DOI:
10.1039/C8AN00729B
(Paper)
Analyst, 2018,
143, 4189-4198
Electrical impedance tomography for real-time and label-free cellular viability assays of 3D tumour spheroids
Received
19th April 2018
, Accepted 21st July 2018
First published on 24th July 2018
Abstract
There is currently a need to culture cells in 3D to better mimic the behaviour of cells growing in the natural environment. In parallel, this calls for novel technologies to assess cell growth in 3D cell culture. In this study, we demonstrated both in silico and in vitro that cell viability inside large cell spheroids could be monitored in real time and label-free with electrical impedance tomography (EIT). Simulations using a single shell model and the effective media approximation (EMA) method were performed to prove the performance of EIT on spheroid imaging and viability monitoring. Then in vitro experiments were conducted to measure in real time a loss of cell viability in MCF-7 breast cancer spheroids when exposed to Triton X-100 and validate with conventional biochemical assays. It is shown that EIT has a spatial resolution of 1.14% and it could monitor the cell mortality over 20% of a spheroid under laboratory noise level. The reconstructed conductivity images for cell mortality induced by the chemical are clear and match the result in the cellular metabolic viability assay. Furthermore, the image reconstruction speed in the experiment was less than 0.3 seconds. Taken together, the results show the potential of EIT for non-destructive real-time and label-free cellular assays in the miniature sensor, providing physiological information in the applications of 3D drug screening and tissue engineering.
A Introduction
There is currently a need to move away from traditional two-dimensional (2D) cell culture towards cell culture systems that better mimic the behaviour of cells growing in the natural environment.1,2 For instance, three-dimensional (3D) tumour cells are considered to have higher radio- and chemo-resistance than cancer cells cultured at 2D because of the 3D cell–cell and cell–matrix interactions,3,4 which is pointed out as one of the most important reasons for the low success rate in anticancer drug discovery and development.5 Because 3D spheroid culture models are closer to an in vivo like morphology, they can better reflect the biological mechanisms of cell migration, differentiation and viability.6
End-point destructive assays, such as histopathology, scanning electron microscopy (SEM) and transmission electron microscopy (TEM), can be used to evaluate frozen and fixed cells within a 3D structure.7 But currently, there is no straightforward method to assess cell growth and cell activity in live 3D structures. Confocal and fluorescence microscopies, the methods of choice for 2D culture, are limited to a penetration depth of a few micrometres (<50 micrometers) in dense, highly scattering tissues. Consequently, multiphoton microscopy and light sheet microscopy, in combination with fluorophores, are a better choice to image cells within spheroids.1 Alternatively, optical coherence tomography can be used to scan large spheroids and it was recently shown that cell viability could be inferred from the optical signal.8 This technique required analysing multiple scans at the same location and has therefore limitations in terms of the scanning rate. Simpler methods have been used to go towards high-throughput imaging. For example, spheroid drug response has been recorded with phase-contrast imaging by monitoring changes in size and integrity9 but with no clear indication with regard to change in cell viability. Therefore, at the moment, cellular metabolic activity assays based on luminescence or fluorescence are the states of the art to analyse drug response in spheroids.10,11 However, they don't allow for real-time monitoring of the drug response. In general, advanced optical imaging technologies are not very well suited to implement multiplexed real-time cell viability assays for 3D samples.
In recent years, impedance measurements were applied to monitor microtissue spheroids continuously.12–15 This technique is based on the measurement of the electrical properties of biological cells and the external culture medium. The electrical properties of biological cells under radio-frequency AC fields are attributed to the interfacial polarization arising from the presence of an insulating double-lipid bilayer membrane that separates two ionic media, i.e. the cell cytoplasm and the surrounding medium.16,17 By applying an AC current to the sensing area through a pair of electrodes, the impedance of the cells is measured through the same pair of electrodes or the adjacent electrode pair, which can be used to deduce cell concentration,18,19 cell viability20,21 and other cell activities.22 It is generally agreed that impedance measurements are a convenient, non-destructive and reliable approach for cell-based assays with high temporal resolution. The latest studies reported that impedimetric analyses were successfully applied to estimate the size,12,13 proliferation15 and drug response14 of 3D microtissue spheroids. Currently, most of the existing impedimetric studies are based on the analysis of measurements taken at only one pair of electrodes. They can only give a single lumped impedance value of the entire sample under test instead of a visual image giving an insight into the spatial distribution. It is necessary to improve the achieved spatial resolution.23
In this study, we demonstrated a novel, real-time and label-free cellular assay based on 2D EIT that can spatially resolve cell viability for single 3D spheroids. We first carried out finite element (FE) simulations to figure out the feasibility of EIT for single spheroid imaging and viability monitoring as well as its detection limits. Then, cohesive MCF-7 spheroids were cultured and introduced in a miniature sensor for practical experiments. The lysis buffer Triton X-100, which can cause cell death in a short time, was added to the sensor to validate the capability of EIT in real-time monitoring. Finally, the cellular metabolic viability assay was performed on the spheroids to verify the correctness of the results.
