2013, 138, 123–128 RSC.
-
http://www.aarp.org/health/conditions-treatments/info-2017/color-changing-tattoos-health-monitoring-fd.html
.
- Z.-M. Zhang, J.-J. Cai, G.-H. Ruan and G.-K. Li, J. Chromatogr. B: Anal. Technol. Biomed. Life Sci., 2005, 822, 244–252 CrossRef PubMed.
- M. Kusano, E. Mendez and K. G. Furton, J. Forensic Sci., 2013, 58, 29–39 CrossRef PubMed.
- P. Mochalski, K. Unterkofler, H. Hinterhuber and A. Amann, Anal. Chem., 2014, 86, 3915–3923 CrossRef PubMed.
- K. Liddell, Postgrad. Med. J., 1976, 52, 136–138 CrossRef PubMed.
- W. Z. D. Stitt and L. A. Goldsmith, Arch. Dermatol., 1995, 131, 997–999 CrossRef.
- P. J. Honig, I. J. Frieden, H. J. Kim and A. C. Yan, Pediatrics, 2003, 112, 1427–1429 CrossRef PubMed.
- B. W. M. Moeskops, M. M. L. Steeghs, K. Van Swam, S. M. Cristescu, P. T. J. Scheepers and F. J. M. Harren, Physiol. Meas., 2006, 27, 1187 CrossRef PubMed.
- C. Di Natale, A. Macagnano, R. Paolesse, E. Tarizzo, A. Mantini and A. D'Amico, Sens. Actuators, B, 2000, 65, 216–219 CrossRef.
- Y. Y. Broza, L. Zuri and H. Haick, Sci. Rep., 2014, 4, 1–6 Search PubMed.
- P. Martínez-Lozano and J. F. de la Mora, J. Am. Soc. Mass Spectrom, 2009, 20, 1060–1063 CrossRef PubMed.
- L. Dormont, J.-M. Bessière and A. Cohuet, J. Chem. Ecol., 2013, 39, 569–578 CrossRef PubMed.
- P. Mochalski, J. King, K. Unterkofler, H. Hinterhuber and A. Amann, J. Chromatogr. B: Anal. Technol. Biomed. Life Sci., 2014, 959, 62–70 CrossRef PubMed.
- S. Riazanskaia, G. Blackburn, M. Harker, D. Taylor and C. L. P. Thomas, Analyst, 2008, 133, 1020–1027 RSC.
- A. M. Curran, S. I. Rabin, P. A. Prada and K. G. Furton, J. Chem. Ecol., 2005, 31, 1607–1619 CrossRef PubMed.
- H. Kataoka, Anal. Sci., 2011, 27, 893 CrossRef PubMed.
- A. D'amico, R. Bono, G. Pennazza, M. Santonico, G. Mantini, M. Bernabei, M. Zarlenga, C. Roscioni, E. Martinelli and R. Paolesse, Skin Res. Technol., 2008, 14, 226–236 CrossRef PubMed.
- C. Di Natale, R. Paolesse, P. Comandini, G. Pennazza, E. Martinelli, S. Rullo, M. Roscioni, C. Roscioni and A. Finazzi-Agro, Med. Sci. Monit., 2005, 11, CR366–CR375 Search PubMed.
- C. Turner, Expert Rev. Mol. Diagn., 2011, 11, 497–503 CrossRef PubMed.
- N. Yamane, T. Tsuda, K. Nose, A. Yamamoto, H. Ishiguro and T. Kondo, Clin. Chim. Acta, 2006, 365, 325–329 CrossRef PubMed.
- C. Turner, B. Parekh, C. Walton, P. Španěl, D. Smith and M. Evans, Rapid Commun. Mass Spectrom., 2008, 22, 526–532 CrossRef PubMed.
- Z. Zhang and G. Li, Microchem. J., 2010, 95, 127–139 CrossRef.
- S. G. Gordon, K. Smith, J. L. Rabinowitz and P. R. Vagelos, J. Lipid Res., 1973, 14, 495–503 Search PubMed.
- K. Smith, G. F. Thompson and H. D. Koster, Science, 1969, 166, 398–399 Search PubMed.
- U. R. Bernier, M. M. Booth and R. A. Yost, Anal. Chem., 1999, 71, 1–7 CrossRef PubMed.
- U. R. Bernier, D. L. Kline, D. R. Barnard, C. E. Schreck and R. A. Yost, Anal. Chem., 2000, 72, 747–756 CrossRef PubMed.
- M. Shirasu, S. Nagai, R. Hayashi, A. Ochiai and K. Touhara, Biosci., Biotechnol., Biochem., 2009, 73, 2117–2120 CrossRef PubMed.