B Materials and methods
B.1 Electrical impedance tomography
EIT is an imaging technique that can reconstruct the conductivity distribution of the spheroids based on the boundary measurements.24 The mathematical theory of EIT is composed of two parts: the forward problem and the inverse problem.
The forward problem corresponds to the calculation of the electrical potentials on the electrodes from a known injected current and the conductivity distribution. It shows how effectively the change in each region contributes to voltage measurements. Solving the forward problem involves the construction of the Jacobian matrix, which is required in the image reconstruction in the inverse problem. The relationship between the boundary voltage v and the magnitude of the complex conductivity distribution |σ*| inside the sensor can be described by the nonlinear deterministic observation model as:
where
j is the excitation current density at the boundary electrodes. The absolute value is used in the equation because only the magnitude value of the measurement is used in the image reconstruction in this study. In the context of difference EIT imaging,
25eqn (1) can be linearized under the condition of a small conductivity perturbation Δ|
σ*|:
| 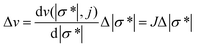 | (2) |
where Δ|
σ*| ∈
Rn×1 is the perturbation of the conductivity distribution of the sensing area, which is segmented into an
n voxel mesh, Δ
v ∈
Rm×1 is the boundary voltage difference before and after the perturbation and
J ∈
Rm×n is the sensitivity matrix calculated based on the Geselowitz sensitivity theorem:
26 |  | (3) |
where
k is the
kth voxel, ∇
vk(
Ix) is the electric potential at the
kth voxels when current
I is stimulated by the
xth electrode pair and ∇
vk(
Iy) is the electric potential at the
kth voxels when current
I is stimulated by the
yth electrode pair.
The inverse problem corresponds to the calculation of the conductivity distribution from the known injected current and related voltage measurements. The usual approach is to find a stable value of |σ*| so that the difference between the simulated boundary voltages v = f(|σ*|, j) and the measurement values v is minimised. The objective function is expressed as:
| ϕ(Δ|σ*|) = ½||JΔ|σ*| − Δv||22. | (4) |
Since the inverse problem is “ill-posed” and the solutions of the inverse problem are not unique, an additional penalty term should be introduced to the function as a constraint. Considering the spheroid is spatially sparse in the sensor and the presence of noise in the measurements, the sparsity constraint is relaxed and the iterative Basis Pursuit Denoising model27 is applied in this study.
| 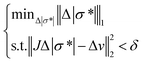 | (5) |
where
δ is the parameter associated with the level of the measurement noise.
Eqn (5) can be solved using the spectral projected gradient for an
l1 minimization method.
27
B.2 Effective medium approximation of MCF-7 cell spheroids
Cell are complex heterogeneous entities and thus must be simplified into a model to calculate their electrical properties.17 In this study, the MCF-7 cancer cell was fitted into the single shell model28 as shown in Fig. 1(a). Based on this model, the cytoplasm of the cell was composed of a homogeneous conductive medium. The relationship between the electrical properties of the spheroids and their physiological characteristics can be accurately controlled. Here, we define that the effective complex conductivity σ* and the complex permittivity ε* of an object are calculated from its effective conductivity σ and effective relative permittivity ε: | σ* = jωε0ε* = σ + jωε0ε | (6) |
where ε0 is the permittivity of vacuum.
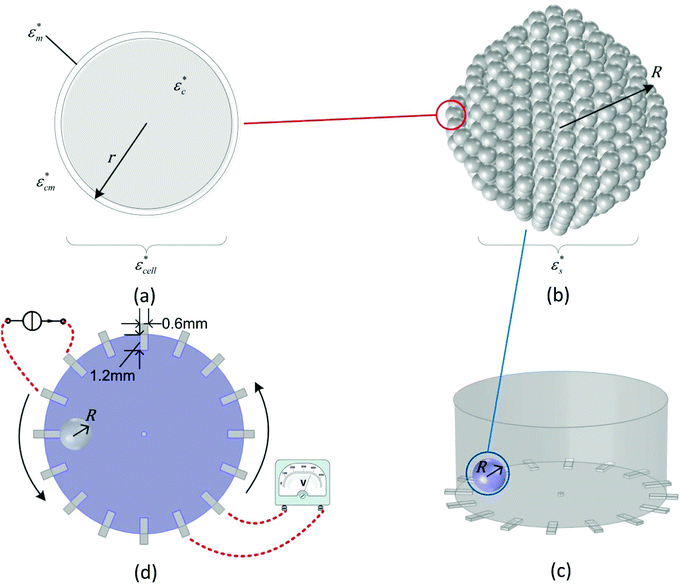 |
| Fig. 1 Simulation models for (a) a single cell, (b) a tumour spheroid and the EIT sensor in (c) stereo view and (d) top view. | |
Hence, the equivalent homogeneous complex permittivity of the whole cell
can be given using the Maxwell Garnett equation in eqn (7):
| 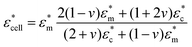 | (7) |
where

is the complex permittivity of the cytoplasm,

is the complex permittivity of the cell membrane and
v = (1 −
dm/
r)
3, in which
r is the outer radius of the cell and
dm is the thickness of the membrane.
In the multicellular spheroid model, each MCF-7 cell is considered as a sphere. The cells were surrounded by an external culture medium and evenly distributed within a spheroid of radius R (Fig. 1(b)). The volume fraction P of the cells over the spheroid depends on the number of cells x in the spheroid, which is given by P = x(r/R)3. To estimate the permittivity of the whole spheroid, Hanai's equation16 is preferable in the EMA, since it takes the high volume fraction into account and it is suitable for cells in different morphologies:
| 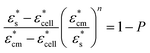 | (8) |
where

and

are the complex permittivity of the culture medium and the whole spheroid, respectively. The morphology parameter
n is set to 1/3 for the spherical MCF-7 cancer cells.
Eqn (6)–(8) are used to determine the FE simulation parameters in the next section, and in particular the effective permittivity of the cell spheroid.
B.3 Evaluation of EIT for spheroid imaging
The FE simulations to evaluate the performance of EIT in spheroid monitoring were performed in the COMSOL Multiphysics software with an AC/DC module in the frequency domain study. A cylindrical EIT sensor model was built with 15 mm and 7 mm diameter and height, respectively, to simulate the sensor filled with 1.2 ml liquid solution. 16 rectangular electrodes (dimensions: 1.2 × 0.6 mm2) were evenly distributed at the boundary of the substrate in the chamber and the circular ground electrode was designed in the centre of the well with a diameter of 0.4 mm. A tumour spheroid with the radius R was placed on the substrate of the sensor (Fig. 1(c)). The conductivity and the permittivity of the culture medium, the cell membrane and the cytoplasm of the viable cell were set as σcm = 1.5 S m−1, εcm = 80, σm = 0 S m−1, εcm = 5, σc = 1.5 S m−1, and εc = 80, respectively. The size of the cells and the volume fraction were defined as r = 10 μm, dm = 5nm and P = 46% based on the characteristics of the MCF-7 breast cancer cells29 and the tightly packed tumour spheroids.30 In order to reduce the computational cost, the cell spheroid shown in Fig. 1(b) was considered as a sphere and its effective permittivity
was calculated based on eqn (7) and (8).
In the simulation, the adjacent drive method31,32 was adopted for the current stimulation and voltage measurements, which increased the sensitivity to the cellular response near the electrode. A 10 kHz current with an amplitude of 1 mA was applied to a pair of electrodes and the electrical properties of the spheroid were measured from the successive adjacent electrode pair (Fig. 1(d)). By switching the current stimulation positions, totally 104 independent measurements were obtained as a data set for image reconstruction.
B.4 Impedance measurement system
Impedance measurements were carried out in a cylindrical miniature EIT sensor with microelectrode arrays33 at the substrate (Fig. 2(a and b)). The diameter and the height of the chamber were 15 mm and 10 mm, respectively, while the number and sizes of the gold-plated electrodes were the same as the setting in the FE simulations. The adjacent current stimulations and voltage measurements were performed with the high-speed EIT system (Fig. 2(c)) developed at the University of Edinburgh.34 In this study, the frequency of the stimulation current was set to 10 kHz and the frame rate was 30 fps. To test the performance of the miniature sensor, 1.2 ml culture medium was added to the sensor and the boundary voltages on the electrodes were continuously measured through the system for 1 hour. The SNR of each channel can be calculated using eqn (9): | 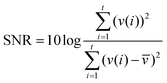 | (9) |
where v(i) and
are the voltage measured at the time i and the mean voltage of this channel, respectively.
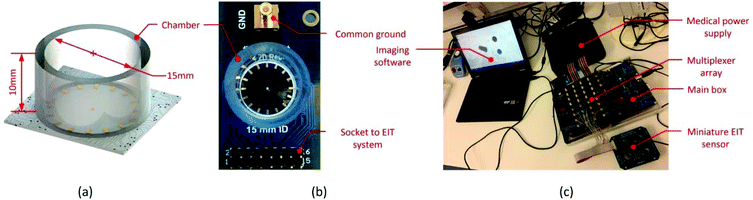 |
| Fig. 2 Pictures of (a) the schematic illustration, (b) the manufactured miniature EIT sensor, and (c) the high-speed EIT system. | |
B.5 Cell line maintenance
The MCF-7 breast cancer cells were obtained from ATCC (Middlesex, UK) and were routinely grown in T-25 flasks in a humidified 5% CO2 incubator at 37 °C. The culture medium was a low glucose Dulbecco's Modified Eagle Medium (DMEM) with glutaMAX (Thermo Fisher Scientific, Waltham, MA USA) supplemented with 10% (v/v) fetal bovine serum (FBS) and 1% (v/v) penicillin/streptomycin. After the cells attained confluence, they were subcultured using 0.25% trypsin.