- A. N. Thomas, S. Riazanskaia, W. Cheung, Y. Xu, R. Goodacre, C. L. Thomas, M. S. Baguneid and A. Bayat, Wound Repair Regen., 2010, 18, 391–400 CrossRef PubMed.
- H.-G. Byun, K. C. Persaud and A. M. Pisanelli, ETRI J., 2010, 32, 440–446 CrossRef.
- J. G. Logan, M. A. Birkett, S. J. Clark, S. Powers, N. J. Seal, L. J. Wadhams, A. J. Mordue and J. A. Pickett, J. Chem. Ecol., 2008, 34, 308 CrossRef PubMed.
- A. Voss, K. Witt, C. Fischer, S. Reulecke, W. Poitz, V. Kechagias, R. Surber and H. R. Figulla, Conf. Proc. IEEE Eng. Med. Biol. Soc., 2012, 7, 6346852 Search PubMed.
- A. Voss, V. Baier, R. Reisch, K. von Roda, P. Elsner, H. Ahlers and G. Stein, Ann. Biomed. Eng., 2005, 33, 656–660 CrossRef PubMed.
- A. D'Amico, C. Di Natale, A. Macagnano, F. Davide, A. Mantini, E. Tarizzo, R. Paolesse and T. Boschi, Biosens. Bioelectron., 1998, 13, 711–721 CrossRef.
- A. D'Amico, C. Di Natale, R. Paolesse, A. Macagnano and A. Mantini, Sens. Actuators, B, 2000, 65, 209–215 CrossRef.
- C. Di Natale, R. Paolesse and A. D’Amico, Sens. Actuators, B, 2007, 121, 238–246 CrossRef.
- H. Ulmer, J. Mitrovics, U. Weimar and W. Göpel, Sens. Actuators, B, 2000, 65, 79–81 CrossRef.
- M. Pardo, L. G. Kwong, G. Sberveglieri, K. Brubaker, J. F. Schneider, W. R. Penrose and J. R. Stetter, Sens. Actuators, B, 2005, 106, 136–143 CrossRef.
- M. Holmberg, F. Winquist, I. Lundström, J. W. Gardner and E. L. Hines, Sens. Actuators, B, 1995, 27, 246–249 CrossRef.
- H. Ulmer, J. Mitrovics, G. Noetzel, U. Weimar and W. Göpel, Sens. Actuators, B, 1997, 43, 24–33 CrossRef.
- C. Jin, P. Kurzawski, A. Hierlemann and E. T. Zellers, Anal. Chem., 2008, 80, 227–236 CrossRef PubMed.
- C. Jin and E. T. Zellers, Anal. Chem., 2008, 80, 7283–7293 CrossRef PubMed.
- N. P. Pont, C. A. Kendall and N. Magan, Mycopathologia, 2012, 173, 93–101 CrossRef PubMed.
- C.-L. Li, Y.-F. Chen, M.-H. Liu and C.-J. Lu, Sens. Actuators, B, 2012, 169, 349–359 CrossRef.
- H.-L. Lu, C.-J. Lu, W.-C. Tian and H.-J. Sheen, Talanta, 2015, 131, 467–474 CrossRef PubMed.
- K. Scholten, L. K. Wright and E. T. Zellers, IEEE Sens. J., 2013, 13, 2146–2154 CrossRef.
- R. Gutierrez-Osuna and A. Hierlemann, Annu. Rev. Anal. Chem., 2010, 3, 255–276 CrossRef PubMed.
- D. C. Meier, B. Raman and S. Semancik, Annu. Rev. Anal. Chem., 2009, 2, 463–484 CrossRef PubMed.
- R. E. Pogue, D. P. Cavalcanti, S. Shanker, R. V. Andrade, L. R. Aguiar, J. L. de Carvalho and F. F. Costa, Drug Discovery Today, 2017, 23, 187–195 CrossRef PubMed.
- P. K. Drain, E. P. Hyle, F. Noubary, K. A. Freedberg, D. Wilson, W. R. Bishai, W. Rodriguez and I. V. Bassett, Lancet Infect. Dis., 2014, 14, 239–249 CrossRef PubMed.
- D. Kuupiel, V. Bawontuo and T. P. Mashamba-Thompson, Diagnostics, 2017, 7, 58 CrossRef PubMed.
- P. B. Luppa, C. Müller, A. Schlichtiger and H. Schlebusch, TrAC, Trends Anal. Chem., 2011, 30, 887–898 CrossRef.
- K. J. Johnson and S. L. Rose-Pehrsson, Annu. Rev. Anal. Chem., 2015, 8, 287–310 CrossRef PubMed.
Footnotes |
† Electronic supplementary information (ESI) available. See DOI: 10.1039/c8cs00317c |
‡ These authors contributed equally to this study. |
|
This journal is © The Royal Society of Chemistry 2018 |
Click here to see how this site uses Cookies. View our privacy policy here.