B.6 Tumour spheroid formation
The liquid overlay technique was adopted to form cell spheroids on the hydrogel surface.35 1% (w/v) agarose dissolved in deionized water was sterilised by heating to 120 °C under 100 kPa for 15 minutes. It was then pipetted to the 24-well plate with the volume of 400 μl per well, following with 1 ml of culture medium. To accelerate the cohesion of the spheroid, the culture medium was optimized to a high glucose DMEM based culture medium (HG culture medium). The cells cultured in the T-25 flasks were trypsinised and counted on the haemocytometer. Cell suspensions with 104 cells were seeded onto each microplate well. The spheroid cultures were maintained under horizontal stirring with the Orbi-Shaker BT4000-E (Benchmark Scientific, New Jersey, USA) at 120 rpm. The size of the spheroids is controlled by the culture period.
B.7 Chemical insult and the reagent
Triton X-100 is a non-ionic, non-denaturing detergent widely used in biochemical applications for lysing cells. Triton X-100 can change live cell permeability and morphology at a low concentration, while a high concentration of the detergent will solubilize the cell membrane proteins, which eventually results in cell death by necrosis.36,37 In this study, the Triton X-100 solution is used to study cell viability in a time-lapse fashion. 2% (v/v) Triton X-100 solution is prepared by pre-mixing 0.2 ml Triton X-100 electrophoresis reagent liquid (Alfa Aesar, Lancashire, UK) with 9.8 ml HG culture medium. The conductivities of the Triton X-100 solution and the HG culture medium at room temperature are 2 S m−1 and 2.07 S m−1, respectively.
B.8 Cellular metabolic viability assay
To assess the cellular metabolic viability of the MCF-7 spheroids, a PrestoBlue Cell Viability Reagent (Thermo Fisher Scientific, Waltham, MA, USA) was used in the endpoint study. The reagent was mixed with the HG culture medium in a 1
:
9 mixing ratio following the product information sheet. The spheroids were incubated in the mixture for 60 minutes at 37 °C for the PrestoBlue assay. Fluorescence was measured at 525 nm (EX)/580 nm (EM) for control and spheroids with and without treatment. A higher fluorescence value (RLU) means a higher cellular metabolic viability of the MCF-7 spheroid under test.
C Results and discussion
C.1 FE simulations of the measurement range
Prior to monitoring spheroid viability in real-time, it is important to examine the theoretical detection limit of EIT for the cell spheroids. Hence, we performed two simulations to figure out the spatial sensitivity and viability detection limit of EIT, which could be used as a reference for parameter setting in the real-time cellular assay.
C.1.1 Spatial sensitivity.
The spatial sensitivity of the EIT measurement evaluates the performance of EIT in reconstructing the size and position of the spheroids. The sensor was first filled with a culture medium as a reference, and then the spheroids in different sizes were introduced to the substrate of the sensor as shown in Fig. 1(c and d). Voltage measurements were taken from the electrodes for EIT image reconstruction. Based on the calculation of eqn (9), the average SNR of the system was 52.63 dB. Hence, Gaussian noise was added to the boundary voltages to achieve the signal-to-noise ratio (SNR) of 50 dB to simulate the conditions of practical experiments. The spatial sensitivity of the reconstructed image was evaluated by the correlation coefficient (CC) between the true image and the reconstruction:38 | 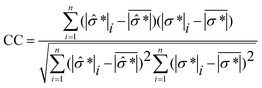 | (10) |
where |
*| and |σ*| denote the reconstructed and true conductivity distribution in the sensor while
and
are the mean values of |
*| and |σ*|, respectively. A higher CC means that the similarity between the actual size and position of the spheroid is closer to the reconstructed value, which means the reconstructed image is a better representation of the spheroid under study. We define the smallest spheroid that can generate the highest CC values as the spatial sensitivity of the measurement because its signal is strong enough to tolerate the disturbance of the measurement noise.
Fig. 3 shows the reconstructed images in the presence of MCF-7 cell spheroids with increasing radius. Based on the electrical characteristics of viable cells as mentioned in section B.3, the effective conductivity of the viable cell spheroid
is equal to 0.595 S m−1, which is lower than the conductivity of the culture medium
. Hence, the introduction of the spheroid results in the conductivity drop in the corresponding area. In the presence of spheroids with a small radius (Fig. 3(a and b)), the resulting disturbance to the boundary voltage is weak and the signal level is similar to the noise level. In Fig. 3(c and d), larger spheroids generate stronger signals to the boundary voltages, and better image quality is generated. The correlation coefficient histogram (Fig. 3(e)) indicates that the minimum radius of the spheroid that can maintain a high image quality is 0.8 mm, which means that the spatial sensitivity of the measurement is 1.14% of the sensor surface area.
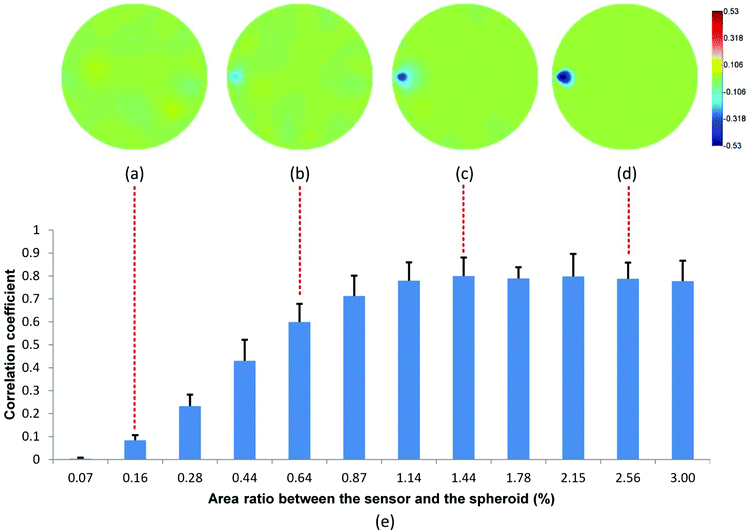 |
| Fig. 3 Reconstructed images for the spheroids in sizes of (a) 0.16%, (b) 0.64%, (c) 1.44% and (d) 2.56% of the sensor and (e) their correlation coefficient with the true image based on 20 measurement samples for each size of the spheroid (n = 20). | |
C.1.2 Detection limits of spheroid viability.
The purpose of this simulation is to evaluate the sensitivity of EIT measurement to the conductivity change of the spheroid caused by cell death. A viable cell spheroid with a radius R of 1.2 mm (area ratio of 2.56%) was simulated inside the sensor filled with culture medium and a cytotoxic agent as shown in Fig. 1(c and d). Since the volume of the biochemical agent was far smaller than that of the culture medium, we assumed that the conductivity of the background solution was equal to the conductivity of the culture medium
. Then, we simulated the effect of the cytotoxic agent with a gradual viability loss of the spheroid down to 0% (cell mortality rate increased from 0 to 100%) by modifying their dielectric property parameters in the simulation. Since the membrane of dead cells lost resistance to the low-frequency electric current, its conductivity and permittivity were increased to σm = 1.5 S m−1 and εm = 80. The conductivity of the spheroid with partial dead cells was updated with eqn (8) and it increased linearly with the cell mortality rate. EIT was used to reconstruct the conductivity change of the spheroid to deduce the cell mortality under a 50 dB noise level. A threshold based conductivity mask was applied to the reconstructed images to locate the spheroid and to calculate the average conductivity change of the spheroid associated with cell death.
According to Fig. 4(a–e), the conductivity variation due to the cell mortality can be detected and reconstructed in the correct position when the mortality rate was over 20%. The variation increases with the cell mortality, as we expected. Fig. 4(f) shows the relationship between the reconstructed conductivity variation and the cell mortality rate plotted with 30 spheroid samples. A good correlation exists between the reconstructed conductivity variation and the cell mortality rate at 20% mortality rate and above. Hence, EIT reconstructed conductivity variation can be used as an indicator of cell mortality in the spheroids.
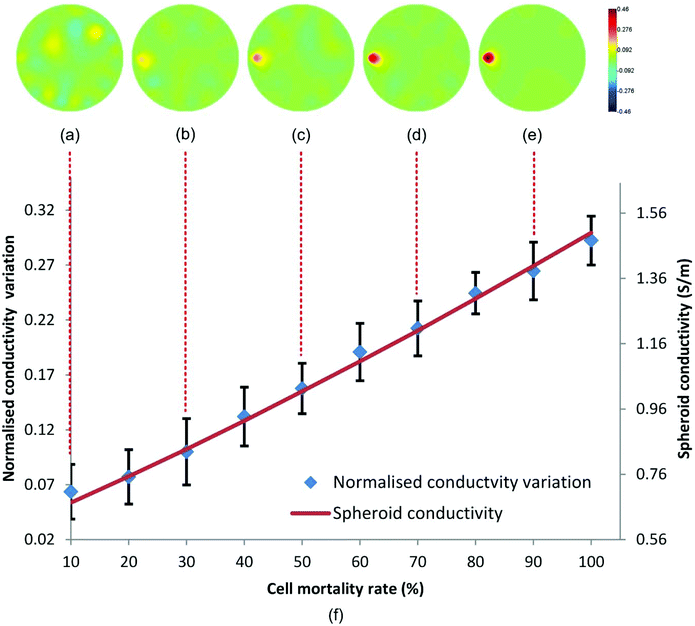 |
| Fig. 4 Reconstructed images for spheroids at (a) 10%, (b) 30%, (c) 50%, (d) 70% and (e) 90% mortality rates, and (f) the comparison diagram between the reconstructed conductivity variation and the cell mortality rate (n = 30). | |
C.2 Experimental results
C.2.1 Real-time imaging of spheroid viability.
In this section, experiments with MCF-7 spheroids were carried out to validate the FE simulations and the feasibility of EIT for real-time imaging. The MCF-7 cells were cultured in the incubator for 6 days using the optimized liquid overlay method to form spheroids with a radius of around 1.2 mm (Fig. 5). In order to monitor the dynamic course of cell death, the spheroids were introduced to the miniature sensor with 1.2 ml 2% Triton X-100 solution (experimental group). In the control group, the spheroids were introduced to the miniature sensor with 1.2 ml HG culture medium. Reference was taken immediately after that. Both the experimental group and the control group have two samples.
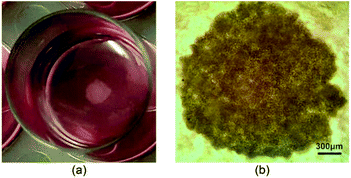 |
| Fig. 5 An MCF-7 spheroid sample cultured in a 24-well plate, (a) a digital camera image and (b) an optical contrast microscopy image; scale bar corresponds to 300 μm. | |
Fig. 6(a) shows the reconstructed images for the response of the MCF-7 spheroids in the 2% Triton X-100 solution (experimental group) and the HG culture medium (control group). These images show the difference in conductivity between the reference and the conductivity at the selected time points. 300 images in total were reconstructed for each sample and the processing time for each image was less than 0.3 seconds. As expected, the conductivity of the spheroids in the Triton X-100 solution has a significant increase while the conductivity of the spheroids in the HG culture medium remains unchanged. In the experimental group, the conductivity of the spheroids starts to increase at about 2 minutes until it reaches a plateau between 0.04 and 0.05 at 22 minutes. The increase is caused by the destruction of the insulating cell membranes after cell death. This result is consistent with the previous MCF-7 chemical response data in 2D,39–41 but a longer response time was observed, likely due to the 3D structure of the spheroids. The standard deviation around the mean could be explained by the individual differences between two spheroids in morphological characteristics, cell concentrations and initial cell viabilities. In Fig. 6(b), the chemical insult over time is reconstructed in real-time with a small fluctuation. One reason for the fluctuation is that the reconstruction parameter δ in eqn (5) in this experiment is fixed in order to compare the conductivity changes in different groups, so it is not optimized for each group to obtain the best image quality. Comparing the RLU in the control group and the experimental group shows that the viabilities of the MCF-7 spheroids almost drop to zero after a 30 minute treatment in the 2% Triton X-100 solution (Fig. 6(c)). These data showed that EIT can monitor and analyse the cell viability on 3D cell spheroids in real time.
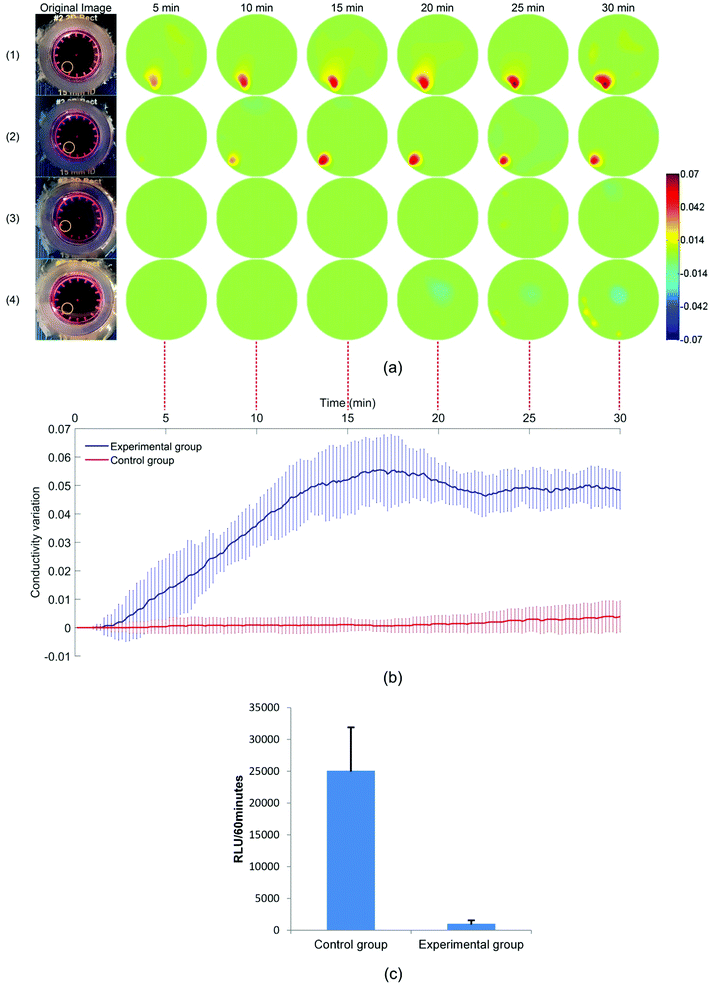 |
| Fig. 6 Reconstructed images for the spheroid samples in the (a1–2) 2% Triton X-100 solution (experimental group) and (a3–4) HG culture medium (control group), (b) the conductivity variation of the spheroids (n = 2) and (c) the cellular metabolic viability assay for the spheroids. | |
C.3 Discussions
In this study, a 2D EIT image reconstruction model is applied to reconstruct the conductivity distribution images. This setting projects the change in the sensor into 2D space. This method was chosen because it significantly reduces the computational time required to reconstruct an image when compared to 3D EIT reconstruction,42 and is therefore more suitable for a new technology to monitor in real-time and label-free cellular viability. Since the conductivity of the background medium is invariable during the measurement, the conductivity variation in the sensor is mainly attributed to the change of the cells. Therefore, the 2D conductivity distribution in the images can directly reflect the conductivity variation of the 3D cell spheroids.
Spheroid position in this study is selected to optimize the signal intensity of the EIT measurements and to improve the image reconstruction with the adjacent drive method. To measure samples located at the centre of the sensor, the polar-offset current drive43 or opposite current drive31 should be applied to maintain high sensitivity in the central area. In future studies, optimization will be performed to automatically detect the position of the samples and choose the best current stimulation pattern to increase the sensitivity of the measurements.
Taken together, the results show that the viability and integrity of the 3D cell spheroids can be monitored in real time and in their cell culture environments with EIT. This method is sensitive to the physiological variation of the cell spheroids. It allows the continuous monitoring of the spheroid integrity and size, which is a parameter of interest alongside cell viability.9 Cell mortality caused by a chemical insult can be reconstructed in real time through its correlated conductivity variations in the spheroid. Being able to monitor the response of MCF-7 spheroids to the Triton X-100 solution confirmed the ability of EIT to perform a real-time and label-free cellular assay. Although its spatial resolution is not as high as other imaging techniques such as confocal and fluorescence microscopies, it does have several distinct advantages over the existing techniques including cost, non-destruction, portability, high temporal resolution and potential for multiplexing and long-term high throughput screening. Overall, it maintains the advantages of other impedimetric measurement techniques while overcoming the lack of spatial resolution.
D Conclusions
In this study, we have developed an EIT sensor and associated methodologies to monitor in real time and non-destructively cell viability in 3D cell spheroids. This technique is demonstrated in a single-well format, but it could be easily multiplexed to a multi-well plate format. We first analysed the relationship between cell viability and spheroid conductivity through the EMA theory, and evaluated the feasibility of EIT for spheroid imaging in FE modelling simulation. Then, experiments were performed that validated our approach and established the potential and suitability of EIT to monitor real-time and label-free transient changes in cell viability induced by a chemical insult within large 3D spheroids. To the best of our knowledge, this is the first study to perform FE simulations with tightly packed tumour spheroids, and to demonstrate the real-time and label-free monitoring of conductivity variation of 3D cell spheroids following a chemical insult. This takes a step forward towards the miniaturisation of impedance imaging to enable the on-line monitoring of cellular activities in a 3D environment, and the integration of the EIT technique to drug-screening platforms and tissue engineering.
Conflicts of interest
There are no conflicts to declare.
Acknowledgements
This research was supported by alumni and friends of the University of Edinburgh through an Innovation Initiative Grant (Grant ID: GR003345).
References
- F. Pampaloni, E. G. Reynaud and E. H. Stelzer, Nat. Rev. Mol. Cell Biol., 2007, 8, 839 CrossRef PubMed
.
-
K. Bardsley, A. J. Deegan, A. El Haj and Y. Yang, in Multi-Parametric Live Cell Microscopy of 3D Tissue Models, Springer, 2017, pp. 3–18 Search PubMed
.
- V. Koshkin, L. E. Ailles, G. Liu and S. N. Krylov, J. Cell. Biochem., 2016, 117, 59–65 CrossRef PubMed
.
- K. Shield, M. L. Ackland, N. Ahmed and G. E. Rice, Gynecol. Oncol., 2009, 113, 143–148 CrossRef PubMed
.
- M. Hay, D. W. Thomas, J. L. Craighead, C. Economides and J. Rosenthal, Nat. Biotechnol., 2014, 32, 40–51 CrossRef PubMed
.
- B. M. Baker and C. S. Chen, J. Cell Sci., 2012, 125, 3015–3024 CrossRef PubMed
.
- L. E. Smith, R. Smallwood and S. Macneil, Microsc. Res. Tech., 2010, 73, 1123–1133 CrossRef PubMed
.
- N. J. Martucci, K. Morgan, G. W. Anderson, P. C. Hayes, J. N. Plevris, L. J. Nelson and P. O. Bagnaninchi, Adv. Biosyst., 2018, 2, 1700212 CrossRef
.
- J. Friedrich, C. Seidel, R. Ebner and L. A. Kunz-Schughart, Nat. Protoc., 2009, 4, 309–324 CrossRef PubMed
.
- T. R. Olsen, B. Mattix, M. Casco, A. Herbst, C. Williams, A. Tarasidis, D. Simionescu, R. P. Visconti and F. Alexis, Acta Biomater., 2015, 13, 188–198 CrossRef PubMed
.
- C. Holmes, J. Daoud, P. O. Bagnaninchi and M. Tabrizian, Adv. Healthcare Mater., 2014, 3, 572–580 CrossRef PubMed
.
- S. C. Bürgel, L. Diener, O. Frey, J.-Y. Kim and A. Hierlemann, Anal. Chem., 2016, 88, 10876–10883 CrossRef PubMed
.
- Y. R. Schmid, S. C. Bürgel, P. M. Misun, A. Hierlemann and O. Frey, ACS Sens., 2016, 1, 1028–1035 CrossRef
.
- K. F. Lei, B.-Y. Lin and N.-M. Tsang, RSC Adv., 2017, 7, 13939–13946 RSC
.
- K. F. Lei, M.-H. Wu, C.-W. Hsu and Y.-D. Chen, Biosens. Bioelectron., 2014, 51, 16–21 CrossRef PubMed
.
- K. Asami, Prog. Polym. Sci., 2002, 27, 1617–1659 CrossRef
.
- K. Heileman, J. Daoud and M. Tabrizian, Biosens. Bioelectron., 2013, 49, 348–359 CrossRef PubMed
.
- A. Rahman, J. Register, G. Vuppala and S. Bhansali, Physiol. Meas., 2008, 29, S227 CrossRef PubMed
.
- E. Krommenhoek, J. Gardeniers, J. Bomer, A. Van den Berg, X. Li, M. Ottens, L. Van der Wielen, G. Van Dedem, M. Van Leeuwen and W. Van Gulik, Sens. Actuators, B, 2006, 115, 384–389 CrossRef
.
- R. Sharma, T. Blackburn, W. Hu, K. Wiltberger and O. D. Velev, Biomicrofluidics, 2014, 8, 054108 CrossRef PubMed
.
- F. A. Alexander Jr., E. G. Huey, D. T. Price and S. Bhansali, Analyst, 2012, 137, 5823–5828 RSC
.
- J. A. Stolwijk, C. Hartmann, P. Balani, S. Albermann, C. R. Keese, I. Giaever and J. Wegener, Biosens. Bioelectron., 2011, 26, 4720–4727 CrossRef PubMed
.
- C. Canali, C. Mazzoni, L. B. Larsen, A. Heiskanen, Ø. Martinsen, A. Wolff, M. Dufva and J. Emnéus, Analyst, 2015, 140, 6079–6088 RSC
.
-
J. Zhao, et al., “Sparse regularization for small objects imaging with electrical resistance tomography”, Imaging Systems and Techniques (IST), 2013 IEEE International Conference on, IEEE, 2013 Search PubMed
.
-
D. S. Holder, Electrical impedance tomography: methods, history and applications, CRC Press, 2004 Search PubMed
.
- D. B. Geselowitz, IEEE Trans. Biomed. Eng., 1971, 38–41 Search PubMed
.
- E. Van Den Berg and M. P. Friedlander, SIAM J. Sci. Comput., 2008, 31, 890–912 CrossRef
.
- K. Asami, J. Non-Cryst. Solids, 2002, 305, 268–277 CrossRef
.
- A. Geltmeier, B. Rinner, D. Bade, K. Meditz, R. Witt, U. Bicker, C. Bludszuweit-Philipp and P. Maier, PLoS One, 2015, 10, e0134999 CrossRef PubMed
.
- M. Pavlin, N. Pavselj and D. Miklavcic, IEEE Trans. Biomed. Eng., 2002, 49, 605–612 CrossRef PubMed
.
- R. Harikumar, R. Prabu and S. Raghavan, Int. J. Soft Comput. Eng., 2013, 3, 2231–2307 Search PubMed
.
- T. Sun, S. Tsuda, K.-P. Zauner and H. Morgan, Biosens. Bioelectron., 2010, 25, 1109–1115 CrossRef PubMed
.
- Y. Yang, J. Jia, S. Smith, N. Jamil, W. Gamal and P. Bagnaninchi, IEEE Sens. J., 2016, 1–1 Search PubMed
.
- Y. Yang and J. Jia, Rev. Sci. Instrum., 2017, 88, 085110 CrossRef PubMed
.
- E. C. Costa, V. M. Gaspar, P. Coutinho and I. J. Correia, Biotechnol. Bioeng., 2014, 111, 1672–1685 CrossRef PubMed
.
- D. Koley and A. J. Bard, Proc. Natl. Acad. Sci. U. S. A., 2010, 107, 16783–16787 CrossRef PubMed
.
- Y. Huang, N. S. Sekhon, J. Borninski, N. Chen and B. Rubinsky, Sens. Actuators, A, 2003, 105, 31–39 CrossRef
.
- W. Yang and L. Peng, Meas. Sci. Technol., 2003, 14, R1 CrossRef
.
- M. Brischwein, S. Herrmann, W. Vonau, F. Berthold, H. Grothe, E. R. Motrescu and B. Wolf, Lab Chip, 2006, 6, 819–822 RSC
.
- T. Schwarzenberger,
et al.
, J. Phys.: Conf. Ser., 2010, 224(1) Search PubMed
, 012141.
- T. Schwarzenberger, P. Wolf, M. Brischwein, R. Kleinhans, F. Demmel, A. Lechner, B. Becker and B. Wolf, Physiol. Meas., 2011, 32, 977 CrossRef PubMed
.
- A. Borsic, E. Attardo and R. Halter, Physiol. Meas., 2012, 33, 1703 CrossRef PubMed
.
- C. Canali, K. Aristovich, L. Ceccarelli, L. Larsen, Ø. Martinsen, A. Wolff, M. Dufva, J. Emneus and A. Heiskanen, J. Electr. Bioimpedance, 2016, 7, 59–67 CrossRef
.
|
This journal is © The Royal Society of Chemistry 2018 |
Click here to see how this site uses Cookies. View our privacy policy here.